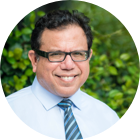
MMS • Lexy Kassan
Article originally posted on InfoQ. Visit InfoQ
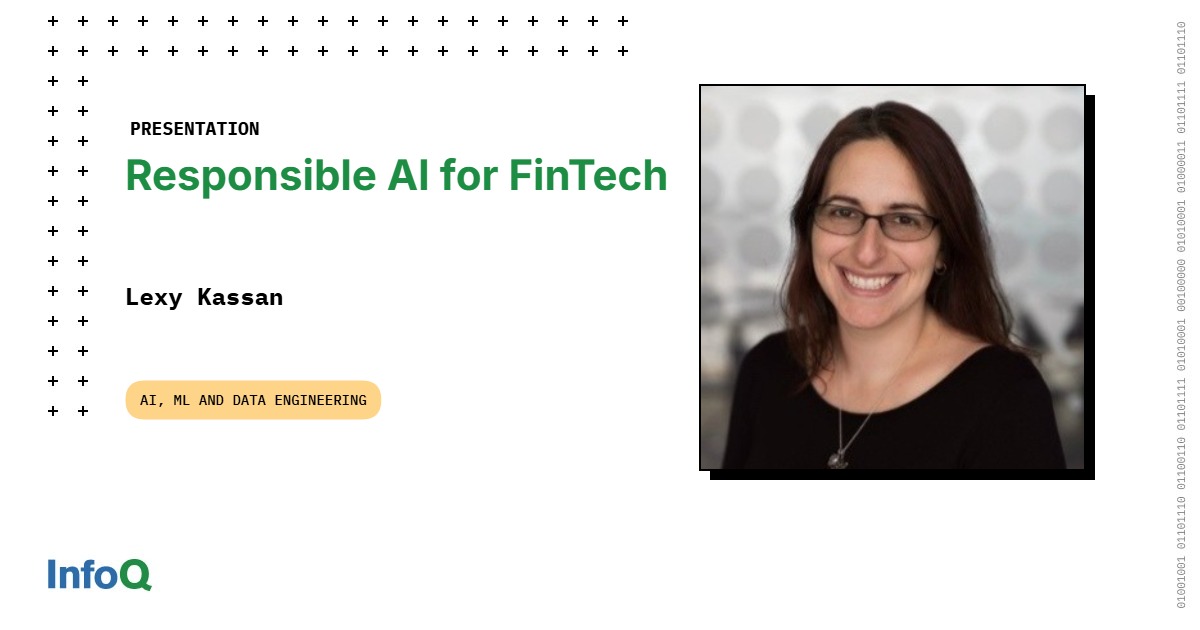
Transcript
Kassan: Internally at Databricks, I call myself the governess of data. The topics I end up covering are things like responsibility and capability maturity, and stuff like that. It sounds like I’m everyone’s nanny, hopefully more of the like Mary Poppins style than the ones that get taken away on umbrellas. I’ll talk a little bit about responsible AI, some of the things that we’re seeing now. How organizations are approaching that. A little bit of a regulation update. Obviously, there’s quite a lot going on in the space, and in particular, for FinTech, there are some specific things in there as well. Then, the response that we’re seeing in the industry and how that’s being approached.
Responsible AI
I was going to start with responsible AI. I like to lay the groundwork of what we’re talking about. One of the things to think about this, just to level set, 80% of companies are planning to increase their investment in responsible AI. Over the last few years, especially, we’ve seen a tremendous increase, of course, in the desire to use AI, and a parallel increase in the need to think about what that means from a risk perspective. How do you get the value of AI and the capabilities that it will drive for your organization and unlock that value, without creating a massive reputational risk for your company, or potentially business, and financial risks? When we think about responsible AI, we think of in multiple levels. A lot of people talk about the ethics. That’s where I started, actually. I was in data science ethics. I had a podcast for it for several years.
As a data scientist who came into the industry when things weren’t quite such a buzz, it was so important to see where could it go. There’s multiple levels. At the very bottom is where we see regulatory compliance. We have to follow the guidelines that are set in place to be compliant with regulation. Otherwise, we’re going to be out of business. That’s how it works. In the ethics space, this is like, what should we do? If we could make AI act the way we would act and have the same values that we have, what should we do? Getting there, as we may have seen in the last year and a half, is very challenging. Really, where we end up is, what can we do? What are the things that we can put in place to be responsible with AI and put in place guardrails that we actually can enforce, knowing that the aspiration is to get to ethics, but the realistic intention and goal is to be responsible. That’s where we’re at. That 80% of companies are looking to say, “Yes, I have to be compliant. That I’ve already invested in. What else can I do?” This is that next step.
I break this down to four levels. If you think about coming from the top down. At the top, you have the program, that’s really the, if we could do anything, if we could be the most ethical people that we intend to be, what does that look like for our company? It’s setting the stage on the ethical principles and the vision of what our organization wants to achieve and intends to be. This is where, at those top level, you typically see somewhere between four and six ethical principles in a given company. Some group of people, probably in the C-suite, maybe the board, are setting out some high-level principles, like, we want to be fair and unbiased. We want to be transparent. We want to be human centric. These kinds of very big, vague, floaty ideas.
Then, at some point, you have to translate that down. You say, that’s great and all. What does that mean to those of us who are actually in the organization? What is it we have to do to be whatever vague concept you’ve just thrown out there? That’s where you see policies. This is that translation down one level to say, what are the frameworks, what are the rules of the road? Are there specific things that that means we can or can’t do as an organization? Do we have to say, every time we’re going to create an AI, we need to evaluate it against this policy and say, is this allowed? Is this ok for us in our organization? Every organization is going to handle this a little differently.
Beneath that, the next level of translation is then, how do we create processes to enforce the policy? What does it look like to start enacting that on a daily basis? Do we have an AI review board that looks at this and says, does a given application of AI conform with the policy, conform with therefore the principles? Do we have auditing over time? How does this change other processes in our organization, like procurement, or like cybersecurity, for example? This is where you have all the different processes that the organization is going to operate under.
Then, at the bottom, you’ve got the practice, the actual hands to keyboard building these things. What is the way that we’re going to actually implement responsible AI? What are the tools and the templates and the techniques that we’re going to use to evaluate AI as we start to build it into our organization? What you find is that this middle section is what ends up being called AI governance. It’s governing the practice. It’s governing to make sure that these tools, templates, and techniques, abide by the processes and follow that process that is then aligned to the policy that then hopefully gets you closer to your principles. This is the stack of responsible AI.
In terms of principles, I’ve summarized from a lot of different frameworks these that end up being roughly what you find. It’s usually some subset of these. Basically, this is the picklist that ends up happening at the highest levels of, which of these do we think is most important? We’ve seen a lot more emphasis, for example, on security lately. Because there’s a lot of concern about, how do we make sure that IP is not infringed, that our information is not getting put out there, that our customers’ information is not going to be exposed? This safety and security ends up being a big part of that. Of course, we’ve also seen that in privacy as well. Now, with additional regulation, compliance is taking on more meaning, has more aspects to it. Other things that we find, for example, is efficiency.
Typically, building AI takes a lot of power, takes a lot of processing, takes a lot of money. Not the thing that most organizations, especially FinTechs, want to spend to do this. If they can get the capability less expensive, they want to. Efficiency is something that’s been talked about more because the energy costs of creating and maintaining and doing inference with AI, especially large language models, is becoming a bigger concern. How do we power these things? If efficiency becomes one of your principles, then you look at how you minimize that to still get the same outputs while not impacting the environment and also not impacting your budget.
Regulation Update
Quick update on regulation. Again, 77% of companies see regulatory compliance and regulation as a priority for AI. Of course, with the EU AI Act, that’s increasing. Anyone who does business with, or near, or on EU citizens, this is going to be a concern with the EU AI Act coming in. We’ve also seen new regulation elsewhere, and I’ll do a little bit of a mapping on that. Of course, in that ladder, the first step is, can we be compliant? With regulation changing, what it means to be compliant is changing. Of course, companies are saying, yes, we should probably still also be compliant with whatever happens. This is probably not up to date. It changes literally daily. We’re seeing regulation all over the place. The patterns that it takes is different.
In the States, what we’ve seen up to this point is more an indication that existing laws need to be abided, even if you’re using AI to do the thing. In Europe, they’re taking a different approach and saying, we’re going to have this risk based hierarchical view of how AI can and should be applied. In China, they’re saying, you can do AI as long as it is in accord with the party aspirations and how they think about the way they want their society to run. In fairness, that’s how responsible AI and regulation works. Everybody has their view of what does it mean to be responsible? What values should we be enforcing? Not universal, which makes it, again, very difficult if you’re operating in a multinational environment. We’re also starting to see additional regulations coming in in Japan, in India, in Brazil, lots of different approaches. In Australia, of course. Tons of different ways that this actually ends up implemented, and the things that you need to comply with will be different by jurisdiction.
I’ll go through a couple. Please understand that for a lot of them, preexisting laws do still apply. This is what I was talking about. In the states, if you look at the way they’re handling it, they basically say, if something was illegal before when a human was doing it, it’s still illegal if an AI does it. You’re not allowed to discriminate based on protected classes, if you’re a human. You’re not allowed to discriminate algorithmically if you’re using AI. That’s how that goes. Data and consumer protection still apply. You don’t get to just blast people’s information out there, because the AI accidentally did it, which we’ve seen. Intellectual property still applies. All the copyright disputes and IP infringements that have been spinning up in litigation still applies on AI. Anti-discrimination. Anything criminal.
For example, there’s a lot of worry about, could AI tell someone how to do something terrible that would constitute criminal behavior? There’s a lot of concern around, how do you guard against your AI saying something it shouldn’t, that it probably knows about, but you don’t want anyone to know it knows about, just based on how it was trained. There’s a lot of filtering that goes on for that. Then antitrust and unfair competition. Basically, with antitrust, it’s saying, you can’t use AI to create a non-competitive environment, despite the fact that the AI itself might be doing that.
EU AI Act: four categories of risk. You’ve probably heard about this. How many people have looked up the EU AI Act? It’s new to some people. Four categorizations. In the top left over there, unacceptable risk, is stuff that like it’s just disallowed, flat out disallowed. It’s things like behavioral profiling and all kinds of stuff with biometrics and stuff like that. The idea is that if you’re materially distorting behavior, or you’re doing things that are really privacy invading, those are prohibited. Just can’t do. Unless you’re the government or the military or a bunch of other things, just asterisks all over this slide. Imagine asterisks everywhere, like it’s snowing. Snowing caveats. In that category, hopefully in your organization, you’re not dealing with any of those things. If you are, probably think about ways to take them out now, because this is going to happen. This is going to come into effect in a year or so, year and a half. High risk.
In high risk, what it’s basically saying is, if there’s the potential for this to influence someone’s means of earning a living, means of living, health, safety, access to critical infrastructure, those kinds of things, you have to go through a very rigorous documentation process called the conformity assessment. This is where a fair bit of FinTech organizations are going to end up having something to do, and probably in the limited risk, which I’ll get to. The conformity assessment is a lot.
That said, finance has been doing impact assessments and compliance documentation for decades. I’ve been there. It sucks, but you do it. You put out reams of documentation about how you got to the conclusion you got to. Why this model was the best one of all the things you tested. Why these features were selected. What their relative importance is. How you’ve tested for disparate impact, all that good stuff. It’s that plus a bunch of other things that go into the conformity assessment. The documentation that you’ve done so far, drop in the bucket. My hope, personally, is that we can then train an LLM to help generate the documentation, and then we can figure out which bucket that falls into. I’m thinking minimal. That’s the high-risk group. There are some caveats on that as well.
Limited risk, basically, if it’s not one of the other two, but it interacts with a person, you have to tell the person, “You’re interacting with an AI”. The fun one I think about here is, a few years ago, Google did a demonstration of what was a Duo, or something like that, where they had an AI application that called and made a booking at a restaurant, or something like that. If that were the case under the EU AI Act, the phone call would sound like, “Hi, this is Google Duo calling. This is an AI. I would like to book a reservation for this particular person on this date and time”. Because you have to tell them. This is true then for all the chatbots that are being created and all these use cases that are coming in now where there’s an interaction with a person. Similarly, it would work for internal. Even if you’re not necessarily displacing customer service, for example, if you’re augmenting your customer service staff, you have to tell your customer service staff, this is an AI chatbot that’s giving you a script.
This is not a preprogrammed defined rules engine, this is an AI. Just so they’re aware. Then everything else is minimal risk. For example, things like fraud detection fall into minimal risk, according to the way that the current structure has been laid out. These are very vague. There are examples in the act, if you want to read through the 200 and some odd pages of it. I don’t recommend it unless you have insomnia, in which case, have at. The majority of what this will do, I think, again, not a lawyer, my own interpretation is a lot of how this is going to come together, will happen in litigation. As new applications come online once this is enforced, we will probably see a lot of the rules get a little bit more clear as to what they consider high risk, unacceptable risk, lower risk, and so forth. Because right now, there’s some vague understandings of what we think people are going to try and do with AI, but it’s not specific yet.
In the act, there are also specific rules around general-purpose AI and foundation models. If you’re training a general-purpose or a foundation model on your own, first of all, I would like your budget. Secondly, there are specific rules around how you have to be transparent, and how many flops you can have, and all kinds of crazy stuff. That’s for the few that want to actually build a model, which at Databricks we did. We had to actually look at that stuff.
The other one I wanted to talk about, specific to FinTechs, is consumer duty. This came in, I think, last year. It has some interesting implications for responsible AI. First of all, it says, design for good customer outcomes. How do you define a good customer outcome? How do you know that that customer outcome avoids foreseeable harm? This is going back to that conformity assessment, disparate impact assessment, all these things that you have to prove are doing the right thing for your customers. The next part is demonstrating your supply chain.
With AI, the supply chain gets a little nebulous, so you have to think about, what is your procurement process? How do you track what data is coming in, being used in your AI, how it’s being labeled, how it’s being featurized, how it’s being vectorized or chunked, or whatever you’re using, to be able to actually put it into an AI application. For FinTech, there’s this extra bit. Again, I think it’s probably mostly there for most financial services companies, including FinTech, because, again, we’ve been subject to this stuff for a long time. Things to think about with our new technologies is, how is this actually going to play out over time?
FinTech Response
It does bring us into the response, though, from FinTech. More stats, because I’m a numbers nerd. According to a survey of FinTechs, they expect a 10% to 30% revenue boost in the next 3 years, most leaders in FinTech, based on the use of generative AI specifically. This is not uncommon. I’ve heard this 30% thing bandied about. I think McKinsey had another one that was like, 30% efficiency from using generative AI. You’re going to save 30% of whatever costs, and all this stuff. Maybe. The reputation of FinTech is two things. There’s the disruption angle. From a technology perspective or digital native perspective, it’s also open source. When you think about how you’re going to achieve this 10% to 30% in a way that others aren’t, so you want to be disruptive. You don’t want to be the next J.P. Morgan, who’s saying, yes, we can incrementally improve our efficiency by 10%. FinTech is saying, no, we want to do something massively different, completely different from what the big guys are doing, disrupt it.
Often, especially in early stage, you want to go for something that you can build and control. It’s actually an advantage now, because when you talk about knowing and ensuring your supply chain for AI, being able to have transparency, driving towards responsibility. The more you use open source and can see the code and can see the data and can see the weights and can show all of that, the better you are able to take that, use it to your advantage, prove the supply chain. Go through the conformity assessments, and disrupt, so that you’re not the one sitting there going, yes, I will incrementally improve my efficiency, and I might get a 5% decrease in some sort of cost. We’re seeing, in FinTech, a lot of interest in the open-source models, a lot of interest in being able to build and fine-tune, especially for LLMs. Of course, that’s always been there for machine learning, and data science, and so forth. I’ve not yet met a FinTech using SaaS, which I’m very grateful for, but just taking advantage of what’s available.
That said, as a disruptor, there’s nothing holding you back from saying, we think there’s a 20% reduction in workforce that we could actually achieve. What’s interesting here is that it’s the unspoken bit in other industries, but in FinTech, it’s actually moving in that direction. There’s a lot of noise about it. Going from processes that had been manual through to augmenting people, and then eventually automating those capabilities. A great example of that was Klarna. A couple months ago, Klarna’s CEO, it was actually on their own website, on their blog, said, we have a chatbot that’s been handling two-thirds of our customer service requests over the last couple of months. It’s gotten better quality. There have been fewer issues where people had to come back and talk to somebody again.
We’re looking at that, and we’re seeing that it could replace 700 workers. They were public about this. Why? Because FinTech, most digital natives, are known for disrupting. They rely on technology. It’s that techno solutionism of being able to say, yes, we want to do this. Thinking back to those principles, does that make you human centric or not? These are the decisions that end up having ramifications for what you do with AI. If you’re saying we’re customer centric, we want to make sure that the humans that we’re serving are our customers, but that means potentially not serving our employees in the same way, not ensuring their continued work in this company.
Although, frankly, what he said was those were all outsourced people, so they don’t count as our employees, which, ethically vague. There’s a reason I always wear gray when I give talks about responsible AI, everything’s gray. This is something that’s very much happening now. I can tell you that they’re not the only company saying we think there’s a workforce reduction. I spoke with another very large organization not long ago that said we have 2000 analysts, but we think if we put in place the right tooling, we put in place the right AI, we could drop that to about 200. They’re not saying it publicly. They’re not telling their analysts, your job is on the line, but it’s still there. It’s happening now.
How do we move through this pattern together? From a responsibility perspective, first thing, establish your principles. That includes, how much transparency are you going to give, to whom, in what? These are the policies internally you need to set up. For organizations that have risk management, which FinTech should, extend your risk management framework to include AI. That’s happening now. They’re evaluating, what are the risks that we’re taking on when we put AI into place. Identify what I call no-fly zones. There are some organizations that are saying, we’re probably not in the high-risk camp most of the time, and we don’t really want to go through this conformity assessment stuff. If we could just never use AI in anything to do with HR, that’d be great. Because the moment it touches something like employment status, or pay, or performance, conformity assessment is required. Sometimes it’s just not worth it. Cross-functionally implementing responsible AI. There’s a lot in that one bullet.
This is something that, again, at the start, we’re seeing a lot more security getting involved. We’re seeing CISOs, legal teams, compliance, AI, governance, all coming together to figure out, what do we do? How do we safeguard the organization? How do we look at risk management differently? Do we bring in the risk officers in conjunction with all these other groups? Build your AI review board, including all these cross-functional folks, so that you can establish a holistic approach.
Then, set up practical processes. Saying, we’re just going to do a conformity assessment for every single AI that we ever have, just in case, probably not practical. May or may not need to do it, again, unless the magic LLM that happens someday is able to do all that documentation for you, which, here’s hoping. Try to think about what are all the teams that actually need to come together to help you solve for responsible AI. Especially in FinTech, a lot of this already exists. You’ve got risk management frameworks. You’ve got a model risk management capability. You’ve probably done some amount of documentation of this stuff before. You’ve got a lot of the components, all the people in different teams that could be part of this.
Questions and Answers
Ellis: You said 80% of companies are investing in ethical or responsible AI, does that mean 20% are investing in irresponsible AI?
Kassan: Twenty-percent probably have the hubris to think that they’ve already invested enough.
Ellis: When you talked about limited risk informing, what are you seeing with AIs dealing with AI? Because you talk about AIs dealing with humans a lot. Will you see a stage where AIs have to inform each AI that they’re dealing with an AI? How does that all work? Or what are you seeing with regulations around AIs dealing with AIs?
Kassan: I haven’t seen a tremendous amount of regulation around that area. What I have seen is more that AI will govern AI. It’s that adversarial approach of having an AI that says, explain to me why you said this, as a second buffer. Because a human can’t be there all the time to indicate, yes, this is a good response, or, no, it’s a bad response, in a generative nature. When you’re doing things like governing and checking and evaluating the responses after somebody’s prompted and saying, is this a response that we would want? It’s actually more scalable and effective to have another AI in place as the governor of that. That’s something I am seeing that’s come up quite a bit. It’s things like algorithmic red teaming. Yes, you could have someone try and sit there and type, but humans are constrained as to how quickly we can type and think up the next use case and the next thing we want to try and trick it to do. AIs can do that a lot faster. If we say to one AI, trick that one into saying this, it will find ways, and it’ll do it quick.
Participant 1: We started thinking about enforcing the policy of adding more use cases using generative AI. We came to a conclusion that we have to create a committee to approve every use case. What’s your takeaway about creating that type of committee?
Kassan: That’s the AI review board idea. A couple of things that that body would do. One is set up the policies, so making sure that you have that framework at the start. Also, looking at the processes. At what point do different business areas need to come to the board and say, we have this idea for a new AI application. Maybe they’ve already chatted with somebody who’s from the AI team, data science, machine learning, whatever it might be, to say, we think we want to solve it this way, so that the AI review board can then say, does this realistically conform with the policies, and processes, and so forth. It becomes that touchpoint. There are two issues with that, though. One is, if you don’t have a solid set of information on how you’re going to manage and mitigate risks, that can cause a lot of looping.
The other is, if they don’t meet often, that can cause a bit of a time suck. The two of those compound. You want to make sure that this is something where there’s enough of a framework there, and this comes back to the risk management framework. Understanding, categorizing, and quantifying the risks that you’re taking and understanding what can be done to mitigate them. Having enough information when you first go to that AI review board to say, here’s the stuff that you’re going to need as inputs to risk management. Here are the mitigations we’re planning. Here’s what we’re looking at, so that they can say, yes, go ahead. Then also just making sure they meet pretty frequently.
Participant 2: Do you think we will see a lot of chatbots giving financial advices in the future. What are the regulations in that area?
Kassan: There’s a lot of discussion around AI giving financial advice. There are regulations already in place about robo-advising. It’s been out there. Robo-advising has been out for some time, even without generative AI. I think the regulation will still apply. That said, there’s enough information for us to see that, yes, it’s something that, if the regulation allows in the jurisdiction, companies will absolutely be approaching it. I think the question there becomes one of, how many permutations of advice do you really want to offer? How customized does that become? What other information do you give access to that chatbot? For robo-advising, really, any of that, you’d want to have sufficient, up-to-date information so that it’s not giving advice on, for example, stock performances from six months ago. It’s looking at what’s happening now.
You want to make sure it’s constantly getting information. How many different places and how much information you’re going to feed it on an ongoing basis, because there’s a cost to that? It’s thinking about, what is that going to get you? How much do you want to do? Or, do you set it as almost like a rules-based engine, the way that a lot of robo-advisors do anyways, which is, instead of saying, these specific things would constitute a good portfolio for you. You say, you’re in this risk category, risk appetite category, so we recommend this fund, or whatever, and it’s a picklist. I think it’ll depend on the regulation. Like I said earlier, there are different approaches in every jurisdiction. It’s certainly a use case that comes up quite a bit.
Participant 3: There seems to be two schools of thought around bias, you either eliminate from the dataset beforehand, or you work through and let it be eliminated afterwards. What’s your thought on that?
Kassan: Neither is possible. Bias is something that we try to mitigate, but it’s very challenging, because the biases that you’re trying to take out mean that you’re putting in other biases. Good luck. It’s a vicious cycle. Bias is something that everyone has, various cognitive biases, contextual biases, and so forth. The more we try and do something about it, the more it’s just changing what bias is represented. It’s not to say don’t do it or don’t try to mitigate it, but just be aware that there will be biases. Certain of them are illegal in various countries, and those are the ones you definitely want to mitigate. That’s really what it comes down to.
Participant 4: When we get past the AIs coming for the job of the person operating the phone to the person who’s doing financial trading and moving up the stack. How do we avoid the financial war game scenario where the bots just trade away all the money?
Kassan: Generative AI is not necessarily going to be doing the trading immediately. That’s something that is a little bit different. You’re talking about different styles of agents at that point. It is helpful to think about what limits you put on these systems, so those no-fly zones. Do you allow AI to trade at certain levels? For example, how much do you automate? How much do you augment a human? Or, do you have a human in the loop where the AI says, I think it’d be prudent to do X, Y, and Z, but somebody has to approve it.
Participant 4: Do the regulations prevent someone from making the bad decision that says, I’m going to try and go fully automated on this, when the responsible thing is, keep a human in the loop.
Kassan: Some of it does. To the point earlier around robo-advising or financial advice, certain jurisdictions say you’re not allowed to give financial advice in a chatbot, for example. At that point, great. You’re not automating, you might be augmenting. Instead of a chatbot saying it directly to the person. You’ve got a financial advisor who types something in, and the chatbot says to them, here’s what I’d recommend for this client. Then they get to say, yes or no. Sometimes, depending on the jurisdiction, that’s what you’d end up seeing.
Participant 5: With LLMs being adopted quite widely, do you see that the onus being on being compliant, running against those companies that are building these LLMs, so they have like ISO, or some sort of certification that embeds that confidence that they’re compliant to a certain level.
Kassan: Compliance is an interesting one on this, because compliance is on a per application level at this point. You don’t get certified as a company that you are compliant with the AI regulation. You have to have every single AI certified, if you’re part of the conformity assessment. Every one of them has to go through that. You don’t get a blanket statement saying, stamp, you’re good. I think with respect to LLMs, the probability of things going more awry than in classical machine learning and data science is higher. I think there’s a higher burden of proof to say that we’ve done what we can to try and limit and to try to be responsible with it. It’s certainly acknowledged in all the regulations, but it’s going to be harder. Frankly, when you look at who’s on the hook, the actual liability of what happens, it’s still people. A lot of the regulation has actually been quite clear that it’s still a person, or people who are responsible for the actions of the AI.
Good example of that was the whole debacle with Air Canada and the bereavement policy that the AI said, yes, just go ahead and claim that it’s bereavement. You can say, within 90 days, and we’ll refund your money. They’re like, your AI said it. Go on, you got to honor it. I think there’s more of that. The regulation has been clearer, especially with some of the newer regulation coming out, saying it has to be a natural person that’s responsible. When it comes to financial services here, at least, as I understand it, it’s the MDs who carry that, which is a lot of risk when you look at it at scale.
Antitrust is an interesting one that I think about, not from the perspective of those implementing AI and trying to create anti-competitive environments for their potential competition. More so for the accessibility of AI as a competitive advantage that a lot of the organizations that we’re seeing that are startup or FinTech or digital native and whatnot, they’re going towards open source, partly because you have the ability to use it without having to spend millions of dollars to do it. It creates actually a more competitive environment to have open-source models and to be able to leverage some of these capabilities, so that if somebody does have a new, disruptive idea that would require the use of AI and require the use of LLMs, there’s a means of entry into that market. I personally think that it’s a helpful tool to have these kinds of open-source models to get new entrants into the market, so that it’s not reliant on this token-based economy of having to pay for a proprietary application for AI.
See more presentations with transcripts