Month: June 2024
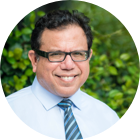
MMS • RSS
Posted on mongodb google news. Visit mongodb google news
Park Avenue Securities LLC trimmed its position in MongoDB, Inc. (NASDAQ:MDB – Free Report) by 9.4% in the first quarter, according to its most recent filing with the SEC. The fund owned 1,963 shares of the company’s stock after selling 204 shares during the period. Park Avenue Securities LLC’s holdings in MongoDB were worth $704,000 as of its most recent filing with the SEC.
Other hedge funds also recently modified their holdings of the company. Transcendent Capital Group LLC acquired a new stake in MongoDB in the fourth quarter valued at $25,000. Blue Trust Inc. increased its stake in shares of MongoDB by 937.5% in the fourth quarter. Blue Trust Inc. now owns 83 shares of the company’s stock valued at $34,000 after buying an additional 75 shares during the period. Beacon Capital Management LLC raised its position in shares of MongoDB by 1,111.1% during the fourth quarter. Beacon Capital Management LLC now owns 109 shares of the company’s stock worth $45,000 after acquiring an additional 100 shares during the last quarter. Raleigh Capital Management Inc. lifted its stake in shares of MongoDB by 156.1% in the third quarter. Raleigh Capital Management Inc. now owns 146 shares of the company’s stock worth $50,000 after acquiring an additional 89 shares during the period. Finally, GAMMA Investing LLC bought a new stake in MongoDB in the fourth quarter valued at approximately $50,000. Institutional investors and hedge funds own 89.29% of the company’s stock.
Insider Transactions at MongoDB
In other news, CRO Cedric Pech sold 1,430 shares of the business’s stock in a transaction on Tuesday, April 2nd. The shares were sold at an average price of $348.11, for a total transaction of $497,797.30. Following the completion of the transaction, the executive now directly owns 45,444 shares in the company, valued at approximately $15,819,510.84. The transaction was disclosed in a document filed with the Securities & Exchange Commission, which can be accessed through the SEC website. In other MongoDB news, CAO Thomas Bull sold 170 shares of the business’s stock in a transaction that occurred on Tuesday, April 2nd. The shares were sold at an average price of $348.12, for a total value of $59,180.40. Following the completion of the transaction, the chief accounting officer now owns 17,360 shares of the company’s stock, valued at approximately $6,043,363.20. The transaction was disclosed in a filing with the SEC, which is available through this link. Also, CRO Cedric Pech sold 1,430 shares of the firm’s stock in a transaction on Tuesday, April 2nd. The shares were sold at an average price of $348.11, for a total transaction of $497,797.30. Following the sale, the executive now owns 45,444 shares in the company, valued at approximately $15,819,510.84. The disclosure for this sale can be found here. Over the last ninety days, insiders have sold 60,976 shares of company stock valued at $19,770,973. 3.60% of the stock is currently owned by corporate insiders.
MongoDB Stock Performance
NASDAQ MDB opened at $244.15 on Friday. The company’s 50 day moving average is $306.14 and its 200-day moving average is $367.45. The firm has a market cap of $17.91 billion, a P/E ratio of -86.89 and a beta of 1.13. MongoDB, Inc. has a 12-month low of $214.74 and a 12-month high of $509.62. The company has a quick ratio of 4.93, a current ratio of 4.93 and a debt-to-equity ratio of 0.90.
MongoDB (NASDAQ:MDB – Get Free Report) last posted its earnings results on Thursday, May 30th. The company reported ($0.80) earnings per share (EPS) for the quarter, hitting the consensus estimate of ($0.80). The firm had revenue of $450.56 million for the quarter, compared to the consensus estimate of $438.44 million. MongoDB had a negative return on equity of 14.88% and a negative net margin of 11.50%. Sell-side analysts predict that MongoDB, Inc. will post -2.67 earnings per share for the current year.
Analyst Ratings Changes
A number of equities research analysts have weighed in on MDB shares. Citigroup lowered their target price on MongoDB from $480.00 to $350.00 and set a “buy” rating for the company in a report on Monday, June 3rd. Scotiabank cut their target price on shares of MongoDB from $385.00 to $250.00 and set a “sector perform” rating on the stock in a research note on Monday, June 3rd. Monness Crespi & Hardt raised shares of MongoDB to a “hold” rating in a research note on Tuesday, May 28th. Guggenheim upgraded shares of MongoDB from a “sell” rating to a “neutral” rating in a report on Monday, June 3rd. Finally, Loop Capital reduced their target price on MongoDB from $415.00 to $315.00 and set a “buy” rating for the company in a research note on Friday, May 31st. One investment analyst has rated the stock with a sell rating, five have given a hold rating, nineteen have given a buy rating and one has issued a strong buy rating to the company. According to data from MarketBeat.com, the stock has an average rating of “Moderate Buy” and a consensus price target of $361.30.
Read Our Latest Research Report on MDB
MongoDB Company Profile
MongoDB, Inc, together with its subsidiaries, provides general purpose database platform worldwide. The company provides MongoDB Atlas, a hosted multi-cloud database-as-a-service solution; MongoDB Enterprise Advanced, a commercial database server for enterprise customers to run in the cloud, on-premises, or in a hybrid environment; and Community Server, a free-to-download version of its database, which includes the functionality that developers need to get started with MongoDB.
Featured Articles
Want to see what other hedge funds are holding MDB? Visit HoldingsChannel.com to get the latest 13F filings and insider trades for MongoDB, Inc. (NASDAQ:MDB – Free Report).
Receive News & Ratings for MongoDB Daily – Enter your email address below to receive a concise daily summary of the latest news and analysts’ ratings for MongoDB and related companies with MarketBeat.com’s FREE daily email newsletter.
Article originally posted on mongodb google news. Visit mongodb google news
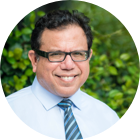
MMS • Tony Ponton
Article originally posted on InfoQ. Visit InfoQ
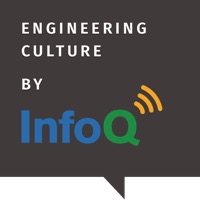
Subscribe on:
Transcript
Shane Hastie: Good day, folks. This is Shane Hastie for the InfoQ Engineering Culture podcast. For a pleasant change, I am sitting down with somebody relatively close to me. It’s just one country away, not many, many hours. I’ve got the pleasure today of talking with Tony Ponton. Tony and I have known each other for decades.
Tony Ponton: That’ll do.
Shane Hastie: But Tony, first of all, welcome. Thanks for taking the time to talk to us today.
Tony Ponton: Oh, it’s a brilliant pleasure. I’m really looking forward to it, Shane. You know, I’ll always talk to you. Doesn’t matter where, when.
Shane Hastie: Thank you. All right. So you and I obviously know each other pretty well, but I suspect most of our audience wouldn’t have heard of you. So, who’s Tony?
Introductions [00:48]
Tony Ponton: That’s fair. I’m Tony Ponton, I’m an executive principal for a consultancy. I like to say with the Agile thing, I’ve been doing this Agile thing for … I’m in my third decade is how I put it, Shane, rather than saying that I’m old. And I grew up starting the Agile journey in very early world, it was XP before we really even knew what Agile really was. So I’ve been doing that with enterprises and organizations across the world, and recently, I wrote a book with my co-author, Phil Gadzinski, who couldn’t be with us tonight. He’s on a houseboat somewhere enjoying himself. So he left me to my own devices. He’ll be sorry for that.
Shane Hastie: The book, Govern Agility: Don’t Apply Governance to Your Agile, Apply Agility to Your Governance. Bold words.
Tony Ponton: Yes, I guess it goes to the heart of why we wrote the book, really, and we call it Govern Agility rather than Governance Agility or Governance Agile, or whatever you want to call those things, because when we’re talking about it, let’s just take that first thing as we’re talking about it tonight. When we’re talking about governance or governing, we’re talking about governing the system as a whole. So for the listeners, we’re not really just talking about projects and PMOs and those types of things and portfolios. Not that they’re not part and parcel, those are very important parts to that, but governing the system as a whole means the whole of the organization. And therefore, that’s why we talk about the fact that you don’t want to put governance in your Agile and choke the ability, or I call it disenable your ability to use the agility that your organization has by creating complexity.
Shane Hastie: Why does governance create complexity?
Good governance systems should not create complexity [02:33]
Tony Ponton: Well, that’s a good question. I’m glad you asked me that, Shane. I could see the cogs turning there. So good governing systems shouldn’t create complexity, but they do. And there’s a few reasons, and when you read the book, we actually spent a fair piece at the beginning explaining where we see that, where we’ve seen it, why we’ve seen it, which places us in the realm of why we wrote the book. A lot of organizations have had governing systems in place for a very long time, and they suffer from what I call organizational detris. It’s that buildup of the system on top of the system, on top of the system, because something went wrong. So we did something to stop that. Now we put something to control that, then we put something to control that. In fact, they end up with so many layers of governing throughout their systems from portfolio to enterprise to right down to the teams, people are doing things they don’t even know why they’re doing, how they’re doing it.
And the buzzword at the moment is flow, right? We all know that and we all know what flow is or have been working with it for a very long time. But the reality is the old saying about you can only be as Agile as your most Agile leg. It also goes to the other spaces. What happens is organizations, they go with this goal of being transformation and we’re going to transform our organization into a more Agile or a more agility based organization or adaptive, whichever one of those buzzwords you want to use, right?
Shane Hastie: Digital today, isn’t it?
Organisations often have contradictory pressures between strategy, governance and operations [03:58]
Tony Ponton: Yes, that’s it. There’s another one. What they actually end up is transitional, because what they do nine times out of 10 is it’s what I call the three-speed economy. You’ll hear me talk about it a lot. The three-speed economy is really like this. They usually have their systems of strategy and leadership, and generally most organizations know where that’s going. It could be cleaner, it could be neater, it could be better. If they don’t, then we’ve got a whole different conversation.
They then turn to shaping where the systems of work get done, because that’s the easy place to start. So they build tribes and cross-functional teams and all the things that we talk about, not that I’m against all of that, that works great. But what they leave behind is the governing systems and the funding systems, and they’re usually going in a separate way. And worst-case scenario, your funding is going one way and your governing systems are going the other way. And believe me, I’ve seen that a number of times as you have. And so essentially what happens is then your ability to enable your agility actually hits what I call the complexity ceiling.
You get bound into this complex utterance of all of the governing systems and the funding systems and not thinking of it as a whole. So what we’re really looking for when we talk about Govern Agility is having those systems shaping and moving in the same way from your strategic intent to your delivery. And there’s that sort of extra piece that connects to it, it’s what we call the collaborative connective tissues of the organization. It’s the horizontal flow of information plugging into the vertical flow of information, which is the strategic intent to your execution, the execution back to your strategic intent.
And so what we see is that that gets truncated quite regularly as well through the amount of restructures, repoints, realigns, have I got them all? Was that all the terms there? I’m sure there’s other ones you can think of for that one. So every time you pull something apart, you actually break your collaborative connective tissues. And usually as that all gets pulled apart, the governing systems don’t change either. So they’re still applying the same governing systems that they’ve had in place for X amount of years, and yet they’re creating this agility. So I guess that’s where we came from when we were thinking about it and looking at organizations and we were seeing this happening at many organizations across the world.
Shane Hastie: Governance exists for good reasons in organizations. How do we, I want to say, sharpen it or make it more effective and avoid some of that complexity?
Governing the system should be part of the everyday work [06:31]
Tony Ponton: Here’s the thing, I’m going to say something quite controversial, which I’m sure you’re going to enjoy, but I’ve been in the Agile community as I said, this is my third decade. And I think as agilists, we have been remiss in thinking about the governing systems or governance as a whole. And often it gets pushed to the side as something that we’ll get to it or those governance people can go do that thing, but we’re going to do our Agile thing. And I’ve heard that and I’ve seen that in organizations. So when you talk about sharpening it, well, the first thing is to actually bring it closer to the mix. The governing of the system should be part of your everyday work, not an operational overhead. And the minute it becomes an operational overhead, then you’re actually creating bottlenecks that actually choke the daylights out of your system.
The other thing with that is it’s looking at it in terms of your governing systems and not just, as I said, not just these checks and balances in the book. We look at it through a set of lenses and we’ll talk about those I suppose, the five lenses, which we call the stanchions. We’ll talk about those in a minute. So the answer to your question there is you have to design your governing systems to the context of your organization, but to the context of enabling your ability to use your agility. And I think that’s the important statement here.
Shane Hastie: So what does that look like in practice? If I’m a large financial institution, subject to Sarbanes-Oxley and various other bits of legislation depending on where we are in the world, we’re tasked with looking after other people’s money perhaps, and we don’t want to take chances with that.
Tony Ponton: Yes. In no way should we, and in no way are we saying you don’t have those checks and balances. But there’s also the guide rails that allow you to bring it close in person, place, and time is what we talk about, right? Closer to the people that do the work, that know what’s happening and are able to make decisions that allow you to expediently understand the risk and make those interventions to the system. And that’s what we’re talking about is those short sharp cycles that allow you to adapt and change. I’ve never met anyone in the C space or even program managers who when you say them, wouldn’t you like to be able to understand what’s happening daily, weekly, fortnightly basis rather than find out a quarter or your steering committee or your CAB or whatever that be that’s held once a month that everything has gone to putty, right?
And we all know the watermelon story, everything’s green and then it’s red. Well, I’ve taken it a step further. What happens in a lot of these systems is that they drop it on the floor and the red goes everywhere and then it becomes code brown and it hits the fan and then we have an organizational issue. And I think we can all think of organizations who found themselves in that drama, found themselves in the press recently as well. So in no way should you not be doing that. But it’s about how do we shorten the cycles that’ll allow us to adapt and use the agility and enable the agility and the flow of our organization to have that information so that we’re all moving in the same shape and size, if that makes sense.
Shane Hastie: You talk about the five lenses, the five stanchions. What are those five stanchions?
Information flow which allows speedy decision making is the key to effective governance [09:48]
Tony Ponton: Let me start with what is a stanchion? I’m surprised you didn’t ask me that, because a lot of people have. Then when we were putting this together, we had some serious debates over what we would call the lenses, whatever it might be. The reason we settle on stanchions, if you look it up, stanchion is a pole that actually you use to hold a structure up and usually those stanchions then fall away.And so we see that as their containers or scaffolding to enable what you’re adjusting your agility to.
And there are five key ones of those that we look at that we have distilled out of what we’ve been doing over the last three decades as the things that will make the change in your agility to allow that adaptability, to allow you to put the Agile in the governance run. So the first of those is sensible transparency, and we use the word sensible, because if you’re in an organization, talk about radical transparency.
Sensible Transparency [10:42]
I know the book, I know the thoughts around that and some of the conversations are fantastic, but the words radical transparency strike fear into the heart of CEOs and CIOs anytime you use that. But sensible transparency for us is thinking about what you’re going to be transparent about and being transparent about what you can’t be. But it’s looking at it through the lens of how do we get the transparent flow of information that allows us to make these expedient decisions. And so starting to look at things like using Obeya’s, digital Obeya’s these days, we’ve got all of that flow of information.
Setting our systems up, and we’ll talk about that, because that’s another lens, obviously, but allowing us to have that ability to have the transparency of information that alleviates what we call the bifurcation of information. So usually, people at the top tend to know where they’re going and what they know, and the people at the bottom tend to know what’s happening with their work, but it’s not necessarily a line at the top. And so we’ve got this split, right?
And what we’re trying to do is make sure that as collaborative connective tissues are in place so that we can use that, because the stock in trade of agility, as it was always told to me, is information flow. That’s what Agile does. It gives you information flow. You can look at all the other things everybody talks about, but the reality is it gives you information that allows you to make speedy decisions. It allows you to understand progress and it allows you to understand the killers of work, dependencies, risks, etc. So you want to set that transparency. So from the bottom of the organization to the top of the organization and across the organization, you can see that in a transparent way.
And we do that in a sensible way, because obviously you say there’s legislative things, some of those things you can’t tell people about, but you can be sensible about that and say, “Well, here’s X.” So it’s having that transparent view, a window into the organization. So radiating information from the organization to the organization, is a great saying one of my friends, James McMenamin (shout out) uses.
Conductive Leadership [12:38]
So that’s the first one. And then we talk about leadership. So we talk about it in terms of conductive leadership, and that’s an interesting turn of phrase. I think a lot of people have asked me about that as well. The reason we talk about conductive leadership is not that I have anything against intent-based leadership or there’s so many leaderships I can come up with for you. Servant leadership, please, that’s again, as an agilist, I think that’s another thing that we as an agilists have done ourselves wrong with. There is no CEO, CIO. There’s no leader that I’ve ever talked to that wants to be called a servant. I mean, I don’t pick up after my kids. You’re basically innovating that they’re going to be servant to the people and do everything for them. And then you have this long-winded discussion about, “Well, we really mean…” So as Agile, I think we haven’t done ourselves wrong.
We talk about in terms of conducting, because we found that that was a great analogy to what we’re talking about with leadership. We want to change the frame of leadership from the typical command and control. We all know that, right? And when you introduce agility to your organization, by rote it disseminates control. That’s what it does, because we’re focusing it down into whether the people get closest to the work.
So what that only leaves is command as a lever to pull. And you’ll hear, I must, you should, you will, you have to, you’ve got to. And the minute that starts happening again, then you’re disenabling your ability to use the agility in your organization. And in your governing systems, right throughout the organization, you need to look at that in a different leadership style, thinking about how you can be more conductive. And we saw a fantastic video which is attributed in the book of a conductor, and it was a gentleman explaining how the conductor actually manages the orchestra. It’s not just a waving stick for fun.
The thing I talk about to people all the time is does the conductor actually play the notes? No. Does he play the instruments? No. But he does help the actual orchestra themselves, put it together in a way that it makes sense and he guides them through the flow of it and he makes interventions. So he does interventions in a different way. If you watch him and next time you’re watching him, I’ll pick on you, Shane, because you happen to be in the room, but Shane plays a bum note. You get a little double tap from his stick just to let you know you didn’t quite get that.
Tony Ponton: Yes, you do it again, you get a very strong stick and he’s made an intervention without actually getting stuck in the execution. And I guess that’s the bottom line is that we’re looking to change that leadership to what we call more conducery, right? You’re not in the actual getting stuck in execution, which you see a lot of leadership doing and bringing the ability to make those decisions, intent-based leadership, leading with intent as David Marquet talks about. Setting the guide rails, not guardrails. So I use that turn of phrase when I talk about leadership and guardrails are the last thing that you see on a very steep chasmic road, where you could drive off it.
And you’re living in New Zealand, Shane, you know what I’m saying, right? By the time you hit those, that’s your last resort. What we want to do is more think about it in terms of the new cars with the fantastic lane control that I always switch off, because it drives me nuts. But anyway, it pulls you back into line. It just course-corrects, makes that adjustment and it gives you the guide rails of where you need to go.
So we’re talking about setting those guide rails, leading with intent, being more conducery in the terms of leadership, using the transparency. So of course these things are all interlinked and using the transparency to help you make those decisions as leaders expediently. So that’s that piece of the puzzle. And of course then we talk about the patterns of work and systems of work, right? And essentially you have to actually think about how you set your systems of work up to enable your ability to use your agility.
You need to set your systems of work up so they enable flow, but they also produce the flow of information, transparency and enable the leadership to be able to be part of that system so that they can understand what’s happening as well. So it’s moving the entire system of work and thinking about how it works in conjunction rather than being truncated in that original example I gave you about the three speed economy, if you like.
Data-Driven Reasoning [16:53]
I’m being careful, because I know I could go forever on each of these, there’s chapters and chapters in the book. And of course, then that brings you to data-driven reasoning. So here’s the thing, when we were looking at the data piece of it and we came up with data-driven decisions, and then it was data-driven reasoning and the reason we settled on it in the way that we did was because the words out there at the moment, everybody’s heard it, data-driven reasoning, they don’t hear that much. What they hear is data-driven decisions. They hear data-driven intent. Well, you can have intent and you can have decisions, but the reality is you actually have to have the data that allows you to reason and it allows you to use that data in a way that allows you to actually reason in terms of introspecting it, understanding it, creating insights, improving, being able to make speedy decisions and reasoning.
And you’ve got smart people, your hire smart people, right? So if you just sit on the data and use the data to make your decisions, then you’re literally taking smarts out of it as well. And when you think about it, when you think about that in terms of AI coming for us as well, this reasoning factor, it’s going to give you a plethora of data and information back in speed, but you actually have to think about reasoning, if that makes sense.
Humanity as the Cornerstone [18:07]
So they all got to be working in concert as well. So what we talk about it is that if one is out of kilter with the other, then your organization is out of kilter and your governing systems are out of kilter and you find yourself in a complexity hole. That brings us to the middle or the cornerstone as we call it, and this should strike to every good agilists heart is humanity is the cornerstone. And the reason we say that is because quite often in terms of governing and governance and governing the system, it becomes a very transactional, mechanistic kind of system.
And the reality is you actually have a blip in the radar and that’s the humans, the ghost in the machines, I talk about it, right? Humans will do human things and humans will make human decisions. So you need to actually enable the humanity in it and enable the people. We talk about trust, but verify, which is an old proverb, came out of the Reagan. We’re going to trust you to do X, but we will verify. So the governing system should be more around verification of than actually the management of and the micromanagement of and grinding your people to a halt, because you’re actually so governance based around what they’re doing and how they’re doing it within the organization rather than thinking about how you can leverage the actual people themselves.
And we talk about that aligns with all of the other stanchions that we talked about, because the reality is you want to bring it close in person, place, and time. Your governing systems need to be closest to where the people are who do the work at the time the work gets done, because that will allow you to make those expedient decisions. That’ll allow you to create flow and that will allow you to manage the risks, the dependencies, issues, all of those things that we talk about when we talk about governance and funding and those types of things. Obviously, I’m giving you a whistle-stop of it there’s a lot more depth to that, but hopefully that was a quick explanation, Shane.
Shane Hastie: Yes. So let’s bring this really practical. I think of our audience, many of them are technical influencers. They’re not able to change the structure of the organization, but they’re sitting down trying to get work done on a day by day basis. Why should they keep?
Why this matters for technologists [20:18]
Tony Ponton: Because the reality of it is that these are the things that are stopping them from getting their work done on a day-to-day basis or slowing their ability to make the work down. Or they’re in a situation where they’re so beholding to the decision-making matrixes that have been put in place, that they’re actually disenabled in terms of their unhappiness around the work as well. And I talked to them, I was one of these people. I started off in enterprise and I remember those situations where I was just going, “Why are we doing this? Why are we doing this?” You would become almost disenabled to go, “Oh, well just going to do it, because I got to do it and I’ll do it, but I’ll do it in the most minimal way that I have to.” And so I think that’s why they should care because if they don’t, then the system itself will continue. If you push hard enough on the system, the system itself will push back.
Shane Hastie: So if I am in that technical influencer, team lead, maybe middle to senior manager in a technology part of an organization, how can I influence bringing these ideas in?
How to influence bringing in new ideas [21:21]
Tony Ponton: The way that you can do that is start to make small change anytime. It goes back to asking the questions that will help people see the light, I suppose is the question. Or I could make a shameless plug and say, “Bring me in,” but I won’t go there. But the reality is making small changes that allow the organization to see those changes. Somebody asked me the other day in an interview that I was in was, what are the three things that you tend to ask?
And it’s the same thing I say to those leaders, can you see your single demand view? Do you understand all of the work that’s coming at you or when it’s coming at you? Do you understand your demand versus your capacity versus your ability to deliver? Not only can you, but can the people above you and the people above those. And I hate to do the above, above, because that’s sort of like, all right, well let’s just face it, that’s how it works, right?
But the reality of that is so you can start to make some changes and start to make those things visible and the light bulb start popping on. So I’ve worked with teams when we was thinking about these things where I’ve gone, “Well, how can we make some change here?” So we started making the capacity very, very clear versus the demand versus the work that was coming at them versus the in progress and making them very, very transparent against their ability to create flow.
Because immediately you start to see that thing, people start to ask the right questions. So then that is a catalyst of the conversation. And we talk about it, you want to do this in a very principled way. You don’t want to just go at it ad hoc, because the other thing that I see people just run into it and that’s the dangerous thing as well.
So we in no way say that you should just throw all the cards up in the air and do that. If you’re thinking about it in a very principled way of thinking about how you can make some of those changes, well let’s decide what we’re going to decide. What are those relevant activities? What are the phases we need to apply that decision into? And then go, “Okay, well if we know what that is, then decide how to decide.” Let’s make an effort here to govern the actual logic, not the decision itself.
So think about that, design your mechanisms to ensure that the logic is very consistent, very clear, very transparent. Transparency comes in again, so I always talk about you want clarity and you want consistency, that’s really important. And then decide who decides. Who can decide on these things. Set up these decision-making frameworks and allow the decision to be made as close, in person, close in time as I was talking about.
I was in with an organization some time ago, very governmental organization is what we’ll say. And that particular government definitely has the hierarchy of X. And there are some very good reasons to your point before where they hold certain decisions. And when we were in that particular conversation, someone said, “Well, I can’t do that, because he has to make all the decisions.” And he actually turned around and said, “Well, you know what? I do have to make that decision, but you can make those decisions and you can bring the information back to me so I can help you make that decision.”
And so all of a sudden that changed the frame of reference. So decide who decides, and then big thing is decide when to decide. We should make them at the most appropriate times. We want to make the short cycle decision and we also want to make not too late, not too soon. So again, I like to give you organizational context around it.
Working with an organization one stage where they basically couldn’t get anything through the flow, because their organization’s steering committee only met once every two months and then they had to prepare a 965-page document of x, x, x, x, x, x, x. And they were filling this stuff out and nobody knew why they were filling it out. When we sat down with the actual steer co and said, “Well, can we shift it to the left? Can we make this shorter cycle and do they need to fill…” And they were going, “We don’t even read that stuff.”
So I think that’s a really important thing that you do, and that’s why we talk about making your governance a really seamless integration, make it part of the everyday flow of work. And these middle leaders that you’re talking about, they have the ability to do that. They don’t want to make it an operational overhead, but how do we make that part of what we do in our flow?
Shane Hastie: A lot of good ideas.
Tony Ponton: I’m an ideas man, I always said that, Shane.
Shane Hastie: If people want to continue the conversation, where do they find you?
Tony Ponton: You can find me and Phil Gadzinski on LinkedIn. And you’ll find a Govern Agility page on LinkedIn as well. But we’re always happy to talk, reach out to us, please. We’re happy to have those conversations, because we only touched the tip of the iceberg today.
Shane Hastie: Indeed. Tony, as always, it’s a pleasure talking. Thanks so much for taking the time to talk to us today.
Tony Ponton: Shane Hastie, it has been an absolute pleasure as always.
Mentioned:
.
From this page you also have access to our recorded show notes. They all have clickable links that will take you directly to that part of the audio.
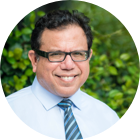
MMS • Nischal HP
Article originally posted on InfoQ. Visit InfoQ
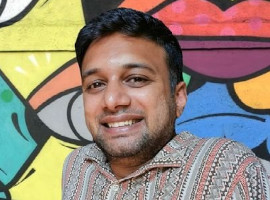
Transcript
HP: I’m going to be talking a little bit about the work that we’re doing at scoutbee in building large language models or enabling large language models and generative AI applications in the enterprise landscape. Before we get all technical and go down that road, moat is the water around the castle that you see here. I wanted to take a little bit of attention away from large language models and talk about black swan events. The black swan events are unpredictable, they have severe consequences. The fun around black swan events is that when it really happens, you look at it, and you connect the dots back and say this was bound to happen. In the supply chain space, up to about 5, 6 years ago, 80% of the supply chain was predictable in the sense people knew when to expect delivery, they didn’t have a massive supply chain break. Twenty percent were surprises. Unfortunately, or maybe fortunately for us at scoutbee, this has flipped. You see 80% of surprises and 20% of predictability. You might be wondering, maybe he’s making these numbers up. Let me walk you through some painful experiences we’ve all had to share in the last 5 years. COVID-19 happened, and I think everybody up until then thought supply chain was this thing that just existed somewhere, until they went to a supermarket and couldn’t find pasta to eat, or worst case, toilet paper was missing. You wondered, what happened? You saw that the entire medical system went under struggle, face masks, ventilators, everything was missing. You started to read a little bit about supply chain. You start seeing Financial Times, Bloomberg, everybody started covering, Wired Magazine started talking about supply chain. We live in a time where the last few months have been terrible in terms of climate, forest fires everywhere, lots of floods. There’s an ongoing war. We are maybe far from it, but it’s causing a lot more disruption than any of us imagined. The other part which also happened, is one of the busiest waterways in the world is the Suez Canal, where a ship just decided to go sideways. For weeks, there was quite some struggle in getting the ship on track and looking at supply chain issues. When you look at these situations, and you ask yourself, how do we handle these events? You’re going to need a wee bit more than direct integration with ChatGPT. All of these problems cannot be solved by just enabling a large language model API.
Background
I’m Nischal. I am the Vice President of Data Science and Engineering at scoutbee. We’re based out of Berlin in Germany. I’ve been building enterprise AI and MLOps for the last 7 years, in the supply chain space for the last 3.
Before that, in the insurance and the med-tech industry. What’s the purpose of the talk? We’ve not found the only solution, we do think solving a problem of this scale requires a multitude of solutions. The goal is to present how we are enabling generative AI applications and enabling large language models as part of our product stack. As takeaways for all of you, the presentation is going to be broken down into two phases. The first phase is how we manage the entire data stack. The second is, how do you start thinking about reliability in large language models? How do you build safety nets, because the users are not consumer users, they’re business users. They’re used to reliability. They’re used to enterprise software. How do you bring that in the generative AI space? For those of you wondering, who are not working in this space, it might seem like we are in a big bubble that’s about to pop at some point. The market analyst thinks otherwise. Generative AI is here to stay. For at least the next 18 months, 67% of the IT leaders want to adopt generative AI. One-third of them want to make it their top priority.
Defensible Moats
A little bit about defensible moats before I jump into the data stack. Warren Buffett looks for economic castles protected by unreachable moats. He invests in companies that have these moats. Wardley mapping is a very interesting tool to think about strategy. You have evolution on the x axis, and you have the value chain on the left. A decade ago, I think in 2011, 2012, when I started working in the field of data science, everything that was related as an IP was basically feature engineering, statistical models, and you did a lot of regression work, and that was your IP. There was not a commodity. The data that went along with it was what you were actually focused on. A few years after that, the deep learning era kicked in, and we stopped wondering about all of the features that we handcrafted and built to serve our applications. That was not your moat anymore. Your moat was essentially thinking about networks, and how do you build your loss functions? It still required quite some data, so you still had a defensible moat if you had traffic and data coming in, but your features were not your moats anymore, it became a commodity. What’s happened with OpenAI coming up with ChatGPT, is that your deep learning models that were your IP also are not your IP anymore, you’re not in the race to build new models. Of course, there’s a lot of room for innovation in the deep learning space. At the moment, if you’re competing with the likes of ChatGPT, and Meta Llama 2, essentially, it’s a race that maybe a few companies can have because of the access to the data that these companies have, which means that you need to be smarter in terms of understanding, where do you want to build your moat?
What is the commodity that you can use off the shelf? Where do you get stronger? This has just been the journey of large language models in the last 2, 3 years. Just in the last 6 months, I think, as I started making this presentation to now, there’s probably two or three new large language models in this space. You can see that building these models themselves are not a defensible moat for us.
Full Data Stack – System of Records
The introduction to the full data stack. This is going to be broken down into three segments, system of record, system of intelligence, and system of engagement, based on the inspiration from a blog post called, “The New Moats,” moats that came out from Greylock ventures. For us, we’re bringing data for our customers, and our customers being the Unilever, Walmart, Audi, and a lot of these large organizations. For them, we bring data from different places. We bring data from ERP systems. I know a whole lot of you have forgotten that ERP exists, but they still do. Lots of companies run on very large ERP installations. They have document stores. They have a ton of custom data systems. You’d be shocked to understand how many custom data systems they have. A bunch of these systems are still not on the cloud, so they’re all sitting in data centers that are managed by a group of people. We saw a few years ago, there was a shortage of COBOL programmers as well. That’s because these systems are still powering a lot of these large organizations. What you see with this is not really a data mesh architecture in these organizations, actually a little bit of data mesh architecture. The data is duplicated. It’s incorrect. It’s not up to date. They have different complexities in their own space, and they’re heterogeneous, which means every enterprise customer we’re working with, and actually the teams within these organizations themselves, they’re all speaking a very different language. They’re all looking at the same data points in their space. It’s hard to understand what it means. The first thing we wanted to do, and we started this journey about two-and-a-half years ago, is to standardize this data language and build a semantic layer. We invested in this piece of technology, we call as the knowledge graph, which was championed by Google and Amazon and everybody in the previous decade. We put together a connection between the data points, and worked on the ontology, on creating this knowledge graph. In the Netflix talk, presented by Surabhi, she spoke a little bit about future proofing your technology and actually taking the bets and investing. This bet that we did two-and-a-half years ago actually started to pay off with the generative AI landscape. I’ll cover as we move through the presentation how that happened.
We couldn’t fit the ontology we have on a slide because it was just too big. This is to give you a sense of what a knowledge graph can look like. This is from the supply chain space. As you can see, there’s data coming from customers, third party, supply chain event, organizational data. The relationship between data points themselves completely change the meaning of the data. The good thing with knowledge graphs is that you’re actually trying to design how the real world works. You’re trying to design how entities in the real world look like. If you think about a manufacturing company that’s dependent on other manufacturing companies, putting them as a record in a relational database table is actually bad practice because they are a real-world entity. A real-world entity has more than just being a record in a database table. In order to enable these knowledge graphs, just briefly touching the technology part, we partner with Neo4j Aura. We are bringing our data in large batches with Apache Airflow. We’re doing data validations with Pydantic. We are scaling that processing with Polars. Polars and Pydantic are some of the new libraries in the Python landscape. We’ve done some data observability with Snowflake. Because we want to build a ton of different applications on top of this, we expose all of this data through GraphQL.
System of Intelligence
We’ll jump into the system of intelligence layers. We have a machine learning inference layer, and we have an agent-based framework. This is where things start to get interesting on the machine learning and the generative AI side. From the machine learning inference layer, so we’re doing the traditional machine learning workloads of converting unstructured data to structured data. We are running smaller transformer models, because we have 170 billion transformer models now. We’re doing something very small in the likes of RoBERTa, and a bunch of other language models to extract information that we think are appropriate for our domain. The scale at which this is operating is at web scale because we actually crawl the internet respectfully and ethically. We’re based out of Europe, there are GDPR laws that kick in. We’re looking at about a billion pages every 3 to 6 months. We’re extracting about 675 million entities. This builds our internal knowledge graph, about 3 billion relationships. They need to be refreshed every few months. That’s the traditional machine learning inference layer that we have. With generative AI kicking in, we started hosting an open source large language model called as Llama 2 from Meta. There’s a reason why we went with hosting something ourselves. The space that we operate in, without the access of system of records, there is very little value that we can actually bring to our customers. When you use ChatGPT and the likes without domain knowledge, without access to all of this internal information, you can bring some value, but the moment you want to start working with intellectual property, organizations don’t want to work with organizations where data is being shipped off somewhere. We are in a position where every single data point that we look at has to be managed and maintained by us. This added another challenge on our machine learning inference layer, which is, we look at a very different observability metric for supporting inferencing with large language models. We’re talking about, how many tokens can you process per millisecond? What’s your throughput of tokens per minute? How large a GPU do you need to actually run this model? Currently, we are running on one big machine, which is about 48GB GPUs. Then we are also running another flavor of it on SageMaker. This is the scale at which our machine learning inference layer has to work. In order to support this, we are working with using Hugging Face transformers library and a bunch of other packages from Hugging Face. We built our own with PyTorch. We’re running our ML workloads with Spark, our MLOps workflows with MLflow, and S3, and Snowflake. The thing that we started to realize the moment we started adding the LLM layer is that this ecosystem is not sufficient for us. We are starting to move away from Spark workloads to actually building machine learning inference workloads with Ray and Airflow. The other thing that’s coming up is we’re moving away from MLOps to LLMOps. I’ve put an asterisk there because I’ll talk about LLMOps in a non-traditional way as part of this presentation. Then the traditional way of LLMOps, which is still people are figuring out what that even means. That’s our machine learning inference layer.
As part of system of intelligence, we bring a new layer, which is actually very nascent, so the agent-based ecosystem. What are agent-based ecosystems, or what are generative AI apps? The ethos that we have at scoutbee is we want humans to be involved to review the outputs of accuracy, suss out bias, and actually ensure that these large language models are operating as intended. The goal for us is to not displace or replace humans, it’s for us to augment them with capabilities they didn’t have, so that they can actually use this to solve harder problems, than search for a data field across 100 different systems. That shouldn’t be the nature of work that people in these organizations have to do. What are we building as part of the agent-based ecosystem? We’re building conversational AI, supported by multi-agent architecture and RAGs. I’ll walk through each one of what that means. Before we jump into what is a multi-agent, what’s a typical agent structure? Who of you here have not worked with ChatGPT, or prompts, or any of this in the last few months? Just a quick introduction. When you’re thinking about an agent, when you write a prompt, or when you ask ChatGPT a question, what you’re typically doing is writing a prompt. When you think about an agent, an agent is something that you’re designing in order to solve a particular problem. This agent can have multiple prompts. You also provide a persona where you say, I am this so and so person. You want to keep the conversation clean, and you provide an instruction in terms of the problem this agent is actually designed to solve, and you give them a set of prompts that they have to look at in order to solve that problem. You can think about an agent as a person tasked with a job to perform, and giving them all the necessary tools and data to solve that problem. A multi-agent is, you go from one person to multiple specialized group of people. Instead of having one agent that does everything, you have multiple agents that talk to each other in order to solve a problem. You have an agent that is summarizing. You have an agent that does analytical work. Then, you have an agent that’s refining. A user creates a prompt and then the agents take over and help you solve that problem.
As part of the multi-agent architecture, I’ll talk about RAGs as well, is, in the multi-agent architecture, we use a flavor for every agent called as Re+Act, not to be confused with the web framework React. Re+Act actually stands for reason and act. Why reason and act? One of the things that the large language models do so much better and why they’re all the rage right now is, because of the amount of data they have been trained on, and because of the amount of prompts that have been generated by experts, they’ve built the capability to actually reason through their analysis and their solution. This is an example of what that looks like, is when you ask a large language model a question, what you can also force the large language model to do is to reason out how it got to that answer. It comes up with a thought. It acts on it. It observes from that thought what to do next, and builds a chain of thoughts until it reaches the final answer. Why did we have to do this? Our business users need to know why we are asking them the question that we are when we are trying to solve a problem. They need to know how we reached a certain solution, and not from going from A to B, because the wonderful thing that large language models can also do is they can be notorious at generating or hallucinating very coherently. It looks like the answer is real, but it’s factually incorrect, so you need a step of reasons to get there.
We spoke about conversational AI and multi-agent and the style that we’re using, and there was another acronym that I mentioned, they’re called as RAGs. RAG stands for Retrieval Augmented Generation. What this actually means is that large language models know a lot, but they have no idea or they have no access to the system of records that you actually have access to. In order to bring facts, in order to make sure large language models are not hallucinating all the answers, when you ask a certain question, you can also provide a small group of documents or a subset of documents that you think contain the answer to that question, to a large language model. The large language model then takes the question, takes a small set of documents that you’ve identified, and then using that, generates an answer with the facts presented in that document. All of the document question-answer, and the applications that people are building on top of their datasets, there’s a lot of work that’s going on in RAGs. That’s why vector databases are suddenly one of the coolest databases out there now, but they’ve been around for quite some time. We’ve used vector databases, not as vector databases, but we’ve been storing and working with vectors for quite some time. Now you have the capability of finding this subset of documents pretty quickly. Now, betting on being future proof and future ready, and investing in some technology where you go from strength to strength. We’re doing RAGs, not only with vector databases, I’ll talk about that next, but also with our knowledge graphs that we’ve built as part of our system of records. When a user is having this conversation, and they’re working with asking us questions, the thing that we can actually do, is because the knowledge graphs are representations of real-world entities with semantic meaning, we can actually convert that query and identify a subgraph on the knowledge graph that we can pick up to actually answer that question. The technology that we invested in, in the last two-and-a-half years now, has enabled us to build generative AI applications on top of it. We do the same thing with documents that we get, where we also have a vector database. It’s not one tool that does it all. We have different datastores. They come into action depending on the kind of question that’s being asked where the system of records actually live.
Quickly moving on from the agent-based ecosystem. This is a very common architecture pattern that you will see for most of the large language model applications that you’re building. You have your datastores sitting in different databases, and you need a way to connect to these different datastores quite easily. LlamaIndex is a framework that helps you do this. Your agents can make use of LlamaIndex to talk to this system of records. Your agents themselves, there’s been a lot of work that’s gone in the last year or so in coming up with frameworks such as LangChain, and there’s a lot of other OpenAI functions and everything that’s come out where we’ve designed the agents using that framework. Basically, your agents are talking to the user, and they are enabling an interaction with large language models. At the same time, whenever there is a requirement of data to answer the question, they’re making use of LlamaIndex. Of course, you can with something called as Llama Hub. We’ve not investigated or invested in Llama Hub because we bring data from a very different place. You could actually integrate all of the APIs from Slack, Notion, Salesforce, a bunch of these other places to bring data through Llama Hub.
The tools and technologies that we use as part of the agent-based ecosystem layer, is we work with LangChain. There’s a whole debate in the community if LangChain’s too bulky, if it’s something that you should use, not use. At the moment, it’s made our jobs a whole lot easier to work with large language models, especially given that we are running our own version of Llama 2 in our ecosystem. Then we have the Llama 2 from the Hugging Face library. We’ve also put it on AWS SageMaker. If you want to quickly get some of these models up and running, Hugging Face inference containers running on SageMaker with some of these models probably takes you 5 or 10 minutes to set up. It’s expensive, but it doesn’t take you much more than that to actually get that up and running. For our first set of applications that we started building, because we didn’t want to invest a whole lot of frontend engineers building the conversational apps, we started working with Streamlit. Streamlit is this framework for building Python data apps. You have the capability to spin up a conversational web app with I think, maybe 20 lines of code, and nothing more than that.
Systems of Engagement
Coming to our last layer of the data stack, we’re talking about systems of engagement. What part of the product are we actually enabling? How do we think about this from a product perspective? We’re looking at two very specific things as part of our product experience here. One is the fact that we’re working with a lot of data. It’s very easy to build complex user interface. With generative AI applications and the agent-based framework that we have, we’re navigating and helping users to move away from complex user interface, to something that is chat based. We’re not entirely chat based, we still have our own flavor of what we call as chat based. This is helping us solve tough problems or complex data problems for our users, by giving them a much neater, cleaner user experience. The second and the most important part of why generative AI is actually essential for us is, I’m not sure how many of you have multiple products as part of your platform layers, but for us, we have three different products. These three products are still in the same space, but they do some very specific set of operations. As a user, they have the necessity to actually use all three different products depending on the kind of problem they were solving. What we have enabled as part of generative AI is that the application that we are building helps us bridge the gap between all of these three different products. It actually helps the users to work through one interface. The application themselves make use of the features coming from different products as and when the user needs them. This is helping us stitch and build a good product, cohesiveness of a product rather than having different products for a narrow solution.
Why do we want to do this? There’s one part being part of the hype, which is good and fun, because the technology teams love it. When you’re building a business, and you want to think about the economies of scale, and you’re investing so much in generative AI and all of these experiments, what we essentially want to do and lead our users into a new direction is, our products were essentially places with the stack up until the machine learning inference, system of records, and product application layer, for a brief second if you just ignored the agent-based ecosystem, they would come and use our systems to search for data. They had a problem, they would come in to search for some data and look at the analytics, the dashboard, so on and so forth. We wanted to help and navigate them from that, to saying, I have this problem. I don’t know how to solve this problem, what can I do? We wanted to support them with all the data that they needed from the stack that we’ve put together.
Recap
We’re going from strength to strength. I think it’s very important to understand large language models, and generative AI should be a tool in your stack, and you still need your entire stack to drive value for your customers. Your defensible moats actually come from building combined power across the layers in the stack.
Feedback and Learnings
Once we did this, and we put a lot of effort in getting our first generative AI applications off the ground, we went into beta testing. This is where magic happened. We started getting feedback. The good thing that we got was our customers enjoyed the experience. They loved the fact that they could chat through an application, work with their data, so on and so forth, but there are concerns. They said, I asked the same question yesterday, and I asked the same question today, your system gives different answers, why? Some of the users are not native English speakers, and sometimes they’re using different text to express the same thing, but the application started behaving differently. They’re used to idempotency. They’re used to the fact that if they do the same things over again, the results they get should be the same. That’s not the case in the area of generative AI and large language models. They also spoke about the quality of conversation. They said, we want to solve a particular problem in this space, but the large language model actually thinks it is in a completely different domain. The intent of the conversation is misunderstood. We’ll talk a little bit about some of the findings that we had.
Reliability in the World of Probability
We realized that if we have to build a stronger moat here and drive value for our customers, we should be able to address their concerns around reliability and build something that is a domain expert, rather than something that is generic. Building trust with generative AI apps, it’s not the easiest things to do, and it’s a work in progress. Building reliability in the world of probabilities is not an easy thing. Enterprise product users are used to reliability in a good form and shape. Now with the generative AI use cases, they are extremely happy to use them, but it creates discomfort because now they have entered a new world of uncertainty of a probabilistic world. The other aspect that really scares them is the fact that the large language model can take you to very different destinations, depending on what you ask of the large language model to do. One could argue that this is the power of the LLMs, but it might not drive the intended value for your customers in an enterprise landscape. Train of thought, so as with humans, if you’re having a long enough conversation, and you ask things in a way that’s maybe confusing or challenging, large language models switch context. They can take you from trying to solve a problem in the supply chain space, to actually taking you to fairyland to write new stories about Narnia. You could really land in very different places with a large language model. The more you use, the more you realize how quickly the large language models can switch context. There’s also the challenge that you need to be able to switch between different agents. This is not trivial either. Large language models or agents can choose to not invoke other agents. The reason they choose to do that is they can start hallucinating. Instead of actually picking up data from the system of records and using that to solve a problem, they might just hallucinate the data themselves. They might say, we know about the supplier working out of the U.S., and the data looks so right that you might assume that it’s factually correct, but it’s not.
We didn’t want to go away from using large language models. We see the power, and we wanted to bring the best of both worlds, where we use the creative and innovation power of the LLMs. At the same time, have control and build reliability and analysis together. How do we start thinking about this? Where do we even go and how do we start? As we were asking ourselves this question, we stumbled upon this concept in large language models called as Graphs of Thought. What this concept is, is very similar to how humans think. Essentially, when you are asked a question, you are in a Bayesian world, you have many answers and many thoughts. Depending on who is asking this question, you go from Bayesian to frequentist, you choose a certain path and a certain answer. The next time when somebody else asks you the same question, you might actually choose a very different path. Depending on the path that you’ve chosen, you put together different thoughts in order to solve a problem. This is something that large language models do as well. What Graphs of Thought paper talks about, it comes from ETH Zürich, is the Re+Act part, which we were talking about, the reason and acting part. They talk about storing this reasoning as a graph, and using the humans to actually tell us if this reasoning is right, so you can fine-tune them later. Of course, this is not the path that we’ve gone into right now. What struck us was the fact that you can actually store the reasoning state, you can store the paths that your large language models have taken. We went from knowing that we have a problem, to actually thinking about the observability part of it, where we said, using this, we can start observing what the large language model is doing. Depending on what the large language model is doing, we can then decide, how do we want to fix it.
This was inspirational for us. It enabled us to think of a plan, to think about the execution as a graph that you could fine-tune over time. As a quick thought experiment, what we realized was, on one side, maybe with thinking about this as graph and controlling it, we can bring some reliability and we can avoid a bit of context switches. This still will not stop the large language model from hallucinating and misinterpreting intent, and being very confident at it. It’s very confident at being wrong. It almost feels like you’re the dumb person on the other side most of the time. You have to be very careful in knowing what’s really going on. Taking that inspiration, and looking at the observability and running these tests, what we realized was that the reasoning that large language models had, we realized there’s a big gap in domain understanding. We have a lot of business and domain knowledge that we couldn’t inject very easily into prompts, which is why you can see prompt engineering being one of the sought after fields right now, where I think salaries are crazy. I saw some organization, I think, paying out a million for some prompt engineer, and there was news all over it. Maybe it was fake or generated by LLM. Converting and taking all of your domain knowledge that is spread between hundreds of people in your organization across years, and bringing them into 1, 2, 10 prompts, is very challenging. You’re always going to miss a certain aspect to it. We saw that with the reasoning with large language models, it jumped very quickly from being told that you are a person working in the supply chain space solving this problem, to thinking that it’s an aeronautical engineer working for Lockheed Martin. It decided to go through different sorts of reasoning.
We said, ok, so we’re going to need some control here. We’re going to have to bring a lot of domain knowledge into this ecosystem. How do we do this? We’ve already worked with knowledge graphs before, so we thought maybe there is a way for us to build a new knowledge graph, which we can call the meta-data knowledge graph. Essentially, we took the problems that we wanted to solve, and from the business knowledge and the experts we had in that domain, put together an ontology that we can use in order to work with a large language model and design a meta-data domain knowledge graph. Essentially, what this knowledge graph has is a problem. All the subproblems that is required to solve that problem. The data the subproblems need in order to solve that problem. Essentially, it’s not a single path. It’s, again, a big graph. Depending on the way the user wants to solve that problem, large language models would essentially guide the user through that process. When you come up with something, and you’re working on it, you have this weird worm in your head that constantly tells you, you’re wrong. “You’re wrong. You’re not seeing something. You’re not thinking about this. Are you sure you want to invest your time and effort into this? What if it backfires?” I’m sure a lot of you are thinking about this when you’re building your large-scale machine learning models. A new paper got released called as Graph Neural Prompting, which talks about a very similar thing that we were doing. There was a big sigh of relief, and it was coincidental, so we were very happy that this came out. We are not doing the Graph Neural Prompting part, but, essentially, it talks about the approach that we take and where you have a very domain specific knowledge graph, you have your question, and you’re augmenting that with your large language model to solve the problems that you wish to solve.
What the meta-data graph would actually do, is that, based on the problem that our user wanted to solve in the particular domain, it would help traverse through a subgraph. The entire traversal itself to different subgraphs would be enabled by a large language model. We put together a quick implementation, and we validated this idea with our users, with our internal team. We had a new additional layer that went on to our data stack, as we speak. This was good. What we saw was that because we started bringing in a whole lot of domain knowledge, the number of context switches reduced. The large language model held a conversation together and tried to solve a complex problem, going step by step with the user, without jumping from one context or one domain to the other. We still did not get the intended reliability we were looking for. There are still hallucinations. In spite of all the nudging and prompting, large language models, at any given point in time, they knew they wanted to perform an action, they knew the data points they required to solve that problem. Essentially, they said, ok, we’ll hallucinate this for you.
How do we reduce the hallucinations? How do we choose the right subgraph? How do we switch the subgraphs in the middle of the conversation? The eyes cannot see what the mind does not know. Essentially, what we did, we went back to our observability. We started thinking about how we are storing the reasoning and managing our entire state. We did what humans do: when you come up with a conclusion, you want to verify if the conclusion that you’ve reached is right or wrong. We did this thing called as chain of verification. We didn’t know this was what it was actually called. We essentially said, at every single point in the work that we are doing, we have to verify and ask another set of prompts to say, is this the right thing that you’ve done? Do you understand the intent? Is this the right data to validate? This is what that looks like. When you ask an LLM, name some politicians who were born in New York. It comes up with a list of presidents. It says Hillary Clinton, Donald Trump, Michael Bloomberg. When you go and nudge the LLM to say, let’s verify this, where was Hillary Clinton born? Where was Donald Trump born, and Michael Bloomberg? You realize that the LLM says, Hillary Clinton was actually born in Chicago, Donald Trump in Queens, New York, and Michael Bloomberg in Boston. You realize the first answer it gave you was incorrect, because it picked up a very different understanding of what the question meant. With the chain of verification, you can actually verify every single action that you’re performing with your large language model. Because we had the meta-data graph, because we had the chain of verification in place, what we could do was to introduce a planner. One of the other aspects that was important for us, when we picked up the subgraph, one of the challenges with business users, and when you try to solve a big problem with generative AI applications, is that you don’t really know the road that the user is being asked to walk. Now that we had the process modeled on a graph, we showed the major milestones that the user has to go through much before the user actually hit the milestone. There was a certain aspect of certainty in using the generative AI application that guided our users and reduced their anxiety, that we have a 10-minute conversation to only realize we’ve made all the wrong choices in life. We didn’t want them to have that. Essentially, we introduced a planner in the mix.
With this, what we did was we unlocked another layer in our data stack. As you can see, just enabling a very lightweight integration into something like ChatGPT, or Anthropic, or any of these systems, you will not find the value that you can actually drive for your customers with a very simple, easy integration. You will have to build and invest quite a lot, if you want to build generative AI applications in the enterprise space that actually drives value. With these two layers, what we did was we brought in a little bit more reliability and predictability to our probabilistic world. We’re still in the process of measuring the appropriate baseline to see how we have improved. What we’ve seen from one of these papers is that with everything that we’ve taken, they’ve seen a 13.5% increase in the performance. If you fine-tuned it a little bit more, then close to about 15%, 16% of improvement. The other win that we had was that our data science team that’s been working on this with the first version of the apps, and when they handed it out to the users and the sales team, they were like, we have no idea what’s going to happen. Now with this, they’re like, we know what’s going to happen. We have some sense of reliability here. It’s predictable in the way it needs to behave, to a certain degree. This is still work in progress. The stage 2, we want to implement human in the loop feedback. We want to grow our meta-data graph with the combination of users and LLMs itself, so that essentially we have the wide array of the entire knowledge graph that’s there.
Summary
Without access to your full stack of data in building this entire thing, it might be very hard for you to build a defensible moat. Enabling LLMs requires a lot of effort. Making them reliable, predictable, and harmless requires way more effort and innovation. The generative AI space is starting up now. It’s a very exciting time we live in. With great power comes great responsibility, so all of us practitioners have to make sure to take the reliability, predictability, and observability part quite seriously, and make LLMs safer for everyone to use.
Questions and Answers
Participant 1: I saw that you moved back to a single agent architecture from multi-agent. Of course, recently, the developments in that space with Auto-GPT, MetaGPT especially this stuff from Microsoft, and I see a general trend from, as much as single agents having lots of specialized agents, do you guys think you’re going to return back to that in the future, augment things like setting the operating procedures for your communication. What are your thoughts on that direction?
HP: What we did was we reduced the amount of space that a large language model can operate in. Basically, to increase the reliability and predictability, we started adding constraints. When we started adding constraints, we saw that we didn’t really need the switch to go from one agent to the other. Because at any given point in time, the meta-data knowledge graph is nudging and prompting the agent to know what is the task it needs to solve. We’re basically building prompts on the fly, depending on how the user is navigating to solve the problem. We don’t really need different agents, because one agent does everything. It knows what to summarize. It knows when to go into large text prompting, when to provide a list, when to go pick up from a system of records. It is being guided by the meta-data knowledge graph and the chain of verification. When we didn’t have those two things, we definitely couldn’t make do with a single agent, we had to do multi-agent. It depends on the domain that you’re working and how much knowledge you have in that domain to design that graph. If you’re working in a very open-ended space, then I think it is good to have multi-agent than single agent.
Participant 2: I know a lot of the stuff that we’re building have latencies [inaudible 00:52:17], and then when you add a verification layer, so the latency question, so I was wondering how you deal with that.
HP: You can solve all the problems, making it a technical engineering one, or you can solve the problem as a combination of user experience, product experience, and the engineering space. When the verification is happening, we let the user know the verification that is happening by constantly providing them a summary. We say, this is the problem you want to solve. If you’re having this long conversation, we guide them through having the summary on the side, saying, you started with this problem, you gave us this input, this is what we are trying to do. Now we are constantly expanding that, as and when you’re going through the conversation. That user experience that we enabled helps us in a way to mask the fact that you need millisecond latency. You have to be a little smart in how you handle this, but that’s helping us now. Let’s talk when this is hitting production and there are hundreds of people using this.
See more presentations with transcripts
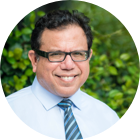
MMS • RSS
Posted on mongodb google news. Visit mongodb google news
MongoDB, Inc. (NASDAQ:MDB – Get Free Report) Director John Dennis Mcmahon sold 10,000 shares of MongoDB stock in a transaction on Monday, June 24th. The stock was sold at an average price of $228.00, for a total transaction of $2,280,000.00. Following the sale, the director now directly owns 20,020 shares of the company’s stock, valued at approximately $4,564,560. The transaction was disclosed in a legal filing with the SEC, which is accessible through the SEC website.
MongoDB Stock Performance
Shares of MDB stock opened at $244.15 on Friday. MongoDB, Inc. has a 12 month low of $214.74 and a 12 month high of $509.62. The company’s fifty day simple moving average is $306.14 and its 200-day simple moving average is $367.45. The firm has a market cap of $17.91 billion, a PE ratio of -86.89 and a beta of 1.13. The company has a quick ratio of 4.93, a current ratio of 4.93 and a debt-to-equity ratio of 0.90.
MongoDB (NASDAQ:MDB – Get Free Report) last posted its quarterly earnings data on Thursday, May 30th. The company reported ($0.80) EPS for the quarter, hitting analysts’ consensus estimates of ($0.80). The company had revenue of $450.56 million during the quarter, compared to the consensus estimate of $438.44 million. MongoDB had a negative net margin of 11.50% and a negative return on equity of 14.88%. Equities research analysts expect that MongoDB, Inc. will post -2.67 EPS for the current fiscal year.
Analyst Ratings Changes
A number of equities research analysts recently commented on MDB shares. Redburn Atlantic reiterated a “sell” rating and issued a $295.00 price objective (down previously from $410.00) on shares of MongoDB in a report on Tuesday, March 19th. Stifel Nicolaus cut their price target on shares of MongoDB from $435.00 to $300.00 and set a “buy” rating on the stock in a research report on Friday, May 31st. Tigress Financial boosted their price objective on shares of MongoDB from $495.00 to $500.00 and gave the company a “buy” rating in a report on Thursday, March 28th. Monness Crespi & Hardt upgraded shares of MongoDB to a “hold” rating in a report on Tuesday, May 28th. Finally, Robert W. Baird reduced their price target on shares of MongoDB from $450.00 to $305.00 and set an “outperform” rating on the stock in a research report on Friday, May 31st. One equities research analyst has rated the stock with a sell rating, five have assigned a hold rating, nineteen have given a buy rating and one has given a strong buy rating to the company. Based on data from MarketBeat, the company has a consensus rating of “Moderate Buy” and an average target price of $361.30.
Read Our Latest Stock Report on MDB
Institutional Inflows and Outflows
Several institutional investors have recently made changes to their positions in MDB. Transcendent Capital Group LLC bought a new position in shares of MongoDB in the fourth quarter valued at $25,000. Blue Trust Inc. increased its stake in shares of MongoDB by 937.5% during the 4th quarter. Blue Trust Inc. now owns 83 shares of the company’s stock worth $34,000 after purchasing an additional 75 shares during the last quarter. Beacon Capital Management LLC lifted its stake in MongoDB by 1,111.1% in the 4th quarter. Beacon Capital Management LLC now owns 109 shares of the company’s stock valued at $45,000 after purchasing an additional 100 shares during the last quarter. YHB Investment Advisors Inc. acquired a new position in MongoDB during the first quarter worth approximately $41,000. Finally, GAMMA Investing LLC bought a new position in shares of MongoDB during the 4th quarter worth approximately $50,000. Institutional investors and hedge funds own 89.29% of the company’s stock.
MongoDB Company Profile
MongoDB, Inc, together with its subsidiaries, provides general purpose database platform worldwide. The company provides MongoDB Atlas, a hosted multi-cloud database-as-a-service solution; MongoDB Enterprise Advanced, a commercial database server for enterprise customers to run in the cloud, on-premises, or in a hybrid environment; and Community Server, a free-to-download version of its database, which includes the functionality that developers need to get started with MongoDB.
Featured Stories
Receive News & Ratings for MongoDB Daily – Enter your email address below to receive a concise daily summary of the latest news and analysts’ ratings for MongoDB and related companies with MarketBeat.com’s FREE daily email newsletter.
Article originally posted on mongodb google news. Visit mongodb google news
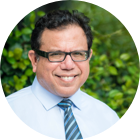
MMS • Sergio De Simone
Article originally posted on InfoQ. Visit InfoQ
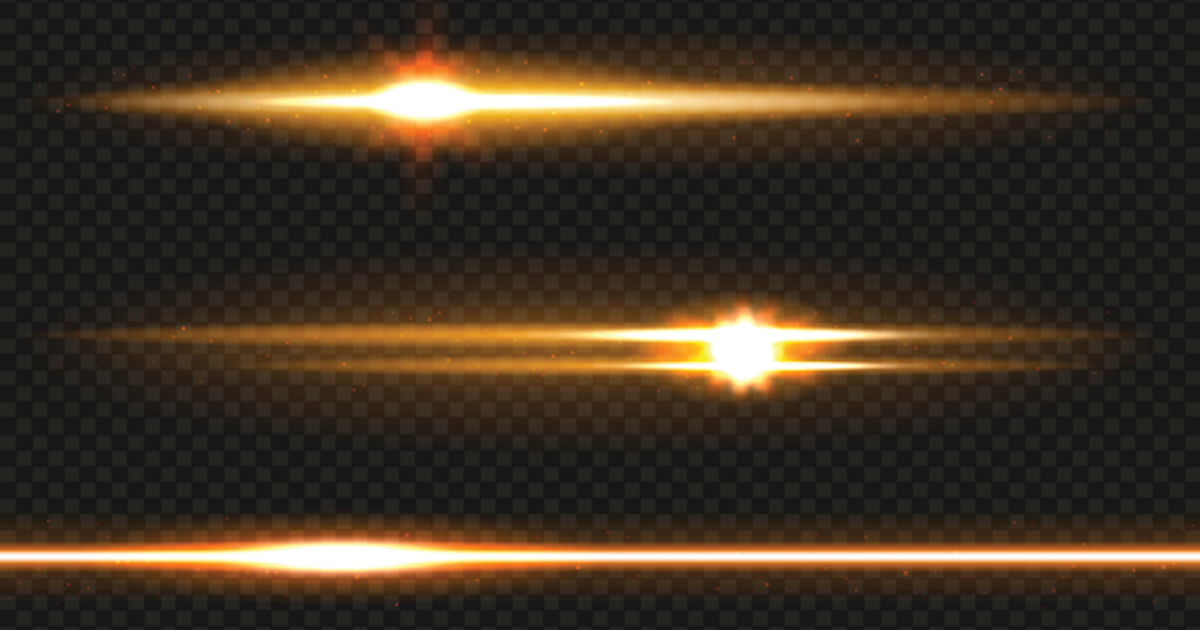
In his WWDC 2024 talk, Apple’s Languages and Runtimes team lead and Swift Core Team member Ted Kremenek introduced the language’s new data-race safe mode, which promises to help developers create concurrent programs free of data races thanks to a new compile-time static detector.
As Kremenek explains, the road to data-race safety has been paved across several Swift versions, starting with the introduction of async/await
and Actors in Swift 5.5, sendable
distributed actors in Swift 5.6, custom executors and isolation assertions in Swift 5.9, and finally full-data isolation and isolated globals in Swift 5.10.
All those features come together in a new opt-in compiler mode that enables full data-race safety. Swift 6 makes concurrent programming dramatically easier, says Kremenek, by identifying data-race conditions at compile time to prevent different parts of the code from accessing and modifying shared data.
The reason for this new mode being opt-in is that data-race safety may require changes to existing code— albeit in many cases they will be “narrow”, says Kremenek —so developers can decide when it is best to enable the new mode to tackle any data-race issues their code might have.
Alternatively, developers can opt-in to the new mode on a per-module basis, which is possible by enabling the compiler’s actor isolation and Sendable
checking as warnings while still using the Swift 5 language mode.
Apple also provided a few guidelines to help developers through the process of migrating an existing project to Swift 6 and deal in an orderly way with the seemingly huge amount of warnings that the compiler may generate.
The main idea is to migrate modules one by one, starting with those that are less depended upon by other modules. This will make most of the required changes local to the module. Another useful criterion to select which modules to start with is considering whether any modules include unsafe global state or trivially-Sendable types, since those can cause many warnings across a project.
If the number of warnings is still too high to be tackled comfortably, the Swift compiler provides three finer-grained switches to focus on specific types of problems. You can enable them one at a time as a progressive path towards enabling complete concurrency checking. They include removing Actor Isolation Inference caused by Property Wrappers, applying strict concurrency for global variables, and inferring Sendable for methods and key path literals.
While it is well understood how global variables may be a cause of problems in a concurrent context, the other two compiler options address subtler behaviors that are specific to Swift. In particular, property wrappers may change how the Swift compiler infers isolation for the container class, in a rather opaque way to the developer and this would bring unexpected potential issues (or warnings, when the static checker is enabled). Similarly, inferring sendability for partial and unapplied methods as well as key path literals addresses a few corner cases. You can get the full discussion in the linked Swift Evolution Proposals.
As a final note, if your Swift codebase is not yet up to date with the concurrency features brought by the latest language releases, now is the time to start using them. Apple suggests adopting advanced concurrency support incrementally, starting with wrapping callback-based functions to make them directly usable from an async context, adopting isolation for your classes temporarily using internal-only isolation, and so on.
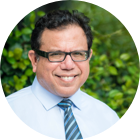
MMS • RSS
Posted on mongodb google news. Visit mongodb google news
MongoDB, Inc. (NASDAQ:MDB – Get Free Report) Director Dwight A. Merriman sold 1,000 shares of the firm’s stock in a transaction on Thursday, June 27th. The shares were sold at an average price of $245.00, for a total transaction of $245,000.00. Following the sale, the director now directly owns 1,146,003 shares of the company’s stock, valued at $280,770,735. The transaction was disclosed in a document filed with the SEC, which is available at this link.
MongoDB Stock Performance
MDB stock traded up $3.63 during trading on Thursday, reaching $244.15. 2,169,590 shares of the company’s stock were exchanged, compared to its average volume of 1,534,545. MongoDB, Inc. has a 1-year low of $214.74 and a 1-year high of $509.62. The firm has a market cap of $17.91 billion, a PE ratio of -85.59 and a beta of 1.13. The company’s fifty day moving average price is $307.97 and its 200-day moving average price is $368.31. The company has a quick ratio of 4.93, a current ratio of 4.93 and a debt-to-equity ratio of 0.90.
MongoDB (NASDAQ:MDB – Get Free Report) last posted its quarterly earnings data on Thursday, May 30th. The company reported ($0.80) earnings per share for the quarter, hitting the consensus estimate of ($0.80). The business had revenue of $450.56 million during the quarter, compared to the consensus estimate of $438.44 million. MongoDB had a negative net margin of 11.50% and a negative return on equity of 14.88%. On average, research analysts expect that MongoDB, Inc. will post -2.67 EPS for the current fiscal year.
Institutional Inflows and Outflows
A number of hedge funds have recently made changes to their positions in the stock. Norges Bank bought a new position in shares of MongoDB during the 4th quarter valued at about $326,237,000. Jennison Associates LLC grew its position in MongoDB by 14.3% in the first quarter. Jennison Associates LLC now owns 4,408,424 shares of the company’s stock worth $1,581,037,000 after buying an additional 551,567 shares during the last quarter. Axiom Investors LLC DE acquired a new position in MongoDB in the fourth quarter worth approximately $153,990,000. Swedbank AB bought a new position in shares of MongoDB in the first quarter valued at $91,915,000. Finally, Clearbridge Investments LLC lifted its holdings in shares of MongoDB by 109.0% during the first quarter. Clearbridge Investments LLC now owns 445,084 shares of the company’s stock valued at $159,625,000 after purchasing an additional 232,101 shares during the last quarter. Institutional investors and hedge funds own 89.29% of the company’s stock.
Analyst Ratings Changes
Several research firms recently commented on MDB. Mizuho cut their price target on MongoDB from $380.00 to $250.00 and set a “neutral” rating on the stock in a research note on Friday, May 31st. Piper Sandler reduced their target price on MongoDB from $480.00 to $350.00 and set an “overweight” rating for the company in a research note on Friday, May 31st. Needham & Company LLC reaffirmed a “buy” rating and issued a $290.00 price target on shares of MongoDB in a report on Thursday, June 13th. Redburn Atlantic reissued a “sell” rating and issued a $295.00 target price (down previously from $410.00) on shares of MongoDB in a research report on Tuesday, March 19th. Finally, Loop Capital reduced their price target on shares of MongoDB from $415.00 to $315.00 and set a “buy” rating on the stock in a research report on Friday, May 31st. One investment analyst has rated the stock with a sell rating, five have issued a hold rating, nineteen have given a buy rating and one has given a strong buy rating to the stock. According to MarketBeat.com, MongoDB has an average rating of “Moderate Buy” and a consensus target price of $361.30.
Get Our Latest Stock Report on MongoDB
About MongoDB
MongoDB, Inc, together with its subsidiaries, provides general purpose database platform worldwide. The company provides MongoDB Atlas, a hosted multi-cloud database-as-a-service solution; MongoDB Enterprise Advanced, a commercial database server for enterprise customers to run in the cloud, on-premises, or in a hybrid environment; and Community Server, a free-to-download version of its database, which includes the functionality that developers need to get started with MongoDB.
See Also
This instant news alert was generated by narrative science technology and financial data from MarketBeat in order to provide readers with the fastest and most accurate reporting. This story was reviewed by MarketBeat’s editorial team prior to publication. Please send any questions or comments about this story to contact@marketbeat.com.
Before you consider MongoDB, you’ll want to hear this.
MarketBeat keeps track of Wall Street’s top-rated and best performing research analysts and the stocks they recommend to their clients on a daily basis. MarketBeat has identified the five stocks that top analysts are quietly whispering to their clients to buy now before the broader market catches on… and MongoDB wasn’t on the list.
While MongoDB currently has a “Moderate Buy” rating among analysts, top-rated analysts believe these five stocks are better buys.
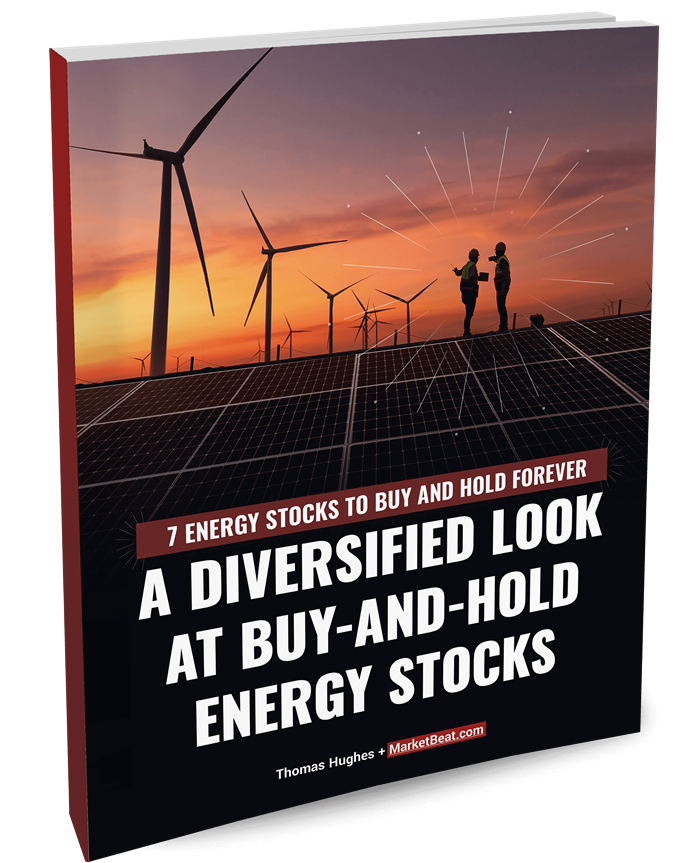
Do you expect the global demand for energy to shrink?! If not, it’s time to take a look at how energy stocks can play a part in your portfolio.
Article originally posted on mongodb google news. Visit mongodb google news
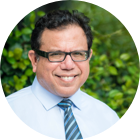
MMS • RSS
Posted on mongodb google news. Visit mongodb google news
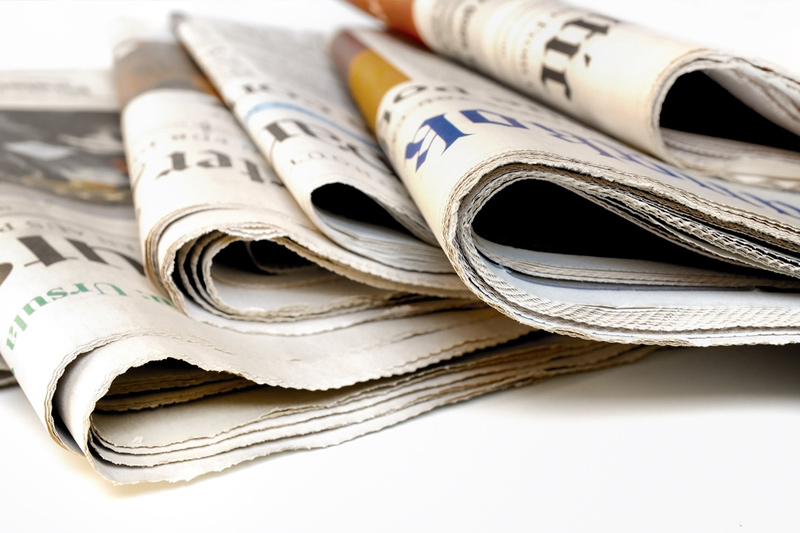
On June 25, Merriman sold 598 shares at a price of $226.31 per share. Following this transaction, his direct ownership in the company stood at 1,146,186 shares. Two days later, on June 27, he sold an additional 1,000 shares, priced at $245.00 each. After these sales, Merriman’s direct holdings in MongoDB decreased slightly to 1,146,003 shares.
The filings also revealed that Merriman acquired 817 restricted stock units (RSUs) as part of the company’s non-employee director compensation policy. These units represent the right to receive an equivalent number of shares of Class A common stock and are set to vest on the earlier of the first anniversary of the grant date or the date of MongoDB’s 2025 annual stockholders’ meeting, provided Merriman continues his service to the company.
In addition to his direct holdings, Merriman has indirect ownership through The Dwight A. Merriman 2012 Trust, which benefits his children and holds 522,896 shares. Another 95,000 shares are held by The Dwight A. Merriman Charitable Foundation, over which Merriman has voting and investment power.
The sales were made in accordance with a Rule 10b5-1 trading plan, a mechanism that allows company insiders to sell shares at predetermined times to avoid accusations of insider trading.
Investors and MongoDB watchers may keep an eye on insider transactions as they often seek to understand the confidence levels of company executives and directors in the business’s prospects.
In other recent news, MongoDB has been the subject of multiple analyst adjustments following its first-quarter earnings report. KeyBanc maintained its Overweight rating on MongoDB with a steady price target of $278, highlighting MongoDB’s potential in the medium term due to its offerings in online transaction processing databases and vector search capabilities. Meanwhile, Scotiabank reduced its price target for MongoDB to $250, maintaining a “Sector Perform” rating, advising investors to adopt a “wait and see” approach due to a slower operational start and more moderate activity from end-users.
Citi also reduced its price target for MongoDB to $350 while maintaining a Buy rating, citing weaker consumption trends and the smallest revenue beat in the company’s history. However, Citi remains optimistic about MongoDB’s potential for growth in the second half of the year. Guggenheim upgraded MongoDB stock from Sell to Neutral, attributing the downgrade in guidance and the company’s performance to temporary go-to-market headwinds rather than broader macroeconomic issues.
Baird adjusted its price target on MongoDB shares to $305 while keeping its Outperform rating, expressing confidence in MongoDB’s long-term potential, especially in the area of artificial intelligence workloads. Lastly, Piper Sandler reduced its price target for MongoDB to $350 while retaining an Overweight rating, acknowledging macroeconomic challenges but considering MongoDB’s year-to-date decline as a more attractive risk-reward balance. These are the recent developments in MongoDB’s financial outlook.
As MongoDB, Inc. (NASDAQ:MDB) navigates the dynamic landscape of database technology, recent insider transactions have drawn attention to the company’s financial health and future prospects. Reflecting on the recent stock sales by Director Dwight A. Merriman, it’s valuable to consider the broader financial context of MongoDB as revealed by InvestingPro data and tips.
An intriguing highlight from the InvestingPro Tips is MongoDB’s position of holding more cash than debt on its balance sheet, which suggests a strong liquidity position that could support the company’s growth initiatives and provide a buffer against market volatility. Moreover, despite the stock’s recent price fluctuations, analysts forecast that MongoDB will be profitable this year, indicating potential for the company’s financial turnaround and the realization of its strategic goals.
From the InvestingPro Data, MongoDB’s market capitalization stands at $17.91 billion, underscoring its significant presence in the industry. The company’s revenue growth remains robust with a 29.15% increase over the last twelve months as of Q1 2023, reflecting its ability to expand its market share and innovate its offerings. However, the company’s P/E ratio is currently negative at -87.37, which may raise concerns about valuation among investors, especially in light of the fact that the stock is trading at a high Price/Book multiple of 14.11.
Given the mixed signals from the market performance and financial metrics, investors may find it beneficial to explore the full range of InvestingPro Tips available for MongoDB. With 20 analysts having revised their earnings downwards for the upcoming period, it’s crucial to stay informed on the latest analyses and forecasts. Subscribers to InvestingPro can access these insights and more, and by using the coupon code PRONEWS24, new users can receive an additional 10% off a yearly or biyearly Pro and Pro+ subscription.
To gain a deeper understanding of MongoDB’s trajectory and to make more informed investment decisions, readers are encouraged to explore the comprehensive list of 13 additional InvestingPro Tips available on the platform.
This article was generated with the support of AI and reviewed by an editor. For more information see our T&C.
Article originally posted on mongodb google news. Visit mongodb google news
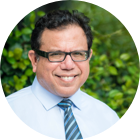
MMS • RSS
Posted on mongodb google news. Visit mongodb google news
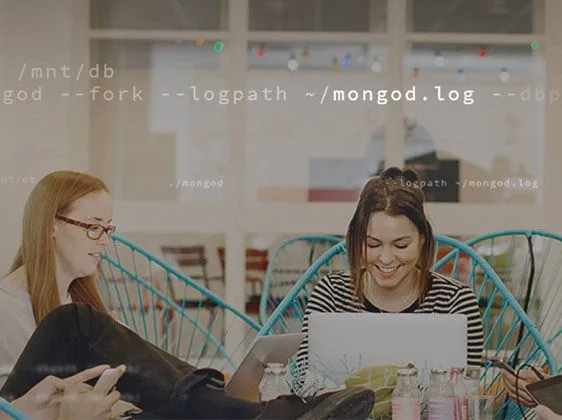
The worldwide data management software market is massive (According to IDC, the worldwide database software market, which it refers to as the database management systems software market, was forecasted to be approximately $82 billion in 2023 growing to approximately $137 billion in 2027. This represents a 14% compound annual growth rate). At MongoDB we are transforming industries and empowering developers to build amazing apps that people use every day. We are the leading developer data platform and the first database provider to IPO in over 20 years. Join our team and be at the forefront of innovation and creativity.
MongoDB is hiring a Senior Manager, Employee Experience to join our Americas Employee Experience organization. The role will report into the Senior Director of Americas Employee Experience and will be responsible for scaling business processes while supporting employees and managers to optimize the experience of working for MongoDB. You will be the face of the People Team to MongoDB employees and managers serving as the main point of contact for escalated inquiries and concerns, performance management, employee well-being, and regional compliance. This role requires a proactive and collaborative individual with excellent communication skills, capable of leading and guiding the HR team in maintaining a compliant and ethical workplace. This is a new role being created to support MongoDB’s continued growth and expansion.
The Employee Experience Team at MongoDB is the face of HR to the approximately 5,000 employees globally. The team is responsible for providing full employee life cycle service delivery from onboarding to separation management in partnership with the rest of the People Team including the following CoEs: Recruiting, Total Rewards, Learning & Development, Employee Engagement & Inclusion, HR Business Partnering and HR Operations. The team is also responsible for our Workplace sustainability and community/events efforts, Employee Relations, policy and process enhancements including regional compliance as well as developing programs to enhance manager capability across the company.
Key Responsibilities:
- Partnership: Partner with global HRBPs and COEs to support achieving the people goals of assigned business units in the Americas
- Service Delivery: Serve as the main point of contact for escalated employee and people manager questions and concerns for your assigned business unit
- Performance Management: Coach and enable managers to set clear expectations, provide regular feedback, and manage employee performance
- Employee Relations: Support our commitment to a safe and balanced workplace by evaluating employee complaints to make appropriate recommendations to address the matter. Conduct mediation sessions when necessary
- Process Improvement: Develop and evolve MongoDB People processes and systems to continue elevating the employee and manager experience
- Management: Effectively lead and manage direct report(s), fostering a collaborative and productive work environment while actively supporting their professional development
- Compliance: Stay updated on employment law and current legislation related to Human Resources, providing valuable advice to managers, identifying risks, and suggesting alternative solutions. Contribute to the evaluation, development, and enhancement of company policies as needed
- Culture Ambassador: Focus on manager and leadership enablement; support and promote a values-based culture and effective hybrid working environment in partnership with Workplace and local leadership
- Coaching: Act as a trusted advisor, applying your HR expertise and understanding of MongoDB’s business to effectively partner with leaders to provide guidance, support, and coaching to drive people development and business results
- Knowledge: Maintains knowledge of trends, best practices, regulatory changes, and new technologies in human resources, talent management, and employment law
Requirements
- Bachelor’s degree in HR or related field with at least 10 years of well-rounded experience in progressive HR roles with at least 5 years in a leadership position. Must have strong performance management, coaching, business partnership, and HR operations background. Experience in a high growth technology business is a plus. Relevant certifications are a double plus
- Exceptional communication and interpersonal skills with the ability to influence and engage others
- Strong understanding and working knowledge of employment laws in the US is a must, combined with the proven ability to interpret and guide employment matters, manage grievances, disputes and investigations in alignment with local employment law and regulations
- Passion for & demonstrated expertise in developing and implementing HR programs and driving operational excellence for a high growth and complex company. We are still building the bridge as we walk, and we need someone who has the organizational & project management skills that are required to do that and enjoys the exhilaration that comes with it
- Critical thinking skills are important. This includes the ability to analyze a situation or problem, identify the root cause, break solutions down into achievable milestones and make informed data-driven decisions
- Adaptability and flexibility for two reasons – we are a company that is scaling in a market that is constantly evolving and this role partners with employees at all levels of the organization. You will need to shift regularly between tactical and strategic priorities, deal with a lot of change and focus on driving outcomes across a wide range of levels in the company
- Ability to handle sensitive and confidential information with discretion
- You need to be comfortable in an accelerated learning environment and be self-motivated and assertive to succeed
To drive the personal growth and business impact of our employees, we’re committed to developing a supportive and enriching culture for everyone. From employee affinity groups, to fertility assistance and a generous parental leave policy, we value our employees’ wellbeing and want to support them along every step of their professional and personal journeys. Learn more about what it’s like to work at MongoDB, and help us make an impact on the world!
MongoDB is committed to providing any necessary accommodations for individuals with disabilities within our application and interview process. To request an accommodation due to a disability, please inform your recruiter.
MongoDB, Inc. provides equal employment opportunities to all employees and applicants for employment and prohibits discrimination and harassment of any type and makes all hiring decisions without regard to race, color, religion, age, sex, national origin, disability status, genetics, protected veteran status, sexual orientation, gender identity or expression, or any other characteristic protected by federal, state or local laws.
MongoDB’s base salary range for this role is posted below. Compensation at the time of offer is unique to each candidate and based on a variety of factors such as skill set, experience, qualifications, and work location. Salary is one part of MongoDB’s total compensation and benefits package. Other benefits for eligible employees may include: equity, participation in the employee stock purchase program, flexible paid time off, 20 weeks fully-paid gender-neutral parental leave, fertility and adoption assistance, 401(k) plan, mental health counseling, access to transgender-inclusive health insurance coverage, and health benefits offerings. Please note, the base salary range listed below and the benefits in this paragraph are only applicable to U.S.-based candidates.
MongoDB’s base salary range for this role in the U.S. is:
$81,000—$112,000 USD
Article originally posted on mongodb google news. Visit mongodb google news
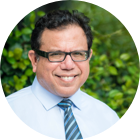
MMS • RSS
Posted on mongodb google news. Visit mongodb google news
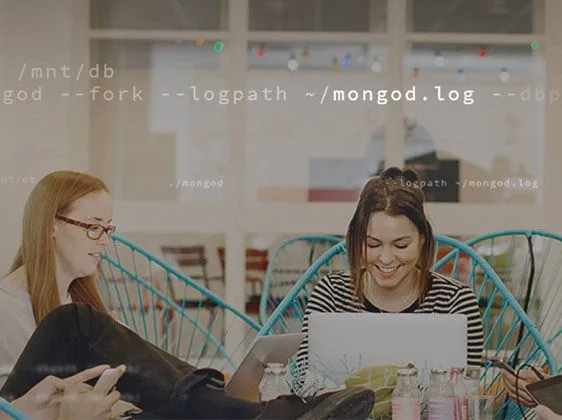
The worldwide data management software market is massive (According to IDC, the worldwide database software market, which it refers to as the database management systems software market, was forecasted to be approximately $82 billion in 2023 growing to approximately $137 billion in 2027. This represents a 14% compound annual growth rate). At MongoDB we are transforming industries and empowering developers to build amazing apps that people use every day. We are the leading developer data platform and the first database provider to IPO in over 20 years. Join our team and be at the forefront of innovation and creativity.
MongoDB is hiring a Senior Manager, Employee Experience to join our Americas Employee Experience organization. The role will report into the Senior Director of Americas Employee Experience and will be responsible for scaling business processes while supporting employees and managers to optimize the experience of working for MongoDB. You will be the face of the People Team to MongoDB employees and managers serving as the main point of contact for escalated inquiries and concerns, performance management, employee well-being, and regional compliance. This role requires a proactive and collaborative individual with excellent communication skills, capable of leading and guiding the HR team in maintaining a compliant and ethical workplace. This is a new role being created to support MongoDB’s continued growth and expansion.
The Employee Experience Team at MongoDB is the face of HR to the approximately 5,000 employees globally. The team is responsible for providing full employee life cycle service delivery from onboarding to separation management in partnership with the rest of the People Team including the following CoEs: Recruiting, Total Rewards, Learning & Development, Employee Engagement & Inclusion, HR Business Partnering and HR Operations. The team is also responsible for our Workplace sustainability and community/events efforts, Employee Relations, policy and process enhancements including regional compliance as well as developing programs to enhance manager capability across the company.
Key Responsibilities:
- Partnership: Partner with global HRBPs and COEs to support achieving the people goals of assigned business units in the Americas
- Service Delivery: Serve as the main point of contact for escalated employee and people manager questions and concerns for your assigned business unit
- Performance Management: Coach and enable managers to set clear expectations, provide regular feedback, and manage employee performance
- Employee Relations: Support our commitment to a safe and balanced workplace by evaluating employee complaints to make appropriate recommendations to address the matter. Conduct mediation sessions when necessary
- Process Improvement: Develop and evolve MongoDB People processes and systems to continue elevating the employee and manager experience
- Management: Effectively lead and manage direct report(s), fostering a collaborative and productive work environment while actively supporting their professional development
- Compliance: Stay updated on employment law and current legislation related to Human Resources, providing valuable advice to managers, identifying risks, and suggesting alternative solutions. Contribute to the evaluation, development, and enhancement of company policies as needed
- Culture Ambassador: Focus on manager and leadership enablement; support and promote a values-based culture and effective hybrid working environment in partnership with Workplace and local leadership
- Coaching: Act as a trusted advisor, applying your HR expertise and understanding of MongoDB’s business to effectively partner with leaders to provide guidance, support, and coaching to drive people development and business results
- Knowledge: Maintains knowledge of trends, best practices, regulatory changes, and new technologies in human resources, talent management, and employment law
Requirements
- Bachelor’s degree in HR or related field with at least 10 years of well-rounded experience in progressive HR roles with at least 5 years in a leadership position. Must have strong performance management, coaching, business partnership, and HR operations background. Experience in a high growth technology business is a plus. Relevant certifications are a double plus
- Exceptional communication and interpersonal skills with the ability to influence and engage others
- Strong understanding and working knowledge of employment laws in the US is a must, combined with the proven ability to interpret and guide employment matters, manage grievances, disputes and investigations in alignment with local employment law and regulations
- Passion for & demonstrated expertise in developing and implementing HR programs and driving operational excellence for a high growth and complex company. We are still building the bridge as we walk, and we need someone who has the organizational & project management skills that are required to do that and enjoys the exhilaration that comes with it
- Critical thinking skills are important. This includes the ability to analyze a situation or problem, identify the root cause, break solutions down into achievable milestones and make informed data-driven decisions
- Adaptability and flexibility for two reasons – we are a company that is scaling in a market that is constantly evolving and this role partners with employees at all levels of the organization. You will need to shift regularly between tactical and strategic priorities, deal with a lot of change and focus on driving outcomes across a wide range of levels in the company
- Ability to handle sensitive and confidential information with discretion
- You need to be comfortable in an accelerated learning environment and be self-motivated and assertive to succeed
To drive the personal growth and business impact of our employees, we’re committed to developing a supportive and enriching culture for everyone. From employee affinity groups, to fertility assistance and a generous parental leave policy, we value our employees’ wellbeing and want to support them along every step of their professional and personal journeys. Learn more about what it’s like to work at MongoDB, and help us make an impact on the world!
MongoDB is committed to providing any necessary accommodations for individuals with disabilities within our application and interview process. To request an accommodation due to a disability, please inform your recruiter.
MongoDB, Inc. provides equal employment opportunities to all employees and applicants for employment and prohibits discrimination and harassment of any type and makes all hiring decisions without regard to race, color, religion, age, sex, national origin, disability status, genetics, protected veteran status, sexual orientation, gender identity or expression, or any other characteristic protected by federal, state or local laws.
MongoDB’s base salary range for this role is posted below. Compensation at the time of offer is unique to each candidate and based on a variety of factors such as skill set, experience, qualifications, and work location. Salary is one part of MongoDB’s total compensation and benefits package. Other benefits for eligible employees may include: equity, participation in the employee stock purchase program, flexible paid time off, 20 weeks fully-paid gender-neutral parental leave, fertility and adoption assistance, 401(k) plan, mental health counseling, access to transgender-inclusive health insurance coverage, and health benefits offerings. Please note, the base salary range listed below and the benefits in this paragraph are only applicable to U.S.-based candidates.
MongoDB’s base salary range for this role in the U.S. is:
$81,000—$112,000 USD
Article originally posted on mongodb google news. Visit mongodb google news
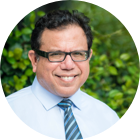
MMS • RSS
Posted on nosqlgooglealerts. Visit nosqlgooglealerts
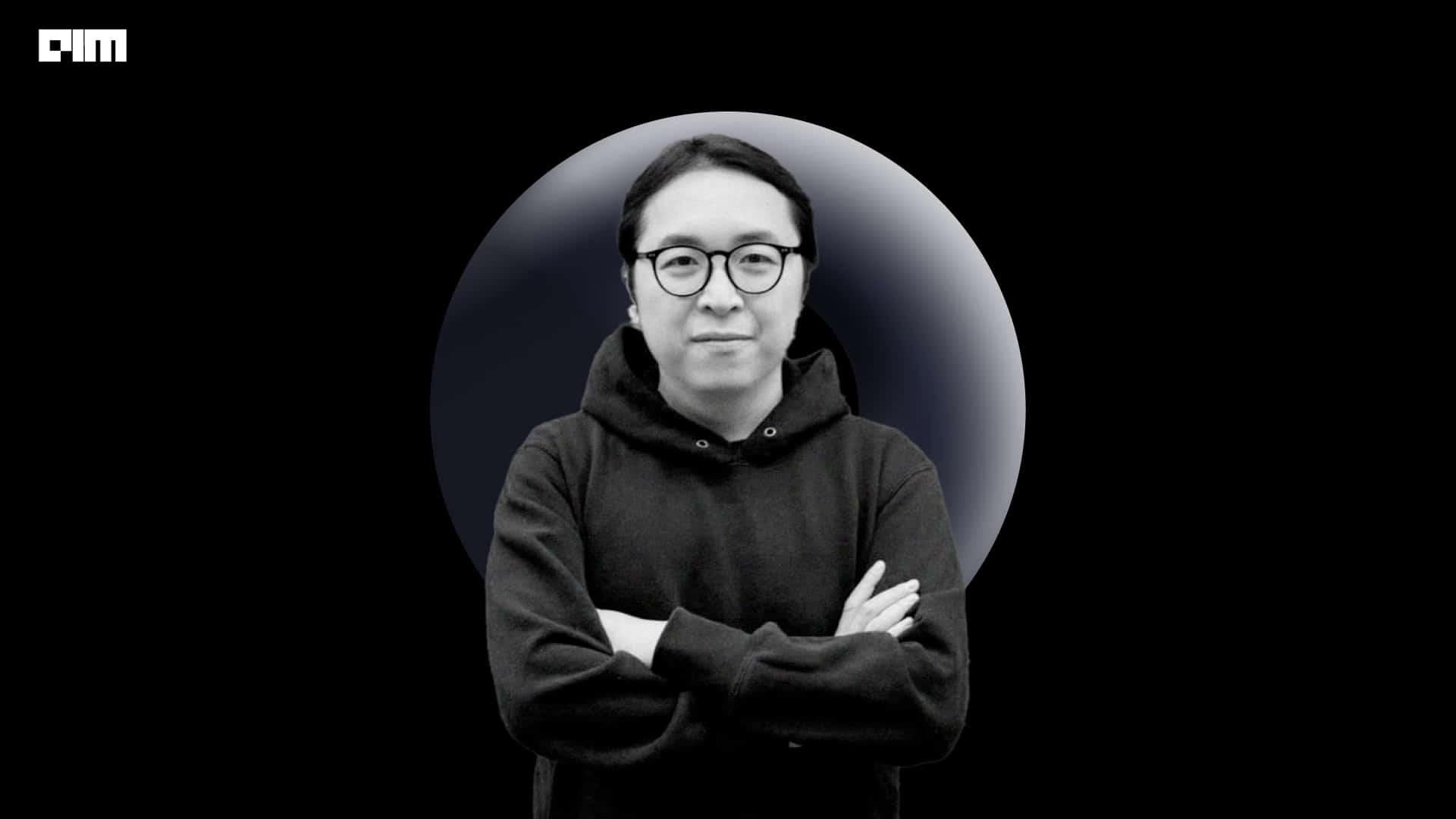
Even though database solutions have evolved over time, developers are constantly seeking solutions that are flexible, easily scalable, and provide real-time analytics.
TiDB, which is an advanced distributed SQL database developed by PingCAP, claims to solve all these problems for developers. Its biggest selling point is that it offers a compelling blend of horizontal scalability, MySQL compatibility, and real-time analytics capabilities.
Competitors like CockroachDB lack a built-in real-time analytics engine. While MongoDB does support basic analytics capabilities, it may encounter difficulties when handling complex analytical workloads or extremely large datasets.
The concept behind TiDB, as described by Ed Huang, the co-founder and chief technology officer at PingCAP, originated nearly a decade ago from the challenges he personally encountered in leveraging databases.
Back then, he was employed by a startup where he managed database clusters heavily reliant on MySQL at the time.
“Our business operations were deeply tied to relational databases due to their complex logic. However, our data was growing rapidly, necessitating sharding (a technique that spreads data across numerous MySQL instances),” Huang said in an exclusive interview with AIM.
This meant every few months, the database size would double, requiring them to rebalance and move data constantly.
TiDB is Inspired by Google
Huang reveals that this was when he came across two Google papers, which served as an inspiration for TiDB (where ‘Ti’ stands for Titanium).
“About ten years ago, I came across Google’s papers on Spanner and F1—new SQL databases that offer traditional SQL interfaces but are incredibly scalable under the hood. I realised this was the direction we needed to go—a solution that could handle our scaling needs without sacrificing SQL functionality,” Huang said.
Hence, by merging the strengths of distributed or NoSQL databases with those of traditional databases, Huang aimed at creating a new database that application developers would embrace.
“We saw this integration as the future after being inspired by these research papers. This led us to embark on an open-source project to develop a new database from scratch, ensuring compatibility with MySQL. Our extensive experience with MySQL also motivated us to initiate what would become TiDB,” Huang added.
TiDB Architecture
The overall architecture of TiDB is decoupled into two layers: the storage layer and the key value layer. “I’m really proud to say that I wrote the first line of code for TiDB. We built it completely from scratch, forming a brand new community around it,” Huang said.
TiDB’s architecture is designed to manage extensive datasets while accommodating both transactional and analytical workloads seamlessly.
It has a distributed key-value storage system similar to databases like Cassandra or MongoDB, ensuring data is stored across multiple servers for scalability and resilience against failures.
“Another notable aspect of TiDB is its capability to handle both OLTP (Online Transaction Processing) and MySQL-compatible workloads, as well as OLAP (Online Analytical Processing) or analytics workloads concurrently.
“This is made possible by its dual storage engine architecture within the storage layer. One is the key-value-packed TiKV storage engine, optimised for transactional processing. There’s another storage engine known as TiFlash, designed specifically for handling analytics queries efficiently,” Huang added.
Databricks Loves TiDB
Over 3,000 customers currently leverage TiDB, hundreds of whom are PingCAP’s paying customers. Some notable users of TiDB include Databricks, Airbnb, LinkedIn, Dailymotion, and Capcom.
Huang reveals the US remains the biggest market for TiDB, however, companies in other geographies also leverage the open-source database.
“Databricks is one of our biggest adopters in the US. Actually, all of Databricks’ metadata is supported by TiDB. Another big customer we have in the US is Pinterest. Currently, we manage hundreds of terabytes of data for Pinterest, assisting them in migrating from HBase to TiDB,” Huang revealed.
TiDB sees higher adoption among customers using legacy NoSQL databases. Most of the customers paying for TiDB services are from the Banking, Finance, Security and Insurance (BFSI) sector.
“In the past, companies relied on Oracle, MySQL, or other legacy databases. Nowadays, with the shift towards mobile platforms, data volumes have significantly increased, posing challenges for infrastructure, especially in sectors like finance,” Huang said.
These industries often have extensive legacy code built on SQL, making it difficult to transition to NoSQL interfaces seamlessly.
“They still require SQL compatibility for their codebase but now need scalability and robust data consistency at financial-grade levels. Japan’s largest payment company relies on TiDB. We also see great adoption in the e-commerce and gaming industry,” Huang added.
TiDB in India
Flipkart, one the largest e-commerce companies in India, revealed in a blogpost they have leveraged TiDB to scale to 1 million QPS. The e-commerce giant also faced scaling challenges which were met by vertically scaling the MySQL cluster. However, they saw TiDB as the solution.
“Flipkart has been using TiDB as a hot store in production since early 2021 for moderate throughput levels of 60k reads and 15k writes at DB level QPS. We set out to demonstrate the feasibility of using TiDB as a hot SQL data store for use cases with very high QPS and low latency requirements for the first time,” the company said in the blog post.
“Another large logistics company in India is also our customer, they are managing terabytes of data and using our real-time analytics capability. We also have a few SaaS companies using our cloud service,” Huang said.
India is home to many high-growth cloud-native companies that could benefit from TiDB. Moreover, TiDB’s real-time analytical capabilities could be an attractive prospect for many SaaS companies.
“They prefer not to establish multiple data warehouses or utilise various data sources separately for analytics. Our goal is to offer a unified platform where they can use a single system to gain real-time insights seamlessly. As far as I know, other databases like MongoDB or CockroachDB do not come with a real-time analytics engine,” he concluded.