Category: Uncategorized
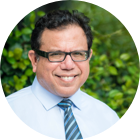
MMS • RSS
Posted on mongodb google news. Visit mongodb google news
MongoDB, Inc. (NASDAQ:MDB – Get Free Report)’s share price gapped up prior to trading on Friday . The stock had previously closed at $366.13, but opened at $382.44. MongoDB shares last traded at $380.18, with a volume of 333,939 shares traded.
Analyst Ratings Changes
Several equities research analysts recently issued reports on MDB shares. Citigroup lifted their price objective on MongoDB from $515.00 to $550.00 and gave the company a “buy” rating in a research note on Wednesday, March 6th. KeyCorp lowered their price target on MongoDB from $490.00 to $440.00 and set an “overweight” rating on the stock in a research report on Thursday, April 18th. UBS Group restated a “neutral” rating and set a $410.00 price target (down previously from $475.00) on shares of MongoDB in a research report on Thursday, January 4th. Loop Capital started coverage on MongoDB in a research report on Tuesday. They set a “buy” rating and a $415.00 price target on the stock. Finally, Redburn Atlantic restated a “sell” rating and set a $295.00 price target (down previously from $410.00) on shares of MongoDB in a research report on Tuesday, March 19th. Two equities research analysts have rated the stock with a sell rating, three have issued a hold rating and twenty have issued a buy rating to the stock. According to data from MarketBeat, the company presently has an average rating of “Moderate Buy” and an average price target of $443.86.
View Our Latest Research Report on MDB
MongoDB Price Performance
The company has a current ratio of 4.40, a quick ratio of 4.40 and a debt-to-equity ratio of 1.07. The stock has a market capitalization of $27.95 billion, a PE ratio of -147.63 and a beta of 1.19. The firm has a 50 day moving average price of $381.23 and a 200 day moving average price of $390.50.
MongoDB (NASDAQ:MDB – Get Free Report) last posted its quarterly earnings data on Thursday, March 7th. The company reported ($1.03) EPS for the quarter, missing the consensus estimate of ($0.71) by ($0.32). The company had revenue of $458.00 million during the quarter, compared to analysts’ expectations of $431.99 million. MongoDB had a negative return on equity of 16.22% and a negative net margin of 10.49%. Research analysts anticipate that MongoDB, Inc. will post -2.53 earnings per share for the current year.
Insider Activity at MongoDB
In related news, Director Dwight A. Merriman sold 1,000 shares of the stock in a transaction dated Thursday, February 1st. The shares were sold at an average price of $404.20, for a total value of $404,200.00. Following the sale, the director now directly owns 527,896 shares of the company’s stock, valued at approximately $213,375,563.20. The transaction was disclosed in a filing with the Securities & Exchange Commission, which is accessible through the SEC website. In other news, Director Dwight A. Merriman sold 2,000 shares of the stock in a transaction that occurred on Monday, April 8th. The shares were sold at an average price of $365.00, for a total transaction of $730,000.00. Following the transaction, the director now directly owns 1,154,784 shares in the company, valued at $421,496,160. The transaction was disclosed in a legal filing with the SEC, which is available through the SEC website. Also, Director Dwight A. Merriman sold 1,000 shares of the stock in a transaction that occurred on Thursday, February 1st. The shares were sold at an average price of $404.20, for a total transaction of $404,200.00. Following the transaction, the director now owns 527,896 shares in the company, valued at $213,375,563.20. The disclosure for this sale can be found here. In the last quarter, insiders sold 91,802 shares of company stock valued at $35,936,911. Insiders own 4.80% of the company’s stock.
Institutional Investors Weigh In On MongoDB
Several institutional investors have recently bought and sold shares of the company. Quadrant Capital Group LLC lifted its stake in shares of MongoDB by 5.6% during the fourth quarter. Quadrant Capital Group LLC now owns 412 shares of the company’s stock worth $168,000 after buying an additional 22 shares during the period. EverSource Wealth Advisors LLC raised its holdings in shares of MongoDB by 12.4% during the fourth quarter. EverSource Wealth Advisors LLC now owns 226 shares of the company’s stock worth $92,000 after purchasing an additional 25 shares during the last quarter. Insigneo Advisory Services LLC raised its holdings in shares of MongoDB by 2.9% during the third quarter. Insigneo Advisory Services LLC now owns 1,070 shares of the company’s stock worth $370,000 after purchasing an additional 30 shares during the last quarter. Yousif Capital Management LLC raised its holdings in shares of MongoDB by 3.9% during the fourth quarter. Yousif Capital Management LLC now owns 792 shares of the company’s stock worth $324,000 after purchasing an additional 30 shares during the last quarter. Finally, Valley National Advisers Inc. raised its holdings in shares of MongoDB by 5.9% during the fourth quarter. Valley National Advisers Inc. now owns 597 shares of the company’s stock worth $244,000 after purchasing an additional 33 shares during the last quarter. 89.29% of the stock is currently owned by institutional investors and hedge funds.
About MongoDB
MongoDB, Inc, together with its subsidiaries, provides general purpose database platform worldwide. The company provides MongoDB Atlas, a hosted multi-cloud database-as-a-service solution; MongoDB Enterprise Advanced, a commercial database server for enterprise customers to run in the cloud, on-premises, or in a hybrid environment; and Community Server, a free-to-download version of its database, which includes the functionality that developers need to get started with MongoDB.
Recommended Stories
This instant news alert was generated by narrative science technology and financial data from MarketBeat in order to provide readers with the fastest and most accurate reporting. This story was reviewed by MarketBeat’s editorial team prior to publication. Please send any questions or comments about this story to contact@marketbeat.com.
Before you consider MongoDB, you’ll want to hear this.
MarketBeat keeps track of Wall Street’s top-rated and best performing research analysts and the stocks they recommend to their clients on a daily basis. MarketBeat has identified the five stocks that top analysts are quietly whispering to their clients to buy now before the broader market catches on… and MongoDB wasn’t on the list.
While MongoDB currently has a “Moderate Buy” rating among analysts, top-rated analysts believe these five stocks are better buys.
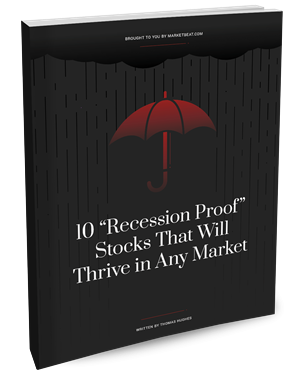
Which stocks are likely to thrive in today’s challenging market? Click the link below and we’ll send you MarketBeat’s list of ten stocks that will drive in any economic environment.
Article originally posted on mongodb google news. Visit mongodb google news
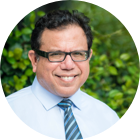
MMS • Sergio De Simone
Article originally posted on InfoQ. Visit InfoQ
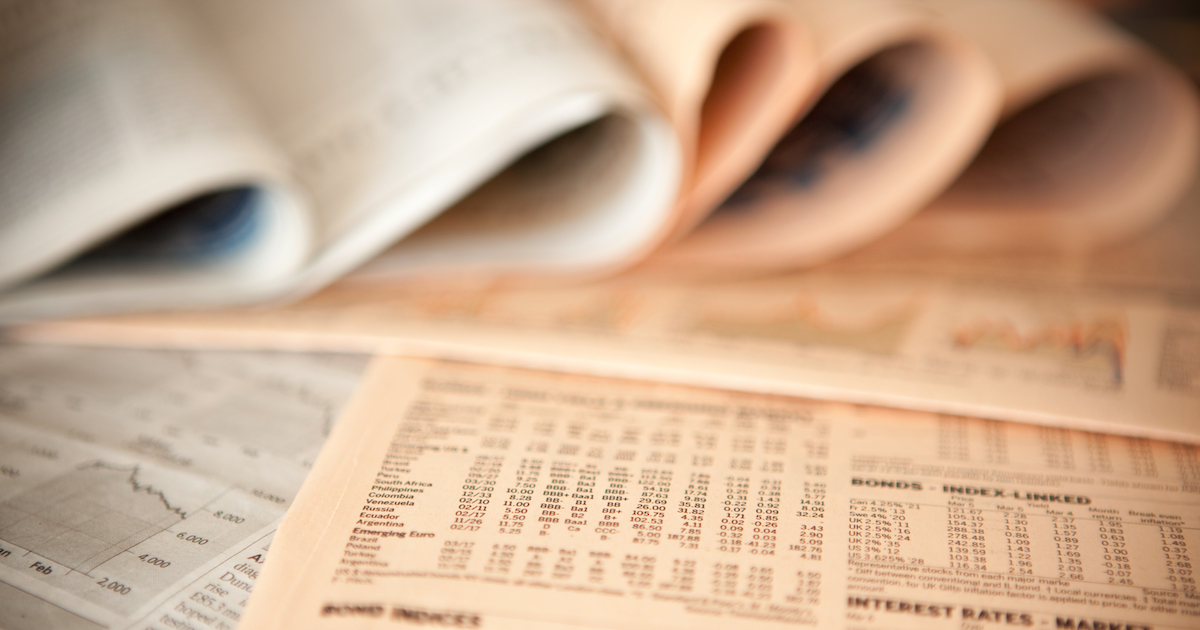
JetBrains IDE Services aims to help enterprises manage their JetBrains tool ecosystem more efficiently and boost developer productivity at the enterprise scale through the integration of AI, remote collaboration, and more.
JetBrains IDE Services is a centralized suite of tools, including IDE Provisioner, AI Enterprise, License Vault, Code With Me Enterprise, and CodeCanvas. JetBrains says it is now making it generally available after testing it in beta with several large JetBrains customers and addressing their feedback.
IDE Provisioner makes it easier to centralize IDE management across versions, configurations, and plugins and helps avoid unapproved or outdated versions being used within the organization. IDE Provisioner also supports a private plugin repository where you can configure which plugins are publicly available and which are only visible to authenticated users.
AI Enterprise gives enterprises control over security, spending, and efficiency for AI-driven features such as code generation and task automation, and allows them to choose the best-in-class LLM provider.
License Vault aims to automate the distribution of licenses for JetBrains IDEs across the entire organization. It supports three licensing models: pre-paid, fully postpaid, and mixed IDE licensing. Additionally, it offers the option of using floating licenses, which are released to the pool of available licenses after 20 minutes of no use.
Code With Me Enterprise offers real-time collaboration solutions for developers with a focus on security for remote workers. It supports pair programming in either full-sync or follow mode; includes a teacher-student scenario; and allows up to five coders to edit the same file simultaneously.
Finally, CodeCanvas is a self-hosted remote development environment orchestrator aiming to simplify the setup and management of dev environments.
IDE Services consists of three components: the IDE Services Server, the Toolbox App, and a plugin for IntelliJ-based IDEs.
The IDE Services Server is available as a Docker image and provides the core functionality that can be run using Docker Compose or Kubernetes. The Toolbox App, available for Windows, macOS, and Linux, is installed on developer machines and is used to download, update, and configure IntelliJ-based IDEs. The plugin for IntelliJ-based IDEs has three main capabilities: receiving the IDE builds recommended by the organization, including settings and plugins; getting access to pre-approved plugins; and setting up secure collaboration sessions for Code With Me Enterprise.
As a final note, JetBrains IDE Services also provides a REST API which enables running all essential operations on the IDE Services Server, JetBrains says.
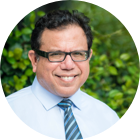
MMS • RSS
Posted on mongodb google news. Visit mongodb google news
Press Release, April, Orbis Research -The comprehensive report on the global Open Source Big Data Tools market meticulously examines various critical aspects, aiming to provide a thorough understanding of the market landscape to industry stakeholders. With a keen focus on the strategies adopted by key players, geographical expansion initiatives, market segmentation dynamics, competitive forces, manufacturing intricacies, and pricing strategies, each section of the research study is meticulously crafted to uncover pivotal insights.
Request a sample report @ https://www.orbisresearch.com/contacts/request-sample/7185077
One of the primary areas of exploration within the report is the examination of market dynamics, where a deep dive into the drivers, constraints, trends, and opportunities shaping the global Open Source Big Data Tools market is conducted. Through a blend of qualitative analysis, which delves into the intricacies of consumer behaviour and industry trends, and quantitative analysis, which provides numerical data to quantify market trends, the report offers a comprehensive understanding of the markets trajectory. Moreover, in addition to traditional market analyses, the report also incorporates strategic frameworks such as SWOT analysis, PESTLE analysis, and Porters Five Forces analysis to provide a holistic perspective on the market dynamics.
Open Source Big Data Tools market Segmentation by Type:
Language Big Data Tools
Data Collection Big Data Tools
Data Storage Class Big Data Tools
Data Analysis Big Data Tools
Others
Open Source Big Data Tools market Segmentation by Application:
Bank
Manufacturing
Consultancy
Government
Other
Direct Purchase the report @ https://www.orbisresearch.com/contact/purchase-single-user/7185077
The assessment of leading players within the global Open Source Big Data Tools market is another crucial aspect covered in the report. This evaluation involves a detailed examination of various facets of the players operations, including their market share, recent strategic moves such as product launches and partnerships, innovations in product offerings, mergers and acquisitions, and their target markets. Furthermore, the report provides an exhaustive analysis of the product portfolios of these key players, shedding light on the specific products and applications they prioritize within the market.
Key Players in the Open Source Big Data Tools market:
MongoDB Inc.
AQR Capital Management
Apache
RapidMiner
HPCC Systems
Neo4j?Inc.
Atlas.ti
Qubole
Qualtrics
Pentaho
Cloudera
Google
GitHub
Kaggle
Greenplum
Additionally, the report presents dual market forecasts, offering insights into both the production and consumption sides of the global Open Source Big Data Tools market. These forecasts are based on meticulous analysis of various factors influencing market demand and supply dynamics, including technological advancements, regulatory changes, and shifting consumer preferences. Moreover, the report goes beyond mere forecast numbers to provide actionable recommendations for both new entrants and established players in the market, helping them navigate the complexities of the global Open Source Big Data Tools market landscape effectively.
Do You Have Any Query Or Specific Requirement? Ask to Our Industry Expert @ https://www.orbisresearch.com/contacts/enquiry-before-buying/7185077
The report serves as a vital repository of essential statistics regarding the current market status of Open Source Big Data Tools manufacturers, offering invaluable guidance and insights for companies and individuals operating within or interested in the industry. It represents a comprehensive source of information that aids in navigating the complexities of the market landscape and making informed decisions.
At its core, the report offers a foundational overview of the industry, encompassing its definition, applications, and underlying manufacturing technology. By providing a clear understanding of these fundamental aspects, it lays the groundwork for deeper insights into the market dynamics and trends.
About Us
Orbis Research (orbisresearch.com) is a single point aid for all your market research requirements. We have a vast database of reports from leading publishers and authors across the globe. We specialize in delivering customized reports as per the requirements of our clients. We have complete information about our publishers and hence are sure about the accuracy of the industries and verticals of their specialization. This helps our clients to map their needs and we produce the perfect required market research study for our clients.
Contact Us:
Hector Costello
Senior Manager – Client Engagements
4144N Central Expressway,
Suite 600, Dallas,
Texas – 75204, U.S.A.
Phone No.: USA: +1 (972)-362-8199 | IND: +91 895 659 5155
Email ID: sales@orbisresearch.com
Article originally posted on mongodb google news. Visit mongodb google news
Microsoft’s Azure Strength Could Rub Off On This Warren Buffett-Backed Stock And Another …
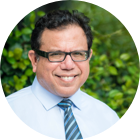
MMS • RSS
Posted on mongodb google news. Visit mongodb google news

Software giant Microsoft Corp. MSFT reported Thursday after the market close strong third-quarter results, sending its stock higher.
On Cloud Nine: Microsoft attributed the strong quarterly performance, primarily to its Cloud business. “This quarter Microsoft Cloud revenue was $35.1 billion, up 23% year-over-year, driven by strong execution by our sales teams and partners,” CFO Amy Hood said in a statement.
Microsoft’s Azure public cloud and other cloud services saw both GAAP and constant currency revenue growth of 31%.
Speaking on the earnings call, CEO Satya Nadella said, “Azure again took share as customers use our platforms and tools to build their own AI solutions.” He also noted that more than 65% of the Fortune 500 now use Azure OpenAI service.
Commenting on the Azure performance, Piper Sandler’s Brent Bracelin said the 31% growth exceeded the guidance for a 28% increase and reinforced his bullish view that Microsoft remained “best-positioned to monetize robust AI secular tailwinds while capitalizing on a first-mover advantage.”
Bracelin estimated that Azure AI contributed over a $5 billion annualized run rate.
See Also: Best Artificial Intelligence Stocks
Ancillary Beneficiaries: Calling Snowflake, Inc. SNOW and MongoDB, Inc. MDB as “Azure upside derivatives to watch,” Bracelin said these two companies have the most indirect exposure to consumption activity trends across the Cloud Titan cohort.
“Majority of revenue for SNOW is consumption-based while about 66% of revenue mix for MDB is consumption-based,” he said.
Bozeman, Montana-based Snowflake is a data-as-a-service company — backed by Warren Buffett‘s Berkshire Hathway — offering a cloud-based data storage and analytics service and New York-based MongoDB is a provider of commercial support for the source-available database engine MongoDB.
In premarket trading on Friday, Snowflake rose 3.19% to $157.37 and MongDB climbed 3.55% to $379.13, according to Benzinga Pro data. Microsoft rallied 4.34% to $416.34.
Photo via Shutterstock
Market News and Data brought to you by Benzinga APIs
© 2024 Benzinga.com. Benzinga does not provide investment advice. All rights reserved.
Article originally posted on mongodb google news. Visit mongodb google news
Presentation: Sleeping at Scale – Delivering 10k Timers per Second per Node with Rust, Tokio, Kafka, and Scylla
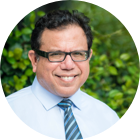
MMS • Lily Mara Hunter Laine
Article originally posted on InfoQ. Visit InfoQ
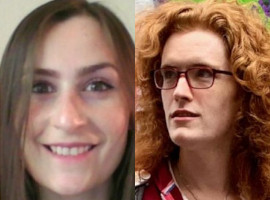
Transcript
Mara: My name is Lily Mara. I’m an engineering manager at a software company here in the Bay Area called OneSignal. We are a customer messaging company. We help millions of application developers and marketers connect with billions of users. We send around 13 billion push notifications, emails, in-app messages, and SMS every single day. I started using Rust professionally in 2016. I started using it as my main daily programming language professionally in 2019, when I started at OneSignal. I’m the author of the book “Refactoring to Rust” that is available now in early access at manning.com.
Outline
What are we going to be talking about? We’re going to be talking about how we built this scalable, high throughput timer system at OneSignal. We’re going to be talking about the motivation behind building it, in the first place. The architecture of the system itself. How the system performed. How we scaled it up, and some future work that we are maybe thinking about doing in the system.
Building a Scalable, High Throughput Timer System (OneSignal)
Let’s jump back in time a little bit. It’s 2019, I’ve just started at OneSignal. I’ve just moved to sunny California from horrible, not sunny Ohio. Everybody in OneSignal, everybody in the customer messaging sphere is talking about the concept of journey builders. If you’re not a content marketer, you might not know what a journey builder is, so just a quick crash course. This is a no-code system that allows marketers to build out customer messaging flows. This is a flowchart that’s going to be applied at a per user level. This is very similar to what a marketer might put together themselves. Over here on the left, every user is going to start over here. There’s, immediately going to be dumped into a decision node. We allow our customers to store arbitrary key-value pairs at the user level. In this case, a customer might be storing what’s your favorite color as one of those key-value pairs. We’ll make a decision based on that. Let’s say for the sake of argument that this user’s favorite color was blue, we’re going to send that user a push notification that says, “There’s a 20% off sale on blue shirts, we know how much you love blue. Aren’t you so excited about the blue shirt sale?” Then the next thing that’s going to happen is we are going to wait 24 hours. We’re going to do nothing for a day. This was the missing piece. We thought we understood how to build the event system, we understood how to build the node walking tree, but we didn’t have a primitive in place in our network for scheduling, for storing a bunch of timers and expiring them performantly. This is what we’re going to be building today. After we do that 24 hour wait, we are going to have another decision node. We’re going to say, did that particular user click on that particular push notification we sent them? If they did, we will say, “Mission accomplished. Good job, journey. You did the engagement.” If they didn’t, we’ll send them an SMS that has more or less the same message. You might want to do this because sending an SMS has a monetary cost associated with it. Carriers will bill you for sending SMS. Twilio will bill you for sending SMS. Push notifications have basically zero marginal cost. If you want to use SMS, you might want to use it as a second-order messaging system to get in contact with your customers. That’s a journey builder. That’s what we’re going to try to enable the construction of today.
What are the requirements on this timer system that we’re going to build? We want to be able to store billions of concurrent timers because we want to be able to support the billions of user records that we already have. We want to be able to expire those timers performantly. We want to minimize data loss because, of course, we don’t want to be dropping timers on the floor. We don’t want people to get stuck in particular nodes of their journeys. We want to integrate well with the rest of the data systems that we have at OneSignal. We’re a relatively small company. We don’t have the resources to have a team for every particular data system that’s out there. We don’t want to adopt 50 completely different open source data systems. Let’s get in the headspace a little bit. We realized very crucially that if we wanted to build a timer, we had to think like a timer. We took the project and we set it down for a year, and we didn’t think about it that hard. We prioritized other initiatives.
Jumping forward once again, we’re in the beginning of 2021. We have the resources to start investigating this project. We have to make a decision, are we going to build something completely from scratch ourselves, or are we going to buy an off-the-shelf system? Is there an open source timer, an open source scheduling system that we can just use? The first place we looked were generic open source queuing systems like Sidekiq and RabbitMQ. We already operate Sidekiq very heavily at OneSignal. It’s the core of our delivery API, and a lot of our lower throughput scheduling jobs. These general-purpose queuing systems, they had a lot of features that we didn’t really need, we didn’t want to pay to have to operate. They were lacking in the area that was the most important to us, and that was performance. We didn’t think that these systems were going to scale up to the throughput that we were expecting this timer system to have. The published performance numbers for things like RabbitMQ just seemed orders of magnitude off from what we wanted out of this system. We knew from experience what Sidekiq was like to scale, and we didn’t think that was going to be good enough. Based on the published numbers, we thought that other things weren’t going to be good enough. We opted to build something for ourselves, we’re going all the way.
Existing Systems (OneSignal)
Once again, let’s look at these requirements. We want to be able to store tons of timers, expire them performantly, minimize data loss, and interoperate with the rest of our system. Let’s talk about the rest of the systems. What is the prior art for system design at OneSignal? We have a lot of things written in Rust. We actually currently, but not at the time, had a policy around only using Rust for new systems. At the time, we were also spinning up new services in Go. We use Apache Kafka very heavily for our async messaging applications. We also use gRPC for all of our internal synchronous RPCs. Also, as a part of the larger journeys’ initiative, we were going to be using Scylla, which is a C++ rewrite of Apache Cassandra.
Ins & Outs
Let’s talk about the big blocks, how the data are flowing in and out of the system. Probably, sensibly, the input to the system is a timer. A timer is an expiry time, a time to end that, and a thing to do once it has ended an action. What are the actions? Initially, we came up with a pretty short list that is sending a message like a notification, email, SMS, in-app message, or it’s adding a tag to a particular user. We realized that we might come up with more actions in the future so we didn’t necessarily want to constrain ourselves to a fixed list. We also realized we might come up with actions that had absolutely nothing to do with journeys, absolutely nothing to do with messaging. That this timer system, this scheduler system has a broad range of applicability, and we wanted to leave the door open for us to take advantage of that. We imposed a new requirement on ourselves, we said we wanted this system to be generic. Given that this system is going to be generic, what is an action? What does it look like? How does it work? In addition to being generic, we wanted it to be simple. That meant constraining what an action is, even though it’s flexible.
We didn’t want to give people a whole templating engine or scripting library, or something like that. We wanted it to be pretty straightforward, you give us these bits, and we will ship them off when the time comes. Are we going to let people send HTTP requests with JSON payloads? All of our internal services use gRPC, so probably not that. Maybe they’ll be gRPC requests then. Both of these systems suffer from the same, in our mind, critical flaw, in that these are both synchronous systems. We thought it was really important that the outputs of the timer system be themselves asynchronous. Why is that? As timers start to expire, if there’s multiple systems that are being interfaced with, say notification delivery and adding key-value pairs. If one of those systems is down, or is timing out requests, we don’t want, A, to have to design our own queuing independence layer in the timer system, or, B, have our request queues get filled up with requests for that one failing system, to the detriment of the other well behaving systems. We wanted the output of this to be asynchronous. We opted to use Apache Kafka, which as I mentioned, is already used very heavily at OneSignal. We already have a lot of in-house knowledge and expertise on how to operate and scale Kafka workloads. It gave us a general-purpose queuing system that was high performance. A really key benefit is it meant that the timer system was isolated from the performance of the end action system. What about these inputs? What about the timers themselves? Because all the timers are being written into the same place by us, we can own the latency of those writes, so this can be a synchronous gRPC call.
Interface
The interface, broadly speaking, looks like this. External customers make a gRPC request to the timer system. They’re going to give us an expiry time, and they’re going to give us an action, which is a Kafka topic, Kafka partition, and some bytes to write onto the Kafka stream. Then later on, when that timer expires, we’re going to write that Kafka message to the place that the customer has specified. We’ll delve into the magic box of the timer system as we go through this. Hopefully, later on, presumably at some point, the team that enqueue the timer will have a Kafka Consumer that picks up the message and acts on it. The really important thing here is the consumer picking up the message and acting on it is totally isolated from the timer system, dequeuing the message and shipping it across the wire. If a Kafka Consumer is down or not performant, that really has nothing to do with the timer system. It’s not impacting us at all.
Internals
Let’s delve into the internals of this a little bit. How are we going to store timers after they come across the gRPC interface? How are we going to expire timers to Kafka after they’ve been stored? What are the key health metrics of the system? Let’s get into it. I’d first like to talk about the timer expiry process. We know we’re going to be writing timers to Kafka. We know there’s a gRPC service on the other side that has some storage medium attached to it for these timers. We want to try to build something that’s relatively simple, relatively straightforward for pulling these things out of storage and expiring them to Kafka. How are we going to do this? We came up with this architecture as our first pass. We’re still abstracting the storage away. We have our gRPC service, we’ve added a couple new endpoints to it. There’s a get timers endpoint that takes in a timestamp, and it’s going to serve us back all the timers that expire before this timestamp. Every minute, this new scheduler box over here, that’s a scheduling service written in Rust, it’s going to send a gRPC request up to our service. It’s going to say, give me all the timers that expire before 10 minutes into the future. If it’s currently 4:48, we’re going to say, give me all the timers that expire before 4:58. It’s going to take all those timers, write them into a pending area in memory, and have some in-memory timers. Then it’s going to expire those out to Apache Kafka as those timers expire. Once they are expired and shipped off to Kafka, we are going to send a delete request up to the gRPC service to remove that particular timer from storage so that it’s not served back down to the scheduler.
We viewed this as a relatively simple solution to the problem, because we thought that this timer system was going to be pretty tricky to implement in the first place. We were a bit incredulous when we came up with this solution. We weren’t sure how we were going to represent the timers in memory. We weren’t sure how we’re going to avoid doubling-enqueuing. Once we just coded up a first pass, we realized that it was actually so much simpler and so much more performant than we thought it was going to be. We basically created an arena for these pending timer IDs in memory. We had an infinite loop with a 1-second period. We pulled all the timers from the gRPC interface, looped over them, and checked to see if they were known or not. If they were not known to the instance, we would spawn an asynchronous task using tokio. Use their built-in timer mechanism. When that timer expires, we would produce the Kafka events, delete the timer from the gRPC interface. Then there was a bit of additional synchronization code that was required to communicate this back to the main task so that we could remove that particular timer ID from the hash set. That was the only complicated piece here. The real service implementation is really not a whole lot more complicated than this. We were pretty impressed that it was quite so simple.
How does this thing actually perform? What do the numbers look like? Using the built-in default tokio async future spawning, and sleep_until functions, we tried to spawn a million timers, and measure both the latency and the memory utilization. We found that it took about 350 milliseconds to spawn a million timers. That ate about 600 megabytes of memory, which is a relatively high amount of memory for a million timers. It’s about 96 bytes per timer, which seems a bit heavy. We decided that this was good enough performance metrics to go out with. We were not going to invest too heavily at this point in ultra-optimizing from here. What key performance metrics did we identify once we were ready to ship this thing? The first one was the number of pending timers in that hash set. The number of things that we are watching right now. This was really important for us when we started getting out of memory kills on this timer, because we had not put any backpressure into the system, so if there were too many timers expiring at say 4:00, 4:00 rolls around, you try to load those all into the scheduler. Scheduler falls down. Scheduler starts back up. It tries to load the timers again, and it keeps falling over. We use this metric to identify what’s falling over at a million, let’s tell it to not load any more than 600,000 into memory. The other one was a little bit less intuitive. It was the timestamp of the last timer that we expired to Kafka. We use this to measure the drift between the timer system and reality. If it’s currently 4:00, and you just expired a timer for 3:00, that means your system is probably operating about an hour behind reality. You’re going to have some customers who are maybe asking questions about why their messages are an hour late. This was the most important performance metric for the system. This is the one that we have alerting around. If things start to fall too far behind reality, we’ll page an engineer and have them look into that.
The Storage Layer
Next, I’d like to talk about the storage layer for the system. Thinking about the requirements of this, we wanted to support a high write throughput. The goal that we had in mind was about 10,000 writes per second. From our experience operating Postgres, we knew that this was definitely possible to do with Postgres, but it was a big pain in the butt if you didn’t have a lot of dedicated Postgres staff, and a lot of infrastructure dedicated to clustering in Postgres. We didn’t really want to use Postgres for this. We wanted something that was going to be simple to scale, so that when we needed additional capacity, we could just throw it at the wall and have it stick. We wanted something that would be simple to maintain, so zero downtime upgrades were going to be really important to us. We knew that we were going to be making relatively simple queries that might serve back a bunch of results. What is a simple query? What is the kind of queries that we’re going to be making? The scheduler is going to be doing queries that look like, give me all of the timers that are expiring in the next 10 minutes. That is not a very complicated query. It’s not, give me all the timers that are expiring in the next 10 minutes for this particular customer, that are targeting these 10,000 users in these time zones, relatively straightforward queries. We didn’t necessarily need all the querying, filtering power of something like Postgres or another relational database.
In the end, we picked Scylla. This was already something that we were spinning up as a part of the larger journeys project. Even though we didn’t have existing in-house experience operating Scylla, we knew that we were going to be developing it as another business line item. One thing that we had to think about with adopting Scylla was that the data modeling approach for Scylla and Cassandra are very different from something like Postgres. We need to think pretty hard about how we’re going to be writing these data to the tables and how we’re going to be querying it afterwards. When you’re doing data modeling in a relational database, it’s a lot easier to just think about, what are the data and how do they relate to each other? You can, generally speaking, add any number of joins to a Postgres query, and then add any number of indices on the other side to make up for your poor data modeling. You don’t really have this luxury with Scylla, or Cassandra. They use SSTables. They don’t provide the ability to do joins. They really aren’t much in the way of indices other than the tables themselves. Ahead of time, as we were writing the data to the database, we need to be thinking about how we’re going to be querying it on the other side. The query we’re going to be doing is fetch all timers about to expire.
What does about to expire mean in this sense? If we think about the basic elements that we just said were a part of a timer, it’s got an expiry timestamp. It has a binary data blob. It has a Kafka topic and partition that the message is going to be written to. Most of the architecture work here was done by the Apache Cassandra team. My exposure to this ecosystem has all been through Scylla, so I’m going to attribute things to Scylla that were certainly done by people on the Apache Cassandra team. In Scylla, we have data that’s distributed amongst a cluster of nodes. As you query the data in the nodes, generally speaking, each query, we want it to hit a single node. We don’t want to be merging data together. We don’t want to be searching across all the nodes for particular pieces of data. Generally speaking, we want to know ahead of time where a particular row is going to land in the cluster.
How do we do that? How do we distinguish where a row is going to go? There’s a couple different layers of keys that exist on each row in Scylla, and we’re going to use those. The primary key has two parts, which is like a relational database, we have a primary key on each row. The first part is the partitioning key, that’s going to determine which node in the cluster a row is going to land on, and where on that node it’s going to go. It’s going to group the data into partitions that are shipped around as one unit. This is composed of one or more fields. There’s also a clustering key that determines where in the partition each row is going to go. That’s used for things like sort ordering. That’s optional, but it also can have a variable number of fields in it. Generally speaking, the kinds of queries, the high performance read queries that we want to be doing, you need to include the partition key, an exact partition key in each read query that you’re doing. You’re not having a range of partition keys. You’re not saying, give me everything. You need to provide, give me this partition key. The query we’re performing is get all the timers about to expire. What does about to expire mean? It means we need to pre-bucket the data. We need to group our timers into buckets of timers that expire around the same time, so that we can query all those timers together.
We’re going to be bucketing on 5-minute intervals. For example, a timer expiring at 4:48 p.m. and 30 seconds, we’re going to bucket that down to 4:45. Everything between 4:45 and 4:50, those are going to land in the same bucket. We are still going to store the expiry time, but we’re also going to have this bucket that’s specifically going to be used for storage locality. We can’t have the bucket alone be the primary key, because just like every other database that’s out there, primary keys need to be unique in tables. If the 5-minute bucket was the sole primary key, you can only have one timer that existed per 5-minute bucket. That’s not a very good data system. We’re going to introduce a UUID field that’s going to be on each row, and that’s going to take the place of the clustering key. That’s going to determine, again, where in the partition each row is going to land.
Our final table design looked like this. We had those same four fields that we talked about initially. We also introduced two new fields, this row, UUID fields, and the bucket fields, which is, again, the expiry timestamp rounded down to the nearest 5 minutes. You can see that on the primary key line down there, we have first the bucket field. That’s the partitioning key. Second, we have the clustering key field, which is the UUID field. What do the queries look like that we’re going to be doing on this table? We’re going to be getting all the fields off of each timer row inside of each bucket, inside of this 5-minute bucket that starts at 4:45. The eagle-eyed among you might already be noticing a problem with this. If it’s currently 4:48, and we get the timers that are in the bucket starting at 4:45. How are we going to do this 10-minute lookahead interval thing? How are we going to fetch the timers that start at 4:50 and 4:55? Because a 10-minute interval is necessarily going to span more than one, 5-minute data bucket. Further complicating things, this system is not necessarily always real time. It might be the case that this system is so far behind reality, which, in some cases, that might only be a couple seconds. It might be the case that there are still some existing timers that are falling into buckets that already ended. If it’s 4:45 and 10 seconds, and you still have an existing timer that was supposed to expire at 4:44 and 59 seconds, you still have to be able to fetch that out of Scylla. Because maybe the scheduler is going to restart, and it’s not going to be able to use the one that’s floating around in memory.
How are we going to pull all the buckets and get the data? We can’t just query the currently active bucket. We need to find out what buckets exist, which buckets that exist fall within our lookahead window. We need to query all of those for their timers. We introduced another table, a metadata table that was just going to hold every single bucket that we knew about. This is going to be partitioned just by its single field, the bucket timestamp. This was just going to give us access to query what buckets currently exist. Every time we insert data into our tables, we are going to do two different writes. We’re going to store the timer itself. We’re also going to do an insertion on this bucket table. Every insert in Scylla is actually an upsert. No matter how many millions of times we run this exact same query, it’s just going to have one entry for each particular bucket because they all have the same primary key. What do our queries look like? We’re first going to have to query every single bucket that exists in the database, literally every single one. That’s going to come back into the memory of our gRPC service. We’re going to say, of those buckets that exist, which ones fall into our lookahead window. That’s going to be the four buckets from 4:40 to 4:45. We’re going to query all the timers off of those. We’re going to merge them into memory of the gRPC service, and then ship them down to the scheduler.
If we put this all together into one cohesive system view. On the external team side, we have a thing that creates timers, that’s going to send a gRPC request across the wire to our service, that’s going to store a timer in our Scylla database alongside a corresponding bucket. Then, every minute, our scheduler is going to call the get timers gRPC method, with a lookahead window. It’s going to add the timers that fall into that window to its pending area in memory. When those in-memory timers expire, it’s going to write them out to an Apache Kafka topic. Eventually, maybe there’ll be a Kafka Consumer that picks that message up. This system, as I’ve described it, existed for about a year, maybe a-year-and-a-half without any major issues, modules that out of memory problem. We didn’t have to do any major scaling operations. It didn’t have any really big problems. We mostly just didn’t think about it after it was out there and running. We were pretty happy with it. Eventually, we started to think about adding more users to our journeys’ product. We started to think about using this timer system to support more use cases than just journeys. We realized that we would have to do some scaling work in this because what I’ve described has some poor scaling tendencies.
Jumping Forward, Q1 2023
Laine: My name is Hunter Laine. I’ve been an engineer on Lily’s team at OneSignal for about two-and-a-half years. In a past life, I was a marketing operations manager in Prague.
We’re going to take another leap forward in time to Q1 of this year. We have this effective timer service up and running pretty smoothly. It’s capable of storing billions of concurrent timers and expiring them in a performant manner, while minimizing data loss, and easily integrating with the rest of our systems. It’s essentially a set timeout function that’s available across our entire infrastructure, without any use case-specific limitations. It sounds pretty good. We thought so too, so we decided it was time to actually start doing that integrating with the rest of our systems.
The Case for Scaling Up
We send about 13 billion notifications a day, so we wanted to use the timer service to ease a significant amount of load on our task queues. This could be useful for a myriad of things, from retrying requests on failures across multiple services, to scheduling future notifications, and many other areas we were already excited about. If we were going to use the timer service in these many critically important areas, we needed to ensure that it could handle a lot more timers reliably than it currently could. We needed to scale up. The original motivation for and use of the timer service was to enable these journey builders, no-code systems generally use as a marketing tool. These systems constitute a significant number of timers that we were storing and retrieving. However, when compared to the task of integrating with our delivery systems, it represented a relatively small scale of use. At the scale of use, we had opted for this, again, slightly more simplified architecture to avoid dealing with the more complex coordination required to make the timer service fully scalable. Specifically, when we talk about scaling issues, we will be focusing more on the scheduler portion.
Scaling the timer service vertically was no problem at all. We could and did add resources to both the gRPC service portion and the scheduler as needed. Scaling the gRPC service portion horizontally was also no trouble. We could easily add a pod or four to handle an increase in create, get, and delete requests from multiple clients. The slight hitch that we were now facing was that the scheduler was not quite so simple to scale horizontally. We’d not yet done the work to allow for multiple schedulers to run at the same time. See, each scheduler needs to ask the gRPC service for timers at some set interval. It does no one any good if each individual scheduler is asking for timers and getting all the same ones back. Then we’re just duplicating all the work, instead of sharing the load. Plus, it certainly doesn’t seem like a desirable feature to enqueue each message to Kafka multiple times as we see here. We needed to do a bit of a redesign to allow for multiple schedulers to share the task of scheduling and firing timers with each in charge of only a particular subset of the overall timers. How do we do that? If we wanted multiple schedulers to run in conjunction, we needed to find a way to group timers by more than just time, so that those schedulers could be responsible, each one, for requesting and retrieving only a particular group of timers.
First, we needed to make some adjustments to how our data was stored so the timers weren’t stored just by the time that they were to be sent out, those 5-minute bucket intervals, but by some other grouping that different schedulers could retrieve from. We made some adjustments to our Scylla table schemas so that when a new timer is created, it is inserted into a bucket by both that time interval, and now a shard. While we were adjusting these schemas, we also actually decided to shrink that bucket interval from the 5 minutes we’d been using to 1-minute bucket intervals. This was because we were already noticing that our Scylla partitions were getting larger than we would like. We would like to keep our Scylla partitions in general relatively small, as this leads to more efficient and less resource intensive compaction. We determined which shard a timer belongs to by encoding its unique UUID to an integer within the range of the number of shards we have. We actually have our shards set to 1024, with timers pretty evenly distributed among them. Each scheduler instance is then responsible for an evenly distributed portion of those shards. We went to this granularity and this many shards, also in an effort to keep those Scylla partitions relatively small. This means we have a more efficient way to parse a far greater number of timers. This update also makes the bookkeeping of the buckets table much more efficient, and means that when querying for timers, we look much more particularly at a particular shard and time to retrieve from. We then just needed to adjust our get timers request so that when we do request those timers, we do it not just by time, as we were before, but in addition by that shard, so that we can get a particular subset of the overall timers.
We now have a system whereby timers are stored by both time and shard, and a way by which to retrieve those timers by both time and shard. We’re there? Just one big problem. Each scheduler instance needs to have state so that it can reliably ask for the same subset of timers every time it asks. How does each scheduler know who it is? This was important because if a scheduler instance were to restart for any reason, it needed to start back up as the same scheduler so it could pull the same timers. If we have multiple instances pulling the same subset of timers, we’re back at square one. If scheduler 2 were to restart for any reason, now thinking that it’s scheduler 1, when we already have a scheduler 1 up and running and pulling timers for scheduler 1, we’re again duplicating work and erroneously firing timers multiple times. Plus, even worse here, no one is looking after the shards assigned to scheduler 2.
In order to solve this, we deployed a new version of the scheduler as a stateful set in Kubernetes, which, among other things, gave us a stable unique name for each instance of the scheduler every time it started up, with each name ending in a zero-indexed value up to the number of replicas. Each scheduler could then take that value and calculate a range of shards that it’s responsible for retrieving timers from. Importantly, this means that each shard of timers and therefore each individual timer will only ever be retrieved by one scheduler instance. We now have this system where we store timers by both time and shard, where we have retrieval requests that can get a subset of timers by both time and shard. Now, schedulers that have state and can therefore reliably request the same subset of timers every time they ask. We have achieved full scalability.
Performance Characteristics
With these changes made to the architecture of the timer service, adding new nodes to the scheduler is as simple as increasing the replica count in the config file, which makes scaling the timer service both vertically and horizontally, possible and simple to do as we continue to use it in more parts of our system. Because we now have multiple instances of both the gRPC service portion and the scheduler, we’ve made the timer service much less susceptible to serious outage if a node were to go down. Previously, we only had one scheduler, so if it went down, it was it. There were no timers being retrieved, or processed, or fired, and no messages being enqueued to Kafka by the timer service until that node were to restart. Now, because we have multiple instances, each in charge of only a particular subset of the overall timers, if a node goes down, it has much less impact on the overall functioning of the system. On single pod performance, each scheduler node is capable of handling about 10,000 timers per second. Frankly, that’s without even pushing it, which makes horizontally scaling incredibly powerful. Each gRPC instance handles about 17,000 requests per second really without trouble.
Callouts
There are a few things to note about our timer service as it exists today. First, the timer service has an at-least-once guarantee. This is because there’s a space between the scheduler enqueuing its message to Kafka, and then turning around and requesting that that timer be deleted. If the scheduler were to restart for any reason between those two actions, or if the gRPC service has some communication error between the scheduler or between Scylla when processing that delete, the timer will fail to be deleted and will again be retrieved and fired. Because of this, the timer service does expect that all downstream consumers manage their own idempotence guarantees. Another callout about the timer service is that timers will fire close to but not exactly at the time they’re scheduled. This is because schedulers still need to pull in timers at that periodic interval via that get timers request. We currently have each scheduler set to pull in the next 10 minutes of timers every 1 minute. Possibly the biggest callout about the timer service as it exists today, is that once a timer has been retrieved by the scheduler, there’s no method by which to cancel it. This is because the only way to delete or cancel a timer in this system the way we have it currently, is via that delete timer request on the gRPC service, which deletes from the database. Therein, if the timer has already been retrieved from the database and is now in the scheduler, there’s no way to currently stop it from firing.
Future Potential
The timer service has proven to be incredibly powerful within our own internal systems. As such, we really believe it could be useful to other organizations and individuals. There’s definite potential that we will move to open source it in the near future. As we continue to grow as an organization, we intend to use the timer service as we create new services, but want to dedicate resources to integrating it more broadly across our existing infrastructure in order to streamline. We also would like to add and fine-tune features such as perhaps the ability to cancel the timer at any point in the lifecycle.
What We Have
We’re about four years on now, from that original conception of a timer service to enable those journey builders. We now have an incredibly robust system that’s capable of storing billions of concurrent timers and expiring them in a performant manner, while minimizing data loss, easily integrating with the rest of our systems, and, importantly, scaling simply both vertically and horizontally to accommodate our future use.
Questions and Answers
Participant: How did you handle when you added the new nodes to the scheduler? If you have 4 running in each kit, 250 charge, you add a fifth one, now they’re going to be 200? How did you keep them from stepping on each other’s toes as you increased that number?
Mara: We would take all of the nodes down at the time that a restart occurred, and start them back up. There would maybe be 30 seconds of potential timer latency. We weren’t super concerned about millisecond accuracy of the schedulers in this case.
Participant: One of the interesting places for scheduling [inaudible 00:41:14].
Mara: I was the manager on the team, at the time we were initially building out this project. The engineer that was in charge of the implementation, he was running all these complicated data structures past me, and I suggested, did you try the most naive possible approach? Did you try spawn a future and wait on a task? He was like, that can’t possibly perform well enough for our needs. I said, why don’t you try it and we’ll see if it performs well enough? It did. We actually didn’t really continue to evaluate more complicated data structures because the easiest one worked for us.
Participant: How do you handle the [inaudible 00:42:38] that replicated the time zones, or anything related here. Did you sidestep or how did you handle that?
Mara: That was totally sidestepped. These timers were all in UTC. These were all server-side events. Every timer that’s in this system, is a timer that was scheduled by another team at OneSignal. If somebody cared about something being time zone sensitive, they cared about, you send a notification at 8 a.m. in the user’s time zone, they would have to figure out when 8 a.m. on the user’s time zone was on a UTC clock.
Participant: You mentioned that you have a secondary table that you store all the tickets, and you request all the tickets [inaudible 00:43:34] for next interval you want to fetch. That’s essentially like a full table scan?
Mara: That is basically a full table scan. The thing that’s saving us from really having a bad time on that is the fact that the number of entries on that table is much more constrained than the number of entries on the timers table. There’s going to be a maximum of one entry on that table for every 5-minute interval. I don’t think the timer system actually has a limit on how far out you can schedule timers. The systems that currently enqueue timers I believe do have limits on how long they will allow you to schedule out a timer. Like journeys will not allow you to, say, send a notification then wait for 30 days. That just puts a constraint on the number of things that are allowed to live in the dataset. Yes, it’s potentially very expensive.
Participant: I have a few questions about the database structure itself. It spawns data in the case of Cassandra? It must be more performant than the Scylla database. At the backend, how many nodes do you have, and what the replication factor is? [inaudible 00:45:06]
Mara: We have just a single data center, I believe four timers. We have I think six nodes with Scylla. I’m not sure if we did any of our own benchmarking of Scylla versus Cassandra. I think at the time, our CTO had quite a strong aversion to Java. I believe we actually run zero Java code in production, and adopting Scylla versus Cassandra was partially motivated by that desire. We have been quite happy with Scylla since we’ve adopted it.
Participant: [inaudible 00:46:06]
Mara: A timer would be returned by the gRPC service multiple times until it was expired, until that delete timer method was called for that particular timer. That, I suppose, is another inefficiency of the system. There’s a bunch of data retransmission. Again, this was done in the name of the simplicity of the system. That is something that probably could be removed in the future if we wanted to optimize that a bit more.
Participant: It’s basically that there is a possibility here of some crashing bug in a particular [inaudible 00:47:20], if one of your scheduled nodes stopped and crashed, do you have a mechanism of recovering that, or that is not a problem?
Mara: That hasn’t been a problem for us, I think because the API of a timer is so small. The variance in each timer is time and data and Kafka settings basically. We don’t give you more Kafka settings than topic and partition. The attack surface is really small. We haven’t had any instances of a malformed timer that was causing issues for the service. If a particular node of the timer system was just crash looping, we would basically just have to page an engineer and have them look into it. There’s not auto-healing built into it.
See more presentations with transcripts
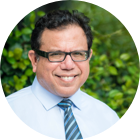
MMS • RSS
Posted on mongodb google news. Visit mongodb google news
Strs Ohio reduced its stake in shares of MongoDB, Inc. (NASDAQ:MDB – Free Report) by 11.1% in the 4th quarter, according to the company in its most recent 13F filing with the Securities and Exchange Commission (SEC). The fund owned 1,610 shares of the company’s stock after selling 202 shares during the quarter. Strs Ohio’s holdings in MongoDB were worth $658,000 at the end of the most recent quarter.
Several other large investors have also recently bought and sold shares of the stock. Vontobel Holding Ltd. increased its holdings in MongoDB by 9.3% in the 4th quarter. Vontobel Holding Ltd. now owns 4,615 shares of the company’s stock valued at $1,887,000 after buying an additional 391 shares during the period. Sumitomo Mitsui Trust Holdings Inc. increased its holdings in MongoDB by 2.6% in the 4th quarter. Sumitomo Mitsui Trust Holdings Inc. now owns 178,693 shares of the company’s stock valued at $73,059,000 after buying an additional 4,600 shares during the period. Louisiana State Employees Retirement System bought a new stake in MongoDB in the 4th quarter valued at $2,330,000. Simplicity Solutions LLC increased its holdings in MongoDB by 10.0% in the 4th quarter. Simplicity Solutions LLC now owns 1,070 shares of the company’s stock valued at $437,000 after buying an additional 97 shares during the period. Finally, Perigon Wealth Management LLC increased its holdings in MongoDB by 1.8% in the 4th quarter. Perigon Wealth Management LLC now owns 2,848 shares of the company’s stock valued at $1,164,000 after buying an additional 51 shares during the period. 89.29% of the stock is currently owned by institutional investors and hedge funds.
Insider Buying and Selling at MongoDB
In related news, CFO Michael Lawrence Gordon sold 10,000 shares of the stock in a transaction that occurred on Thursday, February 8th. The shares were sold at an average price of $469.84, for a total value of $4,698,400.00. Following the sale, the chief financial officer now directly owns 70,985 shares in the company, valued at approximately $33,351,592.40. The sale was disclosed in a filing with the Securities & Exchange Commission, which is accessible through this hyperlink. In related news, CFO Michael Lawrence Gordon sold 10,000 shares of MongoDB stock in a transaction that occurred on Thursday, February 8th. The shares were sold at an average price of $469.84, for a total transaction of $4,698,400.00. Following the transaction, the chief financial officer now owns 70,985 shares of the company’s stock, valued at approximately $33,351,592.40. The transaction was disclosed in a legal filing with the Securities & Exchange Commission, which can be accessed through this hyperlink. Also, Director Dwight A. Merriman sold 2,000 shares of MongoDB stock in a transaction that occurred on Monday, April 8th. The stock was sold at an average price of $365.00, for a total transaction of $730,000.00. Following the transaction, the director now directly owns 1,154,784 shares in the company, valued at approximately $421,496,160. The disclosure for this sale can be found here. In the last three months, insiders have sold 91,802 shares of company stock worth $35,936,911. Company insiders own 4.80% of the company’s stock.
Analysts Set New Price Targets
MDB has been the topic of several analyst reports. Guggenheim upped their target price on shares of MongoDB from $250.00 to $272.00 and gave the stock a “sell” rating in a report on Monday, March 4th. JMP Securities reaffirmed a “market outperform” rating and issued a $440.00 target price on shares of MongoDB in a report on Monday, January 22nd. Redburn Atlantic reaffirmed a “sell” rating and issued a $295.00 target price (down previously from $410.00) on shares of MongoDB in a report on Tuesday, March 19th. Needham & Company LLC reaffirmed a “buy” rating and issued a $465.00 target price on shares of MongoDB in a report on Thursday. Finally, Loop Capital initiated coverage on shares of MongoDB in a report on Tuesday. They issued a “buy” rating and a $415.00 target price on the stock. Two analysts have rated the stock with a sell rating, three have given a hold rating and twenty have issued a buy rating to the company. Based on data from MarketBeat.com, the stock currently has an average rating of “Moderate Buy” and an average target price of $443.86.
Get Our Latest Analysis on MongoDB
MongoDB Stock Performance
MongoDB stock opened at $366.13 on Friday. The company has a current ratio of 4.40, a quick ratio of 4.40 and a debt-to-equity ratio of 1.07. MongoDB, Inc. has a 1 year low of $215.56 and a 1 year high of $509.62. The company has a market cap of $26.67 billion, a PE ratio of -147.63 and a beta of 1.19. The company’s 50-day moving average is $381.23 and its two-hundred day moving average is $390.50.
MongoDB (NASDAQ:MDB – Get Free Report) last released its earnings results on Thursday, March 7th. The company reported ($1.03) earnings per share (EPS) for the quarter, missing analysts’ consensus estimates of ($0.71) by ($0.32). MongoDB had a negative net margin of 10.49% and a negative return on equity of 16.22%. The business had revenue of $458.00 million for the quarter, compared to analyst estimates of $431.99 million. Equities analysts anticipate that MongoDB, Inc. will post -2.53 earnings per share for the current year.
MongoDB Profile
MongoDB, Inc, together with its subsidiaries, provides general purpose database platform worldwide. The company provides MongoDB Atlas, a hosted multi-cloud database-as-a-service solution; MongoDB Enterprise Advanced, a commercial database server for enterprise customers to run in the cloud, on-premises, or in a hybrid environment; and Community Server, a free-to-download version of its database, which includes the functionality that developers need to get started with MongoDB.
Further Reading
Want to see what other hedge funds are holding MDB? Visit HoldingsChannel.com to get the latest 13F filings and insider trades for MongoDB, Inc. (NASDAQ:MDB – Free Report).
Receive News & Ratings for MongoDB Daily – Enter your email address below to receive a concise daily summary of the latest news and analysts’ ratings for MongoDB and related companies with MarketBeat.com’s FREE daily email newsletter.
Article originally posted on mongodb google news. Visit mongodb google news
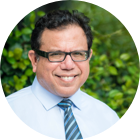
MMS • Kimberly Hendrick
Article originally posted on InfoQ. Visit InfoQ
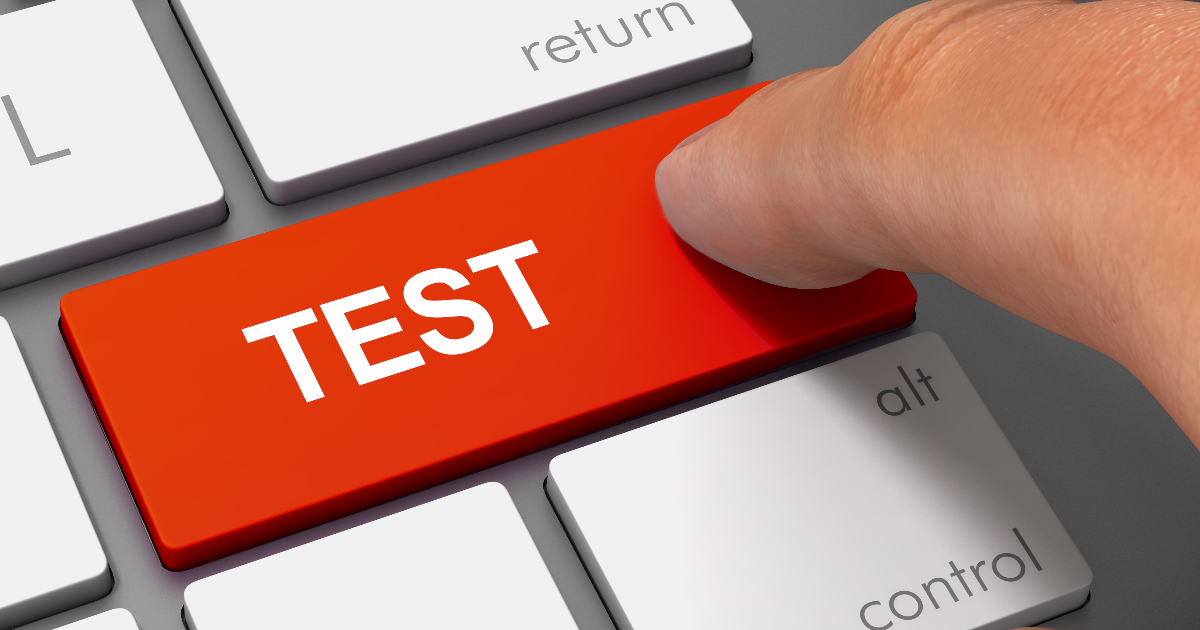
Key Takeaways
- Don’t repeat yourself, or “DRY”, is a useful principle to apply to both application code and test code.
- The misapplication of the DRY technique can make tests hard to understand, maintain, and change.
- While code duplication may not be so harmful to your tests, allowing duplication of concepts causes the same maintainability problems in test code as in application code.
- When applying DRY to tests, clearly distinguish between the three steps of a test: arrange, act, and assert.
- TDD provides many benefits and can promote a shorter feedback loop and better test coverage.
Those of us who write automated tests do so for many reasons and gain several benefits. We gain increased trust in the correctness of the code, confidence that allows us to refactor, and faster feedback from our tests on the design of the application code.
I’m a huge proponent of TDD (Test Driven Development) and believe TDD provides all the benefits stated above, along with an even shorter feedback loop and better test coverage.
One crucial design principle in software development is DRY – Don’t Repeat Yourself. However, as we will see, when DRY is applied to test code, it can cause the test suite to become brittle – difficult to understand, maintain, and change. When the tests cause us maintenance headaches, we may question whether they are worth the time and effort we put into them.
Can this happen because our test suite is “too DRY”? How can we avoid this problem and still benefit from writing tests? In this article, I’ll delve into this topic. I will present some indications that a test suite is brittle, guidelines to follow when reducing test duplication, and better ways to DRY up tests.
Note: I won’t discuss the definitions of different types of tests in this article. Instead, it focuses on tests where duplication is common.
These are often considered unit tests but may also occur in tests that don’t fit a strict definition of a “unit test.” For another viewpoint on types of tests, read A Simpler Testing Pyramid: Getting the Most out of Your Tests.
What is DRY?
DRY is an acronym for “Don’t Repeat Yourself,” coined by Andy Hunt and Dave Thomas in The Pragmatic Programmer. They defined it as the principle that “every piece of knowledge must have a single, unambiguous, authoritative representation within a system.”
The advantage of DRY code is that if a concept changes in the application, it requires a change in only one place. This makes a codebase easier to read and maintain and reduces the chances of bugs. Beautiful, clean designs can emerge when domain concepts are represented in a single place in the application.
DRY Application Code
DRY is not always easy to apply. Indeed, duplication in code that looks similar can tempt us to create unnecessary abstractions, leading to more complicated code instead of a cleaner design. One useful criterion to consider is that DRY is concerned with reducing code duplication from concept duplication and not reducing duplication of typing. This idea may guide its application while avoiding common pitfalls.
For example, we often use literal values in our code. Is the number 60
that appears in several locations an instance of duplication, or does it have different meanings in each case? A helpful evaluation can be to ask: “If the value had to change, would we want it to change everywhere?” 60
will (hopefully) always be the number of seconds in a minute, but 60
somewhere else may represent a speed limit. This integer is not a great candidate to pull into a globally shared variable for the sake of DRY.
As another example, imagine a method that loops over a collection and performs an action. This method might look a lot like another method that loops over the same collection and performs a slightly different action. Should these two methods be extracted to remove the duplication? Perhaps, but not necessarily. One way of looking at it is if a feature change would require them both to change simultaneously, they are most likely closely related and should be combined. But it takes more than looking at the code “shape” to know if it should be DRYed up.
Reasoning in terms of duplication of concepts helps avoid wrong decisions.
DRY Tests
DRY in test code often presents a similar dilemma. While excessive duplication can make tests lengthy and difficult to maintain, misapplying DRY can lead to brittle test suites. Does this suggest that the test code warrants more duplication than the application code?
DRY vs. DAMP/WET
A common solution to brittle tests is to use the DAMP acronym to describe how tests should be written. DAMP stands for “Descriptive and Meaningful Phrases” or “Don’t Abstract Methods Prematurely.” Another acronym (we love a good acronym!) is WET: “Write Everything Twice,” “Write Every Time,” “We Enjoy Typing,” or “Waste Everyone’s Time.”
The literal definition of DAMP has good intention – descriptive, meaningful phrases and knowing the right time to extract methods are essential when writing software. However, in a more general sense, DAMP and WET are opposites of DRY. The idea can be summarized as follows: Prefer more duplication in tests than you would in application code.
However, the same concerns of readability and maintainability exist in application code as in test code. Duplication of concepts causes the same problems of maintainability in test code as in application code.
Brittle Example
Let’s review some brittle test code written in Kotlin.
The below example shows a common pattern that may present differently depending on the testing language and framework. For example, in RSpec, the long setUp()
method may be many let!
statements instead.
class FilterTest {
private lateinit var filter: Filter
private lateinit var book1: Book
private lateinit var book2: Book
private lateinit var book3: Book
private lateinit var book4: Book
private lateinit var author: Author
private lateinit var item1: Item
private lateinit var item2: Item
@BeforeEach
fun setUp() {
book1 = createBook("Test title", "Test subtitle",
"2000-01-01", "2012-02-01")
book2 = createBook("Not found", "Not found",
"2000-01-15", "2012-03-01")
book3 = createBook("title 2", "Subtitle 2", null,
"archived", "mst")
createBookLanguage("EN", book1)
createBookLanguage("EN", book3)
author = createAuthor()
book4 = createBook("Another title 2", "Subtitle 2",
null, "processed", "", "",
listOf("b", "c"), author)
val user = createUser()
createProduct(user, null, book4)
val salesTeam = createSalesTeam()
createProduct(null, salesTeam, book4)
val price1 = createPrice(book1)
val price2 = createPrice(book3)
item1 = createItem("item")
createPriceTag(item1, price1)
item2 = createItem("item2")
createPriceTag(item2, price2)
val mstDiscount = createDiscount("mstdiscount")
val specialDiscount = createDiscount("special")
createBookDiscount(mstDiscount, book1)
createBookDiscount(specialDiscount, book2)
createBookDiscount(mstDiscount, book2)
}
@Test
fun `filter by title`() {
filter = Filter(searchTerm = "title")
onlyFindsBooks(filter, book1, book3, book4)
}
@Test
fun `filter by last`() {
filter = Filter(searchTerm = "title", last = "5 days")
onlyFindsBooks(filter, book3)
}
@Test
fun `filter by released from and released to`() {
filter = Filter(releasedFrom = "2000-01-10",
releasedTo = "2000-01-20")
onlyFindsBooks(filter, book2)
}
@Test
fun `filter by released from without released to`() {
filter = Filter(releasedFrom = "2000-01-02")
onlyFindsBooks(filter, book2, book3, book4)
}
@Test
fun `filter by released to without released from`() {
filter = Filter(releasedTo = "2000-01-01")
onlyFindsBooks(filter, book1)
}
@Test
fun `filter by language`() {
filter = Filter(language = "EN")
onlyFindsBooks(filter, book1, book3)
}
@Test
fun `filter by author ids`() {
filter = Filter(authorUuids = author.uuid)
onlyFindsBooks(filter, book4)
}
@Test
fun `filter by state`() {
filter = Filter(state = "archived")
onlyFindsBooks(filter, book3)
}
@Test
fun `filter by multiple item_uuids`() {
filter = Filter(itemUuids = listOf(item1.uuid, item2.uuid))
onlyFindsBooks(filter, book1, book3)
}
@Test
fun `filtering by discounts with substring`() {
filter = Filter(anyDiscount = listOf("discount"))
assertTrue(filter.results().isEmpty())
}
@Test
fun `filtering by discounts with single discount string`() {
filter = Filter(anyDiscount = listOf("special"))
onlyFindsBooks(filter, book2)
}
@Test
fun `filtering by discounts with non-existent discount`() {
filter = Filter(anyDiscount = listOf("foobar"))
assertTrue(filter.results().isEmpty())
}
@Test
fun `filtering by discounts with multiple of the same discount`() {
filter = Filter(anyDiscount =
listOf("mstdiscount", "mstdiscount", "special"))
onlyFindsBooks(filter, book1, book2)
}
private fun onlyFindsBooks(filter: Filter, vararg foundBooks: Book) {
val uuids = foundBooks.map { it.uuid }.toSet()
assertEquals(uuids, filter.results().map { it.uuid }.toSet())
}
}
When studying code like this, it’s common to first focus on the setup steps, then digest each test and figure out how they relate to the setup (or vice versa). Looking at only the setup in isolation provides no clarity, nor does focusing on each test individually. This is an indication of a brittle test suite. Ideally, each test can be read as its own little universe with all context defined locally.
In the above example, the setup()
method creates all the books and related data for all the tests. As a result, it is unclear which books are required for which tests. In addition, the numerous details make it challenging to discern which ones are relevant and which are required for book creation in general. Notice how many things would break if the required data for creating books were to change.
When focusing on the tests themselves, each test does the minimum to call the application code and assert the results. The specific book instance(s) referenced in the assertion is buried in the setUp()
method at the top. It’s unclear what purpose onlyFindsBooks
serves in the tests. You might be tempted to add a comment on these tests to remind you of the relevance of each book’s attributes in each test.
It was clear that the initial developers had good intentions creating the objects all in one place. If the initial feature only had two or three filters available, creating all the objects at the top might have made the code more concise. As the tests and objects grew, however, they outgrew this setup method. Subsequent filter features led developers to add more fields to the books and expect whichever book suited the test to return. Imagine trying to figure out which object was meant to be returned as we began to compose different combinations of the filters together!
To figure out what onlyFindsBooks()
does, you’ll need to scroll more to find the hidden assertions. This method has enough logic that it takes a minute to connect the dots between what is passed in from the test and what the assertion is.
Finally, the filter instance declaration is far from the tests.
For example, let’s focus on this test for filtering by language:
@Test
fun `filter by language`() {
filter = Filter(language = "EN")
onlyFindsBooks(filter, book1, book3)
}
What makes book1
and book3
match the criteria of language = "EN"
that was passed in? Why wouldn’t book2
also come back from this call? To answer those questions, you need to scroll to the setup, load the entire context of all the setup into your mind, and then attempt to spot the similarities and differences between all the books.
Even more challenging is this test:
@Test
fun `filter by last`() {
filter = Filter(searchTerm = "title", last = "5 days")
onlyFindsBooks(filter, book3)
}
Where does “5 days
” come from? Is it related to a value hidden in the createBook()
method for book3
?
The author of this code applied the DRY technique to extract duplication but ended up with a test suite that is hard to understand and will break easily.
What to Look For
Many clues in the above code indicate that DRY has been misapplied. Some indications that tests are brittle and need refactoring include:
- Tests are not their own little universe (see Mystery Guest): Do you find yourself scrolling up and down to understand each test?
- Relevant details are not highlighted: Are there comments in tests to clarify relevant test details?
- The intention of the test is unclear: Is there any boilerplate or “noise” required for setup but not directly related to the test?
- Duplicate concepts are duplicated: Does changing application code break many tests?
- Tests are not independent: Do many tests break when modifying one?
Solutions
In this section, we will present two possible solutions to the problems described above: the Three As principle and the use of object methods.
Three As
Tests may be seen as having three high-level parts. Often, these are referred to as the “Three As“:
- Arrange – any necessary setup, including the variable the test is focused on
- Act – the call to the application code (aka SUT, Subject Under Test)
- Assert – the verification step that includes the expectation or assertion.
These steps are also referred to as Given, When, and Then.
The ideal test has only three lines, one for each of the As. This may not be feasible in reality, but it’s still a worthwhile objective to keep in mind. In fact, tests that match this pattern are easier to read:
// Arrange
var object = createObject()
// Act
var result = sut.findObject()
// Assert
assertEquals(object, result)
Object Creation Methods
Strategic use of object creation methods can highlight relevant details and hide irrelevant (but necessary) boilerplate behind meaningful domain names. This strategy is inspired by two others: Builder Pattern and Object Mother. While the example code we reviewed earlier uses methods to build test objects, it lacks some key benefits.
Object creation methods should:
- Be named with a domain name that indicates which type of object it creates
- Have defaults for all required values
- Allow overrides for any values used directly by tests
Let’s change one of the tests from our example code to follow the Three As and use object creation methods:
@Test
fun `filter by language`() {
var englishBook = createBook()
createBookLanguage("EN", englishBook)
var germanBook = createBook()
createBookLanguage("DE", germanBook)
var results = Filter(language = "EN").results()
val expectedUuids = listOf(englishBook).map { it.uuid }
val actualUuids = results.map { it.uuid }
assertEquals(expectedUuids, actualUuids)
}
The changes made here are:
- We modified the
createBook()
method to hide the boilerplate and allow overriding of the relevant details of the language value (thecreateBook()
definition is not shown). - We renamed book variables to indicate their relevant differences.
- We inlined the
filter
variable to make the Act step visible. This also allows it to be a constant instead of a variable, thus decreasing mutability. - We inlined the
onlyFindsBooks()
method and renamed temporary variables. This allows the separation of the Act step from the Assert step and clarifies the assertion.
Now, the three steps are much easier to identify. We can easily see why we are creating two books and their differences. It is clear that the Act step is looking only for "EN"
and that we expect only the book’s English version to be returned.
At four lines of code, the Arrange step is longer than ideal. Even though it is four lines long, they are all relevant to this test, and it’s easy to see why. We could combine creating a book and associating the language into a single method. This makes the test code more complex and tightly couples the creation of books with languages in our test code, so it may cause more confusion than clarity. If, however, “book written in language” is a concept that exists in the domain, this might be the right call.
The logic in the Assert step could be better. That’s enough logic and noise to make it hard to understand if it were to fail.
Let’s extract those two areas and see how it looks:
@Test
fun `filter by language`() {
val englishBook = createBookWrittenIn("EN")
val germanBook = createBookWrittenIn("DE")
val results = Filter(language = "EN").results()
assertBooksEqual(listOf(englishBook), results)
}
private fun createBookWrittenIn(language: String): Book {
val book = createBook()
createBookLanguage(language, book)
return book
}
private fun assertBooksEqual(expected: List, actual: List) {
val expectedUuids = expected.map { it.uuid }
val actualUuids = actual.map { it.uuid }
assertEquals(expectedUuids, actualUuids)
}
This test requires nothing in the setUp()
method, making it easy to understand without scrolling. You can dive into the details of the helper methods (createBookWrittenIn
and assertBooksEqual
), but the test is readable even without doing so.
As we apply these changes throughout the rest of the test suite, we’ll be forced to consider which books with which attributes are required for each test. The relevant details will stand out as we continue.
We may look at all the tests together and feel uncomfortable that we’re creating so many books! But we’re ok with that duplication because we know that while it looks like a duplication of code, it is not a duplication of concepts. Each test creates books representing different ideas, e.g., a book written in English vs a book released on a certain date.
Benefits
Our setup method will be empty, and each test will be readable in isolation. Changing our application code (e.g., the book constructor) will only require changing the method in one place. Changing the setup or expectation of a single test will not cause all the tests to fail. The extracted helper methods have meaningful names that fit into the Three As pattern.
Guidelines
Here is a summary of the key guidelines that we followed, as well as additional guidelines:
- Each test matches the Three As pattern: Arrange, Act, Assert. The three-part pattern (setup, action, expectations) should be easily distinguishable when looking at the test.
Arrange
- Setup code does not include assertions.
- Each test clearly indicates relevant differences from other tests.
- Setup methods do not include any relevant differences (they are instead local to each test).
- Boilerplate “noise” is extracted and easy to reuse.
- Tests are run and fail independently. Tests are each their own tiny universe with all the context they need.
- Avoid randomness that causes tests to be non-deterministic. Test failures should be deterministic to avoid flaky tests that fail intermittently.
Act
- The SUT (Subject Under Test) and the main thing being tested (target behavior) are easy to identify.
Assert
- Favor literals (hardcoded) values in assertions instead of variables. An exception is when well-named variables provide additional clarity.
- Tests don’t have complicated logic or loops. Loops create interdependent tests. Complicated logic is brittle and hard to understand.
- Assertions don’t repeat the implementation code.
- Consider fewer assertions per test. Breaking up a test with a large set of assertions into multiple tests with fewer assertions provides more feedback on the failures. Multiple assertions may indicate too many responsibilities in the application code.
- Prefer assertions that provide more information when they fail. For example, one assertion that the result matches an array provides more information than multiple assertions that count the items in the array and then verify each item individually. Tests stop on the first failure, so feedback from subsequent assertions is lost.
A Note about Design
Sometimes, it is difficult to follow the above guidelines because the tests are trying to tell you something about the application design. Some test smells that provide feedback to the application code design include:
If this:
- Too much setup could indicate a large surface area being tested; too much is being tested.
- Wanting to extract a variable (thus coupling tests) because a literal is being tested repeatedly may indicate the application has too many responsibilities.
Then:
- Consider that the application code has too many responsibilities and apply the Single Responsibility principle.
If this:
- Comments are necessary to make the test understandable
Then:
- Rename a variable, method, or test name to be more meaningful
- Consider application code refactoring to provide more meaningful names or split up responsibilities
Additionally, don’t be afraid to wait until removing duplication feels “right.” Prefer duplication until it’s clearer what the tests are telling you. If an extraction or refactor goes wrong, it may be best to inline code and try again.
A Note about Performance
One more reason developers are driven to extract code duplication is performance concerns. Certainly, slow tests are a cause for concern, but often, the worry of creating duplicate objects is overinflated, certainly when compared to the time spent maintaining brittle tests. Respond to the pain caused by a lot of test setup by redesigning the application code. This results in both better design and lightweight tests.
If you do encounter performance problems with tests, begin by investigating the reasons for the slowness. Consider whether the tests are telling you something about the architecture. You may find a performance solution that doesn’t compromise the test clarity.
Conclusion
DRY is a valuable principle to apply to both application code and test code. When applying DRY to tests, though, clearly distinguish between the three steps of a test: Arrange, Act, and Assert. This will help highlight the differences between each test and keep the boilerplate from making tests noisy. If your tests feel brittle (often break with application code changes) or hard to read, don’t be afraid to inline them and re-extract along more meaningful domain seams.
It is important to remember that good design principles apply to application and test code. Test code requires the same ease of maintenance and readability as application code, and while code duplication may not be so harmful to your tests, allowing duplication of concepts causes the same problems of maintainability in test code as in application code. Hence, the same level of care should be given to the test code.
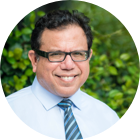
MMS • Jenna Zeigen
Article originally posted on InfoQ. Visit InfoQ
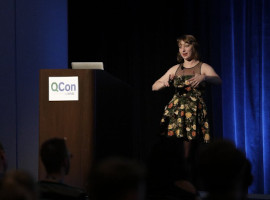
Transcript
Zeigen: My name is Jenna Zeigen. This talk is, several components are rendering. I am a staff software engineer at Slack, on our client performance infrastructure team. The team is only about 2 years old at this point. I was one of the founding members. I’ve been working on performance at Slack full time for a little bit longer than that. Before I was on the client performance infrastructure team, I was on Slack search team, where I worked a lot on the desktop autocomplete experience that you may know and love. It was on that team where I really cut my teeth doing render performance and JavaScript runtime performance. Since that feature does more work than you would expect an autocomplete to do on the frontend, and it has to do it in a very short amount of time. I had a lot of fun doing that and decided that performance was the thing for me.
Performance
What is performance? In short, we want to make the app go fast, reduce latency, have buttery smooth interactions, get rid of jank, no dropped frames. There’s all sorts of goals for performance work. As I like to say, my number one performance rule about how to make performance happen is to do less work. It all comes down to no matter what your strategy, you’re trying to do less work. Then the why, which is really why I wanted to have this slide. It seemed like there needed to be more words on it. The why is so that our users have a great experience. It’s really easy to get bogged down when you’re doing performance work in the numbers. You want that number of milliseconds to be a smaller number of milliseconds. You want the graph to go in the right direction. It’s important to keep in mind that we are doing performance work, because we want our users to have a good experience. In Slack’s case, we want channel switches to be fast. We want typing and input to feel smooth. We don’t want there to be input delay. It should feel instantaneous. Keep that in mind as we go through this talk and try to stay rooted in that idea of why we are doing all of this and why I’m talking about this.
Slack (React App on Desktop)
First, some stuff about Slack. Slack, at its core is a channel-based collaboration tool. There’s a lot of text. There’s also a lot of images, and files, and now video conferencing. The Slack desktop app isn’t native, it’s an Electron app, which means it’s a web application like you would have in a browser being rendered by a special Chromium instance via the Electron framework. This means the Slack desktop app is the same application that you would use in Chrome, or Firefox, or Safari, or your browser of choice. It’s using good-old HTML, CSS, and JavaScript, just like in a browser. This means that we’re also subject to the same performance constraints and challenges as we face when we are coding frontends for browsers.
How Do Browsers Even? (JavaScript is Single Threaded)
Now I’m going to talk a little bit about browsers. What’s going on inside of a browser? One of the jobs of a browser is to convert the code that we send it over the wire into pixels on a page. It does this by creating some trees and turning those trees into different trees. It’s going to take the HTML and it’s going to turn it into the DOM tree, the document object model. It’s also going to do something similar to the CSS. Then, by their powers combined, you get the render tree. Then the browser is going to take the render tree and go through three more phases. First, layout phase. We still need to figure out where all of the elements are going to go on the page and how big they’re supposed to be. Then we need to paint those things. The painting phase, which is representing them as pixels on the screen, and this is going to be a series of layers, which then get sent to the GPU for compositing or smooshing all the layers together. The browser will try its best to do this 60 times per second, provided there’s something that has changed that it needs to animate. We’re trying for 60 frames per second or 16.66 milliseconds, and 16 milliseconds is a magic number in frontend performance. Try and keep that number in mind as we go through these slides.
These 60 frames per second only happens in the most perfect of conditions, for you see, renders are constrained by the speed of your JavaScript. JavaScript is single threaded, running on the browser’s main thread along with all of the repainting, layout, compositing that has to happen. Everything that gets called in JavaScript in the browser is going to get thrown onto the stack. Synchronous calls are going to go right on, and async callbacks like event handlers, input handlers, click handlers, are going to get thrown into a callback queue. Then they get moved to that stack by the event loop once all the synchronous JavaScript is done happening. There’s also that render queue that’s trying to get stuff done, 60 frames per second, but renders can’t happen if there’s anything still on the JavaScript callback. To put it differently, the browser won’t complete a repaint if there’s any JavaScript still left to be called in the stack.
That means that if your JavaScript takes longer than 16 milliseconds to run, you could potentially end up with dropped frames, or laggy inputs if the browser has something to animate, or if you’re trying to type into an input.
Performance, a UX Perspective
Performance is about user experience. Let’s take it back to that. Google’s done a lot of research as they do on browsers and user experience. They’ve come up with a model of user experience called the RAIL model. This work was also informed by Jakob Nielsen and some of his research on how users perceive delay. According to the RAIL model, you want to, R, respond to users’ actions within 100 milliseconds, or your users are going to start feeling the lag. This means that practically, you need to produce actions within 50 milliseconds to give time for other work. The browser has a lot of stuff to do. It’s a busy girl. You got to give it some breathing room on either side to get your JavaScript done and get all the work done that you ask it to do. On the animation frame side, the A in RAIL is for animation, you need to produce that animation frame in 16 milliseconds, that magic 16 milliseconds, or you could end up dropping frames and blocking the loop and animations could start to feel choppy. This practically means that you need to get all that setup done in 10 milliseconds, since the browsers need about 6 milliseconds to actually render the frame.
Frontend Performance
I would be remiss if I didn’t take this slight detour. A few years ago, I was reading this book called, “The Every Computer Performance Book.” It said that, in my experience, the application is rarely re-rendered, unless the inefficiency is egregious, and the fix is easy and obvious. The alternative presented in this book was to move the code to a faster machine, or split the code over several machines. We’re talking about the client here. We’re talking about people’s laptops. We don’t have that luxury. That’s just simply a nonstarter for frontend. Unlike on the backend, we don’t have control over the computers that our code is running on. We can’t mail our users’ laptops that are up to spec and whatever. People can have anything from most souped up M2, all the way down to a 2-core machine with who knows what other software is running on that computer, competing for resources, especially if it’s a corporate owned laptop. We still got to get our software to perform well, no matter what. That’s part of the thrill of frontend performance.
React and Redux 101
I mentioned earlier that Slack is a React app, so some details about React. React is a popular, well maintained, and easy to use component-based UI framework. It promotes modularity by letting engineers write their markup and JavaScript side by side. It’s used across a wide variety of applications from toy apps, through enterprise software like Slack, since it allows you to iterate and scale quickly. Its popularity also means that it’s well documented and there’s solid developer tooling and a lot of libraries that we can bring in if we need to. Six years ago, when Slack was going through a huge rewrite and rearchitecture, it was like the thing to choose. Some details about React that are going to come in handy to know, components get data as props, or they can store data in component state. As you see here, the avatar component gets person and size as props. You can see in the second code block there, it’s receiving Taylor Swift and 1989 as some props. There’s not an example here of storing component state, but that’s also another way that it can deal with its data. Then, crucial detail, like core bit about React is that changes to props are going to cause components to re-render. When a component says, ok, one of my props is different, it’s going to then re-render so it can redisplay the updated data to you the user.
In a large application, like Slack, this fragmented type of storing data in a component itself, or even just purely passing data down via props, could get unwieldy. A central datastore is quite appealing. We decided to use a state management library called Redux, that’s a popular companion to React and is used to supplement component state. Instead, there’s a central store that components can connect to. Then data is read from Redux via selectors, which aid in computing connected props. A component can connect to Redux. You see that useSelector example there on the code block. We passed it the prop of ID and the component is using that ID prop to then say, Redux, give me that person by ID. That is a connected prop making avatar now a connected component.
Let’s explain this with a diagram. You have Redux in the middle, it’s the central datastore. Then there are a whole bunch of connected components that are reminiscent of Slack. Actions are going to get dispatched to Redux which causes reducers to run, which causes a Redux state to get updated. Dispatches are the result of interacting with the app or receiving information over the wire like an API over the WebSocket. Actions will continue to get dispatched, which, again, updates Redux. Then, when Redux changes, it sends out a notification to all the components that subscribe to it. I like to call this the Redux bat signal. Redux will send out its bat signal to all of the components that are connected to it. Then, everything that’s connected to Redux, every single component is going to then rerun all of its connections. All of the connected components are going to recalculate, see if any of them have updated. This is a caveat, it will only do this if it has verified that state has changed. That’s at least one thing. It will only do this if state has actually changed. Then, central tenant of React, components with change props will re-render. Again, if a component thinks that its data is now different, it will re-render. Here’s a different view, actions cause reducers to run, which then updates the store. The store then sends out the subscriber notification to the component, which then re-render. Then actions can then be sent from components or over the wire via API handlers. This process, this loop, this Redux loop that I like to call it, is going to happen every single time there is a dispatch, every single time Redux gets updated, that whole thing happens.
You might start to see how this could go wrong and start to cause performance issues. After that, we are seeing that Redux loops are just taking way too long to happen. Unsurprisingly, what we’re seeing just like, at rest, like you don’t even have to be doing anything. You could have your hands off the keyboard, and just like maybe the app is receiving notifications and stuff over the WebSocket or via API, just hands off the keyboard, even at p50, we are seeing that the Redux loop is taking 25 milliseconds, which is more than 16. We know that we’re already potentially dropping at least one frame, at least 50% of the time, that’s what p50 means. Then at p99, so 1% of the time, we are taking more than 200 milliseconds. We’re taking, in fact, 210 milliseconds to do all of this work, which means that we’ve blown through, we’ve doubled that threshold in which humans are going to be able to tell that something is going wrong. We’re going to start to drop frames. If you try to type into the input, they’re going to be feeling it.
What did we do? Like any good performance team, we profiled. The classic story here is you profile, you find the worst offending function, the thing that’s taking up the most amount of time. You rinse, repeat until your app is faster. In this case, what we had here was a classic death by a thousand cuts. You might say, there’s those little yellow ones, and that purple one. The yellow ones are garbage collections. The purple one is, we did something that caused the browser to be a little bit mad at us, we’ll just say that it’s a recalculation of styles. Otherwise, it’s just this pile of papercuts. We had to take some novel approaches to figuring out how to take the bulk out of this. Because there wasn’t anything in particular that was taking a long time, it was just a lot of stuff.
How can we just, again, make less work happen during every loop? We took a step back and figured out where performance was breaking down. Ultimately, it comes down to three main categories of things that echo the stages of the loop. One, every change to Redux results in a Redux subscriber notification firing. That’s the core problem with Redux. Then we spend way too long running selectors. There’s a lot of components on the page, they all have a lot of connections. Too much work is happening on every loop, just checking to see if we need to re-render. Then, three, we are spending too long re-rendering components that decide that they need to re-render, often unnecessarily. The first thing is, too many dispatches. For large enterprises, we can be dispatching hundreds of times per second. If you’re in a chatty channel, maybe with a bot in it that talks a lot to a channel, you can be receiving a lot every second. This Redux out of the box, it’s just going to keep saying, dispatch, update all the components, dispatch, update all the components. That just means a lot of updates. Every API call, WebSocket event, any clicks, switching the channel, subscriber notification, switching channels, sending messages, receiving messages, reactjis, everything in Slack: Redux, Redux, Redux. Then this leads to again, every connection runs every time Redux notifies. Practically, we ran some ad hoc logging, that we would never put in production. Practically, it’s 5,000 to 25,000 connected props in every loop. This is just how Redux works. This is why scaling it is starting to get to us. Even in 5,000, if every check takes 0.1 milliseconds, that’s a long task. We’ve blown through that 50 milliseconds. The 50 milliseconds is a long task. At that point, once you get to 50 milliseconds, like their browser performance APIs, if you hook into them, they’re like, maybe you should start making that a shorter task. Yes, just again, way too much work happening.
Then all of this extra work is leading to even more extra work. Because, as I said, we’re having unnecessary re-renders, which is a common problem in React land, but just have a lot of them. This happens because components are receiving or calculating props that fail equality checks, but they are deep-equal or unstable. This can happen, for example, if you calculate a prop via map, filter, reduce, what you get from a selector right out of the Redux store isn’t exactly what you need. You want to filter out everyone who isn’t the current member from this list of members today. If you run a map, as you might know, that returns a new array. Every single time you do it, that is a new array that is going to fail reference equality checks. That means the component thinks something is different and it needs to re-render. Bad for performance. There’s all differing varieties of this type of issue happening. Basically, components think that data is different when it actually isn’t.
Improving Performance by Doing Less Work
How are we making this better? Actually, doing less work. There are two attacks that we’ve been taking here. First, we’re going to target some problem components. There are components that we know are contributing to this pile of papercuts more than others, then, also, we know that these mistakes are happening everywhere, so we also need to take a more broad-spectrum approach to some of these things. First, targeting problem components. There’s one in particular that I’m going to talk about. What do you think is the most performance offending component in Slack? It is on the screen right now. It’s not messages. It’s not the search bar. It is the channel sidebar. We had a hunch about this. If you look at it, it doesn’t look that complicated, but we had a hunch that it might be a naughty component. Through some natural experiments, we discovered that neutralizing the sidebars or removing it from being rendered alleviated the performance problems with people who were having particularly bad performance problems. Kind of a surprise. Naively, at face value, sidebar looks like a simple list of channels. It looks like some icons, and maybe some section headings and some channel name, and then maybe another icon on the right. It was taking 115 milliseconds. This was me, I put my sidebar into bat mode, which was showing all of the conversations. Usually, I have it on unreads only performance tip, have your sidebar in unreads only. To make it bad, I made my sidebar, sidebar a lot longer. We found that there’s a lot of React and Redux work happening. This was a bit of a surprise to me. I knew the sidebar was bad, but I thought it was going to be the calculating what to render that was going to stick out and not the, we’re doing all this React and Redux work. Calculating what to render is taking 15 milliseconds, which is almost 16 milliseconds. Either way, this is not fun for anyone. There was definitely some extra work happening in that first green section, the React and Redux stuff.
Again, lots of selectors. We found through that same ad hoc logging that folks who had 20,000 selector calls, when we got rid of their sidebar, that dropped to 2,000. That is quite a 90% increase in improvement. That made us realize there’s some opportunities there. This is mainly because inefficiencies in lists compound quickly. There are 40 connected prop calculations in every sidebar item component, so the canonical like channel name with the icon, and that doesn’t even count all of the child components of that connected channel.
Forty times, if you have 400 things in your sidebar, that’s 16,000. A lot of work to try and dig into. We found that a lot of those selector calls were unnecessary. Surprise, isn’t it like revolutionary that we were doing work that we didn’t need to do? One of my specifically pet peeve, which is why it’s on this slide, is instead of checking experiments, so like someone had a feature flag on or didn’t. Instead of doing that once at the list level, we’re doing it in every single connected channel component, and maybe that was a reasonable thing for them to do. Maybe the experiment had something to do with showing the tool tip on a channel under some certain condition or something. We didn’t need to be checking experiment on every single one.
Then, also, there were some cases where we were calculating some data that was irrelevant to that type of channel. For instance, if you have the pref on to like show if someone’s typing in a DM in your sidebar, we only do that for DMs, it has nothing to do with channels. Yet, we would go and grab that pref, you would see like, does a person have that pref on? Even though it was a public channel, we were never going to use that data. We were just going to drop it on the floor. Surprise, we moved repeated work that we could up a level, we call it once instead of 400 times, and created more specialized components. Then we found some props that were unused. Then, all of this fairly banal work. I’m not standing up here and saying we did some amazing revolutionary stuff. It ended up creating a 30% improvement in sidebar performance, which I thought was pretty rad. We didn’t even touch that 15-millisecond bar on the right. Then, ok, but how did that impact overall Redux subscriber notification time? It made a sizable impact, over 10% across the board over the month that we were doing it. That was pretty neat to see that just focusing on a particular component that we knew was bad was going to have such a noticeable impact. People, even anecdotally, were saying that the performance was feeling better.
What’s Next: List Virtualization
We’re not done. We want to try revirtualizing the sidebar, this technique, which is to only render what’s going to be on the screen with a little bit of buffering to try and allow for smooth scrolling, actually had a tradeoff for scroll speed and performance. There’s some issues that you see if you scroll too quickly. We just were like, virtualization isn’t worth it, we want to focus on scroll speed. When actually now we’re seeing that maybe we took the wrong side of the tradeoff, so we want to try turning on list virtualization. List virtualization will be good for React and Redux performance, because fewer components being rendered means fewer connected props being calculated on every Redux loop, because there’s less components on the page trying to figure out if they need to re-render.
What’s Next: State Shapes and Storage
Another thing that we want to try that really targets that 15-millisecond section that we didn’t really touch with this work is to figure out if we can store our data closer to the shape that we needed for the sidebar. We store data like it’s the backend, which is reasonable. You get a list of channels over the wire, and like, I’ll just put it into Redux in that way. Then I will munge it however I need it for the use case that I in particular have. Engineers also tend to be afraid of storage. I think this is a reasonable fear that people have that like, “No, memory.” If I store this thing with 100 entries in it, we might start to feel it in the memory footprint, when in fact, it’s not the side of the tradeoff that you actually need at that point. We have this question of how can we store data, so it serves our UI better, so we cannot be spending so much time deriving the data that we need on every Redux loop? For example, why are we recalculating what should be shown in every channel section on every loop? Also, we store every single channel that you come across. This might be the fault of autocomplete. I’m not blaming myself or anything. We say, give me all the channels that match this particular query, and then you get a pile of channels back over the wire, and they’re not all channels that you’re in, and we store them anyway. Then to make your channel sidebar, we have to iterate through all of the channels that you have in Redux, while really the only ones we’re ever going to need for your channel sidebar is the ones that you’re actually in. Little fun tidbits like that.
Solutions: Batched Updates, Codemods, and Using Redux Less
As I said, just focusing on problem components isn’t going to solve the whole problem. We have a scaling problem with Redux, and we need to figure out what to do with that. It’s diffused, so it’s everywhere. It’s across components that are at the sidebar. We need some broader spectrum solutions. The first one that seems really intuitive, and in fact, they’re putting this in by default in React 18, is to batch updates. Out of the box, Redux every single time a dispatch happens and a reducer runs is then going to send out that bat signal to all of the components. Instead, we added a little bit of code to batch the updates. We’ve wrapped this call to this half secret React DOM API that flushes the updates.
We wrap this in a request animation frame call, which is our way of saying, browser, right before you’re about to do all that work to figure out where all the pixels have to go on the screen, run this code. It’s like a browser aware way of debalancing the subscriber notification, essentially. I like to joke that this is called the batch signal. Another thing that I think is pretty cool that we’re doing is codemods for performance. Performance problems can be detected and fixed at the AST level. We found that we can find when there are static unstable props. If someone is just creating a static object that they then pass as a prop to a child component, you can replace that out so it’s not getting remade on every single loop. Similarly, we could rewrite prop calculation for the selectors in a way that facilitates memoization. Another example is replacing empty values to an empty array. An empty array does not reference equal empty array, or empty object for that matter. We can replace it with constants that will always have reference equality.
We’re also trying to use Redux less. You might be wondering when this was going to come up. We are investigating using IndexedDB to store more evicted items that we can evict from the cache. Less data in Redux means fewer loops as a result of keeping the items in the cache fresh. Every time something gets stale, we need to get its update over the wire, which causes a dispatch, which causes the subscriber notification. Also, cache eviction is fun, but we could also not store stuff in Redux that we’re never going to use again. Finer-grained subscription would be cool, but it’s harder than it sounds. It would be great if we could say, this component only cares about data in the channel store. Let’s get it to subscribe only to the channel store. With Redux, it’s all or nothing.
Why React and Redux, Still?
Why are we still using Redux? This is a question that we’ve been asking a lot over the past year. We’ve started to tinker with proofs of concepts and found like, yes, this toy thing that we made with this thing that does finer-grained subscription, or has a totally different model of subscription than Redux, it’s a lot faster. Scale is our problem to begin with. Why are we still sticking with React and Redux at this point? React is popular, well maintained, and easy to use. It has thus far served us pretty well, with slather ever-growing team of 200 frontend engineers at this point, build features with confidence and velocity, we chose a system that kind of fits our engineering culture. It’s people friendly. It allows for people on the flip side to remain agnostic of the internals of the framework, which for the most part works for your average, everyday feature work. This is breaking down at scale as we push the limits. These problems are systemic and architectural. We’re faced with this question, we either change the architecture, or we put in the time to fix it with mitigation and education at the place where we have it now. We put in all these efforts into choosing a new framework, or we write our own thing that solves the problems that we have. This too would be a multiyear project for the team to undertake. We’d have to stop everything we’re doing and just switch to another thing if we really wanted to commit to this. There is no silver bullet out there. Changing everything would be a huge lift with 200 frontend engineers and counting. We want our associate engineers to be able to understand things. We want them to be able to find documentation and help themselves. Redux prefers consistency and approachability over performance. That’s the bottom line. Every other architecture makes different tradeoffs that we can’t be sure about, and how anything else would break down at scale either, once we start to see the emergent properties of that architecture that we chose.
Fighting a Problem of Scale at Scale
Where are we now? People love performance. No engineers are like, I don’t want my app to be fast. They’ll want the things that they write to, to work fast. We’re engineers, I think it’s in our blood, essentially. Let’s set our engineers up for success by giving them tools and helping them learn more about performance. I’ve really been taking the attack of creating a performance culture through education, tooling, and evangelism. React and Redux abstract away the internals, so you don’t really need to know about that whole loop. Some of you probably know more about how React and Redux work now than some frontend engineers. I believe that understanding the system contextualizes and motivates performance work, which is why I spent a lot of time in my talk explaining those things to you. The first thing that we can do to make people aware of the issues that are happening is to show them when they’re writing code that could be a performance liability. We’ve done this via Lint rules, remember with the codemods. You can find this stuff via AST and via static analysis. We bring this to their VS Code, with some Lint rules that show them when unstable properties are being passed to children, when unstable properties are being computed. When they’re passing functions or values that are breaking memoization, but they don’t have to. Not everything can be done via static analysis, though. We’ve created some warnings that go into people’s development consoles. While you might not be able to tell from the code itself when props are going to be unstable, we know what the values are at runtime, and we can compare the previous to the current value and be like, those were deep-equal, you might want to think about stabilizing them. We can also tell based on what’s in Redux when an experiment, for example, is finished, and we don’t need to be checking it on every single Redux loop. That’s just one less papercut in the pile.
Once they know what problems are in their code, we’ve been telling them about how to fix these things. These tips and tricks are fairly lightweight. None of these things is a particularly challenging thing to understand. Wrap your component in React memo if it’s re-rendering too much. Maybe use that EMPTY_ARRAY constant, instead of creating a new EMPTY_ARRAY on every single loop. Same with these other examples here. We’ve taken those Lint rules and those console warnings, and we’ve beaconed them to the backend, so now we have some burndown charts. While making people aware of the issues in the first step, sometimes you also need a good burndown chart. I’m not going to say that having your graph isn’t motivational. That’s why we all love graphs in performance land. Sometimes, yes, you do need a good chart that is going down to help light a fire under people’s tuchus. Say the graph is going in the right direction, it’s working. I like to joke that performance work is like fighting entropy, more stuff is going to keep popping up, it’s like Whack a Mole. For the most part, we’re heading in the right direction in the Lint rules and in the console warnings. That’s been really great to see.
I found in my career that performance has been built up as this problem for experts. There’s this hero culture that surrounds it. You go into the performance channel and you say, “I shaved 5 milliseconds off of this API call, look at me, look at how great I am.” This isn’t necessarily a good thing. It’s great to celebrate your wins. It’s great that you’re improving performance and reducing latency. We’re doing ourselves a disservice if we keep performance inaccessible, and that we keep this air around it that like the only way that we solve performance issues is by these huge architectural feats. We have to change the whole architecture if we want to fix performance problems. Because, again, engineers, your teammates care about performance, and they want to help and they want to pitch in. Let’s get a lot of people to fix our performance problems. We had a problem before this problem that came out of the scale of our app, we had all of these papercuts. If we get 200 engineers to fix 200 performance problems, that is 200 fewer papercuts in the pile.
Conclusion
As I was putting this story down on the slides, this has been rattling around in my head, it takes a lot of work to do less work. We could have taken the other side of the story and put in the work to rearchitect the whole application, and that would be a lot of work for my team. It would be a lot of work for the other engineers to readapt and change their way of working and change the code to use a “more performant framework.” Or we could trust our coworkers and teach them, and they’re good engineers. They work at your company for a reason. Let’s help them understand the systems that they are working on. Then they might start to see how the systems break down and then they could start to fix the system when it breaks down.
See more presentations with transcripts
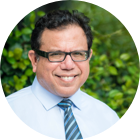
MMS • Chris Swan
Article originally posted on InfoQ. Visit InfoQ

When Microsoft Engineer Andres Freund noticed SSH was taking longer than usual he discovered a backdoor in xz utils, one of the underlying libraries for systemd, that had taken years to be put in place. The United States Cybersecurity & Infrastructure Security Agency (CISA) has assigned CVE-2024-3094 to the issue. The backdoor had found its way into testing releases of Linux distributions like Debian Sid, Fedora 41 and Fedora Rawhide but was caught before propagating into more highly used stable releases. Though there’s evidence that the attackers were pressuring distro maintainers to speed up its deployment.
Evan Boehs provides a detailed timeline and analysis of the attack in ‘Everything I know about the XZ backdoor’, which runs back to 2021 when a GitHub account JiaT75 was created for ‘Jia Tan’. Initial activity from that account was on the libarchive code but in April 2022 ‘Jia Tan’ moved on to XZ creating a patch, and another persona ‘Jigar Kumar’ started pressuring the project maintainer Lasse Collin. Over time, ‘Jia Tan’ took over a substantial part of the ongoing maintenance of XZ and used their position to insert the backdoor using a sophisticated attack against the build process where the code was hidden inside of tests. Earlier efforts at making the code and build process safer and more secure were also undermined, with ‘improved security’ routinely used as the false reason for the changes. Security expert Bruce Schneier links to Thomas Roccia’s infographic in describing it as ‘a masterful piece of work’, and goes on to say:
It affects the SSH remote login protocol, basically by adding a hidden piece of functionality that requires a specific key to enable. Someone with that key can use the backdoored SSH to upload and execute an arbitrary piece of code on the target machine. SSH runs as root, so that code could have done anything. Let your imagination run wild.
This isn’t something a hacker just whips up. This backdoor is the result of a years-long engineering effort. The ways the code evades detection in source form, how it lies dormant and undetectable until activated, and its immense power and flexibility give credence to the widely held assumption that a major nation-state is behind this.
Lasse Collin has provided his own account of events in an XZ Utils backdoor page. It’s somewhat evocative of XKCD 2347 ‘Dependency’ where the stability and security of an entire ecosystem is propped up by a lone maintainer. It’s also a painful illustration of why ‘bus factor’ is an important measure of the health of a dependency, which is why it’s included in measures like the Open Source Security Foundation (OpenSSF) Best Practices. Whoever the attackers are, they took time to identify the weakest link in the software supply chain and exploit the human frailties associated with that.
As the backdoor only got as far as test systems it’s mostly being treated as a ‘near miss’ incident that the industry can learn from. The OpenJS Foundation have published an alert in partnership with OpenSSF ‘Social Engineering Takeovers of Open Source Projects’ where they identify similar attempts to subvert JavaScript projects. Industry veteran Tim Bray looks to the future in proposing “Open Source Quality Institutes” (OSQI) as a means to provide funding and governance for critical open source projects.
Software supply chain security has become a hot topic in recent years, and this attack only serves to highlight why it’s so important. If the back door code hadn’t revealed itself to a diligent engineer by being just a bit too slow, then over the course of months and years it would have left many systems open to the attackers. Though it’s not the only ‘pre auth’ failure to crop up recently, with similar issues impacting Palo Alto (CVE-2024-3400) and Ivanti (CVE-2024-21887).
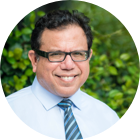
MMS • RSS
Posted on mongodb google news. Visit mongodb google news
MongoDB (NASDAQ:MDB – Get Free Report)‘s stock had its “buy” rating reaffirmed by analysts at Needham & Company LLC in a research report issued on Thursday, Benzinga reports. They currently have a $465.00 target price on the stock. Needham & Company LLC’s price objective suggests a potential upside of 26.88% from the company’s current price.
Other analysts have also recently issued reports about the stock. Citigroup upped their price target on shares of MongoDB from $515.00 to $550.00 and gave the company a “buy” rating in a report on Wednesday, March 6th. Tigress Financial increased their target price on shares of MongoDB from $495.00 to $500.00 and gave the stock a “buy” rating in a report on Thursday, March 28th. Redburn Atlantic reissued a “sell” rating and set a $295.00 target price (down from $410.00) on shares of MongoDB in a research note on Tuesday, March 19th. Guggenheim upped their price objective on shares of MongoDB from $250.00 to $272.00 and gave the stock a “sell” rating in a report on Monday, March 4th. Finally, JMP Securities reaffirmed a “market outperform” rating and issued a $440.00 price objective on shares of MongoDB in a report on Monday, January 22nd. Two investment analysts have rated the stock with a sell rating, three have given a hold rating and twenty have assigned a buy rating to the company. According to MarketBeat.com, MongoDB presently has an average rating of “Moderate Buy” and a consensus target price of $443.86.
Check Out Our Latest Research Report on MongoDB
MongoDB Trading Down 0.8 %
MDB traded down $2.79 during trading on Thursday, hitting $366.50. 620,753 shares of the company’s stock were exchanged, compared to its average volume of 1,374,472. The company has a current ratio of 4.40, a quick ratio of 4.40 and a debt-to-equity ratio of 1.07. MongoDB has a 1 year low of $215.56 and a 1 year high of $509.62. The stock has a 50 day moving average price of $383.39 and a 200 day moving average price of $390.67. The firm has a market cap of $26.69 billion, a P/E ratio of -148.91 and a beta of 1.19.
MongoDB (NASDAQ:MDB – Get Free Report) last released its quarterly earnings data on Thursday, March 7th. The company reported ($1.03) earnings per share (EPS) for the quarter, missing the consensus estimate of ($0.71) by ($0.32). MongoDB had a negative return on equity of 16.22% and a negative net margin of 10.49%. The business had revenue of $458.00 million for the quarter, compared to analysts’ expectations of $431.99 million. As a group, analysts expect that MongoDB will post -2.53 EPS for the current fiscal year.
Insider Buying and Selling at MongoDB
In other news, CAO Thomas Bull sold 170 shares of the business’s stock in a transaction on Tuesday, April 2nd. The stock was sold at an average price of $348.12, for a total transaction of $59,180.40. Following the completion of the transaction, the chief accounting officer now owns 17,360 shares of the company’s stock, valued at approximately $6,043,363.20. The transaction was disclosed in a legal filing with the Securities & Exchange Commission, which is available through this link. In other news, CEO Dev Ittycheria sold 33,000 shares of the business’s stock in a transaction on Thursday, February 1st. The stock was sold at an average price of $405.77, for a total transaction of $13,390,410.00. Following the completion of the transaction, the chief executive officer now owns 198,166 shares of the company’s stock, valued at approximately $80,409,817.82. The transaction was disclosed in a legal filing with the Securities & Exchange Commission, which is available through this link. Also, CAO Thomas Bull sold 170 shares of the business’s stock in a transaction on Tuesday, April 2nd. The stock was sold at an average price of $348.12, for a total value of $59,180.40. Following the sale, the chief accounting officer now owns 17,360 shares in the company, valued at approximately $6,043,363.20. The disclosure for this sale can be found here. Insiders sold 91,802 shares of company stock valued at $35,936,911 in the last three months. Corporate insiders own 4.80% of the company’s stock.
Institutional Investors Weigh In On MongoDB
Several institutional investors and hedge funds have recently made changes to their positions in the business. Jennison Associates LLC increased its position in shares of MongoDB by 87.8% during the third quarter. Jennison Associates LLC now owns 3,733,964 shares of the company’s stock valued at $1,291,429,000 after acquiring an additional 1,745,231 shares during the last quarter. Norges Bank bought a new position in shares of MongoDB in the fourth quarter worth $326,237,000. Axiom Investors LLC DE bought a new stake in MongoDB during the fourth quarter valued at $153,990,000. Clearbridge Investments LLC boosted its stake in MongoDB by 10,827.8% during the fourth quarter. Clearbridge Investments LLC now owns 212,983 shares of the company’s stock valued at $87,078,000 after buying an additional 211,034 shares during the period. Finally, First Trust Advisors LP raised its position in MongoDB by 59.3% during the fourth quarter. First Trust Advisors LP now owns 549,052 shares of the company’s stock valued at $224,480,000 after purchasing an additional 204,284 shares in the last quarter. Institutional investors own 89.29% of the company’s stock.
MongoDB Company Profile
MongoDB, Inc, together with its subsidiaries, provides general purpose database platform worldwide. The company provides MongoDB Atlas, a hosted multi-cloud database-as-a-service solution; MongoDB Enterprise Advanced, a commercial database server for enterprise customers to run in the cloud, on-premises, or in a hybrid environment; and Community Server, a free-to-download version of its database, which includes the functionality that developers need to get started with MongoDB.
Featured Stories
Before you consider MongoDB, you’ll want to hear this.
MarketBeat keeps track of Wall Street’s top-rated and best performing research analysts and the stocks they recommend to their clients on a daily basis. MarketBeat has identified the five stocks that top analysts are quietly whispering to their clients to buy now before the broader market catches on… and MongoDB wasn’t on the list.
While MongoDB currently has a “Moderate Buy” rating among analysts, top-rated analysts believe these five stocks are better buys.
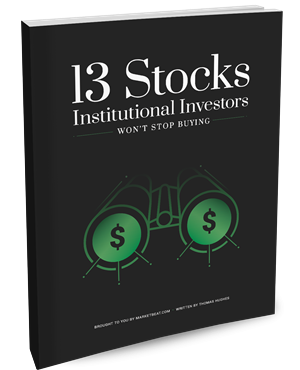
Which stocks are major institutional investors including hedge funds and endowments buying in today’s market? Click the link below and we’ll send you MarketBeat’s list of thirteen stocks that institutional investors are buying up as quickly as they can.
Article originally posted on mongodb google news. Visit mongodb google news