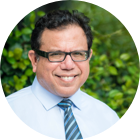
MMS • RSS
Article originally posted on Data Science Central. Visit Data Science Central
This question was recently posted on Quora, and generated a lot of answers. Here is mine:
What differentiates a real doctor from a fake doctor? What about one with no medical degree who cures himself and his family and friends, with better outcomes than official healthcare, for free, and does not call himself a doctor?
Likewise, despite my heavy statistical background (see My data science journey) — Phd, Cambridge PostDoc, numerous publications in top journals, patent owner, former VC-funded data science executive and investor, and 20 years of data science experience in the corporate world at all levels and in all industries, I can say that I disagree with most answers posted by would-be data scientists.
- You can do data science without statistics, read this: Data science without statistics is possible, even desirable
- If you spend 80% of your time cleaning data, you are not a data scientist. Data science is about automating these boring tasks. And automating much more advanced tasks! Everything you learn in a book will eventually be outsourced to robots or automated, be it logistic regression or SVM.
- If you haven’t made at least $150k/year for 5 years in a row doing data science by the time you are 40 years old, then maybe your “data science” activities are not producing the great value you believe they do.
- If you don’t trust your intuition or gut feelings to some extent, either you know your intuition is not reliable (and that’s great to admit it), or you are missing a big part of data science. Data science is a science, and a art. Many calling themselves data scientist today are neither scientists or artists.
- If you don’t have success stories in your portfolio, where you can prove (backed by you CEO and recommendations) that you helped save or generate $1 million in revenue out of data (including finding the right data, asking the right questions to stakeholders to clarify problems in the first place, and get your POC deployed in production mode) then you are not a data scientist. There is no such thing as a junior data scientist.
- Think about this: are you ready to play on the stock market for real, risking your own money, and use “data science” strategies to beat everyone else? You would be playing against very sophisticated data scientists. I did it, also in the real estate market including when everyone got squeezed, and I did not use statistical models, linear or logistic regression in any way to succeed.
- Visualizations are great. Anything that communicates in 30 seconds what others would communicate in months, is like magic.
- In real estate with have this motto: location, location, location. My motto in data science is this: simplicity, simplicity, simplicity. Or: automation, automation, automation.
- If you are searching for the perfect model, just like some people were searching for the philosopher’s stone a while back, you are wasting your time (or trying to protect your job in the short term), and wasting your CEO’s money. Know the 80/20 rule: you can get 95% (I will go as far as to say 110%) of what you can achieve with a simple solution designed in a week, than you could get after spending 2 years on the same project. Do that all the time, it is incredible how much you could accomplish following this rule, and the return you would get — especially, as in my case, you have no boss and all your revenue / added value depends on how efficient you are at generating it.
- You can not successfully design automated data science without significant experience and training. Deploying automated data science is just like climbing Everest solo in winter with no oxygen (it has been done!) What some people call data science nowadays is climbing Everest without any mountain experience, helped by 100 Sherpa and guides who will lift you to the summit even if you are sleeping. That comes with a cost, when it comes to data science: negative ROI, unable to detect fake news, and more.
My 2 cents.
For related articles from the same author, click here or visit www.VincentGranville.com. Follow me on on LinkedIn, or visit my old web page here.
DSC Resources
- Invitation to Join Data Science Central
- Free Book: Applied Stochastic Processes
- Comprehensive Repository of Data Science and ML Resources
- Advanced Machine Learning with Basic Excel
- Difference between ML, Data Science, AI, Deep Learning, and Statistics
- Selected Business Analytics, Data Science and ML articles
- Hire a Data Scientist | Search DSC | Classifieds | Find a Job
- Post a Blog | Forum Questions