Category: Uncategorized
Presentation: Zero Waste, Radical Magic, and Italian Graft – Quarkus Efficiency Secrets
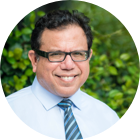
MMS • Holly Cummins
Article originally posted on InfoQ. Visit InfoQ
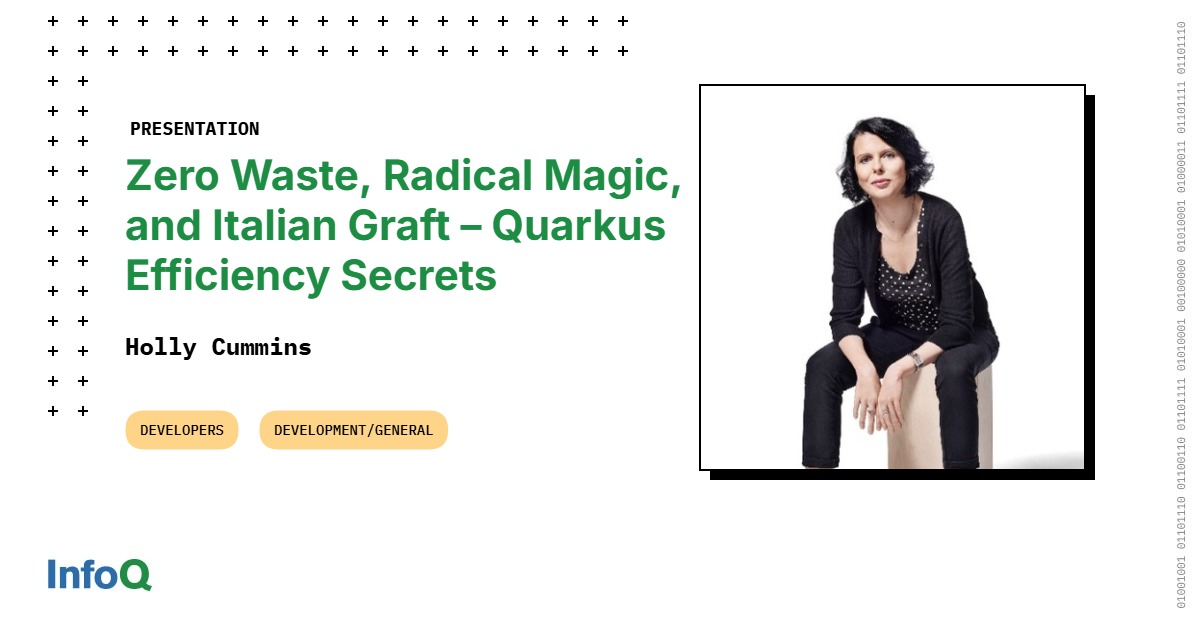
Transcript
Cummins: I’m Holly Cummins. I work for Red Hat. I’m one of the engineers who’s helping to build Quarkus. Just as a level set before I start, how many of you are Java folks? How many of you are using Quarkus? How many of you have not even heard of Quarkus? I’ve worked on Java for most of my career. I’m here to talk about Java. I want to actually start by talking a little bit about Rust. I’m not a Rust developer. I have never developed Rust. I’m not here to criticize Rust, but actually I’m going to start by criticizing Rust. Of course, Rust has so many amazing features. It’s so well engineered. It’s really a needed language. It is incredibly efficient, but Rust does have a problem.
There’s a reason I have never learned Rust, which is, Rust has a reputation for being really hard to learn, and I am lazy. This is something that you see everywhere in the community. People talk about how hard Rust is. It’s too difficult to be widely adopted. Even people who really advocate strongly for Rust will talk about how hard it is. I love the title of this article, “Why Rust is Worth the Struggle”. They start by saying, with Rust, you approach it with trepidation, because it’s got this notoriously difficult learning curve. I love this, “Rust is the hardest language up to that time I’ve met”.
When people talk about Rust, people will tell you that Rust doesn’t have garbage collection, and that’s one of the things that makes it efficient. I have some questions about that. If we start with the assumption that not having garbage collection makes a language performant, which is wrong, but if we start with that assumption, what happens if we add garbage collection to Rust? Now at this point, all of the people who are Rust developers are sort of screaming quietly in the corner, going, why would you do that? What happens if you do that? It turns out, if you do that, Rust becomes much easier to use. They added a layer of garbage collection on top of Rust, and then they had a bunch of volunteers do a coding task. The people who had the garbage collected version were more likely to complete the task, and they did it in a third of the time.
Now I think we really need to rethink the efficiency of Rust, because Rust is very efficient in terms of its computational resources. If you can make something adding garbage collection, is that really an efficient language? Rust maybe is not so efficient. There’s always this tradeoff of, you’ve got your human efficiency and your machine efficiency, and with Rust, they’ve really gone all in on the machine efficiency at the expense of human efficiency. That’s the tradeoff. I don’t like that tradeoff. In fairness to Rust, I think Rust don’t like that tradeoff either, which is why they have all of the things like the really powerful compiler. That’s something that we’ll come back to as well.
Quarkus (Java Framework)
The question is, can we do better? This is where Quarkus comes in. Quarkus is a Java framework. The programming model will be very familiar to you. We have integrations with the libraries that you’re almost certainly already using, like Hibernate, like RESTEasy, but it’s got some really nice characteristics. One of those, and this is probably the thing that people think of when they think of Quarkus, is that Quarkus applications start really fast. You can run Quarkus with GraalVM as a natively compiled binary, or you can run it on OpenJDK. Either way, it starts really fast. If you run it with GraalVM, it actually starts faster than an LED light bulb. Just to give you a scale of how instantaneous the start is. Quarkus applications also have a really low memory footprint. When we used to run on dedicated hardware, this didn’t really matter.
Now that we run in the cloud where memory footprint is money, being able to shrink our instances and have a higher deployment density really matters. If you compare Quarkus to the cloud native stack that you’re probably all using, if you are architecting for Java, we are a lot smaller. You can fit a lot more Quarkus instances in. It’s not just when you compare it to other Java frameworks. When you compare Quarkus even to other programming languages, you can see that we’re competing with Go in terms of our deployment density. Node.js has a higher deployment density than old-school Java, but it’s not as good as Quarkus. This is cool.
There’s another thing that Quarkus is quite good at which we don’t talk about so much, and I wish we would talk about it more, and that’s throughput. If you look at your traditional cloud native stack, you might get about 3000 requests per second. If you are taking Quarkus with the GraalVM native compilation, the throughput is a little bit lower, same order of magnitude, but it’s lower. This is your classic tradeoff. You’re trading off throughput against footprint. This is something that I think we’re probably all familiar with in all sorts of contexts. With native compilation, you get a really great startup time, you get a great memory footprint, but at the expense of throughput.
Many years ago, I worked as a Java performance engineer, and one of the questions we always got was, I don’t like all of this stuff, this JIT and that kind of thing, couldn’t we do ahead-of-time compilation? The answer was, at that time, no, this is a really terrible idea. Don’t do ahead-of-time compilation. It will make your application slower. Now the answer is, it only makes your application a little bit slower, and it makes it so much more compact. Native compilation is a pretty reasonable choice, not for every circumstance, but for some use cases, like CLIs, like serverless. This is an awesome tradeoff, because you’re not losing that much throughput. This is a classic tradeoff. This is something that we see. I just grabbed one thing off core, but we see this sort of tradeoff all the time like, do I optimize my throughput or do I optimize my memory? Depends what you’re doing.
Let’s look at the throughput a little bit more, though, because this is the throughput for Quarkus native. What about Quarkus on JVM? It’s actually going faster than the alternative, while having a smaller memory footprint and a better startup time. That’s kind of unexpected, and so there is no tradeoff, we just made it better. Really, we took this tradeoff that everybody knows exists, and we broke it. Instead of having to choose between the two, you get both, and they’re both better. I always try and think, it’s a double win. I’ve tried a few. I’ve tried 2FA.
Someone suggested I should call it the überwinden. I don’t speak German, and so it sounded really cool to me, but it’s become clear to me now that the person who suggested it also didn’t speak German, because whenever I say it to a German person, they start laughing at me. German’s a bit like Rust. I always felt like I should learn it, and I never actually did. You may think, yes, this isn’t realistic. You can’t actually fold a seesaw in half. You can’t beat the tradeoff. It turns out you can fold a seesaw in half. There are portable seesaws that can fold in half.
What Are the Secrets?
How does this work? What’s the secret? Of course, there’s not just one thing. It’s not like this one performance optimization will allow you to beat all tradeoffs. There’s a whole bunch of things. I’ll talk about some of the ones that I think are more interesting. Really, with a lot of these, the starting point is, you have to challenge assumptions. In particular, you have to challenge outdated assumptions, because there were things that were a good idea 5 years ago, things that were a good idea 10 years ago, that now are a bad idea. We need to keep revisiting this knowledge that we’ve baked in. This, I was like, can I do this? Because I don’t know if you’ve heard the saying, when you assume you make an ass of you and me, and this is an African wild ass.
The first assumption that we need to challenge is this idea that we should be dynamic. This one I think is a really hard one, because anybody knows being dynamic is good, and I know being dynamic is good. I was a technical reviewer for the book, “Building Green Software”, by Anne. I was reading through, and I kept reading this bit where Anne and Sarah would say, “We need to stop doing this because it’s on-demand”. I was thinking, that’s weird. I always thought on-demand was good. I thought on-demand made things efficient. This is sort of true. Doing something on-demand is a lot better than doing it when there’s no demand, and never will be a demand. When you do something on-demand, you’re often doing it at the most expensive time. You’re often doing it at the worst time. You can optimize further, and you can do something when it hurts you least.
This does need some unlearning, because we definitely, I think, all of us, we have this idea of like, I’m going to be really efficient. I’m going to do it on-demand. No, stop. Being on-demand, being dynamic is how we architected Java for the longest time. Historically, Java frameworks, they were such clever engineering, and they were optimized for really long-lived processes, because we didn’t have CI/CD, doing operations was terrible. You just made sure that once you got that thing up, it stayed up, ideally, for a year, maybe two years.
Of course, the world didn’t stay the same. What we had to do was we had to learn how to change the engine while the plane was flying, so we got really good at late-binding. We got really good at dynamic binding, so that we could change parts of the system without doing a complete redeployment. Everything was oriented towards, how can I reconfigure this thing without restarting it? Because if I restart it, it might never come up again, because I have experience of these things.
We optimized everything. We optimized Java itself. We optimized all of the frameworks on top of it for dynamism. Of course, this kind of dynamism isn’t free, it has a cost. That cost is worth paying if you’re getting something for it. Of course, how do we run our applications now? We do not throw them over the wall to the ops team who leave it up for a year, we run things in the cloud.
We run things in containers, and so our applications are immutable. That’s how we build them. We have it in a container. Does anybody patch their containers in production? If someone said to you, I patch my containers in production, you’d be like, “What are you doing? Why are you doing that? We have CI/CD. Just rebuild the thing. That’s more secure. That’s the way to do it”. Our framework still has all of this optimization for dynamism, but we’re running it in a container, so it’s completely pointless. It is waste. Let’s have a look at how we’ve implemented this dynamism in Java. We have a bunch of things that happen at build time, and we have a bunch of things that happen at runtime.
Actually, the bunch of things that happen at build time, it’s pretty small. It’s pretty much packaging and compilation to bytecode, and that is it. All of the other excitement happens at runtime. The first thing that happens at runtime is the files are loaded. Config files get parsed. Properties files get parsed. The YAMLs gets parsed. The XML gets parsed. Then once we’ve done that, then there’s classpath scanning, there’s annotation discovery. Quite often, because things are dynamic, we try and load classes to see if we should enable or disable features. Then we keep going. Then, eventually the framework will be able to build this metamodel.
Then, after that, we do the things that are quite environment specific. We start the thread pools. We initialize the I/O. Then eventually, after all of that, we’re ready to do work. We’ve done quite a lot of work before we did any work, and this is even before we consider any of the Java features, like the JIT. What happens if we start this application more than once, then we do all of that work the first time. We do it again the second time. We do it again the third time. We do it again the fourth time, and there’s so much work each time. It’s a little bit like Groundhog Day, where we’re doing the same work each time. Or it’s a little bit like a goldfish, where it’s got this 30-second memory, and the application has no memory of the answers that it just worked out and it has to do the same introspection each time.
Let’s look at some examples. In Hibernate, it will try and bind to a bunch of internal services. For example, it might try and bind to JTA for your transactions. The first thing it does is it doesn’t know what’s around it, so it says, ok, let me do a reflective load of an implementation. No, it’s not there. Let me try another possible implementation. No, it’s not there. Let me try another implementation. No, it’s not there. It keeps going. Keeps going. It keeps going. Of course, each time it does this reflective load, it’s not just the expense of the load, each time a class not found exception is thrown. Throwing exceptions is expensive, and it does this 129 times, because Hibernate has support for a wide range of possible JTA implementations. It does that every single time it starts. This isn’t just JTA, there are similar processes for lots of internal services. We see similar problems with footprint.
Again, with Hibernate, it has support for lots of databases, and so it loads the classes for these databases. Then eventually, hopefully, they’re never used, and the JIT works out that they’re not used, and it unloads them, if you’re lucky. Some classes get loaded and then they never get unloaded. For example, the XML parsing classes, once they’re loaded, that’s it. They’re in memory, even if they never get used again. This is that same thing. It’s that really sort of forgetful model. There’s a lot of these classes. For example, for the Oracle databases, there’s 500 classes, and they are only useful if you’re running an Oracle database. It affects your startup time. It affects your footprint. It also affects your throughput.
If you look, for example, at how method dispatching works in the JVM, if you have an interface and you’ve got a bunch of implementations of it. When it tries to invoke the method, it kind of has to do quite a slow path for the dispatch, because it doesn’t know which one it’s going to at some level. This is called a megamorphic call, and it’s slow. If you only have one or two implementations of that interface, the method dispatching is fast. By not loading those classes in the first place, you’re actually getting a throughput win, which is quite subtle but quite interesting. The way you fix this is to initialize at build time.
The idea is that instead of redoing all of this work, we redo it once at build time, and then at runtime we only do the bare minimum that’s going to be really dependent on the environment. What that means is, if you start repeatedly, you’ve got that efficiency because you’re only doing a small amount of work each time. That is cool. Really, this is about eliminating waste. As a bonus with this, what it means is that if you want to do AOT, if you want to do native in GraalVM, you’re in a really good place. Even if you don’t do that, even if you’re just running on the JVM as a normal application, you’ve eliminated a whole bunch of wasted, repeated, duplicate, stupid work.
Really, this is about doing more upfront. The benefits that you get are, it speeds up your start. It shrinks your memory footprint. Then, somewhat unexpectedly, it also improves your throughput. What this means is that, all of the excitement, all of the brains of the framework is now at build time rather than at runtime, and there’s lots of frameworks.
One of the things that we did in Quarkus was we said, we have to make the build process extensible now. You have to be able to extend Quarkus, and they have to be able to participate in the build process, because that’s where the fun is happening. I think with anything that’s oriented around performance, you have to have the right plug-points so that your ecosystem can participate and also contribute performance wins. What we’ve done in Quarkus is we have a framework which is build steps and build items, and any extension can add build steps and build items.
Then, what we do is, build steps get declared, and then an extension can declare a method that says, I take in this build step, and I output that build step. We use that to dynamically order the build to make sure that things happen in the right time and everything has the information that it needs. The framework automatically figures out what order it should build stuff in. Of course, if you’re writing an extension, or even if you’re not, you can look to see what’s going on with your build, and you can see how long each build step is taking, and get the introspection there.
Some of you are probably thinking, if you move all of the work to build time, and I, as a developer, build locally a lot, that sounds kind of terrible. What we’ve done to mitigate this is we’ve got this idea of live coding. I’ve been in the Quarkus team for about two years. When I joined the team, I always called live coding, hot reload. Every time my colleagues would get really annoyed with me, and they’d be like, it’s not hot reload, it’s different from hot reload. I think I now understand why. We have three levels of reload, and the framework, which knows a lot about your code, because so much excitement is happening at build time, it knows what the required level of reload is. If it’s something like a config file, we can just reload the file, or if it’s something like CSS or that kind of thing. If it’s something that maybe affects a little bit more of the code base, we have a JVM agent, and so it will do a reload there. It will just dynamically replace the classes.
Or, if it’s something pretty invasive that you’ve changed, it will do a full restart. You can see that full restart took one second, so even when it’s completely bringing the whole framework down and bringing it back up again, as a developer, you didn’t have to ask it to do it, and as a developer, you probably don’t even notice. That’s cool. I think this is a really nice synergy here, where, because it starts so fast, it means that live coding is possible. Because as a developer, it will restart, and you’ll barely notice. I think this is really important, because when we think about the software development life cycle, it used to be that hardware was really expensive and programmers were cheap.
Now, things have switched. Hardware is pretty cheap. Hardware is a commodity, but developers are really expensive. I know we shouldn’t call people resources, and people are not resources, but on the other hand, when we think about a system, people are resources. Efficiency is making use of your resources in an optimum way to get the maximum value. When we have a system with people, we need to make sure that those people are doing valuable things, that those people are contributing, rather than just sitting and watching things spin.
How to Make People Efficient
How do you make people efficient? You should have a programming language that’s hard to get wrong, idiot proof. You want strong typing and you want garbage collection. Then, it’s about having a tight feedback loop. Whether you’re doing automated testing or manual testing, you really need to know that if you did get it wrong despite the idiot proofing, you find out quickly. Then, typing is boring, so we want to do less typing. Java gives us those two, the strong typing and the garbage collection. I just showed that tight feedback loop. What about the typing? With Quarkus, we’ve looked at the performance, but then we’ve also really tried to focus on developer joy and making sure that using Quarkus is delightful and fast. One of the things that we do to enable this is indexing. Indexing seems like it’s actually just a performance technique, but we see it gives a lot of interesting benefits in terms of the programming model.
Most frameworks, if it’s doing anything framework-y and non-trivial, it needs to find all of the classes. It needs to find all of the interfaces that have some annotation, because everything is annotations, because we’ve learned that everything shouldn’t be XML. You also really often have to find all of the classes that implement or extend some class. Annoyingly, even though this is something that almost every Java library does, Java doesn’t really give us a lot of help for this. There’s nothing in the reflection package that does this. What we’ve done is we have a library called Jandex, which is basically offline reflection. It’s really fast. It indexes things like the annotations, but it also indexes who uses you. You can start to see, this could be quite useful.
What kind of things can we do with the index? What we can do is we can go back and we can start challenging more assumptions about what programming looks like, and we can say, what if developers didn’t have to do this and that, and this and that? As an example, a little example, I always find it really frustrating when I’m doing logging that I have to initialize my logger, and I have to say, Logger.getLogger, whatever the call is, and tell it what class it sees. I only half the time know what class I’m programming in, and I get this wrong so often because I’ve cut and paste the declaration from somewhere else.
Then there’s this mistake in the code base, and the logging is wrong. I was like, why do I have to tell you what class you’re in when you should know what class you’re in, because you’re the computer, and I’m just a stupid person? What we’ve done with Quarkus is exactly that. You don’t have to declare your logger. You can just call, capital the static call Log.info, and it will have the correct logging with the correct class information. This is so little, but it just makes me so happy. It’s so nice. I think this is a good general principle of like, people are stupid and people are lazy. Don’t make people tell computers things that the computer already knows, because that’s just a waste of everybody’s time, and it’s a source of errors. When I show this to people, sometimes they like it, and go, that’s cool.
Sometimes they go, no, I don’t like that, because I have an intuition about performance, I have an intuition about efficiency, and I know that doing that kind of dynamic call is expensive. It’s not, because we have the Jandex index, so we can, at build time, use Jandex to find everybody who calls that log.class, inject a static field in them, initialize the static field correctly. Because it’s done at build time, you don’t get that performance drag that you get with something like aspects. Aspects were lovely in many ways, but we all stopped using them, and one of the reasons was the performance of them was a bit scary. We assume that we can’t do this thing that we really want to do because we assume it’s expensive, it’s not anymore. It gets compiled down to that. You can see that that is pretty inoffensive code. I don’t think anybody would object to that code in their code base.
Let’s look at a more complex example. With Hibernate, obviously, Hibernate saves you a great deal of time, but you still end up with quite a bit of boilerplate in Hibernate, and repeated code. Things like, if I want to do a listAll query, you have to declare that for every entity. It’s just a little bit annoying. You think, couldn’t I just have a superclass that would have all of that stuff that’s always the same? What we can do with Hibernate, if you have your repository class, what we can do is we can just get rid of all of that code, and then we can just have a Panache repository that we extend.
That’s the repository pattern where you have a data access object because your entity is a bit stupid. For me, I find an active record pattern a lot more natural. Here I just acquire my entity, and everything that I want to do in my entity is on the entity. That’s normally not possible with normal Hibernate, but with Hibernate with Panache, which is something that the Quarkus team have developed, you can do that. Again, you’ve got that superclass, so you don’t have to do much work, and it all works. One interesting thing about this is it seems so natural. It seems like, why is this even hard?
Of course, I can inherit from a superclass and have the brains on the superclass. With how Hibernate is working, it’s actually really hard. If I was to implement this from scratch, I might do something like, I would have my PanacheEntity, and then it would return a list. The signature can be generic. It’s ok to say, it just returns a list of entities. In terms of the implementation, I don’t actually know what entity to query, because I’m in a generic superclass. It can’t be done, unless you have an index, and unless you’re doing lots of instrumentation at build time. Because here what you can do is you see the superclass as a marker, and then you make your actual changes to the subclass, where you know what entity you’re talking to. This is one of those cases where we broke the tradeoff that machine efficiency of having the index enabled the human efficiency of the nice programming model.
Some people are probably still going, no, I have been burned before. I used Lombok once, and once I got into production, I knew that magic should be avoided at all cost. This is something that the Quarkus team have been very aware of. When I was preparing for this talk, I asked them, under the covers, what’s the difference between what we do and something like Lombok? Several of the Quarkus team started screaming. They know that, with this, what you want is you want something that makes sense to the debugger, and you want something where the magic is optional. Like that logging, some of my team really like it.
Some of my team don’t use it because they want to do it by hand. Panache, some people really like it. Some of the team just use normal Hibernate. All of these features are really optional. They’re a happy side effect. They’re not like the compulsory thing. I think again, this is a question of efficiency. What we see with a lot of these frameworks, or some of these low-code things, is they make such good demos, but then as soon as you want to go off the golden path, off the happy path, you spend so long fighting it that you lose any gain that you maybe had from that initial thing. Really, we’ve tried to optimize for real use, not just things that look slick in demos.
The Common Factor Behind Performance Improvements
I’ve talked about a few of the things that we do, but there’s a lot of them. When I was preparing this talk, I was trying to think, is there some common factor that I can pull out? I started thinking about it. This is my colleague, Sanne Grinovero. He was really sort of developer zero on Quarkus. He did the work with Hibernate to allow Hibernate to boot in advance. This is my colleague, Francesco Nigro. He’s our performance engineer, and he does some really impressive performance fixes. This is another colleague, this is Mario Fusco. He’s not actually in the Quarkus team. He tends to do a lot of work on things like Drools, but he’s given us some really big performance fixes too.
For example, with Quarkus and Loom, so virtual threads, we had really early support for virtual threads back when it was a tech preview. What we found was that virtual threads, you hope that it’s going to be like a magic go faster switch, and it is not, for a number of reasons. One of the reasons is that some libraries interact really badly with virtual threads, and so some libraries will do things like pinning the carrier thread. When that happens, everything grinds to a halt. Jackson had that behavior. Mario contributed some PRs to Jackson that allowed that problem in Jackson to be solved, so that Jackson would work well with virtual threads.
I was looking and I was like, what is that common factor? What is it? I realized they’re Italian. This is a classic example of confirmation bias. I decided the key to our performance was being Italian. Without even realizing it, I looked for the Italians who’d done good performance work. When we do a Quarkus release, we give out a T-shirt that says, I made Quarkus. On the most recent release, we gave out 900 T-shirts. There’s a lot of contributors. A lot of people have done really cool engineering on Quarkus, only some of them were Italian. You don’t have to be Italian to be good at performance, in case anybody is feeling anxious. The title of this talk is Italian graft, and so being Italian is optional, but the graft part is not. This stuff is work. When you’re doing that kind of performance optimization, you have to be guided by the data, and you have to do a lot of graft. You measure, because you don’t want to do anything without measuring.
Then you find some tiny improvement, and you shave it off. Then you measure and you find some tiny improvement, and you shave a little bit of time off. You measure, and then you find some tiny improvement. This was very much what we saw in this morning’s talk as well. It was in C rather than Java, but it was the same thing. If I’m going to profile, then I’m going to find some tiny optimization that I’m going to do. You keep going and you keep going. It’s not easy, so it needs a lot of skill, and it also needs a lot of hard work. I mentioned Francesco, our performance engineer, and he really is like a dog with a bone. When he sees a problem, he’ll just go and go. I think a lot of the rest of us would have gone, “Ooh”, and he just keeps going. He has this idea that what he offers to the team is obsession as a service. You need people like that.
I want to give one example. We run the tech and power benchmark, and what we found was we were behaving really unexpectedly badly when there was this large number of cores. With a small number of cores, our flame graph looked as we hoped. When it was a lot of cores, all of a sudden, our flame graph had this really weird shape, and there was this flat bit, and we’re like, what’s going on there? Why is no work happening in this section of the flame graph? Again, many people would have gone, what a shame? To find out, Francesco and Andrew Haley, another colleague, they read 20,000 lines of assembler. What they found was worth it. They found the pattern that was causing the scalability problem, and the pattern was checking if something is an instanceof.
At this point, hopefully some of you are screaming as well and going, I think there’s a lot of that. That’s not a weird, obscure pattern, that is a very common pattern. Once Franz had found the problematic pattern, he started to look at what other libraries might be affected. We found Quarkus was affected. Netty was affected. Hibernate was affected. Camel was affected. The Java Class library was affected. This was a really big, really bad bug. He found actually that there was an existing bug, but nobody had really realized the impact of it. I think this is partly because it happens when you’ve got like 32 cores, when you’ve got like 64 cores. We’re now much more often running at that kind of scale. It’s a cache pollution problem.
The problem is, when you do this check, the cache that is used for this check is shared across all of the cores. If you’ve got a lot of code running in parallel, basically the cache just keeps getting corrupted, and then you just keep having to redo the work. This was a bad problem. This was not like that saving 2%. This is one of the tech and power benchmarks, and this was running before the fix and running after the fix. You can see we went from 1.8 million requests per second to 5.8 million requests per second. That’s just a small benchmark, but it was a huge improvement.
What we did was, Franz wrote a little tool, because not every instanceof call is problematic. It depends on various factors. He wrote a tool that would go through and detect the problematic case. We ran it through the whole code base, and we started doing the fixes. It’s very sad, because this is fixed in the JVM now, but only on the sort of head, so people won’t get the benefit of the fix for quite a while. We had code that was, for example, like this. Then after the fix, you can see we had to do all of this stuff.
Again, you don’t need to necessarily read the code, but you can just see that the throughput is a lot higher, but the code is a lot longer, so it’s again exactly the same as Alan’s talk. You have this tradeoff. I love it for this one, because the developer did the PR and then they basically apologized for the code that they’re doing in the PR. I’m not a fan of the fix. It’s not idiomatic. It’s difficult to maintain, but it gives us so much more throughput that we have to do it. Again, it’s that tradeoff of machine efficiency against human efficiency. Only in this case, it’s not everybody else’s efficiency, it’s just my team’s efficiency. This is what Anne was talking about when she said, you really want your platform to be doing the hard, grotty, nasty work so that you can have the delightful performance experience. We do the nasty fixes so that hopefully other people don’t have to.
Another thing to note about efficiency is it’s not a one-time activity. It’s not like you can have the big bang, and you can go, yes, we halved the throughput, or halved the cost. Life happens, and these things just tend to backslide. A while ago, Matt Raible was doing some benchmarking, and he said, this version of Quarkus is much slower than the previous version. We thought, that’s odd. That’s the opposite of what we hoped would happen. Then we said, “Are we measuring our performance?” Yes. “Don’t we look to see if we’re getting better or worse?” Yes. “What happened?” What it is, is, if you get that bit of code, is the performance getting better or worse here? It looks like the performance is getting much better. If you look at it over the longer picture, you can see that actually it’s probably getting a little bit worse. Because we had this really big regression that masked a series of smaller regressions.
We had a change detection algorithm that was parametric, and it meant that we missed this regression. We did the work and we fixed it, and we fixed a lot. It was very cool. That was another engineer who was not Italian, called Roberto Cortez. One of the things that Roberto did, which just makes me smile, is, again, it’s about the assumptions. We do a lot of string comparison in config. Config tends to be names based, and so the way any normal human being would do a string comparison is you start at the first character, and then you go. The interesting bit is always at the end. Roberto worked out, if I go from the other end, the config is much faster. I would recommend you all to have a Francesco, to have a performance engineer. You can’t have Francesco, he’s ours, but you need to find your own. It does need investment.
I’ve got one last tradeoff I want to talk about. This is the efficient languages track, but we really do have a green focus here. There’s this classic tradeoff with sustainability between doing the stuff that we want to do and saving the planet. In general, historically, we have always tended to do the stuff we want to do rather than save the planet. I think there is some hope here. I’ve started talking about something called the vrroooom model. Naming is the hardest problem in computer science, because I didn’t think to actually do a Google before I did the name. It turns out there is a vroom model, which is a decision model. That’s with a slightly different spelling than I did. I did 3r’s and 2o’s and stuff, which was another terrible mistake.
If you Google, vrroooom, it thinks you want to do it with the conventional spelling, but then it says, but would you like to search instead for the vrroooom model with the idiosyncratic spline? If you click on that, what do you think happens? The hope is that you get my stuff. The reality is rather different. Everything here, it’s all about cars, and hot babes. That is what you get if you search for the vrroooom model. Even you can see there, that’s a Tesla advert. It says sexy above it. It’s all about sexy cars. Naming, hardest problem in computer science. I should have thought about that.
My vrroooom model, the one that doesn’t involve sexy cars, I really started thinking about this when I looked at the paper. We were talking about this before, and Chris said, you know that stupid paper that compares the programming languages, and there’s a lot of problems with this paper. What I want to show you is not the details of it, but something that I noticed, which is, it has a column for energy and it has a column for time, and they look kind of almost the same.
If you plot it, you can confirm that this trend line is basically straight. It means languages that go fast have a low carbon footprint. We see this with Quarkus. With Quarkus on this graph, we benchmarked the energy consumption of Quarkus native, Quarkus on JVM, the other framework on JVM, the other framework on native. What we did was we had a single instance, and we just fired load at it until it ran out of throughput. The shorter lines are where it ran out of throughput earlier. Lower is better. Lower is the lower carbon footprint. You can see that there’s, again, this really strong correlation. Quarkus on JVM has the lowest carbon footprint of any of these options because it has the highest throughput. It’s the win-win again, that you get to have the really fast language and have the nice programming model and also save the world. We beat the tradeoff.
I just love this that instead of having this opposition between machine efficiency and human efficiency, the one helps us gain the other. If you start with efficient languages, you really need to consider both machine efficiency and human efficiency. When you’re looking at your machine efficiency, you need to challenge your assumptions. Only do work once, obviously. Move work to where it hurts the least. Index. Indexes are so cheap, they’re so good, they solve so many problems. Unfortunately, this isn’t a one-off activity. You do need that continued investment in efficiency. Then, when you look at your human efficiency again, same thing, you need to challenge your assumptions. You need to get those feedback loops as small as you can. Don’t make people tell the computer what the computer already knows, because that’s a waste of everybody’s time.
See more presentations with transcripts
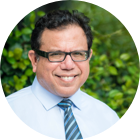
MMS • Daniel Dominguez
Article originally posted on InfoQ. Visit InfoQ
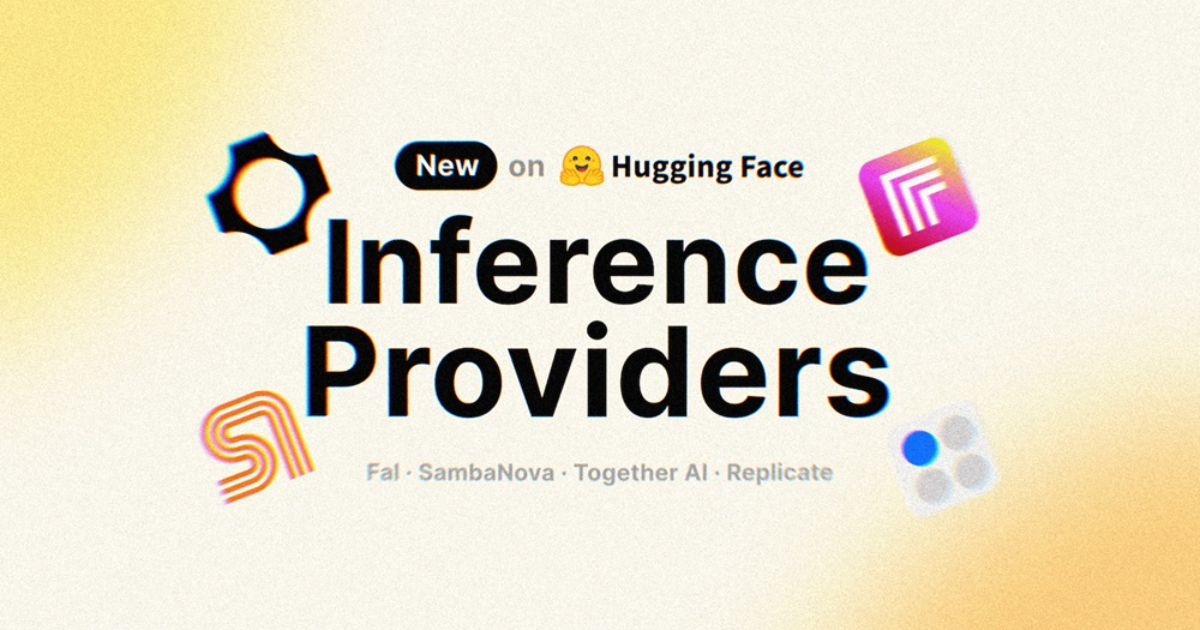
Hugging Face has launched the integration of four serverless inference providers Fal, Replicate, SambaNova, and Together AI, directly into its model pages. These providers are also integrated into Hugging Face’s client SDKs for JavaScript and Python, allowing users to run inference on various models with minimal setup.
This update enables users to select their preferred inference provider, either by using their own API keys for direct access or by routing requests through Hugging Face. The integration supports different models, including DeepSeek-R1, and provides a unified interface for managing inference across providers.
Developers can access these services through the website UI, SDKs, or direct HTTP calls. The integration allows seamless switching between providers by modifying the provider name in the API call while keeping the rest of the implementation unchanged. Hugging Face also offers a routing proxy for OpenAI-compatible APIs.
Rodrigo Liang, co-founder & CEO at SambaNova, stated:
We are excited to be partnering with Hugging Face to accelerate its Inference API. Hugging Face developers now have access to much faster inference speeds on a wide range of the best open source models.
And Zeke Sikelianos, founding designer at Replicate, quoted:
Hugging Face is the de facto home of open-source model weights, and has been a key player in making AI more accessible to the world. We use Hugging Face internally at Replicate as our weights registry of choice, and we’re honored to be among the first inference providers to be featured in this launch.
Fast and accurate AI inference is essential for many applications, especially as demand for more tokens increases with test-time compute and Agentic AI. Open-source models help optimize performance on RDU, enabling developers to achieve up to 10x faster inference with improved accuracy.
Billing is handled by the inference provider if a user supplies their own API key. If requests are routed through Hugging Face, charges are applied at standard provider rates with no additional markup.
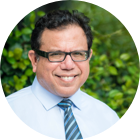
MMS • Robert Krzaczynski
Article originally posted on InfoQ. Visit InfoQ
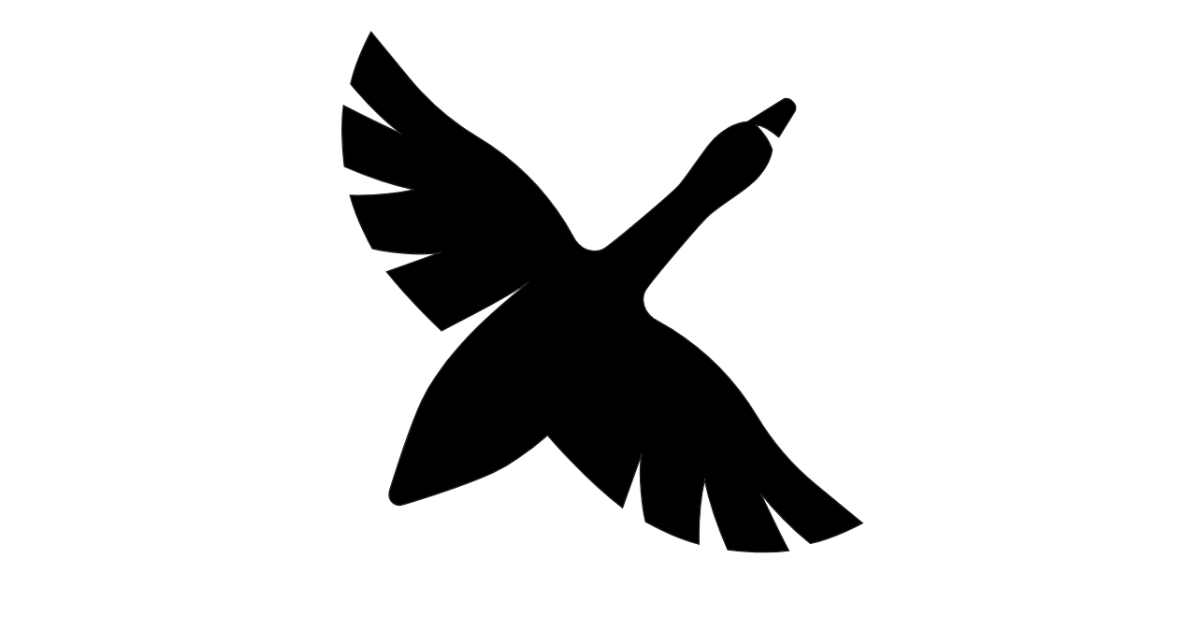
Block’s Open Source Program Office has launched Codename Goose, an open-source, non-commercial AI agent framework designed to automate tasks and integrate seamlessly with existing tools. Goose provides users with a flexible, on-machine AI assistant that can be customized through extensions, enabling developers and other professionals to enhance their productivity.
Goose is designed to integrate seamlessly with existing developer tools through extensions, which function using the Model Context Protocol (MCP). This enables users to connect with widely used platforms such as GitHub, Google Drive, and JetBrains IDEs while also allowing them to create custom integrations. The AI agent is positioned as a tool for both software engineers and other professionals looking to optimize their workflows.
Goose functions as an autonomous AI agent that can carry out complex tasks by coordinating various built-in capabilities. Users can integrate their preferred LLM providers, ensuring flexibility in how the tool is deployed. Goose is designed for easy adaptation, allowing developers to work with AI models in a way that fits their existing workflows.
The agent supports a range of engineering-related tasks, including:
- Code migrations
- Generating unit tests for software projects
- Scaffolding APIs for data retention
- Managing feature flags within applications
- Automating performance benchmarking for build commands
- Increasing test coverage above specific thresholds
As an open-source initiative, Goose has already attracted attention from industry professionals. Antonio Song, a contributor to the project, highlighted the importance of user interaction in AI tools:
Most of us will have little to no opportunity to impact AI model development itself. However, the interface through which users interact with the AI model is what truly drives users to return and find value.
Furthermore, user Lumin commented on X:
Goose takes flight. Open-source AI agents are no longer a side project—they are defining the future. Codename Goose 1.0 signals a paradigm shift: decentralized, non-commercial AI frameworks bridging intelligence and real-world execution. The AI race has been dominated by centralized models with restricted access. Goose challenges that by enabling modular AI agents that can install, execute, edit, and test with any LLM, not just a select few.
Goose is expected to evolve further as more contributors refine its capabilities. The tool’s extensibility and focus on usability suggest it could become a widely adopted resource in both engineering and non-engineering contexts.
Java News Roundup: Java Operator SDK 5.0, Open Liberty, Quarkus MCP, Vert.x, JBang, TornadoVM
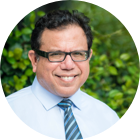
MMS • Michael Redlich
Article originally posted on InfoQ. Visit InfoQ
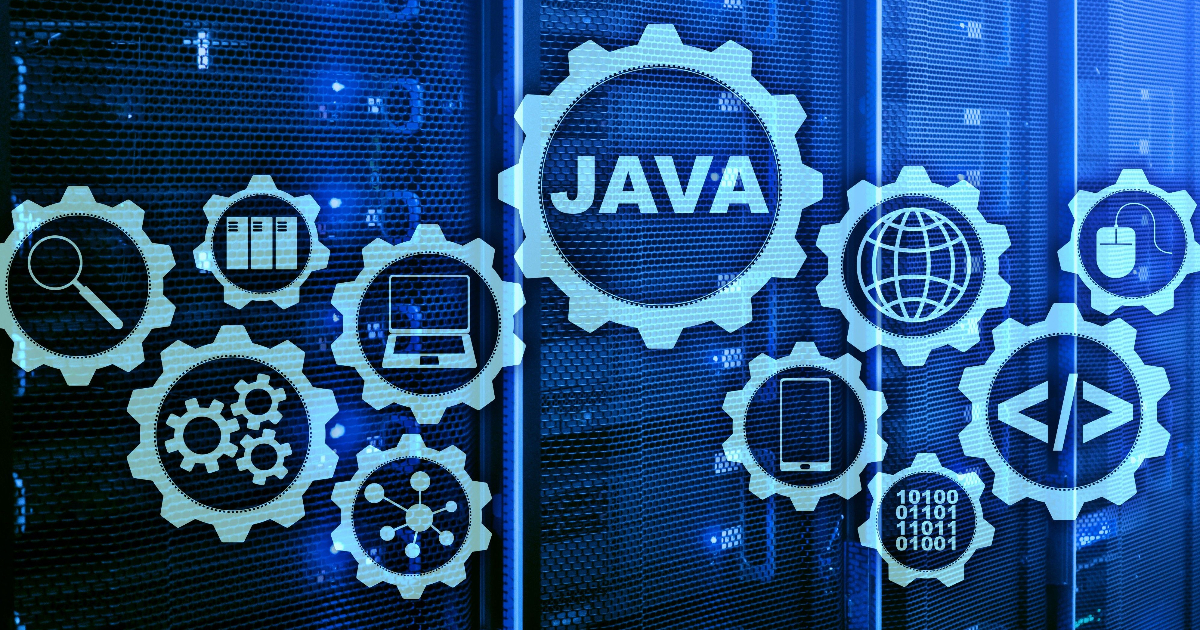
This week’s Java roundup for January 27th, 2025, features news highlighting: the GA release of Java Operator SDK 5.0; the January 2025 release of Open Liberty; an implementation of Model Context Protocol in Quarkus; the fourth milestone release of Vert.x 5.0; and point releases of JBang 0.123.0 and TornadoVM 1.0.10.
JDK 24
Build 34 of the JDK 24 early-access builds was made available this past week featuring updates from Build 33 that include fixes for various issues. Further details on this release may be found in the release notes.
JDK 25
Build 8 of the JDK 25 early-access builds was also made available this past week featuring updates from Build 7 that include fixes for various issues. More details on this release may be found in the release notes.
For JDK 24 and JDK 25, developers are encouraged to report bugs via the Java Bug Database.
TornadoVM
TornadoVM 1.0.10 features bug fixes, compatibility enhancements, and improvements: a new command-line option, -Dtornado.spirv.runtimes
, to select individual (Level Zero and/or OpenCL) runtimes for dispatching and managing SPIR-V; and support for multiplication of matrices using the HalfFloat
type. Further details on this release may be found in the release notes.
Spring Framework
The first milestone release of Spring Cloud 2025.0.0, codenamed Northfields, features bug fixes and notable updates to sub-projects: Spring Cloud Kubernetes 3.3.0-M1; Spring Cloud Function 4.3.0-M1; Spring Cloud Stream 4.3.0-M1; and Spring Cloud Circuit Breaker 3.3.0-M1. This release is based upon Spring Boot 3.5.0-M1. More details on this release may be found in the release notes.
Open Liberty
IBM has released version 25.0.0.1 of Open Liberty featuring updated Open Liberty features – Batch API (batch-1.0
), Jakarta Batch 2.0 (batch-2.0
), Jakarta Batch 2.1 (batch-2.1
), Java Connector Architecture Security Inflow 1.0 (jcaInboundSecurity-1.0
), Jakarta Connectors Inbound Security 2.0 (connectorsInboundSecurity-2.0
) – to support InstantOn; and a more simplified web module migration with the introduction of the webModuleClassPathLoader
configuration attribute for the enterpriseApplication
element that controls what class loader is used for the JARs that are referenced by a web module Class-Path
attribute.
Quarkus
The release of Quarkus 3.18.0 provides bug fixes, dependency upgrades and notable changes such as: an integration of Micrometer to the WebSockets Next extension; support for a JWT bearer client authentication in the OpenID Connect and OpenID Connect Client extensions using client assertions loaded from the filesystem; and a new extension, OpenID Connect Redis Token State Manager to store an OIDC connect token state in a Redis cache datasource. Further details on this release may be found in the changelog.
The Quarkus team has also introduced their own implementation of the Model Context Protocol (MCP) protocol featuring three servers so far: JDBC, Filesystem and JavaFX. These servers have been tested with Claude for Desktop, Model Context Protocol CLI and Goose clients. The team recommends using JBang to use these servers for ease of use, but isn’t required.
Apache Software Foundation
Maintaining alignment with Quarkus, the release of Camel Quarkus 3.18.0, composed of Camel 4.9.0 and Quarkus 3.18.0, provides resolutions to notable issues such as: the Kamelet extension unable to serialize objects from an instance of the ClasspathResolver
, an inner class defined in the DefaultResourceResolvers
, to bytecode; and the Debezium BOM adversely affects the unit tests from the Cassandra CQL extension driver since the release of Debezium 1.19.2.Final. More details on this release may be found in the release notes.
Infinispan
The release of Infinispan 15.1.5 features dependency upgrades and resolutions to issues such as: a NullPointerException
due to a concurrent removal with the DELETE
statement causing the cache::removeAsync
statement to return null
; and an instance of the HotRodUpgradeContainerSSLTest
class crashes the test suite due to an instance of the PersistenceManagerImpl
class failing to start. Further details on this release may be found in the release notes.
Java Operator SDK
The release of Java Operator SDK 5.0.0 ships with continuous improvements on new features such as: the Kubernetes Server-Side Apply elevated to a first-class citizen with a default approach for patching the status resource; and a change in responsibility with the EventSource
interface to monitor the resources and handles accessing the cached resources, filtering, and additional capabilities that was once maintained by the ResourceEventSource
subinterface. More details on this release may be found in the release notes.
JBang
JBang 0.123.0 provides bug fixes, improvements in documentation and new features: the options, such as add-open
and exports
, in a bundled MANIFEST.MF
file are now honored; and the addition of Cursor, the AI code editor, in the list of supported IDEs. Further details on this release may be found in the release notes.
Eclipse Vert.x
The fourth release candidate of Eclipse Vert.x 5.0 delivers notable changes such as: the removal of deprecated classes – ServiceAuthInterceptor
and ProxyHelper
– along with the two of the overloaded addInterceptor()
methods defined in the ServiceBinder class; and support for the Java Platform Module System (JPMS). More details on this release may be found in the release notes and deprecations and breaking changes.
JHipster
Versions 1.26.0 and 1.25.0 of JHipster Lite (announced here and here, respectively) ship with bug fixes, dependency upgrades and new features/enhancements such as: new datasource modules for PostgreSQL, MariaDB, MySQL and MSSQL; and a restructured state ranking system for modules. Version 1.26.0 also represents the 100th release of JHipster Lite. Further details on these releases may be found in the release notes for version 1.26.0 and version 1.25.0.
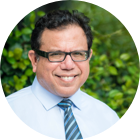
MMS • Apoorva Joshi
Article originally posted on InfoQ. Visit InfoQ
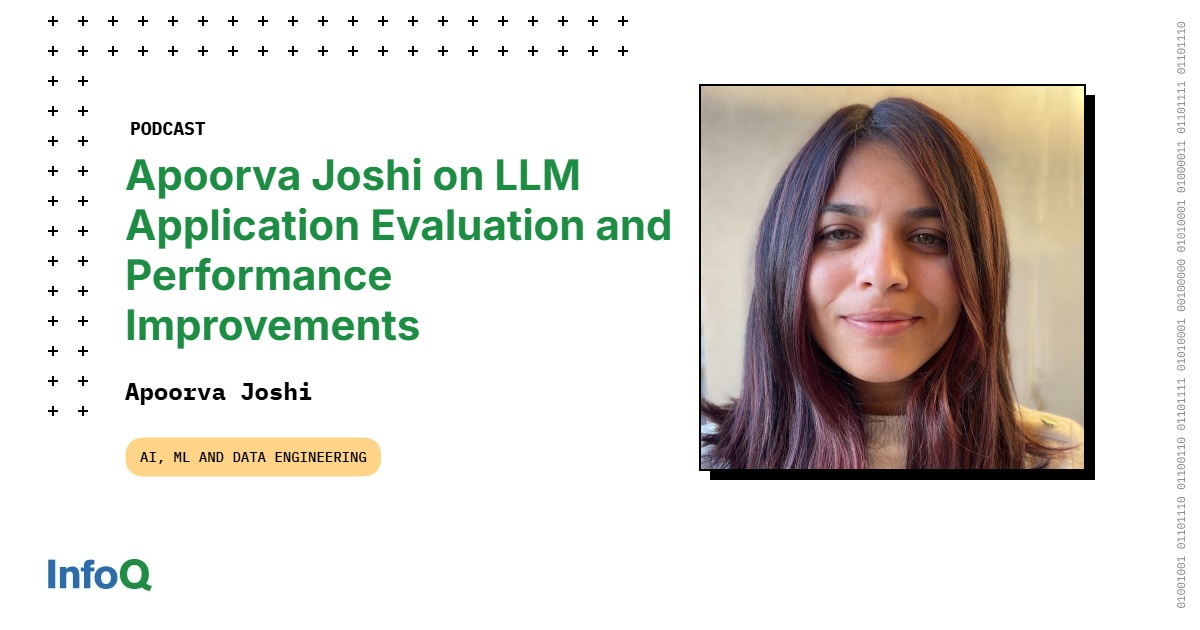
Transcript
Srini Penchikala: Hi, everyone. My name is Srini Penchikala. I am the lead director for AI, ML, and the data engineering community at InfoQ website and a podcast host.
In this episode, I’ll be speaking with Apoorva Joshi, senior AI developer advocate at MongoDB. We will discuss the topic of how to develop software applications that use the large language models, or LLMs, and how to evaluate these applications. We’ll also talk about how to improve the performance of these apps with specific recommendations on what techniques can help to make these applications run faster.
Hi, Apoorva. Thank you for joining me today. Can you introduce yourself, and tell our listeners about your career and what areas have you been focusing on recently?
Apoorva Joshi: Sure, yes. Thanks for having me here, Srini. My first time on the InfoQ Podcast, so really excited to be here. I’m Apoorva. I’m a senior AI developer advocate here at MongoDB. I like to think of myself as a data scientist turned developer advocate. In my past six years or so of working, I was a data scientist working at the intersection of cybersecurity and machine learning. So applying all kinds of machine learning techniques to problems such as malware detection, phishing detection, business email compromise, that kind of stuff in the cybersecurity space.
Then about a year or so ago, I switched tracks a little bit and moved into my first role as a developer advocate. I thought it was a pretty natural transition because even in my role as a data scientist, I used to really enjoy writing about my work and sharing it with the community at conferences, webinars, that kind of thing. In this role, I think I get to do both the things that I enjoy. I’m still kind of a data scientist, but I also tend to write and talk a bit more about my work.
Another interesting dimension to my work now is also that I get to talk to a lot of customers, which is something I always wanted to do more of. Especially in the gen AI era, it’s been really interesting to talk to customers across the board, and just hear about the kind of things they’re building, what challenges they typically run into. It’s a really good experience for me to offer them my expertise, but also learn from them about the latest techniques and such.
Srini Penchikala: Thank you. Definitely with your background as a data scientist and a machine learning engineer, and obviously developer advocate working with the customers, you bring the right mix of skills and expertise that the community really needs at this time because there is so much value in the generative AI technologies, but there’s also a lot of hype.
Apoorva Joshi: Yes.
Srini Penchikala: I want this podcast to be about what our listeners should be hyped about in AI, not all about the hype out there.
Let me first start by setting the context for this discussion with a quick background on large language models. The large language models, or LLMs, have been the foundation of gen AI applications. They play a critical role in developing those apps. We are seeing LLMs being used pretty much everywhere in various business and technology use cases. Not only for the end users, customers, but also for the software engineers in terms of code generation. We can go on with so many different use cases that are helping the software development lifecycle. And also, devops engineers.
I was talking to a friend and they are using AI agents to automatically upgrade the software on different systems in their company, and automatically send the JIRA tickets if there are issues. Agents are doing all this. They’re able to cut down the work from number of days and number of weeks for these upgrades. The patching process is down to minutes and hours. Definitely the sky is the limit there, right?
Apoorva Joshi: Yes.
Current State of LLMs [04:18]
Srini Penchikala: What do you see? What’s the current state of LLMs? And what are you seeing in the industry, are they being used, and what use cases are they being applied today?
Apoorva Joshi: I think there’s two slightly different questions here. One is what’s the current state of LLMs, and then application.
To your first point, I’ve been really excited to see the shift from purely text generation models to models that generate other modalities, such as image, audio, and video. It’s been really impressive to see how the quality of these models has improved in the past year alone. There’s finally benchmarks and we are actually starting to see applications in the wild that use some of these other modalities. Yes, really exciting times ahead as these models become more prevalent and find their place in more mainstream applications.
Then coming to how LLMs are being applied today, like you said, agents are the hot thing right now. 2025 is also being touted as the year of AI agents. Definitely seeing that shift in my work as well. Since the past year, we’ve seen our enterprise customers move from basic RAG early or mid last year to building more advanced RAG applications using slightly more advanced techniques, such as hybrid search, parent document retrieval, and all of this to improve the context being passed to LLMs for generation.
Then now, we are also seeing folks further move on to agents, so frequently hearing things like self-querying retrieval, human in the loop agents, multi-agent architectures, and stuff like that.
Srini Penchikala: Yes. You’ve been publishing and advocating about all of these topics, especially LLM-based applications which is the focus of this podcast. We’re not going to get too much into the language models themselves.
Apoorva Joshi: Yes.
Srini Penchikala: But we’ll be talking about how those models are using applications and how we can optimize those applications. This is for all the software developers out there.
LLM-based Application Development Lifecycle [06:16]
Yes, you’ve been publishing about and advocating about how to evaluate and improve the LLM application performance. Before we get into the performance side of discussion, can you talk about what are the different steps involved in a typical LLM-based application, because different applications and different organizations may be different in terms of number of steps?
Apoorva Joshi: Sure. Yes. Thinking of the most common elements, data is the first obvious big one because the LLMs work on some task out of the box. But at most organizations they want them to work on their own data or domain-specific use cases in industries like healthcare, legal. You need something a bit more than just a powerful language model, that’s where data becomes an important piece.
Then once you have data and you want language models to use that data to inform their responses, that’s where retrieval becomes a huge thing. Which is why things have progressed from just simple vector search or semantic search to some of these more advanced techniques, like again, hybrid search, parent document retrieval, self-querying, knowledge graphs. There’s just so much on that front as well. Then the LLM is a big piece of it if you’re building LLM-based applications.
I think one piece that a lot of companies often tend to miss is the monitoring aspect. Which is when you put your LLM applications into production, you want to be able to know if there’s regressions, performance degradations. If your application is not performing the way it should, so monitoring is the other pillar of building LLM applications.
Srini Penchikala: Sounds good. Once the developers start work on these applications, I think first thing they should probably do is the evaluation of the application.
Apoorva Joshi: Yes.
Evaluation of LLM-based Applications [08:02]
Srini Penchikala: What is the scope? What are the benchmarks? Because the metrics and service level agreements (SLAs) and response times can be different for different applications. Can you talk about evaluation of LLM-based applications, like what developers should be looking for? Are there any metrics that they should be focusing on?
Apoorva Joshi: Yes. I think anything with respect to LLMs is such a vast area because they’ve just opened up the floodgates for being used across multiple different domains and tasks. Evaluation is no different.
If you think of traditional ML models, like a classification or regression models, you had very quantifiable metrics that applied to any use case. For classification, you would have accuracy, precision recall. Or if you were building a regression model, you had means squared error, that kind of thing. But with LLMs, all that’s out of the window. Now the responses from these models are natural language, or an image, or some generated commodity. The metrics, when it comes to LLMs, are hard to quantify.
For example, if they’re generating a piece of text for a Q&A-based application, then metrics like how coherent is the response, how factual is the response, or what is the relevance of the information provided in the response. All of these become more important metrics and these are unfortunately pretty hard to quantify.
There’s two techniques that I’m seeing in the space broadly. One is this concept of LLM as a judge. The premise there is because LLMs are good at identifying patterns and interpreting natural language, they can be also used as an evaluation mechanism for natural language responses.
The idea there is to prompt an LLM on how you wanted to go about evaluating responses for your specific task and dataset, and then use the LLM to generate some sort of scoring paradigm on your data. I’ve also seen organizations that have more advanced data science teams actually putting the time and effort into creating fine-tuned models for evaluation. But yes, that’s typically reserved for teams that have the right expertise and knowledge to build a fine-tuned model because that’s a bit more involved than prompting.
Domain-specific Language Models [10:31]
Srini Penchikala: Yes. You mentioned domain-specific models. Do you see, I think this is one of my predictions, that the industry will start moving towards domain-specific language models? Like healthcare would have their own healthcare LLM, and the insurance industry would have their own insurance language model.
Apoorva Joshi: I think that’s my prediction, too. Coming from this domain, I was in cybersecurity, I used to do a lot of that. This was in the world when BERT was supposed to be a large language model. A lot of my work was also on fine-tuning those language models on cybersecurity-specific data. I think that’s going to start happening more and more.
I already see signals for that happening because let’s take the example of natural language to query. That’s a pretty common thing that folks are trying to do. I’ve seen that usually, with prompting or even something like RAG, you can achieve about, I would say, 90 to 95 percent accuracy or recall on slightly complicated tasks. But there’s a small set of tasks that are just not possible by just providing the LLM with the right information to generate responses.
For some of those cases, and more importantly for domain-specific use cases, I think we are going to pretty quickly move towards a world where there’s smaller specialized models, and then maybe an agent that’s orchestrating and helping facilitate the communication between all of them.
LLM Based Application Performance Improvements [12:02]
Srini Penchikala: Yes, definitely. I think it’s a very interesting time not only with these domain-specific models taking shape, and the RAG techniques now, you can use these base models and apply your own data on that. Plus, the agents taking care of a lot of these activities on their own, automation type of tasks. Definitely that’s really good. Thanks, Apoorva, for that.
Regarding the application performance itself, what are the high level considerations and strategies that teams should be looking at before they jump into optimizing or over-optimizing? What are the performance concerns that you see the teams are running into and what areas they should be focusing on?
Apoorva Joshi: Most times, I see teams asking about three things. There’s accuracy, latency, and cost. When I say accuracy, what I really mean is performance on metrics that apply to a particular business use case. It might not be accuracy, it might be, I don’t know, factualness or relevance. But yes, you get the drift. Because that’s how it is, because there are so many different use cases, it really comes down to first determining what your business cares about, and then coming up with metrics that resonate with that use case.
For example, if you’re building a Q&A chatbot, your evaluation parameters would be mainly faithfulness and relevance. But say you’re building a content moderation chatbot, then you care more about recall on toxicity and bias, for example. I think that’s the first big step.
Improvements here could be, again, depend on what you end up finding are the gaps of the model. Say you’re evaluating a RAG system, you would want to evaluate the different components of the system itself first, in addition to the overall evaluation of the system. When you think of RAG, there’s two components, retrieval and generation. You want to evaluate the retrieval performance separately to see if your gap lies in the retrieval strategy itself or do you need a different embedding model. Then you evaluate the generation to see what the gaps on the generation front are, to see what improvements you need to do there.
I think work backwards. Evaluate as many different components of the system as possible to identify the gaps. And then work backwards from there to try out a few different techniques to improve the performance on the accuracy side. Guardrails are an important one to make sure that the LLM is appropriately responding or not responding to sensitive or off-topic questions.
In agentic applications, I’ve seen folks also implement things like self-reflection and critiquing loops to have the LLM reflect and improve upon its own response. Or even human in the loop workflows, too. Get human feedback and incorporate that as a strategy to improve the response.
Maybe I’ll stop there to see if you have any follow-ups.
Choosing Right Embedding Model [15:02]
Srini Penchikala: Yes. No, that’s great. I think the follow-up is basically we can jump into some of those specific areas of the process. One of the steps is choosing the right embedding model. Some of these tools come with … I was trying out the Spring AI framework the other day. It comes with a default embedding model. What do you see there? Are there any specific criteria we should be using to pick one embedding model for one use case versus a different one for a different use case?
Apoorva Joshi: My general thumb rule would be to find a few candidate models and evaluate them for your specific use case and dataset. For text data, my recommendation would be to start from something like the massive text embedding, or MTEB Benchmark on Hugging Face. It’s essentially a leader board that shows you how different proprietary and open source embedding models perform on different tasks, such as retrieval, classification, and clustering. It also shows you the model size and dimensions.
Yes. I would say choose a few and evaluate for performance and, say latency if that’s a concern for you. Yes, there’s similar ones for multi-modal models as well. Until recently, we didn’t have good benchmarks for multi-modal, but now we have things like MME, which is a pretty good start.
Srini Penchikala: Yes. Could we talk about, real quick, about the benchmarks? When we are switching these different components of the LLM application, what standard benchmarks can we look at or run to get the results and compare?
Apoorva Joshi: I think benchmarks apply to the models themselves more than anything else. Which is why, when you’re looking to choose models for your specific use case, you take that with a grain of salt because the tasks that are involved in a benchmark. If you look at the MMLU Benchmark, it’s mostly a bunch of academic and professional examinations, but that might not necessarily be the task that you are evaluating for. I think benchmarks mostly apply for LLMs, but LLM applications are slightly different.
Srini Penchikala: You said earlier the observability or the monitoring. If you can build it into the application right from the beginning, it will definitely help us pinpoint any performance problems or any latencies.
Apoorva Joshi: Exactly.
Data Chunking Strategies [17:18]
Srini Penchikala: Another technique is how the data is divided or chunked into smaller segments. You published an article on this. Can you talk about this a little bit more, and tell us what are some of the chunking strategies for implementing the LLM apps?
Apoorva Joshi: Sure, yes. I think my disclaimer from before, with LLMs the answer starts from it depends, and then you pick and choose. I think that’s the thumb rule for anything when it comes to LLMs. Pick and choose a few, evaluate on your dataset and use case, and go from there.
Similarly for chunking, it depends on your specific data and use case. For most text, I typically suggest starting with this technique called recursive token with overlap, with say a 200-ish token size for chunks. What this does is it has the effect of keeping paragraphs together with some overlap at the chunk boundaries. This, combined with techniques such as parent document or contextual retrieval could potentially work well if you’re working with mostly text data. Semantic chunking is another fascinating one for text where you try to find or align the chunk boundaries with the semantic boundaries of your text.
Then there’s semi-structured data, which is data containing a combination of text, images, tables. For that, I’ve seen folks retrieve the text and non-textual components using specialized tools. There’s one called Unstructured that I particularly like. It supports a bunch of different formats and has different specialized models for extracting components present in different types of data. Yes, I would use a tool like that.
Then once you have those different components, maybe chunk the text as you would normally do. Then, two ways to approach the non-textual components. You either maybe summarize the images and tables to get everything in the text domain, or use multi-modal embedding models to embed the non-text elements as is.
Srini Penchikala: Yes, definitely. Because if we take the documents and if we chunk them into too small of segments, the context may be lost.
Apoorva Joshi: Exactly.
Srini Penchikala: If you provide a prompt, the response might not be exactly what you were looking for.
Apoorva Joshi: Right.
RAG Application Improvements [19:40]
Srini Penchikala: What are the other, especially if you’re using a RAG-based application which is probably the norm these days for all the companies … They’re all taking some kind of foundation model and ingesting their company data, incorporating it on top of it. What are the other strategies are you seeing in the RAG applications in terms of retrieval or generation steps?
Apoorva Joshi: There’s a lot of them coming every single day, but I can talk about the ones I have personally experimented with. The first one would be hybrid search. This is where you combine the results from multiple different searches. It’s commonly a combination of full text and vector search, but it doesn’t have to be that. It could be vector and craft-based. But the general concept of that is that you’re combining results from multiple different searches to get the benefits of both.
This is useful in, say ecommerce applications for example, where users might search for something very specific. Or include keywords in their natural language queries. For example, “I’m looking for size seven red Nike running shoes”. It’s a natural language query, but it has certain specific points of focus or keywords in them. An embedding model might not capture all of these details. This is where combining it with something like a full text search might make sense.
Then there’s parent document retrieval. This is where you embed and store small chunks at storage and ingest time, but you fetch the full source document or larger chunks at retrieval time. This has the effect of providing a more complete context to the LLM while generating responses. This might be useful in cases such as legal case prep or scientific research documentation chatbots where the context surrounding the user’s question can result in more rounded responses.
Finally, there’s graph RAG that I’ve been hearing about a lot lately. This is where you structure and store your data as a knowledge graph, where the nodes can be individual documents or chunks. Edges capture which nodes are related and what the relationship between the nodes is. This is particularly common in specialized domains such as healthcare, finance, legal, or anywhere where multi-hop reasoning or if you need to do some sort of root cause analysis or causal inference is required.
Srini Penchikala: Yes, definitely. The graph RAG has been getting a lot of attention lately. The power of knowledge graph in the RAG.
Apoorva Joshi: But that’s the thing. Going back to what you said earlier on, what’s the hype versus what people should be hyped about. I think a lot of organizations have a hard time balancing that too, because they want to be at the bleeding-edge of building these applications. But then sometimes, it might just be overkill to use the hottest technique.
Srini Penchikala: Where should development teams decide, “Hey, we started with an LLM-based application in mind, but my requirements are not a good fit?” What are those, I don’t want to call them limitations, but what are the boundaries where you say, “For now, let’s just go with the standard solution rather than bringing some LLM in to make it more complex?”
Apoorva Joshi: This is not just an LLM thing. Even having spent six years as a data scientist, a lot of times … ML in general, for the past decade or so, it’s just been a buzzword. Sometimes people just want to use it for the sake of using it. That’s where I think you need to bring a data scientist or an expert into the room and be like, “Hey, this is my use case”, and have them evaluate whether or not you even need to use machine learning, or in this case gen AI for it.
Going from traditional to gen AI, now there’s more of a preference to generative AI as well. I think at this point, the decision is, “Can I use a small language model or just use an XG boost and get away with it? Or do I really need a RAG use case?”
But I think in general, if you want to reason and answer questions using natural language on a repository of text, then I agree, some sort of generative AI use case is important. But say you’re basically just trying to do classification, or just doing something like anomaly detection or regression, then just because an LLM can do it doesn’t mean you should, because it might not be the most efficient thing at the end of the day.
Srini Penchikala: The traditional ML solutions are still relevant, right?
Apoorva Joshi: Yes. For some things, yes.
I do want to say the beauty of LLMs is that it’s made machine learning approachable to everyone. It’s not limited to data scientists anymore. A software engineer or PM, someone who’s not technical, they can just use these models without having to fine-tune or worry about the weights of the model. Yes, I think that results in these pros and cons, in a sense.
Srini Penchikala: Yes, you’re right. Definitely these LLM models and these applications that use them have brought the value of these to the masses. Now everybody can use ChatGPT or CoPilot and get the value out of it.
Apoorva Joshi: Yes.
Frameworks and Tools for LLM applications [25:03]
Srini Penchikala: Can you recommend any open source tools and frameworks for our audience to try out LLM applications if they want to learn about them before actually starting to use them?
Apoorva Joshi: Sure, yes. I’m trying to think what the easiest stack would be. If you’re looking at strictly open source, you don’t want to put down a credit card to just experiment and build a prototype, then I think three things. You first need a model of some sort, whether it’s embedding or LLMs.
For that, I would say use something like Hugging Face. Pretty easy to get up and running with their APIs. You don’t have to pay for it. Or if you want to go a bit deeper and try out something local, then Ollama has support for a whole bunch of open source models. I like LangGraph for orchestration. It’s something LangChain came up with a while ago. A lot of people think it’s an agent orchestration framework only, but I have personally used it for just building control flows. I think you could even build a RAG application by using LangGraph. It just gives you low-level control on the flow of your LLM application.
For vector databases, if you’re looking for something that’s really quick and open source, and easy to start with, then you could even start with something like Chroma or FAISS for experimentation. But of course, when you move from the prototype of putting something in production, you would want to consider enterprise-grade databases such as my employer.
Srini Penchikala: Yes, definitely. For local, just to get started, even Postgres has a vector flavor of the database called PG Vector.
Apoorva Joshi: Right.
Srini Penchikala: Then there’s Quadrant and others. Yes.
Apoorva Joshi: Yes.
Srini Penchikala: Do you have any metrics, or benchmarks, or resources that teams can use to look at, “Hey, I just want to see what are the top 10 or top five LLMs before I even start work on this?”
Apoorva Joshi: There’s an LLM similar to, what’s the one you were mentioning?
Srini Penchikala: The one I mentioned is Open LLM Leaderboard.
Apoorva Joshi: There’s a similar one on Hugging Face that I occasionally look at. It’s called LLM LMSYS Chatbot Arena. That’s basically a crowdsourced list of evaluation of different proprietary and open source LLMs. I think that’s a good thing to look at than just performance on benchmarks because benchmarks can have data contamination.
Sometimes vendors will actually train their models on benchmark data so certain models could end up looking good on certain tasks than they actually are. Which is why leader boards such as the one you mentioned and LMSYS are good because it’s actually people trying these models on real world prompts and tasks.
Srini Penchikala: Just like anything else, teams should try it out first and then see if it works for their use case and their requirements, right?
Apoorva Joshi: Yes.
Online Resources [27:58]
Srini Penchikala: Other than that, any other additional resources on LLM application performance improvements and evaluation? Any online articles or publications?
Apoorva Joshi: I follow a couple of people and read their blogs. There’s this person called Eugene Yan. He’s an applied scientist at Amazon. He has a blog and he’s written extensively about evals and continues to do extensive research in that area. There’s also a bunch of people in the machine learning community who had written almost a white paper titled What We Learned from a Year of Building With LLMs. It’s just really technical practitioners who’ve written that white paper based on their experience building LLMs in the past year. Yes. I generally follow a mix of researches and practitioners in the community.
Srini Penchikala: Yes, I think that’s a really good discussion. Do you have any additional comments before we wrap up today’s discussion?
Apoorva Joshi: Yes. Our discussion made me realize just how important evaluation is when building just any software application, but LLMs specifically because while they’ve made ML accessible and usable in so many different domains, what you really need on a day-to-day is for the model or application to perform on the use case or task you need. I think evaluating for what you’re building is key.
Srini Penchikala: Also, another key is your LLM mileage may vary. It all depends on what you’re trying to do, and what are the constraints and the benchmarks that are working towards.
Apoorva Joshi: Exactly.
Srini Penchikala: Apoorva, thank you so much for joining this podcast. It’s been great to discuss one of the very important topics in the AI space, how to evaluate the LLM applications, how to measure the performance, and how to improve their performance. These are practical topics that everybody is interested in, not just another Hello World application or ChatGPT tutorial.
Apoorva Joshi: Yes.
Srini Penchikala: Thank you for listening to this podcast. If you’d like to learn more about AI and ML topics, check out the AI, ML, and data engineering community page on infoq.com website. I also encourage you to listen to the recent podcasts, especially the 2024 AI ML Trends Report we published last year. And also, the 2024 Software Trends Report that we published just after the new year’s. Thank you very much. Thanks for your time. Thanks, Apoorva.
Apoorva Joshi: Yes. Thank you so much for having me.
Mentioned:
.
From this page you also have access to our recorded show notes. They all have clickable links that will take you directly to that part of the audio.
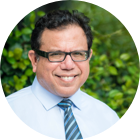
MMS • Sergio De Simone
Article originally posted on InfoQ. Visit InfoQ
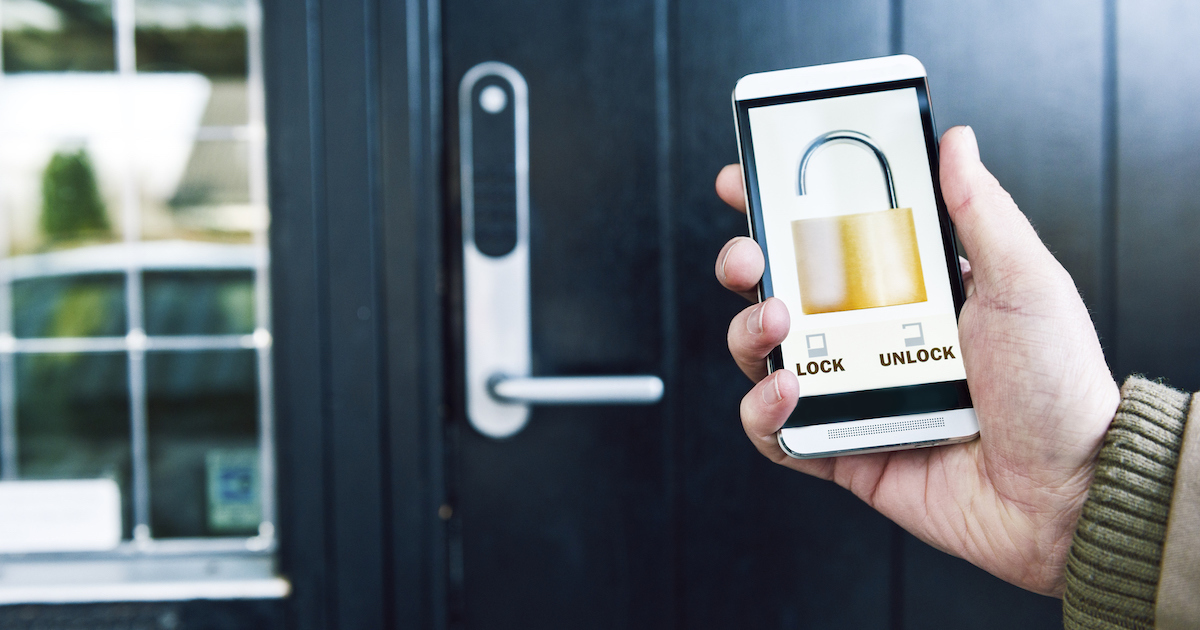
Three months after its launch in beta, the Vertex AI in Firebase SDK is now ready for production, says Google engineer Thomas Ezan, who further explores three dimensions that are essential for its successful deployment to production: abuse prevention, remote configuration, and responsible AI use.
The Vertex AI in Firebase SDK aims to facilitate the integration of Gemini AI into Android and iOS apps by providing idiomatic APIs, security against unauthorized use, and integration with other Firebase services. By integrating Gemini AI, developers can build AI features into their apps, including AI chat experiences, AI-powered optimization and automation, and more.
A few apps are already using the SDK, explain Ezan, including Meal Planner, which creates original meal plans using AI; the journal app Life, which aims to be an AI diary assistant able to convert conversations into journal entries; and hiking app HiiKER.
Although using an AI service may seem easy, it comes with a few critical specific responsibilities, namely implementing robust security measures to prevent unauthorized access and misuse, preparing for the quick evolution of Gemini models by using remote configuration, and using AI responsibly.
To ensure your app is protected against unauthorized access and misuse, Google provides Firebase App Check:
Firebase App Check helps protect backend resources (like Vertex AI in Firebase, Cloud Functions for Firebase, or even your own custom backend) from abuse. It does this by attesting that incoming traffic is coming from your authentic app running on an authentic and untampered Android device.
The App Check server verifies the attestation using parameters registered with the app and then returns a token with an expiration time. The client caches the token to use it with subsequent requests. In case a request is received without an attestation token, it is rejected.
Remote configuration can be useful to take care of model evolution as well as other parameters that could require to be updated at any time, such as maximum tokens, temperature, safety settings, system instructions, and prompt data. Other important cases where you will want to parametrize your app’s behavior are setting the model location closer to the users, A/B testing system prompts and other model parameters, enabling and disabling AI-related features, etc.
Another key practice highlighted by Ezan is user feedback collection to evaluate user impact:
As you roll out your AI-enabled feature to production, it’s critical to build feedback mechanisms into your product and allow users to easily signal whether the AI output was helpful, accurate, or relevant.
Examples of this are including thumb-up and thumb-down buttons and detailed feedback forms in your app UI.
Last but not least, says Ezan, there is responsibility, which means you should be transparent about AI-based features, you should ensure your users’ data is not used by Google to train their models, and highlight the possibility of unexpected behavior.
All in all, the Vertex AI in Firebase SDK provides an easy road into creating AI-powered mobile apps without developers having to deal with the complexity of Google Cloud or switch to a different programming language to implement an AI backend. However, the Vertex AI in Firebase SDK does not support more advanced use cases, such as streaming, and has a simplified API that is close to direct LLM calls. This makes it less flexible out-of-the-box to build agents, chatbots, or automation. If you need to support streaming or more complex interactions, you can consider using Google GenKit, which additionally offers a free tier for testing purposes.
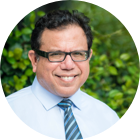
MMS • Renato Losio
Article originally posted on InfoQ. Visit InfoQ
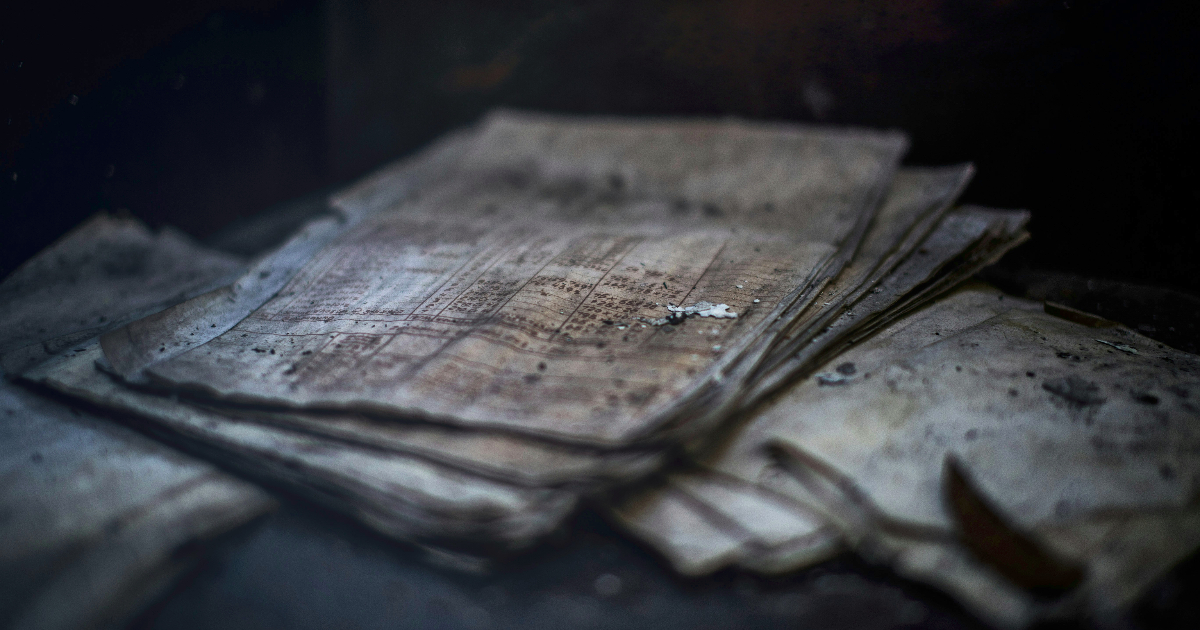
Cloudflare recently published an article detailing their upgrade of developer documentation by migrating from Hugo to the Astro ecosystem. All Cloudflare documentation is open source on GitHub, with opportunities for community contributions.
The developers.cloudflare.com site was previously consolidated from a collection of Workers Sites into a single Cloudflare Pages instance. The process used tools like Hugo and Gatsby to convert thousands of Markdown pages into HTML, CSS, and JavaScript. Kim Jeske, head of product content at Cloudflare, Kian Newman-Hazel, document platform engineer at Cloudflare, and Kody Jackson, technical writing manager at Cloudflare, explain the reasons behind the change in the web framework:
While the Cloudflare content team has scaled to deliver documentation alongside product launches, the open source documentation site itself was not scaling well. developers.cloudflare.com had outgrown the workflow for contributors, plus we were missing out on all the neat stuff created by developers in the community.
In 2021, Cloudflare adopted a “content like a product” strategy, emphasizing the need for world-class content that anticipates user needs and supports the creation of accessible products. Jeske, Newman-Hazel, and Jackson write:
Open source documentation empowers the developer community because it allows anyone, anywhere, to contribute content. By making both the content and the framework of the documentation site publicly accessible, we provide developers with the opportunity to not only improve the material itself but also understand and engage with the processes that govern how the documentation is built, approved, and maintained.
According to the team, Astro’s documentation theme, Starlight, was a key factor in the decision to migrate the documentation site: the theme offers powerful component overrides and a plugin system to utilize built-in components and base styling. Jeremy Daly, director of research at CloudZero, comments:
Cloudflare has open sourced all their developer documentation and migrated from Hugo to the Astro, with the JavaScript ecosystem claiming another victim. No matter how good your documentation is, user feedback is essential to keeping it up-to-date and accessible to all.
According to the Cloudflare team, keeping all documentation open source allows the company to stay connected with the community and quickly implement feedback, a strategy not commonly shared by other hyperscalers. As previously reported on InfoQ, AWS shifted its approach after maintaining most of its documentation as open source for five years. In 2023, the cloud provider retired all public GitHub documentation, citing the challenge of keeping it aligned with internal versions and the manual effort required to sync with GitHub repositories. Jeff Barr, chief evangelist at AWS, wrote at the time:
The overhead was very high and actually consumed precious time that could have been put to use in ways that more directly improved the quality of the documentation.
Gianluca Arbezzano, software engineer at Mathi, highlights the significance of the topic:
If you are thinking: “it is just documentation”, I think you should care a bit more! We deserve only the best! Nice article from Cloudflare about their migration from Hugo to Astro.
Commenting on the Cloudflare article on Hacker News, Alex Hovhannisyan cautions:
I’m sorry but I have to be honest as someone who recently migrated from Netlify (and is considering moving back): the documentation is not very good, and your tech stack has nothing to do with it. End users don’t care what tech stack you use for your docs.
All Cloudflare documentation is available at developers.cloudflare.com.
Surprising Stock Swings: Missed Opportunities and Bold Moves Shake the Market – elblog.pl
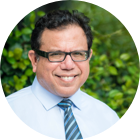
MMS • RSS
Posted on mongodb google news. Visit mongodb google news
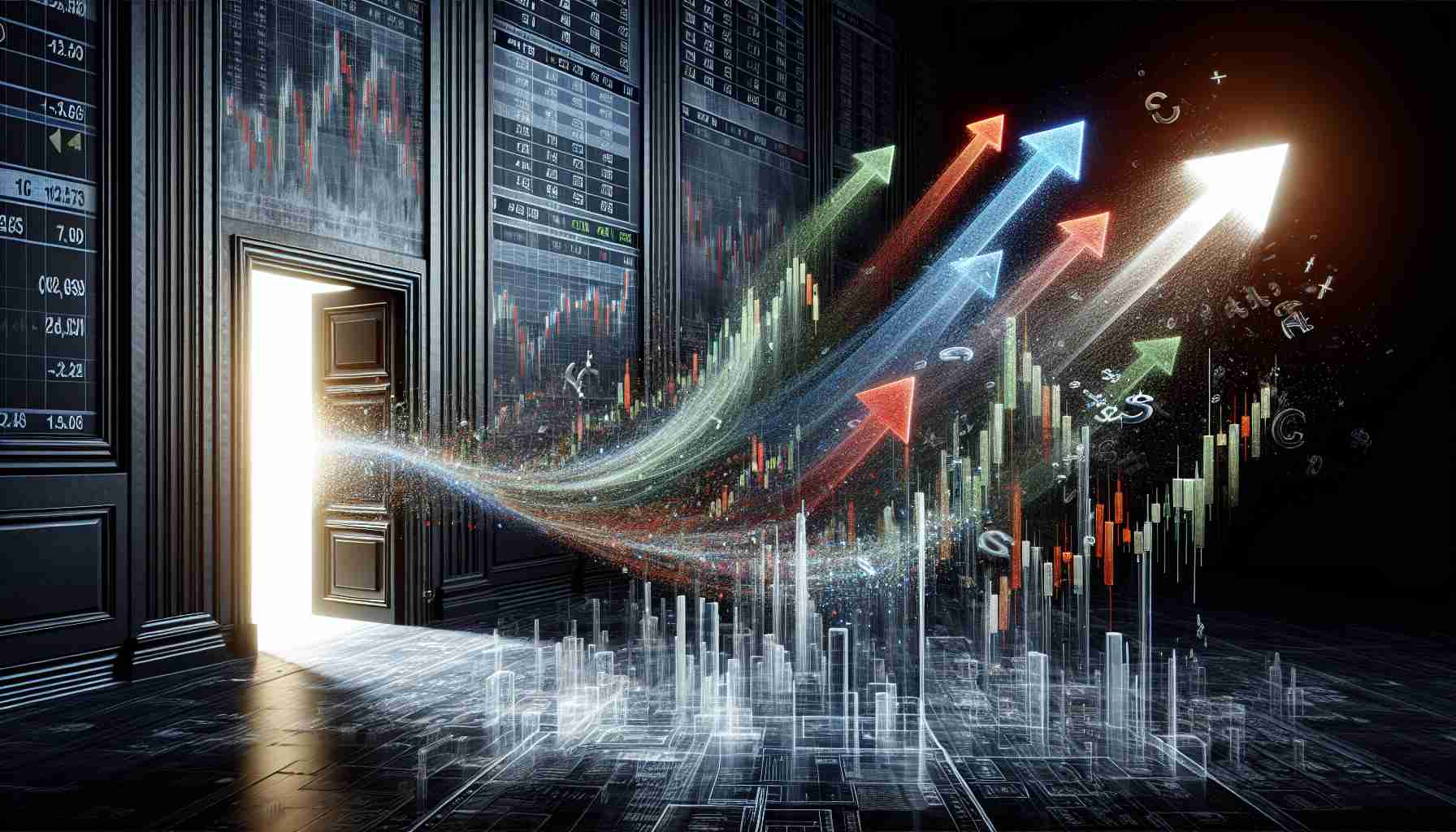
- Momentum stocks experienced setbacks, with the S&P 500 dropping as companies like AppLovin and Palantir faced losses.
- China’s economic policies spark mixed reactions; success could boost companies like Danaher and GE Healthcare.
- DuPont’s plan to split into three entities signals potential growth and innovation.
- Oracle’s report is expected to impact AI stocks, with investor focus also on MongoDB and Toll Brothers.
- CNBC Investing Club offered strategic trade alerts for smarter market navigation.
- Investors are advised to remain cautious, balancing opportunities in AI and restructuring with global uncertainties.
In a week filled with dynamic market shifts, investors watched as momentum stocks hit unexpected roadblocks. The S&P 500 dipped as major players like AppLovin tumbled over 11%, narrowly missing entry into the esteemed index. Traders who speculated on index-related profits found themselves in disappointment, while stocks like Palantir saw notable declines despite initial premarket strength.
Across the globe, all eyes were on China’s economic pulse. The government’s promises of a ‘moderately loose’ monetary policy paired with a ‘proactive’ fiscal stance brought a mix of hope and skepticism. If China successfully rolls out these policies, companies like Danaher and GE Healthcare could see a resurgence in demand, but history urges cautious optimism.
Domestic markets buzzed with DuPont’s bold announcement to split into three distinct entities, shedding light on potentially untapped value. This strategic maneuver hints at a future of sharper focus and innovation, igniting investor interest.
On the tech front, Oracle’s upcoming performance report carries the potential to stir excitement in AI stocks once more. Investors are keenly awaiting the outcomes from other big names like MongoDB and Toll Brothers, poised to sway market sentiment with their insights.
Adding a strategic edge, CNBC Investing Club members received preemptive trade alerts, offering them a chance to navigate the market landscape smarter and faster than the average investor.
As market trends unfold, a conservative approach is advised. While intriguing opportunities in AI and company restructurings like DuPont’s restructure are promising, balancing optimism with vigilance in light of global uncertainties is crucial. Stay informed and ahead by continually engaging with reputable financial news.
The Invisible Forces Shaping Market Waves: What You Need to Know Now
Navigating Market Volatility: Key Insights and Strategies
In recent market developments, investors faced unexpected challenges as momentum stocks hit unforeseen obstacles. The S&P 500 experienced a dip, primarily due to significant losses by companies like AppLovin, which fell over 11% and narrowly missed inclusion in this prestigious index. Speculators banking on index-driven gains faced disappointments, particularly as stocks such as Palantir slipped despite their promising premarket performance.
Concurrently, global attention was riveted on China’s economic strategies. The government’s commitment to a ‘moderately loose’ monetary policy combined with a ‘proactive’ fiscal approach sparked both optimism and skepticism. Should China effectively implement these policies, there may be renewed demand for firms like Danaher and GE Healthcare. However, caution is advised given the potential risks associated with such economic shifts.
Domestically, DuPont made a strategic move by announcing its plan to split into three separate entities. This could unlock previously untapped value, offering a future rife with focused innovation and drawing significant interest from investors.
On the tech front, Oracle’s upcoming performance report is set to potentially reignite interest in AI stocks. There’s keen anticipation around results from MongoDB and Toll Brothers, as these could significantly influence market sentiment.
In a proactive measure, CNBC Investing Club members received early trade alerts, enabling them to maneuver the market landscape more effectively than the average investor.
Given these trends, a conservative investment approach is recommended. The allure of opportunities within AI and strategic company restructurings, like DuPont’s, is clear. However, it’s essential to balance optimism with cautious vigilance amidst global uncertainties, ensuring informed and strategic investment decisions.
Key Questions Answered
1. What are the implications of China’s economic policies for global markets?
China’s pursuit of a ‘moderately loose’ monetary policy paired with a ‘proactive’ fiscal stance is designed to stimulate its domestic economy. Should these policies succeed, they may bolster demand for both international and local companies, particularly in the healthcare and technology sectors. However, past experiences suggest that investors should remain cautiously optimistic to mitigate potential risks.
2. How might DuPont’s restructuring impact investor strategies?
DuPont’s decision to divide into three distinct entities is aimed at creating more specialized and agile business units. This restructuring could unlock hidden value, increase efficiency, and inspire innovation across each unit. For investors, this could mean accessing more focused investment opportunities within DuPont’s spectrum, potentially leading to higher returns if executed successfully.
3. What role does Oracle play in the AI stock market?
Oracle’s performance and developments in AI technology are closely watched by the investment community. The company’s upcoming performance report could act as a catalyst for renewed interest in AI stocks, influencing market trends. Oracle’s strategies and earnings could signal the broader trajectory of AI investments, affecting how investors view potential opportunities and risks in this rapidly evolving sector.
Related Links
– CBC Investment News: Stay updated with business news and analysis.
– DuPont: Learn more about DuPont’s strategic initiatives and corporate developments.
– Oracle: Discover Oracle’s latest advancements and performance insights in the tech industry.
For investors, the ability to adapt and thrive amidst these changes hinges on staying informed and diligently scrutinizing each potential investment avenue. Balancing optimism with caution remains critical as market forces continue to evolve.
Article originally posted on mongodb google news. Visit mongodb google news
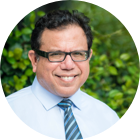
MMS • RSS
Posted on mongodb google news. Visit mongodb google news
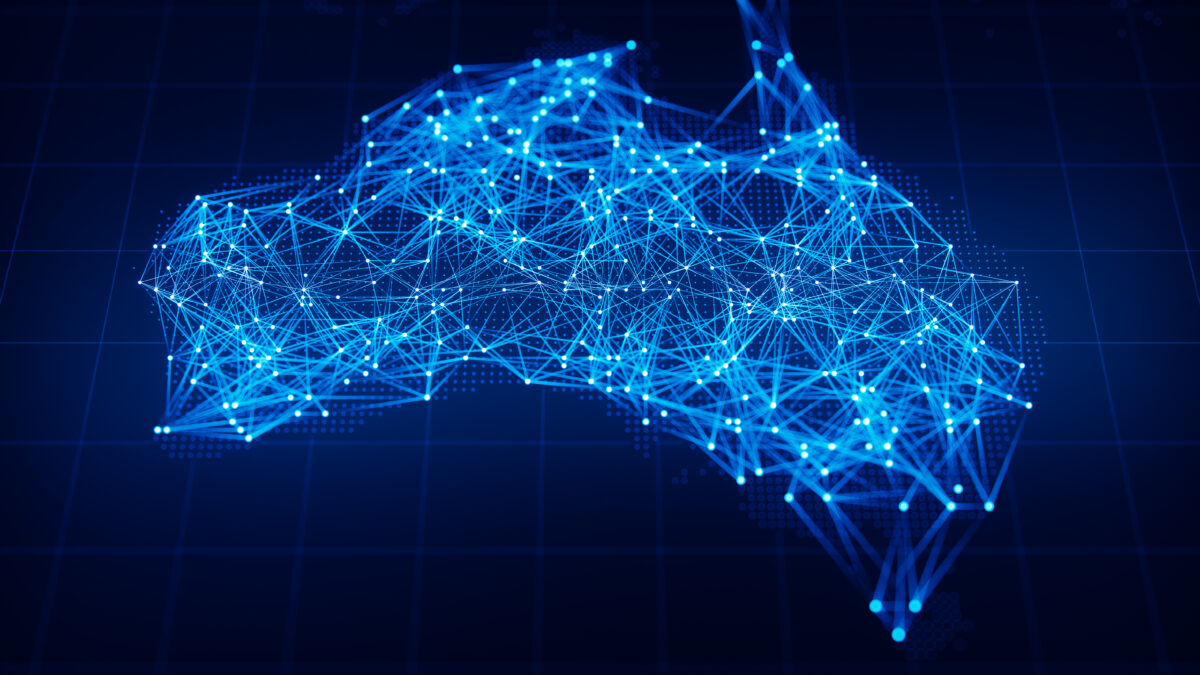
Banked, a global provider of Pay by Bank solutions, has partnered with National Australia Bank (NAB) to launch the PayTo payment option at Amazon’s Australian store.
The initiative aligns with the global rise in account-to-account (A2A) payment transactions, projected to reach 186 billion by 2029 due to their lower costs, enhanced security and better user experience.
Their collaboration is set to raise the profile of Pay by Bank in Australia, using Amazon’s platform to familiarise consumers with this payment method. Customers can now make direct bank-to-bank transactions when shopping on Amazon.com.au, offering a payment experience without the need for card details.
Brad Goodall, CEO of Banked, commented: “Enabling Amazon and NAB to launch PayTo in Australia is a huge step in cementing our position as a truly global Pay by Bank platform. Australia is an important market for us and we have worked closely with NAB to ensure Amazon’s PayTo sets a worldwide benchmark for account-to-account payments at scale.
“As more consumers become aware and familiarise themselves with the Pay by Bank experience through major brands like Amazon, we will see a snowball effect of uptake. This announcement today between NAB and Amazon will leapfrog Australia into a commanding position as an account-to-account payments global leader.”
Using ‘PayTo’
Customers shopping on Amazon.com.au now have the option to use ‘PayTo’ for Pay by Bank transactions directly from their bank accounts. This method bypasses the need for card details, aiming to enhance transaction security and user control. The ‘PayTo’ feature also allows for both visibility and control by enabling secure authorisation of transactions through the customer’s online banking platform.
Once set up as a payment method in their online banking, customers can initiate either one-off or recurring payments directly from their bank account with a single click, processed in real time.
Jon Adams, NAB executive, enterprise payments, also said: “It has been a pleasure working with the Banked team on this implementation. They understand tier one merchants and their global insight and experience puts NAB in a great position to provide the scale, security and customer experience that consumers and merchants like Amazon demand from their payment experiences.”
The Amazon launch caps Banked’s recent expansion in Australia through a partnership with NAB, aimed at boosting account-to-account payments for local merchants. This move also follows Banked’s acquisition of the Australian payment firm Waave, and precedes a strategic partnership with Chemist Warehouse to enhance the Pay by Bank experience by 2025.
Article originally posted on mongodb google news. Visit mongodb google news
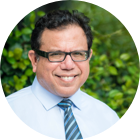
MMS • RSS
Posted on mongodb google news. Visit mongodb google news
MongoDB, Inc. (NASDAQ:MDB – Get Free Report) traded up 7.4% during mid-day trading on Tuesday . The stock traded as high as $285.10 and last traded at $284.28. 819,510 shares were traded during trading, a decline of 43% from the average session volume of 1,427,558 shares. The stock had previously closed at $264.58.
Wall Street Analysts Forecast Growth
Several equities research analysts have weighed in on the company. China Renaissance began coverage on MongoDB in a research note on Tuesday, January 21st. They set a “buy” rating and a $351.00 target price for the company. Loop Capital upped their price objective on MongoDB from $315.00 to $400.00 and gave the stock a “buy” rating in a research report on Monday, December 2nd. KeyCorp lifted their target price on MongoDB from $330.00 to $375.00 and gave the company an “overweight” rating in a research report on Thursday, December 5th. Royal Bank of Canada increased their target price on shares of MongoDB from $350.00 to $400.00 and gave the stock an “outperform” rating in a report on Tuesday, December 10th. Finally, Monness Crespi & Hardt lowered shares of MongoDB from a “neutral” rating to a “sell” rating and set a $220.00 price target on the stock. in a report on Monday, December 16th. Two analysts have rated the stock with a sell rating, four have assigned a hold rating, twenty-three have given a buy rating and two have given a strong buy rating to the company’s stock. According to data from MarketBeat, the stock has a consensus rating of “Moderate Buy” and a consensus target price of $361.00.
Check Out Our Latest Report on MDB
MongoDB Stock Performance
The stock has a market capitalization of $20.35 billion, a P/E ratio of -99.75 and a beta of 1.25. The business has a fifty day simple moving average of $272.39 and a 200-day simple moving average of $269.78.
MongoDB (NASDAQ:MDB – Get Free Report) last posted its quarterly earnings results on Monday, December 9th. The company reported $1.16 EPS for the quarter, topping analysts’ consensus estimates of $0.68 by $0.48. MongoDB had a negative net margin of 10.46% and a negative return on equity of 12.22%. The business had revenue of $529.40 million during the quarter, compared to analysts’ expectations of $497.39 million. During the same period last year, the company posted $0.96 earnings per share. The business’s quarterly revenue was up 22.3% on a year-over-year basis. On average, sell-side analysts anticipate that MongoDB, Inc. will post -1.78 earnings per share for the current year.
Insider Activity
In other MongoDB news, Director Dwight A. Merriman sold 3,000 shares of the business’s stock in a transaction that occurred on Monday, November 4th. The stock was sold at an average price of $269.57, for a total transaction of $808,710.00. Following the completion of the sale, the director now directly owns 1,127,006 shares of the company’s stock, valued at approximately $303,807,007.42. The trade was a 0.27 % decrease in their position. The sale was disclosed in a document filed with the SEC, which is accessible through this hyperlink. Also, CEO Dev Ittycheria sold 8,335 shares of the firm’s stock in a transaction that occurred on Friday, January 17th. The shares were sold at an average price of $254.86, for a total value of $2,124,258.10. Following the completion of the transaction, the chief executive officer now owns 217,294 shares in the company, valued at $55,379,548.84. This represents a 3.69 % decrease in their position. The disclosure for this sale can be found here. Insiders sold a total of 42,491 shares of company stock worth $11,554,190 over the last quarter. 3.60% of the stock is currently owned by corporate insiders.
Hedge Funds Weigh In On MongoDB
Several large investors have recently bought and sold shares of MDB. Hilltop National Bank raised its holdings in shares of MongoDB by 47.2% in the fourth quarter. Hilltop National Bank now owns 131 shares of the company’s stock valued at $30,000 after purchasing an additional 42 shares during the last quarter. Quarry LP lifted its position in shares of MongoDB by 2,580.0% during the 2nd quarter. Quarry LP now owns 134 shares of the company’s stock worth $33,000 after buying an additional 129 shares in the last quarter. Brooklyn Investment Group purchased a new position in shares of MongoDB in the 3rd quarter worth approximately $36,000. GAMMA Investing LLC grew its holdings in shares of MongoDB by 178.8% in the third quarter. GAMMA Investing LLC now owns 145 shares of the company’s stock valued at $39,000 after acquiring an additional 93 shares in the last quarter. Finally, Continuum Advisory LLC increased its position in shares of MongoDB by 621.1% during the third quarter. Continuum Advisory LLC now owns 137 shares of the company’s stock valued at $40,000 after acquiring an additional 118 shares during the last quarter. 89.29% of the stock is currently owned by institutional investors.
About MongoDB
MongoDB, Inc, together with its subsidiaries, provides general purpose database platform worldwide. The company provides MongoDB Atlas, a hosted multi-cloud database-as-a-service solution; MongoDB Enterprise Advanced, a commercial database server for enterprise customers to run in the cloud, on-premises, or in a hybrid environment; and Community Server, a free-to-download version of its database, which includes the functionality that developers need to get started with MongoDB.
See Also
Before you consider MongoDB, you’ll want to hear this.
MarketBeat keeps track of Wall Street’s top-rated and best performing research analysts and the stocks they recommend to their clients on a daily basis. MarketBeat has identified the five stocks that top analysts are quietly whispering to their clients to buy now before the broader market catches on… and MongoDB wasn’t on the list.
While MongoDB currently has a “Moderate Buy” rating among analysts, top-rated analysts believe these five stocks are better buys.
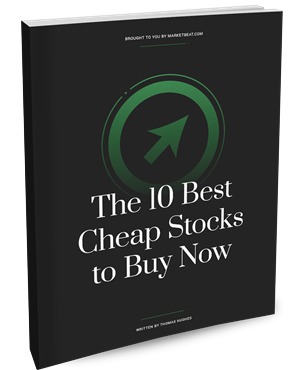
MarketBeat just released its list of 10 cheap stocks that have been overlooked by the market and may be seriously undervalued. Enter your email address and below to see which companies made the list.
Article originally posted on mongodb google news. Visit mongodb google news