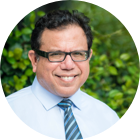
MMS • RSS
Article originally posted on Data Science Central. Visit Data Science Central
Introduction
In a previous post (four quadrants of the Enterprise AI business case) – I laid the foundations of a strategy for deploying Enterprise AI. In this post, we explore how to create an Enterprise AI business case driven by Data. To create this process, I studied the criteria used by VC firms to evaluate AI start-ups and then applied these to deployment of AI in large Enterprises. (VC links/ references included below). The analysis is based on the Enterprise AI workshop in London and remotely
Considerations for Value of AI in the Enterprise
Before we discuss the data considerations for building the AI model, let us first outline the measures of success i.e. how does the AI model create business value
Creation Value by AI is subjective, but a number of considerations apply:
- Is the value reflected in business metrics like conversion, churn and cost savings?
- Do we get significantly improved performance over existing machine learning or rule-based algorithms?
- Does AI improve business processes and thereby create new value?
- Is there a cost benefit in terms of optimizing employee costs?
- Can the AI identify the hidden rules/hierarchy
- Can AI provide near-human or ideally better-than-human, levels of performance?
- Does training data exist? Is it labelled?
- Does the application require a high level of trust (ex: self-driving cars)?
- Does the application need a high level of control? (human intervention as needed)
- Domain complexity – for example the need for extensive feature engineering
- The usage of IoT with AI
- Developing proprietary algorithms
- Impact of regulation on business models including GDPR
- Regulatory transparency – ex explainable AI
- Risk of adoption especially in the non-consumer space. Many existing applications of AI are in the consumer space where the risks are relatively lower (ex chatbots). As AI expands into Enterprise and Healthcare domains, the risk of failure and liability increase substantially.
Data considerations for the Enterprise AI business case
Building on the above considerations, we now consider specific data considerations for building an Enterprise AI business case. “Data is the new oil” is a phrase commonly used but Oil is scarce – and (not all) data is scarce. So, the analogy does not directly apply. However, Crude oil is less valuable. In that sense, the analogy does apply i.e. raw data is less valuable (ex: aggregators like Nielsen are valued less than companies that build products on Data and Algorithms – such as Netflix).
A better analogy is that of a Data moat. More generally, at least the following considerations for Data apply to building a business case for Enterprise AI based on a defensive (Data moat) strategy
- Data availability (ideally accessed by an API)
- Data readiness validated through Data quality rules
- Data governance – supported by Governance rules
- Model governance – delivered through an API / container and refreshed
- The idea of Vertical AI comprising Full stack products, Subject matter expertise, Proprietary data, AI delivers core value
- Performance threshold and the minimum performance of the algorithm
- Stability threshold and data decay – Machine learning models train on examples taken from the real-world environment they represent. If conditions change over time, gradually or suddenly, and the model doesn’t change with it, the model will decay. In other words, the model’s predictions will no longer be reliable.
- Data dimensions: Accessibility; Time to acquire’ Cost to acquire including licenses; uniqueness; Dimensionality of the data;
- Cost of inclusion of all edge cases
- Perishability of data
- Virtuous loop – access to data to improve the algorithm over time
- Prediction horizon: Algorithms making predictions with long time horizons are difficult to evaluate and improve. Most algorithms model dynamic systems and return a prediction for a human to act on.
- Hire people to train the algorithms, either as full=time employees or via mechanical Turk
- Deployment scalability –
- Impact of confounding variables – In statistics, a confounding variable is a variable that influences both the dependent variable and independent variable causing a spurious association. Confounding is a causal concept, and as such, cannot be described in terms of correlations or associations. Models trained on only co-related variables (vs predictive variables) are likely to be impacted by the presence of confounding variables downstream.
- Cost of data integration from data in different formats
- Cost of data integration arising from data in various sources
Conclusions
Data is a key component of the Enterprise AI business case. But as we see above, not all data is created equal -and a number of Data components play a role in the Enterprise AI business case. The analysis is based on the Enterprise AI workshop in London and remotely
References
the-mmc-ventures-ai-investment-framework
ai-entrepreneurs-3-things-to-check
ai-adoption-is-limited-by-incurred-risk-not-potential-benefit/
data-rights-are-the-new-ip-rights/