Month: October 2022
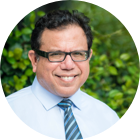
MMS • Renato Losio
Article originally posted on InfoQ. Visit InfoQ
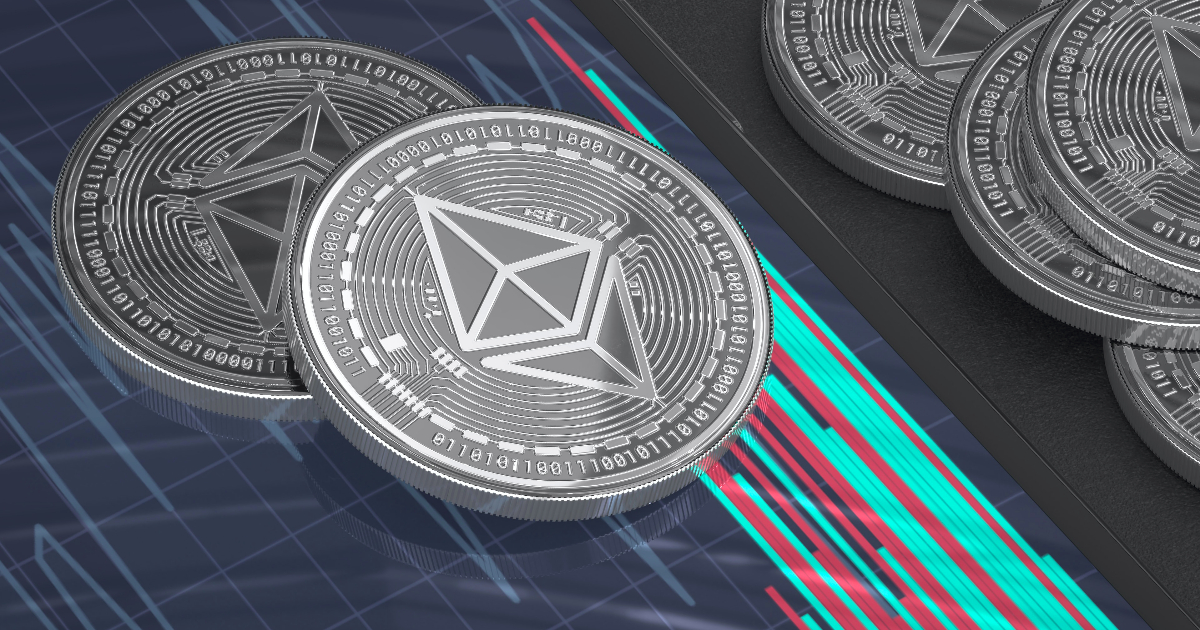
Google Cloud recently announced the private preview of Blockchain Node Engine, a managed node-hosting option for Web3 development. Ethereum will be the first blockchain supported.
Designed to help Web3 developers build and deploy on blockchain-based platforms, the new managed service monitors the nodes and restarts them during outages. Amit Zavery, GM/VP engineering at Google, and James Tromans, director of Cloud Web3 at Google, explain:
While self-managed nodes are often difficult to deploy and require constant management, Blockchain Node Engine is a fully managed node-hosting service that can minimize the need for node operations. Web3 companies who require dedicated nodes can relay transactions, deploy smart contracts, and read or write blockchain data with the reliability, performance, and security they expect from Google Cloud compute and network infrastructure.
According to the cloud provider, the main benefits for Web3 organizations will be streamlined provisioning, managed operations, and secure development, including placing nodes behind a Virtual Private Cloud firewall and integrating with Cloud Armor as a protection against DDoS attacks. Zavery and Tromans add:
Today, manually deploying a node is a time-intensive process that involves provisioning a compute instance, installing an Ethereum client (e.g. geth), and waiting for the node to sync with the network. Syncing a full node from the first block (i.e., genesis) can take several days. Google Cloud’s Blockchain Node Engine can make this process faster and easier by allowing developers to deploy a new node with a single operation.
The preview of the node-hosting service on Google Cloud triggered popular debates on Twitter and Reddit, with some users excited about the new option and others questioning if cloud providers will keep the promise of decentralization. User Lazy_Physicist highlights how the announcement can help spreading nodes among different providers:
You know how people say a concerning quantity of Ethereum nodes are run in AWS? Now you can do the same in Google Cloud. Basically Google just streamlined the provisioning of a node that you can run a validator on. Definitely a centralizing force but usually more options are better.
Earlier this year Google announced the Digital Assets Team to support customers building, transacting and deploying on blockchain-based platforms. Solana and Dapper Labs are among the Web3 companies already running on Google Cloud.
Google Cloud is not the only provider working on managed blockchain options: AWS offers Amazon Managed Blockchain, a service to join public networks or manage private networks using Hyperledger Fabric or Ethereum. Microsoft recently retired Azure Blockchain Service and Azure Blockchain Workbench. User AusIV comments:
If you’re running an application that needs scale and reliability you’re way better off with an RPC gateway than a managed node. If you’re trying to support the network by running a node, managed services are definitely not the way to do it. The network has a problem with the percentage of nodes run in a small handful of service providers.
A form is available to join the private preview of Blockchain Node Engine.
Threat-Detection Tool Falco Now Supports Multiple Event Sources, Syscall Selection, and More
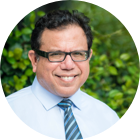
MMS • Sergio De Simone
Article originally posted on InfoQ. Visit InfoQ
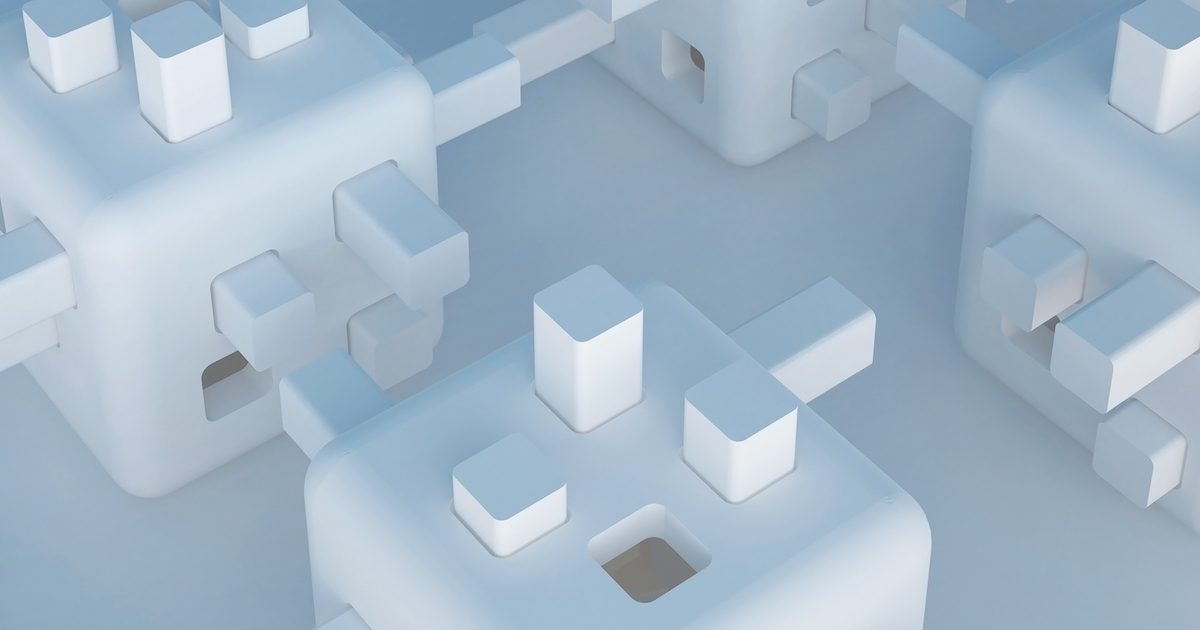
The latest release of Falco adds the ability to handle multiple simultaneous event sources within the same instance, support for selecting which syscalls to capture, a new Kernel Crawler to collect the most recent supported kernel versions, and more.
Up until version 0.33.0, the only way for Falco to consume events from multiple event sources was to deploy multiple instances of Falco, one for each event source. This was especially limiting in the face of Falco’s plugin system, which allowed to go beyond syscall tracing by adding new kind of event sources starting with Falco 0.32.
This is a huge improvement and also brings back support for running syscall and k8s audit logs in the same Falco instance, for all the folks who were interested in doing so.
This new feature introduces a user-facing change in that each Falco instance enables syscall event sources by default, which means you will need to explicitly disable syscalls if you want a plugin-only deployment.
Falco 0.33 also introduces new libsinsp APIs that make it possible to individually select which kernel syscalls and tracepoint events should be collected. This is a step forward in comparison to the previous “simple consumer mode”, which was able to discard events not useful for runtime security purposes. Selecting individual syscalls and events should improve Falco performance and reduces the amount of dropped events.
Related to this, the new release of Falco further attempts to mitigate the issue of dropped events by giving control over the size of the syscall kernel ring-buffer, which is shared memory where drivers buffer events for Falco to consume them at a later point. By tuning the ring-buffer size, you can control how frequently Falco will drop events.
As mentioned, the Kernel Crawler is a new tool that automatically searches for new kernel versions supported for a number of Linux distros. It should make it easier to adopt Falco by simplifying the task of installing kernel modules and eBPF probes for a given kernel version. The Kernel Crawler is used to populate and maintain a database with the build matrix which lists all kernel versions and distros supported by Falco.
The latest Falco release brings many additional new features and improvements, including new drivers for minikube, improved rate limiting for alerts, and new supported syscalls and security rules. Do not miss the official announcement or the changelog for the full detail.
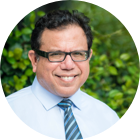
MMS • RSS
Posted on nosqlgooglealerts. Visit nosqlgooglealerts
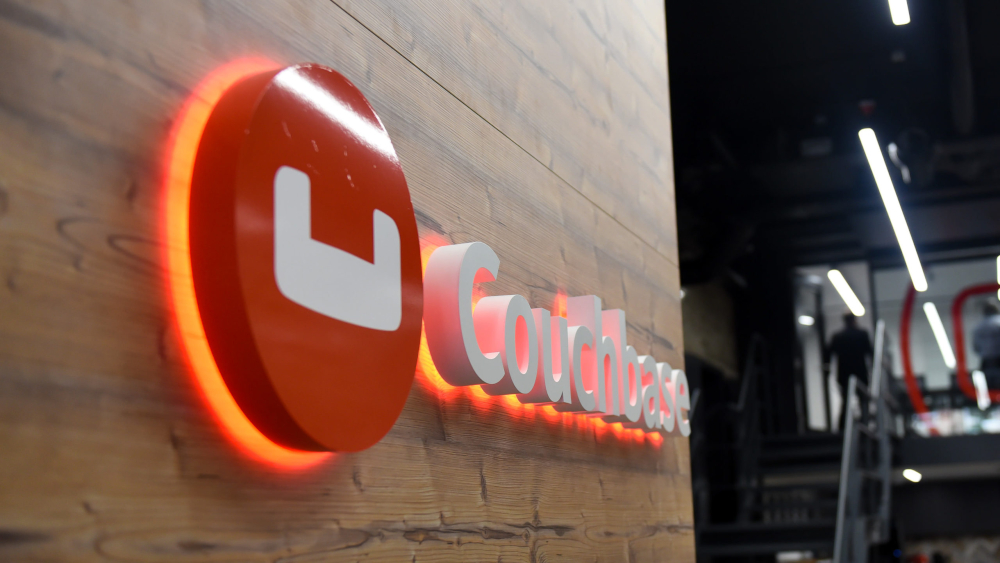
Couchbase Inc. today introduced a new release of Capella, its managed database service, that features an expanded set of data storage and security capabilities.
Nasdaq-listed Couchbase provides a popular NoSQL database of the same name. Capella is a managed, cloud-based version of the company’s database that automates maintenance tasks such as infrastructure management.
Capella is designed to store both structured and unstructured information. Couchbase says the database can complete some queries with latency as low as a few milliseconds. Though Capella is a NoSQL database, it provides the ability to write queries in the SQL language, which makes the database relatively easy to use because many developers are already familiar with the language.
Databases use a software component known as a storage engine to retrieve information for applications and save newly added data. By default, Cappella uses a storage engine known as Couchstore. The new release of Capella that Couchbase debuted today includes a second storage engine, Magma, that can provide better performance for certain enterprise applications.
Databases usually keep information on storage drives when it’s not actively used and move the information to memory when processing is carried out. Capella, in contrast, can skip the process of moving records from storage drives to memory by storing the records in memory from the outset. Skipping this step reduces the number of computations required to analyze data, which improves performance.
Keeping information in memory isn’t always possible. Some enterprise applications have large, complex datasets that cannot be fully stored on a server’s onboard memory chips. The new Magma storage engine that Couchbase has added to Capella is designed to improve the performance of such applications.
When a large dataset can’t be stored in memory, it must be saved to flash or disk drives. The Magma storage engine includes several optimizations that speed up the task of processing data stored in those drives. According to Couchbase, Magma enables Capella to perform some processing tasks up to four times faster than other technologies while using one-10th the memory.
A second set of improvements introduced for Capella today will help developers more easily build applications on the database service. According to Couchbase, the service has been updated with a streamlined interface that draws on design concepts implemented in popular development tools such as GitHub. The upgraded interface also makes it easier to access tutorials and other technical resources.
“As customers continue to invest in digital transformation, developers who are building modern applications need technologies that make them more productive,” said Scott Anderson, senior vice president of product management at Couchbase.
The third major focus of today’s update is helping companies more easily meet cybersecurity requirements.
Couchbase has equipped Capella with administrative controls that meet the requirements set forth in the healthcare sector’s HIPAA data security regulation. According to the company, the controls will make it easier for organizations such as hospitals to store records in Capella. Couchbase has also added a new audit capability that organizations can use to track how important business data is accessed.
Photo: Couchbase
Show your support for our mission by joining our Cube Club and Cube Event Community of experts. Join the community that includes Amazon Web Services and Amazon.com CEO Andy Jassy, Dell Technologies founder and CEO Michael Dell, Intel CEO Pat Gelsinger and many more luminaries and experts.
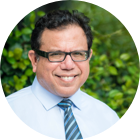
MMS • Susanne Kaiser
Article originally posted on InfoQ. Visit InfoQ
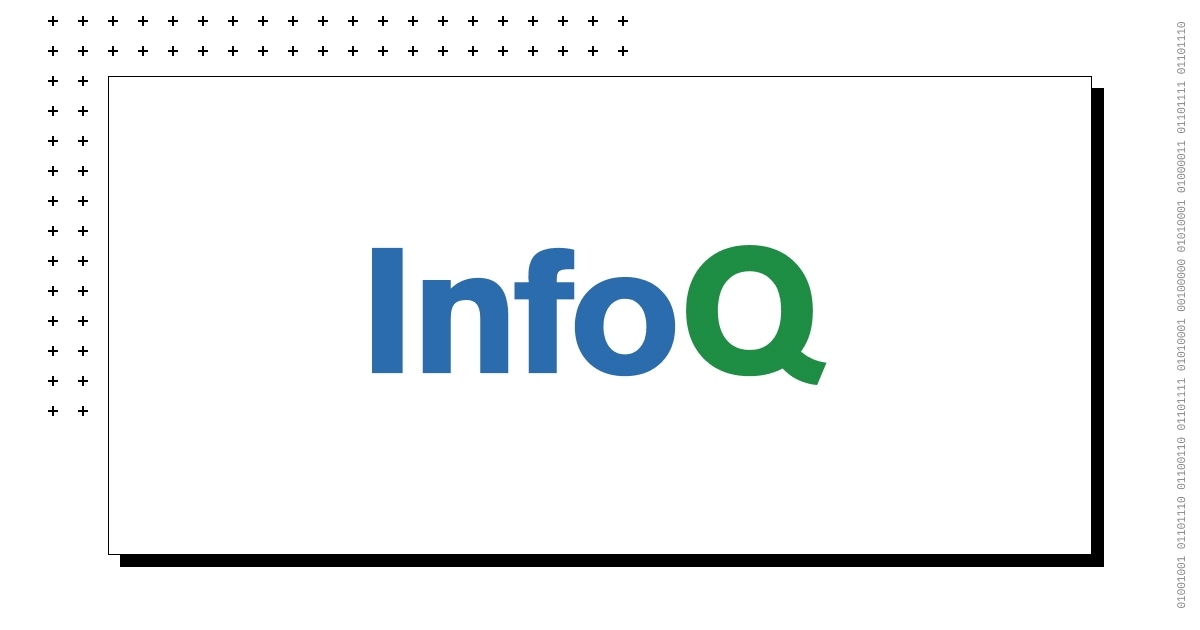
Subscribe on:
Welcome and Introduction [00:04]
Wes Reisz: Domain-Driven Design is an approach to modeling software focused on a domain, or a logical area that defines a problem that you’re trying to solve. Originally popularized in the early 2000s by Eric Evans, DDD’s focus on making software a reflection of the domain it works with has found a resurgence in the isolation and decoupled nature, or at least the promise of it, with microservices. Wardley Maps is a strategic way of visualizing a value chain or business capabilities in the grid characterized by a spectrum towards commodity on one axis, and most commonly something like visibility on another axis. It helps you understand and makes important strategic decisions such as like a build versus buy decision. Finally, team topologies is an approach to organizing business and technology teams for fast flow. It’s a practical kind of step-by-step adaptive model for organizational team design interaction.
When combined, Domain-Driven Design, Wardley Maps, and team topologies provides a powerful suite of tools to help you think about modern software architecture. My name is Wes Reisz, I’m a principal technologist with Thoughtworks and co-host of The InfoQ podcast. Additionally, I chair the QCon San Francisco Software Conference happening in October, and November this year. Today on the InfoQ podcast, we’re talking to Susanne Kaiser. Susanne is an independent tech consultant, software architect, and an ex-startup CTO who has recently been connecting the dots between Domain-Driven Design, Wardley Maps, and team topologies. She recently gave a fantastic talk at QCon London diving into the intersection of the three. So on today’s show, we like to continue the conversation talking about Domain-Driven Design, Wardley Maps, and team topologies. We’ll first tap the recap three, and then we’ll dive into some of the success stories and some of the understanding that Susanne has when she’s talking to the folks about the consulting work. As always, thank you for joining us on your jogs, walks and commute. Susanne, thank you for joining us on the podcast.
Susanne Kaiser: Thank you so much for having me, I’m looking forward to it.
Wes Reisz: Okay. We talked about Domain-Driven Design, Wardley Maps, and team topology in the introduction, at least I did like a high level overview. Anything you want to add to the overview that I kind of gave?
Susanne Kaiser: No, it was a great overview. So I like to hear, to listen to stories that other bring into the table because whenever I make the introduction that it’s like, oh yeah, it’s so either going too far into details. And then the podcast host, they then tend to bring very great summaries, so nothing to add. Great introduction.
What made you bring together Team Topologies, Domain-Driven Design, and Wardley Map? [02:30]
Wes Reisz: Great. There’s so many things that are in software. What made you decide to bring these three things together to kind of a story?
Susanne Kaiser: Yes. So for me, the combination of Wardley Mapping, Domain-Driven Design and team topologies evolved naturally over time, but it was at its core driven by system thinking. So, Dr. Russell Ackoff, one of the pioneers of the system thinking movement, he stated that a system is more than the sum of its parts. It’s a product of their interaction. So the way parts fit together, that determines the performance of system, not on how they perform taken separately. So, and when we are building systems in general, we are faced with the challenges of building the right thing and building the thing right. Right? And building the right thing addresses effectiveness, and addresses questions such as how aligned is our solution to the users and business needs. Are we creating value for our customers? Have we understood the problem and do we share a common understanding and building the thing right?
Focuses on efficiencies, for example, efficiency of engineering practices, and it’s not only crucial to generate value, but also being able to deliver that value. How fast can we deliver changes, and how fast and easy can we make a change effective and adapt to new circumstances. So, the one doesn’t go without the other, but as Dr. Russell Ackoff pointed out doing the wrong thing right is not nearly as good as doing the right thing wrong. So, by considering the whole, and having effectiveness and efficiency in mind to build the right thing right, that we need a kind of like holistic perspective to build adaptive systems. One approach out of many is combining these three perspectives of business strategy with Wardley Mapping, software architecture, and design was Domain-Driven Design, and team organization was team topologies. So, in order to build and design and evolve adaptive socio-technical systems that are optimized for fast flow of change.
Wes Reisz: Yes, absolutely. That really resonates with me, building the right thing and then building the thing right. Those two phrases really resonate with me, a trade-off and understanding what you’re doing and how you’re thinking about these problems. So, where do you start? You have these three things and you start with domain-driven design? Where do you start when you kind of apply these three techniques?
Susanne Kaiser: So, it depends on your context. You can start each of the perspectives. What I like to start with is first analyzing the team situation, regards to their team cognitive load and their delivery bottlenecks that they’re currently facing. So, what of kind of problems do they have right now? Are they are dealing with high team cognitive load because they have to deal with a big ball of mud when you deal with a legacy system which evolved over the time? Are there organized as functional silo teams where handover is involved? Are these large teams or do the teams need to communicate and coordinate with each other when they want to implement and deliver changes? So, this are kind of questions that I like to address first, like analyzing the current situation of your teams. And then the next step is-
How do you talk to teams about cognitive load? [05:32]
Wes Reisz: Let me ask a question before you go there. Let’s talk about cognitive load for a second. How do you get people to understand the cognitive load that they’re under? A lot of teams have been operating in a certain way for so long, they don’t even realize that their cognitive load is so high that they don’t know of any other way. They don’t know how to adapt to that. How do you have that conversation and get people to understand that the cognitive load that they’re seeing is actually a detriment to flow?
Susanne Kaiser: So, there is different aspect that I like to bring into the conversation, for example. So how much time does it need for them to understand a piece of code? How long does it take to onboard new team members? How long does it take to make a change effective and to implement changes and kind of also comes to software quality in teams and in terms of like testing as well. Are there side effects involved that they could not be easily anticipated, and then also bring it back to a Wardley Map itself. So, what kind of components they are responsible for, and if we have our Wardley Map with the value chain matched to the Y axis, and if you use this, your user needs and components that fulfill the user needs directly or facilitating other components in the value chain. And then the evolutions axis going from left to right from Genesis, custom build, product and rental, and commodity.
And the more you are on the spectrum of a left spectrum of your Wardley Map, then you are dealing with high uncertainty, then also unclear path to action. And the components that are located on the right spectrum of your Wardley Map, there you are dealing with mature stable components where you have a more clear path to action. And if your teams are responsible for components that are located on the left spectrum of your Wardley Map, then there’s a potential high cognitive load involved because you need to experiment more, explore more, discover more and applying then merchant and novel practices instead of best and a good practices on the right part.
Wes Reisz: Yes, that really resonated for me in particular, being able to visualize the value chain onto a Wardley Map, and then be able to say things on the left, things that are more towards Genesis require more cognitive load to keep in your mind. Things that are more towards that commodity side, right side, are definitely less. That really resonated to me when you said that. So, I interrupted you, you said step two. Okay. So, that’s step one, applying some things out. What about step two?
Susanne Kaiser: It doesn’t need to necessarily be step two, but one of the steps could be creating a Wardley Map of your current situation and to look at what is your current landscape you are operating in, what are your users, what are the users’ needs? What are the components of fulfill this user needs? And then also to identify the streams of changes in order to optimize for fast flow change, that requires to know where the most important changes in your system are occurring. The streams of changes, and there could be different types of stream of changes. And with the Wardley Map, this visualized activity oriented streams of changes reflected or represented by the user needs. So, if we look at the user needs, these are then the potential stream of changes that we need to focus on when we want to optimize for fast flow of change.
So, that is then first identifying then the stream force changes, the user needs, could be then the next step and using this Wardley Map as a foundation for future discussions, how to evolve then our system. And then we can also go from there to address the problem domain next. And that’s where we are then landing in Domain-Driven Design, and the users and the user needs of a Wardley Map, they are usually representing the anchor of your map, but also constitute the problem domain in regards to Domain-Driven Design. You can then analyze your problem domain and try to distill your problem domain into smaller parts, the sub domains. Different sub domains have different value to the business, so some are more important to the business than others. So, we have different types of sub domains, such as core, supporting and generic. And we can try to identify what are the sub domains that are core, which provides competitive advantage, which tend to change often, which tend to be quite complex and where we should focus on building these parts of our system in-house, because that’s the one that we would like to differentiate ourselves.
So, that requires the most strategic investment so this gives us combined on a Wardley Map, the co-domain related aspects then are then located in Genesis or custom build and needs to be built in-house. Then, supporting a generic that’s then where go to the right spectrum of the Wardley Map, either buying off the shelf product or using open source software for supporting, for example, and then generic, that is something where we can also use go some product and rental, or then commodity and utility where we have can then outsource to, for example, cloud housing services.
Can you give me some examples of bringing these together? [10:17]
Wes Reisz: You’ve used some examples as we’ve talked, let’s try to put this into a journey, like a little example that kind of walks through it. Then as we go through it, I want to ask some questions, things that are about team size, particularly smaller team sizes. When you have a lot of people and large engineering teams, like QCon London had a talk on my track with Twitter that just in the GraphQL API side, they have an enabling team that has 25 engineers just to support the GraphQL side. So, they have a huge team just in one particular area of their API surface, but in smaller organizations, you may not have that kind of depth of talent to be able to pull from. So, as we kind of walk through this, I want to ask kind of drawing on some of that experience you had as a CTO startup, how do you deal with different sizes when you don’t necessarily have huge teams to be able to handle different areas within the platform? So, you used an example I think in your talk, you want to use that as an example? Let’s try to apply this to something. Does an example come to mind?
Susanne Kaiser: In my talk, I was addressing a fictitious example of an online school for uni students, which was at that state, a monolithic big ball of mud, which was run and supported by functional silo teams and running on top of on-premises infrastructure components.
Wes Reisz: Okay. So do you just take this big ball of mud and put it right in the middle of a Wardley Map? How do you start to tease apart those big ball of mud?
Susanne Kaise: I start to map the current state of the online school. This monolithic big ball of mud is, for example, so I start with what users needs first, right? So, the users could be the teachers and the uni students with their user needs of creating course content, or evaluating student progress. Or these students would like to study courses and requesting or receiving help, and also receiving evaluation feedback.
Wes Reisz: So a full slice, but basically a full slice of what the user’s trying to accomplish.
Susanne Kaiser: Exactly, what needs do they have and what is necessary to fulfill this user need? And then I can start with what is necessary to fulfill the user need directly. So, that is at the top of the value chain of the Y axis of our Wardley Map. And when I reflect, when I derive the value chain of the current state, where we have a monolith right now, I start with one component, even knowing that it’s too large, because that’s something that we would like to decompose later on. But at first I would like to bring this big ball of mud as one component, and then bringing in, then the second aspect of Domain-Driven Design, where we split it, decompose it into modular components where we then solution space of strategic design and where we decompose it into modular components within the bounded context.
When it comes to Wardley Maps, how do you think about sizing of the things on the value chain? [12:50]
Wes Reisz: That was my first question, is about sizing. Like, how do you pick the right size? I mean, when you put this big bottle of mud in there, it’s going to be too big, right? So you put that big ball of mud in there, and then you move into kind of the domains and start to break out the bounded context between that big ball of mud. So, that way you can focus on each individual pieces within it?
Susanne Kaiser: The Wardley Map, this is kind of like a continuous improvement, right? So, you have a continuous conversation about it. So is the Wardley Map that they once created will change over the time. So, you can also say, okay, we know that this is a big ball of modern, too large component. You can define it as your scope. Usually when you create a Wardley Map, you also define the scope of your Wardley Map. What is included, what is excluded for example. And you can also say at this point, we are putting in the monolith as one component, we are going to decompose it in a different Wardley Map, or we just replace it. So, if there’s one heuristic is if one component is too large to be handled by a small cross-functional team, that is an indicator that your component is too large. So, if we have this monolithic online school component as one component, it’s an indicator that it’s too large.
So we need to address it then coming to after we know what is our problem domain, what kind of problems we would like to solve, the user needs, we need to then identify where we do high level design decisions and moving to the solution space of strategic design and decompose our big ball of mud into modular components. There we can blend in other techniques from Domain-Driven Design, such as event storming, domain storytelling, user story, mapping, example mapping, and so on to have a conversation about what is behavior that shall sit together? Where we have a boundary around a domain model, the domain model reflecting business rules of a specific area of your system, and where bounded context forms a boundary and could also be then later on be suitable team boundaries. When we come to team topologies, where we blend in the next perspective, making team decisions then.
Wes Reisz: How does it change when maybe you’re just focused on an API, building an API and you don’t have control of say the front end or something along those lines? Does it change? Do you think about it any different when there’s this team abstraction that handles the UI, and then you’re kind of only working at the API surface and below. How do you think about that kind of problem?
Susanne Kaiser: In an ideal situation, there’s end-to-end responsibility for the streamline teams, including the user interface, including the front end, because what we would like to avoid is handover. And when we have front-end teams and back-end teams, it’s definitely a handover involved when we want to make a change effective, that is then distributed to the client that the client can use through the user interface. So, team topologies aims to establish cross-functional teams and that means also that the streamline teams, they need to have at least that much of skills for creating user interface and also having user experience, UX focus as well, involved. That could get support by enabling teams helping them.
For example, they could provide a style guide that can help the streamline teams to not to reinvent the UI wheel every time they introduce a new dialogue and there it is interface. So, there are some standards that enabling teams can provide for example, and help them also to acquire missing capabilities in that regard. But they only there not permanently be available, but instead every other team type from team topologies tried to make the streamline team self-sufficient so that they can focus on their steady flow of future deliveries, flow of changes, autonomously, and then request some help in specific circumstances.
Wes Reisz: Team topologies talks about four different types of team structures that are created. There is a streamline team, which is the main way that work is done. And as you said, it’s cross-functional, it has all these different pieces to it. There’s a platform team, there’s enabling team, and then there’s a complicated sub-process team. So how do, say, like a platform to you, like particularly in a case of a smaller company, I keep coming back to the smaller size because when you’ve got lots of people, you’ve got lots of different ways that you can create this. But in a smaller organization, how do you leverage the streamlined teams with platform teams, for example, to be able to get started? Like, you mentioned some of the best practices and things that you can start with. Can you talk a little bit about kind of that interaction and how some of these tools help you get started with this?
Susanne Kaiser: So first of all, if you have a really small organization, you can still apply it, but you don’t have to have a dedicated platform team from the very first beginning because maybe you only have two streamline teams, right? But you can establish a temporary task force that can provide a sentence viable platform. That’s how Matthew Skelton and Manuel Pais describe in their team topology book, where you provide first this kind of platform that is big enough to fulfill the consumer’s need and not become bigger than needed. And it could start with documentation like how to provision your infrastructure in cloud ecosystem or how to use the serverless framework, or how to use this and that. And with documentation, it can then also describe also standards and best practices. Later on, it can evolve into a digital platform with self-service services, and APIs, and tools that the streamline team can then easily consume. But it does not necessarily have to be full-blown digital platform from the very beginning, but just as big enough as it’s necessary to fulfill the needs of the consumers.
How do you walk the line between standards and standardization? [18:16]
Wes Reisz: As you mentioned there, when you start off in that journey with platform teams, how do you walk the line between standard and standardization? Like you want to have high standards, but as I think you said before, you don’t want a standardization to become like a bottleneck. How do you walk the line between those two things?
Susanne Kaiser: At the moment when you make something mandatory to use, right, that is then where it becomes potentially a bottleneck. So, it’s always like addresses. Does we introduce bottlenecks in our journey because we would like to enable the streamline teams that they are able to focus on fast flow of changes, that they are able to produce a steady flow of feature deliveries. So, if there is a moment where we are blocking them and for example, where we say, no, you are only allowed to use this technologies or that technologies. This is an indication that we might then introduce the next bottleneck that we try to avoid from the very first beginning. For example, one possibility is also when we look at establishing cloud centers of excellence, where we empower teams to innovate on cloud host of infrastructure, we don’t want to block innovation by saying you’re only allowed to use this technologies.
Instead, we would like to spark that these streamline teams can also learn, that we don’t want to hold them back. And I guess the moment where we are not enabling them and supporting them, instead of telling them what to use, it’s a challenge that we address. On the other hand, it’s also like we already had this conversation when we had this DevOps movement, right? Where we have stability on the one side where the operation teams were focusing on and want to keep your production system stable and want to have as minimum, least changes to the system. But the development teams back then wanted to deliver a lot of changes. And so there was a kind of contradiction in between them and also kind of like different forces involved. I guess we have to look at what enables the fast flow of change. I guess that’s the most important focus that they both need to have in mind.
How do you get started with a process like this? [20:12]
Wes Reisz: I want to ask a question that often comes up when I’ve had conversations about this. And it’s about like getting started. When a company, like you talked about, you can start off by talking to the team, you can find out what their friction points are. You talked about them maybe using Wardley Maps and then Domain-Driven Design and some of the concepts and things, the techniques within Domain-Driven Design to be able to dive deeper, like you’ve been storming. So, it’s kind of like a way that you could use these concepts together to be able to be get started, but let’s dive into a specific team on how you get started. If there’s a company that sees this and they know that they can rearchitect this big ball of mud using these strategies that you’ve talked about, and use the Inverse Conway Maneuver to be able to enable fast flow of change and all these wonderful things that you talked about.
But how do you get started? Do you just like come in Monday morning and say, “All right, we’re breaking up into three teams. We’ve got a platform team, we’ve got two enabling teams and go.” I mean, do you start with one team, one uber team knowing full well it’s the team that’s going to live, but not the people on the team? How do you talk to people about day one, and day two, and then day three, when it comes to these strategies?
Susanne Kaiser: First of all, make it transparent, make the change transparent and communicated along the organization. Because I guess the most fear that people have is about change, that they get laid off. So whenever there’s a reorganization in place, because if we are transforming to a team of topologies team types and their interaction modes, there will be reteaming involved. And first of all, I like to make it transparent through the entire organization, what are the team types? What is the interaction mode? When does it make sense that teams are collaborating? When does it make sense to provide excessive service? That is very essential for a transformation? Also there, I would like also to bring in Heidi Helfand’s brilliant book about dynamic reteaming.
She also brings in that you can start with forming one team first on site, for example, by isolating one team, for example. That you can start, for example, with a platform team first where you form first your platform team on a site from members of the back end and the infrastructure team, and then put them on site to discover and assess infrastructure options. For example, if you’d plan to, instead of running the system on premises infrastructure, you would like to migrate to the cloud and have this isolated platform team. As a first team working on the site, they don’t have to follow the processes. They are there to discover and explore new cloud strategies for example, and cloud options that are available and they’re suitable for their first bounded context that they would like to extract.
It can then form next the first streamline teams, collaborating closely together with the platform teams that has been built from first and assessing potential cloud options for the bounded context that the streamline teams is responsible for. And who will be member of which teams is also another question, right? So, Heidi Helfand described a different levels, who decides who will be member of what team. It could be from the top down, from management. It could also be self-selecting process as well, where you let your members of your current teams decide in what teams they would like to become member of. And so there are different levels involve, and also that you can also calibrate when you form a team, when you reteam, you need to also to calibrate your team. So, you can calibrate on different aspects like how you would like to work together, what you like to do, peer programming or more programming.
And what is your mission of your team that you introduce new team members, that they can onboard very easily? And so they have different team calibration sessions that help you to bring the journey forward towards team topologies, team types. But also for example, if you focus on account migration strategy as well, so there are different aspects there where you can gradually transform your existing team incrementally and transform them into team topologies, team type. Also, with the highest level of self-selection then, letting the team members select themselves. That could also be a suggestion, or like that the team members say, “I would like to be into that team.” Then the management can decide in getting this input from the teams and they decide, so there are different levels applicable for forming these teams.
How do you leverage contractors delivering components for the stream-aligned teams? [24:31]
Wes Reisz: I have one more question in this kind of space. What about a company that uses contractors, brings in contractors to deliver units into the value chain? How do you integrate that type of work when you don’t have a streamlined team that has complete code ownership of everything that they’re actually delivering? How do you deal with it?
Susanne Kaiser: If you bring in contractors to the streamline team, you mean?
Wes Reisz: Yes, so like you’ve got a streamline team that owns an area of the business that’s doing some kind of work, but they contract to bring different pieces in. A mobile app that may expose some things, or a capability that might be on a mobile app that maybe they don’t have the like internal experience to develop themselves. How do you deal with that?
Susanne Kaiser: Yes, so it depends what part of the system they are integrated in. So, one thing is that either through well defined APIs of what they are going to provide, so is it excessive server that they provide or not, that they can easily consume or not. But also if they are just temporary support, for example, I would suggest to have them involved where you share the knowledge from the very beginning, having them being part of your peer programming sessions or more programming sessions where you don’t have a team that is building expertise and then leave later, and then the knowledge is gone. So that you incorporate them in your processes, having them involved in your knowledge sharing sessions as well, so that they are becoming part of your team.
And they might rotate later on, also Team Topologies says that you should aim for long life stable teams, but this does not mean that they need to be static. So, team members can switch over the time, either freelancers or contractors from outside, but also Heidi Helfand also recommends to enable or to provide that team members can switch teams because that is one of the opportunities to retain your talents in-house. Right? So, for personal growth you sometimes would like to switch teams and if they can’t grow within your organization, they will find growth opportunities outside of your organization.
Wes Reisz: It’s the team structure that’s long live, not the people on the team. Right?
Susanne Kaiser: Exactly. Yeah.
Wes Reisz: We talked about cognitive load in context of Wardley Maps. We talked about platform and streamlined teams. We talked about standard and standardization. We talked about Domain-Driven Design and then diving deeper into some of the components once you’ve identified some areas that you want to break out. What’d we miss?
Susanne Kaiser: Sometimes I’m asked, so what kind of benefits brings Wardley Map? And it’s also that I like to highlight that first of all, it helps you to visualize potential instability in an associated risk. So for example, if you have a value chain where you have volatile components, for example, bounded context of your core domain are volatile because they’re changing a lot and they have embodied quite high level of complexities because it’s the one that provides competitive advantage. And so if you build these volatile components on top of mature and stable components, that is reflecting a stable system, but if you switch it around, if you have stable components that build up on volatile components, then it is a potential candidate for instabilities and associated risk because you have a stable component, which is expected to be stable and it’s built up on volatile components and all these introduces new changes. And you have to keep the stable component up to date, or you have to keep the stable component stable. That shifts your focus on handling the source of risks.
What are some the patterns you’ve seen when parts of the system are unstable? [27:58]
Wes Reisz: Let’s talk about that for a second. So, when I hear you say that, what are the patterns? Is that a candidate for a complicated sub process team, then to be able to encapsulate that instability and be able to elevate the constraint? Are there patterns to address that you’ve seen?
Susanne Kaiser: First of all, it creates awareness. Maybe it’s on purpose. Maybe you would like to discover a new technologies and that new technology is still in Genesis or custom build. First of all, it makes you aware that you are building stable components on top of volatile components and that could be a potential problem. And then the other thing that I would like to bring in are the efficiency gaps. Like for example, if you are using internally a component in Genesis or custom build, but there is a component in the market that is more evolved in reciting, for example, in commodity and utility. This gives you an indication, a hint that you could be less efficient in your organization, because you’re building on less efficient components that are then reciting on the left part of your Wardley Map, and this is an indication for you.
Wes Reisz: I want to dive into that just a little bit more. Sometimes a component might be very unstable, but it might be more towards commodity. Like you might have a bunch of network partitions or something on top of something, so I guess I’m curious when you talk about from Genesis to commodity, it’s not just Genesis to commodity. It’s like a whole spectrum of things to consider on that left to right? Right. So, how do you talk to people about understanding that yeah, this might be a mature service, but because you have all these network partitions that are there, it actually is further left on the Wardley Map. How do you talk to people about that?
Susanne Kaiser: It’s more about the characteristics and general properties of the components of your Wardley Map. So, it depends on the market perception, and the user perception, and so on. So if you have cloud services, you expect it to be stable and if there is a failure occurring, you are extremely surprised that this failure happens. But if you have brand new product on the market, you expect failures or you can deal with it easier than with a very stable component. Like for example, power on your coming out of the outlet. If there’s no power available, you will definitely be surprised. But if you have a brand new product supporting or something like that, you are kind of expecting that there might be some phase, it doesn’t have to be.
So, there are different characteristics and different properties. Some of Wardley has created this evolution cheat sheet to determine the stage of evolution pack component. So for example, designing for failure as a component, doesn’t necessarily need to be a physical component. It could be activities, it could be data, it could be knowledge, so it could be different activities. Designing for failure is something that is more an activity component. Maybe could be a component of your Wardley Map, of your value chain. If you can apply best practices then is something that goes more on the right spectrum.
Wes Reisz: I love that because you’re describing, if you’re building a stable component on top of something that’s instable, whatever characteristic that you want to describe it, it lets you make very strategic decisions on whether you can elevate that component, whether you can wrap that component into something that might give it the option for failure. It lets you just kind of isolate that and make very strategic decisions on it. So, I really like when you brought up the complexity of dealing with writing something that’s very stable on top of it. It makes a lot of sense to me.
Susanne Kaiser: And that also brings us to the context map of Domain-Driven Design, right? Where we have context map patterns describing the dependencies between bounded context visualizing or making aware the change coupling between bounded contexts. Right? And if you have a bounded context that is more like a supporting sub domain integrating with a quarter domain related bounded context, and there is in context map pattern between those, maybe a partnership or something like that gives you also bringing in context maps with the distribution of your bounded context on the evolution stages gives you an indication whether you have introduced tight change coupling between those systems.
Wes Reisz: Very nice. So Susanne, we’re about at time. So what’s next for you? What’s coming up in the next part of the summer for you?
Susanne Kaiser: Well, yes, finishing my book right now. It’s now in a review process and I need to wrap it up and then also see if you can still publish it this year. Let’s cross fingers. And then also, yeah, looking forward to new opportunities. Yeah. Training and something like that I’m doing also the second half.
Wes Reisz: As always Susan, I thank you so much for all that you do for InfoQ, for QCon and for the larger community as a whole when it comes to microservices, when it comes to thinking about microservices and architecture.
Susanne Kaiser: Thank you so much for having me. It was a pleasure.
.
From this page you also have access to our recorded show notes. They all have clickable links that will take you directly to that part of the audio.
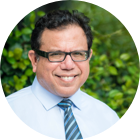
MMS • Gilad David Maayan
Article originally posted on InfoQ. Visit InfoQ
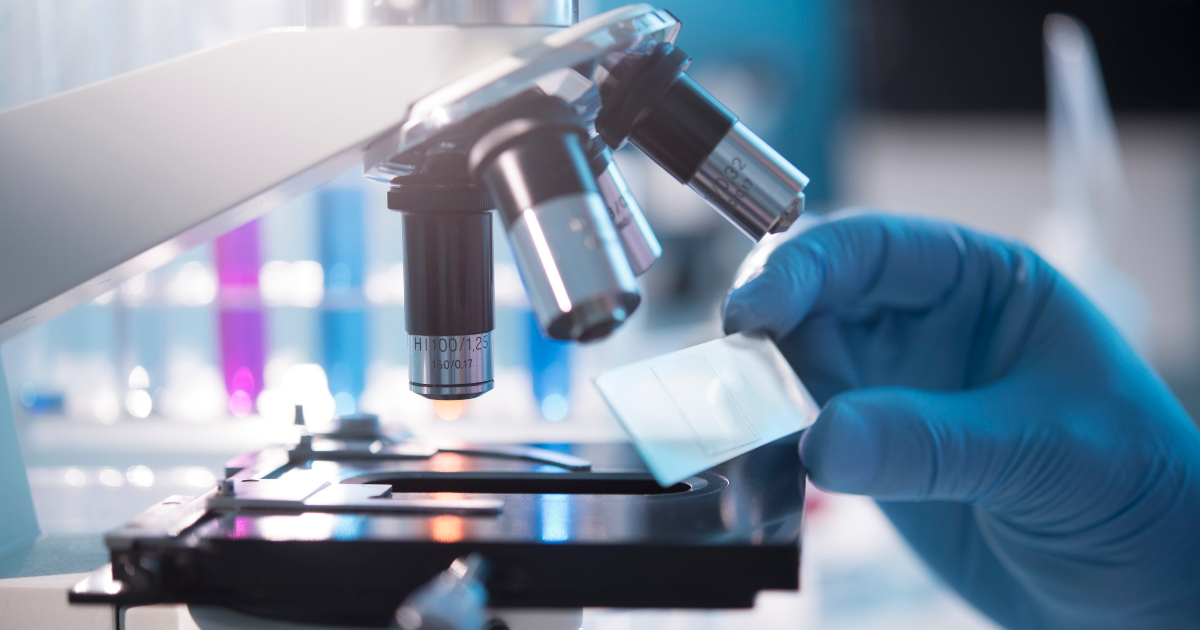
Key Takeaways
- You cannot do GitOps without observability
- Git is the single source of truth for the system’s intended state, while observability provides a single source of truth for the system’s actual state
- Internal observability allows the GitOps controller to identify configuration drift; external observability allows operations staff and other systems to identify changes in the cluster
- Cloud native observability is a new skill and task you must add to your DevOps team
GitOps is a new software development paradigm that promises to streamline and fully automate the software deployment process. Instead of relying on IT staff or unwieldy scripts to provision environments, GitOps defines all environments as code, and deploys the environment together with all applications in a consistent and predictable manner. Everything is managed in source control, using tools that are familiar to most developers.
GitOps promises, and delivers, massive productivity benefits for developers. But just like any new technical approach, the challenge is in the details. One of the complex aspects of GitOps is ensuring sufficient visibility of live environments, to ensure that it can be synchronized with a desired configuration. In this article, I’ll explain why observability is so critical to GitOps, and how ArgoCD, a popular GitOps platform, addresses the observability challenge.
What Are Continuous Delivery and Continuous Deployment?
Continuous delivery prepares a software product for production deployment, making it possible to deploy changes at the push of a button. In traditional setups, this was typically done by merging a change to the master branch (this is known as push deployment).
In newer GitOps environments, it is done by committing a change to a central environment repository, triggering a deployment (this is known as pull deployment).
Continuous delivery creates artifacts that can be deployed to production. This is the next step after continuous integration (CI). It prepares a software release that is ready for deployment, and is only waiting for teams to evaluate the change and decide whether to release it.
Continuous deployment takes this one step further, removing the need for a human to evaluate the new version and push the button to release the software. In continuous deployment, every change is automatically tested and if it meets certain predetermined quality criteria, it is automatically deployed to production.
What Is GitOps?
The GitOps model prescribes the use of source control systems, typically based on Git, for application and infrastructure configuration management. Git version control systems act as the single source of truth for GitOps. Based on this single source of truth, GitOps uses declarative configuration to adjust a production environment to match a desired state.
GitOps automatically manages the provisioning of infrastructure and deployment via Git pull requests. It relies on a Git repository containing the system’s complete state to ensure a full audit trail of system state changes.
The GitOps approach emphasizes developer experience, allowing Dev teams to manage infrastructure with familiar processes and tools used for other software development tasks. GitOps offers almost complete flexibility in the choice of tooling.
What Are the Benefits of GitOps?
There are many reasons to start using GitOps. Most of them are related to the ability to deliver software faster, more reliably, and with higher quality. Here are commonly cited GitOps benefits:
- Increased productivity – GitOps enables fully automated continuous deployment with an integrated feedback loop, which reduces deployment time compared to traditional CI/CD pipelines. According to the State of DevOps report by the DORA research group, acquired by Google, the four characteristics of the highest performing DevOps teams are high Deployment Frequency (DF), low Lead Time for Changes (MLT), low Time to Restore Service(s) (MTTR), and low Change Failure Rate (CR). GitOps can directly improve all four of these metrics.
- Improved developer experience – when operating in a Kubernetes cluster, GitOps removes the need to execute kubectl commands. Instead of having to learn and maintain Kubernetes internals, developers can use familiar tools like Git to manage Kubernetes updates and features declaratively, and any operations on the Kubernetes cluster are carried out automatically by the GitOps controller. New developers can ramp up more quickly and become productive in days instead of months, and experienced developers can rely on their knowledge of existing tools.
- Improved stability – in a GitOps workflow, audit logs are automatically created for all changes. This auditability promotes stability, because it is easy to see what changes resulted in production issues. It can also be used for compliance with any necessary standards such as SOC 2.
- Improved reliability and rollback – Git provides rollback and fork features that allow teams to achieve reliable and repeatable rollbacks. Because Git is the source of truth of the cluster’s configuration, the team has a single source to recover from any production issue. This reduces recovery time from hours to minutes.
- Consistency and standardization – GitOps provides a model for changing infrastructure, applications, and Kubernetes add-ons in a consistent way, providing visibility across the enterprise and ensuring all teams have a consistent end-to-end workflow.
- Security guarantees – Git can sign changes and prove author and origin, and provides strong encryption for tracking and managing changes. This provides a high level of trust over the integrity and security of a Kubernetes cluster.
What Is Observability and How Does it Support GitOps?
Traditional monitoring methods have reached their limits in the context of cloud native application architectures. The focus is shifting from monitoring to observability:
- System monitoring involves detecting a set of known problems by determining the health of the system against predefined metrics. For example, container monitoring aims to answer two questions: what went wrong, and why. Over time, this enables profiling a container to anticipate, predict, and prevent problems before they happen.
- Observability aims to provide an understanding of the state of a system based on three key elements – logs, metrics, and traces. Observability is a characteristic of a system – just like a system can be scalable, reliable, or secure, it can also be observable. In a cloud native environment, observability should be built into applications from day one.
Monitoring and observability are strongly connected. An observable system can be more easily monitored. Monitoring is part of observability, and effective monitoring is a result of an effectively observable system.
Observability provides insights using three main concepts:
- Logs – provide a record of discrete system events.
- Metrics – measure and process numerical and statistical data at set time intervals.
- Traces – provide an event sequence to map the logic path taken.
These three types of insights provide answers to most critical questions, including the current state of the deployment compared to the intended state. They are important for all aspects of the system ranging from intended architecture and configurations to the UI, resources, and behavior.
The Need for Observability in a GitOps Process
The GitOps model emphasizes the ability to simplify complex Kubernetes management tasks. The core concept is the deployment to production via changes to a central Git repository, with changes made to a Kubernetes cluster fully automatically.
To enable a true GitOps process, there is a need for two types of observability:
- Internal observability – the GitOps controller needs to know what is happening in the Kubernetes cluster, for example, in order to compare it with a desired configuration and make adjustments.
- External observability – other systems operating within and outside the cluster need to be aware of workflows automated by the GitOps system. To this end, the GitOps system should publish metrics that cloud native monitoring systems can consume.
How Does Internal Observability Work?
In a GitOps work process, Git is the single source of truth for the system’s intended state, while observability provides a single source of truth for the system’s actual state. Thus, it allows GitOps developers to understand the system’s state in the real world.
If, for example, you intend to have three NGINX pods running in the cluster based on a deployment manifest in your Git repository. The GitOps system will use Kubernetes controllers to determine how many pods are actually running and their current configuration. If it detects the wrong number of instances or any change to pod configuration (this is known as configuration drift), it creates a “diff alert”.
Once the system is aware of a divergence (i.e., a mismatch between the desired and actual number of instances), the diff alerts can trigger the relevant Kubernetes controller. The controller will attempt to synchronize the actual and desired states. Once there are no diff alerts, the system concludes that the actual state matches the desired state, meaning the application is “synchronized”.
The key concept throughout this process is awareness of divergence. You cannot sync or fix the state if you don’t know it is out of sync. Thus, internal observability is critical for enabling GitOps and ensuring the actual state remains up to date.
How Does External Observability Work?
External observability has three elements:
- A monitoring system must be running in the Kubernetes cluster. There are several mature tools that support cloud native environments – a common choice is Prometheus for Kubernetes.
- A GitOps controller making changes to the cluster in accordance with a Git configuration.
- Published metrics generated by the GitOps controller or related systems.
Once these three elements are in place, the monitoring system scrapes metrics from GitOps automation systems in the cluster. This can proactively inform the rest of your ecosystem what changes are taking place. In other words, other systems get a “heads up” that an application is being synchronized, instead of discovering it in retrospect and generating unnecessary alerts.
Let’s see how this works with a popular GitOps project: Argo.
What Is Argo?
Argo is a collection of open source projects that help developers deliver software faster and more securely. Argo is Kubernetes native, making it easy for developers to deploy and publish their own applications.
Argo tools enable continuous deployment with advanced, progressive deployment strategies, allowing developers to define the set of actions required to release a service:
- Argo CD is a GitOps-based continuous deployment tool for Kubernetes. The configuration logic is in Git, and developers can work on their code using the same development, review, and approval workflows they already use in Git-based repositories. Argo CD does not have continuous integration, but integrates with CI systems.
- Argo Rollouts is an incremental delivery controller built for Kubernetes. It enables progressive deployment strategies out of the box, including canary deployments, blue/green deployments, and A/B testing.
- Argo Workflows is a container-native workflow engine for orchestrating parallel tasks on Kubernetes.
- Argo Events is an event-driven workflow automation framework and dependency manager that can manage events from a variety of sources, including Kubernetes resources, Argo workflows, and serverless workloads.
In the context of GitOps, Argo facilitates application deployment and lifecycle management. It makes it possible for developers to operate environments and infrastructure seamlessly, automating deployments, facilitating rollbacks, and enabling easy troubleshooting.
Argo as an Enabling Technology for GitOps
Argo uses Kubernetes manifests to continuously monitor Git repositories, verify commits, proactively fetch changes from repositories, and synchronize them with cluster resources. This synchronous reconciliation process ensures the state of the cluster configuration always matches the state described in Git.
This is the exact definition of GitOps – meaning that Argo allows teams to implement a full GitOps process easily, in their existing Kubernetes clusters, and without changing their existing work processes.
In addition, Argo eliminates the common problem of configuration drift, which occurs when elements in a cluster diverge over time from a desired configuration. These unexpected configuration differences are one of the most common reasons for deployment failures. Argo can automatically revert any configuration drift, or at least show the deployment history of the cluster to identify drift and identify the change that led to it.
Lastly, the Argo project aims to provide a better experience for Kubernetes developers, maintaining a familiar user experience while easily applying advanced deployment strategies. It is implemented as Kubernetes Custom Resource Definitions (CRDs), meaning that it works just like existing Kubernetes objects with extensions that developers can easily learn and use.
To summarize, Argo makes it easier to implement GitOps for the following reasons:
- A more efficient workflow – developers can deploy code using familiar processes and tools.
- Improved reliability and consistency – using an automated agent to ensure that the desired state defined in Git is the same as the state of the cluster.
- Improved productivity – with fully automated CD and no complex setup.
- Reduced deployment complexity – deployment becomes a transparent process that occurs behind the scenes.
- Progressive delivery – in a traditional setup it was very difficult to set up strategies like blue/green or canary deployment, and these are available out of the box in Argo.
Argo CD: GitOps with Observability Built In
Internal Observability in Argo CD
Argo CD receives information about resource status and health via the Kubernetes API Server. When it detects a change between current cluster state and the configuration in Git, it goes through three phases:
- Pre-sync – checking if the change is valid and requires a change to the cluster
- Sync – making a change to the custer
- Post-sync – verifying that the change was made correctly
This process occurs in one or more waves that sweep the entire cluster, looking for changes, and reacting to diffs. The order of resources within a wave is determined by type (namespaces, then Kubernetes resources, then custom resources) and by name.
Within each wave, if any resource is out-of-sync, Argo CD adjusts it and then continues sweeping the cluster. Note that if resources are unhealthy in the first wave, the application may not be able to synchronize successfully.
Argo CD has a delay between each sync wave in order to give other controllers a chance to react to the change. This also prevents Argo CD from assessing resource health too quickly, before it updates to reflect the current object state.
External Observability in Argo CD and Argo Workflows
Argo CD provides a notifications feature, which lets you continuously monitor Argo CD apps and receive alerts about significant changes in the state of an application. It offers a flexible way to set up notifications with templates and triggers – you can define the content of notifications and when Argo CD should send them.
Another part of the Argo project is Argo Workflows, which lets you automate tasks related to CI/CD pipelines in a Kubernetes cluster. Argo Workflows generates several default controller metrics, and lets you define custom metrics to provide information about the state of Workflows.
Argo Workflows generates two types of metrics:
- Controller metrics – provide information about the state of the controller.
- Custom metrics – provide information about the state of your Workflow. You define the custom metrics using the Workflow specifications. The owner of the metric generator is responsible for generating custom metrics.
For example, you can define custom Prometheus metrics and apply them at the Workflow or Template level. These metrics are useful for various cases, including:
- Enforcing thresholds – keep track of your Template or Worklfow’s duration and receive alerts when they exceed your threshold.
- Tracking failures – see how often your Template or Workflow fails across a certain timeframe.
- Metric reporting – set up reports for internal metrics like the model training score and error rate.
Conclusion
GitOps is gaining traction as a mainstream development practice. I showed why observability is an inseparable part of GitOps systems, and described two types of observability:
- Internal observability – required for the GitOps controller to identify configuration drift in the cluster and correct it.
- External observability – required to notify operations staff and other systems of changes made by the GitOps controller.
I briefly showed how both of these are implemented in a popular open source GitOps platform – the Argo project.
GitOps is based on several complex mechanisms, and the best way to wrap your mind around them is to take ArgoCD for a test drive. Check out the official getting started tutorial which shows how to install ArgoCD and deploy a minimal application to a Kubernetes cluster. Try to “mess up” your test cluster and see how Argo picks up the changes and reverts the cluster to your desired configuration.
To go more in depth and understand the ArgoCD sync processes, see the discussion on Sync Phases and Sync Waves in the official documentation.
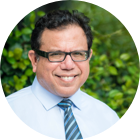
MMS • Sabri Bolkar
Article originally posted on InfoQ. Visit InfoQ
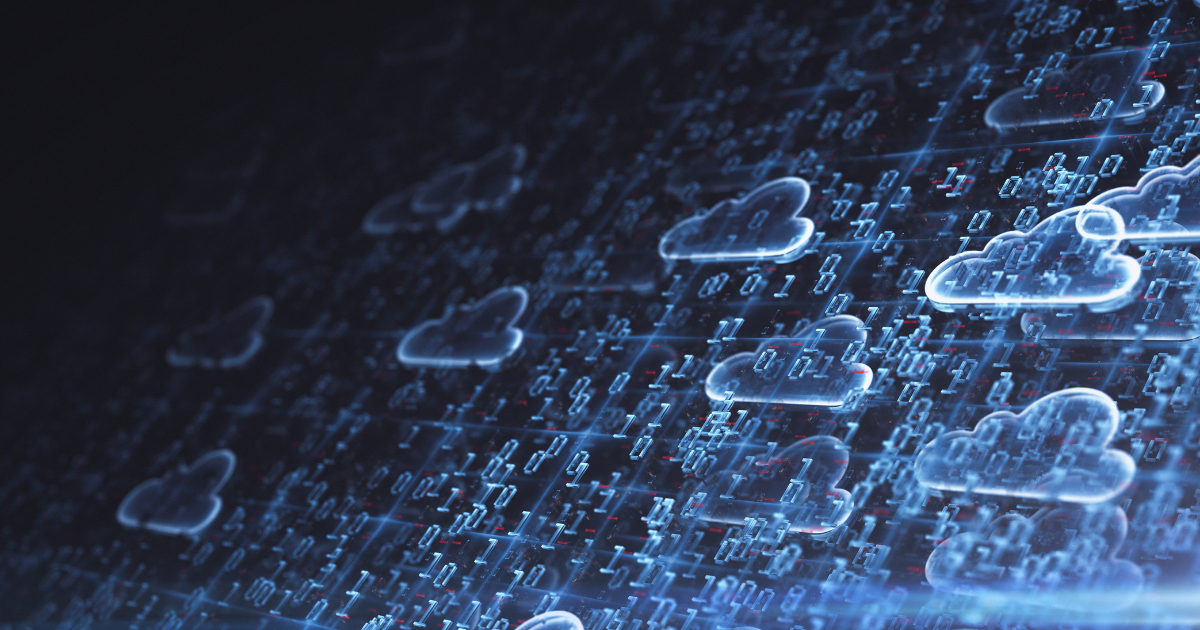
A new open-source library called Alpa aims to automate distributed training and serving of large deep networks. It proposes a compiler where existing model-parallel strategies are combined and the usage of computing resources is optimized according to the deep network architecture.
The network inference loss scales logarithmically with the number of weight parameters and utilized data during training. This led to an increased effort within the deep learning community to develop larger models. To keep up with the growing network size, the scaling of training compute has also accelerated, doubling approximately every 6 months. As accelerator memory capabilities are limited, the main engineering obstacle is the mapping of network parameters to the existing accelerator devices depending on both cluster properties and communication primitives. In particular, this problem surfaces during training as corresponding gradient tensors are also stored and exchanged via the accelerator memory.
There are two main methods to carry out model-parallel distributed training. In the first strategy, functions (e.g. convolutional layers) constituting the computational graph are divided between the devices (aka inter-operator parallelism), and input mini-batches are split into micro-batches where each micro-batch is executed over the same set of functions (aka pipeline) that are placed on multiple devices (e.g. Device Placement Optimization, GPipe). In the second case, the function parameters are divided (aka intra-operator parallelism) and batch inputs are run over different parts of function parameters that are placed on different devices (e.g. GShard, DeepSpeed-ZeRO).
Both strategies have related trade-offs. For example, inter-operator parallelism offers lower network bandwidth usage which can be a favorable scenario for multi-node training. Intra-operator parallelism, on the other hand, minimizes GPU idle time but suffers from higher data exchange requirements. Therefore, it may be more suitable when GPUs are connected with high-bandwidth connection primitives such as NVIDIA NVLink or AMD xGMI. Current training stations are generally made of multiple GPU units with custom inter-GPU connection modules. However, this is not the case on public cloud, hence a hybrid strategy utilizing both inter- and intra-operator parallelism may significantly improve resource usage.
Deep learning libraries continue to release new APIs assisting placement planning for model parameters and input data such as DTensor for Tensorflow and FSDP for PyTorch. The distribution plans can be manually created (e.g. Megatron-LM), but it is beneficial to have auto-generated plans and training schedules especially for very large networks and for AutoML-designed architectures. Alpha can be considered as an attempt to automate such placement procedures. Its compiler leverages both intra- and inter-function parallelism to output runtime-optimized distributed training strategies depending on the cluster and the deep network structure.
Currently, Alpa is built upon Jax that offers automatic composable transformations (i.e. automatic vectorization, gradient computation, SPMD parallelization, and JIT compilation) for side-effect-free function chains applied to the data.
The results presented in the OSDI 22 paper indicate that Alpa provides competitive training strategies when compared to the manual placement and the previous state-of-the-art methods. Additional info can be obtained from the official Google blog and details related to the design decisions are explained on the official documentation. The project also showcases a proof-of-concept OPT-175B model server.
Java News Roundup: OpenJDK Updates, JDK 20 Release Schedule, GraalVM 22.3, JReleaser 1.3.0
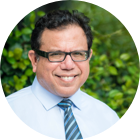
MMS • Michael Redlich
Article originally posted on InfoQ. Visit InfoQ
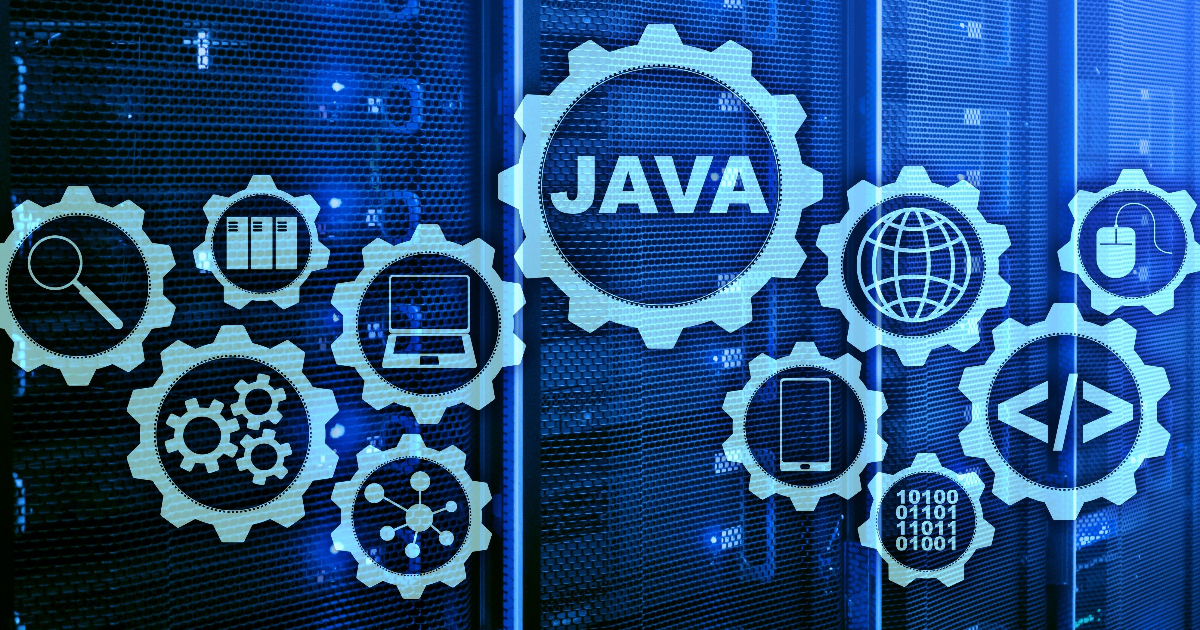
This week’s Java roundup for October 24th, 2022 features news from OpenJDK, JDK 20 release schedule, Build 20-loom+20-34, Spring Integration 6.0-RC1, Spring Tools 4.16.1, GraalVM 22.3, Open Liberty 22.0.0.11 and 22.0.0.12-beta, Eclipse Vert.x 3.9.14, Apache TomEE 8.0.13, JReleaser 1.3.0, Hibernate Search 5.11.11 and 5.10.13, PrimeFaces point releases, JDKMon 17.0.37 and EclipseCon 2022.
OpenJDK
JEP 434, Foreign Function & Memory API (Second Preview), was promoted from its Draft 8293649 to Candidate status this past week. This JEP, under the auspices of Project Panama, evolves: JEP 424, Foreign Function & Memory API (Preview), delivered in JDK 19; JEP 419, Foreign Function & Memory API (Second Incubator), delivered in JDK 18; and JEP 412, Foreign Function & Memory API (Incubator), delivered in JDK 17. It proposes to incorporate refinements based on feedback and to provide a second preview in JDK 20. Updates include: the MemorySegment
and MemoryAddress
interfaces are now unified, i.e., memory addresses are modeled by zero-length memory segments; and the sealed MemoryLayout
interface has been enhanced to facilitate usage with JEP 427, Pattern Matching for switch (Third Preview).
JEP Draft 8295817, Virtual Threads (Second Preview), has been promoted to Submitted status this past week. This JEP, also under the auspices of Project Loom, proposes a second preview from JEP 425, Virtual Threads (Preview), delivered in JDK 19, to allow time for additional feedback and experience for this feature to progress. It is important to note that no changes are within this preview except for a small number of APIs from JEP 425 that were made permanent in JDK 19 and, therefore, not proposed in this second preview.
Similarly, JEP Draft 8296037, Structured Concurrency (Second Incubator), has been promoted to Submitted status. This JEP, also under the auspices of Project Loom, proposes to reincubate this feature from JEP 428, Structured Concurrency (Incubator), delivered in JDK 19, in JDK 20 to allow time for additional feedback and experience. The only change is an updated StructuredTaskScope
class to support the inheritance of scoped values by threads created in a task scope. This streamlines the sharing of immutable data across threads.
JDK 20
Build 21 of the JDK 20 early-access builds was also made available this past week, featuring updates from Build 20 that include fixes to various issues. Further details on this build may be found in the release notes.
Mark Reinhold, chief architect, Java Platform Group at Oracle, formally announced the release schedule for JDK 20 as follows:
- Rampdown Phase One (fork from main line): December 8, 2022
- Rampdown Phase Two: January 19, 2023
- Initial Release Candidate: February 9, 2023
- Final Release Candidate: February 23, 2023
- General Availability: March 21, 2023
For JDK 20, developers are encouraged to report bugs via the Java Bug Database.
Project Loom
Build 20-loom+20-34 of the Project Loom early-access builds was made available to the Java community and is based on Build 20 of JDK 20 early-access builds.
Spring Framework
On the road to Spring Integration 6.0.0, the first release candidate was made available featuring support for: RabbitMQ Streams, Kotlin Coroutines and GraalVM polyglot JavaScript invocations. This version also includes the removal of Spring Data for Apache Geode. More details on this release may be found in the release notes.
Spring Tools 4.16.1 for Eclipse, Visual Studio Code, and Theia has been released featuring early access builds available for Spring Tools 4 on Eclipse 2022-12 milestones. Developers who plan to upgrade from Spring Tools 4.15.3 should follow this migration guide due to a major update in m2e
2.0 that ships with Eclipse 2022-09. Further details on this release may be found in the release notes.
GraalVM
Oracle Labs has released GraalVM 22.3 featuring: support for JDK 19 and jlink
; and Native Image monitoring and developer experience updates. As announced at JavaOne, the GraalVM CE Java code will become part of OpenJDK. This is the last feature release of 2022. More details on this release may be found in the release notes and this YouTube video. InfoQ will follow up with a more detailed news story.
Open Liberty
IBM has promoted Open Liberty 22.0.0.11 from its beta release to deliver: support for JDK 19 and distributed security caching so that multiple Liberty servers can share caches by using a JCache provider. This version also addresses CVE-2022-24839, a vulnerability out of Nokogiri (Rubygem), a fork of the now-defunct org.cyberneko.html
, that raises a OutOfMemoryError
exception when parsing ill-formed HTML markup.
Open Liberty 22.0.0.12-beta has also been released that offers support for six new Jakarta EE 10 specifications: Jakarta Batch 2.1, Jakarta XML Web Services 4.0, Jakarta Server Pages 3.1, Jakarta Standard Tag Library 3.0, Jakarta Messaging 3.1 and Jakarta WebSocket 2.1. There is also support for two updated specifications in the upcoming release of MicroProfile 6.0: JWT Propagation 2.1 and MicroProfile Metrics 5.0.
Eclipse Vert.x
Eclipse Vert.x 3.9.14 has been released that ships with dependency upgrades to GraphQL Java 19.2, Netty 4.1.84.Final, Protocol Buffers Java 3.21.7 and Jackson Databind that addresses CVE-2022-42003, a denial of service vulnerability in Jackson Databind. The 3.9 release train is scheduled to reach end of life by the end of 2022, so developers are encouraged to upgrade to Vert.x 4.x. Further details on this release may be found in the release notes.
Apache Software Foundation
Apache TomEE 8.0.13 has been released featuring: an example on how to work with properties providers; and dependency upgrades that include Jakarta Faces 2.3.18, MyFaces 2.3.10, Hibernate Integration 5.6.9.Final, BatchEE 1.0.2, Tomcat 9.0.68 and SnakeYAML 1.33. More details on this release may be found in the release notes.
JReleaser
Version 1.3.0 of JReleaser, a Java utility that streamlines creating project releases, has been made available featuring: a new WorkflowListener
extension that reacts to workflow events; an option to install additional native-image components; and support for deploying JARs and POMs to Maven compatible repositories. Further details on this release may be found in the changelog.
Hibernate
Versions 5.11.11.Final and 5.10.13.Final of Hibernate Search have been released that feature dependency upgrades to Hibernate ORM versions 5.4.33.Final and 5.3.28.Final, respectively. Version 5.10.13 also provides a fix for a ClassCastException
being thrown when creating a FullTextSession
interface from an EntityManager
interface created by Spring Boot 2.4.0+ and Spring Framework 5.3+.
PrimeFaces
PrimeFaces, a provider of open-source UI component libraries, has provided point releases of PrimeFaces 7.0.30, 8.0.22, 10.0.17, 11.0.9 and 12.0.1. New features and enhancements include: implement an IN
match mode, i.e., filterMatchMode="in"
, for the JpaLazyDataModel
class; and ensure that the emptyLabel
attribute when using the SelectCheckboxMenu
class doesn’t display text.
PrimeVue 3.18.0 has also been released that delivers: accessibility enhancements to all menu components; templating support for FileUpload
; and a responsive Paginator
. More details on this release may be found in the changelog.
JDKMon
Version 17.0.37 of JDKMon, a tool that monitors and updates installed JDKs, has been made available to the Java community this past week. Created by Gerrit Grunwald, principal engineer at Azul, this new version ships with a fix for the detection of GraalVM builds.
EclipseCon
EclipseCon 2022 was held at the Forum am Schlosspark in Ludwigsburg, Germany this past week featuring speakers from the Java community that presented on topics such as Java, The Open Source Way, Cloud Native Technologies and All Things Quality & Security. The conference included the annual Community Day that precedes the conference.
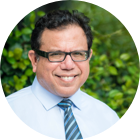
MMS • Steef-Jan Wiggers
Article originally posted on InfoQ. Visit InfoQ
Recently Microsoft announced the public preview of a new version of the Computer Vision Image Analysis API, making all visual image features ranging from Optical Character Recognition (OCR) to object detection available through a single endpoint.
Computer Vision Image Analysis API is part of Microsoft Azure Cognitive Service offering. With the API, customers can extract various visual features from their images. The latest version, 4.0, offers a new feature with OCR, optimized for image scenarios that make OCR easy to use for user interfaces and near real-time experiences. In addition, it now supports 164 languages, including Cyrillic, Arabic, and Hindi languages. The feature recognizes printed and handwritten text in image files (supported formats are .JPEG, .JPG, .PNG, .BMP, and .TIFF).
Next to the OCR feature, also in preview is the “detect people in image” feature. Finally, all the features are available in one API.
Note that Microsoft tests its products with the API. PowerPoint, Designer, Word, Outlook, Edge, and LinkedIn are using Vision APIs to power design suggestions, alt text for accessibility, SEO, document processing, and content moderation.
Besides the OCR and detecting people in image features of Image Analysis, the company also previews another feature: Spatial Analysis. With this feature, developers can create applications that count people in a room, understand dwell times in front of a retail display, and determine wait times in lines.
The upgrade of Computer Vision API is also part of Microsoft’s Responsible AI process and its principles of fairness, inclusiveness, reliability, safety, transparency, privacy and security, and accountability. Other public cloud companies like Google follow the same principle with their responsible AI guidelines.
The company recommends using the new version of the API in the future. Developers can use Image Analysis through a client library SDK by calling the REST API or try out the feature with Vision Studio.
More details on the Computer Vision API are available on the documentation landing page and FAQs. Price details and availability can be found on the pricing page.
Lastly, Andy Beatman, a senior product marketing manager at Azure AI, revealed what will come next in an Azure blog post:
We will continue to release breakthrough vision AI through this new API over the coming months, including capabilities powered by the Florence foundation model featured in this year’s premiere computer vision conference keynote at CVPR.
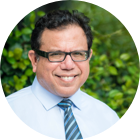
MMS • Edin Kapic
Article originally posted on InfoQ. Visit InfoQ
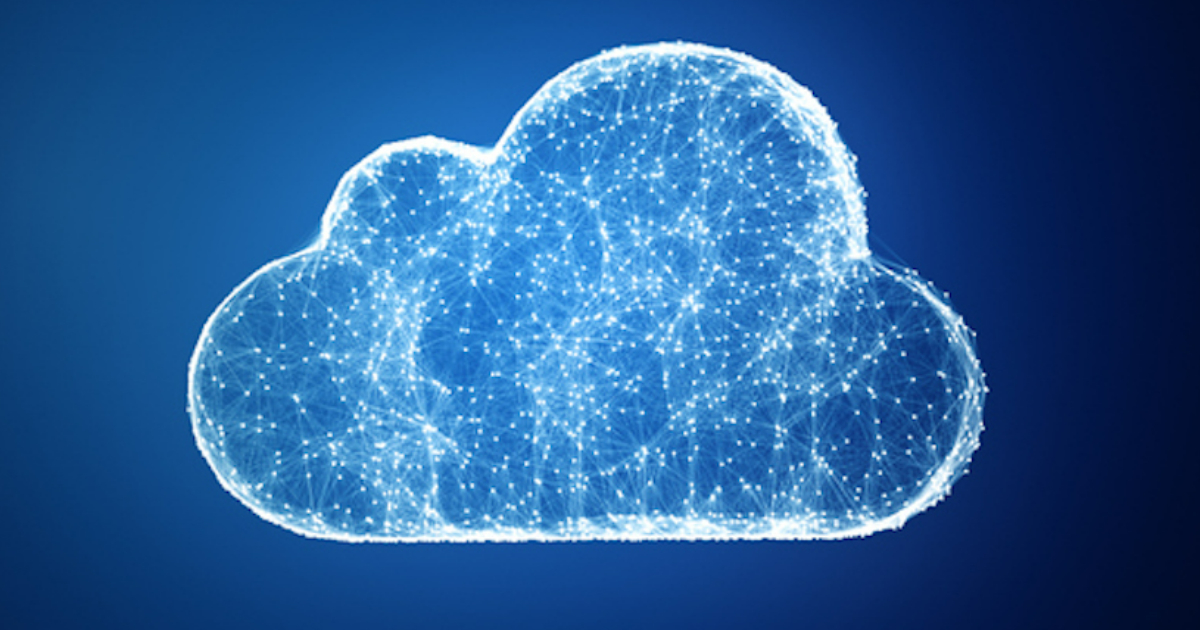
Microsoft announced on September 26th that Azure Functions runtime v4 will support running .NET Framework 4.8 functions in an isolated process, allowing the developers to move their legacy .NET functions to the latest runtime. The isolated process execution decouples the function code from the Azure Functions’ host runtime, which in turn allows running different .NET frameworks within a single environment.
Azure Functions now offer four runtime versions for functions written in .NET:
- v1: Runs on .NET Framework 4.8 only. Supported without end-of-life announcement yet. No security updates.
- v2: Runs on .NET Core 2.1. currently not supported for new functions. No security updates.
- v3: Runs on .NET Core 3.1. Supported until December 2022 (linked to .NET 3.1 end-of-life).
- v4: Runs on .NET 6 but supports all versions of .NET (including .NET Framwork 4.8) when running in isolated mode.
Azure Functions runtime v4 introduces isolated process execution. Until v4, function code runs in the same context of the underlying function host process that’s running on the server. It allows for fast performance and startup time, but it ties the developer to the .NET version that the runtime is using.
Isolated process execution launches the function code in a separate console application on the function host server. The function host then invokes the function code using inter-process communication. According to Microsoft’s announced Azure Functions roadmap update, the in-process and isolated process execution models will coexist in v4 runtime until feature and performance parity is achieved. Once a sufficient parity is achieved, the runtime will only allow isolated process execution.
From the technical perspective there are some adjustments that developers have to do to change their existing functions to run in an isolated process, outlined by Microsoft in a quick guide in the documentation. Functions running inside an isolated process can’t use direct trigger and binding references (such as accessing the BrokeredMessage
when binding to a Service Bus queue). They can only use simple data types and arrays. The original HTTP context is available as an instance of HttpRequestData
class. In the same fashion, writing to a HTTP output is achieved using HttpResponseData
class. The Azure Function runtime manages the mapping of those classes to the real HttpContext
instance. Developers will also have to update unit tests that cover existing in-process function classes, using fake data for incoming HTTP data. One such approach has been explained by Carlton Upperdine on his blog.
The bindings for functions running in an isolated process are different from the bindings of in-process functions. While the in-process ones leverage the underlying Azure WebJob definitions and bindings, isolated process functions must add the Microsoft.Azure.Functions.Worker.Extensions
NuGet packages.
On the other hand, using the isolated process execution will allow developers to build the underlying host pipeline in the Program.cs
file, like the way ASP.NET Core applications build their own execution host. It supports building custom middlewares and injecting dependencies in a straightforward fashion. For convenience, there is a ConfigureFunctionsWorkerDefaults method that sets up the host execution pipeline with integrated logging, a default set of binders, adds gRPC support and correctly sets up the JSON serializer for property casing.
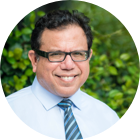
MMS • Sergio De Simone
Article originally posted on InfoQ. Visit InfoQ
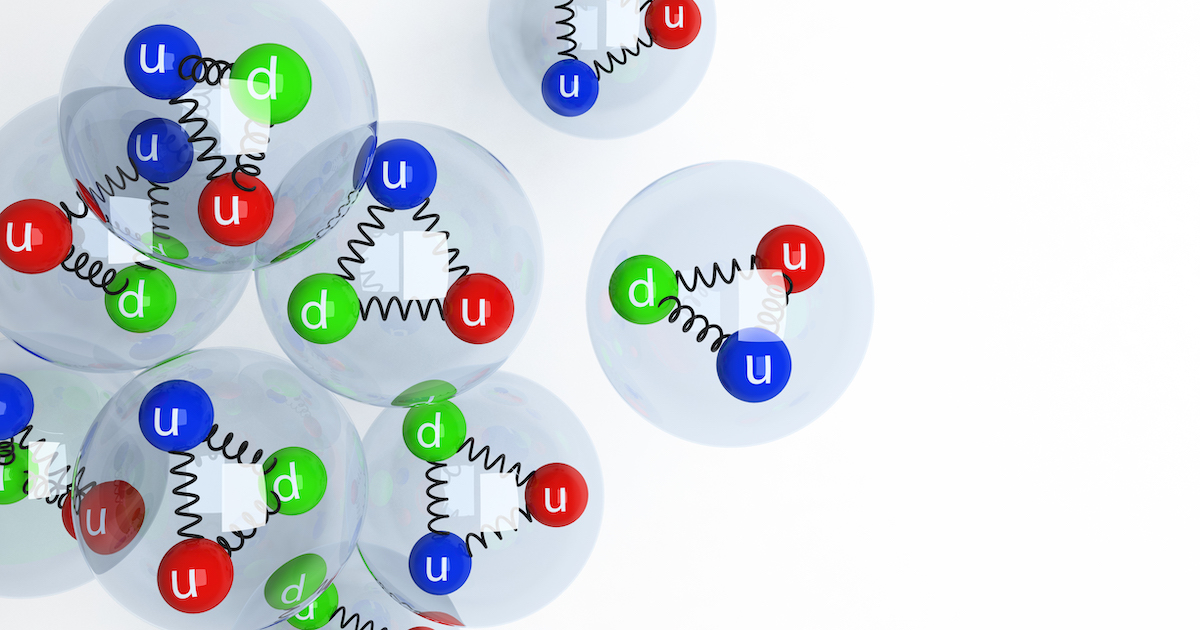
Previously known as AWS Amplify iOS, AWS Amplify for Swift now offers a rewritten API to support Swift async
/await
and make concurrency code more idiomatic. Additionally, the new release introduces beta support on macOS for a number of AWS features, including Auth, Storage, Geo, and others.
AWS Amplify for Swift is built on top of the AWS SDK for Swift, which is still only a developer preview. While insulating the developer from any changes in the underlying layer, AWS Amplify for Swift aims to provide a higher-level, declarative, and use-case centric API to access a number of AWS services, including Analytics, Authentication, DataStore, Geo, and Storage. Both REST and GraphQL are supported to access remote API data.
As mentioned, the most significant improvement in AWS Amplify for Swift is support for the new concurrency features introduced in Swift 5.5. Previous to version 2.0, AWS Amplify relied on a callback-based model for network and asynchronous operations. The adoption of async
/await
will make it easier for developers to write concurrent operations. For example, in the case of authentication:
do {
let signInResult = try await Amplify.Auth.signIn(username: username,
password: password)
if signInResult.isSignedIn {
print("Sign in succeeded")
}
} catch {
print("Sign in failed (error)")
}
AWS Amplify 2.0 also provides better support for debugging authentication and upload/download workflows thanks to a number of architectural improvements. Furthermore, AWS engineers took the chance to remove all calls to obsolete APIs, which makes AWS Amplify a warning-free dependency.
AWS Amplify has a modular architecture, where each supported service is implemented through a plugin. This makes the library theoretically able to support alternative services using the same high-level API, although no additional plugins other than those required for AWS are available.
AWS Amplify for Swift is part of a larger collection of tools AWS offers for mobile iOS and Android app development, including a CLI tool used to configure all AWS services used by the app and a number of UI components for React, React Native, Angular, Vue, and Flutter.