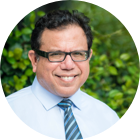
MMS • RSS
Article originally posted on Data Science Central. Visit Data Science Central
Okay, I am weird (tell me something that I don’t know, say most of my friends). For Christmas I wanted a Nike Apple Watch to go with my existing FitBit and Garmin fitness trackers (I look sort of like a cyborg in the photo below…which is always cool).
While I was intrigued by the ability to do all sorts of cool things on the Apple Watch (like take a phone call and talk into my wrist watch like Dick Tracy), the thing that most intrigued me was the ability to buy third-party apps that could yield detailed exercise and health data. I was hoping that this detailed exercise and health data could help me understand what effect particular behaviors or activities (or lack of particular behaviors and activities) were having on my overall health.
Why is this important to me? You can thank articles like “Unexpected Heart Attack Triggers” for my health and exercise anxiety. The article highlighted several things that can trigger a heart attack including:
- Lack of sleep (definitely an issue, especially when I’m traveling so much)
- Migraine Headaches (how can you work in technology and not have headaches)
- Cold Weather (need to find more clients in warmer weather)
- Big, Heavy Meals (with the exception of Chipotle, right?)
- Getting Out of Bed in the Morning (see, I knew that was a big danger!!)
- Alcohol (just like to drink a beer now and then)
- Coffee (I drink Chai Tea Lattes, that’s technically not coffee, and I know that I shouldn’t admit that I drink Chai Tea Lattes)
So there are many items on that above list that could trigger a heart attack, and I enjoy many of the things on that list (like sleeping and eating and the occasional beer). Consequently, I thought I’d put my data science experience to work to monitor my exercise and diet behaviors and predict potential health outcomes.
Personal Fitness Analytics
I tested the downloadable data from each of the three devices. The Fitbit offered the easiest way to download my fitness data (and I have TONS of useful fitness and diet tracking suggestions if anyone at Fitbit, Garmin or Apple ever read this blog!!). The problem with the fitness data is that I can only get daily level data (see Table 1).
Table1: Daily Fitness Tracking Data
I can add more external data to the aggregated fitness data (e.g., days of the week, days when I travel, how much I travel on those travel days) to come up with some simple plots.
For example, Figure 2 shows a visual correlation between the calories that I burn per step and the days that I travel. My assumption is that I burn more calories per step when I am doing something that requires more exertion (like running or climbing steps), so it makes sense that on days when I am traveling, I have less opportunities for highly exertive activities.
Figure2: How Many Calories I Burn Per Step When Traveling
While this information is “interesting,” unfortunately, data at the aggregated daily level is not actionable. If I had more detailed or granular fitness data, I’d like to chart what happens to my heart rate (and related stress levels):
- During an airplane flight
- When racing through an airport to catch a connecting flight
- Waking up very early in the morning while traveling
- Immediately after eating a large meal
- While I’m doing my taxes (I hate doing my taxes)
The problem is that the data provided by my fitness band is aggregated to a level what is not actionable. If I had my fitness data at 5 or 10-minute intervals, then I could more easily spot unusual health outcomes and determine (and eventually predict?) what behaviors (e.g., flying in an airplane, eating large meals, heavy exercise exertion, waking up extremely early) might be causing health concerns.
Power of Granular Data
Big Data and data science are all about granular data because valuable performance and behavioral nuances can be buried in the aggregated data. For example, the chart in Figure 3 shows how additional performance nuances are being uncovered as we transition from a 5-minute to a 1-minute and finally to a 5-second interval in the capture of the performance data.
Figure3: Performance Nuances Uncovered in Granular Data
As the data gets more granular, the behavioral and performance nuances buried in the data start to surface. Data at the 5 minute and 1 minute intervals in Figure 3 tell you very little. Aggregated data is the anti-data science. Data at the 5-second interval highlights some potential performance concerns. In this example, data at the 5-second interval starts to become actionable.
For example, I might notice too sedentary of a heart rate whenever I sit too long on a cross-country flight or my stress level jumping whenever I get another “flight delayed” message while trying to catch a connecting flight. I might then learn to perform some in-seat exercises and walking around during those long flights, or practicing controlled breathing and some simple yoga when enduring yet another flight delay (SFO airport does have a yoga room, and now I know why).
Preparing for an IOT World of Granular Data
Understanding the challenges of capturing and analyzing real-time granular machine and device-generated data will become even more critical as we move into the Internet of Things (IOT), where hundreds of sensors are kicking off tens, hundreds or even thousands of data points per minute. This will force two specific challenges upon those of us coming from the more traditional human-generated big data world:
- Real-time data capture and compression
- Real-time analytics at the edge
For my fitness focus, I might need to expand my Personal Fitness Analysis to capture and analyze more of this detailed data in (near) real-time so that I can become aware of behaviors that are hurting or improving my health and fitness. Ultimately, my goal is to change my behaviors, but I need to understand (and quantify?) what behaviors lead to desirable health and fitness outcomes (e.g., improved blood pressure, lower weight, less stress).