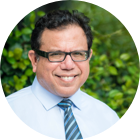
MMS • RSS
Article originally posted on Data Science Central. Visit Data Science Central
Recommendations and Feedbacks
The vast majority of B2C services are quickly discovering the strategic importance of solid recommendation engines to improve the conversion rates and an establish a stronger fidelity with the customers. The most common strategies are based [3] on the segmentation of users according to their personal features (age range, gender, interests, social interactions, and so on) or to the ratings they gave to specific items. The latter approach normally relies on explicit feedbacks (e.g. a rating from 0 to 10) which summarize the overall experience. Unfortunately, there are drawbacks to both cases.
Personal data are becoming harder to retrieve and the latest regulations (i.e. GDPR) allow the user to interact with a service without the collection of data. Moreover, a reliable personal profile must be built using many attributes that are often hidden and can only be inferred using predictive models. Conversely, implicit feedbacks are easy to collect but they are extremely scarce. Strategies based on the factorization of the user-item matrix [3] are easy to implement (considering the possibility to employ parallelized algorithms), but the discrepancy between the number of ratings and number of products is normally too high so to allow an accurate estimation.
Our experience showed that it’s possible to create very accurate user profiles (also for an anonymous user identified only by a random ID) exploiting the implicit feedbacks present in textual reviews. Our main assumption is that reviews which are classified as spam reflect the wishes of a user with respect to a specific experience. In other words, a simple sentence like “The place was very noisy” implies that I prefer quiet places or, adding extra constraints, that, in a specific situation (characterized by n factors), a quiet place is preferable than a noisy one. The approach we are going to present is based on this hypothesis and can be adapted in order to cope with many different scenarios.