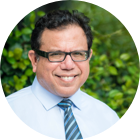
MMS • RSS
Article originally posted on Data Science Central. Visit Data Science Central
Clinical free-text mining
Working with clinical free-text data is not trivial due to several challenges [1]. A minor spelling error can cause a huge difference in meaning; for instance, “Ilium” refers to “the broad, flaring portion of the hip bone, distinct at birth but later becoming fused with the ischium and pubis” [2], whereas “Ileum” represent the “the third and longest portion of the small intestine.”[2] Moreover, clinical abbreviations can cause ambiguity [3]; e.g., PC can mean Pharmaceutical Chemist [4] or Pneumocystis Carinii [5]. In addition, a concept may have different written formats; for instance, falling sickness is an old name for epilepsy [3]. Therefore, data scientists must be more cautious when analyzing clinical free-text data.
References:
1. Menasalvas E, Gonzalo-Martin C. Challenges of Medical Text and Image Processing: Machine Learning Approaches. In: Holzinger A, editor. Machine Learning for Health Informatics: State-of-the-Art and Future Challenges. Cham: Springer International Publishing; 2016. p. 221-42. ISBN: 978-3-319-50478-0.
2. Hazell A. MediLexicon: Pharma-Lexicon International; 2000.
3. Zhu F, Patumcharoenpol P, Zhang C, Yang Y, Chan J, Meechai A, et al. Biomedical text mining and its applications in cancer research. Journal of biomedical informatics. 2013 Apr;46(2):200-11. PMID: 23159498. doi: 10.1016/j.jbi.2012.10.007.
4. Youngson RM. Collins Dictionary of Medicine: HarperCollins; 1992. ISBN: 0004346351, 9780004346359.
5. Stedman TL. Stedman’s medical dictionary for the health professions and nursing: Lippincott Williams & Wilkins; 2005.