Month: January 2025
Presentation: Unleashing the Potential of VR: Building Immersive Experiences with Familiar Tools
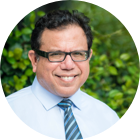
MMS • Ian Thomas
Article originally posted on InfoQ. Visit InfoQ
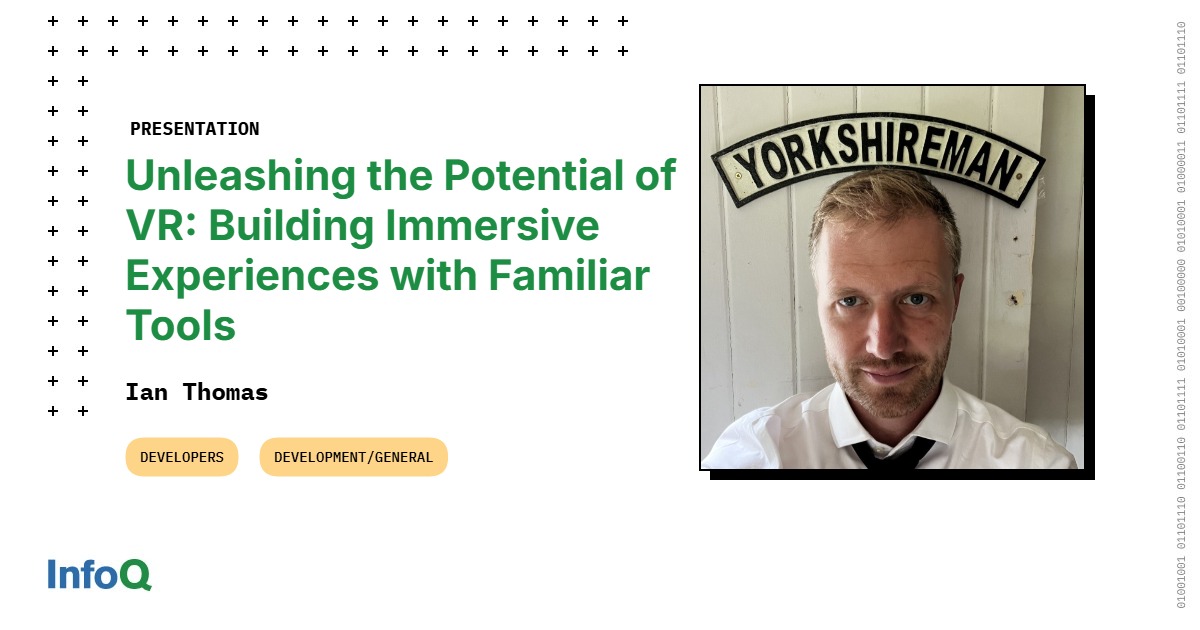
Transcript
Thomas: I’m going to talk about the potential of VR in the context of what we’re building at Meta. First, though, given this is the emerging trends in frontend, let’s go back 15 years to 2009. Some remarkable things happened that year, some less remarkable things. I’m wondering, does anybody remember this at all? This was the coolest thing I’d ever seen at the time. If I play this video, hopefully it will give you a demonstration of how that worked. This was something that North Kingdom produced. It was a Flash experience, if anyone can remember Flash. You held up the sheet of paper, stuff popped out, animations, you could blow on it. It was really cool. This was the most amazing thing I’d ever seen.
At the time, I was working as a designer in an agency. I thought, if an internationally renowned agency can do this, why can’t I? I had a play around with this. This is probably the first introduction I ever had to working in 3D. It was the last until about six months ago. It was really cool, though, and it opened my eyes to what’s possible for different things that you can do with 3D and how you can make immersive, interactive experiences that span between the real world and not.
Obviously, Steve Jobs came along not too long after this, and killed Flash quite dramatically. My nascent interest in that and generative art in Flash stopped, but thankfully, JS was there, and I started to pick up more of this kind of work. This quote from Brendan Eich in 2011 still holds true today, and I think it’s part of the theme of this talk, is that there are technologies that you can pick up as part of developing for more commonplace platforms like the web, that you can then transition and use throughout your development experiences in other platforms too. From that point, web technologies, they’ve come a long way.
Most people, I’m sure, will have experiences building for the web, potentially for mobile as well, maybe even using crossover technologies like Flutter or React Native to build for both using web technologies. The thing to think about is, if you’ve learned these technologies, what do you do if your primary delivery platform isn’t a desktop computer, a laptop computer, or a mobile device? What if it looks a bit more like this, where you’ve got multiple different sensors, you have real-world tracking. It’s head mounted. It’s immersive. You have very non-typical interaction patterns.
I’m Ian. I’m a software engineer at Meta. I work in Reality Labs as part of the Meta for Work area. I work on a product called Workrooms, which I’m going to be talking through, to give you a bit of a flavor about how we’re building some of our apps for VR and AR.
What Is Workrooms?
First, what is Workrooms? This is our immersive, collaborative office. It’s a way for you to be present with your colleagues without actually having to be physically present. I think, as much as I dislike advertising blurb and videos, they’re probably the best way to show you how this product actually works. This will give you a bit of a flavor about what Workrooms is.
We actually provide a few different experiences for Workrooms. There’s the main one I’m going to talk about in VR. There are also some that use the web and video. The web surfaces that we build are fairly standard for how Meta builds web applications. We build on top of a lot of the core infrastructure that powers things like Facebook and Workplace.
The VR surfaces for the Quest, we use Unity as our delivery platform or our environment for building this app. We have to support the different capabilities across the Quest line of headsets. I think we support from the 2 onwards. Then all of our backend systems. There’s a lot of background processing and real-time clients and stuff like that. That’s, again, provided by a lot of the core infrastructure that is powering things like Facebook and Messenger and Work Chat.
The Architecture of Workrooms
Workrooms as an application in a very simplified way. It involves headsets and clients as the main interaction point. You can join via the www client, which is a way for you to use your video camera and microphone. You can engage in a video call. The headsets provide the more immersive experience. We use a variety of different real-time systems in the background to provide Voice over IP or the 360 audio state sync for avatars, and various bits and pieces to support whiteboards and other features of the application. A lot of the backend is in the core stack from Meta. It’s GraphQL and framework TAO.
Obviously, that’s got the support of the scale of Facebook behind it. Developing for web is a standard process, where it’s a well-worn path. In Meta, this is one of the most easy to get going ways of building something. It’s very mature. You can spin up apps. You can work really quickly, use on-demand environments. It’s very familiar to a lot of people. React, obviously, being the primary frontend framework that we use there. Developing for VR is a different story altogether, because it’s far less mature. There’s less support for it across Meta. Then, when you look at the headsets as well, you’ve got new challenges that you have to adapt to. Things like the frame rate. It becomes increasingly important to maintain that, otherwise you can actually cause physical symptoms for people. Things like motion sickness and feeling head-achy and other unwanted side effects. The six degrees of freedom.
When I started working on 3D, again, 6DoF was like, why are we talking about this? It’s 3D, isn’t it? The six degrees of freedom is about tracking the position of your head and the location of your headset in the room. It’s one of the reasons why sometimes you’ll find it funny if you put your headset on when you’re on a flight that things start to whizz around in a really weird way, unless you put it in flight mode. Underlying the stack here we’ve got Unity and the Android open-source platform, which is what VROS is built on top of, and all the XR tech that we’ve got in Meta uses. Essentially, this is an Android phone strapped to your face.
To give you some context about the kind of challenges that you might have when you’re actually thinking about building for this thing. Rendering UI in VR is an interesting problem to consider. The main element that we talk about when thinking about rendering here is the compositor, because when your app is generating its scenes, you’ll end up with an eye buffer similar to this. In fact, you’ll end up with two, because we have two independent displays within the headset itself. If you were to project this straight onto the display as it is, it would look really bad, because they’re so close to your eyes, there’s like a pincushion distortion that’s there.
Instead, the compositor will have a few extra steps in the processing pipeline that eventually applies this barrel distortion to make it so that when the images are presented to the user, they appear to be correct and flat. Obviously, this is not a free operation. There’s quite a bit of work that goes into doing that.
What are the Limiting Factors of Scaling?
How does that affect us? What does that limit? The scaling effects of this mean that we have to think about how many people we can have concurrently in meetings, how many features we add to our applications, whether we enable pass-through because AR adds extra processing requirements. The key thing to bear in mind here is that perhaps when we talk about web, we’re talking about hitting 60 frames per second, but for us, we’re targeting something a little bit higher res, so we want to hit 72, which gives us just under 14 milliseconds to do all the work per frame.
Ideally, we’d like to aim for 90, because it’s an even smoother experience. It helps to make people feel more comfortable within VR. If I break that out into what the render cycle looks like, you can see, there’s a whole bunch of VR tracking going on. The CPU does a lot of work to generate the scene and process all of the business logic that goes on and within the application. Then the GPU will render at the scene. The compositor steps in, and that generates the final displays to go to each eye. What happens when you don’t hit that buffer, you don’t hit that 14 millisecond? If our GPU extends beyond where we want to render, then we drop frames, we have latency there. This is where we increasingly find the experience becomes very jarring for people, and we have to be extremely mindful that if we don’t hit this, that this is where the real-world effects, the physical symptoms can start to affect people dramatically. We can monitor this.
Oculus tooling has loads of great performance debugging tools. This is one that you can see within the application, but there’s also other ones using the Developer Hub, so you can use Perfetto to grab traces from the headset and see what’s going on. Here you can see that frame drop is represented by latency, and it’s relatively low in this example. If you’ve got a particularly heavy workload, and as I mentioned before, AR makes the workload even heavier because of the amount of sensor data it’s processing, this is something that you have to be extremely careful to make sure you’re not introducing excessive latency.
Compositor Layers
One way that you can improve things, especially when you think about elements of your UI that maybe need to be crisper, is to use something called compositor layers. Compositor layers are a way to bypass some of the processing that happens within the compositor itself. They’re a way of saying, I’ve got a texture, and I want to render it to a shape, and I want it to appear at this point in the scene. You avoid having to do some of the sampling that would decrease the quality of the images that are presented to customers.
At a high level, what you see here is the overlay. The quad overlays is a compositor layer. The eye buffer then has holes punched in it, which is where the UI will eventually be displayed. This represents here as these small blue rectangles underneath the compositor. They have the benefit of operating largely independently of the application, so they are guaranteed to run at the full frames per second of the compositor, which is at least as good as the frame rate of the app.
Hopefully, you can see here the difference that this has on the quality of rendering. For things like text, it’s extremely important that we have the ability to drop into this layer to show that the text becomes readable. Because again, we have a limited resolution within the headset itself. It’s incredibly important that when you’re presenting stuff, especially in a work environment, people can read it. You can see here how the compositor layer on the left eye frame buffer leaves an alpha channel. Hopefully, when you flick between the two, you can start to see the difference in the aliasing and the quality of the text.
How We Use JavaScript Across Workrooms
I mentioned about web technology, so let’s have a think about how JavaScript is used across Workrooms. I’ll go back to my high-level diagram here, and pop in some of the technologies that you might find. We can see that JS, React, and Jest play a part in both our web and our headset stacks. That’s because we found that React is a really effective way for us to enable people to work on the apps and build these 2D panels without necessarily having to become deeply expert in building VR applications. If I zoom out to look at a reasonably high-level view of this, the Unity app has a bunch of C# code in it. It also has a bunch of native libraries and native code that we write for performance optimization reasons.
Then, there’s also a whole load of JS, and the JS is largely where the UI resides. You can have a look at a slightly more grown-up version that’s on the Oculus developer portal to see how these things come together. Then they ultimately all talk to the OpenXR runtime that sits within the VROS layer in the headset. React, and specifically React Hermes and Metro, how do we use this? If I look at the UI for Workrooms, the key thing that you’ll notice is there are some 2D flat elements, and these are all the prime elements that are going to be part of the React render case. We have multiple components here that get rendered in different places, and these will sometimes end up as overlay layers in the compositor, or sometimes they’ll be rendered in the application itself.
React VR is a way for us, like I said, to take JS, build a texture and then integrate it within the Unity environment, and so that we can generate lots of UI quickly, and we can use all the familiar tooling that we have with React. It’s a really nice development experience as well, because we can enable things like hot reloading, where previously it would have been a bit more tricky, with something like C#. The renders, as with all these things, they look extremely familiar. If you’ve done any React development over the last however many years, you’ll recognize this instantly.
One of the nice benefits, although React VR is different, we have a different backend to React Native, we do have the ability to use some of the React Native components that are part of other applications, so things like animations can be brought in there. A key thing that’s made some of the things I’ll talk about later really easy to do is, building the render tree allows us to have addressable elements via test IDs, which is something that I’ll go to later on.
Obviously, there’s tradeoffs with this. Why would we choose React over Unity? The main thing is productivity. It is a lot quicker, and it enables familiarity. Engineers who aren’t necessarily native Unity engineers, or haven’t been working in 3D for long, they can onboard a lot more quickly. If we’ve got a UI that we want to share between parts of the web and some of the parts of the VR app, that’s possible too. Hot reloading, like I mentioned, there’s the component inspector. We can get the component hierarchy for test IDs, but it doesn’t completely absolve you of knowing about the underlying platform, so you still have to know a little bit about Unity and C# to be able to be effective.
One of the key nuances here is state management is a little bit different to how perhaps you would do this in a web application, where quite often we would keep state within components. We try and avoid that as much as possible, because of the need for rendering UI and the collaboration between different headsets, so multiple people using the app at the same time. Thankfully, the great John Carmack agrees that React is a good idea. After seeing it get deployed across the Oculus apps that we build, he came out and said that React definitely is a faster way of building UI than Unity. If John thinks it’s good, that’s fine by me.
It makes sense, because if you consider how things need to change and the rapid nature of building UI, they’re right at the fast, thrashy end of this spectrum. We don’t necessarily want to optimize for long, stable infra level stuff. We want to be thinking about how we can enable change as quickly as possible. Developer experience and ease of use definitely factor really highly in our decision making there. Perhaps some of the tradeoffs that we would get there, like performance, we can offset by the fact that we get much better productivity.
Creating a new panel is fairly straightforward. You write the component in JS, register it for Unity use. Then, because everything in Unity is a game object, we need to create one of those and use the component that we generate as an element on the game object, enable things like colliders so that we can have interactions, and then we position it within the scene. I mentioned about state management, and that’s where the React VR modules come in, because we don’t want to have too much of the state living within the JS code. We want to be able to communicate between JS and C# fairly easily. React VR modules allow us to do that in a really straightforward way. You can see here, within the application, you can choose the table layout for the room.
Obviously, this is something that you can’t have one layout and then another person in the meeting have a different layout. This is something that needs to be synchronized across everybody. We want to be able to maintain that state in a central place. Again, these modules are really simple to create. You define the type in JS, run some code generation, that’ll produce a JS class, and it will produce a C# interface. Then we implement that. It provides mocks so we can test and all sorts of other useful bits and pieces.
It’s a really straightforward way of making sure that we can enable easy interop between our C# and our JS layers. Performance is really important. You can see here, we have critical decisions to make around which UI is going to be rendered as a compositor layer, and which UI is going to be rendered as an application layer. We have a limit. There is only a certain number of compositor layers we can support, so we have to be really careful where we choose to use them. Typically, anything that’s going to be front and center, like notifications, we will try and make sure that they are rendered as crisply as possible. We also need to be mindful that we still want to have as much budget left for all the work that’s going on in the background.
Building in Quality
Quality, this is where things get quite interesting. Workrooms at a high level, like I say, is a multiplayer application. When you’re building these multiplayer applications, it seems difficult, it might be difficult to consider how you would test multiplayer scenarios. We also need to bear in mind that it’s not just VR, there’s VC users, there’s web users, and all of these different parts of the stack. They interact with each other, and they need to collaborate in a way that we can test in a useful manner. This is where we can look to some of the tooling that’s provided centrally by Meta. You might be familiar with this. This has been published about how Meta developer environments work, and how we build code and deploy apps. We rely as much as possible in a lot of this core tooling to make sure that we have full support for testability and managing our test environments and building apps that we can use in CI.
The key elements that I’m going to talk about are how we edit and how we test, and then how we review, and how we understand whether we’re shipping something that we think is of high enough quality. When we think about testing, we often talk about pyramids and how we want to balance between unit integration, end-to-end. Clearly, for us, end-to-end tests are going to be extremely important. However, they have some drawbacks, and we have to be really careful in our decision making to know whether we want to go really deep into the end-to-end area, or whether we would be beneficial just thinking about more integration unit tests. The trophy that you see here, has been put forward as a concept I think by Kent C. Dodds, as a way that web products can really benefit from the depth of coverage that you get from an end-to-end test.
If we think about this from a VR perspective, there are some drawbacks there that we have to be really careful to consider. Web, as I said, really mature. There’s loads of great tooling available, good browser support, battle hardened libraries like Puppeteer. There’s lots of value from your end-to-end tests, and they generally are not too flaky or too slow. The size and the scale of the www code base in Meta has proved that there’s lower reward for going for an integration approach here.
The end-to-end testing tends to win for us. VR, on the other hand, there’s less coverage here. It’s not something that’s so widely supported, because we’re quite a small group, really, compared to the web users. Because a lot of the developers are coming from a game development background, there’s still quite a heavy reliance on manual QA and play testing through people actually putting the headset on, creating meetings, joining them, and finding the issues in using human effort rather than automation. We don’t have wider support from a test framework, subset of features. Tests can be very slow. There’s also some really interesting challenges around the fact that they’re going to have to be on physical devices.
One of the problems that I’ve come across in the last few months was that when we bought new devices and they were put into our device labs, they were put at a different orientation. Bear in mind that we track where you are in physical space. That meant that when we were recording test videos and we were trying to assert on things in the environment, you were looking straight at the ceiling rather than at your desk or looking at the VC panel. Emulators are coming. That’s something that we’re investing heavily in, but they’re not quite there yet. One of the crutches that we have is that Unity is obviously a visual environment, so people tend to favor doing the quickest, easiest thing, which is sometimes writing the tests that run against the editor itself. Again, it’s not the same as the deployed artifact. It’s not the same as the thing that someone’s actually going to download and use on their headset.
I’m going to start this running in the background, because it’s a relatively long video. This is the sort of thing that you can see from a test when we write them. You can see we’ve got the two views here that are distorted, as mentioned before, from the compositor, and the environment loads up. We’ve joined the meeting as a single avatar, and then we’re using our test framework to cycle through the different parts of the UI and check that it’s working as expected, that things change, and we can assert on what’s present, what’s not present. We can check whether interactions that happen between JS and C# are working correctly. We can really dig into, is this thing doing what it’s supposed to?
The really cool thing about this is that we can have multiple devices at the same time in the same test doing the same thing, and we can assert that they’re all doing it in the way that we want to, which is critical when you consider that we’re also supporting multiple versions of the Quest headset. Some will have certain features. Some will have more power. The Quest 3, a much better device than the Quest 2 in many ways, but it also has different drawbacks and different things that we need to consider. The editor environment, like I said, is a standardized thing in Meta. This is generally where I spend most of my day. I look at Visual Studio code a lot. What you’ll notice is there’s an awful lot of stuff on the left-hand side, custom extensions. This is the key way that we can start to standardize and make testing easier and building quality easier.
As I said, we need to think about how we test on these physical devices. We’ve got a way now to avoid having people with local versions of the headsets, their instance, or whatever, their Quest 3, their Quest Pro, that they might have configured slightly differently to the way that it gets configured for a consumer. We have the labs available to us, and we can use a tool that we call RIOT, which stands for Run It Over There, and it allows us to lease devices very quickly using a visual workflow in Visual Studio code.
We can lease as many of these as we need to, to run our tests and actually start to use different versions of the device to validate our work. You can see here I’ve got some command line tooling as well. We’ve actually managed to completely inline this workflow. Now when you write a test, you can actually define the devices that you want within it and the application that you want within the test file. Everything is just a case of click and go, once you’ve got your device leased, which is amazingly streamlined.
The reason I’m talking so much about this is, again, this is another familiar tool. This is all built and operates using Jest, a framework that when I first started using it in 2014 felt like it was the worst testing framework in the world. It’s something that I now think is absolutely invaluable, and I spend pretty much my working life thinking about how we can make more of it, because it’s a really critical part of our quality arsenal. End-to-end testing looks a bit like this. As I mentioned, there’s a way for us to lease these devices and have different devices that we talk to. We don’t just limit ourselves to headsets, although that’s the primary focus of my work, is making sure that our tests are operating across multiple headsets at once. You can also have Android devices, iOS devices, and smart glasses, for example.
These tests, they’re very familiar to people, and they rely heavily on the Jest end-to-end framework that we’ve been working with internally. These tests will be familiar to anybody that’s written an end-to-end test for the web. It’s very straightforward. We have to pull our environment through to make sure that we’ve got access to the UI driver. Again, because we can have multiple devices leased for a test, we want to be able to have access to multiple UI drivers. This is the code that powers the test that I showed you in the video earlier. It’s a very straightforward, go find this thing, click it, go find this thing, click it. We can do things like pull back the text. We can assert that something is present, something isn’t present.
Again, this is where the power of the React component tree really helps us out and makes things so that we can understand the state that our application’s in. A little way down the middle there, you can see, I’ve got this sendSystemEvent. This is another way that we bridge between JS and C#. In the background, we have a small web server running on the device during tests. This allows us to say, can you please do this? Or, can you give me this state so I know what kind of seat layout I’ve selected, or have I managed to change the seat position that I’m in? If I zoom out one level and you look at the environment, you actually have access to the VR headset itself, which is another way that we can start to think about play testing in an automated fashion, all the things that we want to do that a user might do in the real world.
You can see here we have doff and don, which I find is quite quaint as an API. You can doff your headset, make sure that the device thinks it’s taken off, and then you can don it again. It’s a way for us to then assert things like, is the right UI appearing when someone puts the headset back on, is our app crashing, that kind of thing? You can see here, this lockView, this is something that I alluded to earlier. We noticed that certain headsets in certain configurations in the labs ended up pointing the wrong way, and so it was difficult to know whether something was actually working or not, because one thing would be perfectly set up, and another device would be pointing out the window or something.
Because we are building on top of Jest, that means we also get the full support of a lot of the developer tooling around the integration and CI part of the Meta life cycle. Here you can see, this is the analysis that we can pull back from a test to see what’s happening. We can put manual checkpoints in so that we know performance markers to see, is this thing getting faster or slower? What’s causing bottlenecks within our application? This is very high level, but you can also dive in to the device specifically. You can use something called dumpsys to go and really dig into the details of what processes we’re running and find out if there are any performance issues there. You can see as well we capture any crashes.
Unfortunately, this one looks like it did have a crash in it, though, weirdly enough, the test passed. There you go, a little hint at some of the interesting challenges around the flakiness of testing on VR. This then integrates further into our CI so that we can see the state of our application at any time and the state of our code that we’re writing. This is the Fabricator UI that’s part of everyone’s core workflow at Meta, no matter whether you’re working on VR, www, low-level infra systems. The key thing here, down at the bottom here, it says it’s landed, and we can see where it is in the deployment cycle. There’s also a whole bunch of really useful information here about which tests get selected and run. When you are deploying these apps and building them for different use cases, and we share a lot of code because we work in a monorepo, the ability for us to have our tests run on other people’s changes is massively important.
The fact that we’ve standardized this device leasing approach, we’ve standardized the way that we go through the process of running tests, and we’ve got the tooling, people will see these tests running on diffs that they didn’t even know were going to touch something to do with Workrooms. We get an awful lot of signals. You can see here, we’ve got 2500 different checks, and lints, and things that have run.
Key Takeaways
The key things from my learnings of VR, is that the React programming paradigm has really unlocked the power for us to bring people on board quickly. I’ve never really dug into game development. I’ve never really dug into C#. I’ve never really worked in any kind of 3D environment at any great depth, but I was able to onboard within several weeks and was productive writing tests, building code, and getting the app deployed. That’s partly because it was so familiar, because React allowed me to do that.
Jest as well, because of the familiarity of Jest and the way that you can leverage the existing experience you’ve had, maybe building for the web or mobile using it, it allowed me to get in and dive into how we can validate the quality of our application quickly. It means that there’s no overhead to onboarding. I can really make efficient tests. I can understand the state of things. I can treat it like a browser, and I can also interact with different devices at the same time. That doesn’t absolve us of learning about the different platforms, so there’s still a lot to do. You still have to understand how things work underneath, things like the compositor, compositor layers, the render pipeline, the problems that come with performance.
You gradually have to onboard to those as well and understand where React benefits you, but also where React maybe offers you some of the limitations. It’s amazing what you can do with the web platform, so investing in your skills in that area is definitely a worthwhile thing. Always bet on JS.
Questions and Answers
Participant 1: Do you use those Jest tests for any of the performance concerns, or is it just like, this button shows up and I can click it?
Thomas: Can we use the Jest tests to understand some of the performance concerns? Yes. The way that we have test suites set up is we have some that run specifically to validate the UI, some to understand how the multiplayer aspects work. We spawn lots of avatars and see what happens if we have loads of people trying to do the same thing at the same time. Then we also have tests that turn off as much of the debugging and insights as possible, and just hammer the environment.
Workrooms as a product is one of the most performance intensive applications that you can put on the Quest platform, because we’ve got video streams coming in. We’ve got multiple avatars that have got real-time data being synced between them. You’ve got remote desktop, so you can bring your laptop or your desktop computer into the environment as well, screen sharing. Then, you can also turn on pass-through. There are all these different things that really stress you out. Being able to set that up using some of the tooling that you have with the VR device API allows us to really go, let’s just run this for 10, 15 minutes and see what happens. How many crashes do we see? Do we see much in the way of drop-offs in the frame rate?
Participant 2: How far do you think we as an industry are able to have VR as the first-class citizen as we have with mobile? How far we are from this? If you believe that it will be possible to render my React Native apps in devices like Quest automagically, like we do with iOS and Android.
Thomas: Can we use React Native to render apps for Quest? How far are we from this being as prolific as mobile?
When you go onto the Quest store and you see various apps, a lot of the panel apps that you see are React apps, and they’re built with React Native. Yes, that’s going to be possible. I don’t know that we have the same capability at the moment that you might think with the Vision Pro, where they can take an iPad app and they can put it into the VR space. It’s not wholly impossible, but yes, from a React Native point of view, we definitely do that. A lot of the 2D panel apps that you will interact with do use that a lot.
VR as a First-Class Citizen
In terms of the proliferation, probably one of the biggest challenges from a product experience perspective is the friction of actually putting the device on and the time it takes you to get into the meeting. If you’re comparing to like a VC call, it’s pretty quick. You click the link and it spins up and your camera comes on and off you go. Matching that, I think, is a key element of making it more of a ubiquitous experience. I think that will apply broadly to different types of applications as well. Once you’re in the environment, and as long as the comfort level is there, the heat in the device isn’t too high, yes, there’s a lot of things that you can do in there that actually make you think, yes, this is my first choice. An example of that is one of the features in Workrooms.
When you launch the app, you go into what we call the personal office, and in there, you can connect using the remote desktop software, and you can have three screens. Those three screens offer you much more real estate, perhaps, than you would have just working on a laptop or whatever. Because the devices are pretty small that you take it to a coffee shop or take it somewhere on holiday. I’ve been using it in my hotel room while I’ve been in London. I’ve now got this really expansive thing. For me, the benefit of working in that means it becomes a natural choice. Over the coming years, as I’m sure, hardware will get better and the displays have higher resolution, that will become more appealing and you’ll think, this is the sort of thing that I will definitely reach for my headset first, and maybe not my laptop, or maybe a combination of both, or mobile.
Participant 3: I’m really curious about how closely related Meta’s teams are around the software and the hardware pieces. For example, as you’re building stuff in React VR, as you’re building Workrooms, how much influence does your group have on in terms of what comes next for Quest v next, or insights you have about what might be available in the future? How closely that tracks.
Thomas: How closely do the software teams work with the hardware teams within Reality Labs? Can I share any insights about the future?
I can’t really share any insights about the future, but if you go onto The Verge, they somehow found all the information about our hardware roadmap last year. Maybe have a look at that.
In terms of the collaboration between software and hardware teams, like most software now, there’s multiple layers of abstraction. The teams that we engage with the most are the VROS teams and some of the teams in the XR world who are looking at the core system libraries to support things like spatial audio, guardian boundaries. We have less influence on the hardware roadmap. Even internally that’s quite well protected and guarded. We do have to know quite early doors what’s coming. If you look at the move from the Quest Pro to the Quest 3, one of the things that we gained was really superior pass-through, but we lost the eye tracking support and the face tracking support.
Which for us as Workrooms, that’s a really great feature, because part of the draw of being in that product and using it as a meeting is the body language and the feeling that you’re actually connected with somebody in the same area, but you’re not necessarily physically located together. The facial aspect of that was really powerful. Knowing what’s coming on the hardware roadmap and maybe understanding the implications that has for us, that’s critical, but it tends to be more of a feed-in rather than us influencing it.
Participant 4: What do you see as the key limiting factor in achieving what you’d like to be able to achieve at the moment. What do you think about what Apple are doing in this space, because it seems to be a different direction to where you’re going.
Thomas: What’s the limiting factor for our products? What are my thoughts on Apple and the Vision Pro and what they’re doing?
I think, personally, the big limiting factor is still the performance, because it is still a fairly low power device that you wear. We’re trying to get them as light as possible. We don’t want to have the battery pack that you clip to your boat, for example. The heat generated makes it uncomfortable and all that stuff. Seeing performance improvements as will naturally happen with hardware evolution is good. The quality of optics as well, making the lenses better and being able to support higher resolution, that will also help. Like I said before, it’s the time it takes for you to get into the environment, because they take a lot of compute to make happen these experiences, not just from the rendering point of view, but just the whole managing state and the real time and data aspect. Any performance gains we can get there to reduce the barrier to entry, I think is the critical thing.
In terms of Apple, I try to use one. I haven’t actually managed to get my hands on one yet. I think it’s really interesting the way that they’ve positioned their apps in their ecosystem to be more around individual usage. I know that there’s a bit more coming now with the personas and FaceTime. It did feel much more like an individual consumption device. I’m not sure I’m 100% on board with the wearing it for family gatherings and taking videos and stuff, because I think that’s a bit weird and intrusive. You might see, I’ve got my Meta Ray-Bans on.
I think this is a far more nice form factor for, “I’m at a family gathering. I’m going to take a quick photo, and I don’t want to get my phone out and be intrusive” .There’s obviously potential here, and there’s a lot we can do. I think the more competition there is, the more people are exploring this area, the more beneficial it will be for everybody. I’m really keen to see where it goes.
Participant 5: How much time do you spend in Workrooms, productive?
Thomas: How much time do I spend in Workrooms, productive? I probably use it for an hour or two a day, depending on the meetings that I’m in. Obviously, there’s an added incentive, because it’s the product that I work on, so I tend to want to dogfood it a bit more. Workrooms as a collaboration platform is widely used across Meta. A lot of it is still video calling, but it is heavily used. We have work from VR Days, where they count as in-office days, so people can have an extra day at home, and they can use their headsets, and they can join meetings in VR. What we found through that is actually people are starting to use the personal office a lot more as well, for reasons like I said, you don’t necessarily have to have three massive physical monitors in your workspace. You can sit there and you can be productive quite easily with it.
The thing that I enjoy about using the product is that I’m more present in the meeting. When you’re on a video call, it’s quite easy to get distracted, change tabs, fiddle with emails, go on to workshops, and the thing, whereas when someone can actually see you and your body language is there, you just are more present. The 360 audio, the spatial audio, I think that was the biggest thing. You can’t really see it in a video or understand it to actually experience it, but because it’s there in those specific places within a room, there’s less crosstalk and that awkward, “I’m sorry, you go”. That just kind of is gone. It’s much more efficient as a collaboration medium as well. Just need the widespread adoption of the devices and people to think about it as their first choice now.
See more presentations with transcripts
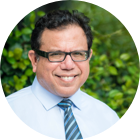
MMS • RSS
Posted on mongodb google news. Visit mongodb google news
Avior Wealth Management LLC trimmed its position in MongoDB, Inc. (NASDAQ:MDB – Free Report) by 90.2% during the fourth quarter, according to its most recent filing with the Securities and Exchange Commission. The institutional investor owned 134 shares of the company’s stock after selling 1,240 shares during the quarter. Avior Wealth Management LLC’s holdings in MongoDB were worth $31,000 at the end of the most recent reporting period.
Several other institutional investors have also modified their holdings of the company. Hilltop National Bank raised its stake in MongoDB by 47.2% during the 4th quarter. Hilltop National Bank now owns 131 shares of the company’s stock worth $30,000 after acquiring an additional 42 shares in the last quarter. Quarry LP lifted its holdings in shares of MongoDB by 2,580.0% in the 2nd quarter. Quarry LP now owns 134 shares of the company’s stock valued at $33,000 after purchasing an additional 129 shares during the last quarter. Brooklyn Investment Group acquired a new stake in shares of MongoDB in the 3rd quarter valued at about $36,000. GAMMA Investing LLC grew its stake in MongoDB by 178.8% during the 3rd quarter. GAMMA Investing LLC now owns 145 shares of the company’s stock worth $39,000 after buying an additional 93 shares during the last quarter. Finally, Continuum Advisory LLC increased its position in MongoDB by 621.1% during the third quarter. Continuum Advisory LLC now owns 137 shares of the company’s stock worth $40,000 after buying an additional 118 shares during the period. 89.29% of the stock is owned by hedge funds and other institutional investors.
MongoDB Price Performance
MDB stock traded down $7.26 during midday trading on Thursday, hitting $271.07. The stock had a trading volume of 2,240,897 shares, compared to its average volume of 1,653,422. MongoDB, Inc. has a 12-month low of $212.74 and a 12-month high of $509.62. The firm has a market cap of $20.19 billion, a PE ratio of -98.93 and a beta of 1.25. The company’s 50-day moving average price is $274.45 and its 200 day moving average price is $269.62.
MongoDB (NASDAQ:MDB – Get Free Report) last released its earnings results on Monday, December 9th. The company reported $1.16 EPS for the quarter, beating the consensus estimate of $0.68 by $0.48. MongoDB had a negative return on equity of 12.22% and a negative net margin of 10.46%. The firm had revenue of $529.40 million during the quarter, compared to analyst estimates of $497.39 million. During the same period in the prior year, the firm posted $0.96 earnings per share. The business’s quarterly revenue was up 22.3% compared to the same quarter last year. As a group, sell-side analysts anticipate that MongoDB, Inc. will post -1.79 EPS for the current year.
Insider Buying and Selling
In related news, CEO Dev Ittycheria sold 8,335 shares of MongoDB stock in a transaction on Tuesday, January 28th. The stock was sold at an average price of $279.99, for a total value of $2,333,716.65. Following the transaction, the chief executive officer now owns 217,294 shares in the company, valued at approximately $60,840,147.06. This trade represents a 3.69 % decrease in their ownership of the stock. The sale was disclosed in a legal filing with the Securities & Exchange Commission, which can be accessed through the SEC website. Also, insider Cedric Pech sold 287 shares of the company’s stock in a transaction dated Thursday, January 2nd. The stock was sold at an average price of $234.09, for a total value of $67,183.83. Following the sale, the insider now owns 24,390 shares of the company’s stock, valued at $5,709,455.10. This represents a 1.16 % decrease in their ownership of the stock. The disclosure for this sale can be found here. Over the last three months, insiders have sold 42,491 shares of company stock valued at $11,554,190. Insiders own 3.60% of the company’s stock.
Analysts Set New Price Targets
A number of equities analysts have recently issued reports on the stock. China Renaissance assumed coverage on shares of MongoDB in a research report on Tuesday, January 21st. They set a “buy” rating and a $351.00 target price on the stock. The Goldman Sachs Group raised their price objective on shares of MongoDB from $340.00 to $390.00 and gave the stock a “buy” rating in a research report on Tuesday, December 10th. Stifel Nicolaus upped their target price on shares of MongoDB from $325.00 to $360.00 and gave the company a “buy” rating in a report on Monday, December 9th. Rosenblatt Securities initiated coverage on MongoDB in a report on Tuesday, December 17th. They set a “buy” rating and a $350.00 price target on the stock. Finally, Mizuho upped their price objective on MongoDB from $275.00 to $320.00 and gave the company a “neutral” rating in a research note on Tuesday, December 10th. Two analysts have rated the stock with a sell rating, four have assigned a hold rating, twenty-three have issued a buy rating and two have assigned a strong buy rating to the company. According to MarketBeat.com, the stock currently has an average rating of “Moderate Buy” and a consensus price target of $361.00.
Read Our Latest Research Report on MongoDB
MongoDB Company Profile
MongoDB, Inc, together with its subsidiaries, provides general purpose database platform worldwide. The company provides MongoDB Atlas, a hosted multi-cloud database-as-a-service solution; MongoDB Enterprise Advanced, a commercial database server for enterprise customers to run in the cloud, on-premises, or in a hybrid environment; and Community Server, a free-to-download version of its database, which includes the functionality that developers need to get started with MongoDB.
Featured Articles
Before you consider MongoDB, you’ll want to hear this.
MarketBeat keeps track of Wall Street’s top-rated and best performing research analysts and the stocks they recommend to their clients on a daily basis. MarketBeat has identified the five stocks that top analysts are quietly whispering to their clients to buy now before the broader market catches on… and MongoDB wasn’t on the list.
While MongoDB currently has a “Moderate Buy” rating among analysts, top-rated analysts believe these five stocks are better buys.
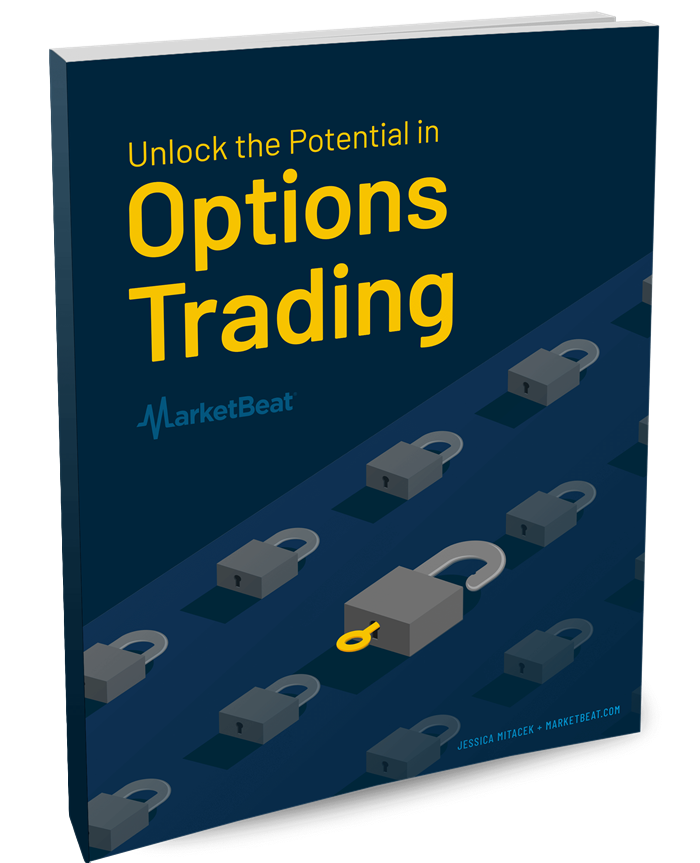
Options trading isn’t just for the Wall Street elite; it’s an accessible strategy for anyone armed with the proper knowledge. Think of options as a strategic toolkit, with each tool designed for a specific financial task. Get this report to learn how options trading can help you use the market’s volatility to your advantage.
Article originally posted on mongodb google news. Visit mongodb google news
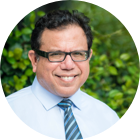
MMS • RSS
Posted on mongodb google news. Visit mongodb google news
Avior Wealth Management LLC trimmed its position in MongoDB, Inc. (NASDAQ:MDB – Free Report) by 90.2% during the fourth quarter, according to its most recent filing with the Securities and Exchange Commission. The institutional investor owned 134 shares of the company’s stock after selling 1,240 shares during the quarter. Avior Wealth Management LLC’s holdings in MongoDB were worth $31,000 at the end of the most recent reporting period.
Several other institutional investors have also modified their holdings of the company. Hilltop National Bank raised its stake in MongoDB by 47.2% during the 4th quarter. Hilltop National Bank now owns 131 shares of the company’s stock worth $30,000 after acquiring an additional 42 shares in the last quarter. Quarry LP lifted its holdings in shares of MongoDB by 2,580.0% in the 2nd quarter. Quarry LP now owns 134 shares of the company’s stock valued at $33,000 after purchasing an additional 129 shares during the last quarter. Brooklyn Investment Group acquired a new stake in shares of MongoDB in the 3rd quarter valued at about $36,000. GAMMA Investing LLC grew its stake in MongoDB by 178.8% during the 3rd quarter. GAMMA Investing LLC now owns 145 shares of the company’s stock worth $39,000 after buying an additional 93 shares during the last quarter. Finally, Continuum Advisory LLC increased its position in MongoDB by 621.1% during the third quarter. Continuum Advisory LLC now owns 137 shares of the company’s stock worth $40,000 after buying an additional 118 shares during the period. 89.29% of the stock is owned by hedge funds and other institutional investors.
MongoDB Price Performance
MDB stock traded down $7.26 during midday trading on Thursday, hitting $271.07. The stock had a trading volume of 2,240,897 shares, compared to its average volume of 1,653,422. MongoDB, Inc. has a 12-month low of $212.74 and a 12-month high of $509.62. The firm has a market cap of $20.19 billion, a PE ratio of -98.93 and a beta of 1.25. The company’s 50-day moving average price is $274.45 and its 200 day moving average price is $269.62.
MongoDB (NASDAQ:MDB – Get Free Report) last released its earnings results on Monday, December 9th. The company reported $1.16 EPS for the quarter, beating the consensus estimate of $0.68 by $0.48. MongoDB had a negative return on equity of 12.22% and a negative net margin of 10.46%. The firm had revenue of $529.40 million during the quarter, compared to analyst estimates of $497.39 million. During the same period in the prior year, the firm posted $0.96 earnings per share. The business’s quarterly revenue was up 22.3% compared to the same quarter last year. As a group, sell-side analysts anticipate that MongoDB, Inc. will post -1.79 EPS for the current year.
Insider Buying and Selling
In related news, CEO Dev Ittycheria sold 8,335 shares of MongoDB stock in a transaction on Tuesday, January 28th. The stock was sold at an average price of $279.99, for a total value of $2,333,716.65. Following the transaction, the chief executive officer now owns 217,294 shares in the company, valued at approximately $60,840,147.06. This trade represents a 3.69 % decrease in their ownership of the stock. The sale was disclosed in a legal filing with the Securities & Exchange Commission, which can be accessed through the SEC website. Also, insider Cedric Pech sold 287 shares of the company’s stock in a transaction dated Thursday, January 2nd. The stock was sold at an average price of $234.09, for a total value of $67,183.83. Following the sale, the insider now owns 24,390 shares of the company’s stock, valued at $5,709,455.10. This represents a 1.16 % decrease in their ownership of the stock. The disclosure for this sale can be found here. Over the last three months, insiders have sold 42,491 shares of company stock valued at $11,554,190. Insiders own 3.60% of the company’s stock.
Analysts Set New Price Targets
A number of equities analysts have recently issued reports on the stock. China Renaissance assumed coverage on shares of MongoDB in a research report on Tuesday, January 21st. They set a “buy” rating and a $351.00 target price on the stock. The Goldman Sachs Group raised their price objective on shares of MongoDB from $340.00 to $390.00 and gave the stock a “buy” rating in a research report on Tuesday, December 10th. Stifel Nicolaus upped their target price on shares of MongoDB from $325.00 to $360.00 and gave the company a “buy” rating in a report on Monday, December 9th. Rosenblatt Securities initiated coverage on MongoDB in a report on Tuesday, December 17th. They set a “buy” rating and a $350.00 price target on the stock. Finally, Mizuho upped their price objective on MongoDB from $275.00 to $320.00 and gave the company a “neutral” rating in a research note on Tuesday, December 10th. Two analysts have rated the stock with a sell rating, four have assigned a hold rating, twenty-three have issued a buy rating and two have assigned a strong buy rating to the company. According to MarketBeat.com, the stock currently has an average rating of “Moderate Buy” and a consensus price target of $361.00.
Read Our Latest Research Report on MongoDB
MongoDB Company Profile
MongoDB, Inc, together with its subsidiaries, provides general purpose database platform worldwide. The company provides MongoDB Atlas, a hosted multi-cloud database-as-a-service solution; MongoDB Enterprise Advanced, a commercial database server for enterprise customers to run in the cloud, on-premises, or in a hybrid environment; and Community Server, a free-to-download version of its database, which includes the functionality that developers need to get started with MongoDB.
Featured Articles
Before you consider MongoDB, you’ll want to hear this.
MarketBeat keeps track of Wall Street’s top-rated and best performing research analysts and the stocks they recommend to their clients on a daily basis. MarketBeat has identified the five stocks that top analysts are quietly whispering to their clients to buy now before the broader market catches on… and MongoDB wasn’t on the list.
While MongoDB currently has a “Moderate Buy” rating among analysts, top-rated analysts believe these five stocks are better buys.
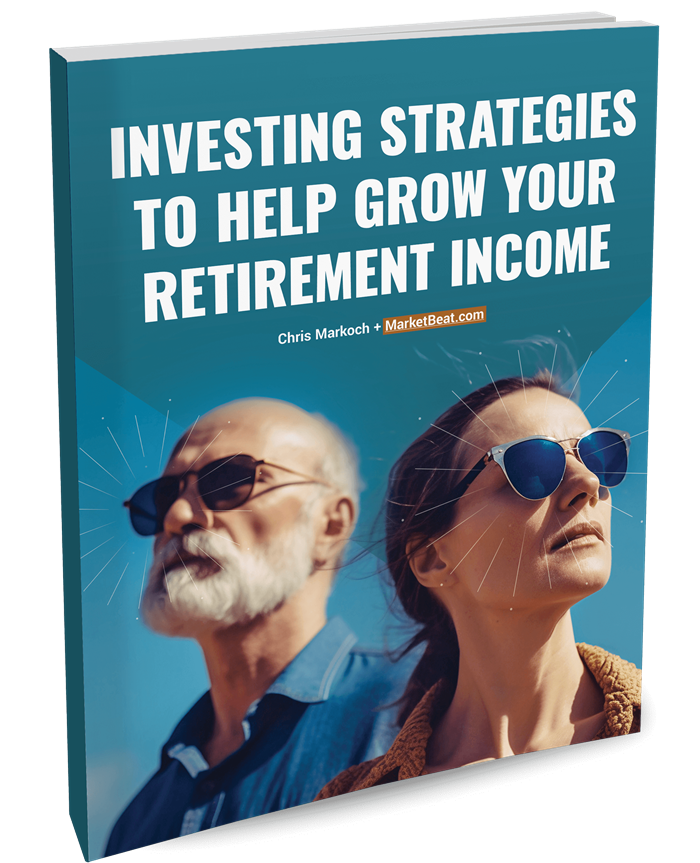
Need to stretch out your 401K or Roth IRA plan? Use these time-tested investing strategies to grow the monthly retirement income that your stock portfolio generates.
Article originally posted on mongodb google news. Visit mongodb google news
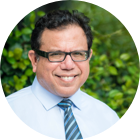
MMS • Sergio De Simone
Article originally posted on InfoQ. Visit InfoQ
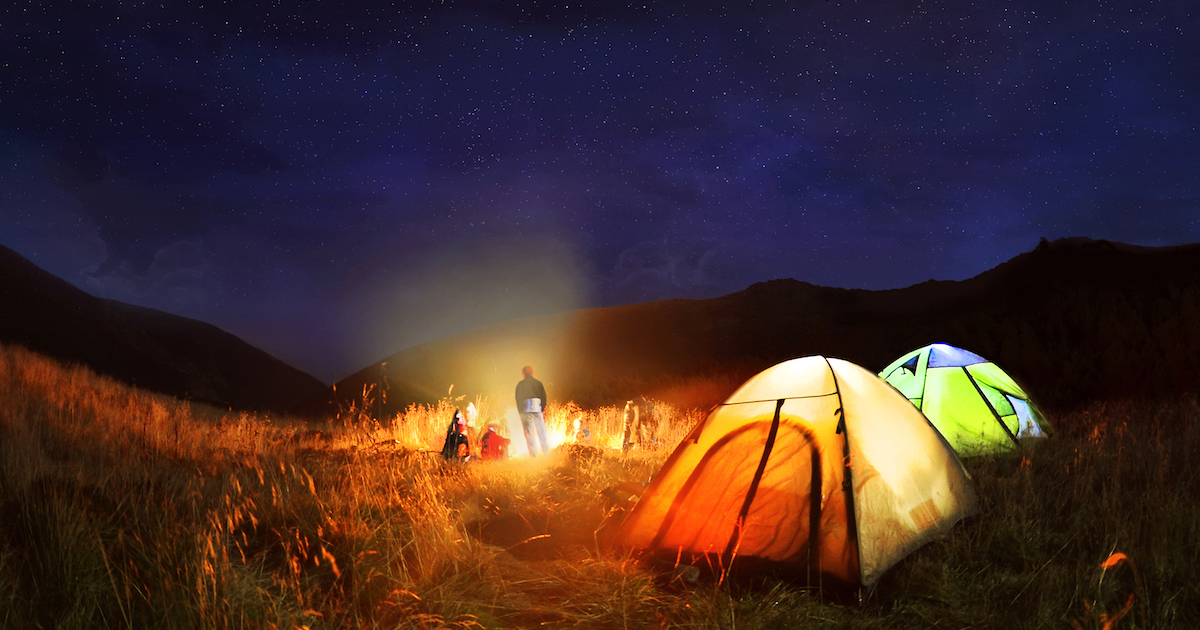
Apple’s new Advanced Commerce API provides iOS developers more flexibility to dynamically manage large content catalogs, creator experiences, and subscriptions with optional add-ons, such as premium features. For a developer to be allowed to use the new API, they must request access to Apple.
The Advanced Commerce API enables apps adopting it to host and manage their catalogs of In-App Purchases and SKUs outside of the App Store. However, they use the App Store commerce system to handle end-to-end payment processing, global distribution, tax support, and customer service. In other words, while the StoreKit In-App Purchase APIs require apps to configure all of their product identifiers in advance, the new Advanced Commerce API makes it possible to manage the catalog of products an app offers dynamically, at runtime.
Advanced Commerce API features are available through requests you make using StoreKit in your app and endpoint requests from your server. To authorize these requests, you generate JSON Web Tokens (JWTs).
To simplify generating JWTs to authorize calls and adopting the App Store Server API, Apple has also open-sourced the App Store Server Library. The library provides an API client to encode requests to and decode responses from the App Store Server and to verify that Apple signed the transaction data coming in responses. It is available in four languages, including Swift, Java, Python, and Node.
Examples of apps that could be eligible to use the new API are apps that provide a large catalog of one-time purchases, such as courses or games; apps that provide a platform for third-party creators to offer and sell their creations; and apps that want to sell add-ons to an existing subscription service, so users can buy a subscription and later add or remove any additional features they wish.
Last year, Apple changed the App Store rules to have all apps use the App Store for their In-App Purchases. This change affected apps like Patreon, Instagram, and others, for which Apple started applying their 30% App Store fee last November. The new Advanced Commerce API will not change this status of things but aims to provide more value to developers to justify that fee.
Build Resilient Systems with Insights on AI, Multi-Cloud, Leadership & Security at QCon London 2025
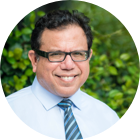
MMS • Artenisa Chatziou
Article originally posted on InfoQ. Visit InfoQ
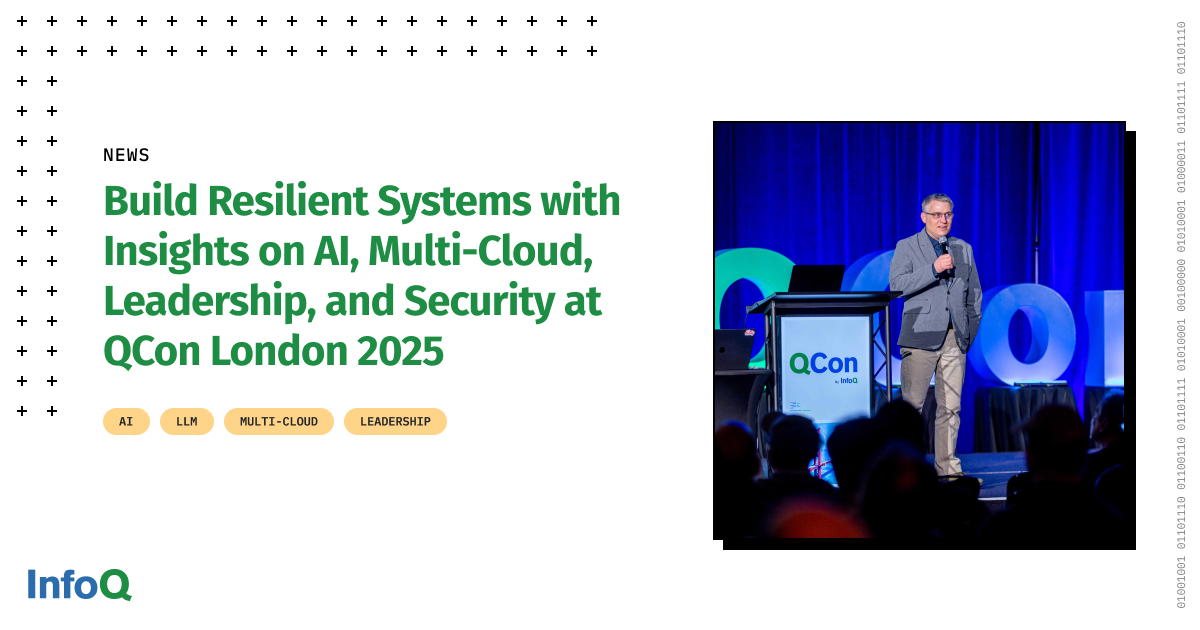
QCon London returns on April 7-11, 2025, bringing together 125+ senior software practitioners to share actionable insights across 15 practitioner-curated tracks focusing on emerging trends in software development.
Designed for senior software engineers, architects, and team leads, QCon will help you adopt the right technologies and practices. Discover how senior software practitioners are tackling modern challenges, from scaling architectures and enhancing developer productivity to securing supply chains and navigating AI integration.
Top Tracks at QCon London 2025: Architecture, Staff+, AI, and More
Architectures You’ve Always Wondered About
This track explores real-world examples of innovative companies pushing the limits with modern software systems. Speakers will share their stories of scaling their systems to handle large amounts of traffic, data, and complexity. QCon London 2025 Track Host: Ian Thomas, software engineer @Meta, with a background in Computer Science with a detour into UX and design, and QCon London 2024 PC Chair.
The Path to Senior Engineering Leadership
What does it take to reach senior leadership in tech? Gain insights into the journey toward senior engineering leadership, including strategies for managing teams, driving technical decisions, and fostering growth. QCon London 2025 Track Host: Leandro Guimarães, senior engineering manager @ClassPass, former chair of QCon in Brazil, working in software development for over 24 years.
AI and ML for Software Engineers: Foundational Insights
Deep dive into the foundational concepts of AI and ML and discover practical ways to integrate these technologies into software development. QCon London 2025 Track Host: Hien Luu, senior engineering manager @Zoox, leading the Machine Learning Platform team, & author of MLOps with Ray.
Engineering Productivity and Developer Experience
Learn how to enhance engineering productivity and create developer experiences that empower teams to deliver high-quality software efficiently. QCon London 2025 Track Host: Blanca Rojo, distinguished engineer and executive director at UBS, specializing in cloud engineering, and AI champion.
Modern Data Architectures
In this track, software leaders will share the actionable insights, cutting-edge strategies, and real-world case studies you need to navigate this evolving landscape. QCon London 2025 Track Host: Fabiane Nardon, data expert, Java Champion by Sun Microsystems & data platform director @totvs, Brazil’s largest tech company.
Connecting Systems: APIs, Protocols, Observability
Explore best practices for designing APIs, implementing protocols, and achieving observability in distributed systems. QCon London 2025 Track Host: Daniel Bryant, platform engineer, co-author of “Mastering API Architecture”, Java Champion, and InfoQ news manager.
Health Tech
Discover how technology is transforming healthcare with insights on optimizing patient outcomes, resilience, and innovations at the intersection of health and technology. QCon London 2025 Track Host: Dr. Jack Kreindler, founder and CEO @Wellfounded Health, chair of Leaders in Health, Extreme Environments Physiologist, & Medical Technology Entrepreneur, Researching the Limits of Human Resilience & Technology for Optimizing Health & Patient Outcomes.
Resilient Engineering Practices for Security against Modern Threats
Learn how to secure your software supply chain by embedding proactive strategies into your workflows to tackle modern vulnerabilities.QCon London 2025 Track Host: Sonya Moisset, staff security advocate @Snyk, a mentor for women in tech, a cybersecurity writer for FreeCodeCamp publications, 4x GitHub Stars, and an active member of the tech community in the UK.
Multi-Cloud and Hybrid Cloud Architectures
In this track, speakers will share real-world examples of multi-cloud adoption, focusing on integration, security, automation, orchestration, and analytics to optimize performance and cost-efficiency. QCon London 2025 Track Host: Dio Rettori, multi-cloud strategy and product @JPMorgan Chase & Co, ambassador for Cloud Native Computing Foundation, advisor for InfinyOn Inc., previously @Solo.io, @Red Hat, and @ Pivotal Software.
Emerging Trends in the Frontend and Mobile
This track will explore cutting-edge trends and best practices for building high-performance, responsive, and engaging frontend and mobile applications that meet the evolving needs of modern users. QCon London 2025 Track Host: Sareh Heidari, senior software engineer at F1 Arcade®, the intersection of Formula 1® racing simulations in a hospitality setting.
Presented by InfoQ, QCon London 2025 shares talks you can trust, free from vendor bias and marketing agendas. Attendees gain actionable insights from real-world experiences shared by practitioners adopting emerging trends and practices.
For more details, visit QCon London 2025. Early bird pricing ends on February 11, 2025.
Presentation: Reducing Developer Overload: Offloading Auth, Policy, and Resilience to the Platform
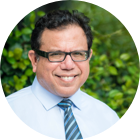
MMS • Christian Posta
Article originally posted on InfoQ. Visit InfoQ
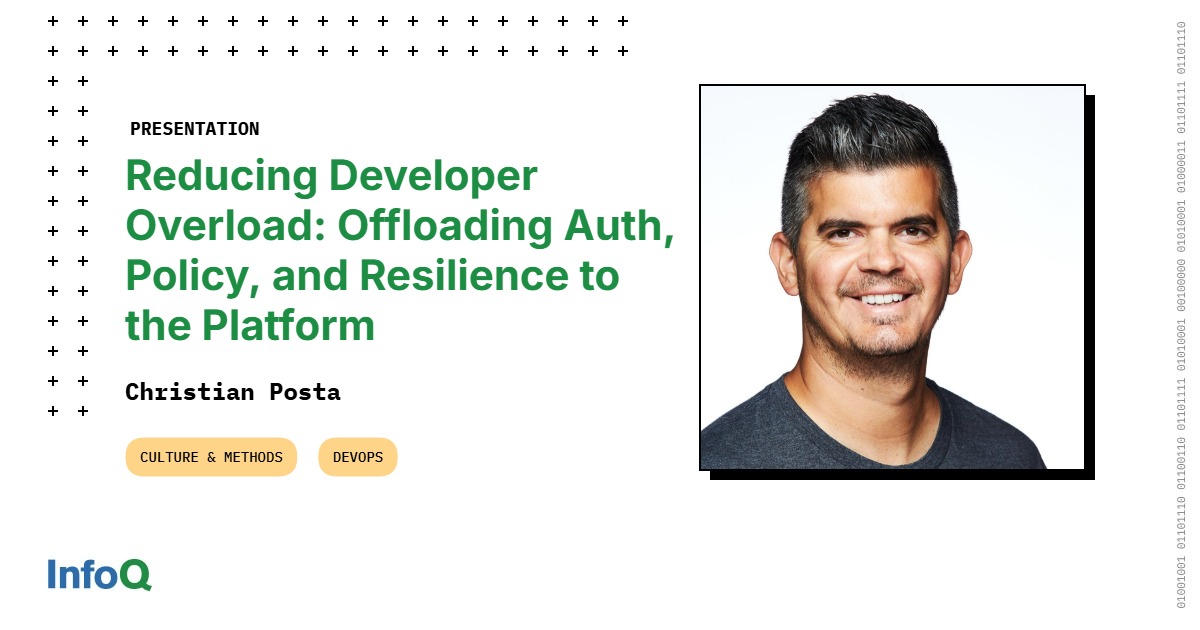
Transcript
Posta: I’m going to be talking about moving off policy and resilience to your internal developer platforms. How many people are working on or use an internal developer platform at the company at which they’re at? I work for a company called solo.io. Solo is based here in Boston, actually, in Cambridge. At Solo, we work on open-source cloud networking technologies. We are very involved in the Istio project, the founders and the lead contributors. We lead the innovation and a lot of the stuff that’s happening in the Istio project, as well as some of the surrounding periphery projects, some of which we’ll be talking about.
Digital Experiences – Driven by APIs and Services
As you know, digital experiences are how we do things today, when we get around. I flew here to Boston. I got on my phone. I booked to travel. How many of you remember what it was like before that? You had to pick up a phone and make a call to a travel agent to book your flights, to book your hotel. You had to call some random number to book a taxi, and hopefully that taxi showed up. Digital experience has made our lives a lot easier today. We save a lot more time, and that’s extremely valuable. APIs and services underpin the apps on our phones and the digital experiences that we use. These organizations, these businesses, they see APIs and services and the infrastructure to get those live and into production as driving a lot of business value, being differentiating.
The developers and the time that they put into building out these new services, new capabilities, are extremely valuable. The challenge with that is getting code and getting these changes into production. I work with organizations that experience those all the time. A big part of that is the siloed nature of their organizations. They build their code. They stage. They get it ready. You got to now make changes to your API management system, to your load balancers, to your firewalls. The way these organizations have been structured aren’t conducive to being able to do this very quickly. They’ve made decisions in their own silos. The integrations between those silos are very complex and brutal and expensive. If you want to get anything done, what do you do? You open tickets, and you sit and you wait.
Hopefully, these teams will go off and go to these UIs, and point and click, and these manual steps to get things done, make the changes, and then eventually you can get things into production. That’s why you’re probably seeing and we see this. We work, like I said, very closely with these organizations that are going down this path of building platform engineering teams. Building internal developer platforms to try to bridge and work across these silos, to try to build internal APIs, to support automation so that they can build the tools, the workflows, the UIs, the experiences for their developers to be able to self-service, and build, deploy their code into production as quickly and safely as possible. They build these paved paths or these golden paths.
The platform engineering team and the platform itself is intended to be a business value accelerator. We want to improve the ability to get these APIs and these services out into production faster. We want to improve efficiencies. We want to maintain or improve compliance, do things like reduce cost, and lock-in, and so on. This is a trend that we’re seeing.
Internal Developer Platforms
How many people have built their own house? It’s not easy. It’s not something I would want to do. I’ve lived through a remodel, and don’t want to do that again. When you build a house, you don’t start with laying electrical wire or putting doors. You start with the foundation. You start building walls and a roof and so on. I think about internal developer portals like a house. It’s a foundation, a platform on which you can get value out of it. Build value. Do things. Work from home. Raise a family. Raise your pets. Sleep comfortably, and then be productive the next day. Internal developer platforms lay that foundation so developers can actually get their work done and be efficient.
The organizations that we work with are trying to improve their cross-team and silo communications. They’re adopting new technologies like containers and cloud, and building automations, CI/CD, putting in observability tools, and so on. There is something missing, and it is fairly glaring when we start working with them. Anybody care to take a guess what’s missing from this list right here? Security is very important. Absolutely. These services need to communicate with each other.
Gregor Hohpe just released a book on platform engineering, platform strategy. He identifies the needs for an internal developer platform, and he calls it the four-leaf clover of engineering productivity, and delivery pipeline, monitoring operations, the runtime, compute, all that stuff. Obviously, very important. You need to solve the communication problem. You need to solve the networking problem. When we work with these organizations, we see this as, they built the foundations, they put the containers and lambda and whatever else they’re going to use, CI/CD, and they still have challenges getting code out into production.
T-Mobile did an internal review of, why is it taking weeks to get changes out into production, even though they’ve adopted these modern platforms? You can go and take a look at this talk where Joe Searcy goes into the details of that research and how they built their platforms to solve some of this. They found that 25% of developers’ time was spent on these nonfunctional requirements, like routing, like security, some reliability stuff. That they would open these tickets, try to get changes into production, and sit and wait. They would find that for production issues and outages and stuff that they had, 75%, three-quarters of those were caused by network misconfigurations.
Modernizing Networking
If you’ve not addressed the networking and communication parts of your platform, you’re still relying on some of the existing ways that don’t quite fit the way you’re building your cloud platforms, then your house is not finished. It’s probably not a good idea to live in it. Some of the outdated assumptions that we run into, especially in the networking and API management space, is those around how you specify policy. Oftentimes, this is implemented as firewalls and firewall rules and so on in these organizations. Things like, we’re going to write our policy in terms of network location or network identity, things like IP addresses. If something in the network changes, those policies become invalidated.
In this very simple case, we’re saying, this IP address can talk to this IP address. What we really mean is service A can talk to service B. If we start adding more workloads to the node that has service B, now we have this drift in policy. Service A can now talk to service C also because it’s deployed on this IP address, but that’s not really what we intended. This is a very simplistic example, and this can get very complicated, but as the network changes, the workloads change and shift and move, you get this policy bit rot, just like you do with code. Another one that frequently pops up in the cloud space is, these IP addresses are ephemeral. A host or a VM can go down and come back up and potentially come up with a new IP address. Or, in Kubernetes, pods will recycle and have new IP addresses. Policies written in terms of those IP addresses are going to be invalidated as IPs get reassigned.
Another one that we see is, these organizations, they’ve implemented these API management systems to solve things for rate limiting and security and observability, and so on, originally intended for external APIs, now they’re using them for internal APIs. Now to go make changes and get changes into production, you have to open tickets. It’s not uncommon that we see organizations saying it takes couple weeks to make changes to their load balancers, to their API management systems, and so on. From a workflow standpoint, this causes bottlenecks. From a technology standpoint, these systems, the tenancy model we see where certain APIs misbehaving will impact other APIs and so on.
From a technology standpoint, there’s bottlenecks and issues there as well. They can force unnatural traffic patterns. We’ve seen this many times, where workloads in a single cluster want to communicate with each other, one API calls another. To do that, they have to be forced out of the cluster, out through load balancers, out into some centralized API gateway, then through the gateway, back down through load balancers, eventually back into the cluster. These workloads might have been deployed on the same host, but for them to get the policies and authentication, authorization, observability, they have to go through these unnatural patterns.
One thing that I pointed out in T-Mobile, is that, developers sometimes they’re like, “I don’t want to open tickets. I don’t want to deal with all this infrastructure stuff. I’ll just write it in my code. I’ll just write the security stuff, the load balancing. I’ll just write the service discovery stuff. I just put it into my code”. That gets expensive, doing across different languages, different frameworks, making the business logic all convoluted, because now you have this networking code in there. From a security standpoint, it’s not that easy to get right. Using tokens, using keys, usernames, passwords, putting that all over the environment, it’s very easy to have a mistake creep in and have a security vulnerability. I blogged about this in much more detail, especially around using JWT tokens as service identity. Using JWT tokens, all that stuff, for user identity, you log in, OAuth, whatever, that’s all good.
For service-to-service communication and workload identity, there’s a lot of things that can go wrong there. What we need for modern networking is something that’s not highly centralized. We don’t need a distributed implementation. We need to tie it into our existing infrastructure as code, GitOps style workflows and automation. We want standard interfaces to be able to integrate with other pieces. There’s not going to be one technology that does everything. We need standard interfaces. We need to do the networking stuff that we’ve been doing already, traffic routing, load balancing, authentication, authorization, rate limiting, observability. We still need those capabilities. If you’ve built your platform and you don’t have those capabilities, then your house is unfinished.
Finishing the House (Istio)
Like I said, I remodeled my house probably about 5 years ago now. It was a bit of a pain. Eventually, we did reinstall new pipes, new electrical, ACs, especially in Phoenix right now. To live comfortably in your house, you need those pieces. The analogy there is, the locks on the doors are like the authentication/authorization for specific requests, for every request between services. You need load balancing, retries, timeouts, circuit breaking, zone-aware load balancing, things like telemetry collection, distributed tracing, logging. Then, like I said, integrating with other parts of the systems. Maybe you have a policy engine like OPA, or Kyverno, or something, or your own homegrown one.
Maybe you have your own existing API gateway, and you need to integrate with that as well. We need nice, standard interfaces, just like when you’re building a house, to assemble these components. It needs to work for all the applications in your environment, regardless of what language, regardless of what framework they’re using. We don’t want the developers to go off and re-implement this themselves. That’s where things like a service mesh come into the picture. Something like Istio, I’ve been working on it for about seven-and-a-half years now, comes into the picture to solve this problem, transparently enabling things like mTLS, mutual authentication, telemetry collection, distributed tracing, and traffic control.
Anybody using Istio? Istio has been out for a bit, and has traditionally been implemented by deploying proxies next to the instances of your workload. If it’s a Java app, next to your JVM. If it’s a Python app, the actual process that runs the Python code. If it’s in Kubernetes, actually inside the pod. Deploying, getting this infrastructure-y stuff into your applications creates this coupling and friction.
First of all, how do you onboard? How do you get applications on? Now you have to inject this thing into it. If you already have other sidecars, do those play nicely together? How do you do upgrades? You got to restart all your apps because you got a new sidecar. Then there’s the performance, there’s the overhead. Sidecars were a necessary evil to implement this type of functionality, but this is the last thing I’m going to talk about sidecars. We’re going to talk more about the functionality of the service mesh, and we’ll dig into a little bit how we implement this functionality without using sidecars. In September 22, I think we announced publicly in the Istio community an implementation of Istio that doesn’t use sidecars. In May, actually, we finally got it to the point where it is now in a state where it’s usable in production. We have people using it in production already.
Demo
Let me just show you a quick demo what that looks like. In my demo app, we have three apps in three different namespaces. We have web API. We have recommendation. We have purchase history. If I go into web API, you’ll see we have an app running there. This signifies the web API team, different teams across the Kubernetes cluster. We don’t have Istio. We don’t have any sidecars or anything installed. Recommendation looks the same. Purchase history actually has two different versions, which we’ll use later in the demo to illustrate routing. In the default namespace, there’s a sleep app that we’ll use as a client, at least in this next step. In httpbin, another sample application. Web API calls recommendation.
Recommendation calls purchase history. If we call web API through the sleep app, we’ll see that, indeed, web API calls recommendation and recommendation calls purchase history here. We’re going to come over here and we’re going to actually start our demo. What we’re going to do is we’re going to install Istio. This will take a second, but you’ll notice in the command here, I’m going to use the new ambient profile. This is going to install Istio’s ingress gateway, which is just a default. It’s an Envoy proxy for getting traffic into the mesh or into the cluster. It’s going to install the Istio control plane, Istiod. Then it’s going to install a couple components that will enable us to do the sidecar-less implementation of Istio. I’ll talk a little bit more about what those components are.
As part of the installation, I want to install Grafana. I want to install some observability apps so that we can see some of the tracing. We’ll give it a second. We’ll take a look. You can see here in the namespace list, Istio system now appears. I click into there. Let’s see, things are still coming up. Grafana’s up. Istiod, right here, the control plane, the ingress gateway, and a few other components, ztunnel. We’ll wait for Kiali to come up. We’ll use Kiali here in a second. The first step we’re going to do is we want traffic into the mesh. We want it to come in through the Istio ingress gateway. We’re going to apply some routing policies to allow traffic in through the ingress gateway. The ingress gateway is exposed on this external IP address.
Once we apply this policy, we’ll end up using that IP address to make the call. The routing policy is very simple, match on a particular host, istioinaction.io. Then, route it to web API. Like I said, traffic comes into web API. Web API calls recommendation, which calls purchase history. We’ll do that. If we actually make a call through that IP address back here, you can see that we’re getting the correct response, and it goes through the Istio ingress gateway. What we’re going to do is we’re going to add our web API, recommendation, and purchase history, to the mesh, and we’re going to do that by labeling each respective namespace. In this case, we’ll also do the default namespace. There are some sample apps there. It will label the recommendation, and then the last one here, purchase history. That’s it. Our apps are now part of the service mesh. There is no sidecar running here, as you can see. This is Istio Ambient mode.
If we come over here to the Kiali console, yes, let’s go ahead and port forward that. Let’s do some port forwarding and get the Kiali console to come up. We don’t have much traffic going through here, so let’s get some traffic. We’ll send about, I think it’s 250 requests through the gateway, and that’s good. We should take a look at our workloads that we have deployed here, web API, recommendation, and purchase history. We see that Kiali recognizes the workloads here. If we look at the Istio config, we can see the Istio config that’s been deployed just not much going on, really, just allowing traffic into the ingress gateway. Then, lastly, if we click on the traffic graph? We still don’t see the traffic. Give it one more run, generate the metrics.
The metrics end up going into Prometheus. Kiali scrapes Prometheus, and should show the traffic flow. There we go. We can see web API calls recommendation, which calls purchase history. What we also see is through the lines between the different services, we see this lock. If I click on these locks and look off to the right-hand side here, we see that Istio is enabled. We have mTLS enabled between these services. The services are using SPIFFE workload identity, which we’ll talk about. We’ve done nothing more than just label the namespaces. We’ve already got the services into the mesh. They’re protected by mTLS, so their traffic’s encrypted. We have workload identity assigned here. That’s pretty good for just labeling a namespace.
Istio Ambient Mode (High-Level Overview)
The way Istio Ambient works, just at a high level, is it deploys an agent to each of the nodes in the cluster. This agent binds directly into the pod’s network namespace. What that means is traffic will leave the pod, but Istio Ambient will have already done some stuff to that traffic, so the ztunnel will have done that. In this case, what it’s doing is matching an mTLS certificate to that pod, and enabling mutual TLS. Once the traffic leaves the pod, it is already encrypted. It is already participating in mTLS. It’s being tunneled to whatever the destination is. Obviously that other side will participate in the mTLS as well. I’m certain I’m going to get this question, so I’ll draw a picture about exactly what the ztunnel is doing. Traffic is not going from the pod to the ztunnel. It is leaving the pod already having been encrypted by the ztunnel.
The ports are opened up inside of the network namespace of the pod, so we get the same behavior that we do with sidecars, sidecars actually deployed into the pod, but without deploying sidecars. That’s great for layer 4 connections mTLS, but what about layer 7, things like request-based routing, or retrying of a request that failed, or JWT validation and authentication and authorization. For that, what Istio Ambient does is it injects a layer 7 proxy that we call the waypoint proxy, into the traffic path.
Since we already control it with the ztunnel, if there are layer 7 policies, we can route it to a layer 7 proxy. That layer 7 proxy, we don’t want to treat that as some big, centralized gateway. What we want to do is have better tenancy for it. In Kubernetes where the default is to deploy a waypoint proxy per namespace, so for each namespace, you have your own layer 7 proxy. If you need more fine-grained tenancy, what you can do is deploy a waypoint proxy per service identity or service account in Kubernetes, for example.
You can control the tenancy of these layer 7 proxies. You don’t get these big centralized API gateways, but you do have API gateway functionality. In these proxies that now live in the network somewhere, they can be scaled. If you want high availability, you scale multiple of these waypoint proxies. You can size the proxies more appropriately to the traffic that is destined, in this case, for pod B. The sidecar approach, you just scale up more pod B’s, but then you get more sidecars, more proxies.
We’ve gone into a lot of performance analysis, resource cost analysis, comparing it to sidecar, comparing it to other service meshes. This was a fairly large deployment. I think we were doing in the order of 112,000 requests per second through this environment and setup. We took measurements of the baseline, what ambient looks like, what other service meshes look like, what the sidecar looks like, for comparison. Because of this optimization, where if you just need mTLS, you don’t have to inject sidecars, you don’t have to do anything, this network path becomes extremely fast. It’s just layer 4. If you need to use layer 7 capabilities and inject the waypoint, this also ends up being faster than the sidecar, because we’re processing HTTP only one time.
Sidecar does it twice, once on each side. The network hop that you take to get to the waypoint, the cost of that, we’ve seen in our performance testing is lower than having the sidecars perform the HTTP parsing, all the stuff that they need to do. Ambient ends up being simpler to onboard, simpler to upgrade and maintain, especially for security patches and all kinds of stuff that you have to do. It’s a fraction of the resources that need to be reserved for CPU and memory to run sidecars, because you don’t run sidecars. Performance is improved, especially in the layer 4 only cases. Actually, security gets a slight improvement as well. I’ll leave you with this link here – https://bit.ly/ambient-book . Lin Sun and I wrote a book, “Istio Ambient Explained”, and it goes into a little bit more detail about Istio Ambient. Go ahead and take a look at the istio.io website. Istio Ambient, like I said, just became available for production usage. It will eventually become the default data plane. Not right now, sidecars won’t go away. That’s the path that we’re on with Istio.
Auth, Policy, and Resilience
Let’s talk in more detail about auth, policy, and resilience, and how moving that to the platform makes a lot more sense, drives down costs, and so on. We’ll look at a few examples. One is Trust Bank. I’m going to use public references, so you can go and if you’re interested look them up. Trust Bank was this new digital bank that was starting up as a joint venture between a bunch of other big banks in Singapore, and they went from nothing to a million users in a very short amount of time. They were cloud first. They built on EKS and AWS, but for their networking components, they used Istio. The problems that they were trying to solve were around compliance, security, encrypted traffic. Things like, they started off with a handful of clusters. They wanted to add more clusters and deploy workloads into more clusters, but they didn’t want downtime for their apps. They wanted to be able to move apps to different clusters, different regions. They started to encounter regulatory concern around data gravity and that kind of stuff as well. They needed to be able to control the routing.
From an authentication, authorization standpoint, they didn’t want to force everything through these centralized systems. They needed a decentralized networking approach. That’s where Istio came into the picture. We talked a little bit about what the existing assumptions and existing approach to defining policy looks like with IP addresses and firewalls and so on. What we want to solve is the service-to-service communication and authentication problem. How does service B know that it actually is service A that’s calling it? That’s where a specification called SPIFFE comes into the picture. SPIFFE is a spec for workload identity and how to get those credentials that prove you are a certain workload. It specifies what they call a verifiable identity document, SPIFFE, verifiable identity document, that is usually in the form of an X.509 certificate. It doesn’t have to be, it can be other formats, but X.509 is a common one.
Then workflows for how does a workload get that document? How does service A prove that it is service A? The way that it works is, service A will request its verifiable identity document, an X.509 cert that says I’m service A. The SPIFFE workflow and the implementations behind the scenes will then go and say, I need proof that you’re service A. I’m going to go do a background check, basically. I’m going to go attest that you are indeed service A. I’m going to go check the machine that you’re running on. I’m going to check the context that you’re running in. I’m going to check the attributes assigned to you. If that all lines up and you really are service A, then I’ll give you this document. That X.509 document is presented by service A to service B, saying, I am service A.
Service B can look at it and check the authenticity of that document. A common way to do that is TLS and mTLS. This is where Istio comes into the picture. Istio can automate the mTLS connection transparently for your applications. Istio does implement SPIFFE, so they work very nicely together. Now if workload A is workload A, is cryptographically provable, and it’s talking with workload B, and we know these identities, these identities are durable, we can write policy about what services are allowed to talk with which other services. In regulated environments, this type of policy is extremely important. Istio allows you to write this type of policy in terms of workload identity in a declarative format that can be automated, fit into your GitOps workflows and pipelines, and so on.
Demo
I’m going to jump into a quick demo that shows what that looks like. Again, we have web API calls recommendation, which calls purchase history. The first thing that we’re going to do is we’re going to lock down all communication in the mesh. We’re going to deny all. In a realistic world, you can’t just come in and shut off all traffic. You can iterate and incrementally add these authorization policies and eventually get to a zero-trust environment where you deny all. For this demo, we’ll start with just lock down all traffic. The only thing we will allow is people can call the ingress gateway. We’ll apply this authorization policy, and we’re going to try to make a call to the gateway.
By the error message, you can’t really tell, but it makes it to the gateway, but that gateway says, I can’t route this to web API. Traffic can’t proceed in the mesh right now, everything is denied. What we want to do is adjust the policy. We want to allow the ingress gateway to call web API. Using an Istio authorization policy, what we’re going to do is do that based on identity, not what cluster this thing’s running on, or what IP address this thing’s running on, or what pod it is. We’re going to do it based on workload identity, the SPIFFE workload identity that I just described. We’re going to allow that traffic for web API. If we allow it and now make a call, we should see traffic go from ingress gateway to web API.
Not surprisingly, the rest of the traffic is still disallowed. Let’s go ahead and add that. We’ll add the policies to allow traffic between the rest of the chain of the services. Now, if I make that call through the gateway, everything proceeds. The calls work again. From the sleep app, which is in the default namespace, I shouldn’t be able to call web API. Ingress gateway can. Other apps cannot. Let’s take a look. I try to call it. That does not work, which is what we expect.
So far, this has been mutual authentication and policy enforcement based on identity, but we can be even more fine-grained than that. We can specify policies about what services can call what other services, and specific endpoints, specific HTTP verbs that they can call, specific headers that have to be present, or not present. We see a little bit of that in this next part. I want to allow the sleep service to call httpbin, but only at /headers, and only if this x-test-me header is present. Now we’re looking at layer 7. We’re looking at the request itself, the details of the request, and we’re going to build some authorization policies in terms of some of those details. In Istio Ambient, if you’re going to do anything with layer 7, you need that waypoint proxy that I mentioned.
This is that layer 7 proxy that gets injected into the network path. Those get deployed in Istio Ambient by default, one per namespace. I just created this waypoint proxy, if I come into the default namespace, we can see sleep, which calls httpbin. Now we’ve included this new waypoint proxy, and we’re going to apply that policy to allow sleep to call httpbin. It won’t work if we call /IP, that was not whitelisted, that was not part of our authorizations. If we call it with the right header on the right path, in this case, the call will go through. We get very fine-grain layer 7 authorization policies declaratively with Istio.
Traffic Control and Traffic Routing
The last section here goes into a little bit more of the traffic control and traffic routing. Intuit has given a number of presentations on how they’ve built their platform using Istio multi-cluster. One of their big use cases is, when they deploy a service, and they make an upgrade, they deploy the new version of that service into a different namespace. They might move it into a different cluster. What they need is globally-aware routing. When we deploy a new service, we don’t want to take an outage. Another company gave a similar talk, but their motivation, their reasons for needing that global routing and failover, were for data gravity and compliance reasons.
GDPR, you have to have your data in a certain region, and if you want to access it, you have to go through and to that that region. Istio is really good at controlling traffic, both load balancing, being zonal aware, being multi-cluster aware, and routing across multiple clusters. When we have traffic control down to the level of a request, we can also implement things like resilience. We can be resilient in terms of load balancing. We can be smart and cost optimized in terms of load balancing. We can also do things like timeouts, retries, and circuit breaking, and offload that from the app developers having to worry about that.
Like I mentioned, globally-aware routing. If a service is talking to a service in one cluster, but the destination service fails, we can fail over to a different cluster transparently, and still have mTLS, still have our authorization policies enforced, and do it in a smart way. We’re not going to automatically fail out to a different region. We would try to prefer locality. We’ll try to prefer zonal affinity. Then fail out to a different region as necessary. I mentioned circuit breaking. If I’m making a call to a service and it’s misbehaving, stop calling it, or at least for a period of time, back off. Then, slowly, try to call back into it.
Demo
This is the last part of the demo. Purchase history has two deployments, a v1 and a v2. If I call that, we see, in this instance, we had a response from purchase history v2. I call it again, also v2 it looks like. We should see it load balance. There we go. There’s a v1, v1, v2, so it’ll load balance about 50% is what Kubernetes does automatically, out of the box. What we want to do is force all the traffic to v1. That’ll be the production available version. We’ll write a default routing rule here that says, 100% of the traffic should go to v1, 0% should go to v2. However, we might want to introduce v2 as a canary. We want to be very specific and fine-grained about what services can call the v2 version.
To do that, we’ll add a match that looks for a specific header, and if it has this header, then we’ll route it to v2. Again, we’re doing Istio layer 7 stuff, so we’re going to add a waypoint proxy into the purchase history namespace. We can see that down here at the bottom. Let’s go ahead and apply that routing rule. Now let’s start making calls. Actually, we have deny all authorization policy. We have to enable that for the waypoint. Now what we’re going to do is call the services 15 times. We’re going to use jQuery to pull out the bodies, we see 15 times in a row. The call ends up going to purchase history v1. If I want to do the canary part, you can’t see it, but at the bottom here, we called the services with this header, which triggers that match in the routing, and routes it to purchase history v2. What if purchase history is misbehaving? What if it’s returning errors? I just deployed a version of purchase history that half the time is going to return 500 errors.
As we can see here, we call it a handful of times. With Istio, we can do timeouts, retries, circuit breaking type thing. Let’s take a look at a retry policy that we will want to add here. If you’re calling purchase history v1, down here in the bottom, you can see, I want to implement retries. I’ll retry up to three times on 500 type errors. If I apply this and then make calls, we should see, every time the calls succeed. They might be failing. We can check the retry metrics. They might be failing in the background, but the retries are kicking in and making it so that the call succeeds eventually.
Conclusion
Istio in general provides a lot of capabilities for fine-grained modern networking. Solving this networking challenge should be part of your modern internal developer platform. You can see that here in the stack. This is how it lines up in block diagrams. One thing I will say, and you’ll notice here, Istio is buried down here in the bottom of the stack. This is networking. Application developers shouldn’t have to know about Istio. The APIs, the workflows, the interfaces, the experiences that you build for your developer platform should enable things like maybe doing a canary release, or publishing telemetry and metrics to Grafana dashboards and so on, so that they can see what’s happening with their services.
Things like security policy are probably not driven by developers, but if your workflow includes that, then your workflow can generate those authorization policies and the details around what services can call which other services or API endpoints. All of that stuff should be automated away. They shouldn’t have to know about this. From a platform like, what are the business outcomes of the platform?
Originally, I mentioned, you want to increase business value. You want to increase compliance. You want to make things more efficient, and reduce cost. From a value standpoint, your code on the developer’s laptop, or in CI does nothing unless it gets into production. You can’t get value out of that. Tools like Istio implementing something like this allows you to do more safe releases, canaries, blue/green deployments. A lot of network telemetry can be pulled back, distributed tracing, and so on. You can make those decisions about whether to go forward and so on.
See more presentations with transcripts
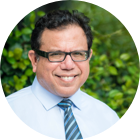
MMS • RSS
Posted on mongodb google news. Visit mongodb google news
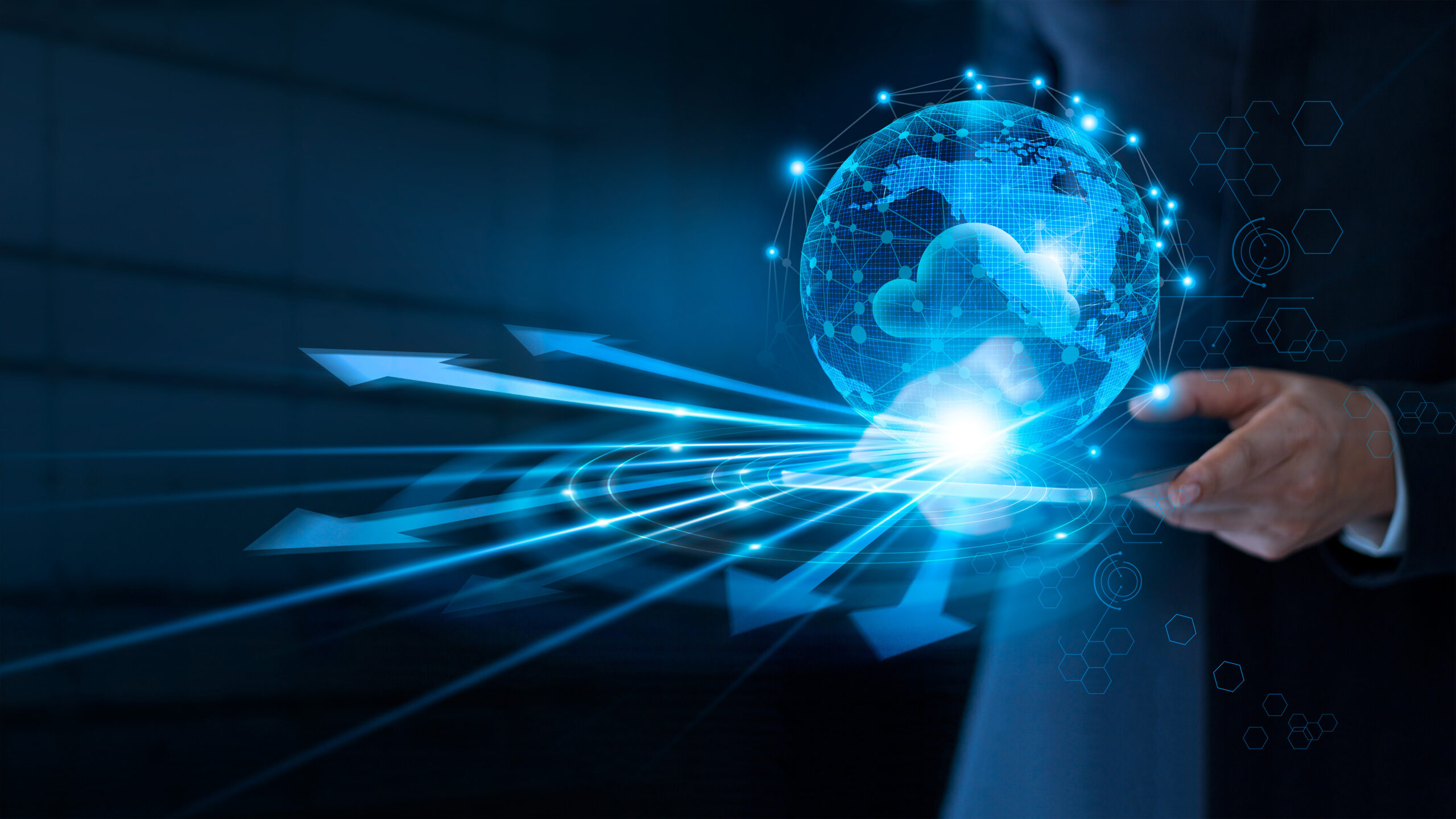
After tech investors licked their wounds yesterday on the launch of the Chinese artificial intelligence (AI) chatbot DeepSeek, today they saw an opportunity from the upheaval.
Software stocks broadly rallied as the stock market bet that costs to run AI infrastructure could come down and efficiencies could improve, benefiting the software companies that rely on that infrastructure and are launching their own AI platforms and looking to leverage the power of agentic AI.
That makes sense, as the AI infrastructure is being built to ultimately run software applications. Among the winners today were MongoDB (NASDAQ: MDB), Salesforce (NYSE: CRM), and GitLab (NASDAQ: GTLB). As of 11 a.m. ET, the stocks were up 8%, 5.4%, and 10.3%, respectively, on the news.
Source Fool.com
Article originally posted on mongodb google news. Visit mongodb google news
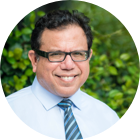
MMS • RSS
Posted on mongodb google news. Visit mongodb google news
MongoDB, Inc. (NASDAQ:MDB – Get Free Report) was up 7.4% during mid-day trading on Tuesday . The stock traded as high as $285.10 and last traded at $284.28. Approximately 819,510 shares changed hands during mid-day trading, a decline of 43% from the average daily volume of 1,427,558 shares. The stock had previously closed at $264.58.
Analyst Upgrades and Downgrades
Several research analysts have weighed in on MDB shares. Wells Fargo & Company upped their price objective on shares of MongoDB from $350.00 to $425.00 and gave the stock an “overweight” rating in a report on Tuesday, December 10th. JMP Securities reaffirmed a “market outperform” rating and issued a $380.00 price target on shares of MongoDB in a research report on Wednesday, December 11th. Robert W. Baird boosted their price objective on MongoDB from $380.00 to $390.00 and gave the stock an “outperform” rating in a report on Tuesday, December 10th. Scotiabank dropped their target price on MongoDB from $350.00 to $275.00 and set a “sector perform” rating on the stock in a report on Tuesday, January 21st. Finally, Cantor Fitzgerald assumed coverage on MongoDB in a report on Friday, January 17th. They set an “overweight” rating and a $344.00 price target for the company. Two equities research analysts have rated the stock with a sell rating, four have issued a hold rating, twenty-three have issued a buy rating and two have issued a strong buy rating to the company’s stock. Based on data from MarketBeat.com, the company currently has an average rating of “Moderate Buy” and a consensus price target of $361.00.
Get Our Latest Analysis on MDB
MongoDB Price Performance
The stock has a market capitalization of $21.11 billion, a PE ratio of -103.48 and a beta of 1.25. The firm’s 50 day simple moving average is $275.02 and its 200 day simple moving average is $269.23.
MongoDB (NASDAQ:MDB – Get Free Report) last posted its quarterly earnings results on Monday, December 9th. The company reported $1.16 earnings per share for the quarter, topping the consensus estimate of $0.68 by $0.48. MongoDB had a negative net margin of 10.46% and a negative return on equity of 12.22%. The business had revenue of $529.40 million during the quarter, compared to the consensus estimate of $497.39 million. During the same period in the previous year, the firm posted $0.96 earnings per share. The firm’s revenue for the quarter was up 22.3% on a year-over-year basis. Analysts expect that MongoDB, Inc. will post -1.79 earnings per share for the current fiscal year.
Insider Buying and Selling at MongoDB
In related news, CEO Dev Ittycheria sold 2,581 shares of the business’s stock in a transaction dated Thursday, January 2nd. The shares were sold at an average price of $234.09, for a total transaction of $604,186.29. Following the transaction, the chief executive officer now owns 217,294 shares in the company, valued at $50,866,352.46. The trade was a 1.17 % decrease in their ownership of the stock. The sale was disclosed in a filing with the Securities & Exchange Commission, which is available through this hyperlink. Also, CAO Thomas Bull sold 169 shares of the firm’s stock in a transaction dated Thursday, January 2nd. The stock was sold at an average price of $234.09, for a total transaction of $39,561.21. Following the completion of the sale, the chief accounting officer now owns 14,899 shares of the company’s stock, valued at $3,487,706.91. This trade represents a 1.12 % decrease in their ownership of the stock. The disclosure for this sale can be found here. Over the last 90 days, insiders sold 34,156 shares of company stock worth $9,220,473. 3.60% of the stock is currently owned by insiders.
Hedge Funds Weigh In On MongoDB
Institutional investors and hedge funds have recently made changes to their positions in the business. Hilltop National Bank grew its position in MongoDB by 47.2% during the 4th quarter. Hilltop National Bank now owns 131 shares of the company’s stock valued at $30,000 after purchasing an additional 42 shares during the last quarter. Quarry LP grew its holdings in shares of MongoDB by 2,580.0% during the second quarter. Quarry LP now owns 134 shares of the company’s stock valued at $33,000 after buying an additional 129 shares during the last quarter. Brooklyn Investment Group bought a new stake in shares of MongoDB during the third quarter valued at approximately $36,000. GAMMA Investing LLC raised its holdings in MongoDB by 178.8% in the 3rd quarter. GAMMA Investing LLC now owns 145 shares of the company’s stock worth $39,000 after acquiring an additional 93 shares during the last quarter. Finally, Continuum Advisory LLC lifted its position in MongoDB by 621.1% in the 3rd quarter. Continuum Advisory LLC now owns 137 shares of the company’s stock valued at $40,000 after acquiring an additional 118 shares in the last quarter. Institutional investors own 89.29% of the company’s stock.
MongoDB Company Profile
MongoDB, Inc, together with its subsidiaries, provides general purpose database platform worldwide. The company provides MongoDB Atlas, a hosted multi-cloud database-as-a-service solution; MongoDB Enterprise Advanced, a commercial database server for enterprise customers to run in the cloud, on-premises, or in a hybrid environment; and Community Server, a free-to-download version of its database, which includes the functionality that developers need to get started with MongoDB.
See Also
This instant news alert was generated by narrative science technology and financial data from MarketBeat in order to provide readers with the fastest and most accurate reporting. This story was reviewed by MarketBeat’s editorial team prior to publication. Please send any questions or comments about this story to contact@marketbeat.com.
Before you consider MongoDB, you’ll want to hear this.
MarketBeat keeps track of Wall Street’s top-rated and best performing research analysts and the stocks they recommend to their clients on a daily basis. MarketBeat has identified the five stocks that top analysts are quietly whispering to their clients to buy now before the broader market catches on… and MongoDB wasn’t on the list.
While MongoDB currently has a “Moderate Buy” rating among analysts, top-rated analysts believe these five stocks are better buys.
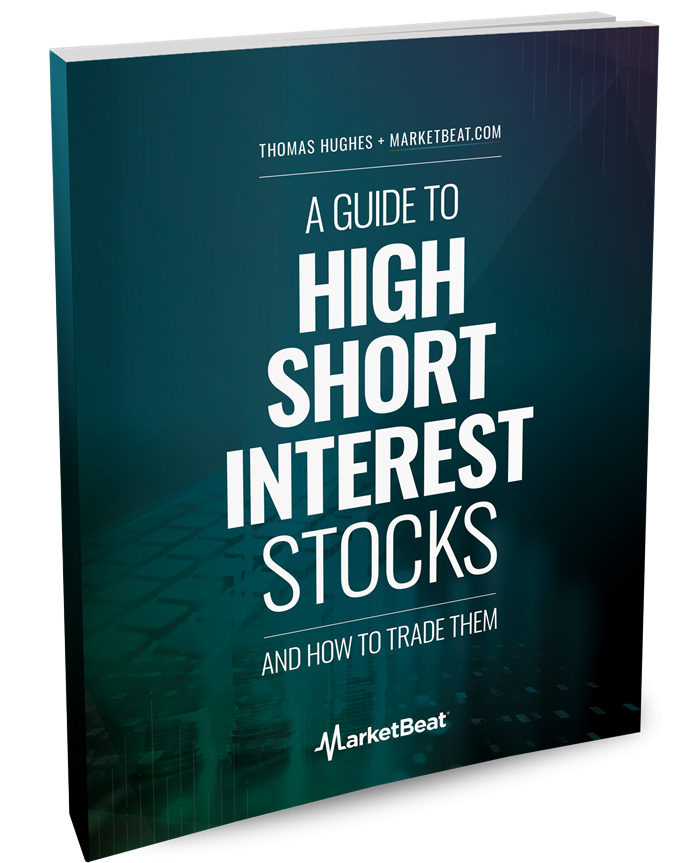
MarketBeat’s analysts have just released their top five short plays for February 2025. Learn which stocks have the most short interest and how to trade them. Enter your email address to see which companies made the list.
Article originally posted on mongodb google news. Visit mongodb google news
Is MongoDB (MDB) Among the Most Promising Growth Stocks According to Wall Street Analysts?
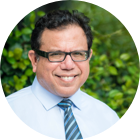
MMS • RSS
Posted on mongodb google news. Visit mongodb google news
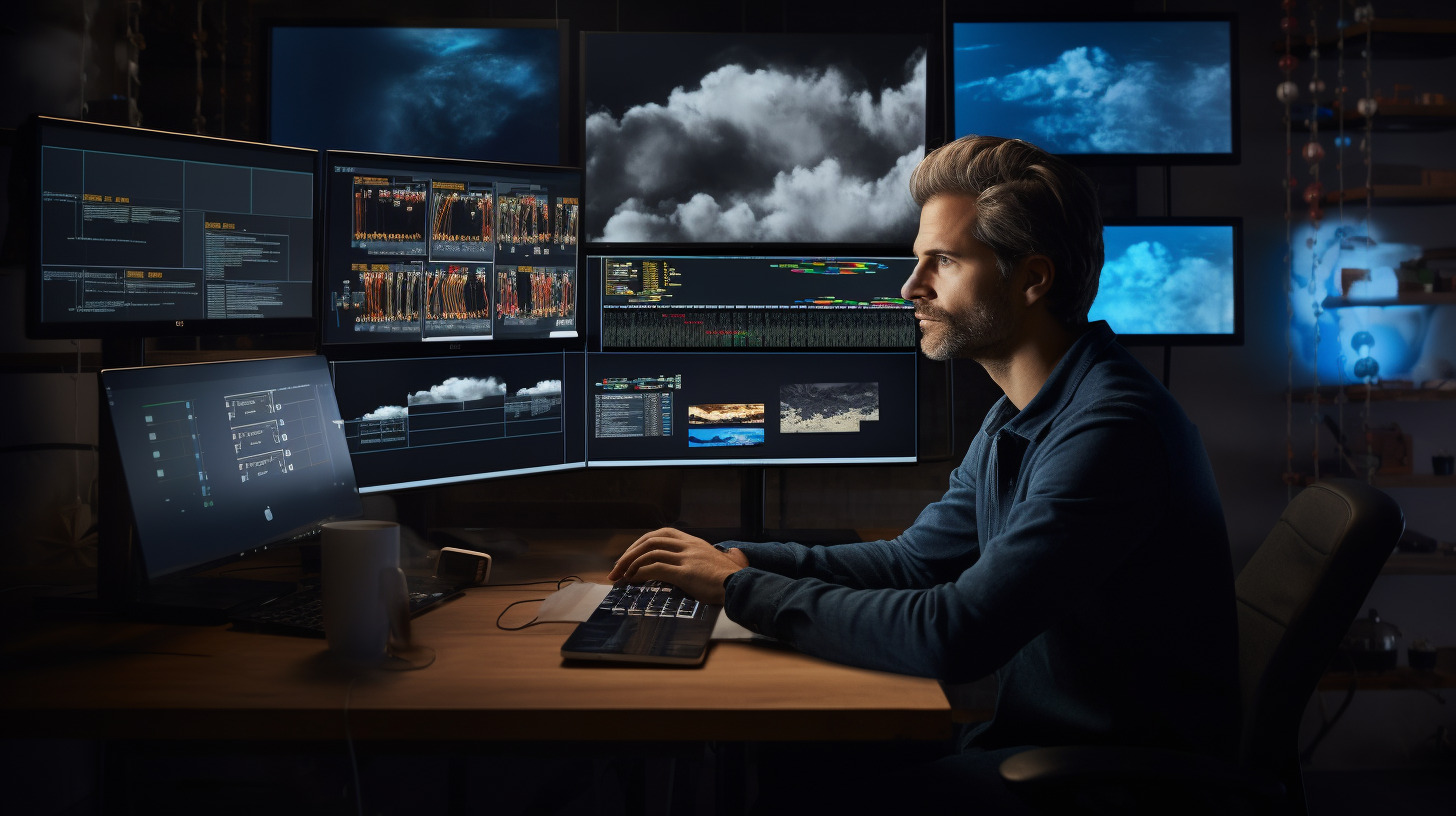
Artificial intelligence is the greatest investment opportunity of our lifetime. The time to invest in groundbreaking AI is now, and this stock is a steal!
The whispers are turning into roars.
Artificial intelligence isn’t science fiction anymore.
It’s the revolution reshaping every industry on the planet.
From driverless cars to medical breakthroughs, AI is on the cusp of a global explosion, and savvy investors stand to reap the rewards.
Here’s why this is the prime moment to jump on the AI bandwagon:
Exponential Growth on the Horizon: Forget linear growth – AI is poised for a hockey stick trajectory.
Imagine every sector, from healthcare to finance, infused with superhuman intelligence.
We’re talking disease prediction, hyper-personalized marketing, and automated logistics that streamline everything.
This isn’t a maybe – it’s an inevitability.
Early investors will be the ones positioned to ride the wave of this technological tsunami.
Ground Floor Opportunity: Remember the early days of the internet?
Those who saw the potential of tech giants back then are sitting pretty today.
AI is at a similar inflection point.
We’re not talking about established players – we’re talking about nimble startups with groundbreaking ideas and the potential to become the next Google or Amazon.
This is your chance to get in before the rockets take off!
Disruption is the New Name of the Game: Let’s face it, complacency breeds stagnation.
AI is the ultimate disruptor, and it’s shaking the foundations of traditional industries.
The companies that embrace AI will thrive, while the dinosaurs clinging to outdated methods will be left in the dust.
As an investor, you want to be on the side of the winners, and AI is the winning ticket.
The Talent Pool is Overflowing: The world’s brightest minds are flocking to AI.
From computer scientists to mathematicians, the next generation of innovators is pouring its energy into this field.
This influx of talent guarantees a constant stream of groundbreaking ideas and rapid advancements.
By investing in AI, you’re essentially backing the future.
The future is powered by artificial intelligence, and the time to invest is NOW.
Don’t be a spectator in this technological revolution.
Dive into the AI gold rush and watch your portfolio soar alongside the brightest minds of our generation.
This isn’t just about making money – it’s about being part of the future.
So, buckle up and get ready for the ride of your investment life!
Act Now and Unlock a Potential 10,000% Return: This AI Stock is a Diamond in the Rough (But Our Help is Key!)
The AI revolution is upon us, and savvy investors stand to make a fortune.
But with so many choices, how do you find the hidden gem – the company poised for explosive growth?
That’s where our expertise comes in.
We’ve got the answer, but there’s a twist…
Imagine an AI company so groundbreaking, so far ahead of the curve, that even if its stock price quadrupled today, it would still be considered ridiculously cheap.
That’s the potential you’re looking at. This isn’t just about a decent return – we’re talking about a 10,000% gain over the next decade!
Our research team has identified a hidden gem – an AI company with cutting-edge technology, massive potential, and a current stock price that screams opportunity.
This company boasts the most advanced technology in the AI sector, putting them leagues ahead of competitors.
It’s like having a race car on a go-kart track.
They have a strong possibility of cornering entire markets, becoming the undisputed leader in their field.
Here’s the catch (it’s a good one): To uncover this sleeping giant, you’ll need our exclusive intel.
We want to make sure none of our valued readers miss out on this groundbreaking opportunity!
That’s why we’re slashing the price of our Premium Readership Newsletter by a whopping 70%.
For a ridiculously low price of just $29.99, you can unlock a year’s worth of in-depth investment research and exclusive insights – that’s less than a single restaurant meal!
Here’s why this is a deal you can’t afford to pass up:
• Access to our Detailed Report on this Game-Changing AI Stock: Our in-depth report dives deep into our #1 AI stock’s groundbreaking technology and massive growth potential.
• 11 New Issues of Our Premium Readership Newsletter: You will also receive 11 new issues and at least one new stock pick per month from our monthly newsletter’s portfolio over the next 12 months. These stocks are handpicked by our research director, Dr. Inan Dogan.
• One free upcoming issue of our 70+ page Quarterly Newsletter: A value of $149
• Bonus Reports: Premium access to members-only fund manager video interviews
• Ad-Free Browsing: Enjoy a year of investment research free from distracting banner and pop-up ads, allowing you to focus on uncovering the next big opportunity.
• 30-Day Money-Back Guarantee: If you’re not absolutely satisfied with our service, we’ll provide a full refund within 30 days, no questions asked.
Space is Limited! Only 1000 spots are available for this exclusive offer. Don’t let this chance slip away – subscribe to our Premium Readership Newsletter today and unlock the potential for a life-changing investment.
Here’s what to do next:
1. Head over to our website and subscribe to our Premium Readership Newsletter for just $29.99.
2. Enjoy a year of ad-free browsing, exclusive access to our in-depth report on the revolutionary AI company, and the upcoming issues of our Premium Readership Newsletter over the next 12 months.
3. Sit back, relax, and know that you’re backed by our ironclad 30-day money-back guarantee.
Don’t miss out on this incredible opportunity! Subscribe now and take control of your AI investment future!
No worries about auto-renewals! Our 30-Day Money-Back Guarantee applies whether you’re joining us for the first time or renewing your subscription a year later!
Article originally posted on mongodb google news. Visit mongodb google news
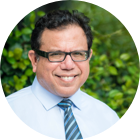
MMS • RSS
Posted on mongodb google news. Visit mongodb google news
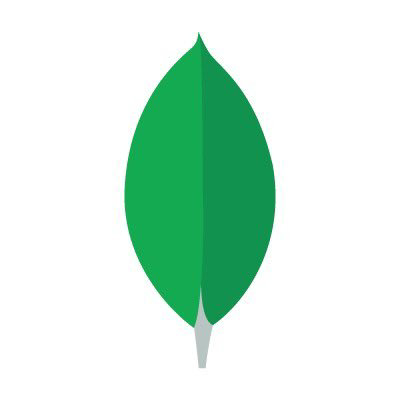
Shares of MongoDB (MDB, Financial), a leading database software company, surged by 6.09% today. This increase is attributed to the heightened discussions surrounding the future of AI, catalyzed by the recent presentation of DeepSeek.
The stock market is realigning its focus on companies like MongoDB (MDB, Financial) that are poised to thrive in the AI space. Regardless of which entity emerges as a frontrunner, MongoDB (MDB) stands to benefit from the overall growth and integration of AI across industries.
MongoDB (MDB, Financial) is well-positioned to capitalize on the increasing demand for cybersecurity, big data, and automation software driven by AI advancements. With a current stock price of $280.69, the company’s performance reflects its potential in these burgeoning sectors.
Analyzing MongoDB’s (MDB, Financial) financials, the company is navigating growth with several positive indicators. Despite having no price-to-earnings ratio due to negative earnings, MongoDB boasts a strong Altman Z-score of 7.34, indicating robust financial health. Additionally, the Price-to-Book (PB) ratio is at 13.9, nearing a five-year low, suggesting potential value for investors.
The company’s operating margins are expanding, and its financial strength is underscored by a Beneish M-Score of -2.71, suggesting it is unlikely to be involved in financial statement manipulation. Moreover, MongoDB’s (MDB, Financial) Price-to-Sales (PS) ratio is close to a two-year low at 10.02, presenting investors with an attractive entry point.
With a market capitalization of $22.49 billion, MongoDB (MDB, Financial) is considered significantly undervalued according to its GF Value of $430.79, offering a promising investment opportunity. The company’s revenue growth remains solid with a 16.2% increase over the past year, highlighting its ongoing momentum in the competitive software sector.
As the company continues to align itself with the evolving digital landscape and AI-driven innovations, MongoDB (MDB, Financial) is strategically placed to maintain its growth trajectory and deliver long-term value to its stakeholders.
Article originally posted on mongodb google news. Visit mongodb google news