Month: April 2024
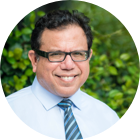
MMS • RSS
Posted on mongodb google news. Visit mongodb google news
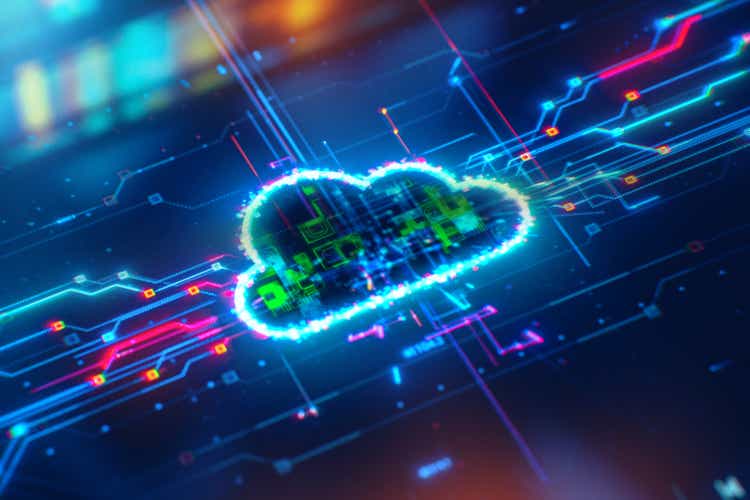
da-kuk/E+ via Getty Images
The strength reported by Microsoft (MSFT) and Google (GOOG) (GOOGL) in their respective cloud computing units last week could have positive implications for the broader cloud computing software industry as a whole, investment firm Baird said.
“Strong data points to kick off earnings season from both Azure and Google Cloud, which should be directionally positive for our cloud data leaders – SNOW, MDB, DDOG and DT – boosting those stocks to end the week,” analysts at the investment firm said, referencing Snowflake (NYSE:SNOW), MongoDB (NASDAQ:MDB), Datadog (NASDAQ:DDOG) and Dynatrace (NYSE:DT).
Snowflake was up 0.8% in mid-day trading, while MongoDB, Datadog and Dynatrace fell at least 1% each.
A consensus of analysts expects Seattle-based Amazon to earn $0.83 on revenue of $142.56B in sales when it reports on April 30, implying a rise of 11.9% during the quarter.
Investors will also look into capital expense for the quarter, especially after the company said it anticipates spending for 2024 to increase year-over-year, primarily driven by increased infrastructure to support AWS growth and additional investments in generative AI among others.
MongoDB is slated to host an investor event on May 2, followed by Datadog, which will discuss its first-quarter results on May 7. Snowflake is set to discuss its first-quarter results of fiscal 2025 on May 22.
Dynatrace has not yet set a date, according to its investor relations website.
More on cloud computing
Article originally posted on mongodb google news. Visit mongodb google news
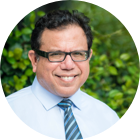
MMS • RSS
Posted on mongodb google news. Visit mongodb google news
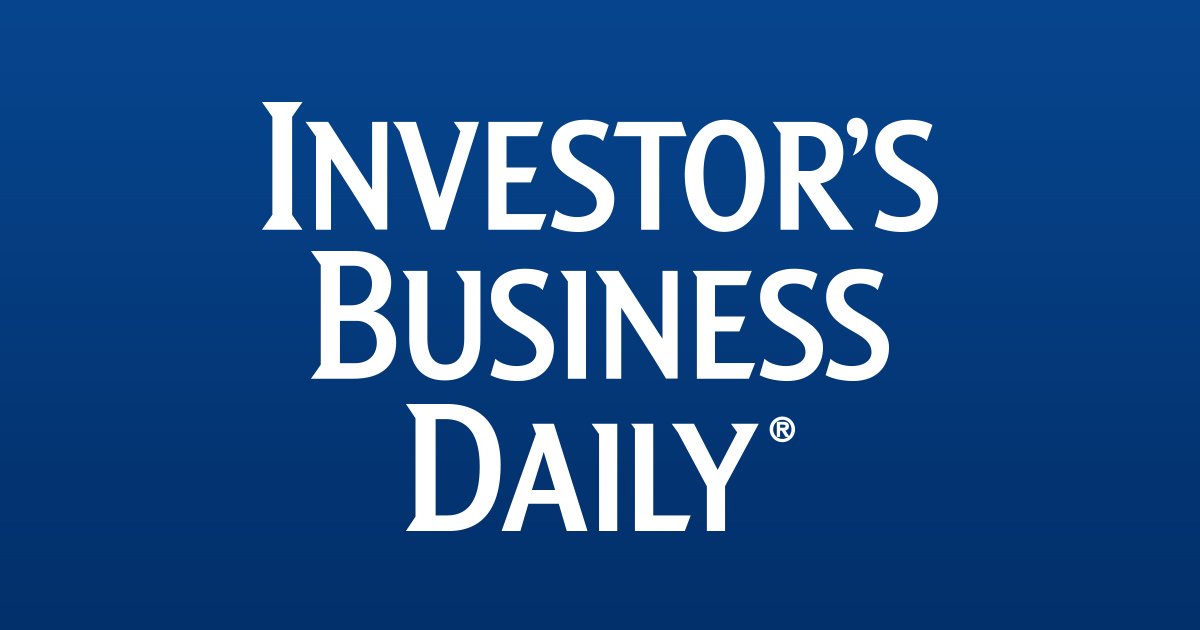
On Monday, MongoDB (MDB) received an upgrade to its Relative Strength (RS) Rating, from 65 to 75.
X
This proprietary rating measures technical performance by showing how a stock’s price action over the last 52 weeks measures up against that of the other stocks in our database.
Decades of market research reveals that the market’s biggest winners often have an 80 or better RS Rating as they begin their largest runs. See if MongoDB can continue to show renewed price strength and clear that threshold.
How To Invest In Stocks In Both Bull And Bear Markets
While now is not an ideal time to invest, see if the stock is able to form a chart pattern and break out.
MongoDB showed 51% EPS growth last quarter. Revenue rose 27%.
MongoDB earns the No. 4 rank among its peers in the Computer Software-Database industry group. Elastic (ESTC) is the top-ranked stock within the group.
RELATED:
IBD Stock Rating Upgrades: Rising Relative Strength
Why Should You Use IBD’s Relative Strength Rating?
How Relative Strength Line Can Help You Judge A Stock
Ready To Grow Your Investing Skills? Join An IBD Meetup Group!
Article originally posted on mongodb google news. Visit mongodb google news
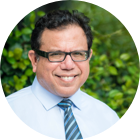
MMS • RSS
Posted on mongodb google news. Visit mongodb google news
Key Insights
- The projected fair value for MongoDB is US$386 based on 2 Stage Free Cash Flow to Equity
- With US$384 share price, MongoDB appears to be trading close to its estimated fair value
- Analyst price target for MDB is US$447, which is 16% above our fair value estimate
How far off is MongoDB, Inc. (NASDAQ:MDB) from its intrinsic value? Using the most recent financial data, we’ll take a look at whether the stock is fairly priced by projecting its future cash flows and then discounting them to today’s value. One way to achieve this is by employing the Discounted Cash Flow (DCF) model. Believe it or not, it’s not too difficult to follow, as you’ll see from our example!
We generally believe that a company’s value is the present value of all of the cash it will generate in the future. However, a DCF is just one valuation metric among many, and it is not without flaws. If you still have some burning questions about this type of valuation, take a look at the Simply Wall St analysis model.
View our latest analysis for MongoDB
Crunching The Numbers
We’re using the 2-stage growth model, which simply means we take in account two stages of company’s growth. In the initial period the company may have a higher growth rate and the second stage is usually assumed to have a stable growth rate. To begin with, we have to get estimates of the next ten years of cash flows. Where possible we use analyst estimates, but when these aren’t available we extrapolate the previous free cash flow (FCF) from the last estimate or reported value. We assume companies with shrinking free cash flow will slow their rate of shrinkage, and that companies with growing free cash flow will see their growth rate slow, over this period. We do this to reflect that growth tends to slow more in the early years than it does in later years.
Generally we assume that a dollar today is more valuable than a dollar in the future, so we discount the value of these future cash flows to their estimated value in today’s dollars:
10-year free cash flow (FCF) estimate
2024 | 2025 | 2026 | 2027 | 2028 | 2029 | 2030 | 2031 | 2032 | 2033 | |
Levered FCF ($, Millions) | US$103.0m | US$131.1m | US$235.1m | US$591.8m | US$713.0m | US$1.11b | US$1.42b | US$1.71b | US$1.97b | US$2.19b |
Growth Rate Estimate Source | Analyst x13 | Analyst x15 | Analyst x16 | Analyst x6 | Analyst x4 | Analyst x3 | Est @ 28.43% | Est @ 20.58% | Est @ 15.10% | Est @ 11.25% |
Present Value ($, Millions) Discounted @ 7.3% | US$96.0 | US$114 | US$190 | US$446 | US$501 | US$724 | US$866 | US$973 | US$1.0k | US$1.1k |
(“Est” = FCF growth rate estimated by Simply Wall St)
Present Value of 10-year Cash Flow (PVCF) = US$6.0b
We now need to calculate the Terminal Value, which accounts for all the future cash flows after this ten year period. For a number of reasons a very conservative growth rate is used that cannot exceed that of a country’s GDP growth. In this case we have used the 5-year average of the 10-year government bond yield (2.3%) to estimate future growth. In the same way as with the 10-year ‘growth’ period, we discount future cash flows to today’s value, using a cost of equity of 7.3%.
Terminal Value (TV)= FCF2033 × (1 + g) ÷ (r – g) = US$2.2b× (1 + 2.3%) ÷ (7.3%– 2.3%) = US$45b
Present Value of Terminal Value (PVTV)= TV / (1 + r)10= US$45b÷ ( 1 + 7.3%)10= US$22b
The total value, or equity value, is then the sum of the present value of the future cash flows, which in this case is US$28b. In the final step we divide the equity value by the number of shares outstanding. Compared to the current share price of US$384, the company appears about fair value at a 0.5% discount to where the stock price trades currently. Remember though, that this is just an approximate valuation, and like any complex formula – garbage in, garbage out.
Important Assumptions
Now the most important inputs to a discounted cash flow are the discount rate, and of course, the actual cash flows. You don’t have to agree with these inputs, I recommend redoing the calculations yourself and playing with them. The DCF also does not consider the possible cyclicality of an industry, or a company’s future capital requirements, so it does not give a full picture of a company’s potential performance. Given that we are looking at MongoDB as potential shareholders, the cost of equity is used as the discount rate, rather than the cost of capital (or weighted average cost of capital, WACC) which accounts for debt. In this calculation we’ve used 7.3%, which is based on a levered beta of 1.092. Beta is a measure of a stock’s volatility, compared to the market as a whole. We get our beta from the industry average beta of globally comparable companies, with an imposed limit between 0.8 and 2.0, which is a reasonable range for a stable business.
SWOT Analysis for MongoDB
- Cash in surplus of total debt.
- Shareholders have been diluted in the past year.
- Has sufficient cash runway for more than 3 years based on current free cash flows.
- Current share price is below our estimate of fair value.
- Debt is not well covered by operating cash flow.
Next Steps:
Valuation is only one side of the coin in terms of building your investment thesis, and it ideally won’t be the sole piece of analysis you scrutinize for a company. DCF models are not the be-all and end-all of investment valuation. Preferably you’d apply different cases and assumptions and see how they would impact the company’s valuation. If a company grows at a different rate, or if its cost of equity or risk free rate changes sharply, the output can look very different. For MongoDB, we’ve compiled three important elements you should consider:
- Risks: Consider for instance, the ever-present spectre of investment risk. We’ve identified 2 warning signs with MongoDB , and understanding these should be part of your investment process.
- Future Earnings: How does MDB’s growth rate compare to its peers and the wider market? Dig deeper into the analyst consensus number for the upcoming years by interacting with our free analyst growth expectation chart.
- Other High Quality Alternatives: Do you like a good all-rounder? Explore our interactive list of high quality stocks to get an idea of what else is out there you may be missing!
PS. The Simply Wall St app conducts a discounted cash flow valuation for every stock on the NASDAQGM every day. If you want to find the calculation for other stocks just search here.
Valuation is complex, but we’re helping make it simple.
Find out whether MongoDB is potentially over or undervalued by checking out our comprehensive analysis, which includes fair value estimates, risks and warnings, dividends, insider transactions and financial health.
Have feedback on this article? Concerned about the content? Get in touch with us directly. Alternatively, email editorial-team (at) simplywallst.com.
This article by Simply Wall St is general in nature. We provide commentary based on historical data and analyst forecasts only using an unbiased methodology and our articles are not intended to be financial advice. It does not constitute a recommendation to buy or sell any stock, and does not take account of your objectives, or your financial situation. We aim to bring you long-term focused analysis driven by fundamental data. Note that our analysis may not factor in the latest price-sensitive company announcements or qualitative material. Simply Wall St has no position in any stocks mentioned.
Article originally posted on mongodb google news. Visit mongodb google news
Java News Roundup: WildFly 32, JEPs Proposed to Target for JDK 23, Hibernate 6.5, JobRunr 7.1
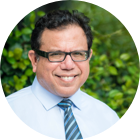
MMS • Michael Redlich
Article originally posted on InfoQ. Visit InfoQ
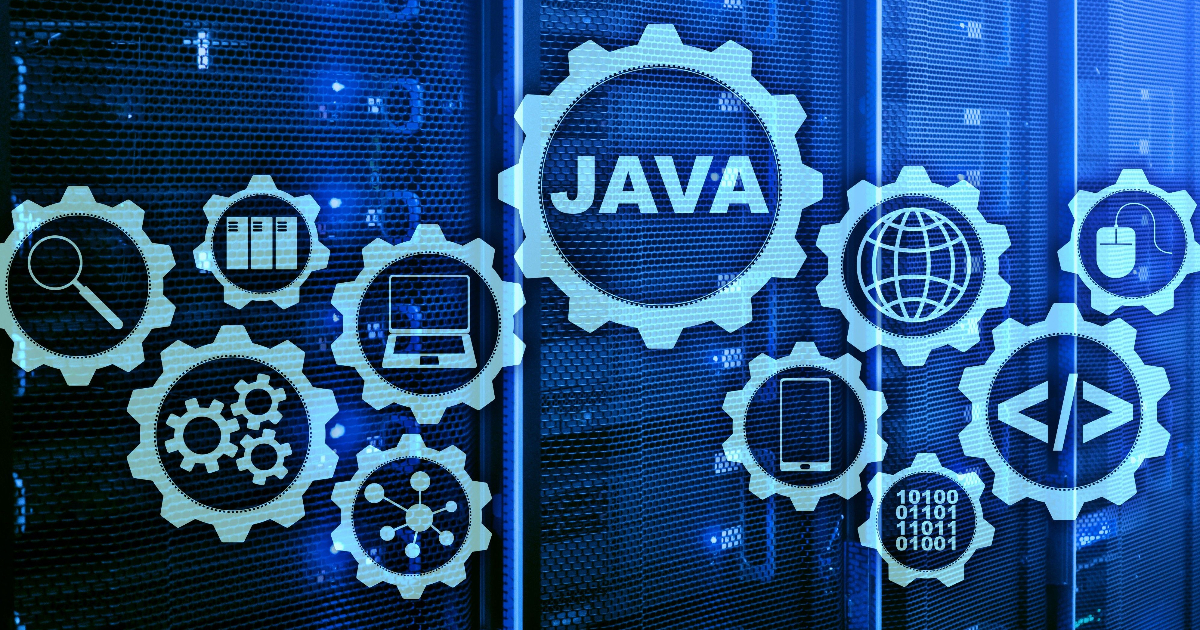
This week’s Java roundup for April 22nd, 2024 features news highlighting: the release of WildFly 32; JEP 476, Module Import Declarations (Preview), JEP 474, ZGC: Generational Mode by Default, and JEP 467, Markdown Documentation Comments, proposed to target for JDK 23; Hibernate ORM 6.5; and JobRunr 7.1.
OpenJDK
One week after having been declared a candidate, JEP 476, Module Import Declarations (Preview), has been promoted from Candidate to Proposed to Target for JDK 23. This preview feature proposes to enhance the Java programming language with the ability to succinctly import all of the packages exported by a module with a goal to simplify the reuse of modular libraries without requiring to import code to be in a module itself. The review is expected to conclude on May 1, 2024.
JEP 474, ZGC: Generational Mode by Default, has also been promoted from Candidate to Proposed to Target for JDK 23. This JEP proposes to use the Z Garbage Collector (ZGC) from non-generational to generational mode by default. The non-generational mode will be deprecated and removed in a future JDK release. This will ultimately reduce the cost of maintaining the two modes such that future development can primarily focus on JEP 439, Generational ZGC. The review is expected to conclude on April 30, 2024. InfoQ will follow up with a more detailed news story.
JEP 467, Markdown Documentation Comments, has been promoted from Candidate to Proposed to Target for JDK 23. This feature proposes to enable JavaDoc documentation comments to be written in Markdown rather than a mix of HTML and JavaDoc @
tags. This will allow for documentation comments that are easier to write and easier to read in source form. The review is expected to conclude on May 4, 2024. InfoQ will follow up with a more detailed news story.
JDK 23
Build 20 of the JDK 23 early-access builds was made available this past week featuring updates from Build 19 that include fixes for various issues. Further details on this release may be found in the release notes.
BellSoft
BellSoft has released versions 24.0.1 for JDK 22, 23.1.3 for JDK 21 and 23.0.4 for JDK 17 of their Liberica Native Image Kit builds as part of the Oracle Critical Patch Update for April 2024 to address several security and bug fixes. A total of 10 CVEs have been resolved. These include: CVE-2023-41993, a vulnerability in which processing web content may lead to arbitrary code execution; and CVE-2024-21085, a vulnerability in which an unauthenticated attacker, with network access via multiple protocols, can compromise Oracle Java SE and Oracle GraalVM Enterprise Edition resulting in the unauthorized ability to cause a partial denial of service.
Spring Framework
Versions 3.3.0-M1 3.2.4 and 3.1.11 of Spring Shell have been released featuring notable resolutions to issues such as: use of the GridView
class with zero column/row sizes causing an item to be placed into the bottom-right when user expects it to be in the top-left; and a race condition and resulting ConcurrentModificationException
, primarily seen on WindowsOS, from the TerminalUI
class when updating the screen. These releases build on Spring Boot 3.3.0-RC1, 3.2.5 and 3.1.11, respectively. More details on this release may be found in the release notes for version 3.3.0-M1, version 3.2.4 and version 3.1.11.
WildFly
The release of WildFly 32 features the version 1.0 release of WildFly Glow, a set of command-line provisioning tools that analyzes deployments and identifies the set of Galleon feature-packs and Galleon layers that are required by applications. Along with bug fixes and dependency upgrades, other new features include: support for the Jakarta MVC 2.1 specification; support for an instance of the Java SSLContext
class that can dynamically delegate to different SSL contexts based on the destination’s host and port; and the ability to create channels defining component versions used to provision WildFly using the WildFly Channel project that may be separately maintained from WildFly’s feature packs. Further details on this release may be found in the release notes. InfoQ will follow up with a more detailed news story.
Micronaut
The Micronaut Foundation has released version 4.4.1 of the Micronaut Framework featuring Micronaut Core 4.4.6, bug fixes, improvements in documentation, and updates to modules: Micronaut Views, Micronaut gRPC, Micronaut Test Resources and Micronaut Maven Plugin. More details on this release may be found in the release notes.
Open Liberty
IBM has released version 24.0.0.4 of Open Liberty featuring: support for JDK 22; and updates to eight (8) Open Liberty guides to use the MicroProfile Reactive Messaging 3.0, MicroProfile 6.1 and Jakarta EE 10 specifications. There were also security fixes for: CVE-2023-51775, a vulnerability in the Javascript Object Signing and Encryption for Java (jose4j
component) before version 0.9.4 that allows an attacker to cause a denial of service via a large PBES2 value; and CVE-2024-27270, a cross-site scripting vulnerability in IBM WebSphere Application Server Liberty 23.0.0.3 through 24.0.0.3 that allows an attacker to embed arbitrary JavaScript code in a specially crafted URI.
Helidon
The release of Helidon 4.0.8 ships with notable changes such as: support for a span event listener with a new SpanListener
interface for improved tracing callbacks; and the use of delegation instead of inheritance from the Java BufferedOutputStream
class to ensure the use of virtual thread-friendly locks in the JDK code and avoids thread pinning due to synchronized blocks in the JDK. Further details on this release may be found in the changelog.
Hibernate
The release of Hibernate ORM 6.5.0.Final delivers new features such as: Java time objects marshaled directly through the JDBC driver as defined by JDBC 4.2 to replace the use of java.sql.Date
, java.sql.Time
or java.sql.Timestamp
classes; a configurable query cache layout to minimize higher memory consumption from storing the full data in the cache; and support for Java records as a parameter in the Jakarta Persistence @IdClass
annotation; and support for auto-enabled filters. This release also includes a technical preview of the Jakarta Data specification that will be included in the upcoming release of Jakarta EE 11.
Apache Software Foundation
The release of Apache Camel 4.4.2 provides bug fixes, dependency upgrades and improvements such as: the ability to set the error handler on the route level to complement the existing error handler on the global lever in the Camel YAML DSL component; and support for the restConfiguration
property in the Camel XML IO DSL component. More details on this release may be found in the release notes.
Similarly, version 4.0.5 of Apache Camel has also been released with bug fixes, dependency upgrades and improvement such as: a resolution to the PubSubApiConsumer
class failing to load the POJO
enum, defined in PubSubDeserializeType
, on some platforms in the Camel Salesforce component; and a more robust way to obtain the correlationID
for brokers in the Camel JMS component. Further details on this release may be found in the release notes.
JobRunr
Version 7.1.0 of JobRunr, a library for background processing in Java that is distributed and backed by persistent storage, has been released to deliver bug fixes, dependency upgrades and new features such as: support for virtual threads when using GraalVM Native mode; and improved initialization of the BackgroundJobServer
class in Spring with improved support for JSR 310, Date and Time API. More details on this release may be found in the release notes.
Details on the new features of JobRunr 7.0.0, released on April 9, 2024 may be found in this webinar hosted by Ron Dehuysser, creator of JobRunr.
JDKUpdater
Versions 14.0.39+69 of JDKUpdater, a new utility that provides developers the ability to keep track of updates related to builds of OpenJDK and GraalVM. Introduced in mid-March by Gerrit Grunwald, principal engineer at Azul, this new release includes: a resolution to an issue related to the latest download view closing problem; and the ability to open the latest version download view from notification. Further details on this release may be found in the release notes.
TornadoVM
TornadoVM has announced that SAPMachine, a downstream distribution of OpenJDK maintained by SAP, has been added to their TornadoVM Installer utility. This complements the existing downstream distributions, namely: Oracle OpenJDK, Amazon Corretto, GraalVM and Mandrel.
Gradle
The first release candidate of Gradle 8.8 delivers: full support for JDK 22; a preview feature to configure the Gradle daemon JVM using toolchains; improved IDE performance with large projects; and improvements to build authoring, error and warning messages, the build cache, and the configuration cache. More details on this release may be found in the release notes.
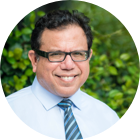
MMS • Sergio De Simone
Article originally posted on InfoQ. Visit InfoQ
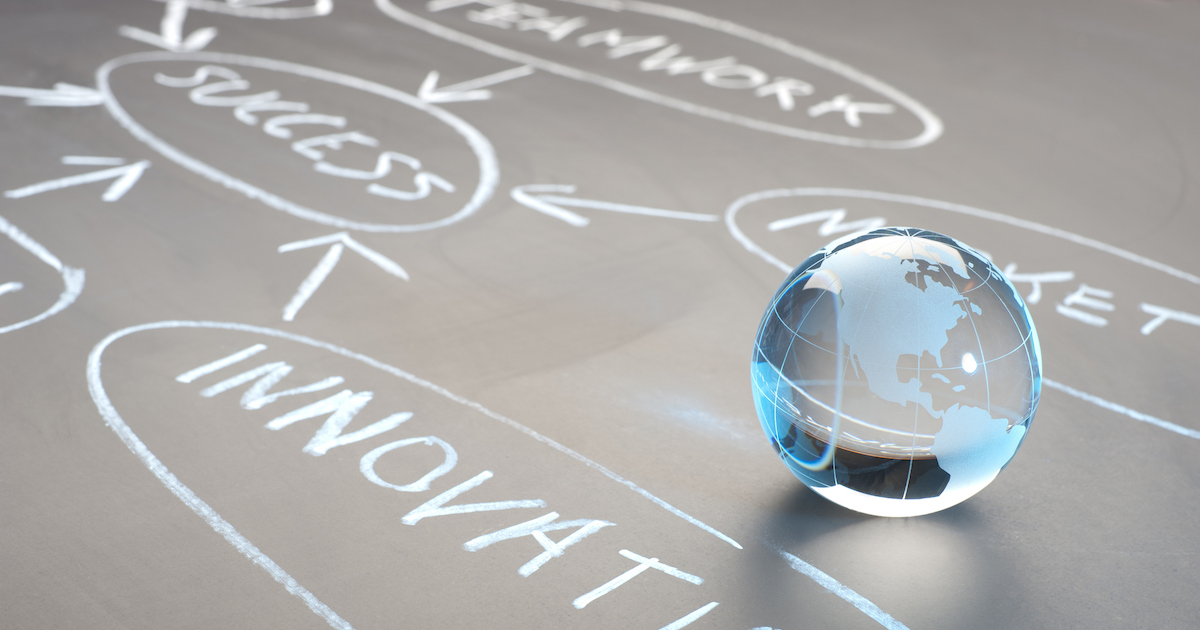
Opening up the operating system that powers its Meta Quest devices to third-party hardware makers, Meta aims to create a larger ecosystem and make it easier for developers to create apps for larger audiences.
Dubbed Meta Horizon OS, the operating system combines mixed reality features with others focusing on social presence. The OS supports eye, face, hand, and body tracking to enable more natural interaction and social presence. It makes it possible to move people’s identities, avatars, and friend groups across virtual spaces and devices, meaning that people can be in virtual worlds that exist across mixed reality, mobile, and desktop devices.
Additionally, it supports more mixed reality-oriented features aimed to blend the digital and physical worlds such as high-resolution Passthrough, Scene Understanding, and Spatial Anchors.
Developers and creators can take advantage of all these technologies using the custom frameworks and tooling we’ve built for creating mixed reality experiences, and they can reach their communities and grow their businesses through the content discovery and monetization platforms built into the OS.
Meta sees its Horizon OS as a key enabling factor for the existence of a variety of specialized devices that better serve customers’ diverse interests in categories such as gaming, entertainment, fitness, productivity, and social presence. Examples of companies that are already building new devices based on Horizon OS include ASUS’s Republic of Games, Lenovo, and Xbox, Meta says.
At the same time, Meta is making it easier for developers to ship software on the platform, specifically by including titles featured on the App Lab in the official Meta Store. The App Lab was originally introduced to allow developers to distribute apps directly to consumers safely and securely, without requiring store approval and without sideloading.
App Lab titles will soon be featured in a dedicated section of the Store on all our devices, making them more discoverable to larger audiences. Some of the most popular apps on the Store today, like Gorilla Tag and Gym Class, began on App Lab.
On the tooling side, Meta began previewing a new spatial app framework to help developers create mixed-reality experiences.
At the hardware level, Horizon OS is tightly tied to the Snapdragon processors that power Meta Quest devices and companies building products using it are expected to use the same hardware and software stack as Meta itself.
Commenting on Meta’s announcement, John Carmack shared his view about what it could entail:
What it CAN do is enable a variety of high end “boutique” headsets, as you get with Varjo / Pimax / Bigscreen on SteamVR. Push on resolution, push on field of view, push on comfort. […] You could add crazy cooling systems and overclock everything. All with full app compatibility, but at higher price points. That would be great!
However, he maintains, “VR is held back more by software than hardware” and the effort required for Meta’s engineers to prepare the system and maintain good communication with partners is likely to slow down the further development of the system.
Other commenters chimed in, following Carmack’s take, to highlight a different scenario where Meta’s announcement could lead to the creation of a new space for VR devices, similarly to what Google achieved launching the Android platform. And almost naturally, when talk is about Meta, it’s hard not to find remarks about privacy, in this case regarding the collection of users’ gaze tracking data.
MongoDB CEO Dev Ittycheria talks AI hype and the database evolution … – Yahoo Lifestyle Canada
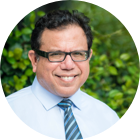
MMS • RSS
Posted on mongodb google news. Visit mongodb google news
A lot has happened since Dev Ittycheria took the reins at MongoDB, the $26 billion database company he’s led as president and CEO since September 2014. Ittycheria has taken MongoDB to the cloud, steered it through an IPO, overseen its transition from open source, launched a venture capital arm, and grown the customer base from a few hundred to something approaching 50,000.
“When I joined the company, it wasn’t clear if people would trust us to be a truly mission-critical technology,” Ittycheria told TechCrunch. “When I joined, it was doing roughly $30 million in revenue; now we’re doing close to $2 billion.”
It hasn’t all been peaches and cream, though. Five months ago, MongoDB was hit by a security breach, which, while relatively contained, did momentarily risk its reputation in an industry where reputation is paramount.
Throw into the mix the whirlwind AI revolution that has engulfed just about every industry, and there was much to discuss when TechCrunch sat down with Ittycheria at MongoDB’s new London office, which opened in Blackfriars last year.
MongoDB’s London office. Image Credits: Paul Sawers / TechCrunch
Vector’s embrace
Databases have come a long way since IBM and Oracle first popularized relational databases more than half a century ago. The internet’s rise created demand for flexible, scalable, and cost-effective data storage and processing, paving the way for businesses such as MongoDB to thrive.
Founded in 2007 by a trio of veterans hailing from online adtech company DoubleClick (which Google acquired for $3.1 billion), MongoDB was initially called 10Gen until a rebrand to the name of its flagship product six years later. It has since emerged as one of the preeminent NoSQL databases, helping companies store and manage large volumes of data.
Prior to joining MongoDB, Ittycheria founded and exited a server automation company called BladeLogic for $900 million in 2008, and went on to serve in various board member and investor roles (including a 16-month stint at Greylock) before joining MongoDB as president and CEO coming on for 10 years ago now. Ittycheria replaced Max Schireson, who stepped down for family reasons after just 18 months in the role.
Built on a document-oriented model, MongoDB has grown off the back of the explosion in mobile and web applications where flexible, dynamic data structures are at play. The current artificial intelligence wave is driving a similar shift, with vector databases the hot new thing in town.
Like NoSQL, vector databases also specialize in unstructured data types (e.g., images, videos, social media posts), but are particularly well suited to large language models (LLMs) and generative AI. This is due to the way they store and process data in the form of vector embeddings, which convert data into numerical representations that capture relationships between different data points by storing them spatially by relevance. This makes it easier to retrieve semantically similar data and allows AI to better understand context and semantics within conversations.
While a slew of dedicated vector database startups have emerged these past few years, the incumbents have also started embracing vector, including Elastic, Redis, OpenSearch, Cassandra, and Oracle. Cloud hyperscalers, including Microsoft, Amazon, and Google have also ramped up support for vector search.
MongoDB, for its part, introduced vector search to its flagship database-as-a-service product Atlas last June, a sign that the company was preparing for the oncoming AI tsunami. This mimics other historical trends where single-function databases emerge (such as time-series) with some utility as stand-alone solutions but that might also be better integrated into a larger multi-purpose database stack. This is precisely why MongoDB introduced support for time-series databases a few years back, and why it’s doing the same with vector.
“A lot of these companies are features masking as products,” Ittycheria said of the new wave of dedicated vector products. “We built that into the platform, and that’s the value — rather using some stand-alone vector database and then your OLTP [online transaction processing] database and then your search database, we can combine all three things into one platform that makes the life of a developer and architect so much easier.”
The idea is that database providers that adopt a multipronged approach can combine all the data in one place, making life easier for developers to work with.
“There’s probably like 17 different types of databases, and probably about 300 vendors,” Ittycheria said. “There’s no customer on this planet that wants to have 17 different databases. The complexity that creates, and the cost of learning, supporting and managing those different technologies becomes overwhelming. It also inhibits innovation, because it creates this tax of complexity.”
MongoDB’s Dev Ittycheria. Image Credits: MongoDB
Too much hype
Despite the preparation, Ittycheria reckons there is too much hype around AI — for now, at least.
“My life has not been transformed by AI,” he said. “Yes, maybe I can write an email better through all those assistants, but it’s not fundamentally transformed my life. Whereas the internet has completely transformed my life.”
The theory is that despite the hullaballoo, it will take time for AI to seep into our everyday lives — and when it does, it will be through applications integrating AI, and businesses building on it.
“I think with the adoption of any new technology, we see value accrue at the bottom layer first,” Ittycheria said. “Obviously, Nvidia is making money hand over fist, and OpenAI has been the most talked about company since they launched ChatGPT. But the real value will come when people build applications on top of those technologies. And that’s the business we’re in — we’re in the business of helping people build applications.”
For now, it’s all about “simple apps,” as Ittycheria puts it. This includes chatbots for customer service, something that MongoDB itself is doing internally with CoachGTM, powered by MongoDB’s vector search, to bring its sales and customer teams instant knowledge about their products. In some ways, we’re currently in the “calculator apps” stage that the iPhone found itself in nearly 20 years ago when the concept of the App Store hit the masses.
“The real sophisticated [AI] apps will be using real-time data, being able to make real-time decisions on real-time events,” Ittycheria said. “Maybe something’s happening in the stock market, maybe it’s time to buy or sell, or it’s time to hedge. I think that’s where we will start seeing much more sophisticated apps, where you can embed real-time data along with all the reasoning.”
The SaaS path
One of the biggest developments during Ittycheria’s tenure has been the transition from a self-deployed model, where customers host MongoDB themselves and the company sells them features and services. With the launch of Atlas in 2016, MongoDB embarked on the familiar SaaS path where companies charge for removing all the complexities of self-hosting. At the time of its IPO the following year, Atlas represented 2% of MongoDB’s revenue — today that figure sits at nearly 70%.
“It’s grown very quickly, and we’ve really built that business as a public company,” Ittycheria said. “What the popularity of Atlas showed was that people are comfortable consuming infrastructure as a service. What that allows them to do is delegate what they consider ‘non-strategic functions,’ like provisioning, configuring and managing MongoDB. So they can focus on building applications that are really transforming their business.”
Another major development came when, a year after going public, MongoDB moved away from an open source AGPL license to a source-available SSPL (server side public license). In some ways, this was the bellwether of what was to come, with countless infrastructure companies going on to abandon their open source credentials to prevent the cloud giants (e.g., Amazon) from selling their own version of the service without giving back.
“We feel very happy about it [the license change],” Ittycheria said. “The reality is that while it was open source, 99.9% of the development is done by our own people — it’s not like communities contributing code. It’s not some simple, trivial application — it’s very complex code, and we need to hire senior, talented people who cost a lot of money. We didn’t think it was fair for us to spend all this money to build this product, then someone takes that free product, monetizes it, and not give us anything back. It was quite controversial in 2018, but looking back, our business has only grown faster.”
And grown it has. As with just about every tech company, MongoDB’s valuation soared during the pandemic, peaking at an all-time high of $39 billion in late 2021, before plummeting south of $10 billion within a year — roughly the same as its pre-pandemic figure.
However, MongoDB’s shares have been in ascendency in the 18 months since, hitting $35 billion just a couple of months ago, before dropping again to around $26 billion today — such is the volatile nature of the stock markets. But given the company’s relatively modest $1.8 billion valuation at the end of its first day of trading in 2017, MongoDB has performed fairly well for public market investors.
Dev Ittycheria with MongoDB colleagues at its 2017 IPO. Image Credits: MongoDB
Four months ago, though, MongoDB revealed a data breach that exposed “some customer account metadata and contact information” — it involved a phishing attack through a third-party enterprise tool (Ittycheria wouldn’t confirm which). This caused its shares to drop 3%, but in the months that followed, MongoDB’s valuation surged back to a two-year high. This highlighted how little impact the breach had on affairs at the company, certainly compared to high-profile data breaches at the likes of Equifax and Target, which hit the businesses hard and forced senior executive departures.
While MongoDB’s cybersecurity incident was significantly smaller in scope, what stood out was how quickly the whole thing went away — it was reported in several outlets (including TechCrunch), but the story disappeared into the foggy ruins of time just as quickly as it arrived.
“Part of the reason is that we were very transparent,” Ittycheria said. “The last thing you want to do is hide information and appear like you’re misrepresenting information. We have lots of banks who put a lot of very sensitive information in our data platform; we’ve lots of other companies that have a lot of sensitive information. So for us, it’s really about making sure that our architecture is robust and sound. And this really forced us to double down. I would never claim that we’re never gonna get hacked again, but we’re doing everything in our power to ensure that it doesn’t.”
Nothing ventured
It’s not unusual for the biggest tech companies to launch their own investment vehicles, as we’ve seen through the years with Alphabet (which has several investment offshoots), Microsoft, Amazon, and Salesforce all ingratiating themselves with the startup fraternity. But a newer wave of enterprise corporate venture firms have entered the fray, too, including Slack, Workday, Twilio, Zoom, HubSpot, and Okta.
In 2022, it was MongoDB’s turn to launch such a fund, and in the two years since, MongoDB Ventures has invested in some eight companies.
“This is for us to build deeper relationships — we work in an ecosystem that consists of large companies and also small companies,” Ittycheria said. “Where we see a small company that we think could be interesting to work with, we say, ‘Hey, we want a chance to invest in you,’ so that extra value’s created. We also are the beneficiaries of creating some of that value.”
MongoDB only has a handful of people in its corporate development team that are mostly focused on the venture fund, and Ittycheria stresses that MongoDB takes a back seat with its investments. It also typically invests alongside other VCs, as it did with its inaugural investment in 2021 (predating the formal launch of its fund), when it quietly joined the likes of Insight Partners and Andreessen Horowitz in Apollo GraphQL’s $130 million Series D round.
“We always take a minority position, we don’t take a board seat, and we don’t set the terms,” Ittycheria said. “But the reason startups are interested in us is because they want to leverage the MongoDB brand. We have thousands of people in the field, so they [startups] can leverage our distribution channels.”
Article originally posted on mongodb google news. Visit mongodb google news
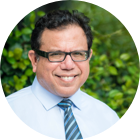
MMS • Claudio Masolo
Article originally posted on InfoQ. Visit InfoQ
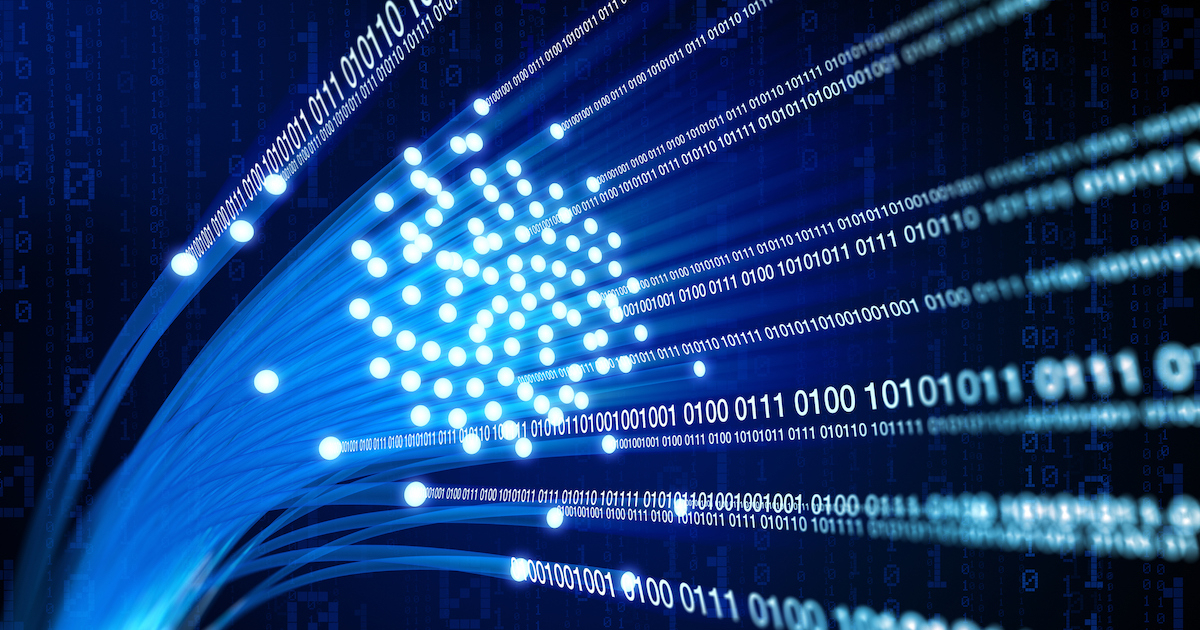
RedHat has announced the general availability of Red Hat OpenShift 4.15, based on Kubernetes 1.28 and CRI-O 1.28. Red Hat OpenShift is an application platform that allows developers and DevOps to build, and deploy applications.
This release introduces some changes for application build like the Red Hat Developer Hub operator, the Integration of role-based access controls (RBAC) via the web interface, the migration to the new Backstage backend system, and the support for viewing installed plugins through the web interface and the compatibility with Azure Kubernetes Services (AKS) and Elastic Kubernetes Services (EKS) for installation.
Red Hat OpenShift Local lets users set up a mini OpenShift cluster on their local machine. This mimics a cloud development environment, providing all the tools you need to build container-based applications.
The versions 2.31 of OpenShift Local, built upon OpenShift 4.14.7, Podman 4.4.4, and MicroShift 4.14.7, benefit from several enhancements:
- Windows GUI Launcher: A new helper tool (win32-background-launcher) simplifies starting OpenShift Local as a background service on Windows machines.
- Bug Fixes: This update addresses issues encountered during daemon initialization.
- Admin Helper Update: The admin-helper tool has been upgraded to version 0.5.2.
- Libvirt Driver Update: OpenShift Local 2.32 uses an updated
crc-libvirt-driver
(version 0.13.7).
Red Hat OpenShift Dev Spaces offers a web-based development environment tailored for Red Hat OpenShift. The latest release: Red Hat OpenShift Dev Spaces 3.11, built on Eclipse Che 7.80 and compatible with OpenShift versions 4.11 to 4.14, empowers cluster admins with enhanced access management capabilities and supports Microsoft Visual Studio Code extensions utilizing OAuth2 authorization code flow. Moreover, it defaults to Java 17 in the universal developer image and streamlines workspace configuration for various Git providers. Similarly, Red Hat OpenShift Dev Spaces 3.12, based on Eclipse Che 7.82, extends the collaborative environment by enabling the sharing of certificates, secrets, and configuration files across users. This release also enhances flexibility by allowing the override of the editor’s image using dedicated URL parameters and introduces support for running Che-Code editor in the Red Hat Universal Base Image 9. Explore the Dev Spaces v3.12 release notes for detailed insights.
The OpenShift Toolkit IDE extension by Red Hat enhances the development experience by facilitating code deployment to OpenShift directly from Visual Studio Code and IntelliJ. This extension integrates seamlessly with OpenShift Serverless Functions, improves Helm Chart deployment, and offers convenient access to Developer Sandbox environments from within the IDE. Explore the extension’s features to boost productivity in your development workflow.
OpenShift Serverless, providing autoscaling and networking for containerized microservices and functions, unveils version 1.32, featuring Knative 1.11. This release introduces Knative Eventing monitoring dashboards, on-cluster function building for IBM P/Z, and custom CA bundle injection for system components. Additionally, it offers new capabilities in technology preview, including Go language support for Serverless Functions and enhanced trigger filters for Event-driven apps. Dive into the release notes for a comprehensive overview of its features.
Red Hat’s migration toolkit for applications 7.0 offers support for multiple languages, enhanced rules syntax, automated classification, and dynamic reporting capabilities. Container images for OpenShift now include Node.js 20, while Java 21 builder and runtime container images are also available. Quarkus 3.8 will introduce Redis 7.2 support, Java 21 support, Arm native support, and OpenSearch Dev services. Additionally, Spring Boot 3.1.x and 3.2.x have been tested and verified for runtimes on OpenShift.
Red Hat OpenShift GitOps allows administrators to configure and deploy Kubernetes infrastructure and applications across various clusters and development life cycles. With the release of OpenShift GitOps 1.11, leveraging Argo CD 2.9.2, users gained access to dynamic shard rebalancing in Technology Preview mode alongside other new capabilities. In the subsequent release, OpenShift GitOps 1.12, built on Argo CD 2.10, users can enjoy several additions, including introducing an Argo CD CLI in technology preview. For an extensive overview of all the new features, refer to the “What’s new in Red Hat OpenShift GitOps 1.12” documentation.
OpenShift Pipelines stands as a cloud-native continuous integration and continuous delivery (CI/CD) solution rooted in Kubernetes, streamlining deployments across multiple platforms by abstracting away implementation complexities. The latest version, Openshift Pipelines 1.14.0, powered by Tekton 0.56, introduces a new web console plugin in technology preview, enabling users to visualize pipeline and task execution statistics. Additionally, it embraces Pipeline as a Code methodology to interface with multiple GitHub instances.
OpenShift Service Mesh 2.5, built on Istio 1.18 and Kiali 1.73, users can expect a host of enhancements:
- General Availability support for Arm clusters, broadening compatibility.
- Expanded observability integrations with the inclusion of Zipkin, OpenTelemetry, and envoyOtelAls extension providers.
- General Availability status for the OpenShift Service Mesh Console plug-in, enhancing management capabilities.
- Developer Preview features including IPv4/IPv6 dual-stack support and the Kiali Backstage plug-in for Red Hat Developer Hub.
- Updated Developer Preview of the Service Mesh 3 Kubernetes Operator (or the Sail Operator), offering improved functionality.
The Red Hat build of OpenTelemetry, based on the OpenTelemetry framework, simplifies telemetry data collection for cloud-native software. Version 3.1 (based on OpenTelemetry 0.93.0) introduces support for the target allocator in the OpenTelemetry Collector. This feature optimizes Prometheus receiver scrape target allocation across deployed OpenTelemetry Collector instances and integrates seamlessly with Prometheus PodMonitor and ServiceMonitor custom resources.
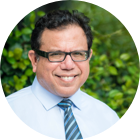
MMS • Renato Losio
Article originally posted on InfoQ. Visit InfoQ
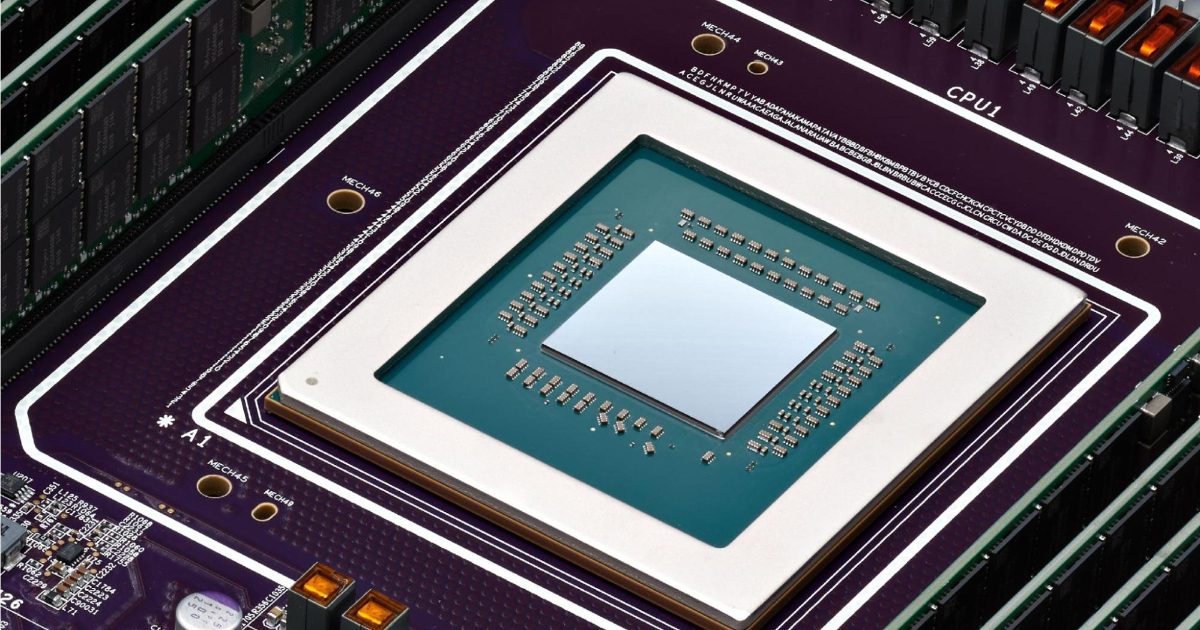
During the recent Google Next ’24 conference, Google unveiled Axion, its first custom Arm-based CPUs designed for data centers. Utilizing the Arm Neoverse V2 CPU architecture, the new processor will be available to customers later this year.
According to the cloud provider, Axion will deliver 30% better performance than the fastest general-purpose Arm-based instances available in the cloud. Additionally, it promises up to 50% better performance and up to 60% better energy efficiency than comparable current-generation x86-based instances. Amin Vahdat, engineering fellow and VP at Google, writes:
Axion is underpinned by Titanium, a system of purpose-built custom silicon microcontrollers and tiered scale-out offloads. Titanium offloads take care of platform operations like networking and security, so Axion processors have more capacity and improved performance for customer workloads. Titanium also offloads storage I/O processing to Hyperdisk, our new block storage service that decouples performance from instance size and that can be dynamically provisioned in real time.
Even if Google claims “giant leaps in performance for general-purpose workloads” no details or benchmarks have been provided. Google is the latest of the cloud hyperscalers developing an Arm-based custom processor, with Axion now competing with AWS Graviton, already at its fourth generation, and the recently announced Microsoft Cobalt. Matthew Kimball, technology analyst, writes:
So, the three largest cloud providers (AWS, Microsoft Azure, Google Cloud) have all become first-party silicon designers. And of course, Oracle Cloud is utilizing Ampere at scale. If you had any doubts about Arm’s standing in the datacenter CPU market – put them to rest.
Axion is built on the standard Armv9 architecture and instruction set and Google promises that customers will be able to use Axion in many managed services including Compute Engine, Kubernetes Engine, Dataproc, Dataflow, and Cloud Batch. Vahdat adds:
Google also has a rich history of contributions to the Arm ecosystem. We built and open sourced Android, Kubernetes, Tensorflow and the Go language, and worked closely with Arm and industry partners to optimize them for the Arm architecture.
On Hacker News, user soulbadguy comments:
Another interesting challenge for intel (and AMD to a lesser extent). Between the share of compute moving to AI accelerator (and NVIDIA), and most cloud providers having in-house custom chips, I wonder how Intel will position itself in the next 5 to 10 years.
Virtual machines based on Axion processors will only be available in preview in the coming months. User mtmail adds:
Eight testimonials and it’s clear the companies haven’t been able to test anything yet.
While the new processors are not yet available to customers, Google claims that several internal services, including BigTable, Spanner, BigQuery, Blobstore, Pub/Sub, Google Earth Engine, and the YouTube Ads platform, have already been migrated to Arm-based servers. No pricing information has been provided.
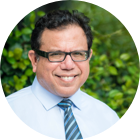
MMS • Sergio De Simone
Article originally posted on InfoQ. Visit InfoQ
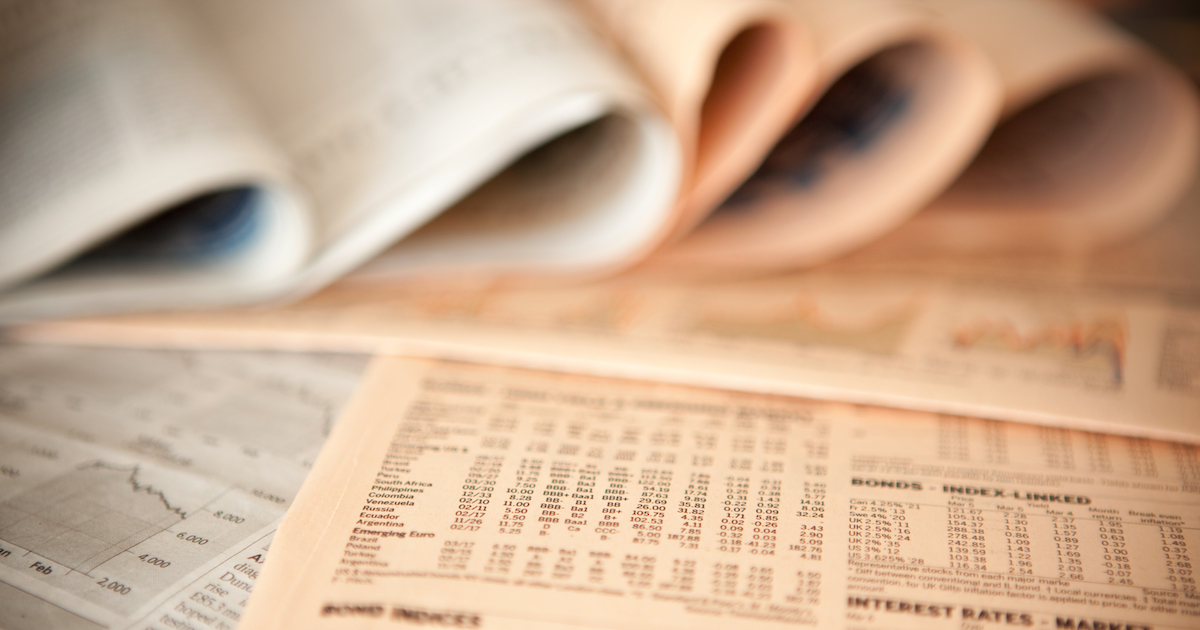
JetBrains IDE Services aims to help enterprises manage their JetBrains tool ecosystem more efficiently and boost developer productivity at the enterprise scale through the integration of AI, remote collaboration, and more.
JetBrains IDE Services is a centralized suite of tools, including IDE Provisioner, AI Enterprise, License Vault, Code With Me Enterprise, and CodeCanvas. JetBrains says it is now making it generally available after testing it in beta with several large JetBrains customers and addressing their feedback.
IDE Provisioner makes it easier to centralize IDE management across versions, configurations, and plugins and helps avoid unapproved or outdated versions being used within the organization. IDE Provisioner also supports a private plugin repository where you can configure which plugins are publicly available and which are only visible to authenticated users.
AI Enterprise gives enterprises control over security, spending, and efficiency for AI-driven features such as code generation and task automation, and allows them to choose the best-in-class LLM provider.
License Vault aims to automate the distribution of licenses for JetBrains IDEs across the entire organization. It supports three licensing models: pre-paid, fully postpaid, and mixed IDE licensing. Additionally, it offers the option of using floating licenses, which are released to the pool of available licenses after 20 minutes of no use.
Code With Me Enterprise offers real-time collaboration solutions for developers with a focus on security for remote workers. It supports pair programming in either full-sync or follow mode; includes a teacher-student scenario; and allows up to five coders to edit the same file simultaneously.
Finally, CodeCanvas is a self-hosted remote development environment orchestrator aiming to simplify the setup and management of dev environments.
IDE Services consists of three components: the IDE Services Server, the Toolbox App, and a plugin for IntelliJ-based IDEs.
The IDE Services Server is available as a Docker image and provides the core functionality that can be run using Docker Compose or Kubernetes. The Toolbox App, available for Windows, macOS, and Linux, is installed on developer machines and is used to download, update, and configure IntelliJ-based IDEs. The plugin for IntelliJ-based IDEs has three main capabilities: receiving the IDE builds recommended by the organization, including settings and plugins; getting access to pre-approved plugins; and setting up secure collaboration sessions for Code With Me Enterprise.
As a final note, JetBrains IDE Services also provides a REST API which enables running all essential operations on the IDE Services Server, JetBrains says.
Presentation: Sleeping at Scale – Delivering 10k Timers per Second per Node with Rust, Tokio, Kafka, and Scylla
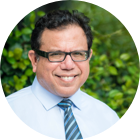
MMS • Lily Mara Hunter Laine
Article originally posted on InfoQ. Visit InfoQ
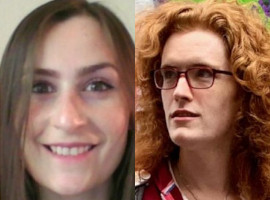
Transcript
Mara: My name is Lily Mara. I’m an engineering manager at a software company here in the Bay Area called OneSignal. We are a customer messaging company. We help millions of application developers and marketers connect with billions of users. We send around 13 billion push notifications, emails, in-app messages, and SMS every single day. I started using Rust professionally in 2016. I started using it as my main daily programming language professionally in 2019, when I started at OneSignal. I’m the author of the book “Refactoring to Rust” that is available now in early access at manning.com.
Outline
What are we going to be talking about? We’re going to be talking about how we built this scalable, high throughput timer system at OneSignal. We’re going to be talking about the motivation behind building it, in the first place. The architecture of the system itself. How the system performed. How we scaled it up, and some future work that we are maybe thinking about doing in the system.
Building a Scalable, High Throughput Timer System (OneSignal)
Let’s jump back in time a little bit. It’s 2019, I’ve just started at OneSignal. I’ve just moved to sunny California from horrible, not sunny Ohio. Everybody in OneSignal, everybody in the customer messaging sphere is talking about the concept of journey builders. If you’re not a content marketer, you might not know what a journey builder is, so just a quick crash course. This is a no-code system that allows marketers to build out customer messaging flows. This is a flowchart that’s going to be applied at a per user level. This is very similar to what a marketer might put together themselves. Over here on the left, every user is going to start over here. There’s, immediately going to be dumped into a decision node. We allow our customers to store arbitrary key-value pairs at the user level. In this case, a customer might be storing what’s your favorite color as one of those key-value pairs. We’ll make a decision based on that. Let’s say for the sake of argument that this user’s favorite color was blue, we’re going to send that user a push notification that says, “There’s a 20% off sale on blue shirts, we know how much you love blue. Aren’t you so excited about the blue shirt sale?” Then the next thing that’s going to happen is we are going to wait 24 hours. We’re going to do nothing for a day. This was the missing piece. We thought we understood how to build the event system, we understood how to build the node walking tree, but we didn’t have a primitive in place in our network for scheduling, for storing a bunch of timers and expiring them performantly. This is what we’re going to be building today. After we do that 24 hour wait, we are going to have another decision node. We’re going to say, did that particular user click on that particular push notification we sent them? If they did, we will say, “Mission accomplished. Good job, journey. You did the engagement.” If they didn’t, we’ll send them an SMS that has more or less the same message. You might want to do this because sending an SMS has a monetary cost associated with it. Carriers will bill you for sending SMS. Twilio will bill you for sending SMS. Push notifications have basically zero marginal cost. If you want to use SMS, you might want to use it as a second-order messaging system to get in contact with your customers. That’s a journey builder. That’s what we’re going to try to enable the construction of today.
What are the requirements on this timer system that we’re going to build? We want to be able to store billions of concurrent timers because we want to be able to support the billions of user records that we already have. We want to be able to expire those timers performantly. We want to minimize data loss because, of course, we don’t want to be dropping timers on the floor. We don’t want people to get stuck in particular nodes of their journeys. We want to integrate well with the rest of the data systems that we have at OneSignal. We’re a relatively small company. We don’t have the resources to have a team for every particular data system that’s out there. We don’t want to adopt 50 completely different open source data systems. Let’s get in the headspace a little bit. We realized very crucially that if we wanted to build a timer, we had to think like a timer. We took the project and we set it down for a year, and we didn’t think about it that hard. We prioritized other initiatives.
Jumping forward once again, we’re in the beginning of 2021. We have the resources to start investigating this project. We have to make a decision, are we going to build something completely from scratch ourselves, or are we going to buy an off-the-shelf system? Is there an open source timer, an open source scheduling system that we can just use? The first place we looked were generic open source queuing systems like Sidekiq and RabbitMQ. We already operate Sidekiq very heavily at OneSignal. It’s the core of our delivery API, and a lot of our lower throughput scheduling jobs. These general-purpose queuing systems, they had a lot of features that we didn’t really need, we didn’t want to pay to have to operate. They were lacking in the area that was the most important to us, and that was performance. We didn’t think that these systems were going to scale up to the throughput that we were expecting this timer system to have. The published performance numbers for things like RabbitMQ just seemed orders of magnitude off from what we wanted out of this system. We knew from experience what Sidekiq was like to scale, and we didn’t think that was going to be good enough. Based on the published numbers, we thought that other things weren’t going to be good enough. We opted to build something for ourselves, we’re going all the way.
Existing Systems (OneSignal)
Once again, let’s look at these requirements. We want to be able to store tons of timers, expire them performantly, minimize data loss, and interoperate with the rest of our system. Let’s talk about the rest of the systems. What is the prior art for system design at OneSignal? We have a lot of things written in Rust. We actually currently, but not at the time, had a policy around only using Rust for new systems. At the time, we were also spinning up new services in Go. We use Apache Kafka very heavily for our async messaging applications. We also use gRPC for all of our internal synchronous RPCs. Also, as a part of the larger journeys’ initiative, we were going to be using Scylla, which is a C++ rewrite of Apache Cassandra.
Ins & Outs
Let’s talk about the big blocks, how the data are flowing in and out of the system. Probably, sensibly, the input to the system is a timer. A timer is an expiry time, a time to end that, and a thing to do once it has ended an action. What are the actions? Initially, we came up with a pretty short list that is sending a message like a notification, email, SMS, in-app message, or it’s adding a tag to a particular user. We realized that we might come up with more actions in the future so we didn’t necessarily want to constrain ourselves to a fixed list. We also realized we might come up with actions that had absolutely nothing to do with journeys, absolutely nothing to do with messaging. That this timer system, this scheduler system has a broad range of applicability, and we wanted to leave the door open for us to take advantage of that. We imposed a new requirement on ourselves, we said we wanted this system to be generic. Given that this system is going to be generic, what is an action? What does it look like? How does it work? In addition to being generic, we wanted it to be simple. That meant constraining what an action is, even though it’s flexible.
We didn’t want to give people a whole templating engine or scripting library, or something like that. We wanted it to be pretty straightforward, you give us these bits, and we will ship them off when the time comes. Are we going to let people send HTTP requests with JSON payloads? All of our internal services use gRPC, so probably not that. Maybe they’ll be gRPC requests then. Both of these systems suffer from the same, in our mind, critical flaw, in that these are both synchronous systems. We thought it was really important that the outputs of the timer system be themselves asynchronous. Why is that? As timers start to expire, if there’s multiple systems that are being interfaced with, say notification delivery and adding key-value pairs. If one of those systems is down, or is timing out requests, we don’t want, A, to have to design our own queuing independence layer in the timer system, or, B, have our request queues get filled up with requests for that one failing system, to the detriment of the other well behaving systems. We wanted the output of this to be asynchronous. We opted to use Apache Kafka, which as I mentioned, is already used very heavily at OneSignal. We already have a lot of in-house knowledge and expertise on how to operate and scale Kafka workloads. It gave us a general-purpose queuing system that was high performance. A really key benefit is it meant that the timer system was isolated from the performance of the end action system. What about these inputs? What about the timers themselves? Because all the timers are being written into the same place by us, we can own the latency of those writes, so this can be a synchronous gRPC call.
Interface
The interface, broadly speaking, looks like this. External customers make a gRPC request to the timer system. They’re going to give us an expiry time, and they’re going to give us an action, which is a Kafka topic, Kafka partition, and some bytes to write onto the Kafka stream. Then later on, when that timer expires, we’re going to write that Kafka message to the place that the customer has specified. We’ll delve into the magic box of the timer system as we go through this. Hopefully, later on, presumably at some point, the team that enqueue the timer will have a Kafka Consumer that picks up the message and acts on it. The really important thing here is the consumer picking up the message and acting on it is totally isolated from the timer system, dequeuing the message and shipping it across the wire. If a Kafka Consumer is down or not performant, that really has nothing to do with the timer system. It’s not impacting us at all.
Internals
Let’s delve into the internals of this a little bit. How are we going to store timers after they come across the gRPC interface? How are we going to expire timers to Kafka after they’ve been stored? What are the key health metrics of the system? Let’s get into it. I’d first like to talk about the timer expiry process. We know we’re going to be writing timers to Kafka. We know there’s a gRPC service on the other side that has some storage medium attached to it for these timers. We want to try to build something that’s relatively simple, relatively straightforward for pulling these things out of storage and expiring them to Kafka. How are we going to do this? We came up with this architecture as our first pass. We’re still abstracting the storage away. We have our gRPC service, we’ve added a couple new endpoints to it. There’s a get timers endpoint that takes in a timestamp, and it’s going to serve us back all the timers that expire before this timestamp. Every minute, this new scheduler box over here, that’s a scheduling service written in Rust, it’s going to send a gRPC request up to our service. It’s going to say, give me all the timers that expire before 10 minutes into the future. If it’s currently 4:48, we’re going to say, give me all the timers that expire before 4:58. It’s going to take all those timers, write them into a pending area in memory, and have some in-memory timers. Then it’s going to expire those out to Apache Kafka as those timers expire. Once they are expired and shipped off to Kafka, we are going to send a delete request up to the gRPC service to remove that particular timer from storage so that it’s not served back down to the scheduler.
We viewed this as a relatively simple solution to the problem, because we thought that this timer system was going to be pretty tricky to implement in the first place. We were a bit incredulous when we came up with this solution. We weren’t sure how we were going to represent the timers in memory. We weren’t sure how we’re going to avoid doubling-enqueuing. Once we just coded up a first pass, we realized that it was actually so much simpler and so much more performant than we thought it was going to be. We basically created an arena for these pending timer IDs in memory. We had an infinite loop with a 1-second period. We pulled all the timers from the gRPC interface, looped over them, and checked to see if they were known or not. If they were not known to the instance, we would spawn an asynchronous task using tokio. Use their built-in timer mechanism. When that timer expires, we would produce the Kafka events, delete the timer from the gRPC interface. Then there was a bit of additional synchronization code that was required to communicate this back to the main task so that we could remove that particular timer ID from the hash set. That was the only complicated piece here. The real service implementation is really not a whole lot more complicated than this. We were pretty impressed that it was quite so simple.
How does this thing actually perform? What do the numbers look like? Using the built-in default tokio async future spawning, and sleep_until functions, we tried to spawn a million timers, and measure both the latency and the memory utilization. We found that it took about 350 milliseconds to spawn a million timers. That ate about 600 megabytes of memory, which is a relatively high amount of memory for a million timers. It’s about 96 bytes per timer, which seems a bit heavy. We decided that this was good enough performance metrics to go out with. We were not going to invest too heavily at this point in ultra-optimizing from here. What key performance metrics did we identify once we were ready to ship this thing? The first one was the number of pending timers in that hash set. The number of things that we are watching right now. This was really important for us when we started getting out of memory kills on this timer, because we had not put any backpressure into the system, so if there were too many timers expiring at say 4:00, 4:00 rolls around, you try to load those all into the scheduler. Scheduler falls down. Scheduler starts back up. It tries to load the timers again, and it keeps falling over. We use this metric to identify what’s falling over at a million, let’s tell it to not load any more than 600,000 into memory. The other one was a little bit less intuitive. It was the timestamp of the last timer that we expired to Kafka. We use this to measure the drift between the timer system and reality. If it’s currently 4:00, and you just expired a timer for 3:00, that means your system is probably operating about an hour behind reality. You’re going to have some customers who are maybe asking questions about why their messages are an hour late. This was the most important performance metric for the system. This is the one that we have alerting around. If things start to fall too far behind reality, we’ll page an engineer and have them look into that.
The Storage Layer
Next, I’d like to talk about the storage layer for the system. Thinking about the requirements of this, we wanted to support a high write throughput. The goal that we had in mind was about 10,000 writes per second. From our experience operating Postgres, we knew that this was definitely possible to do with Postgres, but it was a big pain in the butt if you didn’t have a lot of dedicated Postgres staff, and a lot of infrastructure dedicated to clustering in Postgres. We didn’t really want to use Postgres for this. We wanted something that was going to be simple to scale, so that when we needed additional capacity, we could just throw it at the wall and have it stick. We wanted something that would be simple to maintain, so zero downtime upgrades were going to be really important to us. We knew that we were going to be making relatively simple queries that might serve back a bunch of results. What is a simple query? What is the kind of queries that we’re going to be making? The scheduler is going to be doing queries that look like, give me all of the timers that are expiring in the next 10 minutes. That is not a very complicated query. It’s not, give me all the timers that are expiring in the next 10 minutes for this particular customer, that are targeting these 10,000 users in these time zones, relatively straightforward queries. We didn’t necessarily need all the querying, filtering power of something like Postgres or another relational database.
In the end, we picked Scylla. This was already something that we were spinning up as a part of the larger journeys project. Even though we didn’t have existing in-house experience operating Scylla, we knew that we were going to be developing it as another business line item. One thing that we had to think about with adopting Scylla was that the data modeling approach for Scylla and Cassandra are very different from something like Postgres. We need to think pretty hard about how we’re going to be writing these data to the tables and how we’re going to be querying it afterwards. When you’re doing data modeling in a relational database, it’s a lot easier to just think about, what are the data and how do they relate to each other? You can, generally speaking, add any number of joins to a Postgres query, and then add any number of indices on the other side to make up for your poor data modeling. You don’t really have this luxury with Scylla, or Cassandra. They use SSTables. They don’t provide the ability to do joins. They really aren’t much in the way of indices other than the tables themselves. Ahead of time, as we were writing the data to the database, we need to be thinking about how we’re going to be querying it on the other side. The query we’re going to be doing is fetch all timers about to expire.
What does about to expire mean in this sense? If we think about the basic elements that we just said were a part of a timer, it’s got an expiry timestamp. It has a binary data blob. It has a Kafka topic and partition that the message is going to be written to. Most of the architecture work here was done by the Apache Cassandra team. My exposure to this ecosystem has all been through Scylla, so I’m going to attribute things to Scylla that were certainly done by people on the Apache Cassandra team. In Scylla, we have data that’s distributed amongst a cluster of nodes. As you query the data in the nodes, generally speaking, each query, we want it to hit a single node. We don’t want to be merging data together. We don’t want to be searching across all the nodes for particular pieces of data. Generally speaking, we want to know ahead of time where a particular row is going to land in the cluster.
How do we do that? How do we distinguish where a row is going to go? There’s a couple different layers of keys that exist on each row in Scylla, and we’re going to use those. The primary key has two parts, which is like a relational database, we have a primary key on each row. The first part is the partitioning key, that’s going to determine which node in the cluster a row is going to land on, and where on that node it’s going to go. It’s going to group the data into partitions that are shipped around as one unit. This is composed of one or more fields. There’s also a clustering key that determines where in the partition each row is going to go. That’s used for things like sort ordering. That’s optional, but it also can have a variable number of fields in it. Generally speaking, the kinds of queries, the high performance read queries that we want to be doing, you need to include the partition key, an exact partition key in each read query that you’re doing. You’re not having a range of partition keys. You’re not saying, give me everything. You need to provide, give me this partition key. The query we’re performing is get all the timers about to expire. What does about to expire mean? It means we need to pre-bucket the data. We need to group our timers into buckets of timers that expire around the same time, so that we can query all those timers together.
We’re going to be bucketing on 5-minute intervals. For example, a timer expiring at 4:48 p.m. and 30 seconds, we’re going to bucket that down to 4:45. Everything between 4:45 and 4:50, those are going to land in the same bucket. We are still going to store the expiry time, but we’re also going to have this bucket that’s specifically going to be used for storage locality. We can’t have the bucket alone be the primary key, because just like every other database that’s out there, primary keys need to be unique in tables. If the 5-minute bucket was the sole primary key, you can only have one timer that existed per 5-minute bucket. That’s not a very good data system. We’re going to introduce a UUID field that’s going to be on each row, and that’s going to take the place of the clustering key. That’s going to determine, again, where in the partition each row is going to land.
Our final table design looked like this. We had those same four fields that we talked about initially. We also introduced two new fields, this row, UUID fields, and the bucket fields, which is, again, the expiry timestamp rounded down to the nearest 5 minutes. You can see that on the primary key line down there, we have first the bucket field. That’s the partitioning key. Second, we have the clustering key field, which is the UUID field. What do the queries look like that we’re going to be doing on this table? We’re going to be getting all the fields off of each timer row inside of each bucket, inside of this 5-minute bucket that starts at 4:45. The eagle-eyed among you might already be noticing a problem with this. If it’s currently 4:48, and we get the timers that are in the bucket starting at 4:45. How are we going to do this 10-minute lookahead interval thing? How are we going to fetch the timers that start at 4:50 and 4:55? Because a 10-minute interval is necessarily going to span more than one, 5-minute data bucket. Further complicating things, this system is not necessarily always real time. It might be the case that this system is so far behind reality, which, in some cases, that might only be a couple seconds. It might be the case that there are still some existing timers that are falling into buckets that already ended. If it’s 4:45 and 10 seconds, and you still have an existing timer that was supposed to expire at 4:44 and 59 seconds, you still have to be able to fetch that out of Scylla. Because maybe the scheduler is going to restart, and it’s not going to be able to use the one that’s floating around in memory.
How are we going to pull all the buckets and get the data? We can’t just query the currently active bucket. We need to find out what buckets exist, which buckets that exist fall within our lookahead window. We need to query all of those for their timers. We introduced another table, a metadata table that was just going to hold every single bucket that we knew about. This is going to be partitioned just by its single field, the bucket timestamp. This was just going to give us access to query what buckets currently exist. Every time we insert data into our tables, we are going to do two different writes. We’re going to store the timer itself. We’re also going to do an insertion on this bucket table. Every insert in Scylla is actually an upsert. No matter how many millions of times we run this exact same query, it’s just going to have one entry for each particular bucket because they all have the same primary key. What do our queries look like? We’re first going to have to query every single bucket that exists in the database, literally every single one. That’s going to come back into the memory of our gRPC service. We’re going to say, of those buckets that exist, which ones fall into our lookahead window. That’s going to be the four buckets from 4:40 to 4:45. We’re going to query all the timers off of those. We’re going to merge them into memory of the gRPC service, and then ship them down to the scheduler.
If we put this all together into one cohesive system view. On the external team side, we have a thing that creates timers, that’s going to send a gRPC request across the wire to our service, that’s going to store a timer in our Scylla database alongside a corresponding bucket. Then, every minute, our scheduler is going to call the get timers gRPC method, with a lookahead window. It’s going to add the timers that fall into that window to its pending area in memory. When those in-memory timers expire, it’s going to write them out to an Apache Kafka topic. Eventually, maybe there’ll be a Kafka Consumer that picks that message up. This system, as I’ve described it, existed for about a year, maybe a-year-and-a-half without any major issues, modules that out of memory problem. We didn’t have to do any major scaling operations. It didn’t have any really big problems. We mostly just didn’t think about it after it was out there and running. We were pretty happy with it. Eventually, we started to think about adding more users to our journeys’ product. We started to think about using this timer system to support more use cases than just journeys. We realized that we would have to do some scaling work in this because what I’ve described has some poor scaling tendencies.
Jumping Forward, Q1 2023
Laine: My name is Hunter Laine. I’ve been an engineer on Lily’s team at OneSignal for about two-and-a-half years. In a past life, I was a marketing operations manager in Prague.
We’re going to take another leap forward in time to Q1 of this year. We have this effective timer service up and running pretty smoothly. It’s capable of storing billions of concurrent timers and expiring them in a performant manner, while minimizing data loss, and easily integrating with the rest of our systems. It’s essentially a set timeout function that’s available across our entire infrastructure, without any use case-specific limitations. It sounds pretty good. We thought so too, so we decided it was time to actually start doing that integrating with the rest of our systems.
The Case for Scaling Up
We send about 13 billion notifications a day, so we wanted to use the timer service to ease a significant amount of load on our task queues. This could be useful for a myriad of things, from retrying requests on failures across multiple services, to scheduling future notifications, and many other areas we were already excited about. If we were going to use the timer service in these many critically important areas, we needed to ensure that it could handle a lot more timers reliably than it currently could. We needed to scale up. The original motivation for and use of the timer service was to enable these journey builders, no-code systems generally use as a marketing tool. These systems constitute a significant number of timers that we were storing and retrieving. However, when compared to the task of integrating with our delivery systems, it represented a relatively small scale of use. At the scale of use, we had opted for this, again, slightly more simplified architecture to avoid dealing with the more complex coordination required to make the timer service fully scalable. Specifically, when we talk about scaling issues, we will be focusing more on the scheduler portion.
Scaling the timer service vertically was no problem at all. We could and did add resources to both the gRPC service portion and the scheduler as needed. Scaling the gRPC service portion horizontally was also no trouble. We could easily add a pod or four to handle an increase in create, get, and delete requests from multiple clients. The slight hitch that we were now facing was that the scheduler was not quite so simple to scale horizontally. We’d not yet done the work to allow for multiple schedulers to run at the same time. See, each scheduler needs to ask the gRPC service for timers at some set interval. It does no one any good if each individual scheduler is asking for timers and getting all the same ones back. Then we’re just duplicating all the work, instead of sharing the load. Plus, it certainly doesn’t seem like a desirable feature to enqueue each message to Kafka multiple times as we see here. We needed to do a bit of a redesign to allow for multiple schedulers to share the task of scheduling and firing timers with each in charge of only a particular subset of the overall timers. How do we do that? If we wanted multiple schedulers to run in conjunction, we needed to find a way to group timers by more than just time, so that those schedulers could be responsible, each one, for requesting and retrieving only a particular group of timers.
First, we needed to make some adjustments to how our data was stored so the timers weren’t stored just by the time that they were to be sent out, those 5-minute bucket intervals, but by some other grouping that different schedulers could retrieve from. We made some adjustments to our Scylla table schemas so that when a new timer is created, it is inserted into a bucket by both that time interval, and now a shard. While we were adjusting these schemas, we also actually decided to shrink that bucket interval from the 5 minutes we’d been using to 1-minute bucket intervals. This was because we were already noticing that our Scylla partitions were getting larger than we would like. We would like to keep our Scylla partitions in general relatively small, as this leads to more efficient and less resource intensive compaction. We determined which shard a timer belongs to by encoding its unique UUID to an integer within the range of the number of shards we have. We actually have our shards set to 1024, with timers pretty evenly distributed among them. Each scheduler instance is then responsible for an evenly distributed portion of those shards. We went to this granularity and this many shards, also in an effort to keep those Scylla partitions relatively small. This means we have a more efficient way to parse a far greater number of timers. This update also makes the bookkeeping of the buckets table much more efficient, and means that when querying for timers, we look much more particularly at a particular shard and time to retrieve from. We then just needed to adjust our get timers request so that when we do request those timers, we do it not just by time, as we were before, but in addition by that shard, so that we can get a particular subset of the overall timers.
We now have a system whereby timers are stored by both time and shard, and a way by which to retrieve those timers by both time and shard. We’re there? Just one big problem. Each scheduler instance needs to have state so that it can reliably ask for the same subset of timers every time it asks. How does each scheduler know who it is? This was important because if a scheduler instance were to restart for any reason, it needed to start back up as the same scheduler so it could pull the same timers. If we have multiple instances pulling the same subset of timers, we’re back at square one. If scheduler 2 were to restart for any reason, now thinking that it’s scheduler 1, when we already have a scheduler 1 up and running and pulling timers for scheduler 1, we’re again duplicating work and erroneously firing timers multiple times. Plus, even worse here, no one is looking after the shards assigned to scheduler 2.
In order to solve this, we deployed a new version of the scheduler as a stateful set in Kubernetes, which, among other things, gave us a stable unique name for each instance of the scheduler every time it started up, with each name ending in a zero-indexed value up to the number of replicas. Each scheduler could then take that value and calculate a range of shards that it’s responsible for retrieving timers from. Importantly, this means that each shard of timers and therefore each individual timer will only ever be retrieved by one scheduler instance. We now have this system where we store timers by both time and shard, where we have retrieval requests that can get a subset of timers by both time and shard. Now, schedulers that have state and can therefore reliably request the same subset of timers every time they ask. We have achieved full scalability.
Performance Characteristics
With these changes made to the architecture of the timer service, adding new nodes to the scheduler is as simple as increasing the replica count in the config file, which makes scaling the timer service both vertically and horizontally, possible and simple to do as we continue to use it in more parts of our system. Because we now have multiple instances of both the gRPC service portion and the scheduler, we’ve made the timer service much less susceptible to serious outage if a node were to go down. Previously, we only had one scheduler, so if it went down, it was it. There were no timers being retrieved, or processed, or fired, and no messages being enqueued to Kafka by the timer service until that node were to restart. Now, because we have multiple instances, each in charge of only a particular subset of the overall timers, if a node goes down, it has much less impact on the overall functioning of the system. On single pod performance, each scheduler node is capable of handling about 10,000 timers per second. Frankly, that’s without even pushing it, which makes horizontally scaling incredibly powerful. Each gRPC instance handles about 17,000 requests per second really without trouble.
Callouts
There are a few things to note about our timer service as it exists today. First, the timer service has an at-least-once guarantee. This is because there’s a space between the scheduler enqueuing its message to Kafka, and then turning around and requesting that that timer be deleted. If the scheduler were to restart for any reason between those two actions, or if the gRPC service has some communication error between the scheduler or between Scylla when processing that delete, the timer will fail to be deleted and will again be retrieved and fired. Because of this, the timer service does expect that all downstream consumers manage their own idempotence guarantees. Another callout about the timer service is that timers will fire close to but not exactly at the time they’re scheduled. This is because schedulers still need to pull in timers at that periodic interval via that get timers request. We currently have each scheduler set to pull in the next 10 minutes of timers every 1 minute. Possibly the biggest callout about the timer service as it exists today, is that once a timer has been retrieved by the scheduler, there’s no method by which to cancel it. This is because the only way to delete or cancel a timer in this system the way we have it currently, is via that delete timer request on the gRPC service, which deletes from the database. Therein, if the timer has already been retrieved from the database and is now in the scheduler, there’s no way to currently stop it from firing.
Future Potential
The timer service has proven to be incredibly powerful within our own internal systems. As such, we really believe it could be useful to other organizations and individuals. There’s definite potential that we will move to open source it in the near future. As we continue to grow as an organization, we intend to use the timer service as we create new services, but want to dedicate resources to integrating it more broadly across our existing infrastructure in order to streamline. We also would like to add and fine-tune features such as perhaps the ability to cancel the timer at any point in the lifecycle.
What We Have
We’re about four years on now, from that original conception of a timer service to enable those journey builders. We now have an incredibly robust system that’s capable of storing billions of concurrent timers and expiring them in a performant manner, while minimizing data loss, easily integrating with the rest of our systems, and, importantly, scaling simply both vertically and horizontally to accommodate our future use.
Questions and Answers
Participant: How did you handle when you added the new nodes to the scheduler? If you have 4 running in each kit, 250 charge, you add a fifth one, now they’re going to be 200? How did you keep them from stepping on each other’s toes as you increased that number?
Mara: We would take all of the nodes down at the time that a restart occurred, and start them back up. There would maybe be 30 seconds of potential timer latency. We weren’t super concerned about millisecond accuracy of the schedulers in this case.
Participant: One of the interesting places for scheduling [inaudible 00:41:14].
Mara: I was the manager on the team, at the time we were initially building out this project. The engineer that was in charge of the implementation, he was running all these complicated data structures past me, and I suggested, did you try the most naive possible approach? Did you try spawn a future and wait on a task? He was like, that can’t possibly perform well enough for our needs. I said, why don’t you try it and we’ll see if it performs well enough? It did. We actually didn’t really continue to evaluate more complicated data structures because the easiest one worked for us.
Participant: How do you handle the [inaudible 00:42:38] that replicated the time zones, or anything related here. Did you sidestep or how did you handle that?
Mara: That was totally sidestepped. These timers were all in UTC. These were all server-side events. Every timer that’s in this system, is a timer that was scheduled by another team at OneSignal. If somebody cared about something being time zone sensitive, they cared about, you send a notification at 8 a.m. in the user’s time zone, they would have to figure out when 8 a.m. on the user’s time zone was on a UTC clock.
Participant: You mentioned that you have a secondary table that you store all the tickets, and you request all the tickets [inaudible 00:43:34] for next interval you want to fetch. That’s essentially like a full table scan?
Mara: That is basically a full table scan. The thing that’s saving us from really having a bad time on that is the fact that the number of entries on that table is much more constrained than the number of entries on the timers table. There’s going to be a maximum of one entry on that table for every 5-minute interval. I don’t think the timer system actually has a limit on how far out you can schedule timers. The systems that currently enqueue timers I believe do have limits on how long they will allow you to schedule out a timer. Like journeys will not allow you to, say, send a notification then wait for 30 days. That just puts a constraint on the number of things that are allowed to live in the dataset. Yes, it’s potentially very expensive.
Participant: I have a few questions about the database structure itself. It spawns data in the case of Cassandra? It must be more performant than the Scylla database. At the backend, how many nodes do you have, and what the replication factor is? [inaudible 00:45:06]
Mara: We have just a single data center, I believe four timers. We have I think six nodes with Scylla. I’m not sure if we did any of our own benchmarking of Scylla versus Cassandra. I think at the time, our CTO had quite a strong aversion to Java. I believe we actually run zero Java code in production, and adopting Scylla versus Cassandra was partially motivated by that desire. We have been quite happy with Scylla since we’ve adopted it.
Participant: [inaudible 00:46:06]
Mara: A timer would be returned by the gRPC service multiple times until it was expired, until that delete timer method was called for that particular timer. That, I suppose, is another inefficiency of the system. There’s a bunch of data retransmission. Again, this was done in the name of the simplicity of the system. That is something that probably could be removed in the future if we wanted to optimize that a bit more.
Participant: It’s basically that there is a possibility here of some crashing bug in a particular [inaudible 00:47:20], if one of your scheduled nodes stopped and crashed, do you have a mechanism of recovering that, or that is not a problem?
Mara: That hasn’t been a problem for us, I think because the API of a timer is so small. The variance in each timer is time and data and Kafka settings basically. We don’t give you more Kafka settings than topic and partition. The attack surface is really small. We haven’t had any instances of a malformed timer that was causing issues for the service. If a particular node of the timer system was just crash looping, we would basically just have to page an engineer and have them look into it. There’s not auto-healing built into it.
See more presentations with transcripts