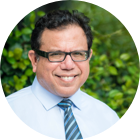
MMS • RSS
Article originally posted on Data Science Central. Visit Data Science Central
Summary: Our recent series of articles on AI strategies shows the options available for the strategic direction of your AI-first company. Here are some thoughts on moving from strategy to implementation, including some useful tools to help in planning.
Hope you’ve been following our latest series of articles describing and comparing the four major strategies for AI-first companies. Now that you’re better equipped to pick a strategy, we offer a few thoughts on moving from strategy to implementation.
To start with, we need to clarify what we mean by AI-first companies. The confusion as usual comes from what exactly you mean by AI.
My favorite quote on this topic:
If you’re talking to a customer it’s AI.
If you’re talking to a VC it’s ML.
If you’re talking to a data scientist it’s statistics.
That about summarizes the lack of clarity around the topic. Basically it depends on who you’re talking to. Personally, when I’m talking to a professional audience of data scientists I go for the more restrictive (and accurate) definition that AI is a subset of ML, the set of techniques based on deep learning and reinforcement learning.
But to make this particular article a little more accessible to the entrepreneurs who may be considering AI-first startups, I’m going to make an exception and use the broader definition (credit Louis Dorard for this particular formulation).
An artificially intelligent product/program is one that makes useful decisions on its own, automatically.
Ok data scientists, if this sounds like prescriptive analytics carried into automated decisioning systems, that’s intentional. In the four major strategies we reviewed, two focused on the narrower technical deep learning/reinforcement learning tools. But we were more persuaded by the two that left open the reality that much can be accomplished, more cheaply and with less complexity with traditional ML techniques. Leaving open the opportunity to expand or enhance those early minimum viable data products with the image, text, and speech capabilities that many of us think of as AI.
AI-First Companies
A quick note about what it means to be an AI-first company. This means starting with the proposition that advanced AI/ML offers routes to solutions that weren’t previously available. And that being AI-first means setting out to exploit exactly those new capabilities, not plastering an AI add-on onto your current offering.
In AI-first we are not giving our customers a general purpose AI/ML tool that they must adapt to solve their problem (horizontal strategy). We are using AI/ML techniques in the technology layer of our more-or-less full stack solution in such a way that it disappears into the plumbing. No data scientist required to operate or interpret results.
I know that having the AI/ML disappear into the infrastructure of a solution may sadden some data scientists. For at least the last decade we’ve been used to having our cool tools and techniques out on full display. But as the field has matured, especially over the last two years, this is just the way it is. The data science is just as important as it’s always been. We just don’t want the customer to have to deal with it.
Some Interesting Planning Tools
First you write the business plan, right? Well, not so much anymore. Business plans are basically linear text documents that record our assumptions and plans. But getting people to read them at all is a challenge, much less participate in the authoring or even keeping up with the changes.
Also, writing a document is probably not the best way for a group of founders with different skills and backgrounds to work out what those assumptions and plans are.
Chances are if you’ve contemplated your own startup you’ve probably encountered canvases, those ubiquitous and seemingly simple diagrams meant to help you work out your proposition on a single page.
These are intended to be communal work spaces (best if blown up to the size of a whiteboard) where a group of planners can discuss and write down their high level assumptions on a ‘single piece of paper’. The benefit of the canvas concept is that the diagram is traditionally divided into all the major categories of things one needs to consider. If you follow through the sections more or less in sequence, you’ll be able to spot the holes and inconsistencies and use it as a discussion guide for planning.
Although we’re going to talk about canvases as tools for planning entire businesses, they are actually equally applicable to planning down to the application level. This might be the case if you’re planning a new AI-based offering from within your current larger company.
The ML Canvas
First there was the Business Model Canvas, then the ever popular Lean Canvas, and now the ML Canvas.
The ML Canvas is the creation of Louis Dorard, an adjunct teaching fellow at the UCL School of Management in London and General Chair at PAPIs.io, the international ML conference. He’s adapted the canvas concept to help you directly with ML/AI startups.
If you spend a minute on the categories Dorard has used you’ll see these are pretty comprehensive as the basis for any AI/ML project.
Like all canvas variations, the center box ‘Goal’ is the starting point. This is where you define the hair-on-fire problem you intend to resolve. Who is the end user, what’s the problem to be solved, why it’s important.
The left side addresses the predictions necessary to solve the problem. The right side is about the required data and how you’ll learn from it.
There are two sections that I thought were particularly well thought out and that’s the split between Offline Evaluation and Live Evaluation.
Ultimately, whether this is to be a traditional ML solution or a deep learning solution, the data science techniques are models and predictions. That’s not the same as reality and all models will have inherent errors, false positives and false negatives.
Since we are making the ML/AI part of the hidden plumbing, the question is how to make our users confident in our results which also means making them aware of this potential for error. Putting trust in our models to correctly make automated business decisions is one of the great and emerging barriers to adoptions.
The Offline Evaluation forces us to think through how we’ll validate the output before we let the solution go live. The Live Evaluation forces us to plan in advance for monitoring and updating the solution once it does go live.
If there’s a shortcoming to the ML Canvas, and it’s only minor, it is that you must deal with one prediction problem at a time. If for example you’re building a comprehensive full stack solution like the predictive maintenance solution described by BCG and reviewed in our previous article, your solution may be based on a series of predictive capabilities, the output of one which may the input to another. Here’s their list of component applications:
- Identifying at-risk component failure prediction.
- Optimizing resource scheduling and staffing.
- Matching technician and Inventory to the maintenance and repair work to be done.
- Ensuring tools and repair equipment availability.
- Ensuring first-time-fix optimization.
- Optimizing parts and MRO inventory.
- Predicting component fixability.
- Optimizing the logistics of parts, tools and technicians.
- Leveraging cohorts analysis to improve service and repair predictability.
- Leveraging event association analysis to determine how weather, economic and special events impact device and machine maintenance and repair needs.
So you’d need a series of ML Canvases and need to keep track of how they nest or layer. That might look in part like this:
You can read much more about Dorard and the ML Canvas here. Curiously the canvas does not explicitly start with a strategy selection but now that you know the alternatives, combine the two to make your own great plan.
Other articles on AI Strategy
Comparing the Four Major AI Strategies
Comparing AI Strategies – Systems of Intelligence
Comparing AI Strategies – Vertical versus Horizontal.
What Makes a Successful AI Company – Data Dominance
AI Strategies – Incremental and Fundamental Improvements
Other articles by Bill Vorhies.
About the author: Bill Vorhies is Editorial Director for Data Science Central and has practiced as a data scientist since 2001. He can be reached at: