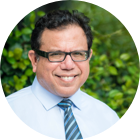
MMS • RSS
Article originally posted on Data Science Central. Visit Data Science Central
On the surface, preventing injuries to professional-caliber athletes would seem to have little in common with preventing operational failures for a machine (i.e., autonomous vehicle, locomotive, airplane, CT Scan). However, both athletes and machines deal with inter-twined complex systems (where the interactions of one complex system can have a ripple effect on others) that can have significant impact on their operational effectiveness.
My son Max, the Director of Sport Science at Resilient Code and Chief Science Officer at Exsurgo Technologies, has turned into quite an analytics nerd (check him out on twitter at @strong_science, but he saves his good stuff for Instagram where you can follow him at “strong_by_science”). Max and Resilient Code co-founder Dr. Dustin Nabhan have been educating me on the use of Agent-based Models (ABM) as a technique to predict and prevent injuries in athletes, especially high-caliber professional athletes. Unlike the weekend warrior like myself, preventing career-ending injuries can translate into tens of millions of dollars of additional income for professional athletes[1].
Here is the conversation with Max that got me thinking more about the similarities between ABM and Digital Twins (Max’s input is in drab, boring grey and mine is in cool, hip blue):
Okay, probably a boring conversation for most families, but now you know what we talk about when we go out to eat! Yea, you don’t wanna sit next to us…
Understanding Agent-based Models (ABM)
During these conversations with Max and Dustin, I was struck by the similarities in using ABM to prevent injuries in the same way that we use Digital Twins (or what we call Asset Avatars) to prevent machine breakdowns, failures, under-performance and unplanned downtime. Let’s start with an overview of Agent-based Models (ABM).
Agent-based models (ABM) are comprised of sub-system models that simulate the simultaneous operations and interactions of multiple agents in an attempt to re-create and predict the performance of complex phenomena. The ABM approach originates from lower (micro) level sub-systems that inter-twine to create a more complex (macro) entity. A key tenet of ABM is that the combination of simple behavioral rules can be used to predict the behavior of complex systems.
Figure 1: “Agent-Based Modeling of Human-Induced Spread of Invasive Species in Agricultural Landscapes
Another central tenet of ABM is that the whole is greater than the sum of the parts. Individual agents are typically characterized as boundedly rational, presumed to be acting in what they perceive as their own interests, such as reproduction, economic benefit, or social status, using heuristics or simple decision-making rules. ABM agents may experience “learning”, adaptation, and reproduction.
Max describes (in detail) how Resilient Code is applying ABM to prevent sports injuries:
“By tying ABM into a cellular constraints model representing an athlete’s load-response profile, a coach and team can begin to look at specific inferential metrics that represent the innate regulatory sub-systems. In an oddly similar way to the HBO hit show “Westworld”, the goal would be to understand the subsystems’ regulatory responses and digitalize how an athlete or human responds an external stressors.
This process of such modeling would hinge on an adequate quantification of external loading data (GPS, game loads, HR response, number of sets and reps, etc.) and then a response profile of subsystems that are required to deal with and adapt to the external load (HRV response, reaction time, jump height, heart rate response to a given load, salivary pH and predispositions) in conjunction with dynamic regulatory filters, which act as either amplifiers or dimmers (diet, sleep, rest, recovery modalities, etc.).
With enough load-response samples (each game/practice/training session/day of life is a sample), the individual can be mapped out. The quantification of external loading for a general human at first appears to be a farfetched idea, but once one understands how often we are being actively tracked (Phone GPS and FitBit), the concept seems uncomfortably plausible. Using the concept of cellular constraints and outcomes (adapt, maintain or degrade) one can begin to map out subsystem response patterns, while at the same time, begin to quantify biological strain (the overall internal demands of the body when dealing with a stressor), which would be an eventual indicator or adaptive potential and general health and wellness.
The development an agent-based model for human beings would then allow for proactive modeling to take place. Thus, ideally not only preventing an injury, which is arguably an external manifestation of a subsystem failure, but also begin to better understand general health and diseases, which is arguably synonymous with the idea of a subsystem failure, like that of an injury, but within a different domain.”
Introducing the Role of the Asset Avatars (Digital Twins)
An Asset Avatar (Digital Twin) is a digital representation of an industrial asset that enables companies to better understand and predict the performance of their machines, find new revenue streams, and change the way their business operates.
In the world of Industrial IoT (IIoT), the Asset Avatar is the foundation for IoT monetization. Digital Twins / Asset Avatars are the economic value and monetization drivers tied to high-value operational use cases. It is around these Asset Avatars that organizations will build intelligent IoT applications such as predictive maintenance, inventory optimization, quality assurance and supply chain optimization (see Figure 2).
Figure 2: Role of Asset Avatars to Power Key Operational IoT Applications
I have written frequently about Digital Twins and the importance of their role in driving IoT success. Check out these blogs from more details:
- Me, Myself and Digital Twins
https://www.linkedin.com/pulse/me-myself-digital-twins-bill-schmarzo/ - Cohort Analysis in the Age of Digital Twins
https://www.linkedin.com/pulse/cohort-analysis-age-digital-twins-bill-schmarzo/
Summary
ABM can be used for multiple athletic use cases: from evaluating talent and potential, to optimizing training and in-sport performance, to preventing injuries and extending careers. And in the true sense, it is optimizing the economics of professional sports management.
In my role as CTO of IoT and Analytics at Hitachi Vantara, I too am looking to optimize the economics of IoT. I will continue to explore the integration (collusion) of Asset Avatars, Analytic Modules, Data Science (Machine Learning and AI), Digital Economics and Design Thinking to drive an organization’s IoT strategy. Or maybe it’s better put this way:
Organizations do not need an IoT strategy; they need a business strategy that exploits the business and operational potential of IoT
Now that’s more like it!
Sources:
“Complexity frontiers, Artificial Intelligence and Agent-Based Modeling”
https://www.datasciencecentral.com/profiles/blogs/complexity-frontiers-artificial-intelligence-and-agent-based
“Agent-based modeling and simulation tutorial – EASSS 2009 – Giuseppe Vizzari”
https://www.slideshare.net/giuseppevizzari/agentbased-modeling-and-simulation-tutorial-easss-2009-giuseppe-vizzari
“Towards a complex systems approach in sports injury research: simulating running-related injury development with agent-based modelling” https://bjsm.bmj.com/content/early/2018/06/18/bjsports-2017-098871
“Dealing with injury: A look at the process involved in treating injured athletes and getting them back on the field”
https://www.sportsbusinessdaily.com/Journal/Issues/2012/04/23/In-Depth.aspx
[1]Sports history is full of examples of professional careers cut short by injuries. The article “The 25 Greatest Sports Careers Ruined by Injury” profiles professional athletes – Bo Jackson, Greg Oden, Danny Manning, Ralph Sampson, Grant Hill, Terrell Davis, Earl Campbell and my personal favorite Pete Maravich – who lost tens of millions of dollars of income due to sports injuries.