Month: April 2025
Presentation: LLM and Generative AI for Sensitive Data – Navigating Security, Responsibility, and Pitfalls in Highly Regulated Industries
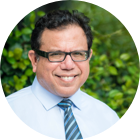
MMS • Stefania Chaplin Azhir Mahmood
Article originally posted on InfoQ. Visit InfoQ
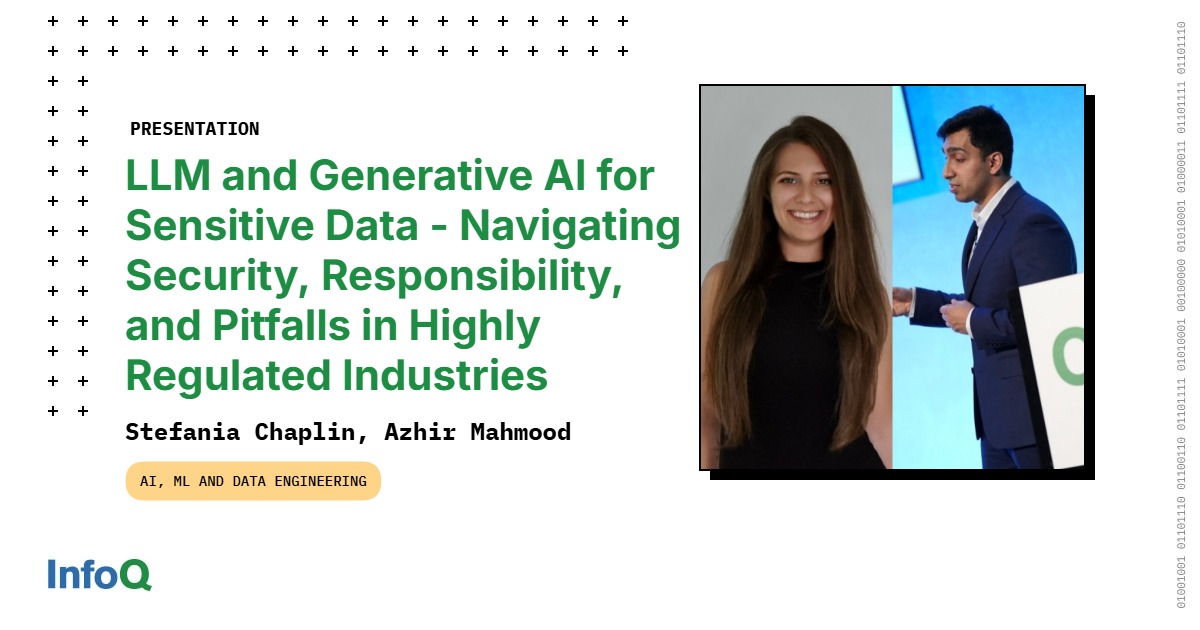
Transcript
Chaplin: Who here is responsible for AI initiatives within their organization? That might be a decision maker, influence, involved somehow? Who is already doing stuff? Has it involved executing, implementing, looking good. Who’s a little bit earlier? Maybe you still have some loopholes, you’re still designing, discovery, thinking about. We’ll try and tailor the talk accordingly.
I am Stefania Chaplin. I am a Solutions Architect at GitLab, where I work with enterprise organizations, predominantly in security, DevSecOps, but also in MLOps as well.
Mahmood: I’m Azhir Mahmood. I’m an AI Research Scientist at PhysicsX. Prior to that, I was a doctoral candidate at UCL developing cutting edge machine learning models. Prior to that, I was in the heavily regulated industry developing my own AI startup.
Chaplin: If you want to follow along with what we get up to, our website is latestgenai.com, where we publish a newsletter with the latest and greatest of everything happening, always from a secure, responsible, ethical, explainable, transparent perspective.
GenAI is Revolutionizing the World
Mahmood: GenAI is revolutionizing the world. It’s doing everything, from hurricane prediction, where NVIDIA were able to forecast Hurricane Lee a week in advance. The team at Isomorphic Labs were able to use AI to predict protein structures. Actually recently, the first ever AI designed drug was developed by the Hong Kong startup, Insilico. It’s currently going through FDA trials. At PhysicsX, we use AI for industrial design and optimization, really accelerating the speed at which we can invent new things, but understanding how AI is really transforming the world of engineering and science. How is it changing the world of business? Allen & Overy, they introduced ChatGPT 4 to 3000 of their lawyers.
Since then, with Microsoft, they developed contract matrix and are using it for drafting, reviewing, and analyzing legal documents. They’ve now launched it to their clients. At McKinsey, Lilli is able to condense all of McKinsey’s knowledge, about 100,000 documents, and allow it to be called within mere moments. That’s really reduced the amount of time associates are spending preparing for client meetings by about 20%. It’s really unlocking a whole new area of creativity for them. Bain & Company, within their recent M&A report, they found about 50% of M&A companies are leveraging AI, especially within the early stages. They’re doing everything from sourcing, screening, and due diligence. Now, having seen how AI can really revolutionize your business?
What Are Highly Regulated Industries, and Types of Sensitive Data?
Chaplin: What are highly regulated industries and types of sensitive data? These are industries that have government regulations, compliance. They have standards and laws, and these are in place for consumer protection, public safety, fair competition, and also environmental conservation. Some examples of the industries. Who here is in finance? Medical or pharmaceutical? Defense, government, and utilities? Anything I didn’t say, anyone missing? I think I’ve got everyone covered. In terms of types of sensitive data, and the important thing when it comes to machine learning and AI, you are only as good as your data, so if you have bad data, how are you going to train your models? Especially when it comes to sensitive data, there are many types, and you’re going to have to treat them differently. For example, maybe you need to start obfuscating or blanking out credit card details. You need to think about your data storage, how you’re going to store it.
Also, types, so things like PII, name, email, mobile, biometrics, religion, government, ideologies, sexual orientation. There’s a lot of different types of PII out there. From an organizational perspective for business, a lot of companies, especially larger ones, highly regulated, are listed. Any information that could influence the stock price will be counted as sensitive data, because if that is leaked or used nefariously, it will have issues. Within health, hopefully you will have come across the HIPAA regulation, which is one of the stricter ones out there in terms of medical records and what to do. Also, high risk. I work with a lot of defense organizations in my experience at GitLab, and it’s very much, we have a low-risk data, medium, classified. We have our diodes for getting the data around. If you’re going to be using sensitive data as part of your machine learning models, you really need to pay attention to making sure it’s safe, secure, and robust.
The AI Legislation Landscape
The legislation landscape, and this has been evolving over the last five, six years. I’m starting off with GDPR. GDPR, if you were working in a customer facing role, it was everything everyone could talk about around 2016, 2017. What are we going to do, personal data? How are we storing it? Is it transferring to our U.S. headquarters? It was one of the first legislations that focused on data. Like I said, good data, good models, good AI. In 2021 we had the AU AI Act proposal. If you’re watching this space, you may have noticed this just got passed into law 2024. This was very much around different risk classifications, so low risk, critical risk. It’s the first time we’re really talking about transparency in legislation. 2023, we had a few things happening. The first one was in D.C. We had the Stop Discrimination Algorithmic Act. Which then evolved to become Algorithmic Accountability Act, which was federal, so across the whole U.S., because what usually starts in D.C. spreads across the U.S, and the world.
With this one, it was very much, we need to understand what AI we’re using, and what are the implications, and what is happening? You see less of the, “It’s a black box. You just put in someone’s name, and it tells you if it’s alone.” No, we really need the transparency and accountability. UK, they’ve taken a bit of a different approach, where it’s very much about the principles. For example, transparency, explainability, responsibility. We’ll be talking about these a bit later. Having fairness, having a safe, secure model. It was very much more focused for innovation and how we use AI.
The final one, United Nations, so this one’s been very interesting. This one was talking about human rights. It was saying, we need to make sure, for one, cease using any AI which infringes on human rights. Also, quotes from the session included, we need to govern AI, not let AI govern us. If you see the way the legislation has evolved from just personal data, transparency, explainability, it’s now become a global human rights issue. What you can see, here we have the map of the world and the vast majority of North and South America, Europe, Asia-Pac, there are legislations either being talked about, being passed. You probably are from one of these countries. It’s also worth noting, where does your company do business? Because a bit like GDPR wasn’t in America, but if you’re an American business doing business with EU, you’re affected. It’s good to keep on top of what the latest and greatest are. I mentioned our newsletter at the beginning.
How to AI?” What is MLOps?
How to AI? What is MLOps? If I was going to ask you, what is an MLOps pipeline? How do you do AI? Who could give me a good answer? I have a little flow, a little bit like a Monopoly board, because you just keep going around. Starting off in the top left and walking around, this is where it’s the data. For example, what is your data source? Is there sensitive data? How are you ingesting that data? Like, we want to look at our financial records, our sales records for this product, or we want to predict customer churn, so we’re going to look at our customer records. That’s great. How are you ingesting or storing that data? Especially with sensitive data, you really need to think securely how you do this. What’s your data? It’s ready, validate, clean it. What are you going to do if there’s null data? What are you going to do if your data’s been manually entered and there are mistakes? How are you going to standardize and curate it? This is majority the data engineer.
What this is meant to show, this flow, is that you have a lot of different people a bit like DevSecOps, really, who are overlapping and getting this done. Now the data is done, then we get our data scientist and ML engineer, so we think about our features. What are we looking at for features, for our ML? Then we have our model. This comes back, should be the final thing on this is our business user, what problems are we solving? Is it regression? Is it classification? Is it anomaly detection? Random Cut Forest is great for that. Once you have your model, train it, validate, evaluate. Ok, we have it ready. We’ve got our data. We’ve got our machine learning. Now let’s do it at scale, so containerize, deploy, make it servable to applications. Then all starts again, because once you’ve got it working once, great, time to keep updating, keep retraining your models.
You can see there are a couple of different users involved in this. Really, when I think of MLOps, you have data, which I’ve spoken about quite a bit. You have your machine learning, something Azhir is very good at. If you only focus on those two, you get back into the problem of, it worked on my machine, which is something that a lot of people have heard from a long time ago in the DevOps world. This is why you need to bring along the best practices from software engineering. Having pipelines, pipelines that can standardize, they can automate, they can drive efficiency. You can add security scanning, pipelines as codes, so that you can do your continuous integration and continuous deployment, so CD4ML. It’s really this overlap, because if you have one data source, one model and one machine, ok great, you’re doing AI. This is very much about how you do enterprise at scale, making sure that is accessible.
When Things Go Wrong
What happens when things go wrong? These are mainly news stories from the last six months, and they cover a few things, so bias, hallucination, and going rogue. We had DPD, January 18th, and a lot of organizations are using a chatbot as part of customer service. Unfortunately for DPD, their chatbot started swearing at the customer and then telling the customer how terrible DPD is as a company. Not ideal, not the type of behavior you’re trying to generate. Bias is a really important area, especially maybe 10 years ago. I got one of my favorite birthday presents I received at the time, a book, “Weapons of Math Destruction.” This is very much talking about the black box. It’s by Cathy O’Neil. It’s great and it’s ok. How do we stop bias, if it’s trained on specific datasets? For example, with image detection, UK passport office exhibited bias based on the color of people’s skin. Hallucinations, I say, a more recent problem. This is a real problem for a few reasons.
A few weeks ago, you had AI hallucinating software dependencies. I’m a developer, and I’m like, yes, PyTorture, that sounds legit. Let me just add that. If I take my developer hat off, I put my hacker hat on, ChatGPT is telling me about a component that doesn’t exist, I know what I’m going to do. I’m going to create that component, and I’m going to put malware in it. Then everyone who uses ChatGPT for development and uses PyTorture, they will be infected. It’s not just dependencies, we can see there was hallucinating a fake embezzlement claim. What was interesting about this, this was the first case of AI being sued for libel. When I speak to organizations who are still earlier in the journey, this is where the lawyers come in and think, what if the AI does something that is suable, or injures someone? This is where the whole ethical and AI headspace comes in. In terms of security, top one, plugins.
Plugins are great, but you need to make sure you’re doing access control. I’ll be talking about this a bit later. Because one of the critical ChatGPT plugins exposed sensitive data, and it was to do with the OAuth mechanism. Who remembers Log4j from a few years ago? Log4j happened just before Christmas, and then, I think this was maybe two years later, PyTorch on Christmas Day, between Christmas Day and the 30th of December, so when not many people are around, there was a vulnerable version of PyTorch. This collected system information, including files such as /etc/passwd and /etc/hosts. Supply chain, this isn’t a machine learning specific vulnerability. This is something that affects all, and what you’ll see in the talk is it’s a lot about the basics. Finally, prompt injection. This is ML specific. What happened to Google Gemini is it enabled direct content manipulation, and it meant that you could start creating fictional accounts, and hackers could see only the prompts that only the developers could see.
Responsible, Secure, and Explainable AI
Mahmood: Now that you know essentially all the problems that can go wrong with machine learning models and generative AI, how do you avoid that? Fundamentally, you want to develop a responsible framework. Responsible AI is really about the governance and the set of principles your company develops when trying to develop machine learning models. There’s a few commonalities. A lot of people focus on human-centric design, fairness and bias, explainability and transparency. Ultimately, you should assure that your responsible AI set of principles align with your company values. What are some responsible AI principles out there? Over here, we’re spanning across Google and Accenture, and you’ll recognize a few commonalities. Say, for example, there is a focus on fairness.
Fundamentally, you don’t want your machine learning model to be treating people differently. There’s a focus on robustness. You want to ensure that the model is constantly working and the architectures and pipelines are functional. Transparency, it should be the case that you should be able to challenge the machine learning model. Every company, from Google and Accenture, seem to have overlaps here. Fundamentally, what you want to avoid is you want to avoid having a situation where you’re developing Terminator, because that doesn’t really align with human values and generally isn’t a good idea. Assuming you want Earth to continue being here.
What are some practical implementations you can use? Be human centric. Ultimately, machine learning, AI, they’re all technologies, and really the importance of it is how actual users experience that technology. It’s not useful if you develop a large language model, and essentially nobody knows how to communicate or interact with it, or it’s spewing swears at people. You want to continuously test. I recognize that the world of AI feels very new, but ultimately, it’s still software, you need to test every component. You need to test your infrastructure. You need to test pipelines. You need to test continuously during deployment. Just because a model may work doesn’t necessarily mean the way it interacts with the rest of the system will get you the result you want. There’s multiple components here.
Also, it’s important to recognize the limitations. Fundamentally, AI is all about the architecture as well as the data. Analyze your data. Identify biases of your model and your data. Make sure you’re able to communicate those biases and communicate the limitations of your system to your consumers and pretty much everyone. Finally, identify metrics. To understand the performance of your model, you need to use multiple metrics, and they give you a better idea of performance. You need to understand the tradeoffs, and thus you’re able to really leverage machine learning to its full capacity.
To summarize, develop a set of responsible AI principles that align with your company values, and consider the broader impact within society. Don’t build something like Terminator. Be human centric. Engage with the broader AI community. Ensure you’re thinking about how people are interacting with these architectures. Rigorously test and monitor every component of your system. Understand the data, the pipelines, how it all integrates into a great, harmonious system.
Now that we understand how to be responsible, how do you be secure?
Chaplin: Is anyone from security, or has worked in security? This might be music to your ears, because we’re going to cover this, and it’s going to cover a lot of the basic principles that are applied across the IT organization. Secure AI, secure and compliant development, deployment, and use of AI. First, I’m going to talk a little bit about attack vectors, popular vulnerabilities, some prevention techniques, and summarize. You can see, this is my simplified architecture where we have a hacker, we’ve got the model data, and we’ve got some hacks going on. Who’s heard of OWASP before? OWASP do a lot of things. Something they’re very famous for is the Top 10 Vulnerabilities. It started off with web, they do mobile, infrastructure as code. They do LLMs as well. This I think was released ’22, or ’23. What you’ll notice with the top 10, some are very LLM specific, for example, prompt injections, training data poisoning. Some are more generic.
In terms of denial of service, that could happen to your models, it can happen to your servers, or your laptop. Supply chain vulnerabilities, so what I mentioned earlier with PyTorch. If I have a look at those, and you have those top 10. We have our users who are actually on the outside. We have different services. We have our data, plugins, extensions, more services. What you’ll notice is there are multiple different attack vectors and multiple places these vulnerabilities can happen. For example, sensitive information disclosure pops up in many times, especially around the data area. We have excessive agency popping up again. In security, you are as strong as your weakest link. You need to make sure every link, every process between these services, users, and data, is as secure as possible, because otherwise you can see, you leave yourself open to a lot of vulnerabilities.
In terms of prevention techniques, so with security, there are a lot of, I call them security basics. For example, access control. Who can do what and how? How are you doing your privileges amongst your users? How are you deciding who can access what data, or who can change the model? Also, you’ve set that up, but what happens if something goes wrong? Monitoring is very important. It actually moved up in the OWASP Top 10. It moved from, I think, number nine to number six, because if you’re not doing logging and monitoring, if/when something goes wrong, how are you going to know? It’s very important, whether it’s for denial of service, for supply chain, for someone getting in your system, to just have logging and monitoring in place. For data specific, you need to think about validation, so sanitization, integrity.
A rule that I do, I worked in security training for a few years, and if everyone just checked that input, are you who you say you are? I know that usually training data comes from here, but are we just verifying that that is who it says it is? That would solve a lot of the different vulnerabilities. Even stuff like upgrading your components, having a suitable patch strategy in place. This is what I meant when I said it’s your security basics, access control, monitoring, patching. If you want to look at a good example of someone who’s got a really good framework, check out Google’s SAIF, Secure AI Framework. You can go online, find it. They’ve got some really useful educational material, because it’s talking about all these concepts. We’re talking about the security foundations, detection and response, defenses, controls, looking at risk and context within business processes.
To summarize, adopt security best practices for your AI and MLOps process. If you’re doing something for your DevSecOps or for your InfoSec, you can probably apply those principles to your MLOps and AI initiatives. I’ve mentioned access control, validation, supply chain verification. Number two, security almost manifesto, you are as strong as your weakest link, so make sure all your links are secure. Finally, check out OWASP and Google SAIF when designing and implementing your AI processes. You can also sign up our newsletter, and we’ll have more information.
Now we’ve talked about secure AI, let’s talk about explainable AI
Mahmood: What is explainability? Fundamentally, explainability is a set of tools that help you interpret and understand the decisions your AI model makes. That’s an overview. Why should you use explainable methods? Number one, transparency. Fundamentally, you should be able to challenge the judgment that your machine learning model is making. Then, trust. It’s great if your machine learning model is a black box, but ultimately, nobody is really going to trust a black box. You want to be able to bridge that barrier. It improves performance, because if you can understand your system, then you can identify components that are weak, and then, as a result, address them. You also are able to minimize risk. By using an XAI framework, it’s the shorthand for Explainable AI, you’re able to also comply with most regulatory and compliance frameworks. That’s a broad overview of its importance. We’re not going to go in too much detail, but here are a few. This is a broad landscape of what the XAI field of research looks like.
Fundamentally, there are model agnostic approaches which treat your machine learning model as a black box and then allow you to interpret that black box. You have your large language model. It’s not initially interpretable. You use a few techniques and you can better understand which components are working and how they’re working, at least. Then there’s model specific approaches. These are tailor-made approaches specific to your architecture. For example, with a model agnostic approach, you could have any architecture. Transformers, those are generally what large language models are. Multiple MLPs, those are quite common deep learning architectures. Then with model specific approaches, it’s specifically tailored to your machine learning architecture or even your domain.
Then, you also have both global analysis, so look at your whole model and attempt to understand it in its completion, and local analysis, which really identify maybe how individual predictions function. Within the model agnostic approach, there’s a common technique, it’s called SHAP. SHAP uses game theory and ultimately helps identify the most important features. You also have LIME. LIME takes in data and then fundamentally looks at how each prediction aligns. It’s really great at identifying for single data points. Then there’s the broader, holistic approach developed by Stanford University, it’s called HELM. It’s actually called Holistic Evaluation of Large Language Models. They look at the whole architecture and have a number of metrics that you can leverage. Then there’s BertViz. BertViz helps you identify the attention mechanism within your model. This is like a whistle-stop tour of explainable AI.
I imagine what you’re probably more interested is how organizations are using explainable AI. In the case of BlackRock, initially they had a black box. The performance of the black box was great. It’s actually pretty superb. What happened was the quants couldn’t explain the decision-making processes to stakeholders or customers, and as a result, that black box model was scrapped. It was then replaced with an XAI process, and that way the decision-making process could be understood. At J.P. Morgan, they’ve heavily invested in XAI. Actually, they have a whole AI institute dedicated to research, which they then integrate. At PhysicsX, we actually leverage domain knowledge. I, for example, may develop an architecture, I get a few results.
Then what will happen is I then communicate with the civil engineer, the mechanical engineer, really understand, does my prediction make sense? Then I leverage that expert judgment to improve my model and understand its failure points. IBM also leverage things called counterfactuals. Say, for example, they might ask a large language model, I have black hair, and it’ll provide some result. The counterfactual of I have black hair is I have red hair or I do not have black hair. That helps you better interpret your model where the data is missing. For example, PayPal and both Siemens have great white papers that really go into details about this whole field.
To quickly summarize, you can use expert judgments and domain knowledge to better interpret and understand the performance of your architecture. It’s a good idea to stay up to date with the latest in research within the field. It’s an incredibly fast-moving field. Make use of model agnostic approaches and model specific approaches. Think about your model in terms of globally, how does the whole architecture work, as well as locally, how does it perform for each individual data point? There’s actually a really great paper by Imperial, called, “Explainability for Large Language Models,” which I imagine many of you might find interesting, and that identifies approaches specific for that domain. Also, you can leverage open-source tools as well as classical techniques. For the case of large language models, there’s BertViz, HELM, LIT, and Phoenix. These are all open-source tools. You can literally go out, download today, and get a better understanding of performance of your model, as well as you can use more classical statistical techniques to understand the input data, the output data and really how things are performing.
The Future of AI
Chaplin: We’ve spoken a little bit about how GenAI is revolutionizing the world, highly regulated industries, sensitive data, regulation, when AI goes wrong. We’ve given you a responsible AI framework that covers responsibility, security, and explainability as well. Let’s talk a little bit about the future of AI. We’re going to take it from two lenses, my view, and then Azhir’s. I come from a cybersecurity background. Maybe I’m biased. How AI can strengthen cybersecurity in the future. Michael Friedrich was talking about AI automated vulnerability resolution. As a developer, vulnerability in my pipeline reflected cross-site scripting. What does that mean? What AI will do, one, it will explain the vulnerability. “That’s what it is. This is what the issue is.” Even better, we have automated resolution. Using GitLab, and there are other tools out there, we will generate a merge request with the remediation. As a developer, my workflow, it’s like, I’ve got a problem. This is what that means. This is the fix. It’s all been done for me. I even have an issue created, and all I need to do is manually just approve.
The reason we have that is because if you have a change, say you’re updating a component from version 2.1 to version 9.1, you might just want to check that with your code, because it’s probably going to introduce breaking changes. That’s why we have the final manual step. That’s from a developer perspective, that’s security. From a more ops perspective, incidence response and recovery. AI is very good at noticing anomalies, and PagerDuty are doing a good job at this, because they can identify something, “Something unexpected is happening. Let’s react quickly.” Maybe we block it. Maybe we’re going to alert someone, because the faster you react the slower the attack vector is.
An example, if anyone remembers the Equifax hack from a few years ago, it was a Struts 2 component, and it took them four months to notice that they had been hacked. You can imagine the damage. It was actually 150 million personal records, which is over double the UK, most of America. This is before GDPR. Otherwise, the fine would have been huge. They lost a third of market cap. This was to do with the attack vector. Finally, NVIDIA, so they are using GenAI as part of their phishing simulation. Using GenAI, you can generate these sandbox cybersecurity trainings, so help to get all of your users as secure as possible. I’m sure everyone’s come across social engineering. My brother-in-law is an accountant, and his whole team are terrified of opening the wrong link and accidentally setting the company on fire. GenAI can really help to speed up these phishing and cybersecurity initiatives.
Mahmood: More broadly, what does the future of AI actually look like? What we’re likely to see is increasing prevalence of large foundation models. These are models trained on immense datasets. They may be then used for numerous domains. They may be multimoded or distributed, but what they’ll be used for is everything from drug development, industrial design. They’ll touch every component of our lives. As they integrate within our lives, we expect AI to become increasingly regulated, especially as they begin to be integrated within health techs, finance, all these highly regulated domains.
Summary
When designing and implementing AI models, think responsibly. Make sure to use a responsible, secure, and explainable framework.
Chaplin: Keep an eye on the legislation and regulations to stay compliant, not only of the country you are based in, but also to your customers.
Mahmood: AI is more than just tech. It’s all about people. It’s how these architectures and models interact with the broader society, how they interact with all of us. Think more holistically.
Chaplin: If you are interested in finding out more, we work with organizations doing MLOps, doing AI design, doing a lot of things, so check out our website.
Questions and Answers
Participant 1: You’re talking about HELM as an explainability framework, but to my knowledge, it’s just an evaluation method with benchmarks on a leaderboard. Can you elaborate a bit on that?
Mahmood: Fundamentally, one way you can think about explainability is really understanding multiple benchmarks. Say, for example, with HELM, I have to read through the paper to make sure I fully interpret everything. If you’re able to understand how maybe changes in your performance, say, for example, you pretrain, you use a different dataset, and then you evaluate it, it gives you more interpretation to your model. That’s a way you can holistically interpret. That’s one way you could leverage HELM.
Participant 1: That doesn’t give you any explainability for highly regulated environments, I think. You compared it with SHAP and LIME, and there you get an explanation of your model and your inference.
Mahmood: Within explainable AI, there’s multiple ways you can think about explainability. There is the framework of interpretability where interpretability is fundamentally understanding each component. I would probably argue, yes, LIME provides you with some degree of interpretability, but explainability is much more of a sliding scale. You have where you might have a white box model, where you understand every component, where you might understand the components of your intention mechanism. While you can also use more holistic metrics to maybe put up your datasets and understand that. Those could be applied to less regulated domains, but still relatively regulated domains. You would, of course, use HELM with other explainable frameworks. You shouldn’t be relying on a single framework. You should be leveraging a wide approach of tools. HELM is one metric, and you can leverage numerous other metrics.
Participant 1: Shouldn’t you promote more the white box models, the interpretable ones, above the explainability of black box models, because LIME, SHAP are estimations of the inference. With the interpretable model, you know how they reason and what they do. I think in a highly regulated environment, it’s better to use white box models than starting with black box models and trying to open them.
Mahmood: The idea is leveraging white box models over black box models, and attempting to interpret black box models. To some degree, I agree. We could leverage white box models increasingly within regulated domains, but what you end up finding is we sacrifice a great deal of performance by leveraging a white box model. It’s not necessarily easy to integrate a white box model into a large language model, that’s still an area of research. You do lose some degree of performance.
Then, within the black box models, yes we have great degrees of performance. Fundamentally, say, for example, in medical vision, it makes more sense to use a CNN, because using a CNN, you’d be able to detect cancer more readily. Using a white box model, yes, it’s interpretable, but it might be the case it’s more likely to make mistakes. As a result, what you’d use then is a domain expert with that black box model. I would probably say it fundamentally depends. It depends on how regulated your industry is. How much performance do you need, again, fundamentally in your domain? There’s a whole host of tools out there.
See more presentations with transcripts
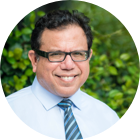
MMS • Ben Linders
Article originally posted on InfoQ. Visit InfoQ
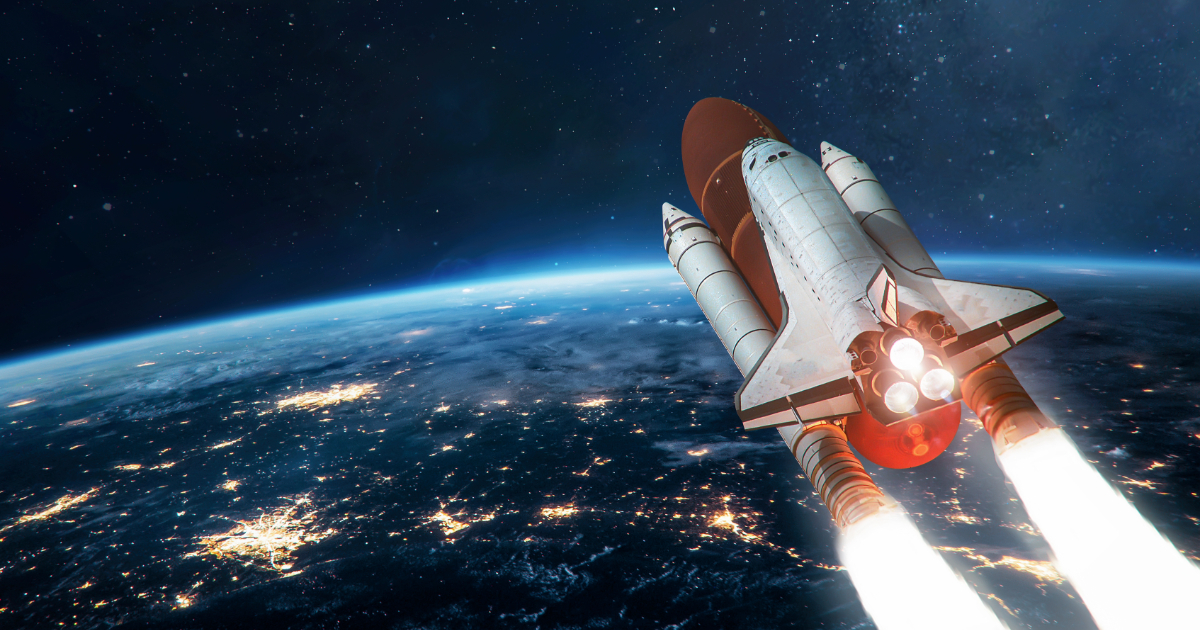
Software development is much different today than it was at the beginning of the Space Shuttle era because of the tools that we have at our disposal, Darrel Raines mentioned in his talk about embedded software development for the Space Shuttle and the Orion MPCV at NDC Tech Town. But the art and practice of software engineering has not progressed that much since the early days of software development, he added.
Compilers are much better and faster, and debuggers are now integrated into our development tools, making the task of error detection much easier, as Raines explained:
There are now dedicated analysis tools that allow us to detect certain types of issues. Examples are static code analyzers and unit test frameworks. We have configuration management systems like “git” to make our day to day work much easier.
Raines argued that many things are the same today as they were when they started writing software for the Space Shuttle. One of the best ways to detect software problems is still with a thorough code review performed by experienced software engineers, he said. Many defects will remain latent in the developed code until we hit just the right combination of factors that allow the defect to show itself. It is imperative to use all the different testing methods available to us to find bugs and defects before we fly, he added.
Raines mentioned that there is one important thing about their software that is very different than most other embedded software:
We cannot easily debug and fix software that is deployed in space! We continually remind ourselves that any testing and debugging that we do on the ground could potentially save a crew when we get to space.
He mentioned that software developers engage with astronauts at many levels during their work. They discuss requirements with astronauts, and talk about how much of a workload they want and how much they can handle. This evaluation allows them to decide on the level of autonomy that the software will have, as Raines explained:
We spend time thinking about how astronauts would recover from various faults. We determine how the harsh environment of space may affect our software in ways that we don’t even have to think about with ground computers.
The hardware used for the major programs is very often generations behind what we have on our phones and on our home computers, Raines said. The software has to be very efficient because they continually struggle with the CPU being saturated. They also run into problems with the onboard networks running out of bandwidth.
C/C++ is the most common computer language used because of its efficiency. Modern compilers help make C code relatively easy to write and debug, Raines said. Since C has been around for a long time, it is well understood and highly optimized on most platforms. There are also spacecrafts that have used Fortran (Space Shuttle flight computers) and Ada (Space Station onboard computers).
The impact of what language is used is a major factor in how to develop and test the code. C/C++ will allow you to do “dangerous” things within the code, as Raines explained:
Null pointers are a constant worry since we have to use them sometimes instead of references.
The most noticeable impact on development is that they need to perform multiple levels of testing on their code, Raines said. They start with unit tests, followed by unit integration tests, then full integration testing, and finally formal verification tests. Each level of testing tends to find different kinds of defects in the software, Raines mentioned.
The impact of failed code can sometimes be a loss of crew or a loss of mission, Raines said. This will weigh heavily on our decisions about how much testing to do and how stringently to perform those tests, he concluded,
InfoQ interviewed Darrel Raines about software development at NASA.
InfoQ: How have changes in the way software development is being developed impacted the work?
Darrel Raines: All of the tools that are available these days make it much easier to concentrate on the important task of making the code work the way we intend it to work.
The adage that the “more things change, the more they stay the same” is an important concept in my job. I am always willing to try new technology as a way of advancing my ability to develop software. But I remain skeptical that the “next big thing” will really make a big difference in my work.
What usually happens is that we make gradual changes over the years that improve our ability to do our work, but we remain consistent with the principles and techniques that have worked for us in the past.
InfoQ: What makes spacecraft software special?
Raines: One example I use with my team is this: if my computer locks up on my desktop, I can just reset the computer and start again. If we lose a computer due to a radiation upset in space, we may not be able to reestablish our current state unless we plan to have that information stored in non-volatile memory. It is a very different environment.
The astronauts, as educated and trained as they are, cannot debug our software during a flight. So we have to be as close to perfect as we can prior to launching the vehicle.
It may mean the difference between a crew coming home and losing them. This difference is what makes spacecraft software special. This is what makes it challenging.
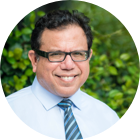
MMS • RSS
Posted on mongodb google news. Visit mongodb google news
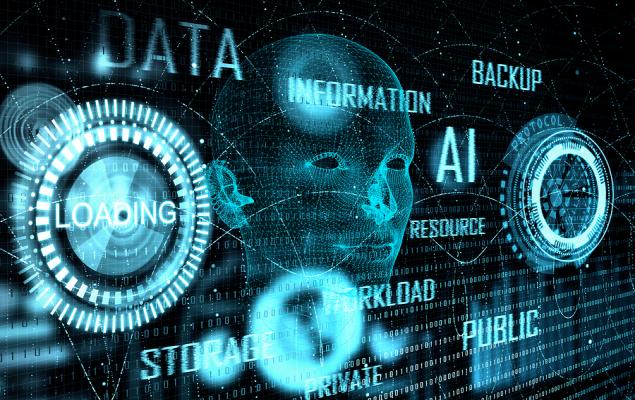
We use cookies to understand how you use our site and to improve your experience.
This includes personalizing content and advertising.
By pressing “Accept All” or closing out of this banner, you consent to the use of all cookies and similar technologies and the sharing of information they collect with third parties.
You can reject marketing cookies by pressing “Deny Optional,” but we still use essential, performance, and functional cookies.
In addition, whether you “Accept All,” Deny Optional,” click the X or otherwise continue to use the site, you accept our Privacy Policy and Terms of Service, revised from time to time.
Article originally posted on mongodb google news. Visit mongodb google news
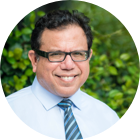
MMS • RSS
Posted on nosqlgooglealerts. Visit nosqlgooglealerts
InfluxData has released InfluxDB 3 Core and Enterprise editions in a bid to speed and simplify time series data processing.
InfluxDB 3 Core is an open source, high-speed, recent-data engine for real-time applications. According to the pitch, InfluxDB 3 Enterprise adds high availability with auto failover, multi-region durability, read replicas, enhanced security and scalability for production environments. Both products run in a single-node setup, and have a built-in Python processing engine “elevating InfluxDB from passive storage to an active intelligence engine for real-time data.” The engine brings data transformation, enrichment, and alerting directly into the database.
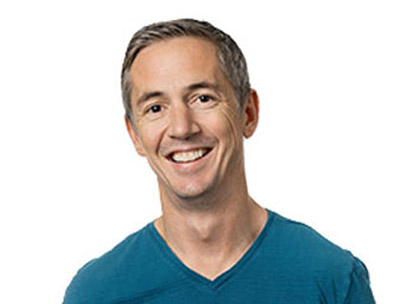
Founder and CTO Paul Dix claimed: “Time series data never stops, and managing it at scale has always come with trade-offs – performance, complexity, or cost. We rebuilt InfluxDB 3 from the ground up to remove those trade-offs. Core is open source, fast, and deploys in seconds, while Enterprise easily scales for production. Whether you’re running at the edge, in the cloud, or somewhere in between, InfluxDB 3 makes working with time series data faster, easier, and far more efficient than ever.”
A time-series database stores data, such as metrics, IoT and other sensor readings, logs, or financial ticks, indexed by time. It typically features high and continuous ingest rates, compression to reduce the space needed, old data expiration to save space as well, and fast, time-based queries looking at averages and sums over time periods. Examples include InfluxDB, Prometheus, and TimescaleDB.
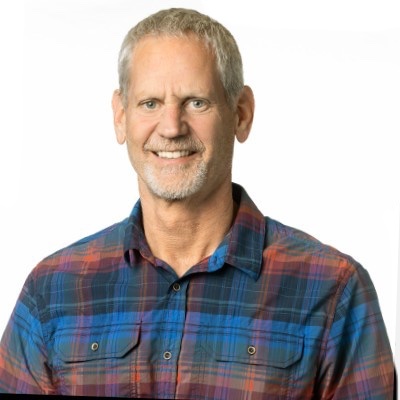
InfluxData was founded in 2012 to build an open source, distributed time-series data platform. This is InfluxDB, which is used to collect, store, and analyze all time-series data at any scale and in real-time. CEO Evan Kaplan joined in 2016. The company raised around $800,000 in a 2013 seed round followed by a 2014 $8.1 million A-round, a 2016 $16 million B-round, a 2018 $35 million C-round, a 2019 $60 million D-round, and then a 2023 $51 million E-round accompanied by $30 million in debt financing.
Kaplan has maintained a regular cadence of product and partner developments:
- January 2024 – InfluxDB achieved AWS Data and Analytics Competency status in the Data Analytics Platforms and NoSQL/New SQL categories.
- January 2024 – MAN Energy Solutions integrated InfluxDB Cloud as the core of its MAN CEON cloud platform to help achieve fuel reductions in marine and power engines through the use of real-time data.
- March 2024 – AWS announced Amazon Timestream for InfluxDB, a managed offering for AWS customers to run InfluxDB within the AWS console but without the overhead that comes with self-managing InfluxDB.
- September 2024 – New InfluxDB 3.0 product suite features to simplify time series data management at scale, with performance improvements for query concurrency, scaling, and latency. The self-managed InfluxDB Clustered, deployed on Kubernetes, went GA, and featured decoupled, independently scalable ingest and query tiers.
- February 2025 – InfluxData announced Amazon Timestream for InfluxDB Read Replicas to boost query performance, scalability, and reliability for enterprise-scale time series workloads.
The new InfluxDB 3 engine is written in Rust and built with Apache Arrow, DataFusion, Parquet, and Flight. We’re told it delivers “significant performance gains and architectural flexibility compared to previous open source versions of InfluxDB.” The engine can ingest millions of writes per second and query data in real-time with sub-10 ms lookups.
The Python engine “allows developers to transform, enrich, monitor, and alert on data as it streams in, turning the database into an active intelligence layer that processes data in motion – not just at rest – and in real-time.” This reduces if not eliminates the need for external ETL pipelines.
Both new products fit well with the existing InfluxDB 3 lineup, which is designed for large-scale, distributed workloads in dedicated cloud and Kubernetes environments and has a fully managed, multi-tenant, pay-as-you-go option.
InfluxDB 3 Core is now generally available as a free and open source download. InfluxDB 3 Enterprise is available for production deployments with flexible licensing options. Read more here.
Google’s Cybersecurity Model Sec-Gemini Enables SecOps Workflows for Root Cause and Threat Analysis
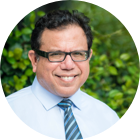
MMS • Srini Penchikala
Article originally posted on InfoQ. Visit InfoQ
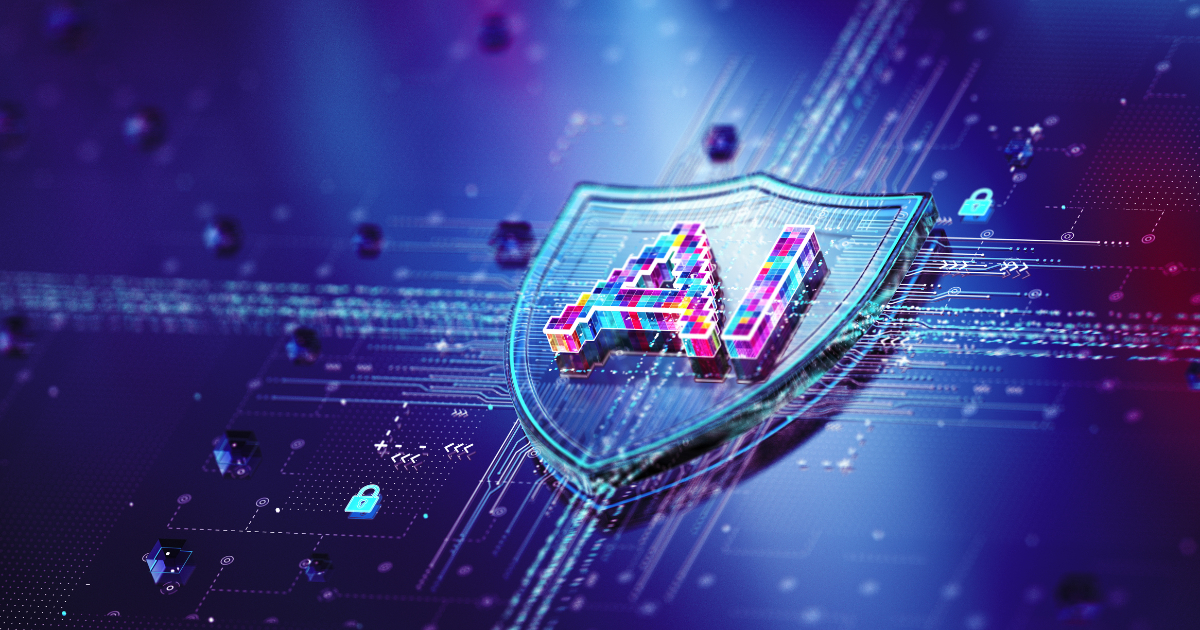
Google’s new cybersecurity model Sec-Gemini focuses on cybersecurity AI to enable SecOps workflows for root cause analysis (RCA) and threat analysis, and vulnerability impact understanding. Google Cybersecurity x AI research lead Elie Bursztein announced last week the release of Sec-Gemini v1.
Security defender teams typically face the task of securing against all cyber threats, while attackers need to successfully find and exploit only a single vulnerability. This asymmetry has made securing systems difficult, time consuming and error prone. AI-powered cybersecurity workflows can help shift the balance back to the defenders by force multiplying cybersecurity professionals. They also help security analysts understand the risk and threat profile associated with specific vulnerabilities faster.
Enabling SecOps workflows requires state-of-the-art reasoning capabilities and current cybersecurity knowledge. Sec-Gemini v1 achieves this by combining Google Gemini’s Large Language Model (LLM) capabilities with near real-time cybersecurity knowledge and tooling. This allows it to achieve a better performance when using it on cybersecurity workflows like incident root cause analysis, threat analysis, and vulnerability impact understanding.
Sec-Gemini v1 leverages various data sources including Google Threat Intelligence (GTI), Open-Source Vulnerabilities database operated by Google (OSV) and Mandiant Threat intelligence data. An example shown on the website highlights Sec-Gemini v1’s answers in response to key cybersecurity questions where the framework, using up-to-date accurate threat actor information, is able to determine that Salt Typhoon is a threat actor and provides a description of that threat actor. The output includes not only vulnerability details (based on OSV data), but also the contextualization of vulnerabilities with respect to threat actors (using Mandiant data). The framework performs well on key cybersecurity benchmarks like Cyber Threat Intelligence Multiple Choice Questions (CTI-MCQ), a leading threat intelligence benchmark and the Cybersecurity Threat Intelligence-Root Cause Mapping (CTI-RCM) benchmark.
Since last year Google has been integrating Gemini product into AI enabled security and compliance strategy programs, including AI-driven security with Google Cloud and Google Security Operations. Last year’s The State of AI and Security Survey Report published by Google Cloud and Cloud Security Alliance (CSA) highlights the AI’s potential to enhance security measures and improve threat detection and response capabilities. Several other organizations including NVIDIA and RedHat are increasingly leveraging AI technologies in cybersecurity use cases to quickly detect anomalies, threats, and vulnerabilities by processing large amounts of data in a short time. Some of these use cases include anomaly detection and AI-assisted code scanning.
It’s important to note that Sec-Gemini v1 is still an experimental cybersecurity model. The team is making it freely available to select organizations, institutions, professionals, and NGOs for research purposes. Google team provided an early access request for their Trusted Tester recruitment program but the forum is currently closed due to the team receiving a lot of requests from the community.
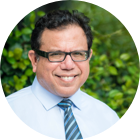
MMS • Anthony Alford
Article originally posted on InfoQ. Visit InfoQ
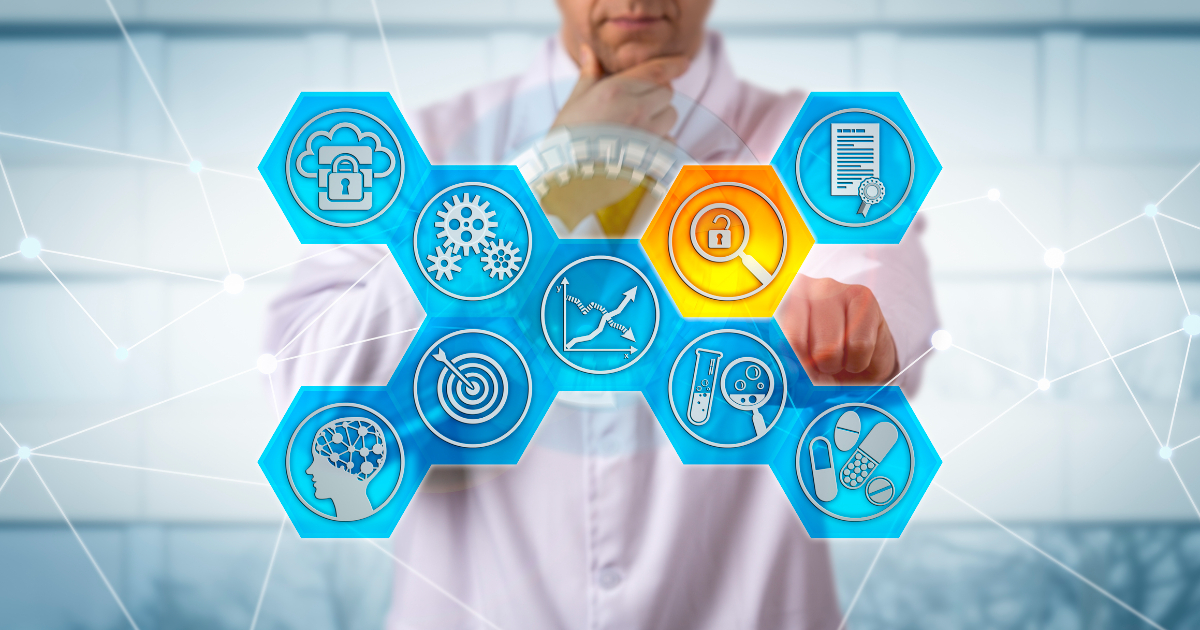
Google released the Agent2Agent (A2A) Protocol, an open-source specification for building AI agents that can connect with other agents that support the protocol. Google has enlisted over 50 technology partners to contribute to A2A’s development.
Google announced the release at the recent Google Cloud Next conference. A2A is billed as a “complement” to Anthropic’s Model Context Protocol (MCP) and defines a client-server relationship between AI agents. Google developed the protocol with help from partners like Salesforce, Atlassian, and LangChain, with the goal of creating an interoperability standard for any agent, regardless of vendor or framework. According to Google,
A2A has the potential to unlock a new era of agent interoperability, fostering innovation and creating more powerful and versatile agentic systems. We believe that this protocol will pave the way for a future where agents can seamlessly collaborate to solve complex problems and enhance our lives. We’re committed to building the protocol in collaboration with our partners and the community in the open. We’re releasing the protocol as open source and setting up clear pathways for contribution.
InfoQ covered Anthropic’s MCP release last year. Intended to solve the “MxN” problem—the combinatorial difficulty of integrating M different LLMs with N different tools—MCP defines a client-server architecture and a standard protocol that LLM vendors and tool builders can follow.
Google’s documentation points out that A2A solves a different problem than MCP does: it “allows agents to communicate as agents (or as users) instead of as tools.” The difference between a tool and an agent is that tools have structured I/O and behavior, while agents are autonomous and can solve new tasks using reasoning. In Google’s vision, an agentic application requires both tools and agents. However, A2A docs do recommend that “applications model A2A agents as MCP resources.”
A2A defines three types of actor: remote agents, which are “blackbox” agents on an A2A server; clients that request action from remote servers; and users (human users or services) that want to accomplish tasks using an agentic system. Like MCP, A2A uses JSON-RPC over HTTP for communication between clients and remote agents. The core abstraction used in the communication spec between agents is the task, which is created by a client and fulfilled by a remote agent.
In a Hacker News discussion, several users compared A2A to MCP; some were not sure what value A2A proved over MCP, while others saw it as a “superset” of MCP and praised its “clear documentation and explanation” compared to MCP. User TS_Posts claimed to be working on A2A and wrote:
[T]he current specification and samples are early. We are working on many more advanced examples and official SDKs and client/servers. We’re working with partners, other Google teams, and framework providers to turn this into a stable standard. We’re doing it in the open – so there are things that are missing because (a) it’s early and (b) we want partners and the community to bring features to the table. tldr – this is NOT done. We want your feedback and sincerely appreciate it!
The A2A source code is available on GitHub. Google also released a demo video showing collaboration between agents from different frameworks.
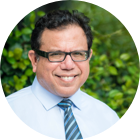
MMS • RSS
Posted on mongodb google news. Visit mongodb google news
Mizuho analyst Siti Panigrahi has revised the price target for MongoDB (MDB, Financial), reducing it from $250 to $190 while maintaining a Neutral rating on the stock. This adjustment is part of a broader assessment ahead of the first-quarter results in the software sector, where price targets have been cut due to a recent compression in software multiples.
Despite the reduction, Mizuho views the recent downturn in the software market as offering an “attractive buying opportunity” for investors. While Mizuho anticipates strong performance reports for the first quarter, it also notes that company management might exhibit caution regarding expectations for the fiscal year.
The firm asserts that ongoing tariff issues are not expected to disrupt the core fundamentals of the software-as-a-service industry, indicating a resilient outlook for the sector despite external challenges.
Wall Street Analysts Forecast
Based on the one-year price targets offered by 34 analysts, the average target price for MongoDB Inc (MDB, Financial) is $294.39 with a high estimate of $520.00 and a low estimate of $180.00. The average target implies an
upside of 86.27%
from the current price of $158.04. More detailed estimate data can be found on the MongoDB Inc (MDB) Forecast page.
Based on the consensus recommendation from 38 brokerage firms, MongoDB Inc’s (MDB, Financial) average brokerage recommendation is currently 2.0, indicating “Outperform” status. The rating scale ranges from 1 to 5, where 1 signifies Strong Buy, and 5 denotes Sell.
Based on GuruFocus estimates, the estimated GF Value for MongoDB Inc (MDB, Financial) in one year is $433.07, suggesting a
upside
of 174.03% from the current price of $158.04. GF Value is GuruFocus’ estimate of the fair value that the stock should be traded at. It is calculated based on the historical multiples the stock has traded at previously, as well as past business growth and the future estimates of the business’ performance. More detailed data can be found on the MongoDB Inc (MDB) Summary page.
Article originally posted on mongodb google news. Visit mongodb google news
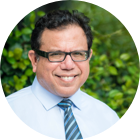
MMS • RSS
Posted on mongodb google news. Visit mongodb google news
Bank of Nova Scotia lessened its position in shares of MongoDB, Inc. (NASDAQ:MDB – Free Report) by 100.0% during the fourth quarter, according to the company in its most recent 13F filing with the Securities & Exchange Commission. The fund owned 11,640 shares of the company’s stock after selling 26,995,360 shares during the period. Bank of Nova Scotia’s holdings in MongoDB were worth $2,708,000 as of its most recent SEC filing.
Other hedge funds also recently made changes to their positions in the company. Hilltop National Bank lifted its holdings in MongoDB by 47.2% in the fourth quarter. Hilltop National Bank now owns 131 shares of the company’s stock worth $30,000 after acquiring an additional 42 shares during the last quarter. NCP Inc. acquired a new stake in shares of MongoDB during the 4th quarter worth approximately $35,000. Wilmington Savings Fund Society FSB bought a new stake in shares of MongoDB in the 3rd quarter worth approximately $44,000. Versant Capital Management Inc boosted its position in MongoDB by 1,100.0% during the 4th quarter. Versant Capital Management Inc now owns 180 shares of the company’s stock valued at $42,000 after purchasing an additional 165 shares during the period. Finally, Morse Asset Management Inc bought a new position in MongoDB during the 3rd quarter worth $81,000. Institutional investors and hedge funds own 89.29% of the company’s stock.
Analyst Ratings Changes
MDB has been the subject of several analyst reports. Cantor Fitzgerald assumed coverage on shares of MongoDB in a report on Wednesday, March 5th. They set an “overweight” rating and a $344.00 price target for the company. The Goldman Sachs Group reduced their target price on shares of MongoDB from $390.00 to $335.00 and set a “buy” rating for the company in a research note on Thursday, March 6th. Stifel Nicolaus cut their price target on MongoDB from $340.00 to $275.00 and set a “buy” rating on the stock in a report on Friday. Macquarie decreased their price objective on MongoDB from $300.00 to $215.00 and set a “neutral” rating for the company in a report on Friday, March 7th. Finally, KeyCorp downgraded MongoDB from a “strong-buy” rating to a “hold” rating in a research note on Wednesday, March 5th. Seven investment analysts have rated the stock with a hold rating, twenty-four have issued a buy rating and one has assigned a strong buy rating to the stock. Based on data from MarketBeat.com, the stock has a consensus rating of “Moderate Buy” and an average price target of $310.74.
Read Our Latest Report on MongoDB
Insider Activity
In related news, Director Dwight A. Merriman sold 1,045 shares of the firm’s stock in a transaction that occurred on Monday, January 13th. The shares were sold at an average price of $242.67, for a total transaction of $253,590.15. Following the completion of the sale, the director now directly owns 85,652 shares in the company, valued at approximately $20,785,170.84. This trade represents a 1.21 % decrease in their ownership of the stock. The transaction was disclosed in a document filed with the SEC, which can be accessed through the SEC website. Also, CEO Dev Ittycheria sold 18,512 shares of the company’s stock in a transaction that occurred on Wednesday, April 2nd. The shares were sold at an average price of $173.26, for a total transaction of $3,207,389.12. Following the completion of the transaction, the chief executive officer now owns 268,948 shares in the company, valued at approximately $46,597,930.48. This represents a 6.44 % decrease in their position. The disclosure for this sale can be found here. Over the last quarter, insiders have sold 58,060 shares of company stock worth $13,461,875. 3.60% of the stock is owned by corporate insiders.
MongoDB Trading Up 0.7 %
NASDAQ MDB opened at $162.24 on Friday. MongoDB, Inc. has a 1 year low of $140.78 and a 1 year high of $387.19. The stock’s 50-day moving average price is $220.46 and its two-hundred day moving average price is $256.10. The company has a market cap of $13.17 billion, a PE ratio of -59.21 and a beta of 1.49.
MongoDB (NASDAQ:MDB – Get Free Report) last issued its quarterly earnings results on Wednesday, March 5th. The company reported $0.19 EPS for the quarter, missing the consensus estimate of $0.64 by ($0.45). MongoDB had a negative net margin of 10.46% and a negative return on equity of 12.22%. The firm had revenue of $548.40 million during the quarter, compared to analysts’ expectations of $519.65 million. During the same quarter last year, the company posted $0.86 EPS. Research analysts forecast that MongoDB, Inc. will post -1.78 EPS for the current fiscal year.
MongoDB Profile
MongoDB, Inc, together with its subsidiaries, provides general purpose database platform worldwide. The company provides MongoDB Atlas, a hosted multi-cloud database-as-a-service solution; MongoDB Enterprise Advanced, a commercial database server for enterprise customers to run in the cloud, on-premises, or in a hybrid environment; and Community Server, a free-to-download version of its database, which includes the functionality that developers need to get started with MongoDB.
Read More
This instant news alert was generated by narrative science technology and financial data from MarketBeat in order to provide readers with the fastest and most accurate reporting. This story was reviewed by MarketBeat’s editorial team prior to publication. Please send any questions or comments about this story to contact@marketbeat.com.
Before you consider MongoDB, you’ll want to hear this.
MarketBeat keeps track of Wall Street’s top-rated and best performing research analysts and the stocks they recommend to their clients on a daily basis. MarketBeat has identified the five stocks that top analysts are quietly whispering to their clients to buy now before the broader market catches on… and MongoDB wasn’t on the list.
While MongoDB currently has a Moderate Buy rating among analysts, top-rated analysts believe these five stocks are better buys.
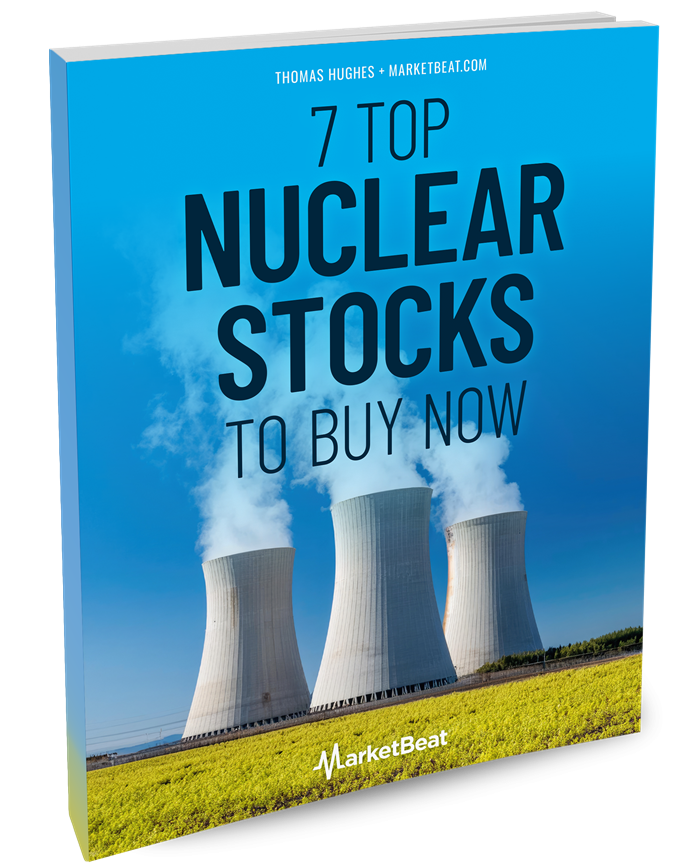
Nuclear energy stocks are roaring. It’s the hottest energy sector of the year. Cameco Corp, Paladin Energy, and BWX Technologies were all up more than 40% in 2024. The biggest market moves could still be ahead of us, and there are seven nuclear energy stocks that could rise much higher in the next several months. To unlock these tickers, enter your email address below.
Article originally posted on mongodb google news. Visit mongodb google news
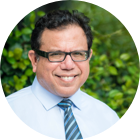
MMS • Sergio De Simone
Article originally posted on InfoQ. Visit InfoQ
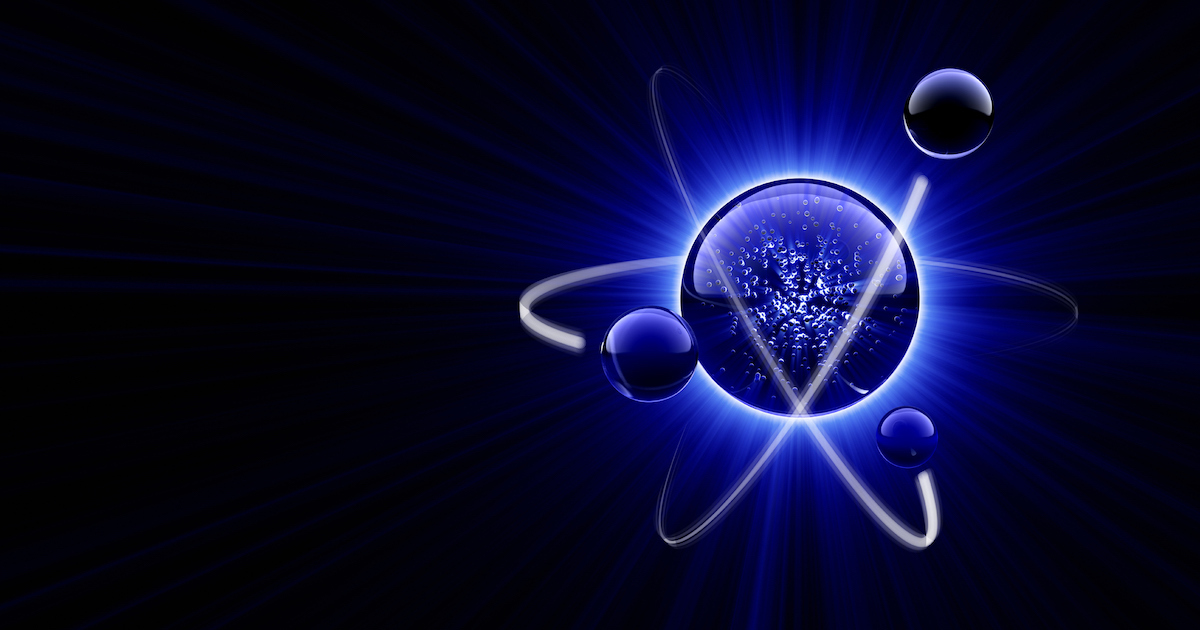
Two recent papers from Anthropic attempt to shed light on the processes that take place within a large language model, exploring how to locate interpretable concepts and link them to the computational “circuits” that translate them into language, and how to characterize crucial behaviors of Claude Haiku 3.5, including hallucinations, planning, and other key traits.
The internal mechanisms behind large language models’ capabilities remain poorly understood, making it difficult to explain or interpret the strategies they use to solve problems. These strategies are embedded in the billions of computations that underpin each word the model generates—yet they remain largely opaque, according to Anthropic. To explore this hidden layer of reasoning, Anthropic researchers have developed a novel approach they call the “AI Microscope”:
We take inspiration from the field of neuroscience, which has long studied the messy insides of thinking organisms, and try to build a kind of AI microscope that will let us identify patterns of activity and flows of information.
In very simplified terms, Anthropic’s AI microscope involves replacing the model under study with a so-called replacement model, in which the model’s neurons are replaced by sparsely-active features that can often represent interpretable concepts. For example, one such feature may fire when the model is about to generate a state capital.
Naturally, the replacement model won’t always produce the same output as the underlying model. To address this limitation, Anthropic researchers use a local replacement model for each prompt they want to study, created by incorporating error terms and fixed attention patterns into the replacement model.
[A local replacement model] produces the exact same output as the original model, but replaces as much computation as possible with features.
As a final step to describe the flow of features through the local replacement model from the initial prompt to the final output, the researchers created an attribution graph. This graph is built by pruning away all features that do not affect the output.
Keep in mind that this is a very rough overview of Anthropic’s AI microscope. For full details, refer to the original paper linked above.
Using this approach, Anthropic researches have come to a number of interesting results. Speaking of multilingual capabilities, they found evidence for some kind of universal language that Claude uses to generate concepts before translating them into a specific language.
We investigate this by asking Claude for the “opposite of small” across different languages, and find that the same core features for the concepts of smallness and oppositeness activate, and trigger a concept of largeness, which gets translated out into the language of the question.
Another interesting finding goes against the general understanding that LLMs build their output word-by-word “without much forethought”. Instead, studying how Claude generates rhymes shows it actually plans ahead.
Before starting the second line, it began “thinking” of potential on-topic words that would rhyme with “grab it”. Then, with these plans in mind, it writes a line to end with the planned word.
Anthropic researchers also dug into why the model sometimes makes up information, i.e. it hallucinates. Hallucination is, in some sense, intrinsic to how models work since they are supposed to always produce a next guess. This implies models must rely on specific anti-hallucination training to counter that tendency. In other words, there are two distinct mechanisms at play: one identifying “known entities” and another corresponding to “unknown name” or “can’t answer”. Their correct interplay is what guards models from hallucinating:
We show that such misfires can occur when Claude recognizes a name but doesn’t know anything else about that person. In cases like this, the “known entity” feature might still activate, and then suppress the default “don’t know” feature—in this case incorrectly. Once the model has decided that it needs to answer the question, it proceeds to confabulate: to generate a plausible—but unfortunately untrue—response.
Other interesting dimensions explored by Anthropic researchers concern mental math, producing a chain-of-thought explaining the reasoning to get to an answer, multi-step reasoning, and jailbreaks. You can get the full details in Anthropic’s papers.
Anthropic’s AI microscope aims to contribute to interpretability research and to eventually provide a tool that help us understand how models produce their inference and make sure they are aligned with human values. Yet, it is still an incipient effort that only goes so far as capturing a tiny fraction of the total model computation and can only be applied to small prompts with tens of words. InfoQ will continue to report on advancements in LLM interpretability as new insights emerge.
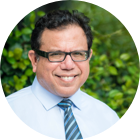
MMS • Robert Krzaczynski
Article originally posted on InfoQ. Visit InfoQ
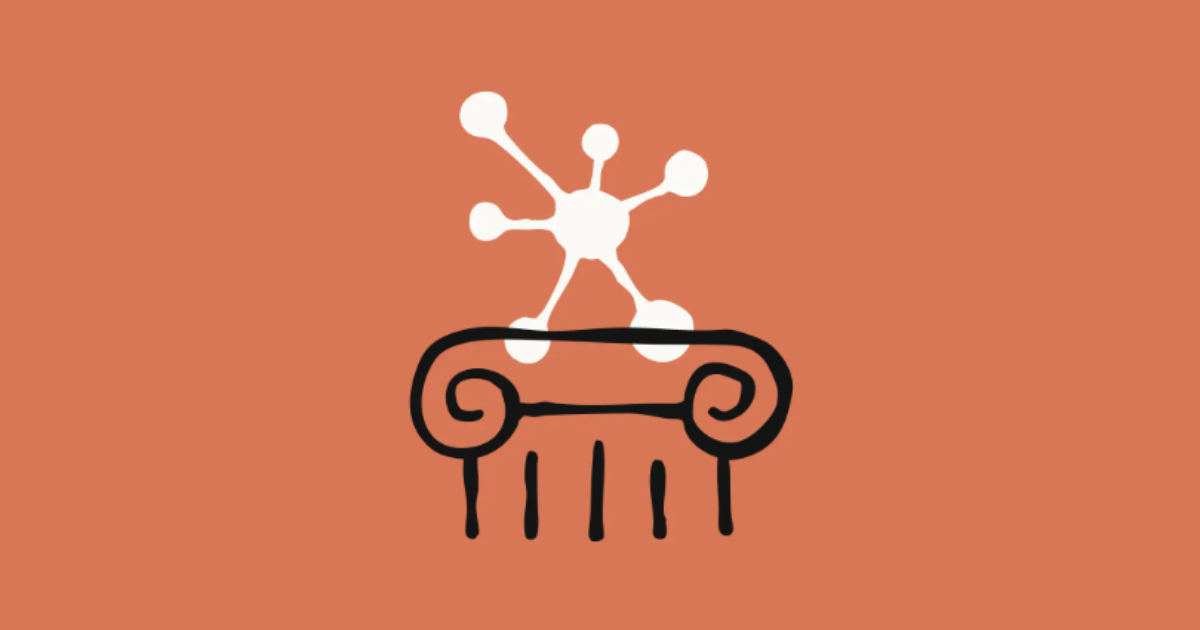
Anthropic has announced the launch of Claude for Education, a specialized version of its AI assistant, Claude, developed specifically for colleges and universities. The initiative aims to support students, faculty, and administrators with secure and responsible AI integration across academics and campus operations.
Claude for Education introduces a new Learning mode designed to promote critical thinking rather than provide direct answers. The feature encourages students to approach problems independently by engaging in Socratic dialogue and focusing on fundamental concepts. According to Anthropic, the goal is to reinforce deeper learning through guided reasoning and structured templates for tasks such as research papers or study guides.
Beyond Learning mode, the initiative also includes broader institutional offerings. Full-campus deployments are already underway at Northeastern University, London School of Economics and Political Science (LSE), and Champlain College, providing students and faculty with access to Claude across a variety of use cases—from thesis drafting and personalized essay feedback to automating administrative workflows and converting policies into FAQs.
Anthropic is also engaging in academic and industry partnerships to integrate Claude into existing educational infrastructure. It has joined Internet2, a nonprofit consortium that supports technology infrastructure for education and research and is collaborating with Instructure, the company behind the Canvas learning management system.
In addition to institutional tools, two new student programs are being launched: the Claude Campus Ambassadors initiative, where students can partner with Anthropic to drive AI-focused efforts on their campuses, and a program that provides API credits for student-led projects using Claude.
Feedback from the community has already started to emerge. Zaid Abuhouran, a U.S. diplomat working in international education and emerging tech, remarked:
I am glad to see that this mode is designed to encourage higher-level thinking and deeper understanding rather than just spitting out answers for students. I would love to see how this mode is used by educators to cultivate more meaningful learning outcomes using AI, and evidence on how it is creating more learning gains and fostering critical thinking among students as compared to traditional learning approaches.
Some questions around access have also been raised. Oxana Y. asked:
Would the AI tools availability be extended to the alumni population as well, or only for currently enrolled students?
In response, Drew Bent, who works on Education & AI at Anthropic, clarified:
At the moment just current students and faculty, but this is something we can look into. Agreed it’d be powerful for alumni!
Claude for Education and Learning mode is now available for institutions interested in exploring the use of AI in a secure, pedagogically aligned environment. Those looking to get started can register their interest through Anthropic’s website.