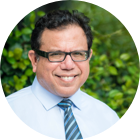
MMS • Anthony Alford Roland Meertens
Article originally posted on InfoQ. Visit InfoQ
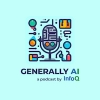
Transcript
Roland Meertens: Okay, so for the fun fact today, I know that the city in Colombo, in Sri Lanka, they created a model of their city using the video game City Skylines. And this is meant as a digital twin. Their citizens can basically use it to understand the impact of decisions such as changing roads, adding more green space. So they created all kinds of digital assets to make the simulation look more realistic.
They changed some parameters of the game. And the funniest change is that they modified the behavior of the traffic to reflect Sri Lankan driving habits, which includes buses may ignore lane arrows, vehicles may enter blocks junctions, vehicles may do U-turns and junctions, 10% of drivers are reckless, vehicles may park on sides of streets, and three wheelers and scooters are introduced.
Anthony Alford: And they drive on the wrong side of the road, no doubt.
Roland Meertens: I mean, 10% of drivers are reckless, I thought was the funniest change they made.
Anthony Alford: That’s optimistic, I imagine.
Roland Meertens: If it’s only 10%.
Anthony Alford: Hate to see those numbers for my hometown.
Roland Meertens: Welcome to Generally AI. This is season two, episode five, and we are going to talk about simulation. It is an InfoQ podcast, and I am Roland Meertens here with Anthony Alford.
Anthony Alford: Hello, Roland.
Roland Meertens: Shall I get started with my simulation topic for today?
Anthony Alford: Please begin.
Sampled Musical Instruments [01:50]
Roland Meertens: All right. So earlier this season, we already talked about sampling music, right?
Anthony Alford: Yes.
Roland Meertens: However, I wanted to take this a step further and talk about how you can simulate playing a real instrument using a virtual instrument.
Anthony Alford: Like Guitar Hero.
Roland Meertens: Oh man. I love Guitar Hero.
Anthony Alford: Or Rock Band or one of those games.
Roland Meertens: Did you ever play that by the way?
Anthony Alford: I tried it once and I was terrible.
Roland Meertens: I was really good at Guitar Hero, and then I started playing more real guitar and then I became terrible at Guitar Hero.
Anthony Alford: Not to interrupt your train of thought, but you’ve probably seen the video of the members of the band Rush playing one of those video games and they’re playing a Rush song.
Roland Meertens: I didn’t see that.
Anthony Alford: They’re so bad that in the video game, the crowd boos them off the stage.
Roland Meertens: Yes, I know that feeling.
Anyway, the bigger problem I have is that not everybody has space in their house for an actual instrument, or alternatively, your neighbors might not like it, like my neighbors. So instead of buying a real piano, you can buy an electric piano or you can buy MIDI keyboards. And the interesting thing is that some of the cheaper models, if you buy a super cheap keyboard, it’ll probably, instead of playing a note, it will just play like a sine wave, call it a day. But of course, real pianists want the expressiveness of a real piano.
Anthony Alford: Yes.
Roland Meertens: So option one to simulate the sound of a real piano is to record the sound of a real piano and then play that. So you can buy all kinds of kits online, like all kinds of plugins for your digital piano. They record it hitting keys on one specific piano in all kinds of different ways with all kinds of different microphones. So I’m going to let you hear an example of that.
How did you like this?
Anthony Alford: That’s very nice. It’s very convincing.
Roland Meertens: Yes. So this is the virtual piano, but then with recordings from a real piano.
Anthony Alford: Okay.
Roland Meertens: If you want this, it’ll cost you $250 for the pack alone.
Anthony Alford: So is it software? Do you use a MIDI controller, and then does this software run somewhere?
Roland Meertens: Yes, so I think this is a plugin. You can use it as a plugin, so you import it into your audio workstation, and then you play with your MIDI controller. So the MIDI controller will tell the computer what key you’re pressing with what velocity, et cetera, et cetera.
Anthony Alford: Very cool.
Roland Meertens: And I know that professional musicians, they all have their favorite virtual recorded piano they like to use, which they think sounds very good. So that’s interesting. I’ve never bought something like this.
Anthony Alford: Well, I don’t know if this is the right time in the podcast to interject it, but I’m sure you’re familiar with the Mellotron.
Roland Meertens: That’s pure sound waves, right?
Anthony Alford: Well, it’s the same idea. It’s an instrument…probably most famously you can hear it on Strawberry Fields Forever by The Beatles, but they did exactly that. They did audio recordings of real instruments. And so if there’s 50 keys on the Mellotron, it’s a keyboard instrument, there would be 50 cassette tapes.
Roland Meertens:Yes, yes. I now know what you mean. So every key has a certain cassette tape, which then starts going down and playing it, but that’s still sampling. And in this case, I guess it’s also just sampling. So you are trying to simulate.
Anthony Alford: It’s simulated in the sense of…basically just play back a recording.
Roland Meertens: Yes. But then per key, you only have one possible sound.
Anthony Alford: Exactly.
Roland Meertens: Whereas this one already took into account with what velocity you press the keys.
Anthony Alford: Oh, okay. So it has a sound per velocity almost, or could be.
Roland Meertens: Yes. So they press each key probably 25 times with different velocities, and then they record all the responses there. And this is why the software is so expensive. It’s not like someone just recorded 88 keys and called it a day. No, no, they did this 88 times 25 times, plus adding the sustained pedal or not.
Anthony Alford: It’s combinatorial explosion here.
Roland Meertens: Yes, I think it’s good value you get here.
Anthony Alford: Yes, I love it.
Roland Meertens: Well, so the other thing I was looking at is instead of sampling piano sounds, I went to the Roland store this weekend where …
Anthony Alford: Did you get a discount?
Roland Meertens: No, I had to pay like $45 for this t-shirt. No. So one thing which they do is they have a virtual drum kit. So instead of clicking on the pad and then hearing a drum, they actually have a real looking drum kit, but then when you smash it, you only hear like “puff”. So you only hear the sound over your headphones.
But I tried playing it and it still feels like a real drum kit. So the cymbals are made out of rubber. So it kind of looks weird, because it doesn’t feel exactly like a cymbal, but it sounds in your ears the same as a cymbal. And one big benefit here is that you can easily select different sounding drum kits.
Anthony Alford: Okay.
Roland Meertens: So if you want more like a metal drum kit, you select it. If you want a smooth jazzy drum kit, you can select it.
Anthony Alford: And this certainly sounds like a good solution if you have neighbors who are noise sensitive. So all they hear is pop, pop, pop, pop.
Roland Meertens: I also think that if you want to sell your house and you have a neighbor who likes to play the drums, buying this for them will probably increase your property value.
Anthony Alford: It’s worth it for that alone.
Roland Meertens: Yes. So it will set you back around $5,000, by the way.
Anthony Alford: Wow.
Roland Meertens: So I have printed it out. If you ever want to start a new hobby and not annoy your neighbors, this is the way to go.
Anthony Alford: Nice. I want to annoy my neighbors with my hobby, so I’m going to get the real thing.
Roland Meertens: In that case, I think their flagship drum kit at the Roland store was about 10k. Yes. But then you get real drums. Anyways, I will let you listen to how the drums sound.
Anthony Alford: Yes.
Not bad.
Roland Meertens: Sounds realistic, right?
Anthony Alford: Yes.
Roland Meertens: I think this is a perfect replacement for real drums, or at least it felt to me like. I would be quite happy.
Anthony Alford: Play along to that.
Simulated Musical Instruments [08:36]
Roland Meertens: No, absolutely. However, this is the simulation and not the sampling episode. So I wanted to know if people ever simulate sounds, and one of the articles I found was by Yamaha.
Anthony Alford: Of course.
Roland Meertens: Well, you say of course, but so Yamaha I think sells digital keyboards, but they also sell real pianos.
Anthony Alford: Yes. And motorcycles.
Roland Meertens: I did actually own a Yamaha scooter as a kid.
Anthony Alford: I mean, whatever. They’ll make it.
Roland Meertens: Yes. I always wonder how they went from instruments to scooters and motorcycles.
Anthony Alford: Or the other way around.
Roland Meertens: Yes. So they do have a webpage. I will link all these things in the show notes, by the way, but they do have a webpage where they talk about this, and the reason they say that they simulate sounds is different than you might think. They do this not because they want to create a virtual keyboard, but they want to know what a piano sounds like before they build it, and they want to know what design decisions impact their pianos.
Anthony Alford: Oh, interesting. That’s very clever.
Roland Meertens: Yes. So their flagship grand piano costs around $50,000. We are already going up in price here now.
Anthony Alford: How much does the motorcycle cost?
Roland Meertens: Yes, indeed. Yes. You get to choose one hobby in life. But yes, so I can imagine that if you want to build such a high quality piano, you want to know beforehand what design decisions impact the sound in which way. So yes, that’s something which I found fascinating is that they say they are using simulation to improve their real physical products.
Anthony Alford: I had never thought of that. That’s very clever.
Roland Meertens: Yes. The other thing, by the way, is that when I was looking at simulated pianos, one other reason you might want to simulate a piano is: imagine you find a super old relic piano from the past, but it’s important for historical context, right? Maybe this is the piano Mozart learned to play, or the piano George Washington imported. I don’t know.
So you don’t want to play it a lot because it might damage the piano. Or maybe it’s partially broken, maybe some keys are missing, some strings are missing and you don’t want to repair it or it’s too difficult to repair it. You can start simulating that piano and maybe simulate over a couple of keys, maybe play it once, and then record the sounds and then simulate the rest of the piano. So there’s definitely reasons that you want to simulate sound beyond “I’m a musician and I want to have a good sounding piano”.
Anthony Alford: Yes, that makes a lot of sense. My mind is just churning over the Yamaha thing. First of all, that’s one of those situations where you can make your model just as detailed as you want, and you could probably still keep going even after you think it’s done.
Roland Meertens: Yes. In that sense, I tried finding academic papers on this topic, but I didn’t find a lot here. I expected the field of simulated music to be quite large, especially because it feels relatively straightforward that you want to simulate a vibration of a string and you want to simulate how felt on the hammer impacts the string, and you want to simulate how the noise spreads through different surfaces.
So it seems like a relatively straightforward way for me as an engineer, but there were not a lot of papers of people comparing their simulation, or at least I didn’t find it.
I found one academic paper called something like Modeling a Piano, and this person had a lot of pages with matrices on what effect everything had on everything. But the paper concluded with, oh, we didn’t implement this, but you should probably use a programming language like Rust or Python or something.
Anthony Alford: I was actually speculating they probably consider it a trade secret, and it’s probably some ancient C++ code that only one old guy about to retire knows how it works.
Roland Meertens: Yes. I do know that, just continuing to talk about Roland, if you buy their amplifiers, you have a lot of artificial pedals in there. So it’s basically a software defined amplifier. And there you even have people who used to work on this technology and who retired, but who are still creating software packages for these amplifiers to make them sound like different types of amplifiers. So you can make your guitar and your amplifier sound better because there are people who are so passionate about this that they keep improving the software.
Anthony Alford: These people make the world go around sometimes.
Roland Meertens: Yes. Shout out for those people, whose names I forgot.
Also, the fun thing about this Modeling a Piano paper is that I found out that it’s basically a third year master’s student at a school of accounting who created a whole mathematical model of this piano playing, but then didn’t implement it.
Anthony Alford: Talk about somebody who just wanted a hobby. And your neighbors won’t mind if that’s your hobby.
Roland Meertens: I think with mathematics, it’s always about giving it a go. Anyway, as a consumer, you can buy simulation software for pianos, and that is that Arturia makes a piano simulator that’s called Piano V3. This one I actually own, and this one can simulate any kind of piano, has a lot of settings like what kind of backplay do you want, how old should the strings be?
And this package also costs $250. So you can either shell out $250 for recordings of a piano or for something which simulates a piano and different types of microphones and positions. Do you want to listen to a small piece?
Anthony Alford: Let’s roll that tape.
That’s very pretty.
Roland Meertens: It is quite expressive, I think. Yes. I do still have the feeling that it feels a bit more sine-wavy, and I have the feeling my MacBook speakers don’t handle it well because it seems that for a lot of the output of this, especially this program, it just seems to vibrate the keys of my MacBook in such a way that is really annoying.
Anthony Alford: You could do it with headphones, I suppose, and it would sound really good.
Roland Meertens: And then my neighbors would also be happier. So yes, that’s the outcome. There is software which can simulate how a piano works.
Anthony Alford: Very cool.
Roland Meertens: Yes.
Anthony Alford: I’ll have to check that out.
Robot Simulation – The Matrix [17:11]
Anthony Alford: Well, Roland, you were talking about Yamaha modeling and simulating the piano before they build it to see how those design decisions affect the sound of the piano.
Roland Meertens: Yes.
Anthony Alford: So imagine you’re doing that, only you’re building a robot or an embodied agent, embodied AI. Nobody does robots anymore. Okay, so when you write code without the help of an LLM…at least when I write code, it doesn’t work 100% correctly the first time.
Roland Meertens: Oh, no.
Anthony Alford: Usually, right? So the downside of having a bug in your robot code could be pretty big. Just like spending $80,000 to create a piano that sounds terrible. You have a bug in your expensive robot. So if your code might cause the robot to run off a cliff, or even worse, the robot might hurt someone.
Roland Meertens: Yes.
Anthony Alford: And probably you’re not the only programmer. You’re a part of a team. Well, everybody wants to try out their code, but maybe you only have one robot because it’s expensive, or you might have a few, but they’re shared.
Or let’s say you’re not even programming the robot because we don’t program robots anymore. The robots learn. They use reinforcement learning. That means that the robot has to do a task over and over and over again, maybe thousands of times or more. That could take a while.
Roland Meertens: Yes.
Anthony Alford: What do we do?
Roland Meertens: I guess given the theme of this episode-
Anthony Alford: The Matrix.
Roland Meertens: The Matrix.
Anthony Alford: We use The Matrix. Okay. It’s a simulator, right? Yes. No surprise, right? Spoiler. So testing your robot control code in a simulator can be a way to address these problems. So we’re going to talk about robot simulators. There’s quite a few different ones available, just like there’s a lot of different piano simulators, and I won’t get into really specific deep dive detail. Instead, we’re going to do high level and talk about what you’ll find in most simulators.
Well, the core component is some kind of world model. The world is usually sparser and more abstract than the real world. It’s got the robot in it, and you’ll put other objects in there. That will depend on what task the robot’s for. If you’re doing an industrial pick and place robot, that’s great. All you need is the workstation and the items that the robot’s picking up. You don’t need to have obstacles and other robots it has to avoid. But if you’re simulating a mobile robot, you’ll need walls, obstacles. If it’s outside, you’ll need buildings, sand traps, people.
Typically in a simulator, you describe these objects in some sort of standard format in a file. You define their geometry and other physical properties. There’s some common file formats for these. There’s Unified Robotics Description Format, URDF. There’s a Simulation Description Format, SDF. So the idea is very similar. With a physical robot, you model it usually as a collection of links and joints. So we’re thinking about a robot manipulator that’s an arm. It has joints and bones.
Roland Meertens: And these are standards for every simulator, or is it-
Anthony Alford: Not every one, but these are for some common popular simulators. But the thing that they accomplish is going to be common to almost any simulator. You have to physically define the objects in the world. And robots in particular usually consist of links and joints. And if you mentally replace “link” with “bone” or “limb”, you could probably describe the position of your body that way, your configuration. You’ve got your arm raised or stretched out, do the YMCA.
Roland Meertens: Yes, with your links and joints.
Anthony Alford: So these attributes define the shape of the robot, how it can move. And you can specify other properties like the mass or moment of inertia of the links. And the reason you do that is because the next key ingredient is the physics engine.
So here’s where we’re calculating the motion of the robot and other objects, the forces on these objects, the forces between objects due to collisions, and things like that. So this is basically Newton’s laws. This is the part where we’re trying to protect the robot, trying to save the robot from damaging itself or from hurting other people. So this is pretty important.
Roland Meertens: Yes. The better the simulator is, the more confident you are that your robot is going to behave safely.
Anthony Alford: Yes. So one important piece of simulating robot motion is called kinematics. There’s two directions of kinematics. There’s forward kinematics, and this is where, for example, with the robot arm, you know the angles of the joints. Forward kinematics computes the 3D position and orientation of the end effector—of the “hand”.
And if you recall our previous episode, we were talking about coordinate systems. This is based on transforming coordinate systems. And by the way, the mathematics involved is matrix multiplication. So there’s the matrix tie in again.
Roland Meertens: Yes. So forward kinematics is quite easy, right? Because you know the exact angle of each motor, and then you know exactly where your robot arm is.
Anthony Alford: That’s right. The opposite of that is inverse kinematics. And that’s where you know what 3D position and orientation you want the end-effector to have. You need to figure out the joint angles to get there. And that is harder to compute because you have to invert those matrices.
So now we’ve got the robot’s motion. So we can command the robot, put the end effector at some 3D point, figure out the joints, angles, and drive it to those angles. But there’s also sensors. So the robots usually have sensors. You’ll have things like LIDAR, sonar, proximity sensors, vision cameras.
So to simulate sensor data, especially for those cameras, you need some sort of 3D graphics rendering. And what’s nice is that a lot of the info you need for the physics of the robot in the world can also be used for the graphics.
So you have to include things like colors and textures and maybe some reflectivity and light sources. And surprise, the mathematics for the 3D graphics are very similar: coordinate transformations and matrix multiplication.
Video Games and Other Simulation Frameworks [23:57]
Anthony Alford: So quiz time. What broad class of software usually includes both physics and 3D graphics besides robot simulator?
Roland Meertens: Is it video games?
Anthony Alford: It’s video games, absolutely. All technological progress is driven by video games. And in fact, some of these game creation frameworks or game engines are actually sometimes used for robotic simulation. There’s one called Unity, and there are several InfoQ news articles that cover embodied agent challenges that are built using the Unity game engine.
Roland Meertens: I’m always surprised that if you think about the costs to build an accurate representation of a world, Grand Theft Auto is actually quite good.
Anthony Alford: I wonder how many people are training their robot using Grand Theft Auto.
Roland Meertens: So it used to be quite a lot.
Anthony Alford: Oh, really?
Roland Meertens: Yes. But they explicitly make it hard to use it as an API because they don’t want people cheating in their games, which is a bit lame because it’s just already an accurate simulator of life in America.
Anthony Alford: Hey, come on! Some of it, maybe.
Roland Meertens: I will tell you this, that I first played Grand Theft Auto and I was like, “The real world can’t behave like this”. And I came to America and I was like, “Ah, some of these things are actually true”.
Anthony Alford: I’m so ashamed.
Okay. Since we’re name-dropping some simulation frameworks, I might as well mention a few others. There’s one called Gazebo.
Roland Meertens: Oh yes, I love Gazebo. Yes.
Anthony Alford: I knew you would be familiar with that. It’s an open source project. It’s maintained by Open Robotics, which also maintains ROS, the Robot Operating System. And InfoQ has a great presentation by a software engineer from Open Robotics that is about simulating with Gazebo and ROS.
Roland Meertens: Yes, I actually invited her to speak at QCon AI.
Anthony Alford: I sometimes often seem to be doing the half of the podcast that you’re the actual expert in.
Roland Meertens: Well, I mean Louise Poubel is in this case the expert, right? I just invited her.
Anthony Alford: Yes, you’re correct. Some of the big AI players, in fact, have their own simulation platforms. NVIDIA has one called IsaacSim, and that’s part of their Omniverse ecosystem.
Meta has one called Habitat, and that one has an emphasis on stimulating spaces like homes where people and robots might interact.
Roland Meertens: And is Habitat easily accessible?
Anthony Alford: It’s open source, yes. They do challenges where they invite people to build virtual robots that operate in the Habitat. Yes.
Roland Meertens: Nice.
Anthony Alford: I don’t know if they’re still doing this, but they tried to pivot to this Metaverse thing, which is basically a simulator, but for people, maybe the real matrix, the alpha version.
Roland Meertens: Yes. Meta is not super active in the robotics space, are they?
Anthony Alford: Again, they’ve built a simulation environment and they’re inviting people to build on it, so maybe.
Roland Meertens: And they already have the technology to do accurate 3D localization. They have all the ingredients to create super cool robots.
Anthony Alford: Yes. So Google is doing robotics. Google, is there anything Google doesn’t do? I don’t know.
So I mentioned ROS, and now as it happens, many of these simulations have integrations with ROS. Because the idea, remember is we’re trying out the robot control software, and that really means the entire stack, which includes the OS.
So you’ve got your control software running on the OS, and instead of the OS interacting with the real robot, sending commands to actual motors and reading physical sensors, instead it can do all that with the simulated robot.
But I keep assuming that we wrote control software. Of course, nowadays, nobody wants to hand code control algorithms. Instead, we want the robot to interact with the environment and learn its own control software. And that’s again, as I said, another reason to use simulation. Because reinforcement learning requires so many iterations.
And, no surprise, there are reinforcement learning frameworks that can integrate with the simulators. So a lot of times these are called a gym or a lab. For example, NVIDIA has a framework called Isaac Lab that works with their Isaac simulator. Meta has a Habitat Lab for Habitat, and probably a lot of us remember OpenAI’s Gym. You could play Atari games and things like that. That’s now called Gymnasium, and it integrates with Gazebo and ROS.
Roland Meertens: Oh, so the OpenAI Gymnasium now also interacts with all these other open simulators.
Anthony Alford: Well, at least somebody has built an integration. So you can find integrations. They may not be … I don’t know how official some of them are.
So the key idea with these reinforcement learning frameworks, these gyms or labs, is that they provide an abstraction that’s useful for reinforcement learning. So basically they provide you an abstraction of an environment, actions, and rewards. And because it’s operating on a simulator instead of the real world, in theory, you can do many episodes very quickly.
Roland Meertens: Yes, I tried this with OpenAI Gym. It was quite fun to play around with.
Anthony Alford: So you’ve simulated pianos to various degrees of success. You may wonder how well robot simulators work in practice. Train a robot in the simulated world, what happens when you let it loose on the real world?
Roland Meertens: What’s the reality gap?
The Reality Gap [29:42]
Yes, that’s exactly right. It’s called sim to real, the reality gap. The robot learns from an imperfect simulation, and so it behaves imperfectly in the real world. So this is an active research area, and there are different approaches.
One that popped up a couple of times in recent work is called domain randomization. Now this is where you apply randomization to different parameters in your simulation. So for example, maybe you have a random amount of friction between some surfaces, or you randomly add or subtract something from the weight or shape of a robot link. And the reason this works according to the researchers is it acts like regularization. So it keeps the model from overfitting.
Roland Meertens: Yes, by adjusting the domain just a bit.
Simulation Makes Dreams Come True [30:31]
Anthony Alford: Mm-hmm. So one final thought: earlier I brought up The Matrix.
Roland Meertens: Yes.
Anthony Alford: I was never a huge Matrix fan. I felt like it violated the laws of thermodynamics to say that the people were power sources. What if that’s not actually what happened? Because we could see in the movie that The Matrix is a simulation environment and humans can learn skills like Kung Fu very quickly, just like bam. So what if that’s what The Matrix was actually originally for.
Roland Meertens: The learning environment?
Anthony Alford: Exactly. So believe it or not, as I was preparing for this podcast, I came across an interesting biology preprint paper. The title is The Brain Simulates Actions and Their Consequences during REM Sleep. So basically the authors found that in mice, there’s a motor command center in the mouse brains, and it’s involved in “orienting movements”. It issues motor commands during REM sleep.
So these are things like turn right, turn left. And they found that although the mouse’s real physical head isn’t actually turning, the internal representation of where the mouse’s head is pointing does move. They look at certain neurons that are responsible for-
Roland Meertens: Interesting.
Anthony Alford: Yes. I don’t know how they—they’re basically reading the mouse’s mind somehow. Anyway, they suggest that “during REM sleep, the brain simulates actions by issuing motor commands that while not executed, have consequences as if they had been. The study suggests that the sleeping brain, while disengaged from the external world, uses its internal model of the world to simulate interactions with it”.
So it seems like dreams are like an organic version of The Matrix. Basically we do know that your memories and what you’ve learned do get affected by sleep, especially REM sleep.
Roland Meertens: Your dreams will come true.
Anthony Alford: They do. And so that’s a better tagline than I had. So we’re going to end it on that.
Roland Meertens: Yes, I like it. Make sleep even more important than you realize.
Anthony Alford: So there we go. Let’s sum it up: Simulations make your dreams come true.
Roland Meertens: Probably.
Anthony Alford: Bam. Headline.
Roland Meertens: Nice.
Grand Theft Auto: Columbo [32:57]
Roland Meertens: All right. Last but not least, some words of wisdom. Do you have some fun facts? Anything you recently learned which you want to share?
Anthony Alford: I don’t have something new, but something that occurred to me while you were talking about simulating musical instruments. Supposedly the theremin, which I think we’ve probably talked about before, the musical instrument you don’t touch, I believe the inventor of that was trying to at least mimic the sound of a cello.
Roland Meertens: Interesting. It’s also fascinating that with the synthesizer, people started trying to mimic existing sounds, but then it became a whole thing on its own to use those artificial sounds as its own separate …
Anthony Alford: Brand new thing: square wave, sawtooth.
Roland Meertens: Square wave. Yes. Yes. It’s its own art form now.
Anthony Alford: Definitely. And in a way, a quite dominant one.
Roland Meertens: Yes, no, definitely. And then also, if you think about different eras, if you want to have an ’80s sound, you are thinking of synthesizers, which are playing …
Anthony Alford: Yamaha FM.
Roland Meertens: The Roland 808s.
Anthony Alford: Oh yes.
Roland Meertens: Yes. No indeed. So it is interesting that the inaccuracies also become their own art form.
Anthony Alford: Very true.
Roland Meertens: Where I must say that I often, as a software engineer, strive for perfection, but maybe sometimes you can stop sooner and then just call it a new thing and then you’re also done.
Anthony Alford: It’s not a bug. It’s a feature.
Roland Meertens: On my side, I found this very interesting video yesterday. We talked about juggling robots in the first season, and I found someone called Harrison Low. He is building a robot which can juggle, and it’s quite interesting to see what he came up with because he has a super complicated system with multiple axes, which can move up and down and throw balls and catch balls again. It can also hold two balls in the air.
It doesn’t sense yet, but it’s very interesting to see his progress. So I will put that in show notes, so you can take a look at that.
Anthony Alford: I wonder if he uses a simulator.
Roland Meertens: I don’t think so, but I’m not sure.
Anthony Alford: Now that’s hardcore.
Roland Meertens: Yes. I guess he has some kind of 3D model, so at least knows how different things impact his 3D model.
Anthony Alford: One would assume.
Roland Meertens: Yes. It’s just annoying with the simulations that the devil always seems to be in the details, that tiny, tiny things tend to be really important.
Anthony Alford: Maybe he could run it in GTA.
Roland Meertens: Yes.
One fun thing, by the way, talking about the reality gap is that I always notice in my machine learning career that the things you think matter are not the things which actually matter.
Anthony Alford: Ah, yes.
Roland Meertens: At some point, for example, with self-driving cars, people ask me, “Oh, what’s important to simulate?” And I was like, “Oh, I don’t know”.
Anthony Alford: You’ll find out.
Roland Meertens: Yes, like, “Oh, I don’t know, like windows are maybe transparent or something”. We noticed at some point that a neural network, we trained to recognize cars. It really knew that something was a car because of the reflective number plates.
But if you ask me what’s the most defining feature of a car, I would be like, “I don’t know, like it has a hood, has four wheels”. Nobody ever says reflective number plates.
Anthony Alford: Interesting.
Roland Meertens: Yes. Shows again that you never know what you really want to simulate accurately.
I guess that’s it for our episode. Thank you very much for listening to Generally AI, an InfoQ podcast. Like and subscribe to it, share it with your family, share it with your friends.
Anything to add to that, Anthony?
Anthony Alford: No, this was a fun one. I really enjoyed this one.
Roland Meertens: All right.
Anthony Alford: I enjoy all of them, but this one was especially enjoyable.
Roland Meertens: Cool. And my apologies to America for comparing you to Grand Theft Auto. And my apologies to Sri Lanka for saying that 10% of drivers are reckless.
Anthony Alford: Grand Theft Auto Colombo.
Roland Meertens: Yes. Well, I guess you could easily say-
Anthony Alford: Show title.
Roland Meertens: Show title. Cool. I think that’s it for today.
Anthony Alford: Oh, too funny.
Roland Meertens: It is too funny.
Mentioned:
.
From this page you also have access to our recorded show notes. They all have clickable links that will take you directly to that part of the audio.