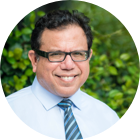
MMS • RSS
Article originally posted on Data Science Central. Visit Data Science Central
Objective
Congestive heart failure (CHF) has been called an “epidemic” and a “staggering clinical and public health problem” (Roger, 2013). It can be defined as the impaired ability of the ventricle to fill or eject with blood. Consequences include difficulty breathing, coughing fits, leg swelling, decreased quality of life, and ultimately death. As life expectancy increases globally, we can only expect to see this syndrome more frequently. Fortunately, the advents of the electronic medical record and machine learning techniques have given us new weapons with which to fight this disease. In this post, let’s discuss three ways to combat CHF using artificial intelligence and related technologies:
- Early prediction and prevention of CHF onset
- Assistance with diagnosing CHF
- Prediction and prevention of CHF exacerbations
Early prediction and prevention of CHF onset
Once CHF is diagnosed, only 10% of patients live past 10 years (Roger, 2013). Therefore, there is much need for detecting patients who are at high risk of developing CHF before its onset and preventing those patients from developing CHF by promoting exercise, a low-salt diet, treatment with medications such as ACE inhibitors and beta-blockers, and increased engagement with physicians.
Several studies have predicted onset of CHF before clinical diagnosis. A 2010 study from the Geisinger clinic in Pennsylvania made models to predict individuals at risk of being diagnosed with CHF within 6 months or longer, using clinical data drawn from electronic health records (Wu et al., 2010). The researchers found that logistic regression and boosting algorithms achieved an AUC of 0.77 for the prediction task.
More recently, a 2016 study out of the Georgia Institute of Technology trained recurrent neural networks to predict the risk of developing CHF within 6 months (Choi et al., 2016). They used clinical event sequences collected over a 12-18 month observation window for over 30,000 patients. They achieved AUCs of 0.77 and 0.88 when using the 12-month and 18-month observation windows, respectively.
Assistance with diagnosing CHF
A definitive diagnosis of CHF is expensive to perform. Echocardiography requires skilled personnel to administer the test, and then a specialist physician (usually a cardiologist or radiologist) must read the study and assess how well the heart is pumping by visually quantifying the ejection fraction (EF), which is the fraction of blood the left ventricle ejects during its contraction. It can be unreliable to determine heart function using the images produced by sound waves, as you can imagine from the echocardiogram image below.
A cardiac MRI, while more expensive, is more accurate in measuring EF and is considered the gold standard for CHF diagnosis; however, it requires a cardiologist to spend up to 20 minutes reading an individual scan.
One approach to improve CHF detection is to sidestep the use of expensive and time-consuming imaging studies. A recent study from Keimyung University in South Korea used rough sets, decision trees, and logistic regression algorithms, and compared their performance to a physician’s diagnosis of heart disease (Son et al., 2012). The algorithms were trained on demographic characteristics and lab findings only. The models achieved over 97% sensitivity and 97% specificity in differentiating CHF from non-CHF-related shortness of breath. That’s astonishingly close to the human performance, with much less data, time, and resources used.
A second approach we should mention is the use of automated algorithms to read the echocardiography and cardiac MRI scans used to diagnose CHF. This problem was the subject of the 2015 Data Science Bowl, sponsored by the data science competition website Kaggle and Booz Allen Hamilton, the consulting company. For more on this competition, you can visit the competition website.
Prediction and prevention of CHF exacerbations
The Internet-of-Things (IoT) is a network of physical devices embedded with sensors, software, and internet connectivity, enabling these devices to exchange data. One emerging application of the IoT is to be able to remotely monitor patient health. A group of researchers at UCLA developed a weight, activity, and blood pressure patient-monitoring system for CHF called WANDA (Suh et al., 2011). Increased weight due to fluid retention, decreased activity, and uncontrolled blood pressure are critical markers of CHF decompensation; WANDA uses weight scales and blood pressure monitors to communicate these patient markers to the patient’s phone via Bluetooth. The phone then sends this data to a backend database in the cloud, along with activity and symptom information about the patient collected from a phone-based app. The data can then be used to perform logistic regression to alert a physician in case the patient is at risk of CHF decompensation. All of this is done privately and securely. This is a prime example of how the IoT and machine learning can be used to combat CHF.
Conclusion
Artificial intelligence can also be used for the following CHF-related applications (Tripoliti et al., 2017):
- Prediction and prevention of myocardial infarction, a major complication of CHF
- Prediction and prevention of hospital readmissions due to CHF
- Prediction and prevention of death resulting from CHF
In conclusion, congestive heart failure (CHF) is one of the leading causes of mortality in the developed world. To fight it effectively, we must use all the tools at our disposal. Artificial intelligence and related techniques provide additional weapons in our arsenal as we fight this catastrophic disease.
References
Choi E, Schuetz A, Stewart WF and Sun J (2017). Using recurrent neural network models for early detection of heart failure onset. JAMIA 24(2): 361-37.
File:Echocardiogram 4chambers.jpg. (2015, November 22). Wikimedia Commons, the free media repository. Retrieved 18:50, August 25, 2018 from https://commons.wikimedia.org/w/index.php?title=File:Echocardiogram_4chambers.jpg&oldid=179925202.
Roger VL (2013). Epidemiology of Heart Failure. Circulation research 113(6): 646-659.
Son C, Kim Y, Kim H, Park H, Kim M (2012). Decision-making model for early diagnosis of congestive heart failure using rough set and decision tree approaches. Journal of Biomedical Informatics 45: 999-1008.
Suh M, Chen C, Woodbridge J, Tu MK, Kim JI, Nahapetian A, Evangelista LS, Sarrafzadeh M (2011). A Remote Patient Monitoring System for Congestive Heart Failure. J Med Syst 35(5): 1165-1179.
Tripoliti EE, Papadopoulos TG, Karanasiou GS, Naka KK, Fotiadis DI (2017). Heart Failure: Diagnosis, Severity Estimation and Prediction of Adverse Events Through Machine Learning Techniques. Computational and Structural Biotechnology Journal 15: 26-47.
Wu J, Roy J, Stewart WF (2010). Prediction modeling using EHR data: challenges, strategies, and a comparison of machine learning approaches. Med Care 48: S106–S113.