Month: February 2025
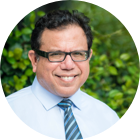
MMS • RSS
Software companies are constantly trying to add more and more AI features to their platforms, and it can be hard to keep up with it all. We’ve written this roundup to share updates from 10 notable companies that have recently enhanced their products with AI.
OpenAI announces research preview of GPT-4.5
OpenAI is calling GPT-4.5 its “largest and best model for chat yet.” The newest model was trained using data from smaller models, which improves steerability, understanding of nuance, and natural conversation, according to the company.
In comparison to o1 and o3-mini, this model is a more general-purpose model, and unlike o1, GPT-4.5 is not a reasoning model, so it doesn’t think before it responds.
“We believe reasoning will be a core capability of future models, and that the two approaches to scaling—pre-training and reasoning—will complement each other. As models like GPT‑4.5 become smarter and more knowledgeable through pre-training, they will serve as an even stronger foundation for reasoning and tool-using agents,” the company wrote in a post.
Anthropic releases Claude 3.7 Sonnet and Claude Code
Anthropic made two major announcements this week: the release of Claude 3.7 Sonnet and a research preview for an agentic coding tool called Claude Code.
Claude Sonnet is the company’s medium cost and performance model, sitting in between the smaller Haiku models and the most powerful Opus models.
According to Anthropic, Claude 3.7 Sonnet is the company’s most intelligent model yet and the “first hybrid reasoning model on the market.” It produces near-instant responses and has an extended thinking mode where it can provide the user with step-by-step details of how it came to its answers.
The company also announced a research preview for Claude Code, which is an agentic coding tool. “Claude Code is an active collaborator that can search and read code, edit files, write and run tests, commit and push code to GitHub, and use command line tools—keeping you in the loop at every step,” Anthropic wrote in a blog post.
Gemini 2.0 Flash-Lite now generally available
Google has announced that Gemini 2.0 Flash-Lite is now available in the Gemini App for production use in Google AI Studio or in Vertex AI for enterprise customers.
According to Google, this model features better performance in reasoning, multimodal, math, and factuality benchmarks compared to Gemini 1.5 Flash.
Google to offer free version of Gemini Code Assist
Google also announced that it is releasing a free version of Gemini Code Assist, which is an AI-coding assistant.
Now in public preview, Gemini Code Assist for individuals provides free access to a Gemini 2.0 model fine-tuned for coding within Visual Studio Code and JetBrains IDEs. The model was trained on a variety of real-world coding use cases and supports all programming languages in the public domain.
The assistant offers a chat interface that is aware of a developer’s existing code, provides automatic code completion, and can generate and transform full functions or files.
The free version has a limit of 6,000 code-related requests and 240 chat requests per day, which Google says is roughly 90 times more than other coding assistants on the market today. It also has a 128,000 input token context window, which allows developers to use larger files and ground the assistant with knowledge about their codebases.
Microsoft announces Phi-4-multimodal and Phi-4-mini
Phi-4-multimodal can process speech, vision, and text inputs at the same time, while Phi-4-mini is optimized for text-based tasks.
According to Microsoft, Phi-4-multimodal leverages cross-modal learning techniques to enable it to understand and reason across multiple different modalities at once. It is also optimized for on-device execution and reduced computational overhead.
Phi-4-mini is a 3.8B parameter model designed for speed and efficiency while still outperforming larger models in text-based tasks like reasoning, math, coding, instruction-following, and function-calling.
The new models are available in Azure AI Foundry, HuggingFace, and the NVIDIA API Catalog.
IBM’s next generation Granite models are now available
IBM has released the next generation models in its Granite family: Granite 3.2 8B Instruct, Granite 3.2 2B Instruct, Granite Vision 3.2 2B, Granite-Timeseries-TTM-R2.1, Granite-Embedding-30M-Sparse, and new model sizes for Granite Guardian 3.2.
Granite 3.2 8B Instruct and Granite 3.2 2B Instruct provide chain of thought reasoning that can be toggled on and off.
“The release of Granite 3.2 marks only the beginning of IBM’s explorations into reasoning capabilities for enterprise models. Much of our ongoing research aims to take advantage of the inherently longer, more robust thought process of Granite 3.2 for further model optimization,” IBM wrote in a blog post.
All of the new Granite 3.2 models are available on Hugging Face under the Apache 2.0 license. Additionally, some of the models are accessible through IBM watsonx.ai, Ollama, Replicate, and LM Studio.
Precisely announces updates to Data Integrity Suite
The Data Integrity Suite is a set of services that help companies ensure trust in their data, and the latest release includes several AI capabilities.
The new AI Manager allows users to register LLMs in the Data Integrity Suite to ensure they comply with the company’s requirements, offers the ability to scale AI usage using external LLMs with processing handled by the same infrastructure the LLM is on, and uses generative AI to create catalog asset descriptions.
Other updates in the release include role-based data quality scores, new governance capabilities, a new Snowflake connector, and new metrics for understanding latency.
Warp releases its AI-powered terminal on Windows
Warp allows users to navigate the terminal using natural language, leveraging a user’s saved commands, codebase context, what shell they are in, and their past actions to make recommendations.
It also features an Agent Mode that can be used to debug errors, fix code, and summarize logs, and it has the power to automatically execute commands.
It has already been available on Mac and Linux, and the company said that Windows support has been its most requested feature over the past year. It supports PowerShell, WSL, and Git Bash, and will run on x64 or ARM64 architectures.
MongoDB acquires Voyage AI for its embedding and reranking models
MongoDB has announced it is acquiring Voyage AI, a company that makes embedding and reranking models.
This acquisition will enable MongoDB’s customers to build reliable AI-powered applications using data stored in MongoDB databases, according to the company.
According to MongoDB, it will integrate Voyage AI’s technology in three phases. During the first phase, Voyage AI’s embedding and reranking models will still be available through Voyage AI’s website, AWS Marketplace, and Azure Marketplace.
The second phase will involve integrating Voyage AI’s models into MongoDB Atlas, beginning with an auto-embedding service for Vector Search and then adding native reranking and domain-specific AI capabilities.
During the third phase, MongoDB will advance AI-powered retrieval with multi-modal capabilities, and introduce instruction tuned models.
IBM announces intent to acquire DataStax
The company plans to utilize DataStax AstraDB and DataStax Enterprise’s capabilities to improve watsonx.
“Our combined technology will capture richer, more nuanced representations of knowledge, ultimately leading to more efficient and accurate outcomes. By harnessing DataStax’s expertise in managing large-scale, unstructured data and combining it with watsonx’s innovative data AI solutions, we will provide enterprise ready data for AI with better data performance, search relevancy, and overall operational efficiency,” IBM wrote in a post.
IBM has also said that it will continue collaborating on DataStax’s open source projects Apache Cassandra, Langflow, and OpenSearch.
Read last week’s AI announcements roundup here.
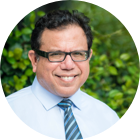
MMS • RSS
For the week ending Feb. 28, CRN takes a look at the companies that brought their ‘A’ game to the channel including IBM, HashiCorp, DataStax, MongoDB, EDB, Intel and Alibaba.
The Week Ending Feb. 28
Topping this week’s Five Companies that Came to Win is IBM for completing its $6.4 billion acquisition of HashiCorp., a leading developer of hybrid IT infrastructure management and provisioning tools, and for striking a deal to buy database development platform company DataStax.
Also making this week’s list is cloud database provider MongoDB for its own strategic acquisition in the AI modeling technology space. EDB is here for a major revamp of its channel program as it looks to partners to help expand sales of its EDB Postgres AI database. Intel makes the list for expanding its Xeon 6 line of processors for mid-range data center systems. And cloud giant Alibaba got noticed for its vow to invest $53 billion in AI infrastructure.
IBM Closes $6.4B HashiCorp Acquisition, Strikes Deal To Buy DataStax
IBM tops this week’s Five Companies That Came to Win list for two strategic acquisitions.
On Thursday, IBM said it completed its $6.4 billion purchase of HashiCorp, a leading provider of tools for managing and provisioning cloud and hybrid IT infrastructure and building, securing and running cloud applications.
IBM will leverage HashiCorp’s software, including its Terraform infrastructure-as-code platform, to boost its offerings in infrastructure and security life-cycle management automation, infrastructure provisioning, multi-cloud management, and consulting and artificial intelligence, among other areas.
The deal’s consummation was delayed while overseas regulators, including in the U.K., scrutinized the acquisition.
Earlier in the week, IBM announced an agreement to acquire DataStax and its cloud database development platform in a move to expand the capabilities of the IBM Watsonx AI portfolio.
IBM said adding DataStax to Watsonx will accelerate the use of generative AI at scale among its customers and help “unlock value” from huge volumes of unstructured data.
“The strategic acquisition of DataStax brings cutting-edge capabilities in managing unstructured and semi-structured data to Watsonx, building on open-source Cassandra investments for enterprise applications and enabling clients to modernize and develop next-generation AI applications,” said Ritika Gunnar, IBM general manager, data and AI, in a blog post.
In addition to its flagship database offering, DataStax’s product portfolio includes Astra Streaming for building real-time data pipelines, the DataStax AI Platform for building and deploying AI applications, and an enterprise AI platform that incorporates Nvidia AI technology. Another key attraction for IBM is DataStax’s Langflow open-source, low-code tools for developing AI applications that use retrieval augmented generation (RAG).
MongoDB Looks To Improve AI Application Accuracy With Voyage AI Acquisition
Sticking with the topic of savvy acquisitions, MongoDB makes this week’s list for acquiring Voyage AI, a developer of “embedding and rerank” AI models that improve the accuracy and efficiency of RAG (retrieval-augmented generation) data search and retrieval operations.
MongoDB plans to add the Voyage AI technology to its platform to help businesses and organizations build more trustworthy AI and Generative AI applications that deliver more accurate results with fewer hallucinations.
“AI has the promise to transform every business, but adoption is held back by the risk of hallucinations,” said Dev Ittycheria, MongoDB CEO, in a statement announcing the acquisition.
MongoDB’s developer data platform, based on its Atlas cloud database, has become a popular system for building AI-powered applications. Last May the company launched its MongoDB AI Applications Program (MAAP), which provides a complete technology stack, services and other resources to help partners and customers develop and deploy at scale applications with advanced generative AI capabilities.
“By bringing the power of advanced AI-powered search and retrieval to our highly flexible database, the combination of MongoDB and Voyage AI enables enterprises to easily build trustworthy AI-powered applications that drive meaningful business impact. With this acquisition, MongoDB is redefining what’s required of the database for the AI era,” Ittycheria said.
EDB Boosts Channel Program Offerings As It Expands Data Platform Sales For AI, Analytical Tasks
EDB wins applause for expanding its channel program, including increasing investments to raise partners’ expertise and go-to-market capabilities, as the company looks to boost adoption of its Postgres-based database platform for more data analytics and AI applications.
The company also launched a new partner portal and “industry success hub” repository of vertical industry customer case studies that partners can draw on.
The upgraded EDB Partner Program is the company’s latest move as it looks to grow beyond its roots of providing a transaction-oriented, Oracle-compatible database to developing a comprehensive data and AI platform.
EDB is a long-time player in the database arena with its software based on the open-source PostgreSQL database. But EDB has been shooting higher for the last 18 months following the appointment of former Wind River CEO Kevin Dallas as the database company’s new CEO in August 2023.
The expanded channel program offerings tie in with last year’s launch of EDB Postgres AI, a major update of the company’s flagship database that can handle transactional, analytical and AI workloads.
Intel Debuts Midrange Xeon 6 CPUs To Fight AMD In Enterprise Data Centers
Intel showed off its technology prowess this week when it launched its new midrange Xeon 6 processors to help customers consolidate data centers for a broad range of enterprise applications.
Intel said the new processors provide superior performance and lower total cost of ownership than AMD’s latest server CPUs.
Using the same performance cores of the high-end Xeon 6900P Series, the new Xeon 6700P series scales to 86 cores on 350 watts while the new Xeon 6500P series reaches up to 32 cores on 225 watts, expanding the Xeon 6 family for a broader swath of the data center market. Intel said its Xeon 6 processors “have already seen broad adoption across the data center ecosystem, with more than 500 designs available now or in progress” from major vendors such as Dell Technologies, Nvidia, Hewlett Packard Enterprise, Lenovo, Microsoft, VMware, Supermicro, Oracle, Red Hat and Nutanix, “among many others.
Intel also debuted the Xeon 6 system-on-chips for network and edge applications and two new Ethernet product lines.
Alibaba Plans To Invest $53B In AI Infrastructure
Cloud computing giant Alibaba Group got everyone’s attention this week when it unveiled plans to invest $53 billion in its AI infrastructure and data centers over the next three years.
Alibaba looks to become a global powerhouse in providing AI infrastructure and AI models with CEO Eddie Wu declaring that AI is now the company’s primary focus.
Alibaba builds its own open-source AI large language models (LLMs), dubbed Qwen, while owning billions worth of cloud infrastructure inside its data centers. The Chinese company recently introduced the Alibaba Cloud GenAI Empowerment Program, a dedicated support program for global developers and startups leveraging its Qwen models to build generative AI applications.
Alibaba said its new $53 billion AI investment exceeds the company’s spending in AI and cloud computing over the past decade.
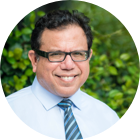
MMS • Robert Krzaczynski
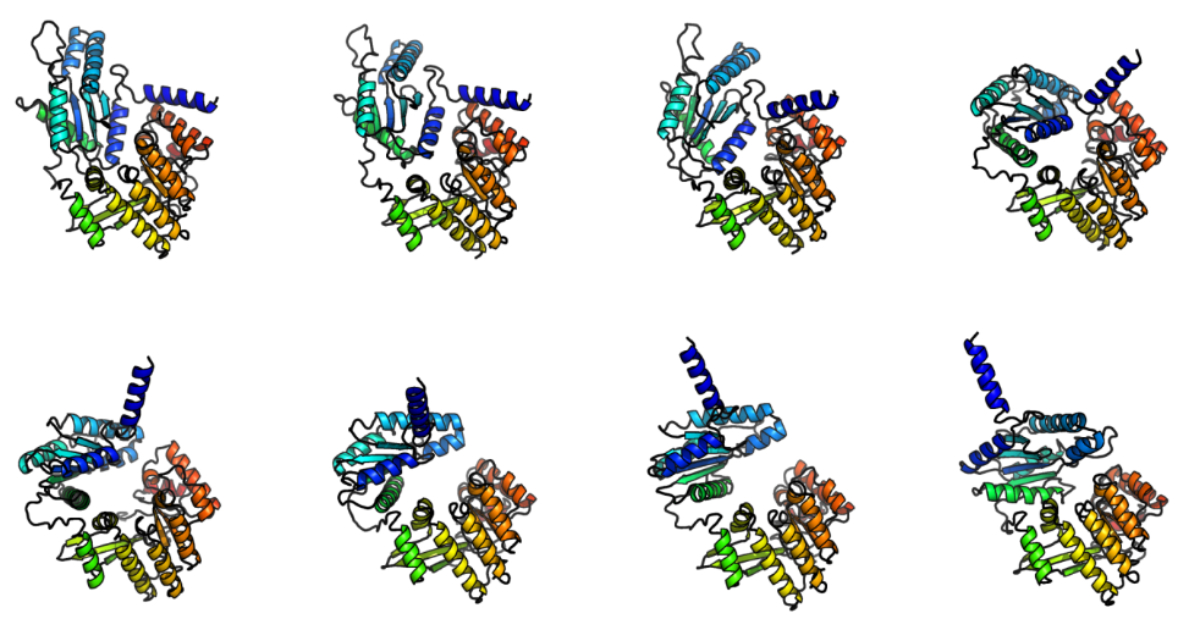
Microsoft Research has introduced BioEmu-1, a deep-learning model designed to predict the range of structural conformations that proteins can adopt. Unlike traditional methods that provide a single static structure, BioEmu-1 generates structural ensembles, offering a broader view of protein dynamics. This method may be especially beneficial for understanding protein functions and interactions, which are crucial in drug development and various fields of molecular biology.
One of the main challenges in studying protein flexibility is the computational cost of molecular dynamics (MD) simulations, which model protein motion over time. These simulations often require extensive processing power and can take years to complete for complex proteins. BioEmu-1 offers an alternative by generating thousands of protein structures per hour on a single GPU, making it 10,000 to 100,000 times more computationally efficient than conventional MD simulations.
BioEmu-1 was trained on three types of datasets: AlphaFold Database (AFDB) structures, an extensive MD simulation dataset, and an experimental protein folding stability dataset. This method allows the model to generalize to new protein sequences and predict various conformations. It has successfully identified the structures of LapD, a regulatory protein in Vibrio cholerae bacteria, including both known and unobserved intermediate conformations.
BioEmu-1 demonstrates strong performance in modeling protein conformational changes and stability predictions. The model achieves 85% coverage for domain motion and 72–74% coverage for local unfolding events, indicating its ability to capture structural flexibility. The BioEmu-Benchmarks repository provides benchmark code, allowing researchers to evaluate and reproduce the model’s performance on various protein structure prediction tasks.
Experts in the field have noted the significance of this advancement. For example, Lakshmi Prasad Y. commented:
The open-sourcing of BioEmu-1 by Microsoft Research marks a significant leap in overcoming the scalability and computational challenges of traditional molecular dynamics (MD) simulations. By integrating AlphaFold, MD trajectories, and experimental stability metrics, BioEmu-1 enhances the accuracy and efficiency of protein conformational predictions. The diffusion-based generative approach allows for high-speed exploration of free-energy landscapes, uncovering crucial intermediate states and transient binding pockets.
Moreover, Nathan Baker, a senior director of partnerships for Chemistry and Materials at Microsoft, reflected on the broader implications:
I ran my first MD simulation over 25 years ago, and my younger self could not have imagined having a powerful method like this to explore protein conformational space. It makes me want to go back and revisit some of those molecules!
BioEmu-1 is now open-source and available through Azure AI Foundry Labs, providing researchers with a more efficient method for studying protein dynamics. By predicting protein stability and structural variations, it can contribute to advancements in drug discovery, protein engineering, and related fields.
More information about the model and results can be found in the official paper.
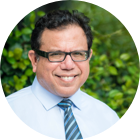
MMS • RSS
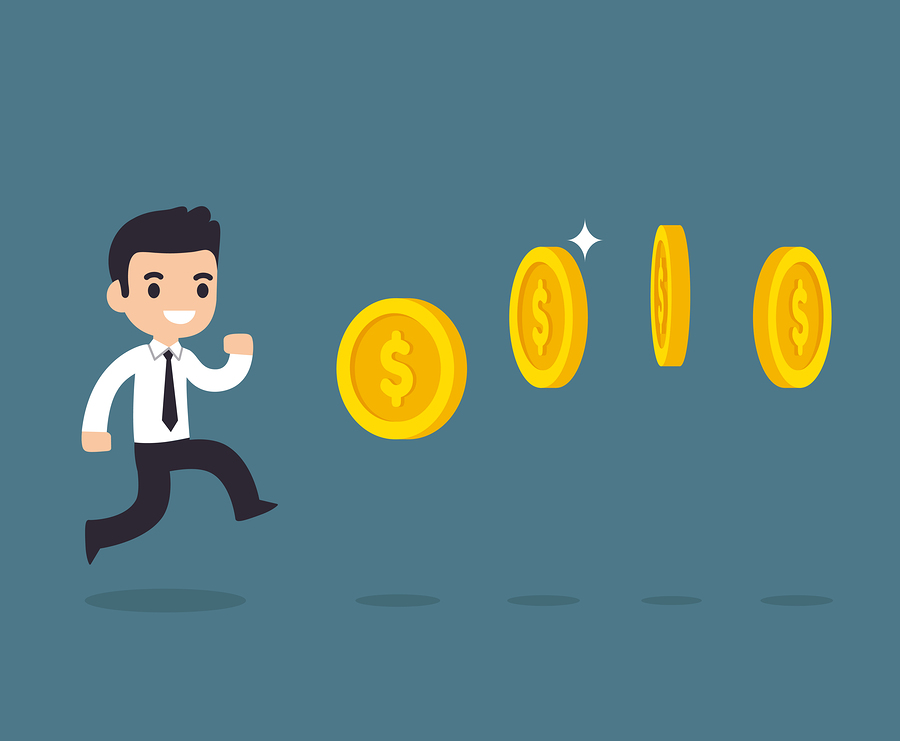
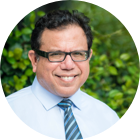
MMS • RSS
Solutions Review Executive Editor Tim King curated this list of notable data management news for the week of February 28, 2025.
Keeping tabs on all the most relevant big data and data management news can be a time-consuming task. As a result, our editorial team aims to provide a summary of the top headlines from the last week in this space. Solutions Review editors will curate vendor product news, mergers and acquisitions, venture capital funding, talent acquisition, and other noteworthy big data and data management news items.
For early access to all the expert insights published on Solutions Review, join Insight Jam, a community dedicated to enabling the human conversation on AI.
Top Data Management News for the Week Ending February 28, 2025
Acceldata Announces Agentic Data Management
It augments and replaces traditional data quality, and governance tools, data catalogs, with a unified AI-driven platform, transforming how enterprises manage and optimize data. By leveraging AI agents, contextual memory, and automated actions, Agentic DM ensures data is always reliable, governed, and AI-ready—without human intervention.
Rohit Choudhary, Founder and CEO at Acceldata.io, said: “Acceldata Agentic Data Management enables true autonomous operations, which is intent-based and context-aware.”
Ataccama Releases 2025 Data Trust Report
The Data Trust Report suggests that organizations must reframe their thinking to see compliance as the foundation for long-term business value and trust. The report found that 42 percent of organizations prioritize regulatory compliance, but only 26 percent focus on it within their data teams. This highlights blind spots with real-world consequences like regulatory fines and data breaches that can erode customer trust, financial stability, and competitiveness.
BigID Announces New BigID Next Offering
With a modular, AI-assisted architecture, BigID Next empowers organizations to take control of their most valuable asset—data—while adapting to the fast-evolving risk and compliance landscape in the age of AI.
Couchbase Integrates Capella AI Model Services with NVIDIA NIM Microservices
Capella AI Model Services, powered by NVIDIA AI Enterprise, minimize latency by bringing AI closer to the data, combining GPU-accelerated performance and enterprise-grade security to empower organizations to seamlessly operate their AI workloads. The collaboration enhances Capella’s agentic AI and retrieval-augmented generation (RAG) capabilities, allowing customers to efficiently power high-throughput AI-powered applications while maintaining model flexibility.
Cribl Unveils New Crible Lakehouse Offering
With Cribl Lakehouse, companies can eliminate the complexity of schema management and manual data transformation while simultaneously delivering ultra-elastic scalability, federated query capabilities, and a fully unified management experience across diverse datasets and geographies.
Google Adds Inline Filtering to AlloyDB Vector Search
Inline filtering helps ensure that these types of searches are fast, accurate, and efficient — automatically combining the best of vector indexes and traditional indexes on metadata columns to achieve better query performance.
GridGain Contributes to Latest Release of Apache Ignite 3.0
Apache Ignite has long been the trusted choice for developers at top companies, enabling them to achieve unmatched speed and scale through the power of distributed in-memory computing. These contributions reflect GridGain’s continued commitment to the open-source distributed database, caching, and computing platform.
Paige Roberts, Head of Technical Evangelism at GridGain, said: “Completely rearchitecting the core of Apache Ignite 3 to improve stability and reliability provides real-time response speed on a foundation that just works. GridGain 9.1 is built on that foundation, plus things from 9.0 like on-demand scaling and strong data consistency that were needed to better support AI workflows. Any data processing and analytics platform that isn’t aimed at supporting AI these days is already obsolete.”
Hydrolix Joins AWS ISV Accelerate Program
The AWS ISV Accelerate Program provides Hydrolix with co-sell support and benefits to meet customer needs through collaboration with AWS field sellers globally. Co-selling provides better customer outcomes and assures mutual commitment from AWS and its partners.
IBM Set to Acquire DataStax
IBM says the move will help enterprises “harness crucial enterprise data to maximize the value of generative AI at scale.”
William McKnight, President at McKnight Consulting Group, said: “DataStax has a very strong vector database. In our benchmark, we found Astra DB ingestion and indexing speeds up to 6x faster than Pinecone, with more consistent performance. It also showed faster search response times both during and after indexing. Furthermore, Astra DB exhibited better recall (accuracy) during active indexing and maintained low variance. Finally, DataStax Astra DB Serverless (Vector) had a significantly lower Total Cost of Ownership (TCO).
IBM was supporting Milvus, but now they have their own vector capabilities.”
Robert Eve, Advisor at Robert Eve Consulting, said: “This seems like a win-win-win for DataStax customers, IBM customers, and both firms’ investors.
As we have seen many times in the database industry, specialized capabilities frequently find homes within broader offerings as market acceptance grows, installed bases overlap, and requirements for shared (common) development tools and operational functionality increase.
This is yet another example.”
MetaRouter Launches New Schema Enforcement Layer
Designed to address persistent challenges in data quality, consistency, and governance, this new tool ensures that data flowing through MetaRouter pipelines adheres to defined schema standards. MetaRouter’s Schema Enforcement tool tackles these challenges head-on by empowering data teams to define, assign, and enforce schemas, preventing bad data from ever reaching downstream systems.
MongoDB is Set to Acquire Voyage AI
Integrating Voyage AI’s technology with MongoDB will enable organizations to easily build trustworthy, AI-powered applications by offering highly accurate and relevant information retrieval deeply integrated with operational data.
Observo AI Launches New AI Data Engineer Assistant Called Orion
Orion is an on-demand AI data engineer, enabling teams to build, optimize, and manage data pipelines through natural language interactions, dramatically reducing the complexity and expertise traditionally required for these critical operations.
Precisely Announces New AI-Powered Features
These advancements address key enterprise data challenges such as improving data accessibility, enabling business-friendly governance, and automating manual processes. Together, they help organizations boost efficiency, maximize the ROI of their data investments, and make confident, data-driven decisions.
Profisee MDM is Now Available in Public Preview for Microsoft Fabric
This milestone positions Profisee as the sole MDM provider offering an integrated solution within Microsoft Fabric, empowering users to define, manage and leverage master data products directly within the platform.
Redpanda Releases New Snowflake Connector Based on Snowpipe Streaming
The Redpanda Snowflake Connector not only optimizes the parsing, validation code, and assembly for building the files that are sent to Snowflake, but also makes it easy to split a stream into multiple tables and do custom transformations on data in flight.
Reltio Launches New Lightspeed Data Delivery Network
Reltio Data Cloud helps enterprises solve their most challenging data problems—at an immense scale—and enables AI-driven innovation across their organizations. Reltio Data Cloud today processes 61.1B total API calls per year and has 9.1B consolidated profiles under management, 100B relationships under management, and users across 140+ countries.
Saksoft Partners with Cleo on Real-Time Logistics Data Integration
The partnership aims to empower Saksoft customers—particularly in the Logistics industry—with CIC, enabling them to harness EDI and API automation alongside real-time data visibility. This will equip them with the agility, control, and actionable insights necessary to drive their business forward with confidence.
Snowflake Launches Silicon Valley AI Hub
Located in the heart of Silicon Valley at Snowflake’s new Menlo Park campus, the nearly 30,000 square foot space plans to open in Summer 2025, and will feature a range of spaces designed for people across the AI ecosystem.
Expert Insights Section
Watch this space each week as our editors will share upcoming events, new thought leadership, and the best resources from Insight Jam, Solutions Review’s enterprise tech community where the human conversation around AI is happening. The goal? To help you gain a forward-thinking analysis and remain on-trend through expert advice, best practices, predictions, and vendor-neutral software evaluation tools.
InsightJam.com’s Mini Jam LIVE! Set for March 6, 2025
From AI Architecture to the Application Layer explores the critical transition of AI from its underlying architectures to its practical applications in order to illustrate the transformations occurring now in the Enterprise Tech workspace.
This one-day Virtual Event brings together leading experts across four thought leader Keynotes and four expert Panels to reveal the nuances of AI Development, from the design of AI to its integration of radical solutions in virtually every tech application.
NEW by Solutions Review Expert @ Insight Jam Thought Leader Dr. Joe Perez – Bullet Train Breakthroughs: Lessons in Data, Design & Discovery
Ford revolutionized automobile manufacturing by observing meatpacking lines and how they moved animal carcasses along their conveyor belts. Adapting that mechanization to his factory, Ford was able to streamline production from 12 hours for each car to a mere 90 minutes, a feat that would change the character of manufacturing forever.
NEW by Solutions Review Expert @ Insight Jam Thought Leader Nicola Askham – Do You Need a Data Strategy and a Data Governance Strategy?
With the increasing importance of data, many organizations are asking whether they need both a data strategy and a Data Governance strategy. I’ve been doing Data Governance for over twenty years now, and I’ll be honest – in the first fifteen years, no one even talked about a data strategy or a Data Governance strategy. But before we dive into the answer, let’s start by getting the basics straight.
NEW by Solutions Review Expert @ Insight Jam Thought Leader Dr. Irina Steenbeek – Key Takeaways from the Free Masterclass: Adapting Data Governance for Modern Data Architecture
I believe that data governance and data management follow a yin-yang duality. Data governance defines why an organization must formalize a data management framework and its feasible scope. Data management, in turn, designs and establishes the framework, while data governance controls its implementation.
What to Expect at Solutions Review‘s Spotlight with Object First & Enterprise Strategy Group on March 13
According to ESG research 81 percent of businesses agree immutable backup storage is the best defense against ransomware. However, not all backup storage solutions can deliver full immutability and enable the quick and complete recovery that is essential for ransomware resilience. With the next Solutions Spotlight event, the team at Solutions Review has partnered with leading Veeam storage provider Object First.
On-Demand: Solutions Review‘s Spotlight with Concentric AI & Enterprise Strategy Group
With the next Solutions Spotlight event, the team at Solutions Review has partnered with leading data security governance platform provider Concentric AI to provide viewers with a unique webinar called Modern Data Security Governance in the Generative AI Era.
Insight Jam Panel Highlights: Best Practices for Ensuring Data Quality & Integrity in the AI Pipeline
They address critical challenges with data provenance, bias detection, and records management while offering insights on legal compliance considerations. Essential viewing for security professionals implementing AI systems who need to balance innovation with data quality requirements.
For consideration in future data management news roundups, send your announcements to the editor: tking@solutionsreview.com.
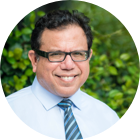
MMS • RSS
Generali Investments Towarzystwo Funduszy Inwestycyjnych grew its position in MongoDB, Inc. (NASDAQ:MDB – Free Report) by 32.3% in the fourth quarter, according to its most recent 13F filing with the Securities and Exchange Commission. The fund owned 12,300 shares of the company’s stock after acquiring an additional 3,000 shares during the quarter. MongoDB makes up approximately 1.7% of Generali Investments Towarzystwo Funduszy Inwestycyjnych’s holdings, making the stock its 17th largest position. Generali Investments Towarzystwo Funduszy Inwestycyjnych’s holdings in MongoDB were worth $2,864,000 at the end of the most recent quarter.
A number of other institutional investors have also made changes to their positions in the company. Jennison Associates LLC increased its holdings in shares of MongoDB by 23.6% in the 3rd quarter. Jennison Associates LLC now owns 3,102,024 shares of the company’s stock valued at $838,632,000 after acquiring an additional 592,038 shares during the last quarter. Geode Capital Management LLC increased its holdings in shares of MongoDB by 2.9% in the 3rd quarter. Geode Capital Management LLC now owns 1,230,036 shares of the company’s stock valued at $331,776,000 after acquiring an additional 34,814 shares during the last quarter. Westfield Capital Management Co. LP increased its holdings in shares of MongoDB by 1.5% in the 3rd quarter. Westfield Capital Management Co. LP now owns 496,248 shares of the company’s stock valued at $134,161,000 after acquiring an additional 7,526 shares during the last quarter. Holocene Advisors LP grew its stake in MongoDB by 22.6% during the 3rd quarter. Holocene Advisors LP now owns 362,603 shares of the company’s stock worth $98,030,000 after buying an additional 66,730 shares during the last quarter. Finally, Assenagon Asset Management S.A. grew its stake in MongoDB by 11,057.0% during the 4th quarter. Assenagon Asset Management S.A. now owns 296,889 shares of the company’s stock worth $69,119,000 after buying an additional 294,228 shares during the last quarter. 89.29% of the stock is currently owned by institutional investors.
Insider Transactions at MongoDB
In other MongoDB news, insider Cedric Pech sold 287 shares of MongoDB stock in a transaction on Thursday, January 2nd. The shares were sold at an average price of $234.09, for a total transaction of $67,183.83. Following the sale, the insider now owns 24,390 shares in the company, valued at $5,709,455.10. The trade was a 1.16 % decrease in their ownership of the stock. The transaction was disclosed in a filing with the Securities & Exchange Commission, which is available at this link. Also, CAO Thomas Bull sold 169 shares of MongoDB stock in a transaction on Thursday, January 2nd. The stock was sold at an average price of $234.09, for a total transaction of $39,561.21. Following the sale, the chief accounting officer now owns 14,899 shares in the company, valued at $3,487,706.91. This represents a 1.12 % decrease in their position. The disclosure for this sale can be found here. Over the last quarter, insiders have sold 41,979 shares of company stock worth $11,265,417. Insiders own 3.60% of the company’s stock.
MongoDB Trading Down 2.2 %
Shares of MongoDB stock opened at $262.41 on Friday. The company’s fifty day simple moving average is $261.95 and its 200-day simple moving average is $274.67. The company has a market cap of $19.54 billion, a price-to-earnings ratio of -95.77 and a beta of 1.28. MongoDB, Inc. has a 1 year low of $212.74 and a 1 year high of $449.12.
MongoDB (NASDAQ:MDB – Get Free Report) last announced its quarterly earnings data on Monday, December 9th. The company reported $1.16 earnings per share (EPS) for the quarter, topping the consensus estimate of $0.68 by $0.48. The business had revenue of $529.40 million for the quarter, compared to the consensus estimate of $497.39 million. MongoDB had a negative net margin of 10.46% and a negative return on equity of 12.22%. MongoDB’s quarterly revenue was up 22.3% on a year-over-year basis. During the same quarter last year, the company posted $0.96 earnings per share. As a group, research analysts forecast that MongoDB, Inc. will post -1.78 earnings per share for the current fiscal year.
Analysts Set New Price Targets
A number of research analysts recently commented on MDB shares. Robert W. Baird upped their price objective on MongoDB from $380.00 to $390.00 and gave the stock an “outperform” rating in a research note on Tuesday, December 10th. Canaccord Genuity Group upped their price objective on MongoDB from $325.00 to $385.00 and gave the stock a “buy” rating in a research note on Wednesday, December 11th. Rosenblatt Securities assumed coverage on MongoDB in a research report on Tuesday, December 17th. They set a “buy” rating and a $350.00 price target for the company. JMP Securities reiterated a “market outperform” rating and set a $380.00 price target on shares of MongoDB in a research report on Wednesday, December 11th. Finally, DA Davidson boosted their price target on MongoDB from $340.00 to $405.00 and gave the stock a “buy” rating in a research report on Tuesday, December 10th. Two analysts have rated the stock with a sell rating, four have assigned a hold rating, twenty-three have assigned a buy rating and two have issued a strong buy rating to the stock. Based on data from MarketBeat.com, the stock currently has a consensus rating of “Moderate Buy” and an average price target of $361.00.
View Our Latest Research Report on MDB
About MongoDB
MongoDB, Inc, together with its subsidiaries, provides general purpose database platform worldwide. The company provides MongoDB Atlas, a hosted multi-cloud database-as-a-service solution; MongoDB Enterprise Advanced, a commercial database server for enterprise customers to run in the cloud, on-premises, or in a hybrid environment; and Community Server, a free-to-download version of its database, which includes the functionality that developers need to get started with MongoDB.
Recommended Stories
Want to see what other hedge funds are holding MDB? Visit HoldingsChannel.com to get the latest 13F filings and insider trades for MongoDB, Inc. (NASDAQ:MDB – Free Report).
This instant news alert was generated by narrative science technology and financial data from MarketBeat in order to provide readers with the fastest and most accurate reporting. This story was reviewed by MarketBeat’s editorial team prior to publication. Please send any questions or comments about this story to contact@marketbeat.com.
Before you consider MongoDB, you’ll want to hear this.
MarketBeat keeps track of Wall Street’s top-rated and best performing research analysts and the stocks they recommend to their clients on a daily basis. MarketBeat has identified the five stocks that top analysts are quietly whispering to their clients to buy now before the broader market catches on… and MongoDB wasn’t on the list.
While MongoDB currently has a Moderate Buy rating among analysts, top-rated analysts believe these five stocks are better buys.
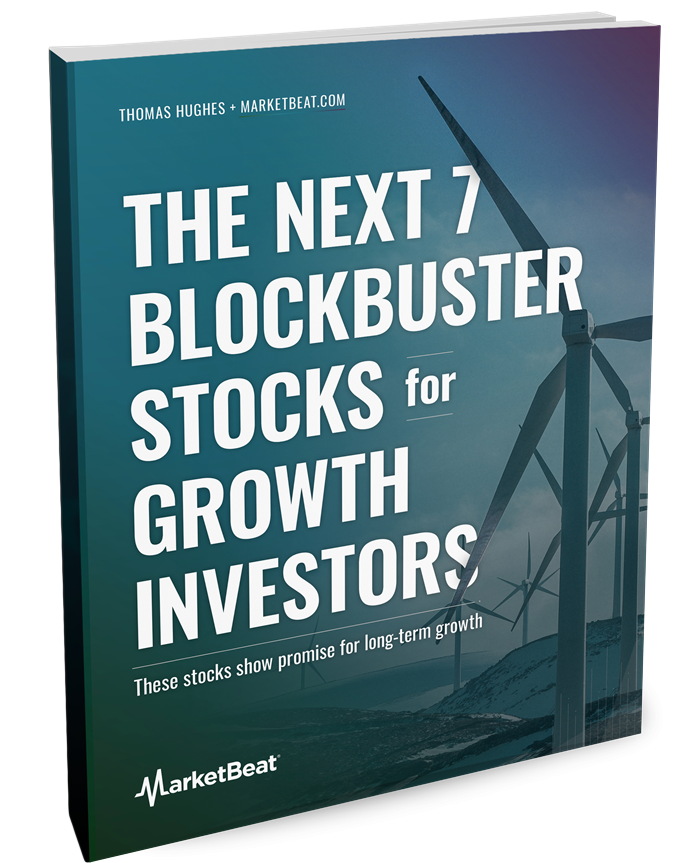
Wondering what the next stocks will be that hit it big, with solid fundamentals? Enter your email address to see which stocks MarketBeat analysts could become the next blockbuster growth stocks.
Google Cloud Introduces Quantum-Safe Digital Signatures in Cloud KMS to Future-Proof Data Security
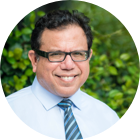
MMS • Steef-Jan Wiggers
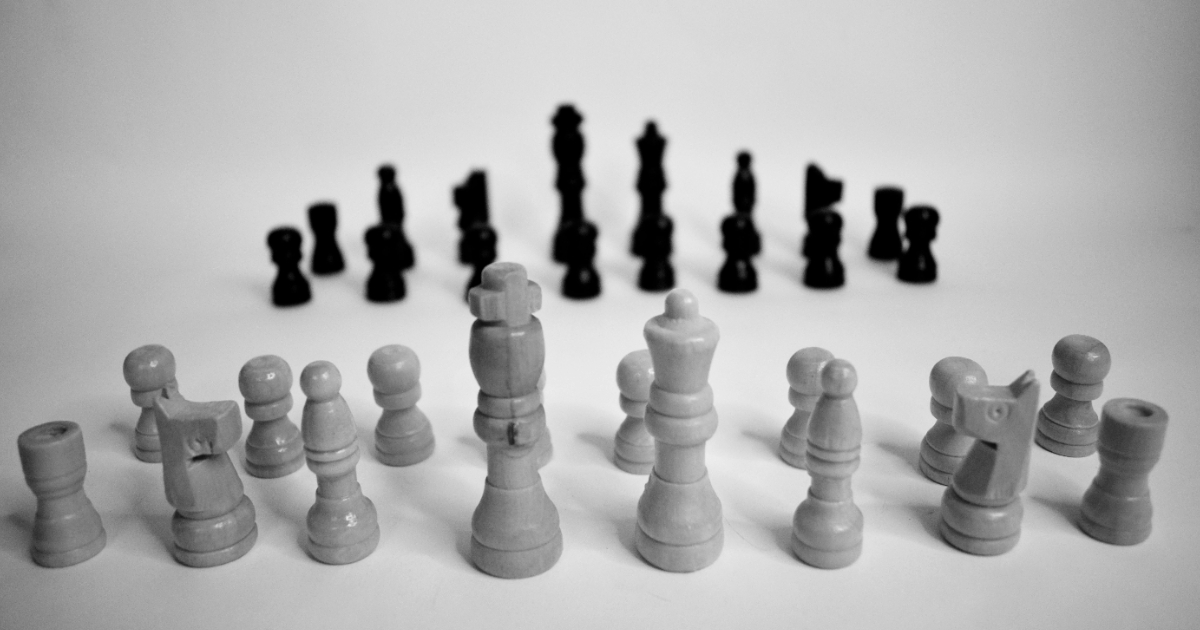
Google recently unveiled quantum-safe digital signatures in its Cloud Key Management Service (Cloud KMS), aligning with the National Institute of Standards and Technology (NIST) post-quantum cryptography (PQC) standards. This update, now available in preview, addresses the growing concern over the potential risks posed by future quantum computers, which could crack traditional encryption methods.
Quantum computing, with its ability to solve problems exponentially faster than classical computers, presents a serious challenge to current cryptographic systems. Algorithms like Rivest–Shamir–Adleman (RSA) and elliptic curve cryptography (ECC), which are fundamental to modern encryption, could be vulnerable to quantum attacks.
One of the primary threats is the “Harvest Now, Decrypt Later” (HNDL) model, where attackers store encrypted data today with plans to decrypt it once quantum computers become viable. While large-scale quantum computers capable of breaking these cryptographic methods are not yet available, experts agree that preparing for this eventuality is crucial.
To safeguard against these quantum threats, Google integrates two NIST-approved PQC algorithms into Cloud KMS. The first is the ML-DSA-65 (FIPS 204), a lattice-based digital signature algorithm; the second is SLH-DSA-SHA2-128S (FIPS 205), a stateless, hash-based signature algorithm. These algorithms provide a quantum-resistant means of signing and verifying data, ensuring that organizations can continue to rely on secure encryption even in a future with quantum-capable adversaries.
Google’s decision to integrate these algorithms into Cloud KMS allows enterprises to test and incorporate quantum-resistant cryptography into their security workflows. The cryptographic implementations are open-source via Google’s BoringCrypto and Tink libraries, ensuring transparency and allowing for independent security audits. This approach is designed to help organizations gradually transition to post-quantum encryption without overhauling their entire security infrastructure.
The authors of a Google Cloud blog post write:
While that future may be years away, those deploying long-lived roots-of-trust or signing firmware for devices managing critical infrastructure should consider mitigation options against this threat vector now. The sooner we can secure these signatures, the more resilient the digital world’s foundation of trust becomes.
Google’s introduction of quantum-safe digital signatures comes at a time when the need for post-quantum security is becoming increasingly urgent. The rapid evolution of quantum computing, highlighted by Microsoft’s recent breakthrough with its Majorana 1 chip, raises concerns over the imminent risks of quantum computers. While these machines are not yet powerful enough to crack current encryption schemes, experts agree that the timeline to quantum readiness is narrowing, with NIST aiming for compliance by 2030.Top of Form
Phil Venables, a chief information security officer at Google Cloud, tweeted on X:
Cryptanalytically Relevant Quantum Computers (CRQCs) are coming—perhaps sooner than we think, but we can conservatively (and usefully) assume in the 2032 – 2040 time frame. Migrating to post-quantum cryptography will be more complex than many organizations expect, so starting now is vital. Adopting crypto-agility practices will mitigate the risk of further wide-scale changes as PQC standards inevitably evolve.
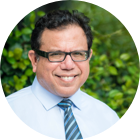
MMS • Shruti Bhat
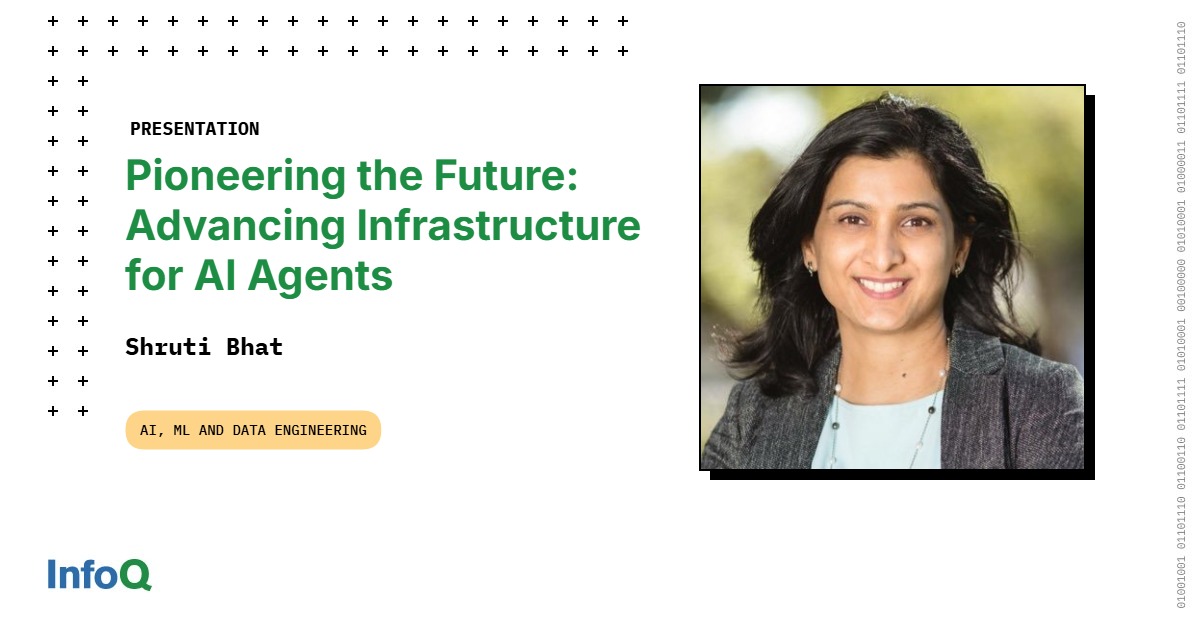
Transcript
Bhat: What we’re going to talk about is agentic AI. I have a lot of detail to talk about, but first I want to tell you the backstory. I personally was at Oracle like eight years ago. I landed there through another acquisition. After I finished integrating the company, I said, let me go explore some new opportunities. I ended up leading AI strategy across all of Oracle’s products. Eight years ago, we didn’t have a lot of answers, and almost every CIO, CDO, CTO I spoke with, the minute we started talking about AI, they’d say, “Stop. I only have one question for you. I’ve been collecting all the semi-structured data, unstructured data, putting it in my data lake, like you guys asked me to, but that’s where data goes to die. How do I go from data to intelligent apps?”
Frankly, we didn’t have a great answer back then. That’s what took me on this quest. I was looking for startups we could acquire. I was looking for who was innovating. I ended up joining Rockset as a founding team member. I was the chief product officer over the last seven years there. We built this distributed search and AI database, which got acquired by OpenAI. Now, eight years later, I’m having a lot of very similar conversations where enterprises are asking, what we have today with AI, LLMs, are very interesting, ChatGPT, super interesting. Doesn’t write great intros, but it’s pretty good for other things. The question still remains, how do I go from my enterprise data to intelligent agents, no longer apps. That’s what we’re going to talk about.
GenAI Adoption
Before I get into it, though, let’s look at some data. Somebody asked me the other day if I believe in AI. I believe in God and I believe in data. Let’s see what data tells us. If you chart out the adoption of some of the most transformative technologies of our times, PCs, internet, and look at it two years out from when it was first commercialized. Like, what was the first commercially available version? For PCs, it goes back to 1981, when the IBM PC was commercialized. Then you plot it out two years later and see what was the rate of adoption. With GenAI, it’s almost twice that of what we saw with PCs and internet. That’s how fast this thing is taking over. That’s both incredibly exciting and creating a lot of disruption for a lot of people. When people now ask me if I believe in AI, I’m basically saying, here’s what the data says. I don’t want to be on the wrong side of history for this one.
GenAI vs. Agentic AI
The previous chart that I showed you, that was basically GenAI. We’re now talking about agentic AI. What’s the difference? The simplest way to think about or just internalize the main concept here is, with GenAI, we talk about zero-shot, and as you start getting better prompt engineering, you’d go into multi-shot. Really with agentic AI, we’re talking about multi-step. Think about how you and I work. If you’re writing code, you don’t just sit down and say, let me go write this big piece of code, and the first time you do it, end-to-end, assume that it’s going to be perfect. No, you take a series of steps. You say, let me go gather some more data around this. Let me go do some research. Let me talk to some other senior engineers. Let me iterate on this thing, test, debug, get some code reviews.
These are all the steps that you’re following. How can you possibly expect AI to just go in zero step and get it perfectly right? It doesn’t. The big advancement we’re seeing now is with multi-step and agentic AI. That brings us to the most simple definition of agent that there is. It can basically act. It’s not just generating text or not just generating images for you. It’s actually taking actions, so it has some autonomy. It can collaborate with other agents. This is very important. As we get into the talk here, you’ll see why the success depends on multi-agent collaboration. Of course, it has to be able to learn. Imagine an agent that’s helping you with your flight bookings. You might ask it to go rebook your tickets, and it might collaborate with a couple more agents to go look up a bunch of flights. It might collaborate with another agent to maybe accept your credit card payment.
Eventually, after it’s done the booking, it realizes it’s overbooked that flight. It has to learn from that mistake and do better next time. This is the most simple definition. We’re all engineers. We like precise definitions. The interesting thing that happened in the industry is this sparked a whole lot of debate on what exactly is the correct definition of an agent. Hien was literally debating against himself in the keynote, and that’s what we like to do. We like to debate. A very simple solution came out, which is, let’s just agree it’s agentic. We don’t really know what exactly an agent looks like, but now we can start looking at what agentic AI looks like.
I really like this definition. We’re not great at naming, but the definition makes sense, because the reality is that this lies on a spectrum. Agentic AI really lies on a very wide spectrum. That’s what your spectrum looks like. We talked a little bit about multi-step. In prompt engineering, what you’re doing is you’re just going zero-shot to multi-shot. You’re really trying to understand, how can I give a series of prompts to my LLM to get better outputs? Now with agentic AI, you’re very quickly saying, maybe I don’t want the same LLM to be called every single time. You might call the same LLM, or you might call a different LLM as you start realizing that different models do better on different dimensions.
Very quickly you realize that if, again, you think about a human, the most fundamental thing that a human does well is reasoning and memory. It’s not enough to have reasoning, you also need memory. You need to remember all the learnings that you’ve had from your past. That’s the long-term memory. Then you need memory between your steps, and that’s just the short-term working memory. The long-term memory typically comes from vector databases. In fact, Rockset, the database that I worked on over the last seven years, was an indexing database. What we did was we indexed all the enterprise data for search and retrieval.
Then, of course, added vector indexing so that you could now do hybrid search. Vector databases are great for long-term memory. There’s still a lot of new stuff emerging on the short-term working memory. That’s just one part of the equation. As you start adding memory, you want learning, and this is what reflection does. Reflection is basically where you say, I’m going to do the feedback loop iteratively and keep going and keep learning and keep getting better, until you get to a point where you’re not getting any more feedback. It can continue. Learning is endless. Every time something changes externally, the learning loop kicks in again, and you keep having more reflection. This is like a self-refined loop, and this is super important for agentic AI.
Planning and tool use is another big area that’s emerging. In fact, when you see LLMs doing planning, it’s quite mind boggling. As you get more senior in your role, you get really good at looking at a big problem and breaking it down into specific things. You think about, how do you chunk it up into smaller tasks? You think about, who do you assign it to? In what sequence does it need to be done? Again, an LLM is really good at doing this kind of planning and getting those steps executed in the right way. The tool use portion is just as important, because as it’s planning, it’s planning what tools to use as well. Those tools are supplied by you, so the quality of your output depends on what tools you’re supplying. Here I have some examples, like search, email, calendaring. That’s not all. You can think about the majority of the tools actually being code execution. You decide what it needs to compute, and you can give it some code execution, and those can be the functions that it calls and uses as tools.
Eventually, you can imagine agents using all these tools to do their work. Again, you control the quality of that output by giving it excellent tools. As you start thinking about this, you get into multi-agent collaboration really fast. When you think about the discussion around agents so far, the reason for this is the multi-agent collaboration gives you much better output. This actually is some really interesting data that was shared by Andrew Ng of DeepLearning.AI. HumanEval is a coding benchmark, so it basically has a series of simple programming prompts. Think of it like something you’d see on a basic software engineering interview, and you can use that to benchmark how well your GPT is performing.
In this case, 3.5 outperforms GPT-4 when you use agentic workflows. That’s really powerful. Most of you know that between 3.5 and 4, there was a drastic improvement. You can almost see that on the screen there. If you look at just zero-shot between 3.5 and 4, the improvement was amazing, but now 3.5 is already able to outperform GPT-4 if you layer on or wrap agentic workflows on top.
As you look at this, the next layer of complexity is, let’s get into multi-agent workflows, because there is absolutely no way an agent can do an entire role. The agent can only do specific tasks. What you really want to do is, you scope the task, you decide how small the task can be, and then you have one agent just focusing on one specific task. The best mental model I can give you is, think about microservices. Some of you may love it. Some of you may hate it. The reason you want to think about microservices is the future we’re looking at already is a micro agent future. You’re not going to have these agents that just resemble a human being. You’re going to have agents that do very specific tasks and they collaborate with each other.
Where Are We Headed? (Evolution and Landscape of Agentic AI)
If many of you have seen some of the splashy demos that came out earlier and then said, this simply does not work in production, this is the real reason that agents failed earlier this year. People tried to create these agents that would literally take on entire roles. I’m going to have an agent that’s going to be an SDR. I’m going to replace all these SDRs. I’m going to be an agent that replaces software engineers. It’s not happening. The reason is, going from that splashy demo to production is extremely hard. Today, the state of the art is very much, let’s be realistic. Let’s break it down to very small, simple tasks. Let’s narrow the scope as much as we possibly can, and let’s allow these micro agents to orchestrate, coordinate, and collaborate with each other. If you imagine that, you can envision a world where you have very specific things.
Going back to your software engineering example, you have an agent that starts writing code. You have another agent that is reviewing the code, all it does is code review. You have another agent that’s planning and scheduling and allowing them to figure out how they break down their tasks. You might even have a manager agent that’s overseeing all of them. Is this going to really replace us? Unlikely. Think of them as your own little set of minions that do your work for you.
However, like somebody asked me, does that mean now I have to manage all these minions? That’s the last thing I want to do. Not really, because this is where your supervisor agents come in, your planner agents, your manager agents come in. You will basically interface with a super minion that can handle and coordinate and assign tasks and report back to you. This is just like the right mental model, as you think about where are we headed and how do we think about the complexity that this is going to create if everybody in the organization starts creating a set of agents for themselves? This was my thesis, but I was really happy to see that this view of the world is starting to emerge from external publications as well.
This was actually McKinsey publishing their view of how you’re going to have all these specialist agents. They not only use the tools that you provide to them, they also query your internal databases. They might go query your Salesforce. They might go query wherever your data lives, depending on what governance you lay on, and what access controls you give these agents.
Agentic AI Landscape: Off-the-Shelf vs. Custom Enterprise Agents
That brings me to, what is the agentic AI landscape as of today? Some of these startups might actually be familiar to you. Resolve.ai had a booth at QCon. I’m by no means saying that these are the winning startups. All I’m saying is these are startups where I’ve personally spent time with the investors, with the CEOs, going super deep into, what are they building? What are the challenges? Where do they see this going? I’ll give you a couple of these examples. Sierra AI is building a customer service agent. SiftHub, which is building a sales agent, very quickly showed me a demo that says, “We’re not trying to create a salesperson. We’re just trying to automate one very specific task, and that is as specific as just responding to RFPs”. If you’ve ever been in a situation where your sales team is saying, “I need input from you. I need to go respond to this RFP, or I need input from you to go through the security review”.
That is a very specific task in a salesperson’s end-to-end workflow, and that’s really where SiftHub is focusing their sales agent. That’s how specific it gets. Resolve.ai is calling it an AI production engineer, but if you look at the demo, it shows you, basically, it’s in Slack with you, so basically, it’s exactly where you live. It’s an on-call support. When you’re on-call, it helps you to remediate. It’s very simple. It’s RCA remediation. It only kicks in when you’re on-call. This is where all the startups are focusing. When you look at enterprise platforms, and the enterprise companies are coming in saying, this is great. Startups are going in building these small, vertical agents.
If you look at an end-to-end workflow in any enterprise, there’s no way these startups are going to build all these little custom agents that they need, so might as well create a way for people to go build their own custom agents. Most of the big companies, Salesforce, Snowflake, Databricks, OpenAI are all starting to talk about custom enterprise agents. How do you build them? How do you deploy them, using the data that already lives on their platform? Each of them is taking a very different approach.
Salesforce coming at it from having all your customer data in there. To Databricks and Snowflake coming at it from a very different perspective, because they’re being your warehouse or your data lake. To OpenAI, of course, coming in with having both the LLM. This is really the choice that you have. Do you use off-the-shelf or do you build your own custom enterprise agents? The real answer is, as long as you can use an off-the-shelf agent, you want to do that. There’s just not going to be enough off-the-shelf agents. In that case, you might want to build a custom agent for yourself. It is really hard.
Custom AI Agents – Infra Stack and Abstractions
That’s what we’re going to talk about. What are the challenges in building these custom agents? Where is the infra stack today? What are the abstractions that you want to think about and layer on? How do you approach this problem space? I’m just going to simplify the infra building blocks here. Most of these are things that you’ve already done for your applications. This is where all the learning that you’ve had in building enterprise applications really transfers over. Of course, at the foundational level, you have the foundation models, that’s what’s giving you the reasoning.
Then you have your data layer, whether it’s your data lake, and of course, your vector database, giving you the memory. The context and memory and the reasoning give you the foundation. As you get into the many micro agents that we talked about, the real challenges are going to show up in the orchestration and governance layer. Because somebody was asking me, again, isn’t it easier for us to just wait for the reasoning to become so good that we don’t have to deal with any of this? Not really. Because if you think about how this is developing, I’m going to give you a real example, it’s not like, let’s say you’ve been hiring high school interns at work. They’re your equivalent of saying, I just have a simple foundational model that can do simple tasks using general knowledge.
As these models get better, it’s not like you’re suddenly getting a computer science engineer or you’re getting some PhD researcher. What you’re really getting is the IQ of that intern has gotten better and higher, but they’re still not super specialized, so you will have to give it the context. You will have to build in the specialization, irrespective of how much advancement you start seeing. You will see a lot more advancement on the reasoning side, but all these other building blocks are still with you. The governance layer here is really complicated. Again, a lot of the primitives that we’ve had on the application governance, it still carries over. How do you think about access controls? How do you think about privacy? How do you think about access to sensitive data? If an agent can act on your behalf, how do you make sure that these agents are carrying over the privileges that were assigned to the person who created the agent? There’s a lot of complexity that you need to think through.
Similarly, on the orchestration side too, when you start thinking about multi-agent workflows, it’s not simply, this agent is now passing information or passing the ball to another agent. What ends up happening is now you suddenly see distributed systems. Because, again, when LLM starts planning the work, it immediately starts distributing it. Now all the orchestration challenges that you’re very familiar with in distributed systems are going to show up here. It’s up to you and how you want to orchestrate all of this.
Then, on the top layer, you will see conspicuously missing, much talk about UIs. Why? Because the way you interact with an agent is going to be very different. Whether it’s conversational or whether it’s chat, you’re going to interact with it in the channels that you live today. You really don’t want to go to a bunch of different tools and log in, and forget the password every single time. You just have an agent, and that agent now is collaborating with a bunch of other agents to get the work done. The real top layer is going to be SDKs and APIs’ access to the tools that you are providing it. This is where it’s up to you to control which tools it can access. What is the quality of the tools that you’re providing it? How do you set up the infra building blocks in a way that is really scalable?
I want to spend a minute on specifically the context question, because you think about where we opened and said, we’re still figuring out how do you bring all of the enterprise context into agentic AI? There are a few different ways to go about it. We talked about prompt engineering. I always say, if you can get away with prompting, you want to stay there. It’s the simplest and most flexible way to get the job done. Many times, prompting is just not enough.
Then you look at more advanced techniques, RAG, or Retrieval Augmented Generation is the simplest, next best alternative. What you basically do with RAG is you’re indexing all your data. Imagine a vector database, Rockset. What we built is a great example here. Imagine you’re indexing your enterprise data so that now, as you’re building your agentic workflows, you’re building in the reasoning alongside your enterprise context. The challenge here is, it’s first very complicated, setting up your RAG pipeline, choosing your vector database, scaling your vector database, not that simple. The other big challenge you’re going to run into is, it’s extremely expensive.
Indexing is not cheap, as you know. Real-time indexing, which is what you run into when you really want agents that can act in real-time. Real-time indexing is very expensive, and you want to keep your data updated. Unless you really need it, you don’t want to go there. Fine-tuning is when you have much more domain-specific needs and training. I’d rather not go there, unless you absolutely have to, because as an enterprise, you’re much more in control of your data, your context, and much better to make a foundational model work for you. There are plenty of good ones out there.
Take your pick, open source, like really fast-paced development there. It’s very expensive too. Training your own model is extremely hard, extremely expensive, and just getting the talent to go train your own models in this competitive environment, forget about it. I think the first three are really the most commonly deployed. Training, yes, if you have extremely high data maturity and domain-specific needs.
The other thing to remember here is, all these building blocks we talked about are changing very fast. If you’re going to expose all of these to your end users, it’s going to be very hard. The best thing you can do is think about, what are the right abstractions from the user’s perspective? I have Salesforce as an example here because I thought they did a good job of really thinking about, what is the end user thinking about? How do they create agents? Really exposing those abstractions to everybody in the enterprise, and then decoupling your infra stack from how users create and manage their agents. The minute you do this decoupling, it gives you a lot more flexibility and allows you to move much faster as your infra stack starts changing.
Advancing Your Infrastructure for Agentic AI
With that, let’s talk more into what are the real challenges in your infra stack? I think we talked about the building blocks, but it looks very simple. It’s pretty complex under the hood. This statement, “Software is eating the world, but AI is going to eat software”, I couldn’t agree more. I’ve been having some conversations with some of the startups doing agentic AI and asking them how their adoption is going. The biggest finding for them is how quickly they’re able to go and show companies that they can go reduce their SaaS application licenses. You have 300 licenses of, name your favorite SaaS vendor, we’ll bring that down to 30 by the end of the year, and 5 the following year.
That’s the trajectory that you’re talking to customers about, and they’re already seeing that. They’re seeing customers starting to retire. One of the more famous ones was the Klarna CEO actually made a statement saying that they are now completely retiring Workday and Salesforce in favor of agentic AI. That is really bold and fast, if they’re able to get there that quickly. That is the thinking. Klarna was actually my customer while we were at Rockset. All of the innovation was happening in the engineering team. The engineers were moving incredibly fast, experimenting with AI, and before you know it, we have the Klarna CEO make a statement like this. This is going to be a very interesting world.
This tradeoff is one of the hardest to make. Having worked on distributed databases for the last few years, we spent so much time thinking about the price, performance tradeoff, and how do you give users more control so they can make their own tradeoffs? Now you have the cost, accuracy, latency tradeoff. Accuracy, especially like going from 95% to 98%, it’s like 10x more expensive. You really have to think deeply about what is the use case, and what tradeoff does it need? Latency, again, real-time is very expensive. Unless your agent needs to answer questions about flight booking and what seats are available right now, you might not need that much real-time.
Maybe you can make do with an hour latency, as long as you set up the guardrails in a way that this agent is going to be an hour behind. Cost, I had this really interesting experience. I was in the room. We were having this really heated debate between product and engineering. The product managers are like, I need this kind of performance. Why can’t you deliver? The engineers were like, you’re out of your mind. There’s no way we can ever get there at that scale. This is ridiculous. After 10 minutes of this, someone asked a very simple question, what is the implicit cost assumption we’re making here? That is really the disconnect between what product managers are saying and what the engineers are saying. Engineers know there’s a fixed budget, and the PMs are only thinking about, I can go charge the customer more, but just give me the performance. This disconnect is very real.
The only advice I have here is, think very deeply about your use case and what tradeoffs you’re making for your use case. Communicate it with all your stakeholders as early and often as you can, because these can’t be implicit assumptions, you have to make sure everybody’s on the same page. Then know that these tradeoffs will keep changing, especially given how fast the models are changing. You’re going to get advancements. These tradeoffs will change. If you made certain assumptions and bake them in, all hell will break loose when the next model comes out.
The previous one was about tradeoffs. Here there’s no or, this is an and. You absolutely need to layer on trust as one of the most important layers here. There are four key components. You’re thinking about transparency. Literally, nobody will adopt agentic AI, or any sort of AI if they cannot understand what was the thought process behind it. What were the steps? If you’ve even used GPT search, it now gives you links that tell you where am I getting this information from. If you want credibility, you want adoption, you have to build explainability right into it. I want to give a shout out to Resolve.ai for their demo, immediately, not only does it do an RCA, it tells you why. Why does it think this is the root cause, and gives you links to all of the information that it’s using to get there.
That’s explainability you want to bake in. Observability is hard. You know all the application logging and monitoring in everything that you’ve built in, how do you now apply that to agentic AI when agents are going in, taking all these actions on behalf of humans? Are you going to log every single action? You probably have to because you need to be able to trace back. What are you going to monitor? How are you going to evaluate? There’s just so many challenges on the observability side. This is where, as senior engineers, all the learnings that you’ve had from application domain, can transfer over here. This is still an emerging field. There aren’t a lot of easy answers. We’ve talked a little bit about governance.
How do you ensure that the agent is only accessing the data it’s supposed to? How do you ensure that it’s not doing anything that is not authorized? How do you make sure that it’s not getting malicious attacks, because anytime you create this new surface vector, you’re going to have more attacks? We haven’t even seen the beginning of what kind of cybersecurity challenges we’re going to run into once you start giving agentic AI more controls. The more you can get ahead of this now, think about these challenges, because this is moving so fast. Before you know it, you’ll have somebody in the company saying, yes, we’ve already built a couple of agents and deployed it here. What about all these other things?
The scalability concerns are also extremely real. When OpenAI was talking to us, I was looking at your scale, and it completely blew my mind. It was 100x more than anything I’d ever seen. That kind of scalability is by design. You have to bake it in from day one. The best approach here is to take a very modular approach. Not only are you breaking down your entire workflow into specific agents, you’re also thinking about, how do you break up your agent itself into a very modular approach so that each of them can be scaled independently, debugged independently?
We talked about the data stack, your foundational model. Make sure every single thing is modular, and that you have monitoring and evaluation built right into it. Because what’s going to happen is, you’re going to have a lot more advancements in every part of your stack, and you have to be able to swap that piece out depending on what the state of the art is. As you swap it out, you have to be able to evaluate the impact of this new change. You’re going to see a lot of regressions if GPT-5 comes out tomorrow, and you go, let me just swap out 4o and just drop in 5. I have no idea what is going to break. I’m speaking about GPT, but you can apply this to Claude. You can apply this to anything.
The next version comes out, you’re going to see a bunch of regressions because we just don’t know what this is going to look like. I think Sam Altman in one of his interviews made a really interesting statement, he said, the startups that are going to get obliterated very soon would be the ones that are building for the current state of AI, because everything is going to change. Especially, how do you think about this when maybe your development cycles are 6 to 12 months, whereas the advancements in AI are happening every month, or every other month.
Unknown Unknowns
That brings us to what we call the unknown unknowns. This is the most difficult challenge. When we were building an early-stage startup at Rockset, we used to say this, building a startup is like driving on a winding road at night with only your headlights to guide you. Of course, it’s raining, and you have no idea what unknown unknowns you’re going to hit. It’s kind of where we are today. The best thing to do is say, what things are going to change? Let’s bake in flexibility and agility into everything that we do. Let’s assume that things are going to change very fast. You can choose to wait it out. You might be thinking, if things are changing so fast, why don’t I just wait it out? You could, but then your competitor is probably not waiting it out. Other people in your company are not going to wait. Everybody is really excited. They start deploying agents, and before you know it, this is going rogue.
Lean Into The 70-20-10 (Successful Early Adopters Prioritize the Human Element)
This is really the most interesting piece as I’ve seen companies go through this journey. What ends up happening is, all of us, we love to talk about the tech, the data stack, the vector databases, which LLM, let’s compare all these models. Let’s talk about what algorithms, like what tools are we going to give to the agents. This is only less than 30%, ideally, of your investment. Where you want to focus is on the people and the process. Because what ends up happening, if you envision this new world where you’re going to have your own personal set of minions, not just you, but everybody in the company starts creating all of these agents or micro agents which are collaborating with each other, and with your agents, and maybe even some off-the-shelf agents that you’ve purchased, can you imagine how much that is going to disrupt the way we do work today?
This whole concept of, let’s open up this application, log in to this UI, and do all of these things, just goes away. That is going to be much harder than anything we can imagine on the agentic AI technology advancements, because ultimately you’re disrupting everything that people know. It was really fun to hear Victor’s presentation on multi-agent workflows. In one slide he mentioned, let’s make sure the agents delegate some of the tricky tasks to humans. I still believe, humans will be delegating work to agents, and not the other way round. How do you delegate tasks to agents? What should you delegate? How do you make sure that agents understand the difference in weights between all the different tasks that it can do? That is going to be really hard.
It is a huge opportunity to stop doing tedious tasks. It is a huge opportunity to get a lot more productive. It comes at the cost of a lot of disruption. It comes with people and processes having to really readjust to this new world of working. What we’re finding is that most of the leaders who are embracing this change are spending 70% of their time and energy and investment into people and processes, and only 30% on the rest. This is a really good mental model to have.
Conclusion
We haven’t talked at all about ethics. Eliezer is one of the researchers on ethics. I think the greatest danger really is that we conclude that we understand it, because we are just scratching the surface. The fun is just beginning.
See more presentations with transcripts
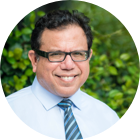
MMS • Courtney Nash
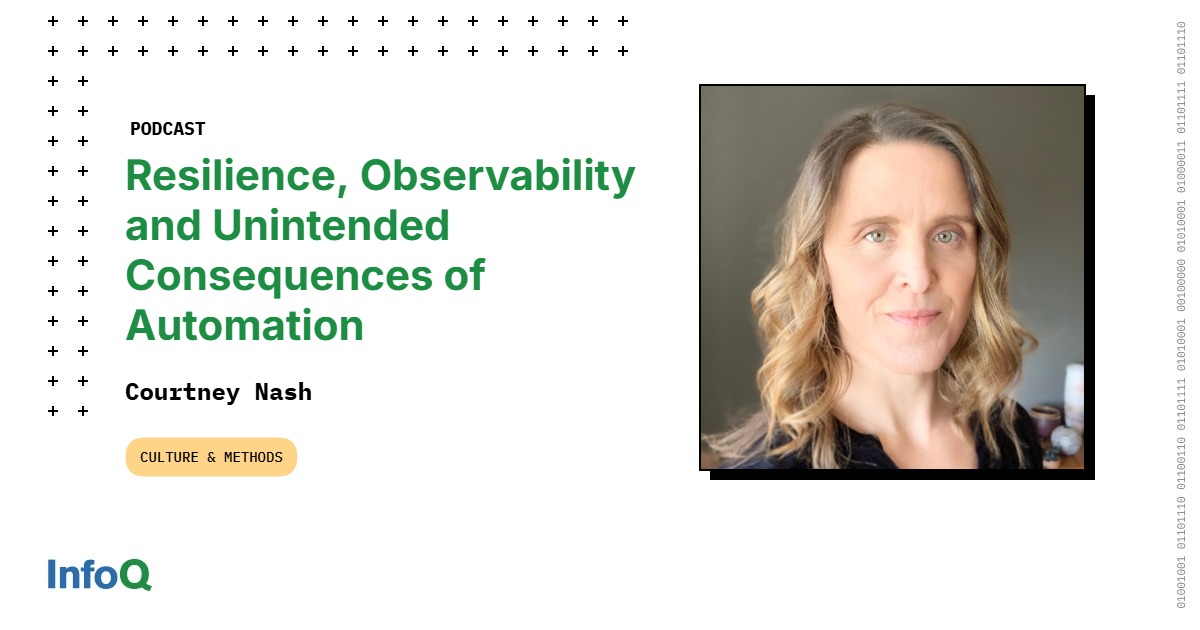
Transcript
Shane Hastie: Good day folks. This is Shane Hastie for the InfoQ Engineering Culture Podcast. Today I’m sitting down with Courtney Nash. Courtney, welcome. Thanks for taking the time to talk to us.
Courtney Nash: Hi Shane. Thanks so much for having me. I am an abashed lover of podcasts, and so I’m also very excited to get the chance to finally be on yours.
Shane Hastie: Thank you so much. My normal starting point with these conversations is who’s Courtney?
Introductions [00:56]
Courtney Nash: Fair question. I have been in the industry for a long time in various different roles. My most known, to some people, stint was as an editor for O’Reilly Media for almost 10 years. I chaired the Velocity Conference and that sent me down the path that I would say I’m currently on, early days of DevOps and that whole development in the industry, which turned into SRE. I was managing the team of editors, one of whom was smart enough to see the writing on the wall that maybe there should be an SRE book or three or four out there. And through that time at O’Reilly, I focused a lot on what you focus on, actually, on people and systems and culture.
I have a background in cognitive neuroscience, in cognitive science and human factors studies. And that collided with all of the technology and DevOps work when I met John Allspaw and a few other folks who are now really leading the charge on trying to bring concepts around learning from incidents and resilience engineering to our industry.
And so the tail end of that journey for me ended up working at a startup where I was researching software failures, really, for a company that was focusing on products around Kubernetes and Kafka, because they always work as intended. And along the way I started looking at public incident reports and collecting those and reading those. And then at some point I turned around and realized I had thousands and thousands of these things in a very shoddy ad hoc database that I still to this day maintain by myself, possibly questionable. But that turned into what’s called The VOID, which has been the bulk of my work for the last four or five years. And that’s a large database of public incident reports.
Just recently we’ve had some pretty notable ones that folks may have paid attention to. Things like when Facebook went down in 2021 and they couldn’t get into their data center. Ideally companies write up these software failure reports, software incident reports, and I’ve been scooping those up into a database and essentially doing research on that for the past few years and trying to bring a data-driven perspective to our beliefs and practices around incident response and incident analysis. That’s the VOID. And most recently just produced some work that I spoke at QCon about, which is how we all got connected, on what I found about how automation is involved in software incidents from the database that we have available to us in The VOID.
Shane Hastie: Can we dig into that? The title of your talk was exploring the Unintended Consequences of Automation in Software. What are some of those and where do they come from?
Research into unintended consequences [03:43]
Courtney Nash: Yes. I’m going to flip your question and talk about where they come from and then talk about what some of them are. A really common through line for my work and other people in this space, resilience engineering, learning from incidents, is that we’re really not the first to look at some of this through this lens. There’s been a lot of researchers and technologists, but looking at incidents in other domains, critically safety critical domains, so things like aviation, healthcare, power plants, power grids, that type of thing. A lot of this came out of Three Mile Island.
I would say the modern discipline that we know of now as resilience engineering married with other ones that have been around even longer like human factors research and that type of thing really started looking at systems level views of incidents. In this case pretty significant accidents like threatening the life and wellbeing of humans.
There were a lot of high consequence, high tempo scenarios and a huge body of research already exists on that. And so what I was trying to do with a lot of the work I’m doing with The VOID is pull that information as a through line into what we’re doing. Because some of this research is really evergreen just because it’s software systems or technology there’s a lot of commonalities in what folks have already learned from these other domains.
In particular, automated cockpits, automation in aviation environments is where a lot of the inspiration for my work came from. And also, you may or may not have noticed that our industry is super excited about AI right now. And so I thought I’m not going to go fully tackle AI head on yet because I think we haven’t still learned from things that we could about automation, so I’m hoping to start back a little ways and from first principles.
Some of that research really talks about literally what I called my talk. Unintended Consequences of Automation. And some of this research in aviation and automated cockpits had found that automating these human computer environments had a lot of unexpected consequences. The people who designed those systems had these specific outcomes in mind. And we have the same set of beliefs in the work that we do in the technology industry.
Humans are good at these things and computers are good at these things so why don’t we just assign the things that humans are good at to the humans and yada yada. This comes from an older concept from the ’50s called HABA-MABA (humans-are-better-at/machines-are-better-at) from a psychologist named Paul Fitts. If anyone’s ever heard of the Fitts list, that’s where this comes from.
Adding automation changes the nature of the work [06:15]
But that’s not actually how these kinds of systems work. You can’t just divide up the work that cleanly. It’s such a tempting notion. It feels good and it feels right, and it also means, oh, we can just give the crappy work, as it were, to the computers and that’ll free us up. But the nature of these kinds of systems, these complex distributed systems, you can’t slice and dice them. That’s not how they work. And so that’s not how we work in those systems with machines, but we design our tools and our systems and our automation still from that fundamental belief.
That’s where this myth comes from and these unintended consequences. Some of the research we came across is that adding automation into these systems actually changes the nature of human work. This is really the key one. It’s not that it replaces work and we’re freed up to go off and do all of these other things, but it actually changes the nature of the work that we have to do.
And on top of that, it makes it harder for us to impact a system when it’s not doing what it’s supposed to be doing, an automated system, because we don’t actually have access to the internal machination of what’s happening. And so you could apply this logic to AI, but you could back this logic all the way up to just what is your CI/CD doing? Or when you have auto-scaling across a fleet of Kubernetes pods and it’s not doing what you think it’s doing, you don’t actually have access to what it was doing or should have been doing or why it’s now doing what it’s doing.
It actually makes the work that humans have to do harder and it changes the nature of the work that they’re doing to interact with these systems. And then just recently some really modern research from Microsoft Research in Cambridge and Carnegie Mellon really actually looked at this with AI and how it actually can degrade people’s critical thinking skills and their ability when you have AI in a system depending on how much people trust it or not.
There’s some really nice modern research that I can also add too. Some of the stuff people are like, “Oh, it came out in 1983”, and I’m like, “Yes, but it’s still actually right”. Which is what’s crazy. We see these unintended consequences in software systems just constantly. I went in to The VOID report and really just read as many as I could that looked like they had some form of automation in them. We looked for things that included self-healing or auto-scaling or auto config. There’s a lot of different things we looked for, but we found a lot of these unintended consequences where software automation either caused problems and then humans had to step in to figure that out.
The other thing, the other unintended consequence is that sometimes automation makes it even harder to solve a problem than it would’ve been were it not involved in the system. I think the Facebook one is I feel like one of the more well-known versions of that where they literally couldn’t get into their own data center. Amazon in 2021 had one like that as well for AWS where they had a resource exhaustion situation that then wouldn’t allow them to actually access the logs to figure out what was going on.
The myth comes from this separation of human and computer duties. And then the kinds of unintended consequences we see are humans having to step into an environment that they’re not familiar with to try to fix something that they don’t understand why or how it’s going wrong yet. And then sometimes that thing actually makes it harder to even do their job, all of which are the same phenomenon we saw in research in those other domains. It’s just now we’re actually being able to see it in our own software systems. That’s the very long-winded answer to your question.
Shane Hastie: If I think of our audience, the technical practitioners who are building these tools, building these automation products, what does this mean to them?
The impact on engineering [10:16]
Courtney Nash: This is a group I really like to talk to. I like to talk to the people who are building the tools, and then I like to talk to the people who think those tools are going to solve all their problems, not always the same people. A lot of people who are building these are building it for their own teams, they’re cobbling together monitoring solutions and other things and trying. It’s not even that they necessarily have some vendor product, although that is certainly increasingly a thing in this space. I was just talking to someone else about this. We have armies of user experience researchers out there, people whose job is to make sure that the consumer end of the things that these companies build work for them and are intuitive and do what they want. And we don’t really do that for our internal tools or for our developer tools.
And it is a unique skill set, I would say, to be able to do that. And a lot of times I learned recently, in another podcast, tends to fall on the shoulders of staff engineers. Who’s making sure that the internal tooling, you may be so lucky as to have a platform team or something like that. But I think I would just, in particular, the more people can be aware of that myth, the HABA-MABA Fitts list, it is, I had this belief myself about automating things and automating computers. And just to preface this, I’m not anti-automation. I’m not, don’t do it, it’s terrible. We should just go back to rocks and sticks. I’m a big fan of it in a lot of ways, but I’m a fan of it when the designers of it understand the potential for some of those unintended consequences.
And instead of thinking of replacing work that humans might make or do, it’s augmenting that work. And how do we make it easier for us to do these kinds of jobs? And that might be writing code, that might be deploying it, that might be tackling incidents when they come up, but understanding what the fancy, nerdy academic jargon for this is joint cognitive systems. But thinking instead of replacement or our functional allocation, another good nerdy academic term, we’ll give you this piece, we’ll give the humans those pieces.
How do we have a joint system where that automation is really supporting the work of the humans in this complex system? And in particular, how do you allow them to troubleshoot that, to introspect that, to actually understand and to have even maybe the very nerdy versions of this research lay out possible ways of thinking about what can these computers do to help us? How can we help them help us? What does that joint cognitive system really look like?
And the bottom line answer is it’s more work for the designers of the automation, and that’s not always something you have the time or the luxury for. But if you can step out of the box of I’m just going to replace work you do, knowing that’s not really how it works, to how can these tools augment what our people are doing? That’s what I think is important for those people.
And the next question people always ask me is, “Cool who’s doing it?” And I answer up until recently was like, “Nobody”. Record scratch. I wish. However, I have seen some work from Honeycomb, which is an observability tooling vendor that is very much along these lines. And so I’m not paid by Honeycomb, I’m not employed by Honeycomb or staff. This is me as an independent third party finally seeing this in the wild. And I don’t know what that’s going to look like. I don’t know how that’s going to play out, but I’m watching a company that makes tooling for engineers think about this and think about how do we do this? And so that gives me hope and I hope it also empowers other people to be, oh, Courtney is not just spouting off all this academic nonsense, but it’s possible. It’s just definitely a very different way of approaching especially developer or SRE types of tooling.
Shane Hastie: My mind went to observability when you were describing that.
Courtney Nash: Yes.
Shane Hastie: What does it look like in practice? If I am one of those SREs in the organization, what do I do given an incident’s likely to happen, something’s going to go wrong? Is it just add in more logs and observability or what is it?
Practical application [14:40]
Courtney Nash: Yes and no. I think of course it’s always very annoyingly bespoke and contextually specific to a given organization and a given incident. But this is why the learning from incidents community is so entwined with all of this because if instead of looking for just technical action item fixes out of your incidents, you’re looking at what did we learn about why people made the decisions they made at the time. Another nerdy research concept called local rationality, but if you go back and look at these incidents from the perspective of trying to learn from the incident, not just about what technically happened, but what happened socio-technically with your teams, were there pressures from other parts of the organization?
All of these things, I would say SREs investing in learning from incidents are going to figure out A, how to better support those people when things go wrong. It’s like, what couldn’t we get access to or what information didn’t we have at the time? What made it harder to solve this problem? But also, what did people do when that happened that made things work better? And did they work around tools? What was that? What didn’t they know? What couldn’t they know that could our tooling tell them, perhaps?
And so that’s why I think you see so many learning from incident people and so many resilience engineering people all talking around this topic because I can’t just come to you and say, “You should do X”, because I have no idea how your team’s structured, what the economic and temporal pressures are on that team. The local context is so important and the people who build those systems and the people who then have to manage them when they go wrong are going to be able to figure out what the systemic things going on are, and especially if it’s lack of access to what X, Y, or Z was doing. Going back, looking at what made it hard for people and also what natural adaptations they themselves took on to make it work or to solve the problem.
And again, it’s like product management and it’s like user experience. You’re not going to just silver bullet this problem. You’re going to be fine-tuning and figuring out what it is that can give you that either control or visibility or what have you. There is no product out there that does that for you. Sorry, product people. That’s the reason investing in learning from their incidents is going to help them the most I would biasedly offer.
Shane Hastie: We’re talking in the realm of socio-technical systems. Where does the socio come in? What are the human elements here?
The human aspects [17:14]
Courtney Nash: Well, we built these systems. Let’s just start with that. And the same premise of designing automation, we design all kinds of things for all kinds of outcomes and aren’t prepared for all of the unexpected outcomes. I think that the human element, for me, in this particular context, software is built by people, software is maintained by people. The through line from all of this other research I’ve brought up is that if you want to have a resilient or a reliable organization, the people are the source of that. You can’t engineer five nines, you can’t slap reliability on stuff. It is people who make our systems work on the day-to-day basis. And we are, I would argue, actively as an industry working against that truth right now.
For me, there’s a lot of socio in complex systems, but for me, that’s the nut of it. That’s the really crux of the situation is we are largely either unaware or unwilling to look at close at how important people are to keep things running and building and moving in ways that if you take these ironies or unexpected consequences of automation and scale those up in the way that we are currently looking at in terms of AI, we have a real problem with, I believe, the maintainability, the reliability, the resilience of our systems.
And it won’t be apparent immediately. It won’t be, oh shoot, that was bad. We’ll just roll that back. That’s not the case. And I’m seeing this talking to people about interviewing junior engineers. There is a base of knowledge that humans have that is built up from direct contact with these systems that automated systems can’t have yet. It’s certainly not in the world we live in despite all the hype we might be told. I am most worried about the erosion of expertise in these complex systems. For me, that’s the most important part of the socio part of the social technical system other than how we treat people. And those are also related, I’d argue.
Shane Hastie: If I’m a technical leader in an organization, what do I do? How do I make sure we don’t fall into that trap?
Listen to your people [19:36]
Courtney Nash: Listen to your people. You’re going to have an immense amount of pressure to bring AI into your systems. Some of it is very real and warranted and you’re not going to be able to ignore it. You’re not going to be able to put a lid on it and set it aside. Faced with probably a lot of pressure to bring AI and bring more automation, those types of things, I think the most important thing for leaders to do is listen to the people who are using those tools, who are being asked to bring those into their work and their workflow. Also find the people who seem to be wizards at it already. Why are some people really good at this? And tap into that. Try to figure out where those sources of expertise and knowledge with these new ways of doing are coming from.
And again, I ask people all the time, if you have a product company, let’s say you work at a company that produces something. You work for big distributed systems companies, but they’re still like Netflix or Apple or whatever, “Do you A/B test stuff before you release it? Why don’t you do that with new stuff on your engineering side?” Think about how much planning and effort goes into a migration or moving from one technology to another.
We could go monolith to microservices, we could go pick your digital transformation. How long did that take you? And how much care did you put into that? Maybe some of it was too long or too bureaucratic or what have you, but I would argue that we tend to YOLO internal developer technology way faster and way looser than we do with the things that actually make us money as that is the perception, the things that actually make us money.
And the more that leaders of technical teams can listen to their people, roll things out in a way that allows you to, how are you going to decide what success looks like? Integrating AI tools into your team, for example, what does that look like? Could you lay down some ground rules for what that looks like? And if you’re not doing that in two months or three months or four months, what do your people think you should be doing? I feel like it’s the same age-old argument about developer experience, but I think the stakes are a little higher because we’re rushing so fast into this.
Technical leaders, listen to your people, use the same tactics you use for rolling out lots of high stakes, high consequences things, and don’t just hope it works. Have some ground rules for what that should look like and be willing to reevaluate that and rethink how you should approach it. But I’m not a technical leader, so they might balk at that advice. And I understand that.
Shane Hastie: If I can swing back to The VOID, to this repository that you’ve built up over years. You identified some of the unintended consequences of automation as something that’s coming up. Are there other trends that you can see or point us towards that you’ve seen in that data?
Trends from the VOID data [22:31]
Courtney Nash: Some of the earliest work I did was really trying to myth-bust some things that I thought I had always had a hunch were not helping us and were hurting us as an industry, but I didn’t have the data for it. The canonical one is MTTR. I wouldn’t call this a trend, except in that everybody’s doing it. But using the data we have in The VOID to show that things like duration or severity of incidents are extremely volatile, not terribly statistically reliable. And so trying to help give teams ammunition against these ideas that I think are actually harmful, they can actually have pretty gnarly consequences in terms of the way that metrics are assigned to team performance, incentivization of really weird behaviors and things that I think just on the whole aren’t helping people manage very complex high stakes environments.
I’ve long thought that MTTR was problematic, but once I got my hands on the data, and I have a strong background in statistics, I was able to demonstrate that it’s not really a very useful metric. It’s still though widely used in the industry. I would say it’s an uphill battle that I have definitely not, I don’t even want to say won, because I don’t see it that way, but I do believe that we have some really unique data to counteract a lot of these common beliefs and things like severity actually is not correlated with duration.
There’s a lot of arguments on teams about how should we assign severity, what does severity need to be? And again, these Goddard’s law things and things like the second you make it a metric, it becomes a target, and then all these perverse behaviors come out of that. Those are some of the past things that we’ve done.
I would say the one trend that I haven’t chased yet, or that I don’t have the data for in any way yet is I really do think that companies that invest in learning from their incidents have some form of a competitive advantage.
Again, this is a huge hunch. It’s a lot, I think, where Dr. Nicole Forsgren was in the early days of DevOps and the DORA stuff where they were like, we have these theories about organizational performance and developer efficiency and performance and stuff, and they collected a huge amount of data over time towards those theories. I really do believe that there is a competitive advantage to organizations that invest in learning from their incidents because it gets at all these things that we’ve been talking about. But like I said, if you want to talk trends, I think that’s one, but I don’t have the data for it yet.
Shane Hastie: You’re telling me a lot of really powerful interesting stuff here. If people want to continue the conversation, where do they find you?
Courtney Nash: Thevoid.community, which is quite possibly the weirdest URL, but domain names are hard these days. That is the easiest way to find all of my past research. There is links to a podcast and a newsletter there. I’m also on all the social things, obviously, and speaking at a few events this year. And just generally that’s the best spot. I post a lot on LinkedIn, I will say, and I’m surprised by that. I didn’t use to be much of a LinkedIn person, but I’ve actually found that the community that are discussing these topics is very lively. If you’re looking for any current commentary, I would actually say that strangely, I can’t believe I’m saying this, but The VOID on LinkedIn is probably the best place to find us.
Shane Hastie: You also mentioned, when we were talking earlier, an online community for resilience engineering. Tell us a little bit about that.
Courtney Nash: There’ve been a few fits and starts to try to make this happen within the tech industry. There is a Resilience Engineering Association. Again, the notion of resilience engineering long precedes us as technology and software folks. That organization exists, but recently a group of folks have put together a Resilience in Software Foundation and there’s a Slack group that’s associated with that.
There’s a few things that are emerging specific to our industry, which I really appreciate because sometimes it is really hard to go read all this other wonky research and then you’ve asked these questions even just today in this podcast, okay, but me as an SRE manager, what does that mean for me? There’s definitely some community starting to build around that and resilience in software, which The VOID has been involved with as well. And I think it’s going to be a great resource for the tech community.
Shane Hastie: Thank you so much.
Mentioned:
.
From this page you also have access to our recorded show notes. They all have clickable links that will take you directly to that part of the audio.
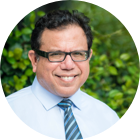
MMS • Pierre Pureur Kurt Bittner
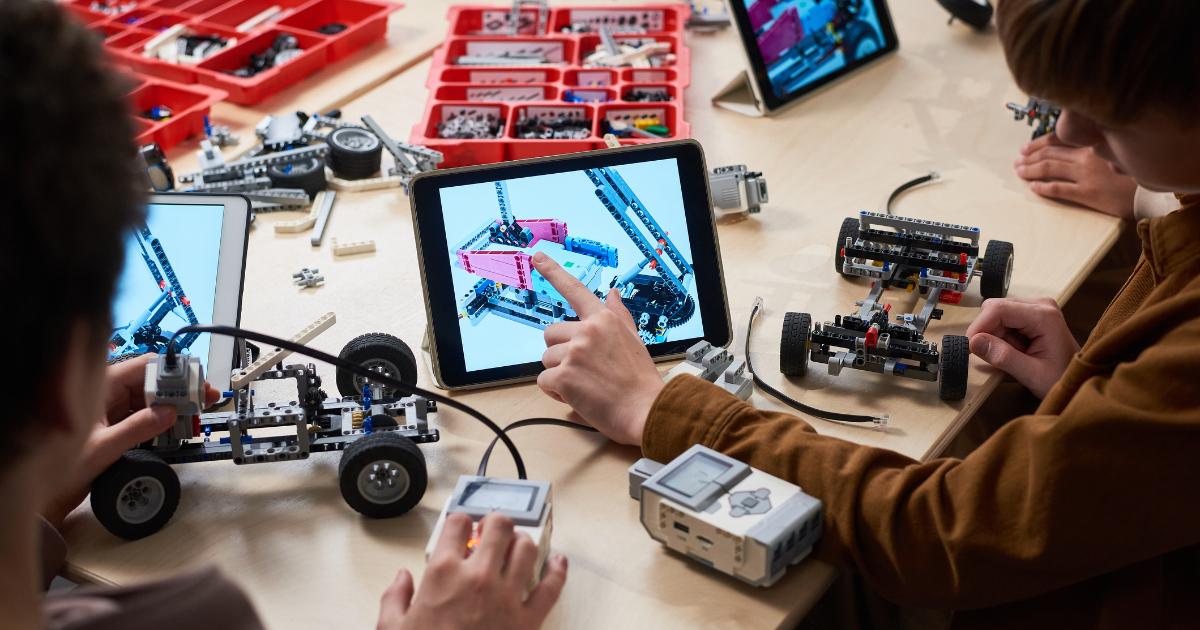
Key Takeaways
- Selling yourself and your stakeholders on doing architectural experiments is hard, despite the significant benefits of this approach; you like to think that your decisions are good but when it comes to architecture, you don’t know what you don’t know.
- Stakeholders don’t like to spend money on things they see as superfluous, and they usually see running experiments as simply “playing around”. You have to show them that experimentation saves money in the long run by making better-informed decisions.
- These better decisions also reduce the overall amount of work you need to do by reducing costly rework.
- You may think that you are already experimenting by doing Proofs of Concept (POCs). Architectural experiments and POCs have different purposes. A POC helps validate that a business opportunity is worth pursuing, while an architectural experiment tests some parts of the solution to validate that it will support business goals.
- Sometimes, architectural experiments need to be run in the customer’s environment because there is no way to simulate real-world conditions. This sounds frightening, but techniques can be used to roll back the experiments quickly if they start to go badly.
As we stated in a previous article, being wrong is sometimes inevitable in software architecting; if you are never wrong, you are not challenging yourself enough, and you are not learning. The essential thing is to test our decisions as much as possible with experiments that challenge our assumptions and to construct the system in such a way that when our decisions are incorrect the system does not fail catastrophically.
Architectural experimentation sounds like a great idea, yet it does not seem to be used very frequently. In this article, we will explore some of the reasons why teams don’t use this powerful tool more often, and what they can do about leveraging that tool for successful outcomes.
First, selling architectural experimentation to yourself is hard
After all, you probably already feel that you don’t have enough time to do the work you need to do, so how are you going to find time to run experiments?
You need to experiment for a simple reason: you don’t know what the solution needs to be because you don’t know what you don’t know. This is an uncomfortable feeling that no one really wants to talk about. Bringing these issues into the open stimulates healthy discussion that shape the architecture, but before you can have them you need data.
One of the forces to overcome in these discussions is confirmation bias, or the belief that you already know what the solution is. Experimentation helps you to challenge your assumptions to reach a better solution. The problem is, as the saying goes, “the truth will set you free, but first it will make you miserable”. Examples of this include:
- Experimentation may expose that solutions that have worked for you in the past may not work for the system you are working on now.
- It may expose you to the fact that some “enterprise standards” won’t work for your problem, forcing you to explain why you aren’t using them.
- It may expose that some assertions made by “experts” or important stakeholders are not true.
Let’s consider a typical situation: you have made a commitment to deliver an MVP, although the scope is usually at least a little “flexible” or “elastic”; the scope is always a compromise. But the scope is also, usually, more optimistic and you rarely have the resources to confidently achieve it. From an architectural perspective you have to make decisions, but you don’t have enough information to be completely confident in them; you are making a lot of assumptions.
You could, and usually do, hope that your architectural decisions are correct and simply focus on delivering the MVP. If you are wrong, the failure could be catastrophic. If you are willing to take this risk you may want to keep your resumé updated.
Your alternative is to take out an “insurance policy” of sorts by running experiments that will tell you whether your decisions are correct without resorting to catastrophic failure. Like an insurance policy, you will spend a small amount to protect yourself, but you will prevent a much greater loss.
Next, selling stakeholders on architectural experimentation is a challenge
As we mentioned in an earlier article, getting stakeholder buy-in for architectural decisions is important – they control the money, and if they think you’re not spending it wisely they’ll cut you off. Stakeholders are, typically, averse to having you do work they don’t think has value, so you have to sell them on why you are spending time running architectural experiments.
Architectural experimentation is important for two reasons: For functional requirements, MVPs are essential to confirm that you understand what customers really need. Architectural experiments do the same for technical decisions that support the MVP; they confirm that you understand how to satisfy the quality attribute requirements for the MVP.
Architectural experiments are also important because they help to reduce the cost of the system over time. This has two parts: you will reduce the cost of developing the system by finding better solutions, earlier, and by not going down technology paths that won’t yield the results you want. Experimentation also pays for itself by reducing the cost of maintaining the system over time by finding more robust solutions.
Ultimately running experiments is about saving money – reducing the cost of development by spending less on developing solutions that won’t work or that will cost too much to support. You can’t run experiments on every architectural decision and eliminate the cost of all unexpected changes, but you can run experiments to reduce the risk of being wrong about the most critical decisions. While stakeholders may not understand the technical aspects of your experiments, they can understand the monetary value.
Of course running experiments is not free – they take time and money away from developing things that stakeholders want. But, like an insurance policy that costs the amount of premiums but protects you from much greater losses, experiments protect you from the effects of costly mistakes.
Selling them on the need to do experiments can be especially challenging because it raises questions, in their minds anyway, about whether you know what you are doing. Aren’t you supposed to have all the answers already?
The reality is that you don’t know everything you would like to know; developing software is a field that requires lifelong learning: technology is always changing, creating new opportunities and new trade-offs in solutions. Even when technology is relatively static, the problems you are trying to solve, and therefore their solutions, are always changing as well. No one can know everything and so experimentation is essential. As a result, the value of knowledge and experience is not in knowing everything up-front but in being able to ask the right questions.
You also never have enough time or money to run architectural experiments
Every software development effort we have ever been involved in has struggled to find the time and money to deliver the full scope of the initiative, as envisioned by stakeholders. Assuming this is true for you and your teams, how can you possibly add experimentation to the mix?
The short answer is that not everything the stakeholders “want” is useful or necessary. The challenge is to find out what is useful and necessary before you spend time developing it. Investing in requirements reviews turns out not to be very useful; in many cases, the requirement sounds like a good idea until the stakeholders or customers actually see it.
This is where MVPs can help improve architectural decisions by identifying functionality that doesn’t need to be supported by the architecture, which doubly reduces work. Using MVPs to figure out work that doesn’t need to be done makes room to run experiments about both value and architecture. Identifying scope and architectural work that isn’t necessary “pays” for the experiments that help to identify the work that isn’t needed.
For example, some MVP experiments will reveal that a “must do” requirement isn’t really needed, and some architectural experiments will reveal that a complex and costly solution can be replaced with something much simpler to develop and support. Architectural decisions related to that work are also eliminated.
The same is true for architectural experiments: they may reveal that a complex solution isn’t needed because a simpler one exists, or perhaps that an anticipated problem will never occur. Those experiments reduce the work needed to deliver the solution.
Experiments sometimes reveal unanticipated scope when they uncover a new customer need, or that an anticipated architectural solution needs more work. On the whole, however, we have found that reductions in scope identified by experiments outweigh the time and money increases.
At the start of the development work, of course, you won’t have any experiments to inform your decisions. You’re going to have to take it on faith that experimentation will identify extra work to pay for those first experiments; after that, the supporting evidence will be clear.
Then you think you’re already running architectural experiments, but you’re not
You may be running POCs and believe that you are running architectural experiments. POCs can be useful but they are not the same as architectural experiments or even MVPs. In our experience, POCs are hopefully interesting demonstrations of an idea but they lack the rigor needed to test a hypothesis. MVPs and architectural experiments are intensely focused on what they are testing and how.
Some people may feel that because they run integration, system, regression, or load tests, they are running architectural experiments. Testing is important, but it comes too late to avoid over-investing based on potentially incorrect decisions. Testing usually only occurs once the solution is built, whereas experimentation occurs early to inform decisions whether the team should continue down a particular path. In addition, testing verifies the characteristics of a system but it is not designed to explicitly test hypotheses, which is a fundamental aspect of experimentation.
Finally, you can’t get the feedback you need without exposing customers to the experiments
Some conditions under which you need to evaluate your decisions can’t be simulated; only real-world conditions will expose potentially flawed assumptions. In these cases, you will need to run experiments directly with customers.
This sounds scary, and it can be, but your alternative is to make a decision and hope for the best. In this case, you are still exposing the customer to a potentially severe risk, but without the careful controls of an experiment. In some sense, people do this all the time without knowing it, when they assume that our decisions are correct without testing them, but the consequences can be catastrophic.
Experimentation allows us to be explicit about what hypothesis we are evaluating with our experiment and limits the impact of the experiment by focusing on specific evaluation criteria. Explicit experimentation helps us to devise ways to quickly abort the experiment if it starts to fail. For this, we may use techniques that support reliable, fast releases, with the ability to roll back, or techniques like A/B testing.
As an example, consider the case where you want to evaluate whether a LLM-based chatbot can reduce the cost of staffing a call center. As an experiment, you could deploy the chatbot to a subset of your customers to see if it can correctly answer their questions. If it does, call center volume should go down, but you should also evaluate customer satisfaction to make sure that they are not simply giving up in frustration and going to another competitor with better support. If the chatbot is not effective, it can be easily turned off while you evaluate your next decision.
Conclusion
In a perfect world, we wouldn’t need to experiment; we would have perfect information and all of our decisions would be correct. Unfortunately, that isn’t reality.
Experiments are paid for by reducing the cost, in money and time, of undoing bad decisions. They are an insurance policy that costs a little up-front but reduces the cost of the unforeseeable. In software architecture, the unforeseeable is usually related to unexpected behavior in a system, either because of unexpected customer behavior, including loads or volumes of transactions, but also because of interactions between different parts of the system.
Using architectural experimentation isn’t easy despite some very significant benefits. You need to sell yourself first on the idea, then sell it to your stakeholders, and neither of these is an easy sell. Running architectural experiments requires time and probably money, and both of these are usually in short supply when attempting to deliver an MVP. But in the end, experimentation leads to better outcomes overall: lower-cost systems that are more resilient and sustainable.