Month: November 2019
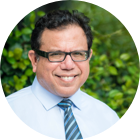
MMS • Dylan Schiemann
Article originally posted on InfoQ. Visit InfoQ
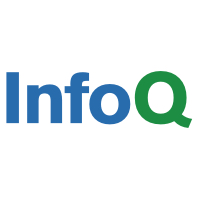
The Wake Lock API prevents some aspect of a device from entering a power-saving state, a feature currently only available to native applications. Chrome 79 Beta updates its experimental support for this feature, adding promises and wake lock types.
Under development since 2015, the Wake Lock API is one of many APIs that strives to give web app developers feature parity with native applications. Most mobile devices quickly sleep when idle to prevent apps from draining a device’s battery. This behavior is generally preferred, but some applications need to keep the device or screen awake to be useful. Use cases include using web apps as audio tours, recipe applications, boarding passes, kiosks, and presentations.
The newest update to the Wake Lock API proposal expands the scope beyond keeping the screen on and resolves potential issues with security and privacy.
To use the Wake Lock API, developers need to enable the #enable-experimental-web-platform-features
flag in chrome://flags
. To see the Wake Lock API in action, enable the feature in Chrome and visit the Wake Lock Demo and view the Wake Lock Demo source code.
The Wake Lock API offers two wake lock types, screen, and system. While somewhat independent, a screen wake lock necessitates that the app continues running. As implied by their names, the screen wake lock prevents the screen from sleeping, whereas the system wake lock prevents the CPU from entering standby mode.
The Wake Lock API was recently updated to support promises and async functions. The WakeLock API is sensitive to page visibility and full-screen mode, and the Wake Lock API provides visibilitychange
and fullscreenchange
events to help developers offer a seamless experience.
Developers are encouraged to consider whether their application requires the Wake Lock API when options that are less performance-intensive exist for certain use cases. For example, an appl with long-running downloads should instead use background fetch, and applications synchronizing data from an external server should instead use background sync.
The Wake Lock API team is looking for feedback. Contributions are welcome via the Web Lock GitHub repo and should follow the W3C contribution guidelines.
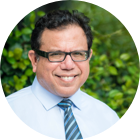
MMS • Sergio De Simone
Article originally posted on InfoQ. Visit InfoQ
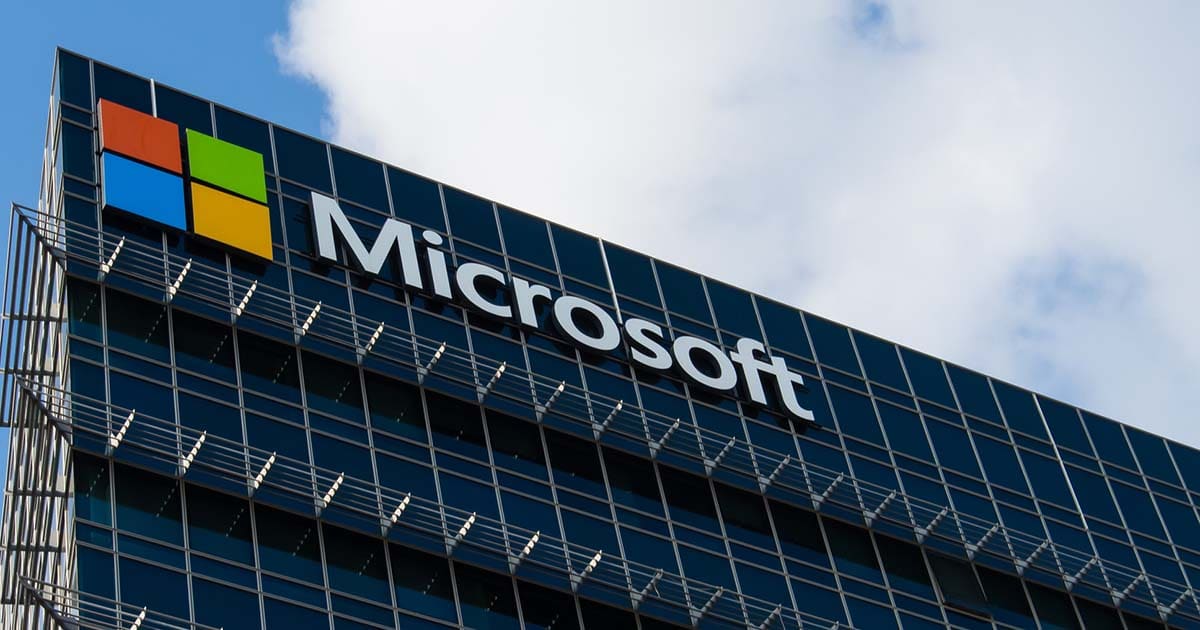
Microsoft has been recently experimenting with Rust to improve the safety of their software. In a talk at RustFest Barcelona, Microsoft engineers Ryan Levick and Sebastian Fernandez explained the challenges they faced to use Rust at Microsoft. Part of Microsoft journey with Rust included rewriting a low-level Windows component, as Adam Burch explained.
According to Fernandez and Levick, the software industry is sitting on insecure technological foundations that are costing a lot of money. A very conservative estimate for Microsoft is each issue discovered in the field costs $150,000. The cost may be even greater for organizations that happen to be the victims of a security vulnerability exploit. This was exactly the case with the British national health care system, which was target of a ransom attack that costed it an estimated $4 billion.
Much of this, they say, is related to the use of C and C++.
C and C++ are extremely great at writing low level systems. They use very little resources on the machine. They are, in fact, really the basis on which we create our systems today but the issue with that, of course, is that they are very, very unsafe and, when they were developed, did not really have safety in mind.
This explains why Microsoft is experimenting with Rust, hoping it can help making software bugs, and specifically those leading to security vulnerabilities, impossible.
Rust allows us to write performant security critical components safely.
This is actually a claim waiting to be proved, say the two engineers, but they hope it will turn out to be true.
A major hindrance towards this goal is it is not possible to rewrite everything from scratch in Rust. Instead, Rust shall coexist with other technologies, which is not always easy. For example, speaking of Windows, a first hurdle is LLVM, Rust compiler, which provides only subpar Windows support. Similarly, Cargo, Rust build tool, cannot manage the whole build system at Microsoft and shall be integrated with their existing build system.
This line of reasoning applies to all Rust tools as well as for shared executables which are encapsulated in DLLs and that are mostly written in C and C++ and support COM, WinRT, and Win32.
In addition to this, there are other challenges that an organization like Microsoft has to overcome, including the human factor. Rust indeed should be adopted also by people who may have been writing C and C++ for many years and shall be convinced of the convenience of switching over.
The good news about this is that before, when we’ve introduced Rust to seasoned C++ programmers, they generally are able to get it rather quickly because it kind of just formalises things that they already have in their head. When people are coming from other backgrounds, it might be a little bit more difficult, but while the learning curve is quite steep, generally people get through it and once they are through that learning curve, they are quite productive.
Adam Burch, software engineer at Microsoft with the Hyper-V Team, shed some light on the kind of projects Microsoft is using Rust for. Burch recounted his experience rewriting a low-level system component of Windows and described it as a breath of fresh air thanks to Rust compiler guarantees, :
The memory and data safety guarantees made by the compiler give the developer much greater confidence that compiling code will be correct beyond memory safety vulnerabilities.
Burch shares Fernandez and Levick’s optimism about C/C++ developers quickly picking up the language and includes couple of interesting practical suggestions for interfacing Rust with C and C++ code and keeping the language use safe, including the generation of Rust data structure to represent C data and around COM APIs.
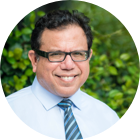
MMS • InfoQ.com
Article originally posted on InfoQ. Visit InfoQ
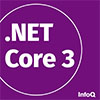
Desktop, Web, Cloud, Mobile, IoT. We have never experienced such diversity in application environments before – each with its own set of particularities, and all of them accessed by an ever-increasing number of users. Considering all the operating systems, protocols, and standards used by these environments, there’s a crescent trend related to cross-platform development: the ability to create one application capable of being seamlessly executed in multiple environments. There is a myriad of problems to be addressed in cross-platform development, varying from which application environments are addressed in each scenario to specifics related to the development stack used by each application. While there is no silver bullet that can address all these problems at the same time, it is expected that one year from now all .NET developers can use only one .NET framework to target the most common application environments currently in place.
Since last year, with the first announcement of .NET Core 3.0, Microsoft has been steering its .NET ecosystem initiatives towards the creation of a truly unified application development platform. With a new approach for Desktop development, .NET Core 3.0 closes some of the gaps of cross-platform applications for the Web. In the next year, other new features are expected to bring other application environments even closer, culminating with the release of .NET 5 – the first version of the intended unified framework. In this eMag, Five authors talk about the current state of .NET Core 3.0 from multiple perspectives. Each author brings their experience and ideas on how different .NET Core 3.0 features are relevant to the .NET ecosystem, both present and future.
Free download
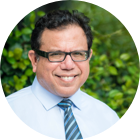
MMS • Carol McDonald
Article originally posted on InfoQ. Visit InfoQ
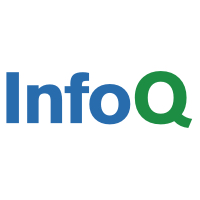
Kirk Borne, principal data scientist at Booz Allen Hamilton, gave a keynote presentation at this year’s Oracle Code One Conference on how the connection between emerging technologies, data, and machine learning are transforming data into value.
Borne explained that with IoT, the value is not in all of the data produced, but instead in the contextual knowledge that sensors give us about the world. With IOT and context knowledge, the goal is to discover and deliver value from data. Value from data is achieved by going from understanding to actions. Emerging technological innovations like AI, robotics, computer vision and more, are enabled by data and create value from data. Borne says that AI is not artificial intelligence but “actionable intelligence”, and data is the fuel for insights for actionable intelligence.
In his talk Borne went into four broad types of discovery from data: class discovery, correlation and causality discovery, anomaly discovery, and association discovery.
Class discovery learns the groupings, and boundaries that separate groups in data. An example of class discovery is disease diagnostics given lab measurements. Learning not only the groupings but also what distinguishes them is the real power with class discovery.
Correlation discovery finds dependencies in data. This is predictive discovery: if x correlates with y, then given x predict y. As you add data, you get insights as to the causal information about why something is happening, and this becomes causation or prescriptive discovery, which is given y find x. Discovering causal variables, or prescriptive analytics, allows you to predict and change outcomes. Examples of getting value through this type of discovery are fraud and machine monitoring.
Anomaly discovery finds regions that the data avoids. The a-ha moment answers the question: what is the data telling me about why it should not be in this spot.
Association discovery finds interesting associations, links in a graph that are indirectly connected. For example a communicates to c through b. Examples of getting value through this type of discovery are recommendations, marketing attribution, and illicit money transfers.
Borne also discussed analytics, the products of data science, and the five levels of analytics maturity: descriptive, diagnostic, predictive, prescriptive and cognitive. Descriptive analytics reports what happened, which sounds boring but is essential for applications like auditing. Diagnostic analyzes why this is happening, why did this change under this condition. Predictive analyzes what will happen. Prescriptive analyzes how to optimize what happens. Cognitive, the highest level, analyzes what is the right action for this data in this context. Analytics by design focuses on products that generate value from data. An example is a chatbot recommendation engine which answers customer FAQs. This can add value by giving a better customer and employee experience.
Lastly, Borne went on to explain dynamic-data-driven applications which combine sensor measurements, machine learning inference prediction, and action. Borne said there is a combinatorial explosion of possible connections among emerging technologies like Robotics, computer vision, IoT, etc, which are building dynamic data driven applications not imagined before such as:
- Robotics combines AI with actions to sensory data. Examples include prosthetics warehousing, and manufacturing.
- Augmented reality combines AI, computer vision and image recognition with actions to superimpose a computer generated image on the user’s view of the real world. Examples include medical procedures and trying out clothes without putting them on.
- IoT sensor data combined with AI examples include: health devices, manufacturing, connected products and farming.
- Machine Learning is also being combined with data to create smart data. Examples include metadata, taxonomies, and breadcrumbs.
Borne concluded his talk with a Larry Ellison quote:
Our mission is to help people see data in new ways, discover insights, unlock endless possibilities.
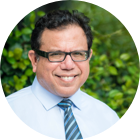
MMS • J Meadows
Article originally posted on InfoQ. Visit InfoQ
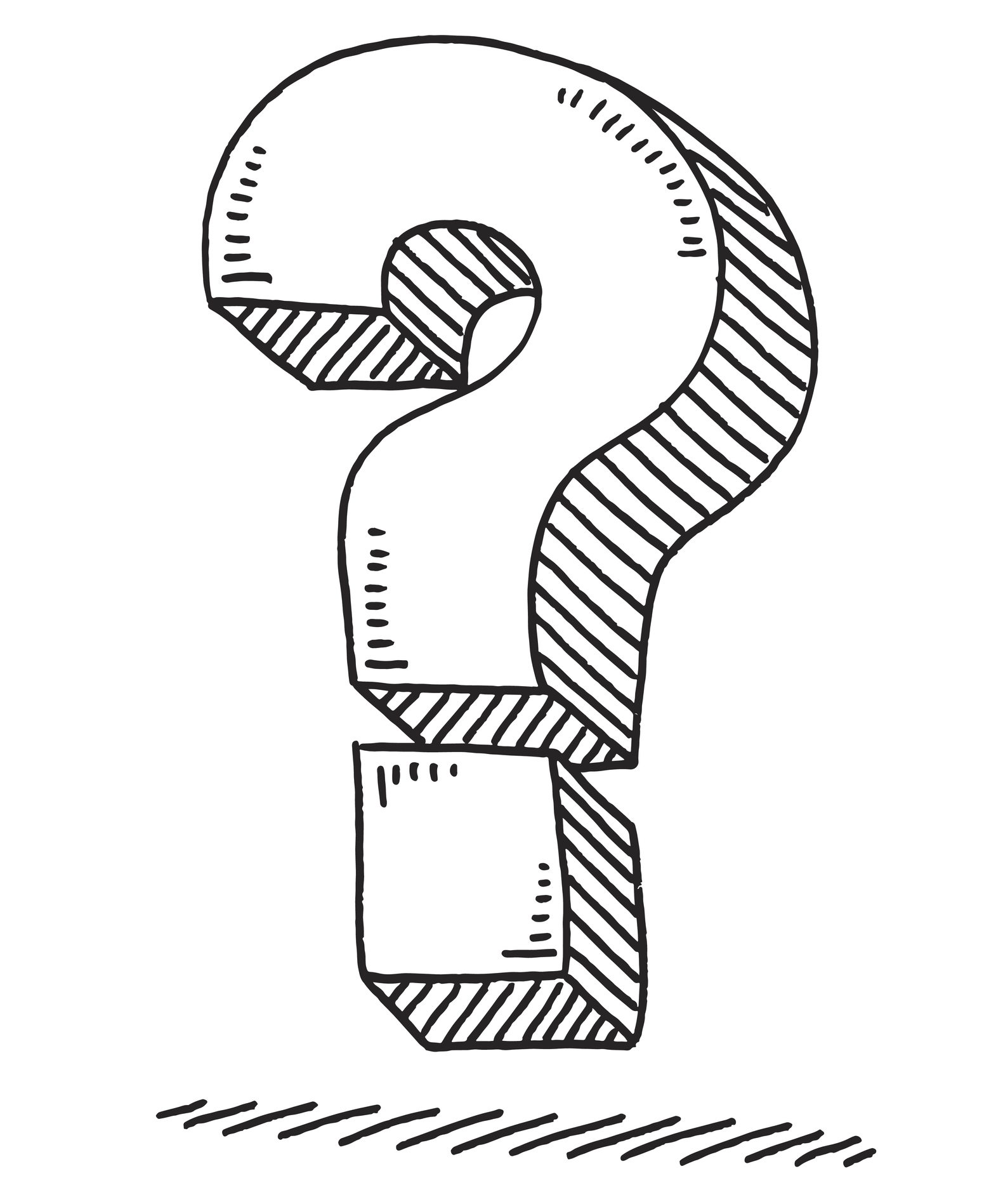
Key Takeaways
- The software industry has a dismal track record when it comes to predicting and planning in the face of uncertainty.
- There are a significant number of biases that prevent us from learning, including cognitive biases and compensation structures.
- Statistical approaches to predictions can be successful if we expend the effort to create learning-based models such as Monte Carlo simulations.
- Highly uncertain environments are best exploited using the iterative learning models inherent to Agile methods.
- Extremely uncertain, non-deterministic environments are best exploited by the incremental learning model of hypothesis testing (Hypothesis-Driven Development) and learning to embrace the discomfort associated with uncertainty.
The Best Laid Plans …
“Prediction is very difficult, especially about the future.”
This quote is often attributed to physicist Neils Bohr. It is also variously attributed to one or all of the following: Mark Twain, Yogi Berra, Samuel Goldwyn, politician Karl Kristian Steincke, or simply an old Danish proverb. That’s a healthy warning that things are rarely as simple as they seem and they usually get more complicated the deeper we go into them. If we can’t even determine who actually cautioned us about the difficulty of predictions then perhaps it’s an indicator that predictions themselves are even trickier.
The software industry operates on predictions at nearly every turn. Yet our track record of success with them is dismal. Depending on which metrics we choose to quote, between 60-70% of software projects are delivered over-budget, over-schedule, or canceled outright 1, often after large amounts of money have been spent.
What causes this and what can be done to increase the odds of success? Before we get into that, let’s review the reasons for making software predictions.
Businesses need to budget and plan. Capital, the lifeblood of a business, must be allocated sensibly toward efforts that will provide the best return. We need to answer questions such as: How much should we spend? Which projects get approval to proceed? Over what time horizon are we allocating? Is it the two weeks of the next sprint or the twelve months of the next fiscal year?
Traditionally, allocation questions were answered by first creating some kind of estimate regarding long-range project cost and scope, formulating a schedule and plan around that, and then deciding whether the plan was worthy of capital. For many reasons, some of which we discuss below, a plan often started unraveling even before its ink was dry.
With the advent of Agile methods such as Scrum, the planning cycle has been reduced to as little as two weeks. Even so, the questions remain about which stories in our backlog are worthwhile. But even shortened release cycles still result in disappointment at least 60% of the time 1. So what is wrong? Why aren’t we improving things? Why can’t we seem to learn how to make things better?
Why We Don’t Learn
Let’s examine what mechanisms drive us to continue with strategies that often fail and leave us stuck in a non-learning cycle. If we can understand the motivations for our actions then it might make it easier to change them and learn something along the way.
We Get Paid Not To
In much of the corporate world, job security and incentives are tied to making accurate predictions, formulating a plan to achieve them, allocating scarce capital, and then delivering on the plan. Additionally, there are often financial incentives to deliver at less than the predicted cost and completion date. As long as pay incentives are tied to predicting and planning the future, predictions and plans will be the bread and butter of business activity, whether they produce the desired outcome or not. In fact, convoluted conclusions likely will be derived that prove their validity, regardless of whether such validity exists.
Unfortunately, the solution to failed predictions is often taken to be the alluringly plausible “we’ll do better next time.” It’s natural to wonder: how many attempts does it require to achieve mastery? At some point, we should realize that a strategy isn’t working and something else needs to be tried. What slows the learning is that compensation is tied to not understanding it. Upton Sinclair captured the essence of this when he wrote (in gender-specific terms):
“It is difficult to get a man to understand something, when his salary depends on his not understanding it.”
If we want to improve our outcomes then we need to change our compensation structures into something that rewards us for learning and move away from structures that reward us for not understanding things.
An anecdote: Once, when describing to an executive how uncertain completion dates are in non-deterministic systems, he turned to me in exasperation and, holding his thumb and forefinger a fraction of an inch apart, said, “You’re telling me that you don’t know when you will finish until you are this close to being done? That’s nonsense.” It’s hard to say who was more disappointed in the conversation. The executive, because to him I seemed to lack a basic understanding of how business works, or me, because the executive seemed to lack a basic understanding of the mathematics of his business. In reality, we were both right, at least from our respective viewpoints. The real problem lay in the architecture of our compensation system that forced us into incompatible beliefs.
The Allure Of Simplicity
No matter how we practice it, software engineering is a difficult business undertaking. It was always thus. Fred Brooks, writing decades ago, concluded that there was “No Silver Bullet” 2 that would eliminate the inherent complexity and difficulty of developing software. And yet here we are, so many years later, still seeking a solution to the complexity, something that will make it simple. This desire for simplicity drives us to create oversimplified plans that discount the likelihood of the unknowns that will derail our project when they suddenly appear, often late in the plan after considerable sums have been spent.
When it comes to predictions, it is alluring to believe that there is a simple, one-size-fits-all solution that will work for everyone and all that is required is rigid adherence to its practices. Yet history shows us that a never-ending parade of simple solutions come and go, falling into and out of fashion with regular occurrence. What this suggests is that the software industry has complex needs and there is no simple, “Silver Bullet” solution that addresses them. From that wondrous wit, H.L. Mencken, we have this admonition to warn us about the allure of simplicity:
“… there is always a well-known solution to every human problem — neat, plausible, and wrong.”
The Sunk Cost Fallacy
Once we have invested time and money to create a prediction then the sunk cost fallacy rears its head. The sunk cost fallacy boils down to this: money already spent cannot be recovered but we are often unable to see that and spend additional money seeking a return on our initial outlay. We are prone to this because our job security usually requires us to prove that we are making wise financial decisions that turn out to be profitable. Worse, the more we spend the more we feel the need to justify our investment, putting us on a spiraling path of ever greater cost. All of this means that we will too often spend money to defend a failed prediction long after it would be better abandoned and the money reallocated to a more sensible idea.
There is an instructive example in the natural world, which has no sunk costs. If something doesn’t work, if it’s maladaptive to its environment, it is dealt a swift and pitiless blow that ends its reign and a new idea quickly replaces it. It’s an example worth remembering next time we find ourselves believing our prediction will come true if we just invest a bit more into it.
The Dogma Trap
Any successful business has, deliberately or accidentally, discovered a set of practices that allow it to exploit a niche in its environment. These practices become codified into a system of rules and organizational hierarchies that are intended to preserve and perpetuate the success of the business. The longer the success, the easier it is for profitable practices to become encoded as dogma. If any of these successful practices involve predictions, then belief in the efficacy of predictions may become dogma as well.
Of course, the cardinal problem with dogma is that, by definition, it is unchanging, thereby blinding us to a changing environment, which has no such definition. And when the environment changes but the dogma doesn’t then it’s a short step to extinction. Avoiding this requires us to reject the formulation of dogma.
But rejecting dogma is often an unwelcome practice in an organization. Those who question it are often labeled as someone who “isn’t a team player” or someone who needs to “get with the program.” Sidelining or removing such people is the typical response. After all, dogma exists in a business because it codifies a strategy that led to success and protecting that success is a principal mission of the organization. When it comes to predictions, however, a reasoned approach would suggest that thoughtfulness, not dogma, should guide decision making.
The Cruelty of Randomness
Prediction typically has two troubling beliefs inherent to it. One, that the future will proceed much like the past and, two, that all past events were expected. In reality, at the moment they were occurring, events that reshaped the future were often entirely unexpected. By forecasting the future we are often assuming that there will be no unknowable and, therefore, unexpected future events. The cognitive trap is that new endeavors seem to be similar to those in the past, making us believe that we have advance knowledge of events that would otherwise surprise us. The problem is that each new endeavor unfolds according to its own internal, and usually unknowable, set of unique characteristics.
If we know what we don’t know then we can simply apply an appropriate fudge factor to account for it and proceed on our way, satisfied that our plan accounts for unknowns. Unfortunately, we are too often unaware of our own ignorance, much less how to plan for it. Additionally, we are incentivized to find reasons that it “failed last time because of X but we have accounted for that this time.” While we may have accounted for X in the latest prediction it’s never X that surprises us the next time. It’s Y or Z or any other unknown. While there are a finite number of alphabetic characters such that we can eventually account for all of them, there is no such upper limit in the possible range of real-world unknowns.
But what if we get lucky and are rewarded with a random success for one of our predictions? If we don’t realize that it’s random, it will inevitably reduce our inclination to try a new strategy because of our natural belief that success was due to our skill instead of chance. That makes it more likely that random successes will be elevated to the status of perfect execution and repeated failures will be rationalized as poor execution. But it’s the randomness of reward we get from the lucky prediction that causes us to try ever harder to reproduce it. Studies show that the humble pigeon will quickly learn the pattern required to peck a lever to release food 3. And if no food ever arrives they will quickly give up. But if the reward is random, if there is no discernible pattern to when pecking the lever releases food, then the pigeons soon are driven into a superstitious frenzy of pecking in trying to determine the pattern. This behavior doesn’t seem terribly dissimilar from repeated attempts to make our predictions come true.
The Charismatic
Add in another human bias: we like confident and charismatic people. Confident, certain, and assertive people are promoted quickly and rise to positions where they influence a company. From there, they orchestrate activities to show that they can predict the future, formulate a plan, and execute on it. When faced with an unknown, they have a certain answer and a plan of action at the ready even if the plan might represent a mismatch between their confidence and their competence. So we marshal resources under their leadership and move ahead full of certitude. Contrast that to those who are uncertain and when asked about an unknown, shrug their shoulders and reply “I don’t know. Let’s do an experiment and see if we can figure it out,” leading us to turn to the charismatic individuals instead of the cautious ones.
An overconfidence bias also comes into play. Charismatic and confident people are likely to be imbued with a sense of superior predictive ability over their compatriots. Rationally, we might look at the 70% failure rate of predictions and decide that we are better off avoiding them because we stand only a 30% chance of success. Highly confident people are instead likely to take a different view, discount years of statistics from many projects, and believe that their efforts will somehow succeed where others failed.
An anecdote: Many years ago, at the tail end of the dot-com bubble, I worked in a startup as a software developer. We were led by a young, energetic, and charismatic CEO who exuded confidence and mastery. At the time, we leased office space in a building that had been shedding software tenants one after the other as each one failed like so many dominoes. There were only a few of us left and the nearly-empty building and parking lot had the eerie feel of a post-apocalyptic setting. It was in this environment that our CEO called an all-hands meeting to reassure the anxious staff that our future was promising. I recall him making an eloquent and impassioned case that filled the room with the belief that we might make it.
In the end, of course, we were no different than any other of the innumerable dot-coms that failed in the wake of the bubble’s bursting. Indeed, our denouement arrived shortly after our CEO’s rousing speech when we failed to receive another round of financing and joined the exodus of the building’s tenants.
Blinkered by confidence and faith in a charismatic leader, many in the company were unable to see what was obvious: we could not survive if we were not profitable. This was clear in hindsight but beforehand it seemed reasonable to believe that we were different and would succeed where so many others recently had failed. It was an instructive lesson in maintaining a reserve of skepticism around charisma.
Being Mistaken, Usually
“Well, we won’t make that mistake again. We even fired some people to make sure it never recurs.” That’s probably true. We won’t make the same mistake because we are prepared for it on the next attempt. The problem is that the first mistake was unknowable before it occurred and the same thing will happen again but this time with a different set of mistakes. The set of new mistakes, to which we will fall victim, is an inexhaustible supply because they are always unknowable in advance. Winston Churchill perfectly described this scenario while addressing Parliament at the dawn of World War II. Members were concerned about repeating the mistakes of World War I and wanted assurance that they would be avoided. Churchill replied:
“I am sure that the mistakes of that time will not be repeated; we should probably make another set of mistakes.”
We are often mistaken and simply don’t yet know it. And being wrong and not knowing it feels just like being right 4. Actually, being right and being wrong are indistinguishable until the moment we are proven wrong. That should sound a note of caution about the inevitability of mistakes.
There is an expression often heard in management meetings and boardrooms: “failure is not an option.” While this is usually intended to discourage half-hearted efforts, it excludes learning and discovery because failure is a necessary ingredient in learning. It also suggests that to admit a mistake means to admit incompetence and possibly lose one’s job. Once this belief system is in place and cemented by financial incentives, it can lead to the idea that failure indicates a historically successful practice is going through a temporary rough patch and we simply need to redouble our efforts so it will once again be successful, even if the real lesson is that we need to change course. Under these conditions, admitting an error and changing course is a difficult thing to do because we are irreversibly invested in our belief system. History is filled with examples of businesses that failed to learn and continued to feed ever greater amounts of precious capital into failed strategies even as those strategies drove them right off a cliff. A moment’s reflection will disabuse us of the notion that we are somehow immune to such folly.
Strategies That Use Learning
So that’s a rundown of some of the reasons why we are often unable to learn and continue with strategies that fail us. But what if we can avoid these pitfalls? Are there strategies that focus on learning? As it happens, there are.
A Deterministic Approach
Historically, software projects used a Waterfall model of development. Requirements were gathered, estimates were made from the requirements, and schedules were created from the estimates. This approach is based on a deterministic view of software projects and that with enough upfront data and analysis, we can make accurate predictions about cost and delivery dates. These projects often began failing early, usually due to inadequate requirements and inaccurate estimates. In the latter case, estimates were often faulty because they were not based on statistically rigorous methods but instead gathered from methods that were little more than guessing.
It turns out, though, that a deterministic view can succeed by using calibrated statistical models gathered from a company’s historical software projects. One common statistical method is a Monte Carlo analysis 5 6. The underlying mathematics are rather complicated but it boils down to this: we gather a set of historical data that typically include parameters like effort and duration. We then run scenarios thousands of times where we randomly vary input parameters to produce a probability distribution that a given amount of work will be completed in a given amount of time. For example, we might derive a distribution that indicates a certain amount of staff effort has a 25% probability of being completed within a month, a 50% probability within two months, and a 90% probability within five months. The key point is that we use historical data, unique to our organization, to calibrate our model and produce probability ranges for outcomes instead of single-point values. Notice how rigorous this approach is compared to someone’s unsubstantiated claim that “I can do that in a week.”
With this approach, we are also choosing to learn. We gather data over time and use it iteratively to teach us about our organization’s capabilities and the cost and time required to perform work. Of course, our model is only as good as the data we use to calibrate it. Vague requirements specifications, poor record-keeping for completed work, and other such shortcomings will yield disappointing results.
A Pseudo-Deterministic Approach
A fully-deterministic approach as described above works well if requirements can be specified in advance and are not subject to frequent revision but this type of project is rarely seen. What if we are working on more typical projects with unclear goals, uncertain specifications, and unknown market needs? Deterministic predictions under those conditions are unlikely to yield satisfactory results.
Enter Agile methods.
Agile methods take a pseudo-deterministic approach to software delivery. Born out of the frustration with repeated failures in traditional Waterfall projects, Agile methods abandon the belief in long-term predictions and planning and instead focus on short-term delivery of working software and adapting to change as it occurs. By using Agile methods, we adopt the philosophy that requirements cannot be determined far in advance but must instead emerge over time.
One of the more popular Agile methods is Scrum 7. Its two-week sprint minimizes project tracking error and drift by shortening timeframes for releases. We reprioritize with every sprint and in so doing effectively reset our project clock, giving us the flexibility to adapt to change.
We can still use Monte Carlo-type methods to predict the volume of stories we can produce 6 but we surrender our belief of one aspect of determinism: that we can generate long-term plans that determine project schedules. Instead, we once again focus on learning by iteratively discovering what we need to deliver.
But have we actually solved the problem of predictions and plans or have we just minimized the impact of being wrong about them? It seems we might still carry with us the same problem but at a smaller scale.
An Evolutionary Approach
We have progressed from the long-term release cycles of traditional methods to the much shorter cycles of Agile methods. We also abandoned the belief in long-term, fixed requirements and chose instead to focus on smaller stories. Both of these changes help us iteratively discover requirements and produce better results. This leads to an obvious question: if a little discovery is a good thing, is more discovery an even better thing?
Enter hypothesis testing.
Hypothesis testing (also called Hypothesis-Driven Development) takes its cues from the greatest experimental laboratory ever devised: evolution. Evolution makes no pretense at being able to predict what the future holds. It simply responds to change by constant experimentation. An experiment that produces a better outcome is rewarded with longevity. A worse outcome is quickly subjected to an ignominious end. If we are willing to surrender our predictive beliefs then evolution has a lot to teach us.
With hypothesis testing, we take a slightly more deliberate approach than the pure randomness of evolution. We proceed as scientists do when faced with the unknown: formulate a hypothesis and subject it to measurement and failure in the real world. If it is falsifiable and can’t be proven false, at least not yet, then it has merit.
There are many ways to implement hypothesis testing 8 9 10 but here is a simple example. We formulate a hypothesis such as “We believe that our customers want a left-handed widget feature on our data portal. We declare our hypothesis to be true if traffic to our portal increases by 5% in one week.” If our hypothesis is correct then we should see at least a 5% bump in traffic within a week. If not, we were wrong and reject our hypothesis and possibly remove the feature. We then reformulate our hypothesis or move on to another one. It’s beyond the scope of this article to provide a detailed how-to of hypothesis testing but the references provide links to articles with instructive examples and best-practices.
With hypothesis testing, we surrender our predictive beliefs that envision how the future will unfold. Instead, we build from the bottom up, testing each small piece as we go, minimizing the risk to capital and cutting losses early. In effect, we make ourselves intellectually humble and admit we have little knowledge of the future. We accept that we don’t know what we don’t know and are unlikely to ever really know much in advance. We can only discover it through experimentation.
Most importantly, hypothesis testing minimizes the biases described above that slow our learning. With it, we actually get paid to learn and use objective data to validate or falsify our ideas. We minimize sunk costs thereby making it less likely to cling to a failed idea. We use randomness to help us learn instead of fooling us into seeking a reward where none is to be found. Charismatic personalities have less sway when objective data is the measuring tool. And finally, being wrong is accepted as the normal state and part of the experiment. In effect, we are using an evidence-based decision system over one based on omnipotence and superstition.
We can further inoculate ourselves against bias by placing strict, consistent limits on the amount of capital allocated to hypotheses and requiring short timeframes for proving them true. Otherwise, we are right back to endeavors that need “just a little more time” or “just a little more money.” Evolution allows no such exemptions. Ultimately, we need to decide if we want to be “right” or make money. We sometimes seek the former while claiming to seek the latter.
Admittedly, this approach doesn’t yield a particularly motivating rally cry like that of the predictive approach’s “Full speed ahead!” By contrast, “Let’s run an experiment” is hardly as energizing. But it has the potential to be more profitable which, perhaps, carries its own motivation.
A Common and Misguided Strategy
“The fault, dear Brutus, is not in our stars,
But in ourselves…”Julius Caesar (Act 1, Scene 2)
Perhaps we have a biased sample set in our industry and hear only the stories of predictive planning nightmares and not the successes, making us believe that the nightmare scenario is the common one. But given so many stories from so many people over so many years, it seems that the scenario is probably representative for many work environments. It contains the worst possible choices and almost always leads to failed outcomes.
Here’s how it occurs: We have a generic, somewhat vague goal like “increase revenue from our website by ten percent next year.” Or maybe it’s more specific like “add a left-handed widget to our data portal because customers will buy it.” Whatever it is, it typically isn’t well-specified and the assumptions underlying the premise are just that: assumptions. And hidden details will surely appear as we begin work. We have done similar features in the past but, crucially, we have never done exactly the same thing before. But that should be “good enough” for the basis of our prediction. We then have one, perhaps two, developers provide a prediction that is little more than an off-the-cuff guess. And then we are off to the races. It often goes like this in predictive environments:
Manager: “How long will it take to write the Widget feature?”
Programmer: “I don’t know, maybe a month.”
Manager: “What? That’s ridiculous! There’s no way it will take that long!”
Programmer: “Well, OK, I can probably do it in a week.”
Manager: “That’s more like it. I’ll put it in the schedule. Do it next week.”
In an Agile environment it might look like this:
Manager: “How many story points are you estimating for the Widget story?”
Programmer: “I don’t know, maybe it’s a thirteen.”
Manager: “What? That’s ridiculous! There’s no way it’s that big!”
Programmer: “Well, OK, it’s probably a three.”
Manager: “That’s more like it. I’ll update the backlog. Do it in the next sprint.”
This is little more than random guessing under extreme duress and creates the worst possible conditions: vague specifications, no rigorous collection of historical data upon which to draw for a careful, statistical analysis, off-the-cuff predictions from one or two programmers, and turning the guess into a commitment to deliver according to a schedule. To this mix, add incentives for managers to “hold developers accountable” for failing to deliver what they never realized was a promise instead of a guess and the understandable fear of punishment for being wrong about their guess once it becomes a commitment. Is it any wonder that failure is an inevitable outcome? They only way it is delivered is by cutting features, heroic overtime, and sacrificing quality. And yet, the lesson is rarely “this isn’t working so we need to try something else.” Instead, it’s often “we need to get better at predictions.”
We get what we pay for. If we are required to use predictions to derive plans then we must invest the time and money to do it right. If we use Agile methods then the delivery of working software must take precedence over predictions. To do otherwise is wishing to get something for nothing. As the Second Law of Thermodynamics makes clear, “There’s no free lunch.”
Know Thine Environment
It is imperative to know the environment in which our businesses are operating. If we work on large, contract-driven projects where timelines are extended and the specifications are well-defined in advance, then quantitative prediction is usually a required skill to survive. On the other hand, if we operate in the more common environment where specifications are vague or non-existent, the market needs are unclear or unknowable, timelines are short and urgent, and competition for market share is fierce, then we should consider a hypothesis-driven approach.
A key problem is that we often misunderstand the mathematical underpinnings of our environment. We often believe that we operate in a deterministic world where more effort will reward us with a successful result. In fact, we often are operating in a non-deterministic, highly empirical world with an unstable foundation that changes with time. Statisticians call this a problem with “a non-stationary base” where the mathematical foundation is not stable and there is no base upon which to anchor our assumptions. Under these conditions, fixed, deterministic methods will not succeed outside of sheer, random luck. For all of the biases listed above, it’s nearly irresistible to believe that we can predict and plan even when we can’t.
Unfortunately, if we are not operating under stable conditions then greater effort put into a prediction has a higher chance of increasing our confidence in its accuracy than it does in improving the accuracy itself. And so we become more certain of our wisdom than we do of our doubt. We are then prone to commit ever more capital to prove that we are right instead of cautiously guarding our resources and applying them when the data tell us we are on the right path.
Knowing the environment in which we operate means that pay incentives are aligned with methods that produce successful outcomes for that environment. We are incentivized to learn, in whatever form it may take for our environment.
Final Thoughts
One of the key difficulties with predictions lies in our natural human reluctance to accept uncertainty. Being in a state of uncertainty and doubt is an extremely uncomfortable place. So we are much more inclined to embrace those who are full of confidence than we are those who shrug and prefer to run an experiment to verify a hypothesis.
The external reality is that the business environment is often governed by uncertainty, unknowable unknowns, and darkness that we must navigate with only the faintest of lights. Our challenge is to accept the disquieting nature of that environment instead of clinging to the comfort of a belief system that provides us with a reassuring but misleading picture.
The road to knowledge is paved with the embrace of uncertainty. If we can learn to live with its discomfort then we open the path to learning. To paraphrase a famous saying: The price of learning is eternal unease.
Footnotes
1Standish Group 2015 Chaos Report.
2“No Silver Bullet — Essence and Accident in Software Engineering”, by Fred Brooks.
Proceedings of the IFIP Tenth World Computing Conference: 1069–1076.
3 ” ‘SUPERSTITION’ IN THE PIGEON“, B.F. Skinner, Journal of Experimental Psychology, 38, 168-172.
4 “On Being Wrong” by Kathyrn Schulz.
5 “A Gentle Introduction to Monte Carlo Simulation for Project Managers” by Kailash Awati.
6 “Web-Based Monte Carlo Simulation for Agile Estimation” by Thomas Betts.
7 “The Scrum Primer” by Pete Deemer and Gabrielle Benefield.
8 “How to Implement Hypothesis-Driven Development” by Barry O’Reilly
9 “Why hypothesis-driven development is key to DevOps” by Brent Aaron Reed and Willy-Peter Schaub
10 “Hypothesis-driven development” by Adrian Cho
About the Author
J. Meadows is a technologist with decades of experience in software development, management, and numerical analysis. The author is an occasional contributor to InfoQ.
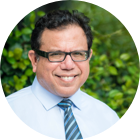
MMS • Arthur Casals
Article originally posted on InfoQ. Visit InfoQ
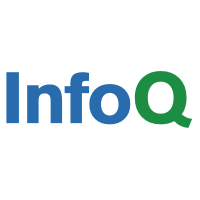
Earlier this month, Pulumi announced the addition of .NET Core to their supported languages. Pulumi is an open-source tool that allows the creation, deployment, and management of infrastructure as code on multiple cloud providers, similarly to HashiCorp Terraform.
Infrastructure as Code (IaC) is the process of managing infrastructure in a descriptive model using configuration files, used by DevOps in conjunction with Continuous Delivery (CD). Most major cloud providers offer their own IoC solution, usually based on JSON or YAML configuration files. These files can be then be integrated into the development process, being maintained and versioned in code repositories. Examples of IaC tools include Chef, Puppet, AWS CloudFormation, and Azure Resource Manager (ARM).
Launched last year, Pulumi is a relatively new player on the IaC scene. According to their website:
The Pulumi Cloud Development Platform is a combination of tools, libraries, runtime, and service that delivers a consistent development and operational control plane for cloud-native infrastructure. Not only does Pulumi enable you to manage your infrastructure as code, but it also lets you define and manage your infrastructure using real programming languages (and all of their supporting tools) instead of YAML.
Similarly to HashiCorp Terraform, Pulumi uses programs for partitioning, configuring, and scaling (provisioning) virtual environments. However, while in Terraform these programs are written in a custom domain-specific language (DSL), Pulumi’s programs are written in general-purpose languages. The addition of .NET Core support allows Pulumi programs to be written using C#, VB.NET, or F#.
Using a general-purpose language allows the integration of IaC with the existing language ecosystem. In the case of .NET, the benefits include integration with existing IDEs (including Visual Studio and Visual Studio Code), NuGet support (both for using existing libraries and distributing IaC programs), and using standard compiler errors.
Using .NET to write IaC programs also allows the use of language-specific resources, such as LINQ and async code. The code excerpt below illustrates how an Azure CosmosDB can be created with a serverless Azure AppService FunctionApp that automatically scales alongside the database:
using System;
using System.Collections.Generic;
using Pulumi;
using Pulumi.Azure.AppService;
using Pulumi.Azure.Core;
using Pulumi.Azure.CosmosDB;
class Program
{
static Task<int> Main(string[] args)
{
return Deployment.RunAsync(() => {
var locations = new[] { "WestUS", "WestEurope", "SouthEastAsia" };
var rg = new ResourceGroup("myapp-rg", new ResourceGroupArgs {
Location = locations[0],
});
var app = new CosmosApp("myapp", new CosmosAppArgs {
ResourceGroup = resourceGroup,
Locations = locations,
DatabaseName = "pricedb",
ContainerName = "prices",
Factory = (location, db) => {
var func = new ArchiveFunctionApp("myapp-func",
new ArchiveFunctionAppArgs {
Location = location,
Archive = new FileArchive("app"),
AppSettings = new Dictionary<string, string> {
["COSMOSDB_ENDPOINT"] = db.Endpoint,
},
},
);
return func.App.ID;
},
});
});
}
// Definitions of CosmosApp and ArchiveFunctionApp elided for brevity.
// Actual runtime application code is stored in the "app" directory.
// See link to the full example at the end of this article.
}
(source: original announcement)
A full CosmosApp example can be found here. In addition to .NET, Pulumi also supports JavaScript, TypeScript, Python, and Go. A full list of all supported cloud providers can be found here. Pulumi is also open source on GitHub.
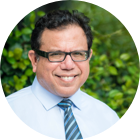
MMS • Ben Linders
Article originally posted on InfoQ. Visit InfoQ
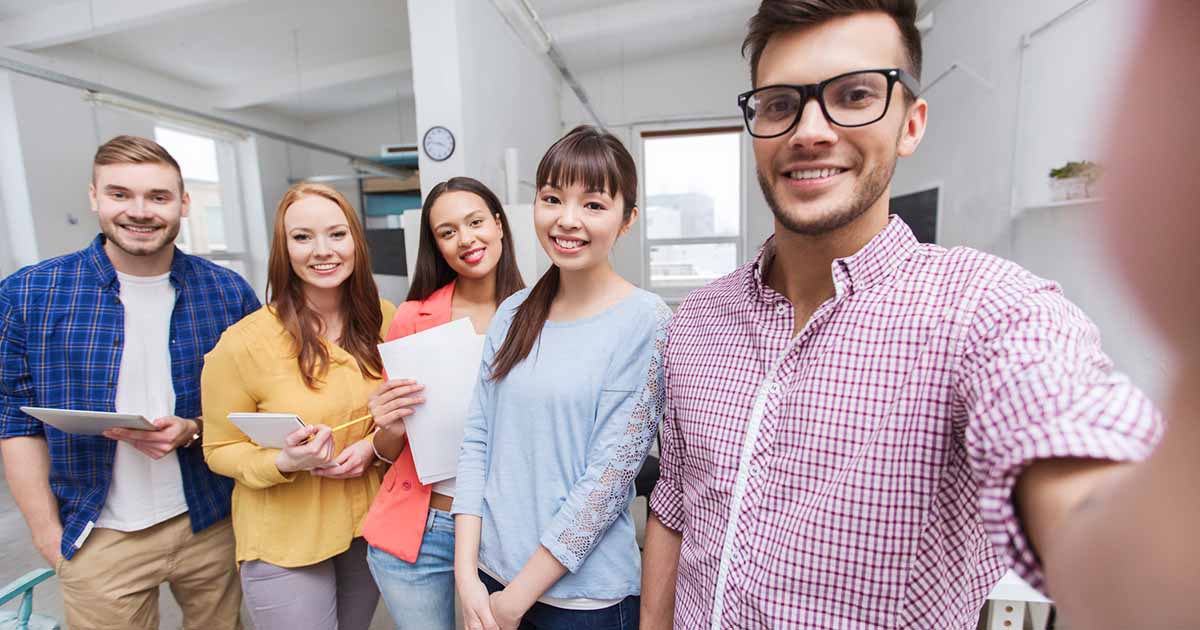
More teamwork, a better vision of daily work, a team that works in a concentrated way, and more pride in doing a job well; these are the benefits that Mélanie Noyel mentioned that their IT team at Acta gained from using Lean. At the Lean Digital Summit 2019 she presented on how they applied Lean to improve the IT team’s daily work.
The IT team is composed of two people for development and three for system and network. The day-to-day work is divided among projects, customer support, development and maintenance for 18 business applications and various IT training.
In early 2018, the situation was disappointing, as Noyel mentioned:
The team was overloaded under support work, customers were not really satisfied, projects dragged on, and the top management pushed for a different way of organizing. Drowned in this situation, we didn’t see how to get out of it.
As Acta was already familiar with Lean, the IT team decided to try the Lean IT Academy, a rendez-vous held once a month in the company to learn how to apply Lean principles to the development and management of IT products and services. “What makes it different is that we see a little of Lean theory and a lot of gemba,” Noyel explained.
Before, we just did day-to-day work; from problem-solving to more problem-solving, without a real global vision. Now, we have defined our goals focused on the opinion of our customers. And we made it visual so that the whole team gets it right and pulls in the same direction.
Noyel mentioned that the team members have become proud of their achievements, building self-confidence from success:
The first PDCAs allowed each team member to realize that they were able to tackle big problems and solve them. Later, satisfied customers started to give positive feedback. Then, the other services started wanting to work like us. We regularly present what we are doing, including at this year’s Lean Digital Summit, and it’s a real reward for the pride of the team.
InfoQ interviewed Mélanie Noyel, head of IT at Acta, after her talk at Lean Digital Summit 2019.
InfoQ: What made you decide to go on a Lean journey?
Mélanie Noyel: The opportunity that we got! The Lean mentality was already familiar within the company. Indeed, Acta is followed by the “Lean Institut France” and benefits from coaching in Lean engineering and Lean manufacturing. Because IT is also a particular domain and because the situation had to be improved, we start talking about another type of coaching for us: the Lean IT Academy. I thought it would help us to take time to start something and to get ideas.
InfoQ: How did the Lean transformation go, can you give some examples of what has changed?
Noyel: A first example is the reliability of our system. One way to measure it is to follow how long we spend on support in a week. We set an objective to reduce it by half in one year. We color a box per 15 minutes spent doing support on a visual graph every evening before leaving work, and we discuss it every morning during the stand up meeting. The goal is to understand what makes us waste time, and trigger PDCA to find the root causes and solve it. We have already met our objective to reduce it by half, but now it becomes harder to continue to reduce the support time.
The stand up meeting is the second great change we made. We set up this time for a regular exchange every morning to organize the work of the day and decide things together. It’s a way to align us on priorities.
To conclude, I mostly changed my mind! I’m no longer here to decide who does what but to make sure that we maintain the things that make our victories possible, and that the obstacles we encounter are overcome with greater ease.
InfoQ: How has the Lean transformation of Acta Mobilier IT team impacted day-to-day work?
Noyel: I think the day-to-day work has nothing to do with how it was before! Today, other offices in the company are starting to follow our example, simply because our image has changed. The other teams perceive us as more organized, more attentive to them, and as a provider of solutions (in IT but also in the organization of the day-to-day work). We also give the image of a united team, efficient and proud of our work. Our joy of living at work creates envy!
InfoQ: Which benefits have you gained?
Noyel: The IT system is more reliable and applications are faster and more intuitive. Our two types of customers are satisfied. Internal customers (other teams and workshop operators) rely on us to improve their professional lives. And external customers (those who buy the products manufactured by the company) are no longer impacted by problems related to IT. But the biggest benefit is for the people of the team! The team is closer together and ready to face bigger challenges.
InfoQ: What have you learned on your journey?
Noyel: You just have to start! Test something even if you’re not sure if it’s good or not. Test and observe what changes, then adapt and test again.
As an example, we tested allocating support to a different person per day. This brought up lots of problems regarding skills, availability, organizing the day, etc… But we learned a lot and we adapted. We did draw many improvements on the management and transfer of skills, on making visual the person assigned to support, etc…
Stay in this dynamic of improvement; celebrate your success and do not be afraid to admit your mistakes. For me, the true Lean theory becomes accessible only to those who really try.
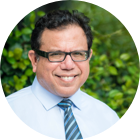
MMS • Steef-Jan Wiggers
Article originally posted on InfoQ. Visit InfoQ
In a recent blog post, Microsoft announced the 1.0 version of Kubernetes-based event-driven autoscaling (KEDA) component, an open-source project that can run in a Kubernetes cluster to provide fine-grained autoscaling (including to and from zero) for every pod and application. KEDA also serves as a Kubernetes Metrics Server and allows users to define autoscaling rules using a dedicated Kubernetes custom resource definition.
Jeff Hollan, principal PM manager, Azure Serverless, author of the blog post, told InfoQ:
KEDA is a key piece of our serverless story. KEDA and the open-sourced Azure Functions runtime provide serverless runtime and scale without any vendor lock-in. It allows enterprises to build hybrid serverless apps running across a fully managed cloud and the edge for the first time ever.
Earlier this year Microsoft announced KEDA in collaboration with Red Hat, which was well-received by users and the community. Hollan told InfoQ:
KEDA was possible because of collaboration with the community at large. Red Hat has contributed significantly to both the design and the code making KEDA work well on Open Shift. In addition, we’ve had dozens of contributors who have helped created new event sources, docs, samples, and features. The result is a tool that is better than any single organization could have created in isolation, and an open and collaborative pattern we feel is vital to bring forward.
Kubernetes is a container orchestrator, and is available on several cloud platforms as a managed service. By default, Kubernetes will only scale based on operational metrics like CPU and memory, and not in relation to application-level metrics such as thousands of messages on a queue awaiting processing. Therefore, developers need to define how their deployments need to scale by creating Horizontal Pod Autoscalers (HPA) – for which they will need to run a metric adapter to pull the metrics from the source that they want. In the case of multiple sources, it can become a challenge, and also requires more infrastructure.
However, with KEDA, Kubernetes can scale pod instances based on the knowledge of other metrics it pulls from a variety of sources. For example, it can pull information from a queue in order to learn how many messages are waiting for processing, and scale accordingly before the CPU load rises. The scaling occurs from 0 to n-instances of the application deployments, based on the configuration in the ScaledObject. Behind the scenes, KEDA adds HPAs when it needs to raise the instances of a deployment. If no instances are required, KEDA will delete the HPA.
Tom Kerkhove, Azure Architect at Codit and one of the main contributors on KEDA, told InfoQ:
You could already use HPAs with metric adapters but had to find out which ones you need and cannot run multiple adapters at the same time – KEDA fixes this by aggregating from various sources.
Source: https://blog.tomkerkhove.be/2019/11/19/keda-v1-autoscaling-made-simple/
Additionally, Kerkhove shared with InfoQ other benefits of leveraging KEDA:
- Another big win is that there was no official Azure Monitor metric adapter, other than James Sturtevant his OSS project or had to use Promitor via Prometheus & the Prometheus metrics adapter.
- TriggerAuthentication now provides production-grade authentication by supporting pod identities like Azure Managed Identity, allowing you to re-use authentication information. Next to that, you can decouple the assigned permissions on your application from what KEDA needs, which reduces the exposure risk.
- Deployments got a lot simpler with Helm 2.x & 3.0 and are available on hub.helm.sh. KEDA is now based on Operator SDK and will be in Operator Hub soon.
- It now supports scaling jobs instead of only deployments.
- It will be donated to CNCF to allow it to grow and to let other vendors jump in and help improve the product by adding more scalers, better usability, etc.
Developers can learn more about KEDA at KEDA.sh or try a step-by-step QuickStart.