Month: December 2024
The companies that had tightly packed the technology they had developed through “patents …
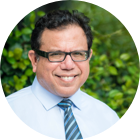
MMS • RSS
Posted on mongodb google news. Visit mongodb google news
![ID Tech company Hopae's digital identity authentication solution is a third-generation digital identity technology that cannot be falsified and escaped privacy infringement problems, enabling easy and convenient identity authentication. [Picture = Hoppae]](https://mobilemonitoringsolutions.com/wp-content/uploads/2024/12/news-p.v1.20241231.0261a95c2ef9465c87b4861c1c4eda3a_P1.png)
The companies that had tightly packed the technology they had developed through “patents” have changed. Open Source, which discloses important technologies and even detailed codes so that anyone can see them, is becoming the basis of a new industry in the software (SW) market.
Open source is free, but many companies are looking for ways to generate revenue through this, creating a business model and even generating revenue.
The U.S. Red Hat is a representative. Red Hat is a global provider of enterprise open-source solutions, including Linux, Cloud, Container, Kubernetes, and more. Red Hat developed an enterprise-class Linux distribution to introduce a subscription model and ensure thorough quality control and long-term support. Later in 1999, IBM loaded Red Hat Linux on corporate servers, and various Wall Street financial institutions also began introducing Red Hat to reduce costs.
Since the first premium enterprise Linux launch in 2002, it became the first open-source technology company to surpass $1 billion in sales in 2012, and IBM acquired Red Hat in 2019 for about $34 billion, the largest in the history of the software company’s acquisition. In particular, Red Hat currently accounts for about 17.5% of IBM’s total actual system sales. This is a significant increase from 9.2% at the time of the acquisition in 2019, meaning Red Hat has a growing share of IBM’s software division.
MongoDB is an open-source database-based cloud service provider. Having grown around the developer community, MongoDB has established a monetization model, providing enterprises including advanced security features, audit functions, and professional support services, operating certification programs.
Since then, it has attracted $150 million from its Series F investment in 2013 and attracted more than 30 customers among the top Fortune 500 companies in 2014. MongoDB’s sales as of the third quarter of 2024 amounted to about 580 billion won.
Companies based on excellent open-source technology are also emerging in Korea. ID Tech company Hopae, which provides digital identity authentication solutions, uses open source as a market entry strategy. The open-source code created by the Hopae team has more than 2 million global downloads and is actively used for various projects.
According to the Software Policy Research Institute, the global open source market is expected to grow from $27.7 billion (about 38.4 trillion won) in 2022 to 75.2 billion dollars (about 104.2 trillion won) in 2028.
Article originally posted on mongodb google news. Visit mongodb google news
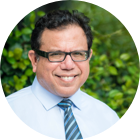
MMS • Anthony Alford
Article originally posted on InfoQ. Visit InfoQ
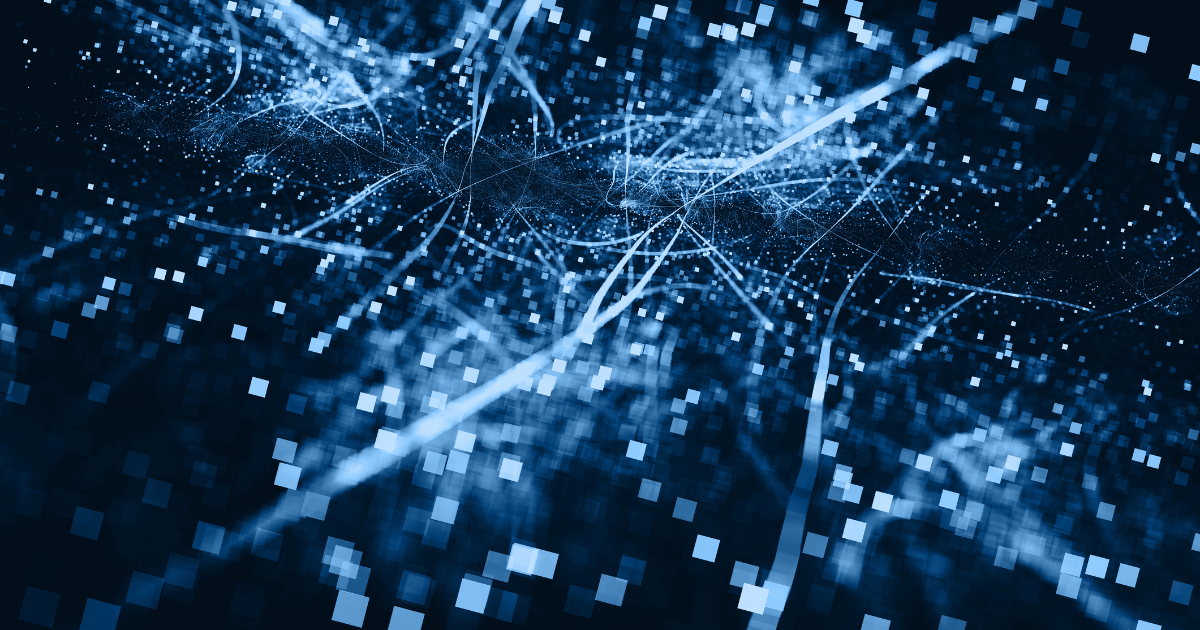
Researchers from InstaDeep and NVIDIA have open-sourced Nucleotide Transformers (NT), a set of foundation models for genomics data. The largest NT model has 2.5 billion parameters and was trained on genetic sequence data from 850 species. It outperforms other state-of-the-art genomics foundation models on several genomics benchmarks.
The InstaDeep published a technical description of the models in Nature. NT uses an encoder-only Transformer architecture and is pre-trained using the same masked language model objective as BERT. The pre-trained NT models can be used in two ways: to produce embeddings for use as features in smaller models, or fine-tuned with a task-specific head replacing the language model head. InstaDeep evaluated NT on 18 downstream tasks, such as epigenetic marks prediction and promoter sequence prediction, and compared it to three baseline models. NT achieved the “highest overall performance across tasks” and outperformed all other models on promoter and splicing tasks. According to InstaDeep:
The Nucleotide Transformer opens doors to novel applications in genomics. Intriguingly, even probing of intermediate layers reveals rich contextual embeddings that capture key genomic features, such as promoters and enhancers, despite no supervision during training. [We] show that the zero-shot learning capabilities of NT enable [predicting] the impact of genetic mutations, offering potentially new tools for understanding disease mechanisms.
The best-performing NT model, Multispecies 2.5B, contains 2.5 billion parameters and was trained on data from 850 species of “diverse phyla,” including bacteria, fungi, and invertebrates as well as mammals such as mice and humans. Because this model outperformed a 2.5B parameter NT model trained only on human data, InstaDeep says that the multi-species data is “key to improving our understanding of the human genome.”
InstaDeep compared Multispecies 2.5B’s performance to three other genomics foundational models: Enformer, HyenaDNA, and DNABERT-2. All models were fine-tuned for each of the 18 downstream tasks. While Enformer had the best performance on enhancer prediction and “some” chromatin tasks, NT was the best overall. It outperformed HyenaDNA on all tasks, even though HyenaDNA was trained on the “human reference genome.”
Besides its use on downstream tasks, InstaDeep also investigated the model’s ability to predict the severity of genetic mutations. This was done using “zero-shot scores” of sequences, calculated using cosine distances in embedding space. They noted that this score produced a “moderate” correlation with severity.
An Instadeep employee BioGeek joined a Hacker News discussion about the work, pointing out example use cases in a Huggingface notebook. BioGeek also mentioned a previous Instadeep model called ChatNT:
[Y]ou can ask natural language questions like “Determine the degradation rate of the human RNA sequence @myseq.fna on a scale from -5 to 5.” and the ChatNT will answer with “The degradation rate for this sequence is 1.83.”
Another user said:
I’ve been trialing a bunch of these models at work. They basically learn where the DNA has important functions, and what those functions are. It’s very approximate, but up to now that’s been very hard to do from just the sequence and no other data.
The Nucleotide Transformers code is available on GitHub. The model files can be downloaded from Huggingface.
Flutter 3.27 Promotes New Rendering Engine Impeller, Improves iOS and Material Widgets, and More
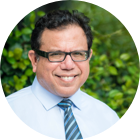
MMS • Sergio De Simone
Article originally posted on InfoQ. Visit InfoQ
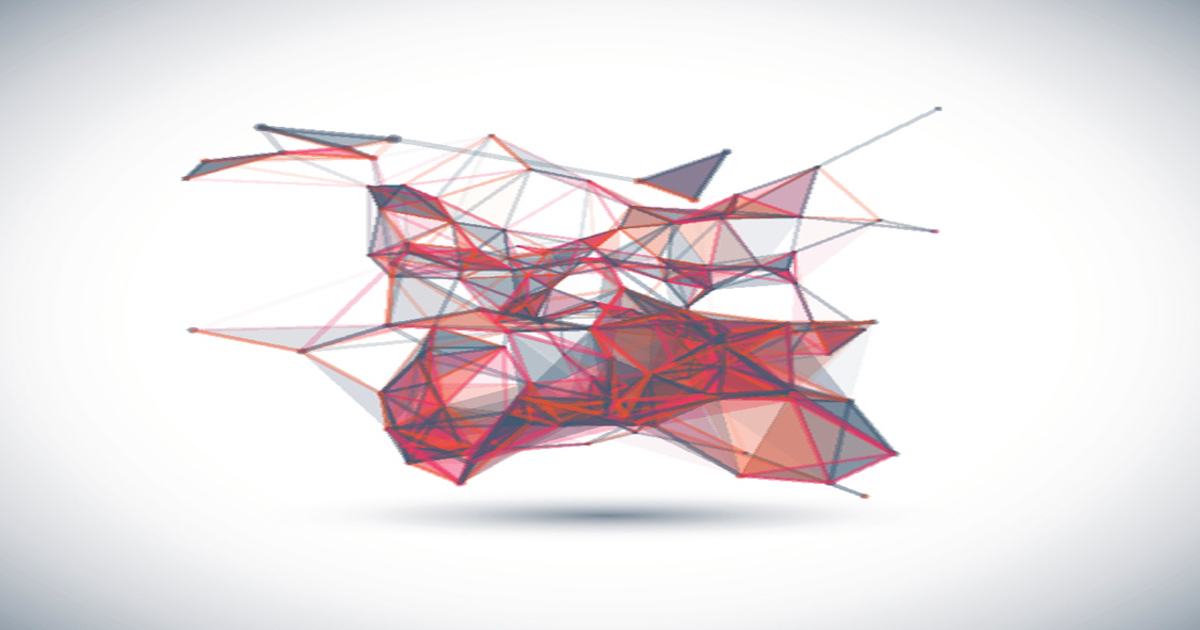
The latest version of Google’s cross-platform UI kit, Flutter 3.27, brings a wealth of changes, including better adherence to Apple’s UI Guidelines thanks to a number of improved Cupertino widgets, new features for CarouselView
, list rows and columns, ModalRoutes
transitions, and so on. Furthermore, the new release makes the Impeller rendering engine the default, with improved performance, instrumentation support, concurrency support, and more.
Cupertino is a collection of widgets that align strictly with Apple’s Human Interface Guidelines. Flutter 3.27 updates a few to increase their fidelity, including CupertinoCheckbox
, CupertinoRadio
, and CupertinoSlidingSegmentedControl
. It also extends CupertinoCheckbox
, and CupertinoSwitch
to make them more configurable, and brings CupertinoButton
on a par with the latest customizability options introduced in iOS 15. Other improvements affect CupertinoActionSheet
, CupertinoContextMenu
, CupertinoDatePicker
, and CupertinoMagnifier
.
On the front of Android-native Material UI, Flutter 3.27 extends CarouselView
with CarouselView.weighted
, which allows you to define more dynamic layouts using the flexWeights
parameter to specify the relative item weight occupied within the carousel view. Additionally, SegmentedButton
can be aligned vertically and a few widgets have been fixed to align better with Material 3 specifications.
Flutter 3.27 also improves ModalRoutes
, text selection, and rows and columns spacing. ModalRoutes
, which have the peculiarity of blocking interaction with previous routes by occupying the entire navigator area, now enable the exit transition from a route to sync up with the enter transition of the new route, so they play nicely together. Text selection now supports Shift + Click gesture to move the extent of the selection to the clicked position on Linux, macOS, and Windows. Rows and Columns may use a spacing
parameter which makes it easier to offset them from each other.
After over one year in preview, the new Impeller rendering engine has been promoted to default on modern Android devices, replacing the old one, Skia. Skia is still available as an opt-in in case of compatibility issues. Impeller attempts to do at compile times a number of tasks that Skia does at runtime, such as building shaders and reflections and creating pipeline state objects upfront, and improves caching to make performance more predictable. It also improves debug support by labeling textures and buffers and being able to capture animations to disk without affecting rendering performance. When necessary, Impeller can distribute single-frame workloads across multiple threads to improve performance.
Going forward we will continue to make improvements to Impeller’s performance and fidelity on Android. Additionally, we intend to make Impeller’s OpenGL backend production ready to remove the Skia fallback.
Other improvements worth mentioning are improved rendering performance on iOS, support for the Swift Package Manager, as well as edge-to-edge and freeform support for Android. Do not miss the official announcement to get the full detail.
Podcast: Key Trends from 2024: Cell-based Architecture, DORA & SPACE, LLM & SLM, Cloud Databases and Portals
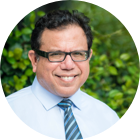
MMS • Daniel Bryant Thomas Betts Shane Hastie Srini Penchikala Ren
Article originally posted on InfoQ. Visit InfoQ
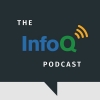
Transcript
Daniel Bryant: Hello and welcome to the InfoQ podcast. My name is Daniel Bryant. I’m the news manager here at InfoQ and my day job, I currently work in platform engineering at Syntasso. Today we have a treat for you as I’ve managed to assemble several of the InfoQ podcast hosts to review the year in software technology, techniques and people practices. We’ll introduce ourselves in just a moment and then we’ll dive straight into our review of architecture, culture and methods, AI and data engineering, and the cloud and DevOps. There is of course no surprises that AI features heavily in this discussion, but we have tried to approach this from all angles and we provided plenty of other non-AI insights too. Great to see you all again. Let’s start with a quick round of intro, shall we? Thomas, do you want to go first?
Thomas Betts: Yes, sure. I don’t think I’ve done my full introduction in a while on the podcast. My day job, I’m an application architect at Blackbaud, the number one software provider for social impact. I do a lot of stuff for InfoQ, and this year a lot of stuff for QCon. So I’m lead editor for architecture design, co-host of the podcast obviously, I was a co-chair of QCon San Francisco this year and a track host for QCon London, so that kind of rounds out what I’ve been up to. Next up, Shane.
Shane Hastie: Thanks, Thomas. Really great to be here with my colleagues again. Yes, Shane Hastie, lead editor for culture and methods. My day job, they call me the global delivery lead for Skills Development Group. Written a couple of books deep into the people and culture elements of things, the highlights for this year. Unfortunately, I didn’t get to any of the QCons, I’m sad about that, and hopefully next year we’ll be there in person. But some of the really amazing guests we’ve had on the Engineering Culture podcast this year have been, we’ll talk a bit about it later on, but that’s probably been some of my highlights. Srini.
Srini Penchikala: Thanks, Shane. Hello everyone. I am Srini Penchikala, my day job is, I work as an application architect, but for InfoQ and QCon, I serve as the lead editor for data engineering and AI and ML community at InfoQ. I also co-host a podcast in the same space, and I am serving as the programming committee member for QCon London 2025 conference, which I’m really looking forward to.
Real quick, 2024 has been a marquee year for AI technologies and we are starting to see the next phase of AI adoption. We’ll talk more about that in the podcast and also I hosted a AI/ML Trends podcast report back in September, so that will be the biggest reference that I’ll be going back to a few times in this podcast. Definitely there are a lot to look forward to. I am looking forward to sharing in today’s podcast the technologies and trends that we should be hyped about and also what is all the hype that we should be staying away from. Next, Renato.
Renato Losio: Hi everyone. My name is Renato Losio. I’m an Italian cloud architect living in Germany. For InfoQ, I’m actually working in the Cloud queue, I’m an editor. And definitely my highlight of the year has to be being the chair of the first edition of the InfoQ Dev Summit in Munich. Back to you, Daniel.
Daniel Bryant: Fantastic. Yes, I’ve already enjoyed the Dev Summit. I was lucky enough to go to the InfoQ Dev Summit in Boston. I’ve worked in Boston for a number of years or worked with a Boston-based company and the content there was fantastic, staff plus in particular sticks in my mind. I know we’re going to dive into that, but I also did the platform engineering track at QCon London this year, which is fantastic. Great Crossing paths with yourself, Srini, I think I’ve met almost everyone this year. Maybe not yourself, Shane, this year. Actually, I can’t remember exactly when, but it’s always good to meet in person and the QCons and the Dev Summits are perfect for that kind of stuff, and I always learn a ton of stuff, which we’ll give the listeners a hint at tonight, right?
Did our software delivery trends and technology predictions from 2023 come true? [04:10]
So today as we’re recording, so I just want to do a quick look back on last year. Every year we record these podcasts, we always say we want to look back and say, “Hey, did our predictions come true?” And when we pulled out this time for last year, we said, “2024, could the use of AI within software delivery becoming more seamless and increasing divide between organizations and people adopting AI and those that don’t, and a shift towards composability and improved abstractions in the continuous delivery space”. So I think we actually did pretty well this year, right? I’m definitely seeing a whole lot of AI, as you hinted at, Srini, coming in there and as you say, every year, Thomas, the AI overlords have not stolen our jobs yet. So not completely in terms of software engineering.
Thomas Betts: I think we’re all still employed, and I’m surprised that quote in there about the separation, I think that’s true. We’re seeing the companies that are doing the innovator early adopter are still doing new things. I think the companies that are more late majority are like, “We want to use it”, but they’re not quite sure how yet. I don’t know if, Srini, you have any more insight into how people are adopting AI?
Srini Penchikala: Yes, that’s very true, Thomas. Yep, definitely AI is here, I guess, but again, it’s still evolving, right? So I definitely see some companies are going full-fledged, some companies are still waiting for it to happen. So as they say that the new trend, and I will talk more about this later in the podcast, the agentic AI. The AI agents that cannot only generate and predict the content and insights, they can also take actions. So the agentic AI is a big deal. So as they say, the AI agents are coming, so whether they’ll overtake our jobs or not, that’s to be seen. But speaking of the last year’s predictions, we had talked about the shift towards AI adoption, right? Adoption has been a lot more this year, but I think we still have some areas where I thought we would be further ahead and we are not. So it’s still evolving.
Shane Hastie: Yes. I see lots and lots of large organizations that are not software product companies putting their toes in and bumping against privacy, security, ethics and not sure how to go forward knowing that they absolutely need to, and often that governance frame slowing things down as they’re exploring, “Well, okay, what does this really mean for us?” And a lot of conservatism in that space.
Daniel Bryant: It’s really funny, Shane, compared to what Renato and I are seeing. So I went to the Google Cloud Summit in London and I only heard AI, AI, AI, AI. If you listen to the hype, and I think, Renato, you covered re:Invent for us recently. I think if you sort listen to the hype, you believe everyone, even the more late adopters are covering AI, Renato, right?
Renato Losio: Yes, I mean, just to give a order of magnitude, I don’t know if they changed the number during the conference, but at a conference like the re:Invent, there were over 800 session about AI/ML. By comparison, there were just about 200 about architecture and even less about serverless. So that gives a bit of direction where the conference was going.
Surprisingly, the itself was not so generative AI focused. They tried to make it difference, probably go back to that later on, but I find it interesting what happened in the last year in the space of AI cloud. But I don’t take responsibility for the prediction of last year because I was not there. But I have to admit that I love to start with looking back at the prediction actually, when I see tech prediction for 2025, actually, I tend to go to the bottom of the article, use it as a reference to the article of the year before because I to see a year later what people predicted if it still does. I love to go back to those topics.
What are the key trends in architecture and design? [08:08]
Daniel Bryant: That’s awesome, Renato, and you will get the privilege this time at the end to talk about your predictions, right? So we’ll hold you to it next year. So enjoy it for the moment, but I think that’s a perfect segue, you mentioned serverless there. Architecture and design is one of our sort of marquee topics. To be fair all the things we’re going to talk about today are marquee topics, but we often look to the InfoQ through an architect lens. And Thomas, you sort of run the show for us there. I know you’ve got a bunch of interesting topics that you wanted to talk about. I think the first one was around cell-based architectures, things like that.
Thomas Betts: Yes, so this was I think something we highlighted in the A&D trends report back in April I think it was, and we ended up having an e-mag come out of it and had a lot of different topics. Some of those were from various articles or presentations at QCons. And just this happens in architecture that we have these ideas that have been around for a while, but we didn’t have the technology to make it easy to implement. And then it becomes easier and then people start adopting it. So the idea of putting all of the necessary components in one cell, and so you minimize the blast radius.
And if one goes down, the next cell isn’t affected, and how do you control it and how do you maintain it? Just like any microservices, distributor architecture, there’s extra complexity involved, but it’s becoming easier to manage, and if you need the benefits, then it’s worth the trade-offs. You’re willing to take on the extra overhead of managing these things. So like I said, that e-mag is full of a lot of different insights, different viewpoints. Again, architects always looking at different viewpoints and ways to look at a problem, so I like that it gives a lot of different perspectives.
Daniel Bryant: We’ve got to named check Rafal [Gancarz] there on that one just quickly, and Rafal did a fantastic job on that.
Thomas Betts: Yes, thanks for calling out Rafal. He did fantastic doing that. The other thing that I remember talking about a few people at QCon London this year, and I think QCon London last year as well, was the green software trends. So fantastic book just came out in April. I think the book signing was the release party at QCon London.
Daniel Bryant: Oh, yes, it was Anne Currie and team, Yes, fantastic.
Thomas Betts: Anne Currie, Sara and Sarah. Sara Bergman and Sarah Hsu were all there together and they actually credited InfoQ with being the force that made the book happen because that was how they were able to all get together and collaborate. So that book about Building Green Software, Adrian Cockroft has talked about this. He’s kind of championing it from here’s how you do it in the cloud, going back to serverless, he advocates make things serverless, make them small, only running when you need it. That kind of philosophy, I think we’re going to start seeing architects have to start thinking about that more and more. It’s going to become more important.
The point that I love about, and Sara, I had a great presentation on this, is that it just makes more efficient software. It makes better software, it makes more sustainable, more maintainable software, all the other abilities we look for, if you build with a green mindset, you get all those other benefits. So if you just say, “Oh, we need to make it green, we need to reduce our carbon footprint, and nobody really cares about that”. Well, all the other things you do care about, they come along for the ride. So start thinking about that way. How do you only run your software when you need to? How do you only write the code you need? So there’s a lot of ideas in there and I think we’re going to start seeing more of that hopefully in the next year. That’s definitely one of my 2025 predictions.
Renato Losio: I think you really raised a good point about software in the sense that it’s seen as well as a proxy to other things like cost for example. I think if you go to your CFO and you say, “We are trying to make our cloud deployment greener”. Probably he won’t care that much. Even if outside the company you might sell that message that you’re generating less CO2 realities. Often it’s really a proxy on cost optimization. When you talk about serverless run just what you need or whatever, choose region that are more carbon effective often are as well the cheapest data center because they’re more efficient. So it’s an interesting approach to looking through the lens of green and not just cost or architecture.
Thomas Betts: Yes, I know Anne has talked a lot about how one of the only measures we have sometimes is what’s our bill? What’s the AWS bill that we get or Azure bill? And if that’s the only measure you have, then use it, that’s better than nothing and it is actually still a pretty good proxy. The cloud providers are getting better at saying, “Here’s how that energy is produced”. But you have to dig for it. I think we’re going to start seeing that become more of a first class, “Here’s your bill and then here’s the carbon footprint of this because you ran it in this data center, which is in US East and it’s running on coal versus somewhere in Europe that’s on nuclear”. Right? So I think that’s going to be interesting to see if we can get to those metrics and people say, “Oh, we can run this somewhere else because there’s a better carbon efficiency and we save a lot of money by doing it”.
Srini Penchikala: All of that is important, I agree with you both the green software and the sustainable computing is a very good time to talk about it in the AI context because as we all know, the large language models that are the main part of GenAI, they do require a lot of processing power. So we have to be more conscious about how much are we really spending and how much value we are getting, right? So between the value of these solutions and what are we really spending in terms of money and energy resources and the environment, right? So how about you Shane? What do you think about green?
Shane Hastie: Green software, ethics and sustainability have been a drum I have wanted and have beaten for the last three years, and it’s been great to see more and more the ability to have those hard conversations. And the challenging within organizations where as software engineers, as the development community, we can actually start to ask for, “Hey, we want to do it this way”. And now as Thomas so smoothly says, we can actually use money as a good excuse, and that helps because without showing the measurable benefits, we’re going to struggle.
Thomas Betts: And Srini, you brought up the AI component and yes, the AI carbon footprint is enormous. Somebody will say it’s the next Bitcoin, it’s just spending a lot of money, but hopefully it’s producing value. The other aspect I thought was interesting, and this was a presentation at QCon San Francisco, was how GitHub Copilot serves 400 million requests per day, and it got into the details of how you actually have to implement AI solutions. So GitHub Copilot, two things. There’s GitHub Copilot Chat, that’s where you open a window. You ask a prompt, it responds, right? It’s like ChatGPT with code, but GitHub Copilot the autocomplete, it’s kind of remarkable because it has to listen for you to stop typing and then suggest the next thing.
And so all of the complications that go underneath that that I hadn’t considered, so they had to create this whole proxy and it’s hanging up HTTP two requests, and if you just use native things like engine X after a hundred disconnects, it just drops the connection entirely and so you’ve lost it. There’s all these low level details, and I think when we get to see AI become more standard as parts of big software, more companies that have these distributed systems are going to run into some of these problems. Maybe not to GitHub Copilot scale, but there’s probably these unintended things that are going to show up in our architecture that we haven’t even thought of yet. I think that’s going to be real interesting to see how AI creates the next level of architectural challenges.
Srini Penchikala: Also, maybe just to add to that, Thomas, maybe the AI can also solve some of those challenges, right? We talk about AI hallucinations and bias, but can AI also help minimize the environmental hallucinations and the ethical biases
Daniel Bryant: Said like a true proponent, Srini, the cause of and solution to most of the world’s problems, Ai, right? I love it.
Thomas Betts: Yes, I think we’re going to see how are we going to use AI as an architect? Can I use it in my day job? Can it help me design systems? Can it help me solve problems? I use it as the rubber duck. If I don’t have someone else that I can get on a call and chat with and discuss a problem, I’ll open up ChatGPT and just start a conversation and say, “Hey, I’m trying to come up with this. What are some of the trade-offs I should consider?” I haven’t gone so far as to say, “Solve this problem”. The hallucination may be completely invalid or it may be that out of the box thinking that you just hadn’t thought of yet, it’s going to sound valid either way. You still have to prove it out and make it work.
What are the key trends in culture and methods? [16:22]
So I think the other part of being an architect, I talked about using AI to do your job, but I think the architectural process has been a big discussion this year. All of the staff plus talks at QCons are always interesting. I think we have good technical feedback, but people love, I personally love the how do I do my job? How do I get better at my job, how do I level up? So we’ve seen some of that in decentralizing decision making. I talked to Dan Fike and Shawna Martell about that. They gave a presentation, they wrote up an article based on that presentation. And, Shane, I can’t remember if you talked to them as well or you talked to somebody else about how to do a better job. How do you level up your staff plus, how do you become a staff plus or principal engineer?
Shane Hastie: Yes, I’ve had a number of people on the podcast this year talking about staff plus and growth both in single track and dual track career paths. The charity majors still going, the pendulum back and forward. When do you make your choices? How do you make your choices? Shameless plug, I had Daniel as a guest talking about people process for great developer experience, technical excellence, weaving that into the way that we work. So all of these things leveraging AI, challenging the importance of the human aspect, that critical thinking, and Thomas, you made the point, the hallucination is there.
Well, one of the most important human skills is to recognize the hallucination and not go down that path to utilize the generative AI and other tools at your fingertips most effectively. Platform engineering, the myth still of the 10 Xx engineer, but with great platform engineering, what we do is we make others 10 times better and there’s a lot of the, I want to say same old people stuff still coming up because fundamentally the people haven’t changed.
Daniel Bryant: That’s a good point.
Shane Hastie: Human beings, we don’t evolve as rapidly as software tools, digging into product mastery. Really interesting conversation with Gojko Adzic about observability at the customer level and bringing those customer metrics right to the fore. So Yes, all of the DORA metrics and all of these others still really important. I had a couple of conversations this year where we’ve spoken about DORA’s great, and it is becoming a target in and of itself for many organizations, and that doesn’t help if you don’t think about all of the people factors that go with it.
Thomas Betts: There’s a law that once you have a named goal, it stops being a useful metric, I’m botching the quote entirely. But it’s like once you have that, it was useful to measure these things to know how well you’re doing, but then people see it as that’s the thing I have to do, as opposed to, “No, just get better and we’ll observe you getting better”.
Daniel Bryant: Goodhart’s law, Thomas.
Thomas Betts: Yep, thank you.
Shane Hastie: And W. Edwards Deming, if you give a manager a numerical target, they will meet it even if they have to destroy the organization to do so.
Thomas Betts: You mentioned the DORA metrics. Why I loved the QCon keynote Lizzie Matusov gave was talking about the different metrics you can measure for psychological safety and how to measure team success. And that’s how you can say, are these teams being productive? And there’s different survey tools out there and there’s different ways to collect this data, but I think she focused on if people feel they’re able to speak up and raise issues and have open conversations, that more than anything else makes the team more successful because then they’re able to deal with these challenges.
And I think that keynote went over really well with the QCon audience, that people understood like, “Oh, I can relate to that, I can make my teams better”. You might not be able to use all the new AI stuff, but you can go back and say, “Here’s how I can try and get my teams to start talking to each other better”. Like you said, Shane, the humans haven’t evolved that fast, software has.
Daniel Bryant: That’s a great quote. On that notion, are you seeing more use of other frameworks as well? Because I’m with you. In my platform engineering day job, I see DORA, every C-level person I speak to knows what DORA is, and for better or worse, people are optimizing for DORA, but I’m also seeing SPACE from [Dr] Nicole Forsgren, et al. I’m seeing DevEx from the DX folks, a few other things. And I mean space can be a bit complicated because there’s like five things, the S, the P, the A, the C and the E. but I think if you pick the right things out of it, you can focus more on the people to your point and the productivity, well, and the happiness as well, right?
Shane Hastie: Yes, we could almost go back to the Spotify metrics. The Spotify team culture metrics have been around for a long time and what I’m seeing is reinventions of those over and over again. And it’s fundamentally about how do we create that psychologically safe environment where we can have challenging conversations with a foundation of trust and respect. And that applies in technical teams of course, but it applies across teams and across organizations and the happiness metrics and there are multiple of those out there as well.
Ebecony gave us some good conversations about creating a joyous environment and also protecting mental health. Burnout is endemic in our industry at the moment, and I think it’s not just in software engineering, it’s across organizations, but mental health and burnout is something we’re still not doing a good job at. And we really need to be upping our organizational gain in that space.
Renato Losio: I think it’s been actually a very bad year in this sense as well with what you mentioned, Shane, about a manager that reached the goal, might destroy a team, make me think that this year one of the key aspects has been all the return to office mandate, regardless if it’s good or not for the company, has been a key element of a large company, became like the new trend.
Shane Hastie: There’s cartoons of people coming into the office and then sitting on Zoom calls because it’s real. The return to office mandates, and this is a strong personal opinion, they’re almost all have nothing to do with value for the organization, and they’re all about really bad managers being scared. And I’m sure I’ve just made a lot of managers very unhappy.
Daniel Bryant: Hey, people join this podcast for opinions, Shane, keep them coming.
Thomas Betts: So many times come across the Conway’s law and all different variations of this. I think Ruth Malan is the one who said that if you have an organization that’s in conflict, the organization structure and conflict of the architecture, the organization is going to win. And I’m wondering what the return to office mandates, how is that going to affect the architecture? I made the quote, I think it was last year, the comment about the COVID corollary to Conway’s law that the teams that can’t work effectively, once we all went remote, they weren’t able to produce good distributed systems because they couldn’t communicate.
Well, now we’re in that world. Everyone has adapted to that. I think we’re seeing more companies are doing distributed systems and the teams don’t sit next to each other. They have to form their own little bubbles in their virtual groups because they’re not sitting at the desk next to each other. If we make people go back to the office, but we don’t get the benefits of them having that shared office space, then what is that going to do to the software? I don’t know if I have an answer to that, but it seems like it’s not going to have a good impact if you’re doing it for the wrong reasons.
Srini Penchikala: Maybe I can bring a cheesy analogy to this, right? We started this conversation with the serverless architecture where you don’t need to run the software systems and servers all the time. They can be ideal when you don’t need them. I think that should apply to us as humans also, right? I read this quote on LinkedIn this morning, I really like this, it says, “Almost everything will work again if you unplug it for a few minutes, including you”. So we as humans, we need to unplug once in a while to avoid the burnout. I mean, that’s the only way we can be productive when we need to work, if we have to take that break or time off.
Daniel Bryant: Yes. Plus one to that, Srini. Changing subjects a little bit. But Shane, I know you wanted to touch on some of the darker human sides of tech too.
Shane Hastie: Yes, I will go there. Cybercrime, the use of DeepFakes, generative AI. I had Eric O’Neill on and the episode is titled, Spies, Lies in Cybercrime and he brings the perspective from an FBI agent and there are some really interesting applications of technology in that space and they’re very, very scary. Personally, I was caught by a scammer this year and they were the social engineering, it worked.
Fortunately, my bank’s fraud detection system is fantastic and they caught it and I didn’t lose any money, but it was a very, very scary period while we were trying to figure that out. And for me, and in Eric’s conversations, it’s almost always the social engineering piece that breaks the barrier. Once you’ve broken the barrier, then the technology comes into play. But he tells a story of a deepfake video in real time that was the chief financial officer of a large global company. So very perturbing, somewhat scary. And from a technical technologist perspective, how do we make our systems more robust and more aware? So again, leveraging the AI tools is one of the ways. So the potential for these tools is still huge.
What are the key trends in AI and data engineering? [27:01]
Daniel Bryant: Perfect segue, Srini, into your space. It’s slightly scary, but well-founded grounding there, Shane. But Yes, I think it’s almost a dual-use technology in terms of for better or worse, right? And, Srini, love to hear your take on what’s going on in your world in the AI space.
Srini Penchikala: Yes, thanks, Daniel. Thanks, Shane, that’s a really good segue. This is probably a repeat of one of the old quotes, Uncle Ben from Spider-Man movie, right? “With power comes responsibility”. I know we kind of keep hearing that, but with powerful AI technologies, how to come the responsible AI technologies. So in the AI space, again, there are a lot of things happening. I encourage our listeners to definitely watch the 2024 trends report we published back in September. We go into a lot of these trends in detail, but just to highlight a couple of things in the short time we have in today’s podcast, obviously the language models are going at a faster pace than ever, large language models, it seems like there’s no end to them. Every day you see a new LLM popping out and the Hugging Face website, you go there, you see a lot of LLMs available for different use cases.
So it’s almost like you need an LLM, large language model to get a handle on these LLMs. But one thing I am definitely curious about and also seeing the trend this year are what are called small language models. So these are definitely a lot smaller in terms of size and the data sets compared to large language models. But they are excellent for a lot of the use cases where again, talking about green software, you don’t want to expend a lot of computing resources, but you can still get similar accuracy and benefits also. So these SLMs are getting a lot of attention. Microsoft has something called Phi-3, Google Gemma, there is the GPT Mini I think. So there are a lot of these small language models are definitely adding that extra dimension to the generative AI. And also these language models are enabling the AI modeling and execution on the mobile devices like phones, tablets, and IoT devices.
Now you can run these language models on a smaller phone or a tablet or laptop without having to send all the data to the cloud, which could have some data privacy issues and also obviously the cloud computing resource and cost. So this is one of the trends I’m watching, definitely keep an eye on this. And the other trend is obviously the one I mentioned earlier called agentic AI, agent-based AI technologies, right? So this is where I think we are going to the next level of AI adoption where we have the traditional AI that’s been used for a long time for predicting the results. And then we have GenAI, which started a few years ago with the GPT and the ChatGPT announcements. So generative AI not only does the prediction, but it also generates the content and the insights, right? It goes to the next level. So I think the agents are going to go one more step further and they can not only generate the content or insights, but also they can act on those insights with or without supervision of humans.
So there are a lot of tasks that we can think of that we don’t need humans to be in the middle of the process. We can have the agents act on those. And also one of the interesting use cases I heard recently is a multi-agent workflow application where each of the agents take the output from the previous agent as the input and they perform their own task. But doing so, they’re actually also giving the feedback to the previous agent on the hallucinations and the quality of the output so the previous agent can pick a different model and rerun the task, right?
So they go through these iterations to improve the quality of the output and minimize the hallucinations and biases. So these multi-agent workflows are definitely going to be a big thing next year. So that’s one other area that I’m seeing. Also, recently, just a couple of days ago, Google actually announced Google Gemini 2.0. The interesting thing about this article is Google’s CEO, Sundar Pichai, he actually wrote a note, so he was kind of the co-author of this article.
So it was a big deal from that standpoint. And they talk about how the agentic AI is going to be impactful in the oral AI adoption and how these agentic AI models like Google Gemini 2.0 and other models will help with not only the content generation and insights, but also actually acting on those insights and performing the tasks. Real quickly on a couple of other topics, the RAG, we talked about this last year, retrieval augmented generation. So I’m seeing a couple of specialized areas in this.
One is multimodel RAG where you can use the RAG techniques for text content and also audio and the video images to make them work together for real-world use cases. And the other one is called graph RAG. Basically use the RAG techniques on knowledge graphs because the graph data is already interconnected. So by using RAG techniques on top of that will make it even more powerful. And I think the last one I want to mention is the AI-powered PCs. Again, AI is coming to everywhere.
So especially in line with the local first architectures that we are seeing in other areas, how much computing can I do locally on the device, whether it’s my smartphone or a tablet or IoT device. So this AI is going to be powering the PCs going forward. We already are hearing about Apple intelligence and other similar technologies. But Yes, other than that AI, like you all mentioned GitHub Copilot, so AI is going to be everywhere in the software development life cycle as well.
I had one use case where multiple agents are used for code generation, document generation and test case generation in a software development life cycle. It’s only going to grow more next year and be even more like a peer programmer. That’s what we always talk about. How can AI be an architect, how can AI be a programmer or how can AI be a QA software engineer. So I think we’re going to see more developments in those areas. So that’s what I’m seeing. I don’t know, Thomas, Shane or Renato, you guys are seeing any other trends in AI space?
Shane Hastie: So I’m definitely seeing the different pace of adoption. As I mentioned right at the beginning, the organizations for whom software is now their core business, but they still think of themselves as not software companies, the banks, the financial institutions and so forth. They’re struggling with wanting to bring in, wanting the benefits and really having to tackle the ethical challenges, the governance challenges, but overcoming those, recognizing those, the limited selection of tools that are available. So one organization, the AI tool they’re allowed to use is Copilot. Nothing wrong with Copilot, there are 79,000 at least other alternates. But even in that sort of big space, there’s a dozen that people should be looking at. And I think one of the risks in that is that they end up narrowing the options and not getting the real benefits.
Thomas Betts: I saw this in my company. We did a very slow rollout of a pilot of GitHub Copilot and they wanted those people to say, “Here, how do we use it? But we’re not going to just let everyone go through it”. And part of it once they said everyone can use it is you have to go through this training on here’s what’s actually happening. So everyone understood what are you getting out of it. Things like the hallucinations, you can’t assume it’s going to be correct, it’s only as good as what you give. But if it doesn’t know the answer, its job isn’t to know the right answer. Its job is to predict the next word, predict the next code. So it’s always going to do that even if that’s not the right thing. So you still have maybe even more oversight than if you had just doing a full request review or review for someone else’s code, right?
We’ve now done Microsoft Copilot as the standard that the company gets to use. I think this is probably the one you’re referring to, everyone can use the generative AI tool to start doing all the things. And because we’re mostly on the Microsoft stack, there’s the integrations with SharePoint and OneDrive and all of that benefit. So there’s reasons to stay within the ecosystem, but again, every employee has to go through just like our mandatory ethics training and compliance training and if you deal with financial data, you have to go through this extra training. If you’re going to use the AI tools, here’s what you need to know about them, here’s how you’re safe about that. And I think that training is going to have to evolve a lot year to year to year because the AI that we have in 2024 is not the same AI we’re going to have in 2026. It’s going to be vastly different.
What are the key trends in cloud technologies? [36:08]
Daniel Bryant: I think it’s a perfect segue from everyone talking about AI. Renato, our cloud space has lost its shine. We used to be doing all the innovative stuff, all the amazing things, and now with the substrate powering all this amazing innovation going on in the AI space. So you mentioned already about the sharp skew at re:Invent as one example. There’s many other conferences, but the sharp skew at re:Invent towards this AI focus. But I’m kind of curious what’s going on outside of that, Renato? What are you seeing that’s interesting in general from re:Invent and from CloudFlare and from Azure and GCP?
Renato Losio: I think that the key point that has been going on for already two, three years is that we have moved from really innovation as in any area to really a more evolutionary approach where we had new feature, but there’s nothing really super new and that’s probably good. I mean we are getting maybe more boring but more enterprise-wise maybe, but that’s the direction. Just an example, I mean, you mentioned re:Invent. People tend to go out of re:Invent, say, “This has been the best re:Invent ever”. Usually because they get more gadgets and whatever else, but that’s usually the main goal talking about sustainability. But even Amazon itself during the keynotes and during the week before re:Invent was highlighting the 10th anniversary of AWS Lambda and 10 years of Amazon Aurora, 10 years of… what was the third one?
I think container service, and then even I think KMS. And those were all announced 10 years ago at re:Invent. If you take a re:Invent today was a great re:Invent, but you don’t have something as revolutionary as Lambda. You have cool things, you have a new distributed database, yes, definitely it’s cool, but you don’t have the same kind of new breaking the world things. It’s more evolutionary thing as well in the AI space. That was of course a key part of it. But yes, there were some new financial models for Bedrock the school, they got better names that even someone like myself that is not the skilled in the area, I can say I can get when I should use a light approved or a micro model.
At least I know that the price probably follows that. But apart from that, it’s quite, I would say evolutionary. Probably the only exception in this space is Cloudflare, at least the way I see it because probably we used to consider just a CDN. We used to consider networking mostly, but actually in the last few years they’re fully fledged cloud provider. Quite interesting services that have been out there at the moment. The other trend I wouldn’t say is for 2025, but it is already here, at least in the data space, in the database space, in the cloud database space. I think this was the year that Postgres became the de facto standard. Any implementation of any cloud provider has to be somehow even pretending to be Postgres.
Daniel Bryant: Indeed.
Renato Losio: That’s the direction. Even Amazon doesn’t mention MySQL for new services for GSQL or even Limitless database earlier this year, used to be their first open source compatible database reference point. Now it’s not anymore. All the vector databases are pointing to us as well. So that’s the direction I see at the moment.
Daniel Bryant: Fantastic.
Srini Penchikala: Quickly, Daniel, I have a comment. Renato, you are right in saying that Postgres is getting a lot of attention. Postgres has a vector database extension called PG Vector, and that is being used a lot to store the vector embeddings for AI programming. And also the database are becoming more distributed in terms of the architecture and also hosting. So I’ve been seeing a lot of databases that need to run on on-prem and in the cloud with all the transactional support and the consistency support, so distributed events are kind of helping in this. So definitely like you said, just like cloud is a substrate for everything else to happen, database engineering, data engineering and databases are the foundation for all of these powerful AI programs to work. So we don’t want to lose focus on the data side of the things.
What are the key trends in DevOps and platform engineering? [40:34]
Daniel Bryant: I’ll cover the platform engineering aspects now. So for me, 2024 has definitely been the year of the portal. Backstage has been kicking around for a while. We had folks like Roadie talking about that. It’s got its own colo day at KubeCon. Now the BackstageCon, I’ve also seen the likes of Port and Cortex emerging, lots of funding going into this space and lots of innovation too. Folks are loving a UI, loving a portal, a service catalog, a way to understand all the services they’ve got in their enterprise and how these things knit together. Now I’ve argued in a few of my talks, there’s definitely a missing middle, which we’re sort of labeling as platform orchestration popping up. And this is the missing middle between something like a UI or a CLI, portal, that kind of good stuff. And the infrastructure layer, things like Terraform, Crossplane, Pulumi, cloud infrastructure in general.
Now I was super excited to see Kief Morris with his latest edition of his book, Infrastructure’s Code talking about this missing middle two and also Camille Fournier and Ian Nowland in their platform engineering book that’s just been published by Rily. Fantastic read. I thoroughly recommend folks get hold of that, but they were also talking about this missing middle as well. So I’m super excited over the next year to see how that develops. Just in general, I’m seeing platform engineering move more into the mainstream. We’re seeing more events pop up. I mean the good folks at Humanitec spun up PlatformCon in 2022. That one is going from strength to strength. There’s also Plat Eng Day at KubeCon now and KubeCon in London coming up next year, we’re going to see an even bigger Plat Eng day I think with two tracks. So I’m super excited about that. I’m definitely at QCon London.
We’re going to dive into platform engineering again, I’ve got a hat tip my good people that were on the track this year, Jessica, Jemma, Aviran, Ana and Andy did an amazing job talking about our platform engineering from their worlds. Topics like APIs came up, abstractions, automation, I often say three A’s of platform engineering, really important. And in particular, Aviran Mordo talks about platform as a runtime. And at Wix they built this pretty much serverless platform and it was a real eye-opener seeing at the scale they’re working at how they’ve really thought about the platform APIs, they’ve really thought about the abstractions to expose the developers. And a whole bunch of the stuff behind the scenes is automated Now it’s all tradeoffs, right? But Aviran, and I saw Andy said the same thing. They’re optimizing for developer experience and not just keeping people happy, but keeping people productive too.
And there’s lots of great research going on around developer experience. I’ve got to hat tip the DX folks, Abi Noda and crew and some great podcasts kicking off in that space. And I’m just really interested about that balance, that sort of business balance I guess with proving out value for the platform, but also making sure developers are enjoying their day-to-day work as well. There’s a whole bunch of platform engineering FOMO that I see in my day-to-day job and people spinning up platform engineering efforts, spinning up platforms without really having business goals associated with them, which I think is a danger. And I’ll hint at some more why I think that’s going later on.
What are our predicted trends for software delivery in 2025? [43:25]
Now it’s that time where we hold you to predictions you are going to make, and next year the InfoQ bonus is based on success or failure of these predictions. So I’d love to go around the room and just hear what you most excited about for 2025 and predictions and we’ll go in the same order if that’s all right. Thomas, we’ll start with you.
Thomas Betts: Yes, I don’t think there’s going to be some dramatic shift. There’s never dramatic shifts in architecture, but I think the sustainability, the green engineering, I think those concepts are just going to start becoming more mainstream. You look at how team topologies and microservices and all these things overlap. All these books start referencing each other, the presentations start talking about each other in the same ideas in different ways. I think we’re going to see architects that just look at it from, “I did this for all of these benefits and I learned to put all these benefits together because they were the right sustainable thing to do and it made my system better”. I want to see that presentation at QCon San Francisco next year of we chose to do some architecture with sustainability in mind, and here’s the benefits we saw from it. Shane.
Shane Hastie: I’m going to build on the people stuff. I think we’re going to see challenges with the return to office mandates. I hope we’re going to see some sensibility coming in that when we bring people together, we bring them together for the right reason and that we get the benefit of those human beings in the same physical space. Doing collaborative work generates innovation. You want to allow that, but you also want to give the space for the work that is more effective when we are remote.
So that combination of the two, and there’s no one size fits all, and organization shifting away from mandates to conversations and let’s treat our people as responsible, sensible adults and trust them to figure out what is going to be the best way of getting stuff done. I want to see the continuing evolution of the team areas, generative AI and other AI tools as partners. I think the agentic AI as a partner is going to be a huge potential and I think we’re going to start to see some good stuff happening in that space with the people. But again, the critical thinking, the human skills becoming more and more important. So what’s the prediction there? It’s maybe more of a hope.
Daniel Bryant: No, I like it, Shane, very positive. It’s very good. Srini, on to you.
Srini Penchikala: Yes. Thanks, Daniel. Yes, definitely on the AI side, I can take a shot at a couple of predictions. I think the LLMs are going to become more business domain specific in a sense, just like we used to see our banking standards, our insurance industry standards, I think we’ll probably eventually start seeing some finance, FinTech LLM or manufacturing LLM, because that’s where the real value is, right? Because a typical ChatGPT program only knows what’s out in the internet. It doesn’t know exactly what my business does, and I don’t want to share my business information out to the outside world.
But I think there will be some of these consortiums that will come together and share at least non-sensitive proprietary information among the organizations, whether it’s manufacturing or healthcare. And they will start to create some base language models that the applications in those companies can use to train, right? So it could be the large language model, like Llama 2 will have something more specific on top of that, and then applications will be built on top of that. So that’s one prediction for me. And the other one is agents. I think agents, agents and agents. Just like that movie Matrix, agents are coming, right? Hopefully these agents are not as nefarious.
Daniel Bryant: Indeed.
Srini Penchikala: They’re not villains, right? Exactly. But Yes, I think we’ll see more. I think it’s the time for all these generative AI, great content to put into action, not by humans but hopefully by computer programs. So that’s another area that I’m definitely looking forward to seeing. Other than that, I think AI will become, like you said, something like a boring cross-cutting concern that will just enable everything. We don’t even have to think about it. And maybe just like the toys we buy sometimes, they say batteries not included. Maybe in the future the application that are not using AI, which will be very few, they will say, this application does not include AI, right? Because everything else will be AI pretty much, right? So those are my couple of predictions.
Daniel Bryant: I like it, Srini, fantastic stuff. Renato, over to you.
Renato Losio: Well, first of all, I will say that these are my tech provision in the cloud for 2025 and beyond, as many good ones say. So I will always have the chance next year to say, “Well, there’s still time”. But I really think that next year will be the first year in the cloud space that for processor Intel won’t be the default anymore. Basically we won’t consider anymore using Graviton or anything else as the alternative would be the de facto on most deployment.
And the second one, giving as well how different cloud provider implemented now distributed database using their own proprietary network and basically taking advantage of the speed they have. I see the cloud provider going towards this distributed system with less regional focus. As an end user, I will probably… As a developer, I won’t carry more long term about the specific region, the geographical area, probably yes, for compliance and many other reasons. But if behind my database is Ohio or Northern Virginia or whatever else, I would probably not care so much.
Daniel Bryant: Thanks, Renato. I like the hedge there or is it a smart move. Well done. So all these, some predictions around the platform engineering space and the good folks at Gartner are saying we’re about to head into the trough of disillusionment in their model of adoption. And I also think this is true. My prediction next year is we’re going to hear more failure stories around platforms and ultimately that’ll be triggered by a lack of business goals associated with the engineering going on. Now, I think this is just part of the way it goes, right? We saw it with microservices, we saw it with DevOps as well.
Ultimately, I think it leads us to a better place. You go into the trough for disillusionment, you hopefully come out the other side on the plateau of productivity and you’re delivering business value and it’s all good stuff. And I think we’re going to bake in a lot of learnings that we sort of temporarily forgotten. This is again, the way of the world.
We often embrace a new technology, embrace a new practice, and we sort of temporarily forget the things we’ve learned before. And I’m seeing in platform engineering, definitely a lack of focus on business goals, but also a lack of focus on good architecture practices, things like coupling and cohesion. And in particular creating appropriate abstractions for developers to get their work done and also composability of the platform.
So I think in 2025, we’re going to see a lot more around golden paths and golden bricks and making it easy to do the right thing to code ship run for developers, deliver business value, and also allow them to compose the appropriate workflow for them. And again, that’ll be dependent on the organization they’re working on. But I’m super excited to see where platform engineering is going in 2025. As always, it’s your pleasure. We could talk all day, all night, I’m sure here, but it’s fantastic just to get an hour of everyone’s time just to review all these things as we close up the year. I’ll say thank you so much for everyone and we’ll talk again soon.
Shane Hastie: Thank you, Daniel.
Srini Penchikala: Thank you.
Renato Losio: Thank you, Daniel.
Thomas Betts: Thank you Daniel, and have a happy New Year.
Mentioned:
.
From this page you also have access to our recorded show notes. They all have clickable links that will take you directly to that part of the audio.
Java News Roundup: Spring AI 1.0-M5, LangChain4j 1.0-Alpha1, Grails 7.0-M1, JHipster 8.8
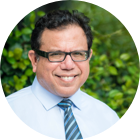
MMS • Michael Redlich
Article originally posted on InfoQ. Visit InfoQ
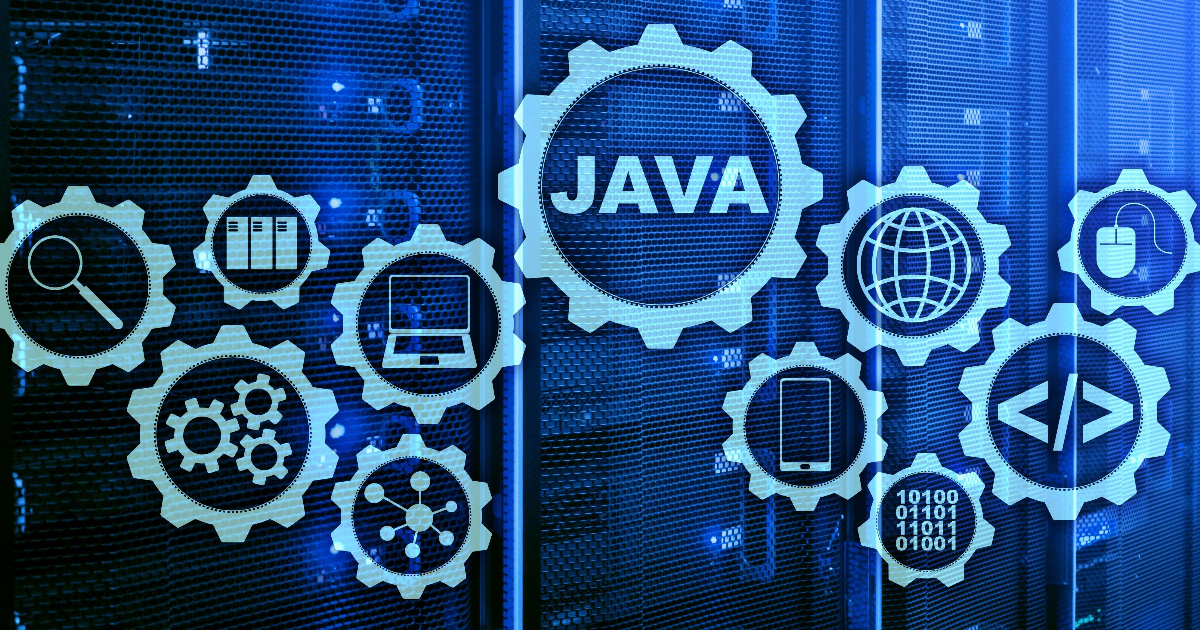
This week’s Java roundup for December 23rd, 2024 features news highlighting: the fifth milestone release of Spring AI 1.0; the first milestone release of Grails 7.0; the first alpha release of LangChain4j 1.0; and the release of JHipster 8.8.
JDK 24
Build 29 remains the current build in the JDK 24 early-access builds. Further details on this release may be found in the release notes.
JDK 25
Similarly, Build 3 remains the current build in the JDK 25 early-access builds. More details on this release may be found in the release notes.
For JDK 24 and JDK 25, developers are encouraged to report bugs via the Java Bug Database.
Spring Framework
Ten days after introducing the experimental Spring AI MCP, a Java SDK implementation of the Model Context Protocol (MCP), to the Java community, the Spring AI team has released a version 0.2.0 milestone. This initial release features: a simplified McpClient
interface such that listing operations no longer require a cursor parameter; and a new SseServerTransport
class, a server-side implementation of the MCP HTTP with the SSE transport specification. Breaking changes include a rename of some modules for improved consistency. Further details on this release may be found in the release notes.
The fifth milestone release of Spring AI 1.0 delivers: incubating support for the Model Context Protocol; support for models such as Zhipuai Embedding-3 and Pixtral; and support for vector stores such as MariaDB and Azure Cosmos DB. There were also breaking changes that include moving the MilvusVectorStore
class from the org.springframework.ai.vectorstore
package to the org.springframework.ai.vectorstore.milvus
package. The Spring AI team plans a sixth milestone release in January 2025 followed by one release candidate before the final GA release.
TornadoVM
The release of TornadoVM 1.0.9 ships with bug fixes and improvements such as: support for the RISC-V 64 CPU port to run OpenCL with vector instructions for the RVV 1.0 board; support for int
, double
, long
and short
three-dimensional arrays by creating new matrix classes; and the addition of a helper menu for the tornado
launcher script when no arguments are passed. More details on this release may be found in the release notes.
Micronaut
The Micronaut Foundation has released version 4.7.3 of the Micronaut Framework featuring Micronaut Core 4.7.10, bug fixes and patch updates to modules: Micronaut Logging, Micronaut Flyway, Micronaut Liquibase Micronaut Oracle Cloud and Micronaut Pulsar. Further details on this release may be found in the release notes.
Grails
The first milestone release of Grails 7.0.0 delivers bug fixes, dependency upgrades and notable changes such as: a minimal version of JDK 17, Spring Framework 6.0, Spring Boot 3.0 and Groovy 4.0; and an update to the PublishGuide
class to use the Gradle AntBuilder
class instead of the deprecated Groovy AntBuilder
class. More details on this release may be found in the release notes.
LangChain4j
After more than 18 months of development, the first alpha release of LangChain4j 1.0.0 features: updated ChatLanguageModel
and StreamingChatLanguageModel
interfaces to support additional use cases and new features; and an initial implementation of the Model Context Protocol. The team plans a GA release in Q12025. Further details on this release may be found in the release notes.
Apache Software Foundation
The Apache Camel team has announced that the version 3.0 release train has reached end-of-life. The recently released Apache Camel 3.22.3, will be the final one. Developers are encouraged to upgrade to the 4.0 release train via this migration guide.
JHipster
The release of JHipster 8.8.0 features: upgrades to Spring Boot 3.4, Angular 19 and Gradle 8.12; experimental support for esbuild in Angular; and improved CSRF token handling for single page applications. More details on this release may be found in the release notes.
Similarly, the release of JHipster Lite 1.24.0 ships with an upgrade to Spring Boot 3.4.1 and new features/enhancements such as: a new module for configuring a Liquibase linter; and the addition of metadata to the preprocessor to resolve a ESLint cache error. Further details on this release may be found in the release notes.
Kubernetes 1.32 Released with Dynamic Resource Allocation and Graceful Shutdown of Windows Nodes
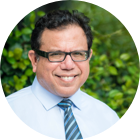
MMS • Mostafa Radwan
Article originally posted on InfoQ. Visit InfoQ
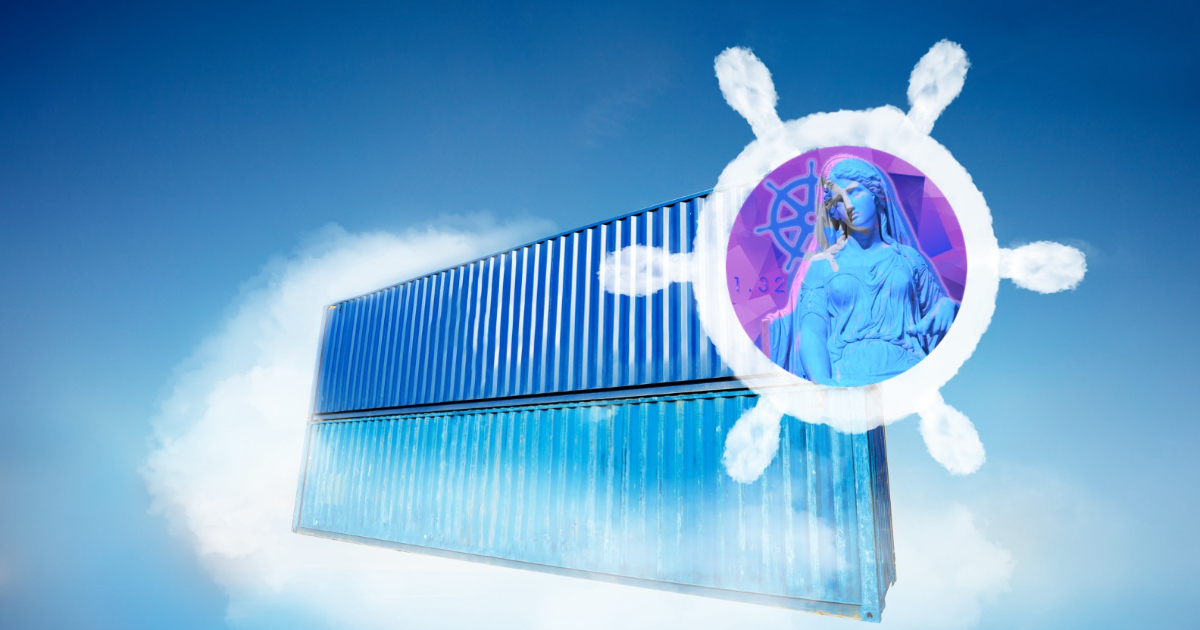
The Cloud Native Computing Foundation (CNCF) released Kubernetes 1.32, named Penelope a few weeks ago. The new release introduced support for the Graceful Shutdown of Windows Nodes, new status endpoints for core components, and asynchronous preemptions in the Kubernetes scheduler.
A key feature in Kubernetes 1.32 is the various enhancements to Dynamic Resource Allocation (DRA), a cluster-level API for requesting and sharing resources between pods and containers. These enhancements improve the ability to effectively manage resource allocation for AI/ML workloads that rely heavily on specialized hardware such as GPUs.
Some alpha features in version 1.32 include two new HTTP status endpoints /statusz
and /flagz
for core components such as the kube-scheduler and kube-controller-manager. This makes gathering details about a cluster’s health and configuration easier to identify and troubleshoot issues.
Another feature entering alpha in this release is asynchronous preemption in the scheduler. This mechanism allows high-priority pods to get the resources needed by evicting low-priority pods in parallel, minimizing delays in scheduling other pods in the cluster.
In addition, an enhancement to Gracefully Shut down Windows Nodes has been added to the Kublet to ensure proper lifecycle events are followed for pods. This allows pods running on Windows nodes to be gracefully terminated and workloads rescheduled without disruption. Before this enhancement, this functionality was limited only to Linux nodes.
The automatic removal of PersistantVolumeClaims(PVCs)
created by StatefulSets
is a stable feature in version 1.32. This streamlines storage management, especially for stateful workloads, and reduces the risk of unused resources.
This release also includes a generally available improvement to the Kubelet to generate and export OpenTelemetry trace data. This aims to make monitoring, detecting, and resolving issues related to the Kubelet easier.
Allowing anonymous authorization for configured endpoints moved to beta in this release. This enhancement is enabled by default in version 1.32 allowing cluster administrators to specify which endpoints can be accessed anonymously.
Additionally, recovery from volume expansion failure is a beta feature in the new release. This improvement allows recovery from a volume expansion failure by retrying with a smaller size, reducing the risk of data loss or corruption throughout the process.
The flowcontrol.apiserver.k8s.io/v1beta3
API related to FlowSchema
and PriorityLevelConfiguration
was removed in the new release. It’s part of the Kubernetes API functionality to deal with an overload of incoming requests. Users are encouraged to migrate to flowcontrol.apiserver.k8s.io/v1
which has been available since version 1.29.
According to the release notes, Kubernetes version 1.32 has 44 enhancements, including 19 entering alpha, 12 graduating to beta, and 13 becoming generally available or stable.
For more information on the Kubernetes 1.32 release, users can refer to the official release notes and documentation for a detailed overview of the enhancements and deprecations in this version or watch the upcoming CNCF webinar by the release team scheduled for Thursday, January 9th, 2025 at 5 PM UTC. The next release version 1.33 is expected in April 2025.
After Rome Failure, VoidZero is the Newest Attempt to Create Unified JavaScript Toolchain
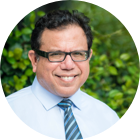
MMS • Bruno Couriol
Article originally posted on InfoQ. Visit InfoQ
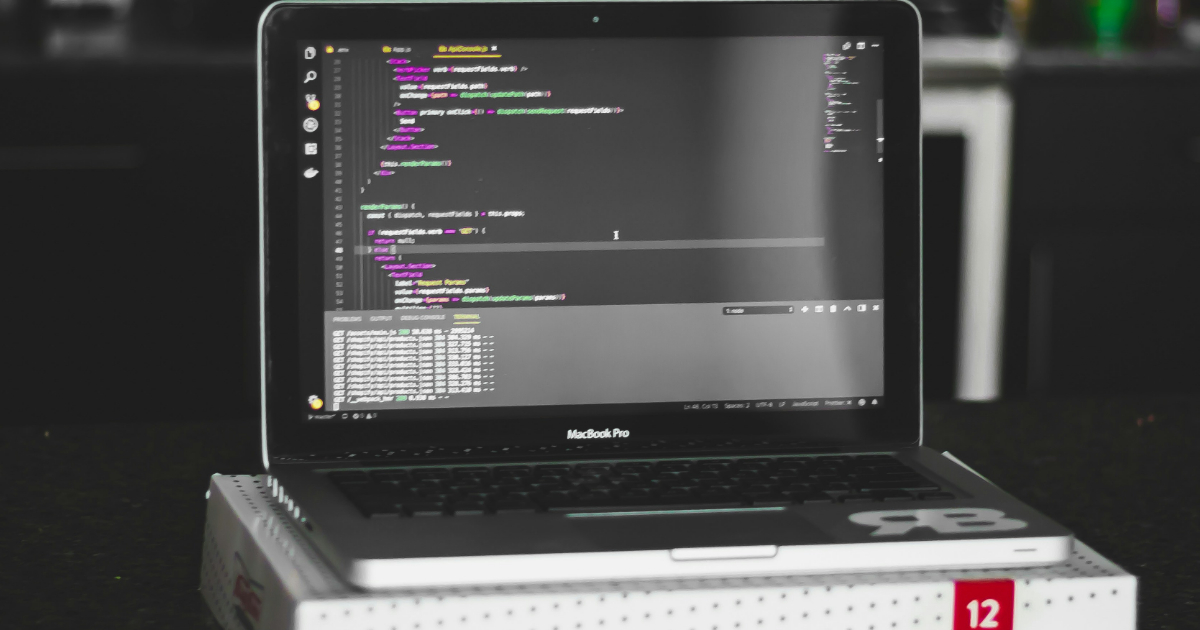
Evan You, creator of the Vue.JS web framework and Vite build tool, recently announced the creation of VoidZero Inc., a company dedicated to building a unified development toolchain for the JavaScript ecosystem. You posits that VoidZero may succeed where Rome, a previous project with similar goals, failed as it would inherit the large user base from the popular Vite toolchain. While VoidZero would release open-source software, the company itself is VC-funded.
VoidZero aims at creating an open-source, high-performance, and unified development toolchain for the JavaScript ecosystem that covers parsing, formatting, linting, bundling, minification, testing, and other common tasks that are part of the web development life cycle. While unified, the toolchain would be made of components that cover a specific task of the development cycle and can be used independently.
High performance would result from using the system development language Rust. Rust’s compile-to-native nature removes layers of abstraction and is credited to run at close-to-native speed. Rust’s memory safety features additionally facilitate concurrently running tasks and better utilization of multicore architectures. Additional performance gains come from better design (e.g., parsing once and using the same AST for all tasks in the development cycle).
The release note also mentions seeking to provide the same developer experience across all JavaScript runtimes. JavaScript is now being run in many different environments, including at the edge. New runtimes have appeared in recent years to reflect those new execution contexts (e.g., Deno, Bun, Cloudflare Workers, Amazon’s LLRT).
You justified its vision on Twitter:
The biggest challenge of a unified toolchain is the zero-to-one problem: it needs to gain critical mass for exponential adoption to justify continued development, but it is hard to cross the chasm before it actually fulfills the vision.
VoidZero does not have this problem, because Vite is already the fastest growing toolchain in the JavaScript ecosystem. And even by pure implementation progress, we’ve already built more than Rome did (before it transitioned into Biome) at this point. I think the premise that JS would benefit from a unified toolchain stands without questions – it’s the execution that matters.
Some developers on Reddit have raised concerns regarding VoidZero’s venture capital backing. The release note mentions that potential revenue incomes would come on top of the released open-source components in the shape of end-to-end solutions targeting the Enterprise segment, which has specific requirements in terms of scale and security. As adoption in the Enterprise is tied to adoption outside of the Enterprise (where developers are sourced from), VoidZero has an incentive to maintain free access to its core offering, beyond the usual benefits of open-source development. Trevor I. Lasn, in an article in which he elaborates on the pros and cons of VC funding, wonders:
[Premium features or enterprise solutions] aren’t necessarily a bad thing. Sustainable open source is good for everyone. But it does raise questions about long-term accessibility and potential lock-in.
The full release note is available online and includes many more technical details together with answers to a list of frequently asked questions.
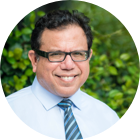
MMS • Sergio De Simone
Article originally posted on InfoQ. Visit InfoQ
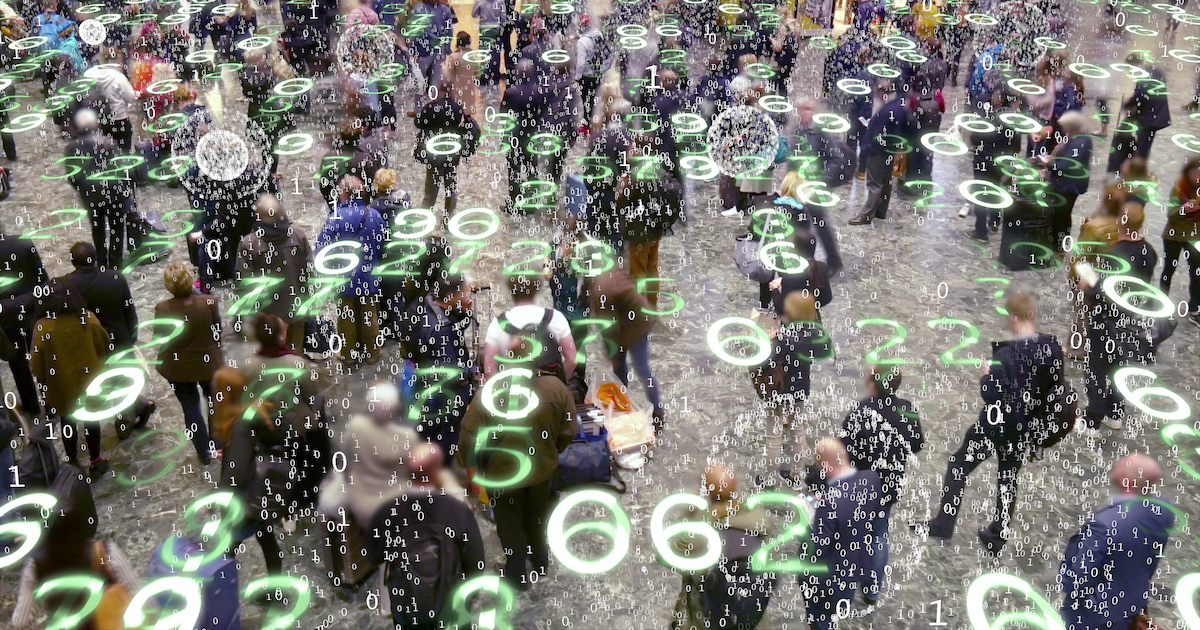
In order to maximize the benefits brought by Kotlin in terms of productivity and safety, Meta engineers have been hard at work to translate their 10 million line Android codebase from Java into Kotlin. One year into this process, they have ported approximately half of their codebase and developed a specific tool, Kotlinator, to automate the process as much as possible.
Instead of translating only actively developed code, which could sound the most convenient approach, Meta engineers decided to go for a full migration to avoid the risk that any remaining Java code could be the source of nullability issues but also to remove the drawbacks of using two different toolchains in parallel and the performance hit of having to compile a mixed codebase.
From the beginning, it was clear to Meta engineers that the support provided by IntelliJ’s J2K translation tool was not enough for such a large codebase and that they needed to automate the conversion process as much as possible. However, J2K provided the foundations for their conversion solution, Kotlinator.
The first step was transforming J2K into a headless tool that could be run on a remote machine. The headless J2K was implemented as an IntelliJ plugin extending the ApplicationStarter
class and calling directly into JavaToKotlinConverter
, as the IntelliJ conversion button does.
Before running the headless J2K, Kotlinator uses a pre- and post-conversion steps to make sure that converted code can build. These steps deal with nullability, apply some known J2K workarounds, and make the generated code more idiomatic.
Both phases contain dozens of steps that take in the file being translated, analyze it (and sometimes its dependencies and dependents, too), and perform a Java->Java or Kotlin->Kotlin transformation if needed.
Meta open sourced some of those conversions so that they could be directly used and also to provide examples of Kotlin AST manipulation through the Kotlin compiler API.
Most of the conversion steps are built using a metaprogramming tool leveraging JetBrains’ Program Structure Interface (PSI) libraries, which can parse files and create syntactic and semantic code models without resorting to the compiler. This is important, say Meta engineers, because in many cases post-processed code would not compile at all, so an alternative method to transform it was required and PSI provided just that.
Any build errors resulting from these steps are handled by interpreting the compiler’s error messages just as a human would do, explain Meta engineers, but in an automated way specified using some metaprogramming.
Instead of simply reducing developers’ effort, these automated steps were also used to minimize the possibility of human error when converting code manually. In the process, Meta engineers collaborated with JetBrains to extend J2K to enable it to run hooks injected by client libraries directly in the IDE.
As Meta engineers explain, a large part of their effort to make Java code translatable to Kotlin is aimed at making it null-safe. This can be achieved simply using a static analyzer, such as Nullsafe or NullAway, to detect all suspect cases, but it is still not enough to eliminate the risk of null-pointer exceptions (NPEs).
NPEs, for example, can arise when some non null-safe dependency passes a null
value into a formally non-nullable parameter to a function. One approach taken by Kotlinator to reduce this risk is “erring on the side of more nullable”, which means defaulting to considering parameters and return types as nullable when the code does not suggest they actually are non-nullable. Additionally, they built a Java compiler plugin that collects nullability data at runtime to identify parameters and return types that could be null
in spite of not being declared as nullable
.
As it is evident from Meta report, translating a large Java codebase into Kotlin is no trivial effort and requires some very advanced engineering. Meta’s journey to make their codebase 100% Kotlin has not come to an end yet but it surely provides the opportunity for some deep understanding of the differences between the two languages and how to transform Kotlin code in a programmatic way. There is much more to this than can be covered here, so do not miss the original article if you are interested in the full details.
Google Cloud Launches Sixth Generation Trillium TPUs: More Performance, Scalability and Efficiency
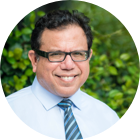
MMS • Steef-Jan Wiggers
Article originally posted on InfoQ. Visit InfoQ
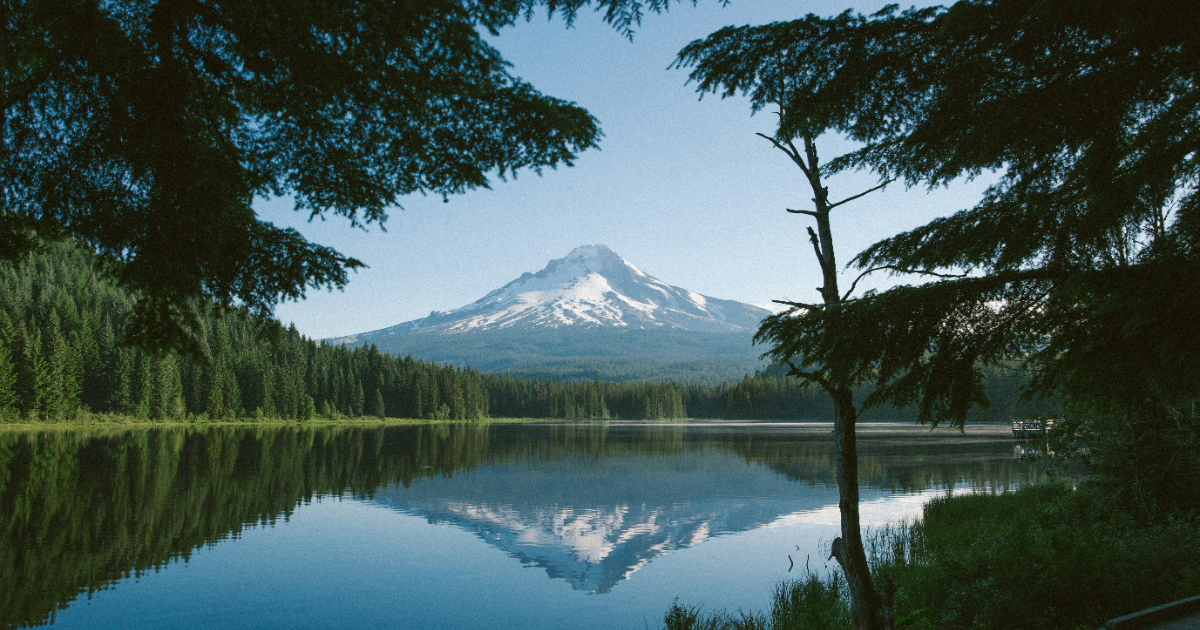
Google Cloud has officially announced the general availability (GA) of its sixth-generation Tensor Processing Unit (TPU), known as Trillium. According to the company, the AI accelerator is designed to meet the growing demands of large-scale artificial intelligence workloads, offering more performance, energy efficiency, and scalability.
Trillium was announced in May and is a key component of Google Cloud’s AI Hypercomputer, a supercomputer architecture that utilizes a cohesive system of performance-optimized hardware, open-source software, leading machine learning frameworks, and adaptable consumption models.
With the GA of Trillium TPUs, Google enhanced the AI Hypercomputer’s software layer, optimizing the XLA compiler and popular frameworks like JAX, PyTorch, and TensorFlow for better price performance in AI training and serving. Features like host-offloading with large host DRAM complement High Bandwidth Memory (HBM) for improved efficiency.
The company states that Trillium delivers training performance over four times and up to three times the inference throughput compared to the previous generation. With a 67% improvement in energy efficiency, Trillium is faster and greener, aligning with the increasing emphasis on sustainable technology. Its peak compute performance per chip is 4.7 times higher than its predecessor, making it suitable for computationally intensive tasks.
Trillium TPUs were also used to train Google’s Gemini 2.0 AI model, with a correspondent on a Hacker News thread commenting:
Google silicon TPUs have been used for training for at least 5 years, probably more (I think it’s 10 years). They do not depend on Nvidia GPUs for the majority of their projects. It took TPUs a while to catch up on some details, like sparsity.
This is followed by a comment that notes that TPUs have been used for training deep prediction models in ads since at least 2018, with TPU capacity now likely surpassing the combined capacity of CPUs and GPUs.
Currently, Nvidia holds between 70% and 95 % of the AI data center chip market, while the remaining percentage comprises different versions like Google’s TPUs. Google does not sell the chips directly but offers access through its cloud computing platform.
In a Reddit thread, a correspondent commented regarding not selling the chips:
That’s right, but I think Google is more future-focused, and efficient AI will ultimately be much more valuable than chips.
In my country, we often say that we should make wood products rather than export wood because making furniture creates more value. I think this is similar: TPUs and AI create more value than the two things alone.
More details on pricing and availability are available on the pricing page.
Podcast: Leveraging AI Platforms to Improve Developer Experience – From Personal Hackathon to AI at Scale
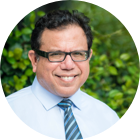
MMS • Olalekan Elesin
Article originally posted on InfoQ. Visit InfoQ
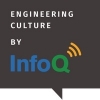
Transcript
Shane Hastie: Good day, folks. This is Shane Hastie for the InfoQ Engineering Culture Podcast. Today I’m sitting down across 12 times zones with Lekan Elesin. Lekan, welcome. Thanks for taking the time to talk to us today.
Olalekan Elesin: Thanks a lot, Shane. Thank you for having me here. To the audience, my name is Lekan Elesin. I think Shane has done a very good job to pronounce the words quite well, to be honest.
It’s not an easy name to pronounce, I’m from the, let’s say the western part of Nigeria, but I stay in Germany. Been in Germany for about seven years. I’m happy to be here.
Shane Hastie: Thank you. So you’re from the western part of Nigeria, you’re in Germany, that’s quite a long journey in many different ways. Tell us about how you got there, what’s your background?
Introductions [01:14]
Olalekan Elesin: So interesting one. So my background is in computer science. I studied computer science as bachelor. So what happened, I think in 2013, I was… In Nigeria, when you graduated from university, you have one year to serve the country, and before my time, I was listening to Bloomberg News, and there was this story called Big Data. This was 2012, 2013, and it was called the sexiest job on the planet back then. And I felt like doing statistics, the odds of being a very, very good software, let’s say programmer was very slim. So I decided to journey into the path of what most people were not aware of back then, which is big data, and that’s how it started.
So computer science graduates turn out to learn how to, back then, a lot of O’Reilly, a lot of InfoQ summits as well, a lot of watching a lot of tutorials on InfoQ as well. My first job was as a software engineer, PHP, but then I learned scalar, also with tutorials from InfoQ, to be honest, as far back as 2013. And from software engineering, I switched to data engineering, and then had an opportunity to work in telecoms, e-commerce, and then education technology.
In 2017, I had an opportunity to move to Germany in an online marketplace, and after three years on the job, I wanted to get a new opportunity, so I decided to opt for product management. So I was data and software engineer, data engineer, product manager, technical product manager. And when I was the technical product manager for an AI platform at the company back then, at some point, there was a decision to reshape the company, or restructure the company, and there was no need for my position anymore.
So I spoke to my then head of engineering, saying, “I created a lot of tutorials internally for the data scientists to use, so is it possible for me to, let’s say, exclude the content that is really, really tailored for the company, and then publish this information externally?” Because it could be valuable for the developer community outside of the company back then. And he gave me the go ahead, so that was how I started writing about my experience using AWS services for machine learning.
And then at some point, someone reached out to me from AWS saying, “Wow, you’ve written a lot. People are appreciating what you’re doing. Are you interested in the AWS Hero program?” So that was how I got into the AWS Hero program. At that point in time, it was also in the middle of the pandemic, and I was getting tired of product management, and I had another opportunity to move back into technical leadership. No offense to all product managers out there, it was just not my thing, I tried it for about nine months. I had an opportunity to lead a data platform in my current company, HRS Group, which is in business travel. And ever since then, I grew from technical lead, to head of, to director, and then leading an entire business unit along with my product management counterpart. So that’s the journey so far.
Shane Hastie: An interesting pathway. Machine learning, product platforms. At the recent Dev Summit, you spoke about leveraging AI platforms for developer experience.
Olalekan Elesin: Yes.
Shane Hastie: Let dig into that.
Personal hackathon – leveraging AI [04:16]
Olalekan Elesin: Definitely. So the talk, it was a very interesting one, because even though I lead multiple teams, at least 5 or 6 teams, software engineering teams, including data and AI team, I still find myself writing code once in a while, on my weekends, trying to keep up-to-date with what is going on, the new technology, just making sure that I’m up-to-date, so I can have productive discussions with the team leads with me, and also with the engineers. And on a Saturday, while watching… For the New Zealanders, you might not like this, I was watching the football, football in the English Premier League, and I wanted to build something, but I wanted to build something that was new to me, and at the same time, I wanted to leverage, let’s say generative AI tools available.
So I launched my VS Code, and then I remembered I had Amazon Q Developer. So from that, I asked it to create the application from scratch, and I was quite surprised. From that perspective, it made me realize what would’ve taken me maybe 4 or 5 hours, going into two days, because I also have the toddler to run after, took me about 30 minutes while watching a game of football at the same time.
But at that point, I realized this could really elevate developer experience, because as much as possible, we like to be creative as engineers, and also as engineering leaders, we want our team members to be creative, and at the same time, to make sure that we get value to the customers as quickly as possible. So this was where the idea behind the talk started from. So for me, it was quite an interesting experience to see that I could watch football on the weekend, at the same time, build an application that I wanted to try out. So this is where it started from.
Shane Hastie: I recall for myself the first time using some of these generative AI tools to do some “real work”. There was almost a feeling of, “ooh – I feel like I cheated”.
Olalekan Elesin: Exactly. Exactly. It happened to me as well. This one was quite funny, so I cannot remember the actual workflow itself that I was trying to build, or the actual application, but I remember it helped me to write at least 200 lines of cloud formation code to deploy the infrastructure. And I felt like, “I don’t want to think about this”. So I put it to Q Developer, and said, “Please write this. It should be able to connect to X, Y, Z, A, B, C”.
And then it wrote it out, and I felt like, “Oh my, this is cool”. And I’m lazy. And I remember smiling, and my partner is in the house, she was like, “Are you sure what you’re doing is correct?” I’m like, “Yes, it’s working”. To your point, it felt like I’m lazy. I’m not sure who said that, that the best engineers are the lazy ones, because they find ways to make it work at scale, with minimal effort possible.
Shane Hastie: So from that personal hackathon almost, experiencing it from the first time, what’s involved in bringing this into actually doing work?
Shifting from personal hackathon to scaling within teams [07:17]
Olalekan Elesin: Good point. So for me, it was a hackathon, but then to scale it within my teams itself, it’s a different experience entirely. Because on one side, we hear people granting interviews, saying, “There will be no developers in the next five years”. On the other hand, we see that this can really be a collaborative effort between engineers and artificial intelligence, and it has a change impact on people.
And one of the stories I heard when it comes to change was, a couple of years back, there was the compact disk. Even before that, there was the video cassette or whatever it’s called. And I remember having this, and so many people built businesses around renting video cassettes, even where I come from in Nigeria. And all of a sudden, things moved from video cassettes… Even we had people manufacturing spinners or rewinders for these tapes. All of a sudden, we had compact disks, and now we had to have compact disks. That meant that there was a lot of change. A lot of things would’ve happened to people that built their businesses around that. Not necessarily thinking about the digital content, but really about the hardware.
And for me, that was a light bulb moment when I am speaking with my teams to make them understand, “You have a concern with regards to this particular technology, but think about it when it works in collaboration with you. Then you see that you’re more productive, and look at it also…”
For us at HRS, look at it from taking the customer view first. “Look at it from how fast do you want to get features into the hands of the customer to validate every assumption you have based on the line of code you’re writing. And if you have one assistant sitting next to you as a pair programmer, enabling you to do creative work, and also helping you to write the code, why not leverage that?” And for me, the hackathon is, for me, scaling it across multiple people, and working through that change mindset is one of the biggest concerns that I think a lot of people don’t talk about.
Shane Hastie: So what’s involved? How do we make that work?
Olalekan Elesin: So this is an interesting question, and it’s difficult to answer. But my view, because we also started scaling it with my teams right now, and also across the engineering teams at the company, is to educate people about the change as much as possible, and get them to pilot it. So what we’re doing right now is getting a few people that we believe are enthusiastic, so the scientific approach. Believe that if you have a few people that can use this to validate the assumptions, that it’ll help them become more… It would elevate their developer experience, or their experience when doing development.
And after validation, doing a lot of trial and error, a lot of expectation… I don’t want to use the word expectation management, but there’s no better word coming to me right now, because there’s this assumption that AI can write all the code. You have to be aware that when you use it, it will make mistakes like every person when doing things initially, and you have to correct it along the way. And that expectation management, and get people to experiment as much as possible, and as quickly as possible, this is, for me, what is important.
A handful of people, get them to try it, get them to set the expectations, and use those people based on their learnings as multipliers across the organization. Not trying to do the big bang, saying, “Everybody, now go use…” Whatever it is called. But we have a handful of people, we have learning experiences from them, and then those individuals are part of different teams where they can then share their knowledge and their experiences with the team members, and also scale that across the organization.
Shane Hastie: As a software engineer who’s defined themselves by writing the code, you’re shifting from coder to editor.
Olalekan Elesin: Yes.
Shane Hastie: That’s quite a big shift.
Engineers shifting from coder to editor [11:07]
Olalekan Elesin: Definitely, it is. It is. So I remember, my… I think it was my second year in computer science in bachelor’s, that we were introduced to assembly language, or something like that. And we got to the final year, someone was telling me I had to write C#, and I’m trying to bring the relationship between what I learned in second year to what I’m being taught in the final year. And even in the final year, I remember we had to write Java, and more and more, I realized that as computer science has evolved with the years, that people have been building abstractions, let’s say low-level languages.
And this is what is happening even from my perspective also, that there are abstractions. And at this point, the abstraction we are getting into is where everyone, as much as possible, that has an understanding of how to build stuff, that has imaginative capabilities, can leverage these tools to become editors, and not necessarily trying to write the code from scratch. For me, this is it. It’s, we build a craft, we like to write the code, but then we need to build another craft, which is becoming editors, and letting something that can do it better, to a certain extent, to write the code, where we guide it across how we wanted to write the code.
Shane Hastie: You did make the point that these tools make mistakes. They make them very confidently.
Olalekan Elesin: Because they don’t know better.
Shane Hastie: Yes. This implies that that problem solving, mistake finding is another, not necessarily new, but a skill we have to build more strength in.
The tools will make mistakes [12:43]
Olalekan Elesin: Yes, of course. Yes, of course. And while you were asking the question, I remember one of the first production errors I made while being a junior engineer, this was when I was in the telecoms industry doing my first job, we had production on-premise data servers, and we wanted to analyze the log files. And I had done a lot of tutorials about Hadoop, and I didn’t know that I needed to move the data from where it is, which was on the production server, into another server, or another virtual machine which was separated from the production environment. So what I did was to install Hadoop Hive on the production server that was serving millions of transactions, and we had a major incident.
It didn’t crash the business, but it was quite an interesting one, but the learning there was that I could make a mistake as a junior engineer. And this is what our folks out there have to realize, that these tools are still learning. And another key learning I took here from that experience I had was one of the senior engineers explaining to me how not to repeat that mistake next time, and how to solve the problem next time, and guiding me through the understanding of, this is what happened, this is how to solve it, this is how to make sure that it doesn’t happen all over again.
And this is what we see with these tools, where… There are some techniques called retrieval-augmented generation, where you can put your data somewhere so that it doesn’t go off tangent with regards to the recommendations it comes with. And there are some mechanisms where you can also fine-tune an existing model, which is helping it to understand better the context. But until that time where it is really affordable for us, or everyone, to train or fine-tune their own model, these things will make mistakes, and also, we should expect that. I think, for me, this is the biggest part of the expectation management that I do with the engineers that I work with on a daily basis, that it’s expected to make mistakes. You have to be comfortable to see that it can make mistake, and be willing enough to take the time to edit what it’s generating for you.
Shane Hastie: When that mistake is subtle, so it’s not going to cause a syntax error, it’s not going to prevent compilation, but it’s one of those, and we’ve had them for a long, long time, requirements bugs effectively, where, “Oh, it’s solving the wrong problem”. What’s the skillset that we need to develop as humans to find those mistakes?
Building the skillset to ask the right questions [15:11]
Olalekan Elesin: Excellent question. I think the skillset we need to develop as humans is asking the right questions. So when I interview candidates as well, when hiring for my teams, we usually ask them, “How do you deal with requirements that are not clear, or problem statements that are not clear?” And this for me is one of the things that differentiate engineers from developers. Engineers always try to understand the problem, developers think about code.
And this is why, for me personally right now, I’m not seeing this coding assistance as engineers yet. They might evolve to that level, but they are more development assistance. And the reason why I take it in the direction to answer this question is that being able to ask the right question, and this is what people call prompt engineering, is one of the skills that we need to add to what we do. If you have engineers that know how to ask the right questions, they would get a lot more productive by using these tools, because they can then ask it to refine, to change a bit, to adjust what the context of how they’re asking the question to get better responses, that they can then edit from these tools. At least this is what I do. So the skill is prompt engineering, but the underlying thinking behind it is being able to ask the right questions.
Shane Hastie: We hear horror stories of security concerns around these tools. How do we make them secure for our own organizations?
Tackling the potential security risks [16:42]
Olalekan Elesin: This is a very tricky one. First, I think at the QCon developer conference also someone asked a similar question. And my first answer is that security is not there to restrain our space, it’s to enable us to do the right thing. And first and foremost is to make sure that if in any tool that anyone is using in an enterprise setting, please ensure that it is aligned with your security objectives or security policies within the company. This is the first thing.
The second is that we need colleagues to think in terms of the customer, and I think in terms of the business itself. Customers want to have more and more control with regards to their data, so if I, as an engineer, I am responsible towards my customer, and I as an engineering team leader, person leading multiple engineering teams, we have a responsibility towards our customer, and my customer says, “I want my data not to be shared with some AI tool”. Simply don’t do it. Also because it has implications on the reputation of the business if that is not done right. So that responsibility, we all need to live with it, and understand that it has implications on the customer, has implications on the business, also has implications on how we do our jobs by ourselves. For me, this is it.
And I remember when I was building the data platform, and then we said, “We want to be security first”. After that, “No, but this is how to do data responsibly”. It’s the same for me, it’s security first, everything else comes after. As long as it’s satisfying the customer objective with regards to security, wake me up in the middle of the night… This is what I tell anybody, “Security first”. And fortunately, I’m also in Germany, so we’re super cautious about data security.
Shane Hastie: What are the, I want to say future risks, considering developer education, for instance? You learned assembler, are we going to teach new developers assembler, or are we abstracting so far away that we almost don’t know what’s happening underneath?
Developer education needs to evolve [18:47]
Olalekan Elesin: Education definitely needs to evolve to make sure it’s relevant for the generation that we’re educating. That said, anyone being educated needs to be curious with regards to, how did we get here? And that curiosity is another trait of really good engineers. So example, one of my own personal experiences was when I started using Apache Spark for big data processing. I remember going through the Spark GitHub repository just to understand how the people that contributed to that amazing project wrote the code. And this was just being curious.
I also remember going through some parts of Hadoop at some point, some parts of other libraries that I used at some point. And I think that irrespective of the fact that we need to educate people with what is needed right now, those engineers that will be chief engineering editor, or however… Editor engineer, however the title might look like, need to be curious to understand, how did we get here?
When I mentioned that I stumbled into product management, my responsibility back then was… Even though I was building an AI platform as a technical product manager, I needed to understand how this AI platform will enabled the company to have a competitive edge. And I remember something on the book by… I’ve forgotten his name, his last name is Porter, that wrote Competitive Strategy. And this was a book written, I’m not sure, 1990 something, just to study, to really now understand how people thought about competitive strategy a while back. And what I’m saying is, engineers need to be curious with regards to understanding, how did we get to where AI is enabling us to write code and solve problems faster than what people 20 years ago, five years ago, 10 years ago could do?
Shane Hastie: How do we train that curiosity?
How do we train for curiosity? [20:37]
Olalekan Elesin: Oh my God, that’s a really… To be honest, this is a question I ask myself every day. The only way that I’ve seen not to lose it is, we need to find a way to not lose the baby part of our brains, the toddler part of our brains. Where I see my toddler, and he’s asking me, “Daddy, why?” And sometimes I want to answer him, sometimes I’m like, “Maybe I need to help him understand the reason why”. And that curiosity, it’s a trait, difficult to train, but it can be coached.
So in some sessions when I’m having, let’s say one-on-ones with the engineering team leads that work with me, or the skip levels, and they make a statement about something that… A problem that they’re struggling with, the first thing I ask is why. And when I have that discussion, the next thing I do is, Iinform them, “I want you to always have this type of approach to problems”. And so asking why, so that you get as close as possible to the first principles, using the first principle thinking. I’m not sure it’s easy to train, but I know, for me, what has worked is being able to coach it with the people I have direct interactions with.
Shane Hastie: How do we help engineers be coachable?
Helping engineers become coachable [21:52]
Olalekan Elesin: Oh my. There is this book called Billion Dollar Coach written about Bill Campbell, and I remember… I think I stumbled on it two years ago, I think he passed on maybe about three years ago or so. I remember stumbling on the book, and I cannot remember in detail about his approach to coaching people and making people coachable, but what has worked for me, and one of the learnings that I’ve made mine is really explaining the why, and to help the engineers understand how I also think, and making them understand that there is a world before now. And the world before now to get into that means to think in terms of first principles. So what’s the minimum basic element of making this work? And really getting them to really, as much as possible, go back to rethinking, because that’s also the foundation of solving the problem.
Another way I differentiate between engineers and developers when I’m interviewing is, does this person really understand the business problem, or the customer problem they’re solving? And this is how I look out for curiosity in engineers again. And if I don’t see it, I’m happy to share feedback, “I need you to understand the business problem. I need you to understand the customer problem”. And then if I’m talking to skip levels, it’s the week from now, “Send me your detailed understanding of what you see as the problem itself that you’re solving, not the task that you’ve been assigned”. And as leaders, we need to constantly push our engineers in that direction to really realize, “What problem am I trying to solve? Not the lines of code that I need to write”.
Shane Hastie: Lekan, a lot of deep thinking and good ideas here. If people want to continue the conversation, where can they find you?
Olalekan Elesin: They can find me on LinkedIn. It’s only one, but my name is longer on LinkedIn, so it’s Olalekan Fuad Elesin, and I respond to messages. I have put an SLA on it maybe in three days, but as quickly as possible, in less than three days I respond to the messages. I’m also not as active on X, but I’m very active on LinkedIn, and this is where… I use my LinkedIn to share my thoughts and also engage as much as possible.
Shane Hastie: Thank you so much for taking the time to talk to us today.
Olalekan Elesin: Thanks a lot, Shane. Thanks a lot for having me. Super interesting discussion.
Mentioned:
.
From this page you also have access to our recorded show notes. They all have clickable links that will take you directly to that part of the audio.