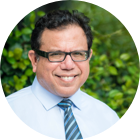
MMS • RSS
Article originally posted on Data Science Central. Visit Data Science Central
Several years ago, my company faced a significant challenge: A large swath of small new entrants relying heavily on data and artificial intelligence provided services faster, cheaper, and more flexibly than we could. They were not slowed down by legacy information systems, archaic business processes, and an outdated workforce. To add insult to injury, the new entrants would use our customer-facing transparency opportunistically to pick up the low hanging fruit, and gradually started to compete with us on our core practices. At the same time, other incumbent market participants had started to innovate.
In this article I would like to share our lessons learned, and discuss how data assets can both be used and should be protected, as to build a defensible competitive data advantage. This is a perspective based on my experience on capital markets and other industries that places innovative technology at the active business foreground as a critical success factor, rather than in a passive support role.
Based on my experience, I believe the questions an organization should be asking are:
- How can we leverage our data with AI?
- How do we build a data moat and protect the flanks?
As noted, a radical shift to data driven business processes was urgently needed in my organization. We had to pioneer new AI-based business processes with and for my teams quickly. We decided to set up a new production centre apart from our existing business and competitors. I decide to hire and manage a dozen persons that specifically had never studied or worked in our industry, to start with fresh and open minds. This team included a high school maths teacher, a statistician, a lawyer, an economist, a farmer, and an accountant. What they had in common was grit and competitiveness.
The above illustration shows some of my team’s lessons learned, and symbolizes how data and AI go hand in hand, wholly depend on each other to add business value, yet should strategically be directed differently. Our business goal is or should be to build on our data assets to drive organizational effectiveness. We aim to accomplish this goal whilst shaping our external data exposure in a manner that enhances product and service offerings yet greatly burdens competitors who try to copy our data, freeload on our platform, or use our public data to pick us off opportunistically.
Looking at how we build on data with AI for internal purposes, three clusters of business value can be identified. I believe leveraging data with AI amounts to making it easy to work with data, automating task execution, and using the data to leverage human talent:
AI Usability
By enhancing ease of access and the interfacing experience with (legacy) information systems, users can spend their time more effectively. Some of these usability systems are colloquially referred to as chatbots. Due to the human-natural language interaction, the user base scope is broadened, as users no longer have to express themselves in a scripted manner as pseudo-programmers. In addition, these systems can help process and present complex information. A practical example of this is how at Watergroep (a water utility) we demonstrated the value of this concept with resource management (cars and meeting rooms) through a native Dutch-speaking chatbot system.
AI automation
Now that a lot of work can be represented through data points, the domain for automation now extends to repetitive knowledge work. At the desktop level this involves automating user interface interactions through so-called robotic process automation tools. Automation is not limited to the digital realm however – for example in logistics robots are replacing human workers. In that setting drones collect visual data, which feeds supply chains and the robots that operate them. On capital markets, the equation was simple: Whereas one human can only manage roughly five repetitive and similar tasks at the same time, one algorithm could manage a virtually infinite amount at the same time – whilst never performing less than average due to a fight with the spouse, working 24/7 without hesitation, nor demanding a hefty bonus. This is why at Goldman Sachs a force of 600 traders could be scaled back to 2, a business process then supported by 200 data engineers.
AI Performance
While a lot of focus in AI has originated from its ability to cut costs, it has also demonstrated its propensity in increasing job effectiveness. This is not just the result of some tasks being automated and the resulting freed up time: The performance-enhancing potential of AI lies in its ability to reduce complexity in data sets for ad-hoc analysis, thereby preventing paralysis by analysis in the big data world. Furthermore, automatic pattern recognition coupled with notification systems can free up cognitive attention so that it can be put to overall better use. An interesting practical (and organic) implementation of such performance-enhancing AI can be found at Codorniu, where the development of the wine grapes needs to carefully controlled for temperature and rainfall influences. There, specific flowers have been selected to grow alongside the ranks that signal through their colours when conditions require intervention or attention through their blooming process. Because of this, the fields do not need to be checked in person as often.
The above three clusters of potential added value through effective use of data helps the organization in making the right decisions quicker whilst expending less resources, thereby enhancing competitive advantage. The question then remains: How do we protect our organization’s external data assets, by providing data transparency in a manner that enhances product and service offerings yet greatly burdens competitors who try to copy our data, freeload on our platform, or use our public data to pick us off opportunistically?
Dynamic Data
While we wish to be transparent to our customers and suppliers, this very same transparency can be abused by competitors that focus on price. By continuously shifting prices regardless of any internal need, we increase the amount of effort that competitors need to expend to keep their prices up to date. While these price-changes can be largely inconsequential to the average customer, being unpredictable markedly increases the amount of resources that competitors need to expend to stay up-to-date. Airlines have largely perfected this dynamic approach to keep each other guessing, although their methods are also geared towards maximizing customer revenue.
Layered Data
In the same way that reality can be layered and multi-faceted, so can your offerings be: On capital markets prices and quantities on offer are rarely firm, as they will change in a fraction of second based on individual orders coming in. An example closer to home for most readers could be a grocer’s discounted offering. Comparing products and prices becomes computationally complex when numerous variables need to be taken into account, such as tailored customer discounts, x-for-y pricing, discount conditions and variable inventory across geography. Algorithms work best when dealing with uniform data with minimal dimensions, so the point to layering is to make comparisons as difficult as possible.
Dense Data
The advent of “big data” tooling was a response to ever-increasing sizes in datasets, so that they became too large and complex to handle with traditional analytical tools and database storage mechanisms. While various solutions have been developed to manage such data and speed up queries, all this big data still needs to be ingested, stored and processed. This still involves a cost. By increasing the amount of data that the organization creates, the “barrier to entry” in required resources to participate on a playing field increases for competitors. There are only so many parties that are able and willing to drink from the data firehose.
In essence, I propose to make it as difficult, expensive, and cumbersome for competitors to collect, process, and store data on your activities, as to hinder their AI. In a world where transparency has become the norm, the new barrier to entry will not be access to data, but having the resources and competence to deal with an ever-increasing data flood.
Conclusion
I feel that the very same digital transformation that I helped pioneer on capital markets is now occuring in a variety of other industries, from agriculture to waste management. While technological progress is inherent to human development, the data and AI wave appears to be different. As a result of ever-increasing data generation, interconnectedness, transparency, in combination with the cost-effective methods to crunch, process, and work with this data, we’ve reached a point where the human cognitive task performance domain is being intruded on by AI.
Looking back at what I did with my AI “skunkworks” teams, I would have put more emphasis sooner on finding the correct incentive systems (gamification of compensation) and would also have focused earlier on aiming for quality in task performance rather than just cost reduction. With my teams I did find a new succesful way of working (and co-existing synergetically) with AI and data, which we referred to as greybox, an aspect of which is highlighted in this article. Regrets and successes aside, I am grateful that this decade of centre-stage exposure to AI has prepared me for driving digital transformations, now that I moved back to the Netherlands after 11 years abroad in various countries, and am helping out customers in a variety of industries.
AI is far from commoditized, so there is ample space and time for having it as your organization’s centre stage to build your competitive data advantage. Incumbents that intervene in time can rely on a healthy financial cushion, an established customer base, execution power through its human resources, and… data. Not just historical data, but also fresh data as generated through daily operations by employees, customers, suppliers, and interactions with competitors. This means that if you act on time you will be in a position to drive change, rather than have it imposed upon you. How will you drive your digital transformation?
About the author
Dr Roger van Daalen is an energetic, entrepreneurial, and highly driven strategic executive with extensive international management experience. He is an expert on industry digital transformations through over a decade of experience with data and artificial intelligence on capital markets and is currently working on transitions to cloud data architectures and applications of machine learning and artificial intelligence more broadly, to pioneer innovative products and services in finance and other industries as to aggressively capture market share and drive revenue growth. Feel free to reach out to him for executive opportunities in the Benelux.
This article was originally posted on LinkedIn