Category: Uncategorized
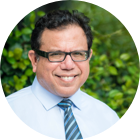
MMS • RSS
Posted on nosqlgooglealerts. Visit nosqlgooglealerts
Postgres vs. MongoDB and the death of SQL?
Hello and welcome to the Cloud Database Report! I’m John Foley, a long-time tech journalist, including 18 years at InformationWeek, who then worked in strategic comms at Oracle, IBM, and MongoDB. I invite you to subscribe, share, comment, and connect with me on LinkedIn.
Happy Valentine’s Day!
Here’s some data for you: Nearly 1 billion roses are expected to be imported into the U.S. for sharing with that special someone, at an average cost of $90.50 per dozen. If you take the flower industry and throw in some chocolates and candle-lit dinners, the economic boost is about $27.5 billion, according to Perplexity. You gotta love that!
It’s the price we pay for expressions of friendship and romance, although in the competitive database industry, we still have our differences. My LinkedIn feed recently has exposed a few areas where people are at odds over platforms and practices. Cupid’s arrows are hitting where it hurts.
Postgres vs. MongoDB
Case in point: There was a lively thread on one of the oldest of database debates: SQL vs. NoSQL.
It was prompted by a post by Peter Zaitsev, founder of Percona, who pointed out that Postgres (a SQL database) is growing in popularity while MongoDB (NoSQL) has been in slight decline. A graph on Zaitsev’s LinkedIn post shows the trend lines.
Percona has a foot in both camps — it supports SQL (Postgres, MySQL) and NoSQL (MongoDB).
Some of the commentary:
“MongoDB’s strategy prioritizes the developer experience, claiming to accelerate time-to-market (TTM) by 10x. Developers love it, but in practice, results often disappoint.”
“Schema less, SQL less world is easy at first glance. But is a big technical debt for the long term. It becomes very painful down the line.”
“Comparing MongoDB and Postgres is naive thing to do. They are for two different purposes and they are not a replacement to each other…”
“I also think MongoDB deserves more credit for eventually delivering on what they marketed — a robust, scale-out cluster for OLTP.”
SQL’s tombstone
That’s the gist of the SQL vs. NoSQL polemic, but not the end of it.
Lekhana Reddy, founder of Storytelling by Data, caused a kerfuffle with a LinkedIn post that headlined “SQL IS DEAD!!” The influencer and tech creator included an image of a tombstone with SQL sketched into it.
What happened that caused SQL to bite the DB dust? According to Reddy, Uber has developed a natural language query tool that obviates the need for the more arcane SQL programming.
Yet, the report of SQL’s death was greatly exaggerated, by Reddy’s own admission. “SQL isn’t dead it’s evolving,” she added.
The hoax drew more than 900 comments on Reddy’s post. Some samples:
“my take: SQL WILL not die before us!”
“SQL and other programming languages are not dead until AI generates its own language and data storage.”
“AI-assisted SQL won’t replace data professionals, it will enhance their capabilities.”
A relationship in the cloud
The good vibes came a day early for SAP and Databricks. When SAP CEO Christian Klein announced the launch of SAP Business Data Cloud in partnership with Databricks, one observer responded simply:
“💙 ❤️”
Quote of the day
I will wrap up with one of my favorite literary quotes below.
“Love loves to love love.”
Do you know who wrote it? Leave a comment.
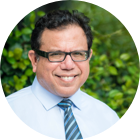
MMS • RSS
Posted on nosqlgooglealerts. Visit nosqlgooglealerts
What Is the Estimated Market Size and Growth Rate for the NoSQL Database Market?
The dimension of the NoSQL database market has seen a significant surge in the past few years. Experts predict a rise from $11.6 billion in 2024 to $15.59 billion in 2025, translating to a compound annual growth rate (CAGR) of 34.4%. Factors contributing to this growth over the historical period involve an escalation in data volume, demand for scalable solutions, the failings of conventional databases, the influx of big data analytics, proliferation of web and mobile apps, desire for flexible data models, and progress in cloud computing technology.
In the course of the coming years, the NoSQL database market is projected to observe remarkable growth, with expectations to reach $50.39 billion in 2029 and a compound annual growth rate (CAGR) of 34.1%. Factors contributing to the anticipated growth during this forecasted period include the rise of IoT devices, the growing application of artificial intelligence, the need for instant data processing, the extension of distributed systems, the increasing growth of e-commerce platforms, a heightened necessity for data security, and advancements in database tech. Notable trends within this period are expected to include the proliferation of multi-model databases, the progression of database automation, the blend with machine learning, the advent of edge computing, increased data privacy features, ground-breaking strides in query optimization, and the broadening of database-as-a-service solutions.
What Are the Forces Behind the Rapid Growth of the NoSQL Database Market?
Expectations of a rise in demand for online gaming and multimedia consumption are contributing to the predicted expansion of the NoSQL database market. Online gaming and multimedia consumption encompass online-accessible media content, such as music, videos, and games, and interactive entertainment. The surge in digitized forms of enjoyment, technological innovations, and the prevalence of high-speed internet worldwide are factors behind this increased demand. The role of NoSQL databases is crucial in managing the vast amounts of unstructured data, real-time interactions, and in ensuring high-performance queries, ultimately resulting in better user experiences and data processing for intricate applications. A study conducted by the Office of Communications (Ofcom), a UK-based governmental broadcasting and telecommunications regulator, in June 2022, stated that individuals in the 13 to 64 age range spent approximately seven and a half hours weekly, equating to about an hour daily, on online gaming. Hence, the rising demand for online gaming and multimedia consumption are key drivers behind the NoSQL database market.
Get Your Free Sample Now – Explore Exclusive Market Insights:
https://www.thebusinessresearchcompany.com/sample.aspx?id=19616&type=smp
Who Are the Dominant Companies Influencing NoSQL Database Market Trends?
Major companies operating in the NoSQL database market are Google LLC, Microsoft Corporation, Amazon Web Services Inc., International Business Machines Corporation, Oracle Corporation, SAP SE, Hewlett Packard Enterprise (HPE), Databricks Inc., MongoDB Inc., Elastic NV, The Apache Software Foundation, Redis Labs Ltd., Neo4j Inc., DataStax Inc., Couchbase Inc., InfluxData Inc., Aerospike Inc., MapR Technologies Inc., TigerGraph Inc., InfiniteGraph Inc., Basho Technologies Inc., VoltDB Inc., OrientDB Inc., Fauna Inc., NuoDB Inc., RavenDB Ltd.
How Is the NoSQL Database Market Evolving?
The key players in the NoSQL database sector are leveraging strategic alliances to augment technology integration and broaden their consumer base. Such partnerships usually symbolize the cooperative endeavor between several organizations pooling their assets, proficiency, and dedication to attain mutual targets. Case in point, in December 2022, Taashee Linux Services Private Limited (TLSPL), a tech and software services company from India, entered into a partnership with open-source NoSQL document database firm RavenDB, based in Israel. This joint venture is designed to grant access to RavenDB’s superior attributes like high-performance, entirely ACID-compliant operations, automatic indexing, and exhaustive text search capabilities to Taashee’s clients. The collaboration will bolster Taashee’s capacity to deliver tailor-made, scalable database solutions aligning with unique business requirements, principally for applications necessitating high transaction precision and efficient data management across multiple platforms.
What Are the Different Segmentations in the NoSQL Database Market?
The NoSQL databasemarket covered in this report is segmented –
1) By Type: Key-Value Store, Document Database, Column Based Store, Graph Database
2) By Organization Size: Small And Medium Enterprises, Large Enterprises
3) By Application: Data Storage, Mobile Apps, Web Apps, Data Analytics, Other Applications
4) By Industry Vertical: Banking, Financial Services, And Insurance (BFSI), Retail And E- Commerce, Healthcare And Life Sciences, Government And Public Sector, Telecom And Information Technology (IT), Manufacturing
Subsegments:
1) By Key-Value Store: Distributed Key-Value Stores, In-Memory Key-Value Stores, Persistent Key-Value Stores, Caching Solutions
2) By Document Database: Schema-Free Document Databases, Self-Describing Document Databases, Multi-Model Document Databases, Search-Optimized Document Databases
3) By Column-Based Store: Wide-Column Stores, Time-Series Column Stores, Column Family Stores, Distributed Column Stores
4) By Graph Database: Property Graph Databases, RDF Graph Databases, Multi-Model Graph Databases, Graph Analytics Platforms
Pre-Book Your Report Now For A Swift Delivery:
https://www.thebusinessresearchcompany.com/report/nosql-database-global-market-report
Which Region Is at the Forefront of the NoSQL Database Market?
North America was the largest region in the NoSQL database market in 2024. The regions covered in the NoSQL database market report are Asia-Pacific, Western Europe, Eastern Europe, North America, South America, Middle East, Africa.
What Is Covered In The NoSQL Database Global Market Report?
– Market Size Analysis: Analyze the NoSQL Database Market size by key regions, countries, product types, and applications.
– Market Segmentation Analysis: Identify various subsegments within the NoSQL Database Market for effective categorization.
– Key Player Focus: Focus on key players to define their market value, share, and competitive landscape.
– Growth Trends Analysis: Examine individual growth trends and prospects in the Market.
– Market Contribution: Evaluate contributions of different segments to the overall NoSQL Database Market growth.
– Growth Drivers: Detail key factors influencing market growth, including opportunities and drivers.
– Industry Challenges: Analyze challenges and risks affecting the NoSQL Database Market.
– Competitive Developments: Analyze competitive developments, such as expansions, agreements, and new product launches in the market.
Unlock Exclusive Market Insights – Purchase Your Research Report Now!
https://www.thebusinessresearchcompany.com/purchaseoptions.aspx?id=19616
Connect with us on:
LinkedIn: https://in.linkedin.com/company/the-business-research-company,
Twitter: https://twitter.com/tbrc_info,
YouTube: https://www.youtube.com/channel/UC24_fI0rV8cR5DxlCpgmyFQ.
Contact Us
Europe: +44 207 1930 708,
Asia: +91 88972 63534,
Americas: +1 315 623 0293 or
Email: mailto:info@tbrc.info
Learn More About The Business Research Company
With over 15,000+ reports from 27 industries covering 60+ geographies, The Business Research Company has built a reputation for offering comprehensive, data-rich research and insights. Our flagship product, the Global Market Model delivers comprehensive and updated forecasts to support informed decision-making.
This release was published on openPR.
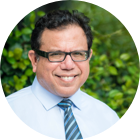
MMS • Pablo Fredrikson
Article originally posted on InfoQ. Visit InfoQ
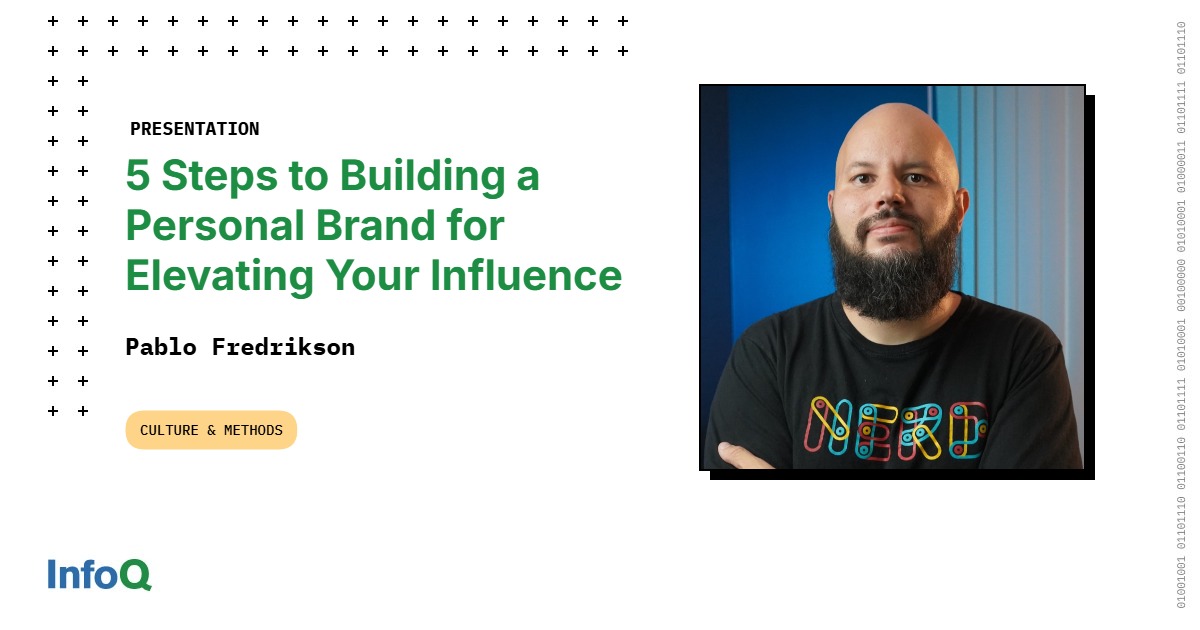
Transcript
Fredrikson: My name is Pablo. I’m going to be talking about building a personal brand. Everyone has a personal brand. You already have a personal brand. Even though maybe you don’t have an online presence and people say, “I don’t have social media. I don’t use LinkedIn, or I don’t use Twitter”, or something like that. That is a personal brand. Or maybe you have like a generic online presence, like a basic LinkedIn profile, a basic Twitter account, or a basic GitHub account, that’s also your personal brand. Or maybe your internal personal brand inside the company, is also really important, the way that you talk, the way that you present yourself with your teammates, it’s also your personal brand.
This is an example. I have a YouTube channel. I have this character or this persona online called peladonerd, which means bold and nerd, easy to remember. This is the way I use Slack on my community Slack. This is just my handle, a picture of a cartoon based on Rick and Morty. This is the way I type on that Slack community. This is my work Slack profile. As you can see, it’s a different one. It has my real name, a real picture. The way I type is also different. I have different personas or different brands depending on my audience.
Why Do You want to Build or Elevate Your Brand?
First, when you want to build your personal brand, you have to know what’s your goal? What’s the idea of your brand? What do you want to do? Maybe you are transitioning roles. Maybe you want to build credibility in a new field. Maybe you are moving from management to technical side. Or maybe you are passionate about music and you want to start a band, or something like that. Or maybe you are someone that is still learning how to develop, or maybe how to program, and it’s going to be a new field that you’re going to be entering. Or maybe you want to be a go-to expert in your field, inside your company, so you want to position yourself as a higher person and a better one. Or maybe you want to just progress in your career. You want to get noticed within your company or even your industry. This is actually really important, in my opinion.
I’ve seen this many times inside and outside my company, seniors that are too quiet and they don’t progress. They are really good technical people. They are perfectly capable, but because they are too quiet or they don’t show off what they are doing, they don’t get noticed, and sometimes they don’t get those promotions, because people don’t know what they’re doing. They know that they are technical experts, maybe, but they are not showing up and not showing their impact inside the company. Getting noticed is really important.
It’s really important as well to learn how to own problems. I remember a few years ago in my previous company, there was a TLS certificate that was going to expire. I remember talking with my manager at the time, and they were asking me, can you fix this problem? I said, let me check with the IT department, because I need to buy a new certificate. That day, the IT department wasn’t available. The person wasn’t available on the phone, so we couldn’t reach him, and we couldn’t buy the certificate because we didn’t have a card. My manager was also a new person, they didn’t have a company card. I didn’t because I was just an engineer. I don’t know why engineers don’t have company cards, but we don’t usually. I remember having this problem. I said, we need to buy this certificate. It’s only 100 bucks, but we don’t have the card. How do we solve this problem? It’s going to expire today, and our site is going down.
My boss was freaking out, “We have to solve this problem. This is something that needs to be fixed”. There was a lot of chaos at that moment. A lot of other things were breaking. I remember saying something like, “Don’t worry. I’ll figure it out. Go and check with your other teammates. Go solve other problems. I don’t know what managers do. Just go and have meetings, and just have a lot of meetings. I’ll solve this problem”. If I think about it right now, it’s obvious, I just paid the certificate with my card. I just bought the certificate, used my personal card. Solved the problem. Changed the certificate, all good. A few days later, the IT department came back, I said, “I had to buy a certificate with my card. You weren’t available”. They say, of course, no worries. We are going to refund you.
A few months later, we were having an offsite. That company was InVision, rest in peace. That company was 1000 people, all of them were remote. Every year or every two years, we had an offsite, and we went to the offsite in Phoenix. I remember passing by the CEO of the company, never had a meeting with him, never talked to him before. He saw me and said, “You’re Pablo, right?” Yes, I am Pablo. “I heard about your certificate thing. Thank you for that. That was awesome”. I said, “What? You know about that?” “Yes, I heard of it. Thank you very much. That was a great way to solve the problem”. I took a picture with him to have this proof. I’m going to tell this story someday, I said. I remember thinking about that and saying that was crazy. I just bought a certificate. I got noticed by the CEO of the company.
Then a few months later, they were talking in CEO meetings, whatever, all-hands, and they were saying like, yes, I know that if there is a problem and you have to check that dashboard, you go to Datadog and grab the dashboard that is made by Pablo, because he created a dashboard, and that is great. Thank you, Paulo. I said, what? This is my best friend now, the CEO of the company. Doing something like that, definitely, I got noticed by him, and it definitely helped. From then I got a promotion. I got messages from him saying, “Great work on this one. Maybe we can talk someday to do something else”.
Going back to, why do you want to build your brand? Maybe you just want to help others, making your company or industry a better place. This is important, because all the things that we do as staff or principal engineers, I believe is to make your company better and more valuable. You’re directly raising the value of the company by doing your job. I have conversations with friends, and they are not in the field, and they ask me, what do you do every day? I say, “I have meetings like managers, and I talk with people”. Why? I say, “I try to fix problems”. Which problems? “I just bought a certificate the other day. That was awesome”. I said, yes, but why?
The thing is that all of those small things, like helping others and making sure that the problems are fixed is adding value to the company. The engineers inside the company are going to be better, and that raises the value of the company. Also, if you are helping others as well outside your company, you are also making the industry a better place.
What Is Your Defining Expertise or Passion?
You need to decide, what do you want to be known for? What’s your defining expertise or passion? If the goal is a tech leadership role, maybe you can be a mentor, or you can try to help others by sharing your technology expertise. It is important to find out, what is important for the company. What does the company need that you can help with? What things that you can do that are unique for you are skills that want to be synonymous with your name? If people think about, let’s solve a problem. What is the dashboard I’m going to use? I’m going to use Pablo’s dashboard, because he knows about this stuff. Just try to get that. Just try to be top of mind for people.
When I was making these slides, I realized that actually these two steps might be interchangeable. You don’t need to first define your goal and then decide what you want to be known for. You can do it the other way around. Also, you can be changing all the time. Maybe after some time, you realize that your goal might change. That way, you can also change what you want to be known for. These are interchangeable, in my opinion.
Background
My name is Pablo. I am a principal SRE at Harness. I have 18 years of experience. I’m also a CNCF ambassador. CNCF is the Cloud Native Computing Foundation that basically created and helped develop and support projects like Kubernetes, Prometheus, and all of those great open-source tools. Because of this online persona and my personal brand, I also got invited to be an ambassador for the CNCF. I also have a YouTube channel where I make videos about these kind of technologies, Docker, Kubernetes, and all that, and also try to share what I do every day at work and what I think is the best way to help people, and make them better engineers and better people as well.
Know Your Audience
You need to definitely know your audience. Who is going to be watching or learning from you? When I started, my YouTube channel was named Pablo Fredrikson, which is my name. Then I changed it to Pelado Nerd. This is funny, because I remember making the first videos and chatting with people. They said, your channel sounds interesting. What’s the name of the channel so I can check it out? I said, Pablo Fredrikson. They said, what? How do you spell? Because in Spanish, Fredrikson is not an easy thing to spell or type. People were like, you have to spell that for me. It sucked a little bit. I realized, that’s a problem. That’s a barrier. People cannot find my channel because they don’t know how to type my name. I said, let’s find an easier way for people to find me. I said, Pelado, which means bold, and Nerd. It’s easy to remember. It’s easy to find.
The funny thing is that I was at KubeCon, talking with a Docker person, and I was mentioning my channel. I said, I make channels about Docker. Actually, my Docker video has like a million views, maybe we can work together. They said, that sounds like a good idea. How do I find your channel? Yes, Pelado Nerd. They say, what? How do I type that? You have to spell it out for me. It was funny because the audience definitely makes a difference. I had to change it depending on who is going to be watching my videos. My videos are all in Spanish, so that’s why it’s my name in Spanish.
Who Should Your Brand Influence?
Who do you want to influence with your brand? Maybe you want to influence your internal audience, which is your company, your industry. You have to say yes to visible projects. You have to build relationships across departments as well. I remember having an offsite a few years ago. We were talking about how to bring more customers to Split, our company. I was chatting with the marketing department, and they had a Twitter account, a LinkedIn account. I said something like, “I do have a YouTube channel where I make videos. I think I know a little bit about social media. Maybe we can work together. We can help each other”.
I had some suggestions of things that we can do in the Twitter account, maybe we can write some tutorials, make some videos, things like that. That was great, because, again, going back to what I do, is, I just try to make the company better, just try to make engineering or just getting customers. The goal of the company at that moment was to get more customers. How can I help to bring more customers? Installing nginx is not going to bring more customers, so I just need to help the company bring more customers. I have some experience with that, so maybe I can help a little bit.
It is really important as well, if you want to build those relationships and you want to be visible, is to join leadership or planning discussions, because you need to know what the company needs. This is something really normal. Usually, engineers don’t know what’s going on in the company. Engineers just install nginx, because they got that ticket that says, install nginx, so just go and do it. They don’t know why. Or maybe they know, but they don’t know the actual goal of the company. At that moment, the goal of the company was to get more customers. Why is installing nginx going to help the company get more customers? Maybe it’s not. Maybe it is. If you have that information, do you know why you are doing it? Maybe you can change it a little bit.
This is just an example, but maybe instead of nginx, Apache will bring more customers because we can have a partnership with some company, or something like that. Maybe instead of nginx, we can do Apache or web server. It is important to know what is the company needing at the moment. For external audience, let’s say that you want to build your personal brand outside the company, maybe for the industry. You are aiming for a broader industry presence. Think about skills that you already know that you can help other people with. Maybe you can write tech blogs, or make videos, speak at meetups or conferences, or maybe just contribute to open-source projects. Just helping people helps. Helping people makes everyone better, and makes the world a better place.
Considerations to Have in Mind
You need to build a presence that reflects you, but also need to be careful. These are a few considerations to have in mind, in my experience. First of all, it’s really hard to separate your job and your online persona. When I was being hired, that was a good thing, of course, but it might be a bad thing as well. Because people say, I don’t like this YouTube video. The video is about things that I don’t like, so we are not going to hire him. In my case, it was a good idea. It’s really hard to separate your job. People in your company will find you online. Be careful with that: things that you share, things that you do online. Be careful with maybe things that you post or tweet, people will find it.
Also, try to align your public brand versus your internal brand. If your public brand is about making videos about Docker and Kubernetes, obviously it will be nice to have the same persona inside the company, you are the guy from Docker and Kubernetes. If they don’t align, maybe it’s time for a change. Maybe it’s time for a change either in your online persona or public persona versus the internal one. Maybe you need to get a new job, or maybe you need to change your online persona or the public one. Obviously, talk with your manager and director about your online persona, make sure they’re ok with it. Have it in writing. This is important as well, because they can say, yes, that’s cool.
Then maybe you get an email from the HR department, or maybe the lawyer of the company saying that it’s not cool. You don’t have any proof about saying, I got a conversation two years ago with my manager who doesn’t work here anymore, and he said it was ok. That’s another great idea. You have to have it in writing. If you’re looking for a new job, also talk about this during the interviews. Again, make sure that they are ok with it. Have it in writing. This is not bragging, or maybe it is a little bit, but you can use it to your advantage.
For example, if you’re having an interview, just say something like, “I built these servers. I built this program inside my company. I also made a video about it in YouTube, if you want to check it out”. They will say something like, you have a YouTube channel? “Yes, I made YouTube videos about this”. People can like that. Maybe they don’t, but because people will find it, it’s better to have that conversation beforehand. Also make sure they’re ok with it.
Find and Share Your Passion Consistently
What’s your passion? What are you going to be sharing? I know that most people have a friend that cannot stop talking about dinosaurs, or cannot stop talking about a topic. You go out, have a meal with them, they say, “This dinosaur is amazing. It has six legs”. You need to find the thing that you’re passionate about, and you cannot stop talking about it, because every time you go have a meeting with them, they can’t stop talking about it. That is something that they want to share. That is something that you can share as well. I remember watching a video, this one is called, “Brown; color is weird”. It has 4.7 million views. I was watching this video, fascinated about it. This guy made a 21-minute video about the color brown. It’s great. You should check it out.
When I was watching this video, being fascinated about it, I was thinking, this guy really likes the color brown, or really likes being nerd about it, about colors, about things. Before that, 10 or 15 years ago, it was impossible for this guy to have an audience, in my opinion. Who is going to be interested about the color brown? Maybe some people are, I am, and maybe you are, but it’s really hard to find the audience. That’s what YouTube makes, or the internet makes in general, finds the audience for your niche, for the things that you’re passionate about. This guy made a video about the color brown, and it has 4.7 million people actually watching this video. I don’t think that that was possible 20 years ago.
Be Ready to Pivot
Also, be ready to pivot. Because maybe your audience will change, maybe the time changes, maybe your dinosaurs change. Maybe you don’t like dinosaurs anymore, you like cars, or you like running. Things like that. I actually started running a couple of years ago. If you saw me two years ago, this guy cannot run. I cannot run that well today. Now I have another YouTube channel where I make videos about running. It’s a small one, but yes, try to share what I do. I like doing it. It’s free to upload in YouTube. Uploading a video before wasn’t possible. It was really hard or really expensive to upload a video and share it with someone. Now you can just put it on YouTube. It’s free. Be ready to pivot. Maybe your interests are going to change. Maybe your audience will change. You can change your content as well.
Wrap-up and Key Takeaways
First, you want to define your goal. Then you want to decide what you want to be known for. You can change this and maybe change your goal and change the second one. Understand and engage with your audience. Be careful what you choose or share. Find and share your dinosaurs. You are probably already learning about dinosaurs in your free time, or the passion that you have. I remember having a conversation with someone, and they asked me, how do you find the time to do everything? Because I have a full-time job, and also have two kids. I have my YouTube channel. I try to run and do other things. How do you find the time to do all of that? The secret is not a secret, it’s just I am already investigating and learning about the things that I want to make videos about every day at my job.
The other day, I was investigating about a technology called APISIX, which is an Apache API gateway. I had to investigate a little bit, install it on a few servers, and then I had to write a documentation, share it with all the engineering platform team. When I was investigating and writing a documentation, I thought about the video that I was going to make about it. Actually, the documentation that I wrote was actually the script for the video. I just was thinking about how to share this technology with my internal company, with my internal teammates, and also, at the same time, how to share this with the public company or the public audience. I wrote the documentation. It was like a video.
If you read that documentation, it said something like, this is called APISIX. It’s an API gateway. What is an API gateway? It’s this and that. What can you do about it? You can do something like this. You’re still not surprised? Maybe you can do something like this. Not surprised yet? You can do something like this. It was a YouTube video, but I wrote it as a documentation. I sent it over. My teammates liked it. You can use the time that you already are getting paid for, which is your job, to investigate about that. Or maybe if your passion is not something that you have in your job, again dinosaurs. You are already investigating that in your free time. Maybe over the weekend, you read about them. You are doing the research that you need in order to share that. Just be a good person. I think that’s my step number six.
If you are a good person, you will help others. If you are a good person, you will care about others, and care about the company, what the company needs, and what the industry needs, and what your teammates need, and what your friends need. If you are a good person, everyone will benefit.
You can start today, actually. Maybe you can write a blog post. Maybe you can share something that you investigated during your work hours and share it online. Again, check with your manager, check with your director, have it in writing. For sure, they will say something like, yes, of course, it’s fine. I have a ton of examples of things that we did during our work hours and our director said, write a blog post about it and share it with others, publicly. Of course, they will have to check it out to make sure that no sensitive information is shared, but you can do it. I’m sure they will say yes. Also, ask a colleague from another department, if they need help with a project that you are interested in.
For example, let’s say that you are interested in marketing. Maybe you have a tech background, but you are interested in social media, so just chat with the marketing department. Say, how can I help? Or maybe you’re passionate about cars. I remember having an interview as well with a guy who I was interviewing. We were talking about documentation. He told me that he loves writing documentation. I don’t know how we got to that point, but he loved writing documentation. I said, really? Why? He said, I love writing documentation. Can you show me an example or something like that? He said, yes, can I send you over later? Yes. After I finished the interview, he sent me the documentation. I didn’t ask for any details. I didn’t say, send me a documentation of how to install whatever. No, I just said, send me whatever.
He sent me a documentation, and said something like, “This is what I did. I created a server. I set it up with Docker Compose. I created a Wikipedia page, hosted it myself. I changed the DNS by doing this and that. Also, I created a domain, pointed it over, and this is the documentation I wrote”. He not only wrote the documentation, he also told me how he did it, and also obviously sharing his knowledge about Docker and all of that. The documentation was about how to change the filter of an air conditioning in a Mustang 98, or something like that. I was like, that’s fascinating, because he didn’t make a documentation on how to install Docker.
He made documentation about what he’s passionate about, and it was his car. The documentation was amazing. He had pictures and everything, all the steps. I don’t know anything about cars, but sounds legit. Seems like a good tutorial on how to change that. I hired him because of that. Sharing something like that, your passion, will definitely make the world a better place. You can get a job, or you can get good things about it.
Talk to your boss about your passions. Maybe they can give you ideas of things that you can do. You say something like, I like cars. We have a new car department, or maybe we have a new customer, it’s a car company. Maybe you can be there and you can just talk to them about cars, whatever. Help someone today. Just go send an email saying something like, do you need help with that? I can give you a hand. I remember a year ago, an application was dying all the time, and it was getting out of memory. Because of Kubernetes, in Kubernetes you can set like a check, and if the pod fails that check, it will kill it and restart it.
Actually, the problem wasn’t too bad, because it was fixing itself all the time. The memory was going up, Kubernetes was killing it. All was good. At the same time, it’s not a good idea to have a service going down all the time. You are using resources. You’re paying for it. It’s obviously better to fix it. I remember having this service going down all the time and saying to the team, fix your service. It’s going down all the time. They said, “Sure, I’ll fix it”. Days go by, the service is still dying. Fix it. “Sure, I’ll fix it”. Few days go over, still the service is going down. I remember saying, let’s change this. Instead of asking them to fix the problem, let’s try to help them to fix the problem. Maybe they don’t know how to do it. I’m not a developer. I don’t know anything about languages. How do I fix this problem?
Obviously, I don’t have the answer, but maybe I can help them. I just went over to their channel and said, seems like the service died again. Do you want to maybe hop into a Zoom call and we can talk about it and see what happens. Sure. We just went into a call, went to Pablo’s dashboard, and checked that, yes, seems like the memory was going up during these times. Seems like it was a specific timeframe. We did some search together. We tried to figure out what was the problem. I gave them information on how to find the problem, and they figured it out an hour later, because they already had the information, but they didn’t know how to find it. I just helped them.
The problem was fixed the day after. By helping them, I benefited myself. My team was happier. I didn’t get paged anymore. Yes, just helping them, easy. I remember after that, the team was actually using that dashboard all the time because they learned about it. They didn’t know about it earlier, and they now know about it. You can check my site, peladonerd.com.
Questions and Answers
Participant 1: It’s interesting to me that all your videos are in Spanish. How does interacting with two different languages, is it a different community? How has that been in general in your YouTube career?
Fredrikson: I don’t interact that much in English, at least on my YouTube channel. I do have some videos in English because I make interviews with all people, like Kelsey Hightower, for example. I also have a few interviews with people from Google. I also have an interview with our Docker guys, and those are in English and have subtitles. The way that I interact with them is just, I have subtitles for the English videos. I try to focus on the Spanish speaking people. I definitely can make English videos, obviously takes more time. There are a few tools that you can use to actually adapt, like dub your voice, and now with AI and all of that it’s easier.
It takes time, of course, and I don’t see that benefit, because I will have to basically dub all of my 400 videos. Currently it’s not possible to add an extra audio track to an already published video. I know this is too nerdy for YouTube. If you upload a video on YouTube, you can add different tracks, but you cannot modify an already published video. The only thing that you can do with an old video is add subtitles. I know that English speaking people don’t like subtitles that much, so I have a problem there. Maybe someday YouTube will allow you to add a track to a published video. In that case, I might do that.
Participant 2: Sometimes people feel they are very busy, and they assign some of the branding aspect to someone. What’s your advice in your career? Maybe advice that you can share, if it is possible or it’s advisable, which part of the aspects can you delegate? I know some people, the content writing, they give to some people to write, and then they just review. Then you put it on your handle, because people are busy. What’s your take on the balance between trying to create everything by yourself and trying to get people to help you, even if eventually you pay them?
Fredrikson: At first, probably you will be doing everything yourself. It’s going to be hard, it’s going to be long, and your videos are going to suck at first, for sure. After some time, you will get used to it, and you will find things that you can delegate. It’s really hard because it’s like your baby. You don’t want to give parts of your baby to someone else. Maybe you can get help from someone you trust. I remember watching a conversation with Mr. Beast, which is one really popular YouTuber. He said something like, it is really stupid for you to be spending eight hours on an edit for a video, plus four hours of recording, two hours investigating whatever, and all of that, because you don’t need to be doing all of those things yourself.
Also, if you can only dedicate eight hours to an edit, that means that it’s limited. The amount of time that you can add to it is limited. If you hire someone else to do the edit, they can maybe invest 24 hours to do the edit, because you don’t have that much time. You can delegate, and that will make the video better. I actually did that. I have my brother. He was looking for a job, and he didn’t know how to edit. I said, you will learn how to edit, and you will edit my videos. I will pay you for it. It’s a person I trust, so I know I can talk with him. Now my videos are better because he edits my videos. He can spend more time on them than the time I was able to spend.
Definitely, at first, you will be doing everything yourself. There are a lot of things that someone else can do. Actually, the only thing that you need to do if you are making a video about yourself is just being in front of the camera, because you cannot hire someone, unless you have a twin brother or something. You have to be there in front of the camera. Everything else can be delegated. You can do something like that.
Participant 3: What goals did you start off with? How did those goals evolve over time?
Fredrikson: At first, I actually made a YouTube channel about vlogging. I watch YouTube all the time. I was following a vlogger called Casey Neistat. It was like really great videos about just going for a run, or having an ice cream. It was fascinating for me. I said, I’m going to do the same thing, just go for a run, get an ice cream, make a video about it, be a millionaire. Didn’t work because people didn’t know me, and obviously my videos weren’t that great either. I said, now that I know that my videos suck if I just go out for an ice cream, maybe I need to get people to know me or like me before they want to see my having an ice cream video. How do I make people find me? I’m going to make a video about Docker, because people will say, “Docker: From Newbie to Pro”, whatever it’s called. That’s actually the name of my video.
People will search for that, find me, hopefully like me. That way they will start seeing my other videos, like passion videos, which are the vlog ones. After some time, I realized that I suck doing those videos, so I just kept doing the Docker ones, because I already had some experience giving talks and all of that. I liked helping people. I liked teaching people about technologies. My YouTube channel changed a little bit to that. Actually, it was a new YouTube channel.
I also have the other channel that I made, about running, which is a mix between the two. Because it’s more vloggy. I’m going for a run. I went to the Lombard Street, the other way, the thing that you have to go up. That’s really hard. I got an alert in my watch, like, “Too hard, you’re doing it today. Your heart rate is really high”. It’s in the middle, because I make videos about running, and also try to teach a little bit about running.
Obviously, I don’t know anything about running. I try to teach the things that I’m learning every day, because I’m a nerd. I like learning. I learn every day. I learn about nutrition. I learn about how to run. I learn about shoes. I learn about all of that. Maybe just share what I’m learning every day. Maybe help some people. I actually got comments on my videos saying something like, “Now that I see that you lost some weight and you are running. I’m also a nerd, I don’t run. I prefer computers and games. Now I tried, and I went for a run. It was 10 minutes. Felt bad, but felt great after that. Thank you”. Trying to help people by showing what you’re doing is always better for everyone. Just be a good person.
Participant 4: How long have you had the YouTube channel? What’s the cadence of how frequently you do a new video? What keeps you motivated to keep producing content on the regular, and not getting burned out?
Fredrikson: Five years for this YouTube channel. The previous one was two or three years before. How often do I make videos? At first, I had a lot of time, because my kid was a baby, so he was sleeping all the time, and he didn’t require me to be running around. I made three videos a week, sometimes. Because I also had a lot of content. Because I wanted to talk about Docker, so let’s talk about Docker, containers, then talk about CPU, memory, then talk about whatever. It was easy for me, because there was a lot of things to talk about.
Then, Kubernetes, let’s talk about services, pods, then deployments, then the stateful set. I had a lot of content. Then my kid grew a little older. Now it requires more time. I also got a new kid now, so I don’t have time. I try to make one video a week, but I don’t record them one a week, because obviously that takes a lot of time. What I do is I try to investigate, batch process all of them. When my kid goes out for soccer practice, and my wife takes my baby with them, that means I have one hour and a half to record videos. I batch, investigate three or four videos. Why I record them when they are not around, because of the screen, the sound, and all of that. I try to record a few, three or four videos in batch, and just send them to my brother. I have a calendar in Notion with a Kanban column like, edit, produce a thumbnail, whatever, and just have the date, so they just move over. He can check on the Kanban and say, what is the schedule, and all of that.
What I do for not getting burnout? It’s hard, because it’s like a startup. It’s like your second job, and if you don’t work, you don’t get money. For a startup, it’s like you have your own company, and you take vacations. It’s really expensive. Because you have to pay for the vacations and also pay for the time that you weren’t working. It’s hard, and you have to find a balance. Try to get breaks. I don’t post videos in January, so I take a month every year. The bad thing about it is that I have to record them in December, and have them ready during February. All of that. It’s not that easy. Find new technologies all the time.
Again, because I’m already investigating all the time during my work hours, I have topics and ideas. I have ideas from all the talks that we’ve seen in this amazing conference. I was at KubeCon, got a few ideas as well. I met with people, or just checked the comments of people, what they are looking for. The topics will never end. You have a lot of things to talk about.
Participant 5: I was wondering about the quality and keeping quality of the brand. You said your first video sucked. I believe you. All of our first videos would suck. Do you go back and delete them afterwards, or do you keep them and say, I was really crap back then, but now it’s much better?
Fredrikson: In my case, I try not to delete them unless there is something that is wrong, like I say something that is not correct. No, I don’t try to delete them. You can go to my channel and see my first video right there. It sucked. It’s a normal evolution. We all evolve over time. I’m sure that you all maybe think about yourself, that you’re in your best looks maybe. You look at a picture of you five years ago, saying, what was I thinking with that hair or with those clothes? It’s normal. You’re evolving. Everyone is evolving all the time and learning new things. You are a better engineer now than before, five years ago. Everything hopefully gets better over time. It’s a normal evolution. Your videos will suck at the beginning. You will get better about it. You will be more efficient.
At first, I set up my videos every time, like all the cameras and the lighting. I had to turn down everything. Now I have everything in one place. The camera is always fixed. The lighting is always fixed. All the lights are fixed. I just press a button, say something to Alexa, and turn on all my lights, just press record, and every video looks the same. It doesn’t take me time to start recording. I removed that variable. Also, the lighting, the sound, everything is in a perfect place. I don’t lose time by adjusting things. Everything is perfect or almost perfect. That allows me as well to improve a little by little every video, because, last week I saw that the lighting wasn’t great here, so I’m going to adjust it a little bit. Now it’s better. Let’s go and try to find small things to improve as well. Every time there is small improvements and the videos are getting better, hopefully.
Participant 2: Also, there is this fear. I don’t know how you deal with it. I’ve written many times. When I write, I’m about to push, then I think that, “There must be something better, there must be a better version of this document out there. Don’t bother. Just keep it”. That slows you down, because you have this feeling. I don’t know how you manage that as well.
Fredrikson: It’s hard. The quote I always think about when I’m thinking about that is, done is better than perfect. Just put a date on it, finish it, and just think about the same one.
See more presentations with transcripts
Podcast: Building Responsible AI Culture: Governance, Diversity, and the Future of Development
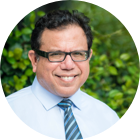
MMS • Inna Tokarev Sela
Article originally posted on InfoQ. Visit InfoQ
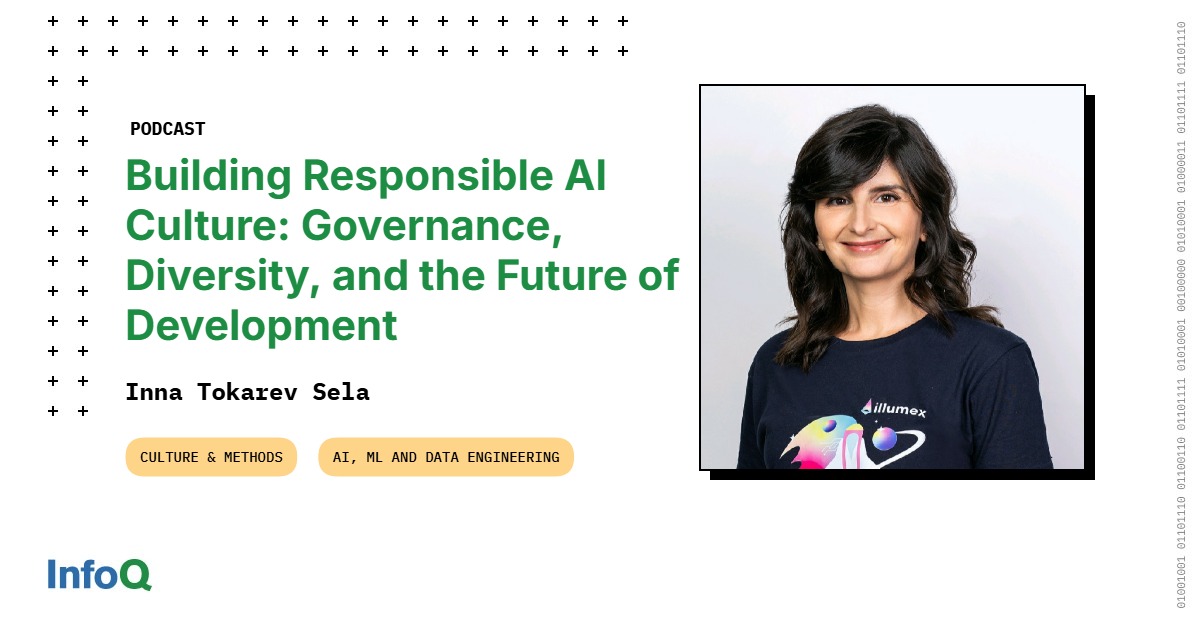
Transcript
Shane Hastie: Good day, folks. This is Shane Hastie for the InfoQ Engineering Culture podcast. Today I’m sitting down with Inna Tokarev Sela across 10 time zones. Inna, welcome. Thank you very much for taking the time to talk to us today.
Inna Tokarev Sela: Hi, Shane. Thank you for inviting me.
Shane Hastie: My normal starting point with these conversations is, who’s Inna?
Introductions [00:44]
Inna Tokarev Sela: Who is Inna? CEO and founder of Illumex. I started the company around four years ago, but I think the most interesting part professionally-wise is to speak about my passion for graphs, run graph technology. During my first degree in physics and computer science, I fell in love with graphs and my research thesis was around that. You might remember we didn’t have all this nice software we use today. So we used Matlab. And what I did is actually simulating geometrical forms over graphs to basically implement operational research methods and make them more efficient.
And since then, I am passionate about the graphs of neural nets and the combinations. This is what I did in my thesis. Basically developing algorithms, which are based in combination of graphs and neural net systems, specifically for healthcare domain.
This was in the beginning of my career and then I started my long journey at SAP. And it was really exhilarating to see how much you can achieve with the access to so many customers. So I was part of SAP HANA cloud platform, a team under the office of CTO. And got lucky to work with companies such as Walmart, Pacific Builder, Lockheed Martin, to help them with the journey to big data and cloud initiatives.
And of course, building use cases and business cases and the strategy around that. This is my background. I’m a mother and we as a family, we live in Tel Aviv, very close to the beach. So I do enjoy first morning and evening walks, seeing sunset. This is indulgences that we have.
Shane Hastie: Tel Aviv is a lovely city. So some key elements of generative AI. And this is the Engineering Culture podcast. So we are not going to talk about the APIs for accessing the AI tools, Sirini and others, my colleagues deal with all of that. Let’s start with the cultural impacts on teams. If we’re bringing generative AI into the software engineering space, what is that impact for us?
Cultural impacts of bringing AI into teams [02:58]
Inna Tokarev Sela: Bringing generative AI to any team bears a lot of cultural change. So given that you cleared up all this privacy issues and show them your tool, it’s important to understand how employees are going to use it. So education, “What’s the art of the possible?”, is imperative to any team. So in development team, we do use generative AI for testing, some code prototyping, but it’s really understanding how you can accelerate the adoption.
I do believe in access acceleration, due to the fact that at least 30% of development are mundane tasks, which are also necessary. So not every backend developer loves to write tests, right? And this is, for sure, something which could be automated. We actually, from my experience, you see lots of excitement in our development team around using generative AI, despite the fact that as a company and especially in development, age is above medium for startups. So we are 35, 37 on average. I see a lot of excitement about this new technology.
Shane Hastie: You blithely passed over let’s sort out the privacy and so forth. But, I know governance is an area that you are passionate about and concerned with. So it’s not that easy to just get the privacy thing right, and other elements of governance. Is it?
Governance when bringing in AI [04:23]
Inna Tokarev Sela: Sure. Governance is a big topic and especially in data, data management analytics space. To me, every aspect of data usage and software development around that historically involves lots of guardrails. So we have governance for data, for analytics, we have different audits and standards, GGBR and SOC 2 and just to name few.
Right now for generative AI, we have lack of standardization on many aspects. So we do have those EU ACT initiatives and other legislation initiatives, which do play some high-level requirements. But. I see that right now the majority of enterprises actually decide on their own standards, separate and we do not have lots of standardization about that.
But in general, I do believe that governance right now is not embedded enough in generative AI practice as it is, due to the fact that generative AI models are black boxes. And when fine-tune when they customize them on your own organizational data or build workflows around them, usually data scientists are mainly focused on feeding those models with test data and understanding the outputs, consistency.
For example, approaches which is called RAG, to make it as customizable as possible to make the models to understand your data. But, they couldn’t care less about governance. They do not understand the models enough even to customize them in majority of the cases, again, because as technology is a black box, they understand more and more about predictorization of this technology.
But, being able to plug in generative AI the same ways as we do plug in data management analytics into access controls, into governance mechanisms, which we already have in our organizations is imperative, I would say even more. So far as practitioners, we didn’t really govern our interactions too much. So we did have secure access of the interactions like security control and malicious software detection and so on and so forth. With generative AI, we match extent also and governance of interactions.
What I mean all those proverbial $1 tickets and SUVs, we need to make sure that not only underlying data access is governed and data quality is governed, but also interaction themselves. Is someone trying to prompt engineer your generative AI, are they asking questions which access data, which they might have access to. But, the model which answers those questions is trained on the data they do not have access to, and so on so forth. So it goes way beyond.
Shane Hastie: So what does that start to look like in practical terms? So who is holding the governance roles? How do we define those rules even?
Inna Tokarev Sela: I do believe that the future of GRC and governance roles as they are today, mainly in setting the standards, setting the standards and monitoring that implementations cater to those standards … I do believe that governance as a discipline, as everyday practice should be actually part of everyone’s job and especially domain experts. So domain experts think about people in sales and marketing and customer support. When you do have your context and reasoning built for generative AI by data scientists, you might want to have workflows, which embed domain expertise.
So why data scientists would decide how churn is calculated? Why data scientists should decide how upsell is defined in sales, right? So domain experts, there are conflicts in data and there are always conflicts in data, especially when connect enough data sources. I do not think that they shouldn’t be resolved on technical team sites. I do believe that domain experts should be involved more, especially because we expect those new generation of tools to provide self-service to data analytics and scale for domain experts. So we need to bring them as part of development as well.
Shane Hastie: It sounds to me, particularly in that transparency, that we’re looking to create or bring in some level of observability in the models, in the AI tool, whether it’s in the training or whether it’s in the processing. How do we do that?
Inna Tokarev Sela: I believe that observability in generative AI implementation should go all three levels, should adjust three levels. The first one is the data layer. Right? So AI-oriented data. If you data is healthy enough, if you have single source of truth, if you do not have single source of truth, of course, speaking about semantic modules, which are probabilistic and you would not like to have randomness around that. So AI-oriented data for starters.
Second layer is the governance. What exactly the policies that you would like to automate on generative AI? Generative AI, of course it’s about scale, so you cannot apply everything manually. You should build automated guard rails to enforce whatever policies you decided to enforce.
And the third one is this explainability layer, because if we speak about intelligence decision-making based on generative AI outputs, if it’s for development, or for business users to make decisions in the daily practice, it’s all about trusting the results. And results, when you have this answer 42, as a black box, you cannot really make decisions based on that.
So observability and transparency also goes to the explainability of generative AI outputs. How my promt was understood? Which data was it mapped to? What is the logic which was deduced from this prompt? Did this prompt goes through some certified semantics, which domain experts waived. So all of that should be in place. So three layers, data layer, governance layer and interaction layer with explainability.
Shane Hastie: I don’t see many organizations doing that at the moment.
Inna Tokarev Sela: I think most of the organization aspire to do it at the moment. I see more and more risk management requirements in generative AI projects, especially in highly regulated industries. And it goes to, of course, risk management goals to the bias and privacy concerns. But, it also goes to liabilities. Liabilities to make decisions based on potentially wrong outputs. So this risk management on accuracy of outputs, explainability of outputs and consistency and compliance of outputs becomes a pinnacle of generative AI implementations.
The second consideration would be total cost of ownership, because it’s very expensive to implement and customize over-the-shelf software for the enterprise needs. So this risk management to me becomes more and more established as a practice. And there are a plethora of solutions evolving in the space, from governance to cybersecurity to more offline tools for policy management and so on and so forth.
Shane Hastie: Dig into that policy management, because if I think from the stance of the technologist implementing this and bringing it in, they have got to provide the framing for these three layers to be in place. So let’s start and you said give us policies. Well, what does that framework, the technical implementation of “apply policies” look like?
Technical implementation of rules & policies [12:35]
Inna Tokarev Sela: Yeah. So think about policies as the vehicle to address a few things. First of all, it’s about quality of data. If data is representative, if it has standards of duplication, duplication levels and so on so forth. But also, if it’s even enough, it’s distributed enough. So for example, all the bias component. There are mechanisms to basically measure that and make sure that the data, which is fed into planting or even into training is even enough. So this is for starters. And this practices with LIME and other techniques already have been used for a while, so they exist. So this some data layer.
On a policies for the governance, think about them as you might want to propagate either your data source policies, or maybe your Azure ID or LDAP group policies that you have for your email use, your SharePoint use and all that. You want to propagate them automatically also for generative AI use and data, which is fed into generative AI.
So not have it as a silo and other silo create specifically for generative AI, but basically connecting it to our existing mechanism in organizations and interactions. Governance policies, they can go to, for example, the condition of patterns. This user coming from specific organization, let’s say, customer success, and suddenly they try to access financial information, maybe on-purpose, may not.
So there are different guardrails, which go not only to access policies, but also to intent, right? To understand the context of user, the context of the problem, the context of the data. And this is where I started my introduction with graphs. This is where graphs complete generative AI semantic models, to provide more and more context with those implementations.
Shane Hastie: A lot there and a lot for us to think about in terms of, what does governance look like in the implementation of generative AI? I think some good advice and a whole lot of things that our listeners can dig into. If I can switch topics a tiny bit, or quite a bit, the gender imbalance in tech. I know that your company is over 50% women. That’s pretty unusual.
Tackling the gender imbalance in tech [15:05]
Inna Tokarev Sela: It’s pretty unusual and intentional. I believe the talent is there and the talent is looking for environment, which can support specific balances. So every demographics requires a setup, which is the most adequate, and COVID taught all of us that we can be more flexible in the work environment from remote standpoint, from the hours standpoint and other aspects as well.
I think majority of software development companies, especially the big ones, software giants, are coming back to five days office policy. And I think it’s going to be discriminative to specific demographics on a scale. So in Illumex we’ve been happy and lucky to have this talent with us, but it does require specific setting to be facilitated, for sure.
Shane Hastie: So what are some of those policies? You mentioned flexibility and so forth. But, what are some of the concrete things that you’ve done at Illumex?
Concrete practices for flexibility [16:08]
Inna Tokarev Sela: It starts from the hiring process. Of course we only hire people who meet our standards. We do have a two days in office policy and those days could be flexible. We do support meetings, which are in core hours. It’s 10:00 AM to 4:00 PM, which means the majority of the people, either it’s fathers or the mothers can attend them without a disrupting the morning routine or the evening routine.
In general, we are flexible about sick days and out-of-office days for whatever reason. And this problem itself, because sometimes educational schedule could be disrupted and especially in this region it happens.
So of course, it’s you need to have home care. On the side, it shouldn’t be gender discriminative, because we want to have the same support for everyone in the company. And our kids ratio, I believe 2.3, among Illumex. We do have the dogs and we also have parents to dogs. So some people have dogs and cats. And to me we should support every needs and cater to the whatever flexibility everyone needs to. And also geographical. So geography wise, some employees might need full remote. Some employees commute could be longer than others and it should be taken into consideration as well.
Shane Hastie: Another thing I know that you are very passionate about and involved in is mentoring programs. How do we design and set up a good mentoring program?
Designing good mentoring programs [17:44]
Inna Tokarev Sela: I do believe in mentorship programs, which are specifically designed for female professionals. And this is due to the fact that communication is still different. So being heard or speak up does not come natural or does not come at ease, at just the same extent. And it’s all ego management in a group dynamics also comes to place. So I do believe in female-oriented networks, despite there is lots of conversations against that. And it bears fruit. So we see more and more female founders.
You can look at data companies are pretty wild growth of those numbers, but we also see data leaders. So chief data officers, heads of data, heads of analytics. Especially in this discipline of data analytics and generative AI, we see more and more female talent and I’m super happy to see that. I think balance is everything.
Shane Hastie: What’s the question I haven’t asked you that you would really like to share with the audience?
Inna Tokarev Sela: I’m really passionate about what future bears with all this new advancements and all those new technologies. It could be scary for some, because the change is accelerating and change is here. To me, as an industry software development, as data management industry, we under this overload of maintenance, of technical depth, of testing of all, let’s say, less creative tasks that we have on our plate every day. So we should embrace this innovation and use generative AI to augment our capacity.
And on a professional level, I’m passionate about application-free future. So who likes to go to 30 different interface over the day and have different tasks and different settings. So this is where I’m personally very passionate about and this is where illumex also helps companies to become closer to this future.
Shane Hastie: Well, a lot to think about there and a lot of good advice for our listeners. If people want to continue the conversation, where do they find you?
Inna Tokarev Sela: I am very active on LinkedIn. The social network has lots to offer, so please do connect to me on LinkedIn and I will be happy to continue the conversation there.
Shane Hastie: Thank you so much.
Inna Tokarev Sela: Of course. Thank you, Shane.
Mentioned:
.
From this page you also have access to our recorded show notes. They all have clickable links that will take you directly to that part of the audio.
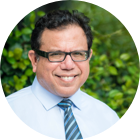
MMS • RSS
Posted on mongodb google news. Visit mongodb google news
Shares of MongoDB, Inc. (NASDAQ:MDB – Get Free Report) have earned an average rating of “Moderate Buy” from the thirty-one analysts that are currently covering the firm, Marketbeat.com reports. Two analysts have rated the stock with a sell rating, four have given a hold rating, twenty-three have assigned a buy rating and two have issued a strong buy rating on the company. The average 1 year target price among brokers that have updated their coverage on the stock in the last year is $361.00.
A number of equities analysts have weighed in on MDB shares. Royal Bank of Canada boosted their price target on MongoDB from $350.00 to $400.00 and gave the stock an “outperform” rating in a research report on Tuesday, December 10th. Needham & Company LLC upped their target price on MongoDB from $335.00 to $415.00 and gave the company a “buy” rating in a research report on Tuesday, December 10th. Guggenheim upgraded MongoDB from a “neutral” rating to a “buy” rating and set a $300.00 price target for the company in a research note on Monday, January 6th. Oppenheimer boosted their price target on MongoDB from $350.00 to $400.00 and gave the company an “outperform” rating in a research note on Tuesday, December 10th. Finally, Barclays cut their price target on MongoDB from $400.00 to $330.00 and set an “overweight” rating for the company in a research note on Friday, January 10th.
Check Out Our Latest Report on MDB
MongoDB Stock Performance
NASDAQ MDB opened at $292.97 on Friday. The stock has a fifty day moving average of $264.56 and a 200-day moving average of $271.42. The stock has a market capitalization of $21.82 billion, a P/E ratio of -106.92 and a beta of 1.28. MongoDB has a 52 week low of $212.74 and a 52 week high of $488.00.
MongoDB (NASDAQ:MDB – Get Free Report) last posted its quarterly earnings results on Monday, December 9th. The company reported $1.16 earnings per share (EPS) for the quarter, topping the consensus estimate of $0.68 by $0.48. MongoDB had a negative return on equity of 12.22% and a negative net margin of 10.46%. The business had revenue of $529.40 million for the quarter, compared to analyst estimates of $497.39 million. During the same period last year, the company earned $0.96 EPS. The firm’s revenue was up 22.3% on a year-over-year basis. Equities research analysts forecast that MongoDB will post -1.78 earnings per share for the current fiscal year.
Insider Transactions at MongoDB
In other MongoDB news, CAO Thomas Bull sold 1,000 shares of MongoDB stock in a transaction that occurred on Monday, December 9th. The shares were sold at an average price of $355.92, for a total transaction of $355,920.00. Following the transaction, the chief accounting officer now owns 15,068 shares of the company’s stock, valued at $5,363,002.56. This trade represents a 6.22 % decrease in their position. The transaction was disclosed in a filing with the Securities & Exchange Commission, which is available through the SEC website. Also, insider Cedric Pech sold 287 shares of MongoDB stock in a transaction that occurred on Thursday, January 2nd. The stock was sold at an average price of $234.09, for a total transaction of $67,183.83. Following the transaction, the insider now directly owns 24,390 shares in the company, valued at approximately $5,709,455.10. This represents a 1.16 % decrease in their position. The disclosure for this sale can be found here. Insiders have sold 43,094 shares of company stock valued at $11,705,293 in the last 90 days. 3.60% of the stock is currently owned by corporate insiders.
Institutional Investors Weigh In On MongoDB
Large investors have recently modified their holdings of the stock. GAMMA Investing LLC boosted its holdings in MongoDB by 178.8% in the 3rd quarter. GAMMA Investing LLC now owns 145 shares of the company’s stock valued at $39,000 after purchasing an additional 93 shares during the period. CWM LLC lifted its holdings in shares of MongoDB by 5.0% during the 3rd quarter. CWM LLC now owns 3,269 shares of the company’s stock worth $884,000 after acquiring an additional 156 shares during the last quarter. Creative Planning lifted its holdings in shares of MongoDB by 16.2% during the 3rd quarter. Creative Planning now owns 17,418 shares of the company’s stock worth $4,709,000 after acquiring an additional 2,427 shares during the last quarter. Bleakley Financial Group LLC lifted its stake in MongoDB by 10.5% in the third quarter. Bleakley Financial Group LLC now owns 939 shares of the company’s stock worth $254,000 after purchasing an additional 89 shares during the last quarter. Finally, Stonegate Investment Group LLC lifted its stake in MongoDB by 5.4% in the third quarter. Stonegate Investment Group LLC now owns 6,979 shares of the company’s stock worth $1,887,000 after purchasing an additional 360 shares during the last quarter. Hedge funds and other institutional investors own 89.29% of the company’s stock.
MongoDB Company Profile
MongoDB, Inc, together with its subsidiaries, provides general purpose database platform worldwide. The company provides MongoDB Atlas, a hosted multi-cloud database-as-a-service solution; MongoDB Enterprise Advanced, a commercial database server for enterprise customers to run in the cloud, on-premises, or in a hybrid environment; and Community Server, a free-to-download version of its database, which includes the functionality that developers need to get started with MongoDB.
See Also
This instant news alert was generated by narrative science technology and financial data from MarketBeat in order to provide readers with the fastest and most accurate reporting. This story was reviewed by MarketBeat’s editorial team prior to publication. Please send any questions or comments about this story to contact@marketbeat.com.
Before you make your next trade, you’ll want to hear this.
MarketBeat keeps track of Wall Street’s top-rated and best performing research analysts and the stocks they recommend to their clients on a daily basis.
Our team has identified the five stocks that top analysts are quietly whispering to their clients to buy now before the broader market catches on… and none of the big name stocks were on the list.
They believe these five stocks are the five best companies for investors to buy now…
Before you consider MongoDB, you’ll want to hear this.
MarketBeat keeps track of Wall Street’s top-rated and best performing research analysts and the stocks they recommend to their clients on a daily basis. MarketBeat has identified the five stocks that top analysts are quietly whispering to their clients to buy now before the broader market catches on… and MongoDB wasn’t on the list.
While MongoDB currently has a “Moderate Buy” rating among analysts, top-rated analysts believe these five stocks are better buys.
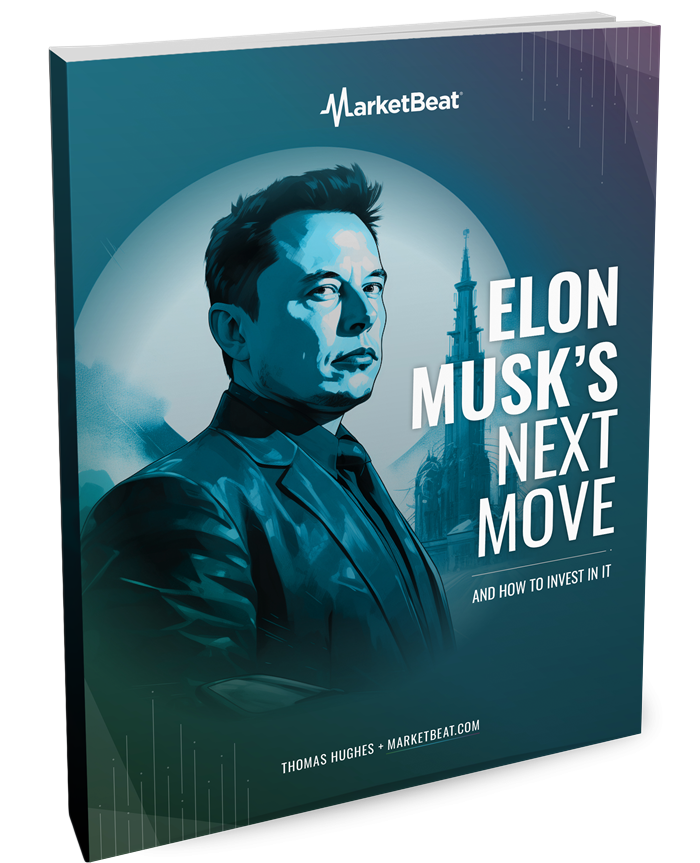
Wondering when you’ll finally be able to invest in SpaceX, Starlink, or X.AI? Enter your email address to learn when Elon Musk will let these companies finally IPO.
Article originally posted on mongodb google news. Visit mongodb google news
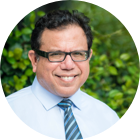
MMS • RSS
Posted on mongodb google news. Visit mongodb google news
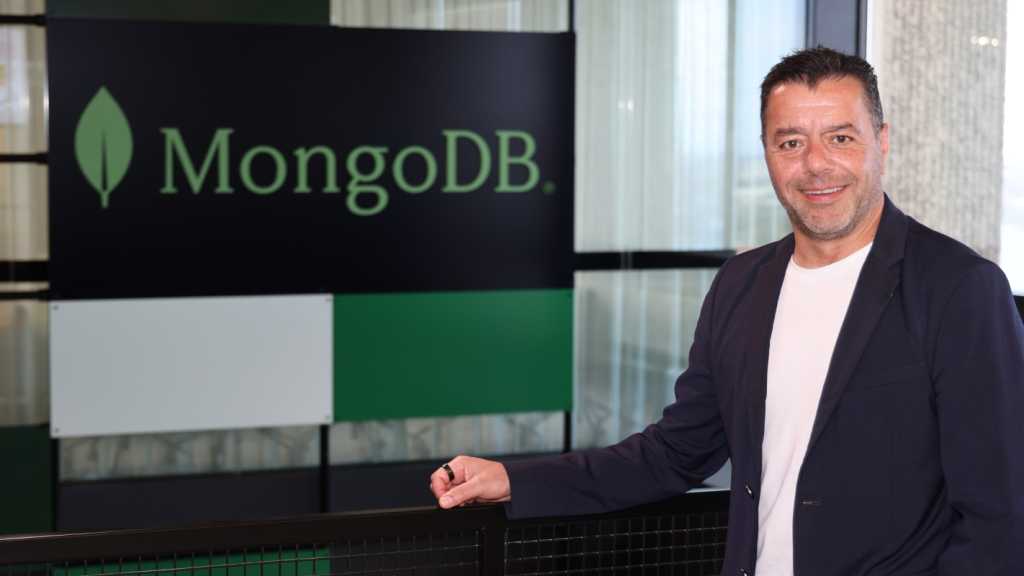
According to MongoDB, these events will include hands-on sessions with customers and partners, internal events and educational sessions for its community and MongoDB user groups.
Meanwhile, MongoDB Asia Pacific senior vice president Simon Eid said, local pilot projects and AI tooling built by the company’s local team are part of a template that is being rolled out globally.
“We’ve now got great new facilities to support MongoDB’s growing world-class teams across engineering, services, support and go-to-market functions,” he said.
Additionally, the company is planning to expand its local research and development teams, with it already holding 160 technical roles in Australia and New Zealand (A/NZ) out of its total of 220 employees.
Article originally posted on mongodb google news. Visit mongodb google news
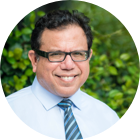
MMS • RSS
Posted on mongodb google news. Visit mongodb google news
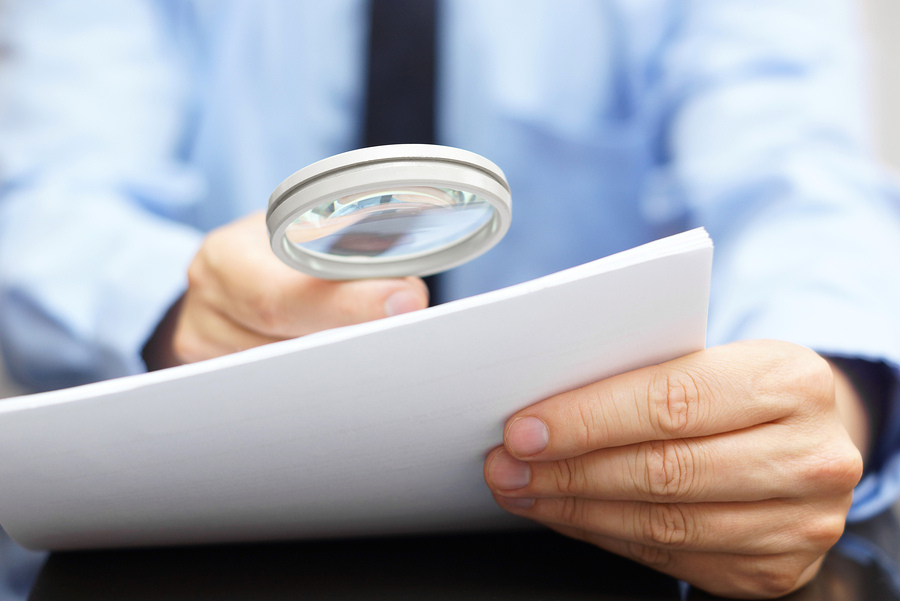
Article originally posted on mongodb google news. Visit mongodb google news
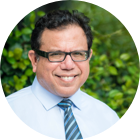
MMS • RSS
Posted on nosqlgooglealerts. Visit nosqlgooglealerts
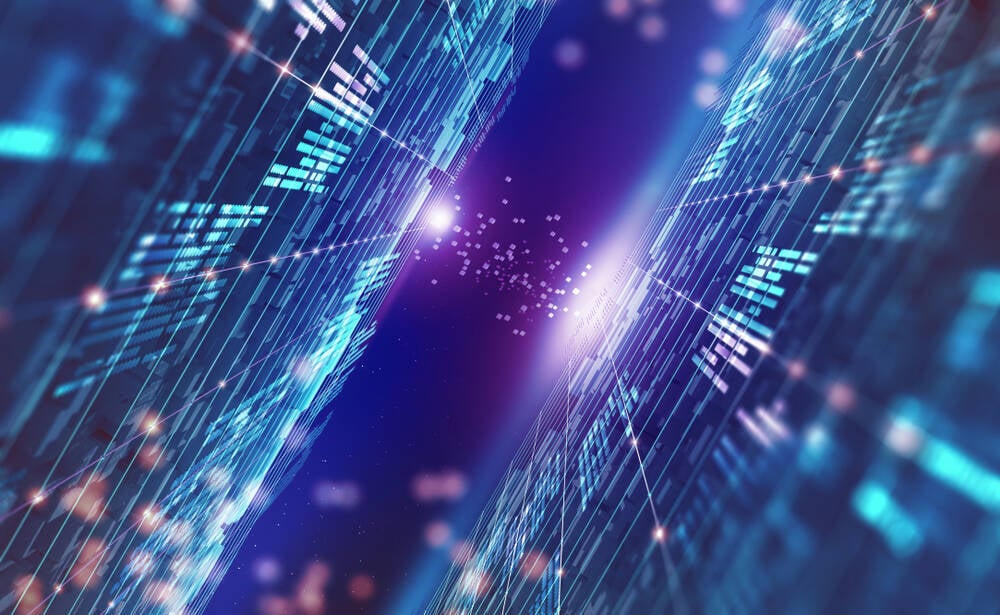
With its 8.0 release, distributed multi-model database Aerospike has added ACID transactions to support large-scale online transaction processing (OLTP) applications in a move it claims is an industry first.
Analysts have backed the release, saying it overcomes a “significant challenge” among distributed NoSQL databases.
Aerospike is a fully in-memory database that supports key-value, JSON document, graph, and vector search models. It began as Citrusleaf in 2010, rebranding as Aerospike in 2012 before going open source under Apache and AGPL in 2014. A commercial Enterprise Edition is also available.
It uses the concept of namespaces, akin to tablespaces in a relational database. Within the namespace reside sets, which are like tables in a relational database.
Aerospike may not be well known. Stack Overflow’s 2024 survey did not rank it among the top 35 databases used by developers. DB-Engines ranks it 61st overall. However, it counts multinational corporations such as PayPal, Barclays Bank, and Airtel among its customers.
Following the Aerospike 8 release earlier this month, chief product officer Srini Srinivasan told The Register that when NoSQL databases started, the idea was to compromise on consistency in order to provide higher performance.
“That was 15 years ago. Over time, every one of these NoSQL databases has added strong consistency features in transactions. Aerospike is focused very heavily on performance at scale. In 2018, we had a strong consistency release for single record operations, and with Aerospike 8 what we have is proper transaction support. This is classical transaction support, which has been around for 30 or 40 years in databases. We added that feature without compromising on the performance, especially for single operations. The performance continues to be the same as it was before this release.”
Some customers were achieving close to 100 million transactions per second on the distributed system, he claimed.
Noel Yuhanna, VP and principal analyst with Forrester Research, said Aerospike was well-known for powering high-performance real-time applications.
“A significant challenge with NoSQL distributed databases has been maintaining data consistency across the cluster, which is why many products support ‘eventually consistent’ databases. However, Couchbase, MongoDB, and YugabyteDB do offer distributed multi-document ACID transactions across clusters. With this announcement, Aerospike empowers customers to run transactional applications in a distributed environment while ensuring strong consistency, an important milestone for Aerospike customers. This provides a compelling value proposition, especially given its attractive TCO, making it an even more appealing choice for more businesses.”
Use cases have started with applications designed to detect fraud across global banking systems, for example, but have spread to gaming, mobile payment systems, and live sports betting.
Henry Cook, Gartner director analyst, said Aerospike’s claims about ACID compliance and distributed performance at scale were credible, and there was growing demand for applications that need this kind of performance.
“As we implement more distributed systems on a global basis, there is a need to support a globally consistent picture of the state of that system. This may be a common status for the underlying global database content, or to provide globally consistent metadata that describes the data and user status.”
Gartner senior director analyst Aaron Rosenbaum said other databases with similar kinds of performance might include CockroachDB or YugabyteDB, which use a PostgreSQL front end and a distributed back end. Meanwhile, hyperscale databases such as Amazon DynamoDB, Azure CosmosDB, and Google Spanner also play in the same category.
The only downside to such a specialist high-performance database might be the size of the vendor.
“Some of the databases come from smaller companies, and therefore, companies need to weigh up any dependency upon them,” Rosenbaum said. “However, the ease of management of the offerings from the hyperscalers is accompanied by lock-in to their cloud and product. Balancing the flexibility of deployment of independent vendors vs the simplicity of management of the hyperscalers is a challenge across data management, especially in this particular segment where distributed access is so powerful.”
Aerospike closed $109 million in VC funding in April last year and $30 million in growth financing in December. Yuhanna said the company was close to breaking even. ®
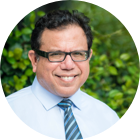
MMS • RSS
Posted on mongodb google news. Visit mongodb google news
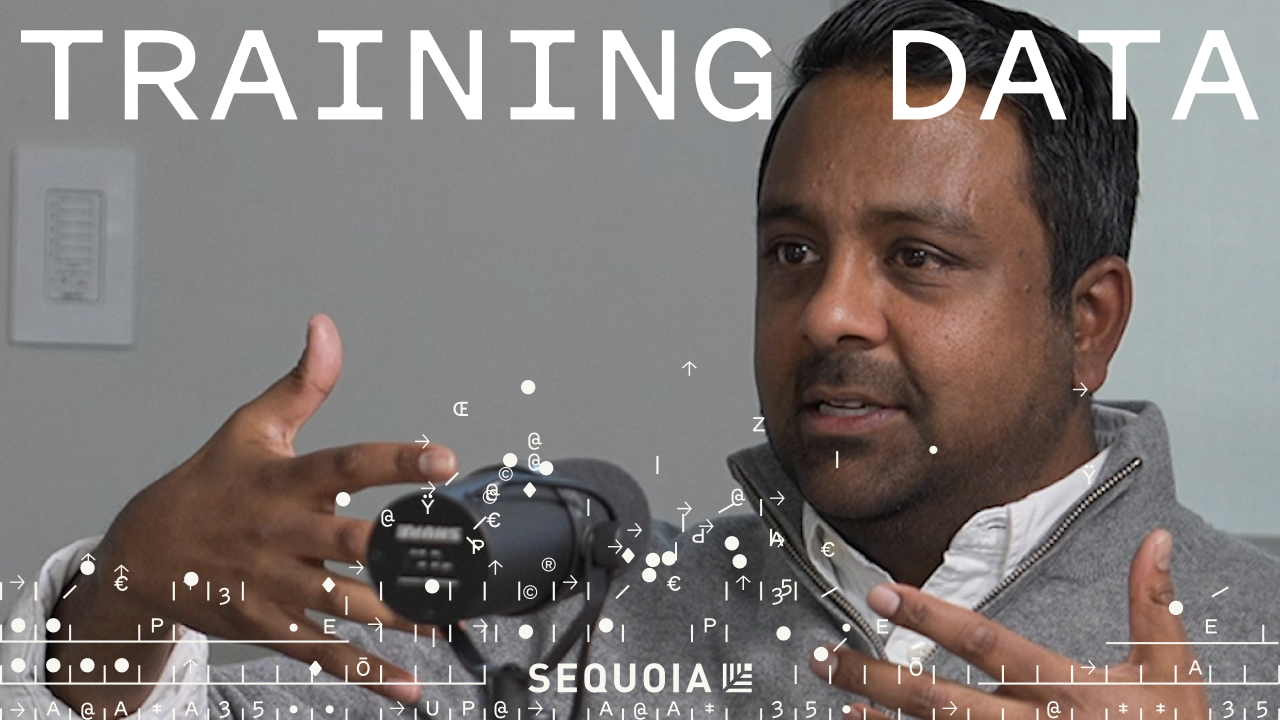
Contents
Sahir Azam: In a world of probabilistic software, you know, the measure of quality is about that kind of last mile. How do you get to 99.99X sort of quality? And so will the domain of sort of quality engineering that we typically associate with manufacturing kind of apply to software? And that really got me thinking in terms of you’re not gonna be able to necessarily get a deterministic result like you would with a traditional application talking to a traditional database. So therefore, the quality of your embedding models, how you construct your RAG architectures, merge it with the real-time view of what’s happening in the transactions in your business, that’s what’s gonna get you that high quality retrieval and result. And unless it’s high quality in a world where it’s probabilistic, I don’t see it going after mission critical use cases in a conservative enterprise. And that is a problem space we’re very focused on right now.
Sonya Huang: Today we are excited to welcome Sahir Azam, who leads product and growth at MongoDB.
Sahir was one of the architects behind Mongo’s successful transformation from on-prem to the cloud, and is now helping to steer Mongo’s evolution in an AI-first world.
Mongo’s journey into vector databases began with semantic search for e-commerce, but has evolved into something much more fundamental: becoming the memory and state layer for AI applications.
We’re excited to get Sahir’s take on the past and future of vector databases and what shape infrastructure will take in a world of AI agents and applications and unlimited software creation.
Sonya Huang: Sahir, welcome to the show. We’re so excited to have you here.
Sahir Azam: Thanks, Sonya. I’m super excited to be here.
Sonya Huang: We’re gonna dig into everything from vector databases to embeddings to knowledge graphs and much, much more on this episode. I’d love to just start with the big picture question and maybe your hot take: Is AI gonna change the database market?
Will AI change application development?
Sahir Azam: That’s an interesting question. I think the related and probably more interesting question is whether it’s gonna change software development in applications. And I think that it really is. You know, I think we’re seeing AI-powered—generative AI-powered applications address a set of use cases that traditional kind of deterministic software hasn’t been able to go after. And I know I’ve read some stuff from Sequoia around the idea of services as software, et cetera in that whole space. And we firmly see that in terms of our early adoption of what we’re seeing in the market. And so that in turn changes the fundamental way we will interact with software, it changes the way business logic of applications will evolve over time with things like agents. And that all has underlying implications on how the database layer will need to transform as well.
Pat Grady: Can we poke on that for just a minute? So I’m curious, since you guys are operating at a layer where you see a lot of what’s being developed? What are people developing today that they could not have developed a few years ago before these capabilities emerged?
Sahir Azam: Yeah, I think on one hand, one trend we’re seeing is certainly that it’s much more easy and efficient to create software than there ever was before. So, you know, the fact that there will be more software in the world means that that’ll have implications in terms of data persistence, storage and processing. So that’s kind of one sort of related piece.
But in terms of use cases, I think the fact that we can now interact with computers in completely different ways beyond just the classic web and mobile applications that we’re all used to, you know, the more interactive experiences, I think the blending of the physical and virtual worlds in ways that I don’t think we’ve really seen yet. Obviously there’s a big trend around how AI impacts robotics. There’s a great blog I read from, I think it was from LangChain the other day, around sort of ambient agents and reacting to signals without necessarily intentional human action.
I think we’re at the very early stages of that top layer of sort of human-computer interaction fundamentally changing. And I think that can now tackle a whole bunch of use cases in terms of improving the productivity of our personal lives, our professional lives, and go after, you know, fundamental productivity that I don’t think traditional software has gone after. And I think that’s the biggest kind of meta change that I think this all has the potential to go after.
Customer use cases
Pat Grady: Do you have a favorite example, either one—either a Mongo customer—obviously you love all of your customers, but do you have a favorite use case either that you’ve seen one of your customers build or a favorite use case that you yourself use?
Sahir Azam: Yeah, I would say generally we’re seeing more—and like most things, we see more sophisticated, advanced use cases tend to come up first in, you know, more risk tolerant, sort of faster-moving startups. But for that reason I’ll pick a couple enterprise use cases that have kind of captured our imagination. One is we worked with a large automaker in Europe and, you know, they have huge fleets of cars globally. They have a bunch of first and third party, you know, mechanics and maintenance sites where people, whether they’re dealers or other sites where people go to get help when their cars are having issues. And the common problem of I hear something funny with my car, like how do I go diagnose this? It means that you typically go in, a mechanic who has expertise has to go kind of tinker around, figure out what it is and then go through a manual to figure out what the remediation steps is, or what parts they have to order to fix it.
Pat Grady: Yeah.
Sahir Azam: We work with them to actually identify an audio embedding model that can allow them to record with a phone the corpus, and semantically match that with a corpus of sounds that are typical problems that are known problems with their cars or any cars, which shrinks down the actual diagnosis time by what typically could take hours if it was a tricky diagnosis, to something that could take now seconds. It’s almost like Shazam for car diagnostics.
Pat Grady: [laughs]
Sahir Azam: And then on the other side of it, instead of looking through PDFs or, you know, physical manuals on what the approved remediation steps are to fix it, now it’s sort of a natural language interface to say, “Okay, this is the issue that we match to. What should I do next in terms of fixing the problem?” And that’s all about unstructured data, semantic meaning of the information both in the problem with that car, and if you extrapolate the business case of that, though, across thousands of dealerships or hundreds of different models and iterations of cars, that’s millions of dollars of potential savings for them, and a better customer experience and consumer sentiment around their brand. And so that was kind of definitely one cool one.
Another one in a more very heavily-regulated industry. Worked with Novo Nordisk, you know, one of the largest pharmaceuticals. You know, obviously getting a drug approved is a highly-scrutinized process. And so there’s this idea of a clinical study report that pharmaceutical companies have to fill out, which typically takes a lot of manual effort to write and structure and review and, you know, kind of get approved. They were basically able to use a large language model, train that against all their approved drugs, all the process they do manually, and now they can get that initial draft of a CSR, as they call it, within a few minutes.
Pat Grady: Hmm.
Sahir Azam: And so it shrinks a lot of just the initial drafting cycles. The quality of that initial draft is higher than what they typically see if it was manually done. And so again, like, you can draw a pretty quick line towards true dollar ROI savings on use cases that are not necessarily even bleeding edge in some aspects of what we’re seeing in the sort of early stage ecosystem, but are being applied in a context of scale in industries that obviously have big implications for them and for their customers.
Pat Grady: Yep.
AI applications: good news or bad news for databases?
Sonya Huang: So now that the shape of these applications is changing and, you know, they’re multimodal, as you said, they’re agentic, they’re ambient agentic, what does that mean for the database layer? And if you wouldn’t mind just giving us the 101 today of, like, the role that databases play for software as we know it today, deterministic software. And what role do you see databases playing in this kind of new evolving market for AI applications?
Pat Grady: Is it good news or bad news?
Sahir Azam: We’re excited. So it’s one point I lightly brought up earlier, which is if there’s more software in the world, which I think generative AI will just make it easier to create more types of software experiences, I think that in general is a tailwind for any data persistence infrastructure technology. It doesn’t necessarily mean that MongoDB or any other particular vendor is automatically going to be the beneficiary. There’s a lot of execution that goes into making sure we’re technologically, and for our partnerships and ecosystems, well set up for that, which is where I spend a lot of my time. But in general, like, more software means more data and needs for persistence of that information. So that’s a very macro sort of, I think, tailwind that we’re definitely excited about.
I think the shift from relatively simplistic gen AI use cases, oftentimes where you’re just interacting via chat with an LLM, it doesn’t necessarily need very advanced kind of data persistence. But as enterprises need to ground the results of their AI applications to proprietary information or to control the result set so the retrieval is of high quality, now there needs to be a lot of interaction with these foundational models and their underlying information about how they run their business. And a lot of that is not necessarily publicly trainable information on the internet.
And so whether that’s advanced or simplistic RAG workflows, whether that’s fine tuning different approaches around post training there, I think there will be more need to interact with an enterprise’s data and foundational models over time, especially as these models become lower latency, and so they interact more with the real-time business data that’s being generated in an organization. And that’s really what we’re seeing in the most advanced companies right now is they’re building really sophisticated ways to control the output of these LLMs based on the use case that they’re trying to drive towards and merging it with the operational data that drives their application or their business.
And so I think we’re still early days in that, in terms of where I think that can go, but I really do fundamentally believe the databases will need to get much better at high-quality retrieval, in particular of unstructured data. Because, you know, when I look at all these embedding models and just what we can do with probabilistic software, it takes the value out of 70 percent of the world’s data, unstructured data, and makes it applicable to applications in a way that just really wasn’t possible before. And I think that’s the real opportunity.
Sonya Huang: What’s the devil’s advocate answer to that? So for example, I’m thinking of Jensen at our first AI Ascent. I think you were at that AI Ascent and he said something like, “Every pixel is gonna be generated, not rendered.” And I think of rendered as, you know, retrieved from a database somewhere. What is the devil’s advocate point of view to the—is it good or bad for databases as generative AI takes off?
Sahir Azam: Yeah, I think the devil’s advocate view to me is less about whether there is a database somewhere behind the scenes, more about where is that abstraction, and is that something that’s occurring a choice of the application developer building that application, or is it abstracted behind some higher level API? Or is that a choice that an LLM makes, in terms of, as it auto-generates software or auto-renders that environment, where does it choose to persist that data?
Sonya Huang: Yeah.
Sahir Azam: But at the end of the day, you know, we like to joke internally, an AI application is still an application. You still need to persist transactions safely to make sure people’s bank balances are accurate. You still need the ability to search information based on text keywords, not only on the semantic meaning. And so I view all these generative AI needs from the data layer as additive, not necessarily substitutive to the needs of a traditional application.
Sonya Huang: And, you know, one of the reasons people love Mongo today is the developer experience, right? If you fast forward the clock, and maybe there’s x-hundred-million human software developers, but there’s trillions of, call it, agentic developers. What makes a good agent developer experience? Like, why would an agent choose to use Mongo as its database, if that even makes—does that make sense as a question?
Sahir Azam: Yeah, I think it does. And it’s something—we think a lot about sort of how the nature of software development will change. And I think one of the things as we move from more simplistic generative AI kind of powered applications to more advanced ones with more agent-driven business logic, state will be more necessary, because now you’re coordinating a more complicated workflow where you need to be able to track the results of a particular piece of a transaction and coordinate that. And all of that requires storing that somewhere and manipulating and updating it over time.
So I think in general things are becoming more state-ful in generative AI applications over time, which is a drag of data and database consumption overall in terms of where things are going. Now I think in terms of the abstraction, I think the question is: If developer experience is the thing that makes any technology really accessible today for human developers, does that same value proposition hold for AI? And I think what we’re seeing, even if you look beyond just the database space, think of, you know, the adoption we’re seeing of some of these, call it AI platform-as-a-service type companies, you know, look at the adoption of things like Vercel v0, or you see things like Replit or whatnot, I think we’re seeing that, at least with early AI-generated software, there’s a preference for great developer experience à la higher levels of abstraction. So I think it’s too early to be definitive on that but, you know, I think we’re seeing some really promising signs.
Pat Grady: Speaking of higher levels of abstraction, I forget who had this one liner. Somebody had the good one liner which was, you know, English is the ultimate layer of abstraction, right? And at the limit you will just be able to describe in plain English, you know, what product requirements you have, and a foundation model will spit out the code required to, you know, build whatever application you want to build. First off, do you believe in that as a future state? And then secondly, is that great news for Mongo because there’s just going to be so much more software and most of it’s going to need a database sitting beneath it? Or is that bad news for Mongo because it neuters some of that development experience that is a good advantage for you? Do you see that playing out, and what does that mean for Mongo?
Sahir Azam: Yeah, I think for databases in general, I feel pretty confident it’s absolutely a tailwind. I think MongoDB specifically, one of the advantages we have is that our data model is really well attuned to managing structured data, semi-structured data, and now with embeddings, unstructured data. So I think we have some fundamental architectural advantages we believe are even more prevalent and important in AI as you’re representing all these forms of data, regardless of whether the software above it that’s interacting with it is human generated or machine generated, so to speak.
Now that being said, we’re certainly not resting on our laurels that that’s gonna happen without us being really intentional about it. So we are working with the whole ecosystem of AI frameworks and model providers to make sure that we are well integrated, whether it’s inference players or dev frameworks, et cetera, to make sure that just like JavaScript and Web 2.0 and Cloud were big tailwinds and are big drivers of our business, that the modern stacks that are being used to generate these applications, MongoDB is well integrated as a default in. So I think there’s a lot of work happening there.
We’re also focused on this idea of what is the equivalent of, you know, quality training or even SEO for LLMs? Meaning if you go scrape the internet to train a code assistant on any technology, is that necessarily what the best practices are? Probably not. But there’s no standard way for a vendor or a technology expert behind a particular area to submit the canonical training data for quality MongoDB code, for example. And so we’re working with some of the labs on methodologies around that. We’re doing things just even without involvement to test sort of what we can be doing to create data sets that allow for the quality of the outputs of these systems to be reliable. Last thing we want is somebody going and saying, “I want to use MongoDB. Help me generate some code for some functionality,” and it’s not high quality, performs poorly. And so there’s various facets of this that I think are very intentional efforts to make sure that our technology fits well as things evolve over the next few years.
Pat Grady: Yeah.
Sonya Huang: So actually to that point, I think there’s been a lot of chatter and increasing chatter that, you know, we’re hitting a wall in terms of just public data globally available.
Sahir Azam: Mm-hmm.
Sonya Huang: There’s a lot of data still left in private enterprise data. You guys sit in the middle of a lot of it. I’m curious how you think about your role in, kind of, that as the market evolves towards this next leg of finding that next trillion tokens’ worth of training data. Do you see yourselves, you know, being a training data provider for your customers? Do you see yourselves partnering with the labs? Are your customers mostly looking to use their data in Mongo for RAG, or are they looking at also training models on the data they have in your systems?
Sahir Azam: Yeah. Definitely, I think, just to be clear, any of the data that we manage on behalf of our customers is owned by our customers. So we’re certainly not taking that data and training any models that are outside of what that customer wants us to train or use for RAG. So I think that definitely is where more of our focus is. And we see a variety of differing things—very simplistic kind of use cases where people are just using core operational data stored in MongoDB or metadata as part of their RAG workflows. We’re seeing obviously a lot of vector adoption; it’s our fastest growing new product area as they try to merge metadata, transactional data and semantic search together into a single sort of system for more quality retrieval kind of use cases, which is sort of, I think, where the market’s going.
And then we see instances where people want to use the data they have in MongoDB and other systems to either fine tune or straight up train smaller models that are specific to a particular use case. And I don’t believe that there’ll be kind of one particular modality that suits every single use case. I think there’s going to be a plethora of different things that customers will begin to optimize for their latency requirements or performance requirements.
Are vector databases a new category?
Sonya Huang: So I think you have the most fascinating seat to what’s happening in the vector database market. We constantly poll our portfolio on what their AI stack is, and consistently Mongo has been the number one vendor that everyone uses for vector databases, so I think you have the deepest and most interesting perspective on this. Maybe, like, from the 20,000 foot view, it seems like people are using LLMs as—you know, they have world knowledge up to some pre-training cutoff date, but beyond that you need RAG and you need vector databases in order to supplement knowledge, to provide specific domain knowledge almost as in information retrieval knowledge source. But if I look at vector databases, they kind of came from the semantic search world and e-commerce and things like that. And so that’s a very different world. So how do you think about—what are people using vector databases for today? Is it a technology of the past that’s being improperly shoehorned into this information retrieval use case, or is it the ideal data structure to kind of be the knowledge infrastructure for LLMs? Like, how do you think this all plays out?
Pat Grady: Yeah, can I ask a quick question on that, too?
Sahir Azam: Of course.
Pat Grady: Did Mongo—I’m aware of Mongo’s vector database because of generative AI and seeing people use it for generative AI.
Sahir Azam: Sure.
Pat Grady: Did you guys have a vector database pre generative AI?
Sahir Azam: We started because of a more classic—classic—semantic search use case.
Pat Grady: Okay.
Sahir Azam: So a few years ago, one of the things we noticed were that many of our customers would use MongoDB as an operational data or any operational data, and side by side with it have an inverted index kind of search engine for full text kind of lexical search. And our customers are basically like, “Why do I have to copy data between these systems to run two different databases just to get the search results I want to empower my application with?” And so being focused on developer experience and simplicity we’re like, this seems like an obvious problem for us to go after.
And so we started there with our search product to really just simplify it so a developer interfaces with one database, but really it has different modalities of indexing and storage that can serve OLTP-type queries as well as full text search queries. Some of our e-commerce, advanced e-commerce customers were the ones then saying, “Okay, that’s great. But I want to start to do semantic similarity search and blend full text lexical search alongside similarity search, because that’s what’s going to give me higher quality search results.” And that’s where we started getting pulled into building the vector capabilities into our engine.
And for us, one of the things we’re always trying to do is remove the need for customers to have multiple systems. So when we say we added this capability, a lot of it goes to how do we integrate it in an elegant way to our data model, how do we extend our query language so it’s very easy for a developer to just feel like it’s not a separate system, they’re just interacting with it as part of their application development.
And so we were down that line, and then obviously the world explodes post ChatGPT. And we were like, “All right, this is going to be even more relevant than we thought.” And so we poured the gas on things, accelerated things, expanded the strategy to be well-integrated into a whole bunch of new frameworks, working a lot more closely with the AI labs, because it is, to Sonya your point, it’s certainly a different use case to leverage vector embeddings or even just metadata or transactional data to integrate to RAG than just a pure semantic search use case.
But as we look at our most advanced customers now in 2025, they’re actually seeing that the integration of all those modalities is really important because you need to filter based on metadata you know about your unstructured data, whatever it is you’re building an application around. There are times when you need to sort by keywords and relevance ranking like a more traditional search engine, and then you need to understand and extract semantic meaning from vector embeddings. And there’s a whole bunch of things around how to improve the quality of that. And only then can their overall application get the percentage quality predictability—especially for large enterprise—to trust putting something in front of their customers, especially in a regulated industry.
And so that’s turned out to be a real advantage to have all of those in a single system, because otherwise it requires a whole bunch of what I call kind of RAG gymnastics to try to tie all these things together, which is possible, but it puts a whole huge burden around the development cycle, what happens in app code. And frankly, you need to be a pretty sophisticated team to figure that out on your own. And so we’re trying to democratize that all by making it just much simpler for the average application developer.
Pat Grady: How do you think about vector versus graph? Are they substitutes? Are they complements? What are the trade offs? Because we see vector-based RAG. We also see graph RAG.
Sahir Azam: Yeah. Yeah, every week goes by and there’s some new sort of approach to higher quality retrieval is kind of what I think everyone’s sort of trying to chase. I think they’re complementary. You know, there are reasons why you want graph relationships because that’s an augmentation of understanding that you may not be able to just infer by the vector embeddings themselves. So we view that as additive, just like pre-filtering based on some sort of metadata you know about your unstructured data and embeddings is additive and improves the quality of results. And so I do view these modalities as very complementary.
Our goal is to just make it simple to combine all of those for a developer so they don’t need to have their graph representations of their objects in one style of database, their metadata in another database, their transactional data in a relational database, and then have to have a separate vector search database and try to rationalize all that, which is kind of what happens. We’re trying to just make that dead simple.
Sonya Huang: Is it fair to simply think about in an agentic system, the LLM as the brain, and the database, whether it’s a vector database or superset of those as the memory? Is it brain and memory? Is that the right abstraction, the right mental model?
More state to persist
Sahir Azam: I think that’s definitely one way to think about it, because absolutely you need to persist memory and state, especially when you have agents that are having more complex workflows and need to drive interaction across multiple endpoints, not necessarily a single foundational LLM with a one-shot call. So you need to persist more of that state. I view them as sort of two pieces of an emerging architecture. You know, you’ve got obviously compute, storage and networking as sort of the underlying primitives, but now there’s this whole set of use cases that foundational LLMs can go after that are more probabilistic in nature, that can automate tasks that knowledge workers would typically have to do manually, which is super powerful. But then that needs to store its state and be grounded and interact with the transaction that the application is driving, and the other information that’s either semi-structured or structured.
And those things together come to create a great application experience and end user experience. It’s not an either/or. I think it’s complementary in a really powerful way, which will only become more important as LLMs become lower latency and faster. Where now you can really use what’s happening in a real world setting to augment the results of an LLM, and much closer to real time than today where it’s just a very different interaction speed.
Sonya Huang: So you’re saying the database is not only the memory for the LLM, but it’s a reflection of world state.
Sahir Azam: Yeah.
Sonya Huang: Like, LLM needs to interact with world state.
Sahir Aazam: Exactly.
Pat Grady: Well, I think that rough framing is consistent with what we’ve talked about internally, which if you think about the bottom as raw infrastructure, compute, network and storage, you think about the top as the application, you’ve got all this stuff in the middle, and for anything that is deterministic, you’re going to be better off with vector database, graph database, relational database, NoSQL database, kind of the traditional database world. For anything that’s more probabilistic, you want something that looks like an LLM. The functionality that gives you is a little bit of human-computer interaction and a little bit of reasoning, which is complementary to what you get from this part of the world.
But I want to take it one step further because it sounds like we’re pretty similar view on this default architecture of the future or kind of this emerging pattern. If you take it one step further, does that imply that the mental model investors should have for the API portion of Anthropic or OpenAI or the other foundation model companies is Mongo? Meaning they’re occupying a similar layer in the stack, they both reside on top of the public clouds, they both reside beneath the application layer. Is Mongo a good frame of reference for what the API businesses of OpenAI and Anthropic would or should or could become over time?
Sahir Azam: Yeah, I think it’s an interesting proxy because you sometimes read, like, okay, the LLM is the new operating system. That never felt logical to me in terms of how application capability and functionality should look. Maybe I’m wrong, things are changing so fast these days. But what we see is really these are side-by-side complementary components that drive and serve the business logic and interaction layer of the application above.
Pat Grady: Yeah.
Sahir Azam: And there’s a whole bunch of use cases obviously that large language models can now reason about and provide human interaction around that weren’t possible before. That’s the amazing, powerful aspect of them. But it doesn’t, you know, in any architecture we’ve seen, supplant the need to have deterministic outputs from structured data to manage transactions and search and all the other data components. It’s really complementary. And I think it’s still early days. I think Sequoia has done a great job sort of writing about as well. We don’t know what the real next generation business models and applications are yet today. I think we’re still seeing the early years of it, and that’s what’s fun, to be able to see all these different early stage companies or these enterprise use cases that I highlighted earlier. Even then, I think there’s a lot more to come.
Pat Grady: Yeah, all hypotheses at the moment.
Sahir Azam: Yes.
Sonya Huang: I mean, speaking of hypotheses, there’s all these hypotheses about, you know, what model architectures are going to leapfrog, and what the next model architectures are going to be. I’m curious your hypotheses on the database side. So we went from nothing to vector databases pretty quickly, it seems like. Do you think we’re going to leapfrog to a new type of data structure for AI, for these AI systems, or do you think this is kind of the ideal architecture?
Sahir Azam: Yeah, I think the fundamental data architecture, at least as far as vectors are concerned, seem to be strong primitives that seem to hold, and where I think we’re still trying to figure out how we extract all the possibility there. Now if something else comes along, certainly open minded to it, but I think it is a primitive in my mind. You know, I think there was a question in the market at some point of, like, all right, is a vector database a whole new segment in the market, or is that going to replace core databases? We view it as a primitive. Like, if you want to manage unstructured data, the combination of the ability to index and vector embeddings combined with high quality embedding models that can represent the meaning of the unstructured data is sort of a new primitive, just like text indexes or B-tree indexes and databases, et cetera.
So we view it as a foundational element. I don’t see that going away. I think how you create high quality results from that data and how you have high quality vector embeddings or how you augment that with other information, there’s a whole lot of evolution happening there right now. And I don’t think that’s by any means settled.
Sonya Huang: I see. So the data structure, the data storage, that’s vectors and the way you store them seems pretty sound. And the thing that’s yet to be optimized is how do you go from all these vectors to ultimately meaning and …
Sahir Azam: Yeah, and I’m not saying there aren’t going to be optimizations or room for innovation and how that can be more efficient, more performant, more cost effective. There’s plenty always in the database space happening there. So I’m not trying to make a statement that there’s certainly innovation going on there. But I think the more interesting thing is when you’re in a world of probabilistic software—and I heard a really interesting take on this from Ben Thompson who writes for Stratechery, where he kind of said in a world of probabilistic software, the measure of quality is about that kind of last mile. How do you get to 99.99X sort of quality? And so will the domain of sort of quality engineering that we typically associate with manufacturing kind of apply to software? And that really got me think in terms of you’re not going to be able to necessarily get a deterministic result like you would with a traditional application talking to a traditional database. So therefore, the quality of your embedding models, how you construct your RAG architectures, merge it with the real time view of what’s happening in the transactions in your business, that’s what’s going to get you that high quality retrieval and result. And unless it’s high quality in a world where it’s probabilistic, I don’t see it going after mission critical use cases in a conservative enterprise. And that is a problem space we’re very focused on right now.
Sonya Huang: How do you think all the innovation in the reasoning model side interplays with what’s happening in your corner of the world?
Sahir Azam: Yeah, I think in terms of whenever there’s reasoning, memory comes into place, long running logic. I think then how reasoning plays into more advanced agentic workflows, all of that needs state as I kind of mentioned earlier. So at a very loose level I think databases are going to be more important to that than just a one-shot simple answer engine from an LLM. So I think that’s the kind of meta trend. As an end user I’m fascinated by these types of reasoning models. I mean, I am definitely a—I know this is very not exactly novel in the last couple weeks, but Google’s Gemini Deep Research and the product experience around that I think is amazing. So I think there’s a lot that can be done there in terms of the user experiences and the types of use cases that applications can build off of that at least the first wave of LLMs that we saw haven’t been able to really drive in terms of adoption.
MongoDB’s business transformation
Pat Grady: Very different direction. So one of the things about your background that people who are listening might not be aware of is that you sort of like architected and led the transformation of MongoDB from being a traditional on prem enterprise software business to being a cloud native consumption-based business, which is now most of MongoDB. And I think any transformation of that magnitude is really hard to pull off, and you guys did it at reasonable scale. And of course now the company has, you know, billions of revenue scale. The reason I’m harping on this a little bit is I think there are probably a lot of enterprises or even a lot of startups who are currently faced with a similar challenge where they need to undergo a transformation of their business. And yours was an on prem to cloud transformation, which not a lot of companies got right. The one we’re looking at now is sort of a non-AI to AI transformation. And so the question is: What made that work for Mongo? Maybe just say a little bit about the nature of the transformation. What made that work for you guys? And do you have any advice for people who are looking at an AI transformation of some sort now?
Sahir Azam: Yeah, I appreciate you bringing that up, and certainly we’re very lucky and fortunate that we were able to make this pretty monumental shift in terms of the business model, the product strategy of the company. And certainly, by all means it required a lot of different people doing a lot of different things to make that happen. But I think one important piece I want to key off is you’re using the word kind of “business transformation.”
Pat Grady: Yeah.
Sahir Azam: That is really important because I think for a lot of companies that have tried to drive this type of a transition, they just view it as “Okay, this is a new SKU, a new product. That’s all I have to worry about.” But I think certainly I took it as a sort of business transformation as the goal here, and therefore we made sure that every functional leader in the organization one, understood that they had a really important part of that transformation and were also accountable for working to think about in a consumption-based cloud-first model, how customer success changes, how our financial model changes, how you can name any single function.
Pat Grady: How did you guys get buy-in in the early days when the thing that generates all the revenue was not this? Like, how did you get people to care?
Sahir Azam: Yeah, absolutely. So one, definitely having strong top-down support. You know, like, it was very clear to the company that launching Atlas, making this transition was a super critical business priority. You know, there’s nothing that gets around the fact that you need that level of top-down consistency. You know, that included empowering me as sort of the person to help drive that. And so when I went knocking on one of my peer’s doors in a particular function, I said, “Hey, I really think we need to fund some headcount here to think about the cloud side of the business,” that I had the sort of ability to kind of drive that level of influence.
But I think what’s important about that is we didn’t treat it as this sort of separate mini BU that’s isolated from the core business. We wanted every functional leader to feel like they were part of that transition and it wasn’t some competing thing for—you know, and they were going to lose some sort of part of the function they ran. So I think that was a really important thing. Certainly it meant a lot more shuttle diplomacy for me versus direct authority, but that was critical to bring the whole company along for that transition as opposed to it just being a starved new business initiative in a corner, which you see sometimes start to happen.
Certainly in terms of the sales organization, the revenue functions in particular, it took a lot of one, just really rolling up sleeves and being a seller, meaning being in the early deals, learning what objections are coming up, whether that’s a product objection we had to go build on the roadmap, or whether it was just an enablement issue or a positioning or messaging exercise or pricing thing. So really taking a mindset of like, you know, our team, the product team launching this is going to be side by side with the sellers and the SAs in every single one of the first deals. And I remember in our smaller New York office at the time, I used to make the rounds every evening and be like, “All right, what’s happening with this deal? What help do you need? Where are we on this? What are you hearing?” And that got a lot of sort of one, all right, the sales team isn’t just being asked by some stranger to do something because it’s important. I was trying to show that I’m in it with them.
And then certainly you have to drive incentives around it. When something’s working and people know how to drive revenue a certain way in any function, there’s going to be so much inertia around that already because the software business is still a growth business for us. So we had to be very intentional about putting SPIFFs, heavy emphasis on enablement, inspection and accountability to make sure enough momentum got built in the new business until we could kind of neutralize it. Because ultimately we’re about customer choice. We don’t want to artificially push a customer that’s on prem to the cloud if they’re not ready. That’s largely out of our control. But in the beginning, we needed the sales team to get a lot of attention on something that they felt was not necessarily the needle mover until we got a certain level of momentum.
Pat Grady: Yeah. Yeah, interesting. The lessons I heard for anybody going through an AI transformation is a lot of top down support, which I imagine requires a lot of conviction that this is where the future is going. Fully integrated, not some projects sitting off in a corner, getting starved for resources, but actually part of the core business. And holistic transformation. It’s not a SKU, it’s a wholesale reinvention of the business in a lot of ways.
Sahir Azam: Right. And some of the most important things were not technology decisions. It was, you know, business model transition. It’s sales enablement to sell to a different segment of the buyer in the organization, different buyer within the organization than we were traditionally. So almost every function had to change in pretty fundamental ways. And I think sometimes an outsized amount of our time went to those things that you wouldn’t think or needed to change that much or that would be easier, versus what you assume to be the hard part, which is how do you deliver a highly reliable cloud database? That’s by no means easy, but that’s the part I think everyone gravitates to. But it’s all these other things around the different functions that drive the business and making sure all those line up in a coherent way that a lot of attention went to.
Sonya Huang: I also think one of the analogies to draw—and tell me if this is just I’m off in la la land, but in our conversations, you were really focused on driving the developer experience through that period of transition, and the developer was going to choose the database for this kind of new mode of operating. It feels like to me, for companies going through the AI transition right now, right now it still is developer developer developer. To your point, developers are choosing AI tools. Eventually, if we have trillions of agents running around, it might be the agent’s experience that’s the thing to really prioritize.
Sahir Azam: Yeah. Especially if agents are the ones who are going to be driving a lot of the business logic without necessarily custom development happening by the organization. I could see that. I think oftentimes from the outside I get the question of how did Mongo go from enterprise to PLG? And I always sort of like, wince at that. You know, I think to me those things are absolutely complementary, and more have to do with where a customer is in their adoption journey or what style of organization they are, whether they’re a technical founder-led, fast-moving startup that doesn’t want to necessarily engage with sales in the beginning of their journey, or whether it’s a large enterprise that’s never going to show up via a self-service type channel. And so we spent a lot of time thinking about the whole system holistically, and trying to map that to how the users and the buyers actually want to engage with us as a company. And so I think a lot of that is what has been behind the cloud transition sort of success. It’s not trying to be too philosophical of saying, “Credit card business customers are the right ones, and enterprise sales? No way.” I mean, it’s neither. Both of them have to be cohesively integrated to reach the global scale of customers that we have at this stage.
Lightning round
Sonya Huang: Should we wrap with some AI rapid-fire questions?
Sahir Azam: All right. Sounds good.
Sonya Huang: Okay. First one: Favorite new AI app.
Sahir Azam: All right, I mentioned that I’m definitely a Gemini Deep Research fan, so that I mentioned. I think that and also Perplexity for me—they’re not new by any definition—in my mind run counter to the okay, thin AI wrappers aren’t really sustainable, because I see a lot of product craft. And I know Gemini obviously has a deep model training behind it, but just the product craft is what I think is really interesting. Like, the way Perplexity makes the user experience, the design sense, for example, is really great as an end user. So I don’t think it’s so simple that AI models are suddenly going to make software go away. I think there’s a lot around adoption and understanding your user, having great design sense. And there’ll be a version of that as we go to other interactive modalities as well, even if it isn’t visual. So I think that’s kind of one thing.
In terms of what’s new to me? I don’t know how new this product is, but somebody last week turned me on to Snipd. S-N-I-P-D. I’m a big podcast listener, and it’s a great example of an application that I think has woven AI really well through the user experience. So it, like, subscribes to all your podcasts. It, like, auto summarizes, it allows—it surfaces up some of the key insights in readable form or in a shortened version. It allows you to take, kind of, notes.
Sonya Huang: We need this. We’ve been looking for this.
Sahir Azam: It’s super cool. Yeah, I just found out about it last week, and I am loving learning how to use it well.
Pat Grady: Love it. Who do you admire most in the world of AI?
Sahir Azam: That’s a tough one. I mean, certainly I think some of the just researchers that see the future and probably have a sense of where things are really going. Every time I listen to them on this podcast or read some of their writing, I feel, like, really excited about the future. And, you know, the typical names there. So I think that cohort of people is always inspirational to me. You know, I think it’s fun to listen to the large company CEOs kind of mudsling a little bit about whether their applications are just systems of record, or who’s going to win the agent race and all of that. So I think it’s interesting to see the battle of titans happening in terms of who are going to really be the incumbents that can survive and thrive versus the ones that may not make the transition. So without naming names, I’d say those are the two most interesting cohorts of leaders that I tend to listen to.
Pat Grady: Fair enough.
Sonya Huang: Okay, agree or disagree? Every developer will become an AI engineer.
Sahir Azam: Agree. You know, I think that traditional machine learning was typically specialized in a centralized ML or data science team, and applied to probably a subset of the use cases that could potentially add value to. What we’re seeing though with generative AI being integrated into applications, whether it’s greenfield or to an existing application, is it’s the average full stack or application developers that are the ones that are responsible for that. So really democratizing that capability across the organization is something we’re trying to do. And so if I had to give a simple answer, I would agree with it.
Sonya Huang: Wonderful. Sahir, thank you so much for joining us today. I think you have…
Sahir Azam: This is super fun.
Sonya Huang: You have really profound theses on how AI is going to change not just databases, but software and technology and the way we interact with technology as a whole, and how that ripples over to the database market. So thank you for taking the time to share your thoughts.
Sahir Azam: Absolutely. Thank you. And happy to be here. And, you know, we’ll see if any of these thoughts actually hold water. Things are moving so fast.
Pat Grady: Awesome. Thank you.
Article originally posted on mongodb google news. Visit mongodb google news
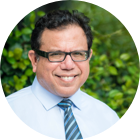
MMS • RSS
Posted on mongodb google news. Visit mongodb google news
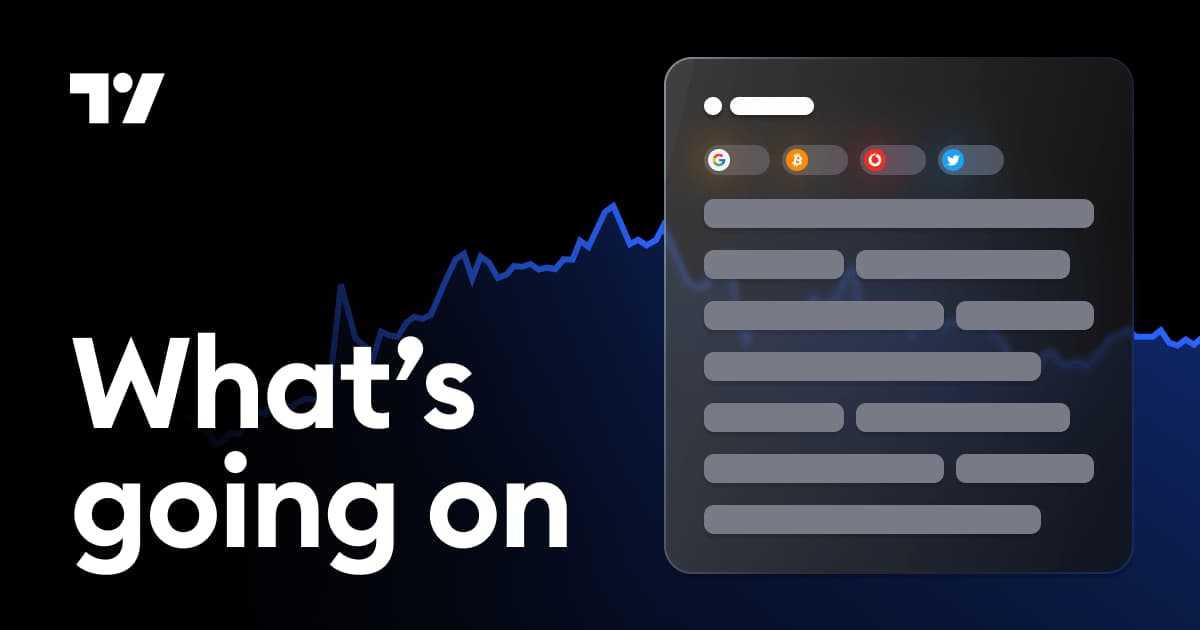
Datadog, Inc., a leading monitoring and security platform for cloud applications, has released its financial results for the fourth quarter and fiscal year ending December 31, 2024. The company reported significant growth in revenue and customer base, alongside strategic product launches and corporate developments.
Financial Highlights
For the fourth quarter of 2024, Datadog reported a revenue of $738 million, marking a 25% year-over-year increase. The GAAP operating income stood at $9 million with a GAAP operating margin of 1%. On a non-GAAP basis, the operating income was $179 million, translating to a 24% operating margin. The GAAP net income per diluted share was $0.13, while the non-GAAP net income per diluted share was $0.49. The company also reported an operating cash flow of $265 million and free cash flow of $241 million.
For the full fiscal year 2024, revenue reached $2.68 billion, a 26% increase from the previous year. GAAP operating income was $54 million with a 2% operating margin, and non-GAAP operating income was $674 million with a 25% operating margin. GAAP net income per diluted share was $0.52, and non-GAAP net income per diluted share was $1.82. Operating cash flow for the year was $871 million, with free cash flow amounting to $775 million.
Business and Operational Highlights
Datadog saw strong growth in its customer base, with 462 customers having an annual recurring revenue (ARR) of $1 million or more, up from 396 a year ago. The company also reported having about 3,610 customers with ARR of $100,000 or more, a 13% increase from the previous year. Key product launches included the general availability of On-Call, a new approach to Cloud SIEM, and the expansion of its Database Monitoring product to support MongoDB databases.
Strategic Initiatives and Corporate Developments
In a significant corporate development, Datadog issued $1 billion aggregate principal amount of 0% Convertible Senior Notes due 2029 in a private placement. The company also highlighted its continued investment in its Amazon Web Services (AWS) monitoring product portfolio and announced the launch of Kubernetes Active Remediation.
Management’s Perspective
Olivier Pomel, co-founder and CEO of Datadog, expressed satisfaction with the company’s performance, stating, “We are pleased with our strong execution in fiscal year 2024, with 26% year-over-year revenue growth, $871 million in operating cash flow, and $775 million in free cash flow.” He also emphasized the company’s commitment to innovation and helping customers with modern observability, cloud security, software delivery, cloud service management, and product analytics.
Future Outlook
Looking ahead to the first quarter of 2025, Datadog expects revenue between $737 million and $741 million, with non-GAAP operating income between $162 million and $166 million. For the full fiscal year 2025, the company projects revenue between $3.175 billion and $3.195 billion, and non-GAAP operating income between $655 million and $675 million. Non-GAAP net income per share is expected to be between $1.65 and $1.70, assuming approximately 369 million weighted average diluted shares outstanding.
SEC Filing: Datadog, Inc. [ DDOG ] – 8-K – Feb. 13, 2025
Article originally posted on mongodb google news. Visit mongodb google news