Month: July 2022
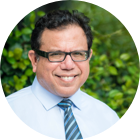
MMS • RSS
Posted on nosqlgooglealerts. Visit nosqlgooglealerts
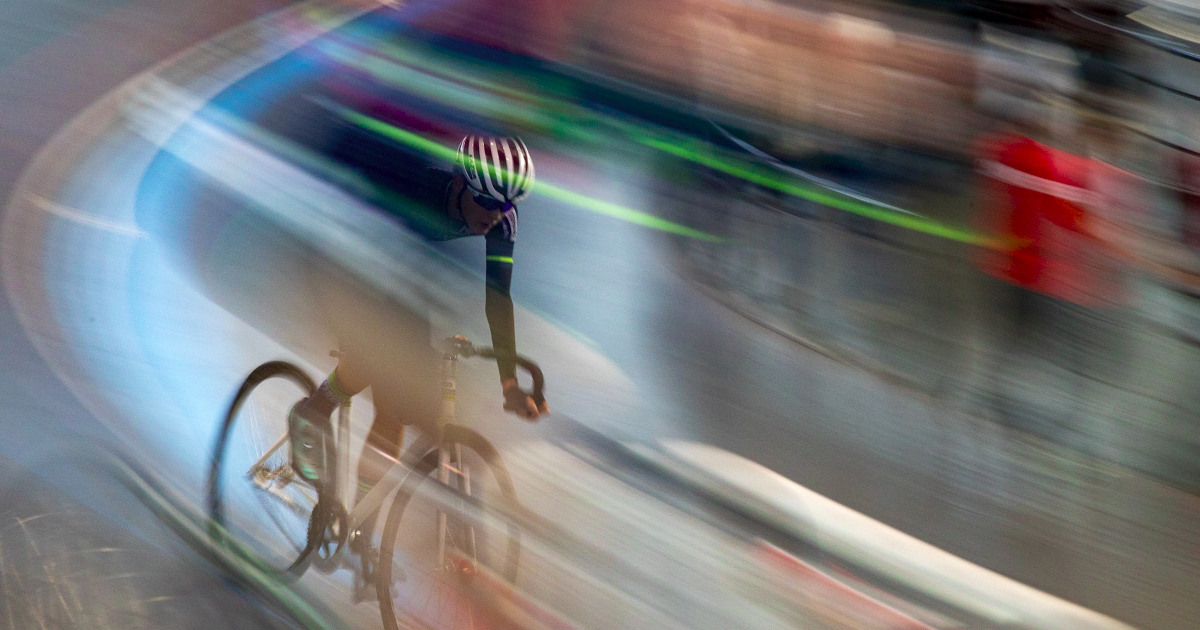
AWS recently announced the general availability of the R6a instances, EC2 designed for memory-intensive workloads like SQL and NoSQL databases.. The new instances are built on the AWS Nitro System and are powered by AMD Milan processors.
With sizes from r6a.large to r6a.48xlarge, the new instances provide up to 192 vCPU and 1536 GiB of memory, twice the limit of the r5a, for vertical scaling of databases and in-memory workloads. Channy Yun, principal developer advocate at AWS, explains:
R6a instances, powered by 3rd Gen AMD EPYC processors are well suited for memory-intensive applications such as high-performance databases (relational databases, noSQL databases), distributed web scale in-memory caches (such as memcached, Redis), in-memory databases such as real-time big data analytics (such as Hadoop, Spark clusters), and other enterprise applications.
As covered recently on InfoQ, the Cockroach Labs’ 2022 cloud report reported that AMD-based instances running Milan processors are the price-for-performance leaders on the major cloud providers and a better choice than Intel ones. According to AWS, the new AMD memory-optimized instances provide up to 35% better compute price performance compared to the previous generation instances and cost 10% less than x86-based R6i instances.
Differently from the previous generation r5a, the r6a instances are SAP-certified for memory-intensive enterprise databases like SAP Business Suite. Mario de Felipe, global director at Syntax, notes:
The not great news is they are not HANA certified. Despite the R family at AWS being focused on DB workloads, and the R family is a great host of SAP databases (Oracle, SQLserver, DB2, or HANA), the HANA database runs on Intel (…) This makes the r6a family the best option available for SAP AnyDB option (excluding HANA).
R6a instances support 40 Gbps bandwidth to EBS in the largest size, more than doubling the R5a limit, and up to 50 Gbps networking. On the largest size, customers can also enable the Elastic Fabric Adapter, the network interface designed for running HPC and ML applications at scale relying on high levels of inter-node communications.
Supporting new AVX2 instructions for accelerating encryption and decryption algorithms, the AMD Milan processor provides Secure Encrypted Virtualization (SEV). Khawaja Shams, co-founder at momento, tweets:
So excited to see always-on memory encryption in the new R6a instances! Glad to see this support go beyond Graviton2/3 & the M6i instances.
The new instances are currently available in a subset of AWS regions: Ohio, Northern Virginia, Oregon, Mumbai, Frankfurt, and Ireland. The on-demand hourly rate goes from 0.1134 USD (r6a.large) to 10.8864 USD (r6a.48xlarge) in the US East regions.
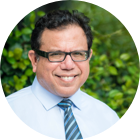
MMS • RSS
Posted on mongodb google news. Visit mongodb google news
Prospera Financial Services Inc purchased a new position in MongoDB, Inc. (NASDAQ:MDB – Get Rating) during the 1st quarter, HoldingsChannel reports. The fund purchased 2,091 shares of the company’s stock, valued at approximately $927,000.
Several other institutional investors have also recently made changes to their positions in the company. Commerce Bank increased its holdings in shares of MongoDB by 1.7% in the fourth quarter. Commerce Bank now owns 1,413 shares of the company’s stock worth $747,000 after purchasing an additional 24 shares during the period. Total Clarity Wealth Management Inc. increased its stake in MongoDB by 6.9% in the first quarter. Total Clarity Wealth Management Inc. now owns 465 shares of the company’s stock valued at $206,000 after acquiring an additional 30 shares during the last quarter. Profund Advisors LLC increased its stake in MongoDB by 5.2% in the fourth quarter. Profund Advisors LLC now owns 647 shares of the company’s stock valued at $342,000 after acquiring an additional 32 shares during the last quarter. Ieq Capital LLC increased its stake in MongoDB by 2.3% in the first quarter. Ieq Capital LLC now owns 1,485 shares of the company’s stock valued at $659,000 after acquiring an additional 34 shares during the last quarter. Finally, Wedbush Securities Inc. increased its stake in MongoDB by 1.8% in the first quarter. Wedbush Securities Inc. now owns 2,253 shares of the company’s stock valued at $999,000 after acquiring an additional 40 shares during the last quarter. Institutional investors own 88.70% of the company’s stock.
MongoDB Stock Performance
MongoDB stock opened at $312.47 on Friday. The company has a current ratio of 4.16, a quick ratio of 4.16 and a debt-to-equity ratio of 1.69. The firm has a 50-day moving average of $275.80 and a 200-day moving average of $338.12. The firm has a market cap of $21.28 billion, a P/E ratio of -64.56 and a beta of 0.91. MongoDB, Inc. has a 1-year low of $213.39 and a 1-year high of $590.00.
MongoDB (NASDAQ:MDB – Get Rating) last posted its earnings results on Wednesday, June 1st. The company reported ($1.15) earnings per share for the quarter, topping analysts’ consensus estimates of ($1.34) by $0.19. The company had revenue of $285.45 million during the quarter, compared to analyst estimates of $267.10 million. MongoDB had a negative net margin of 32.75% and a negative return on equity of 45.56%. MongoDB’s revenue for the quarter was up 57.1% on a year-over-year basis. During the same period in the prior year, the business posted ($0.98) earnings per share. Sell-side analysts expect that MongoDB, Inc. will post -5.08 EPS for the current year.
Insiders Place Their Bets
In other MongoDB news, CRO Cedric Pech sold 350 shares of the firm’s stock in a transaction dated Tuesday, July 5th. The shares were sold at an average price of $264.46, for a total value of $92,561.00. Following the completion of the sale, the executive now directly owns 45,785 shares in the company, valued at $12,108,301.10. The sale was disclosed in a document filed with the Securities & Exchange Commission, which is accessible through this link. In other MongoDB news, Director Dwight A. Merriman sold 3,000 shares of the firm’s stock in a transaction dated Wednesday, June 1st. The shares were sold at an average price of $251.74, for a total value of $755,220.00. Following the completion of the sale, the director now directly owns 544,896 shares in the company, valued at $137,172,119.04. The sale was disclosed in a document filed with the Securities & Exchange Commission, which is accessible through this link. Also, CRO Cedric Pech sold 350 shares of the firm’s stock in a transaction dated Tuesday, July 5th. The stock was sold at an average price of $264.46, for a total transaction of $92,561.00. Following the completion of the sale, the executive now owns 45,785 shares of the company’s stock, valued at $12,108,301.10. The disclosure for this sale can be found here. Insiders sold 77,185 shares of company stock worth $23,594,636 over the last three months. Company insiders own 5.70% of the company’s stock.
Analyst Upgrades and Downgrades
A number of research analysts recently commented on the company. Mizuho reduced their target price on MongoDB from $325.00 to $270.00 and set a “neutral” rating on the stock in a research note on Wednesday, May 18th. Oppenheimer reduced their target price on MongoDB from $490.00 to $400.00 and set an “outperform” rating on the stock in a research note on Thursday, June 2nd. Robert W. Baird assumed coverage on MongoDB in a research note on Tuesday, July 12th. They issued an “outperform” rating and a $360.00 target price on the stock. Needham & Company LLC increased their target price on MongoDB from $310.00 to $350.00 and gave the company a “buy” rating in a research note on Friday, June 10th. Finally, Morgan Stanley reduced their price objective on MongoDB from $378.00 to $368.00 and set an “overweight” rating on the stock in a research note on Thursday, June 2nd. One research analyst has rated the stock with a sell rating, one has assigned a hold rating and sixteen have given a buy rating to the company. According to data from MarketBeat.com, the company currently has a consensus rating of “Moderate Buy” and a consensus price target of $401.17.
MongoDB Company Profile
MongoDB, Inc provides general purpose database platform worldwide. The company offers MongoDB Enterprise Advanced, a commercial database server for enterprise customers to run in the cloud, on-premise, or in a hybrid environment; MongoDB Atlas, a hosted multi-cloud database-as-a-service solution; and Community Server, a free-to-download version of its database, which includes the functionality that developers need to get started with MongoDB.
Featured Stories
Want to see what other hedge funds are holding MDB? Visit HoldingsChannel.com to get the latest 13F filings and insider trades for MongoDB, Inc. (NASDAQ:MDB – Get Rating).
Receive News & Ratings for MongoDB Daily – Enter your email address below to receive a concise daily summary of the latest news and analysts’ ratings for MongoDB and related companies with MarketBeat.com’s FREE daily email newsletter.
Article originally posted on mongodb google news. Visit mongodb google news
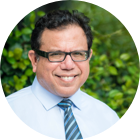
MMS • Renato Losio
Article originally posted on InfoQ. Visit InfoQ
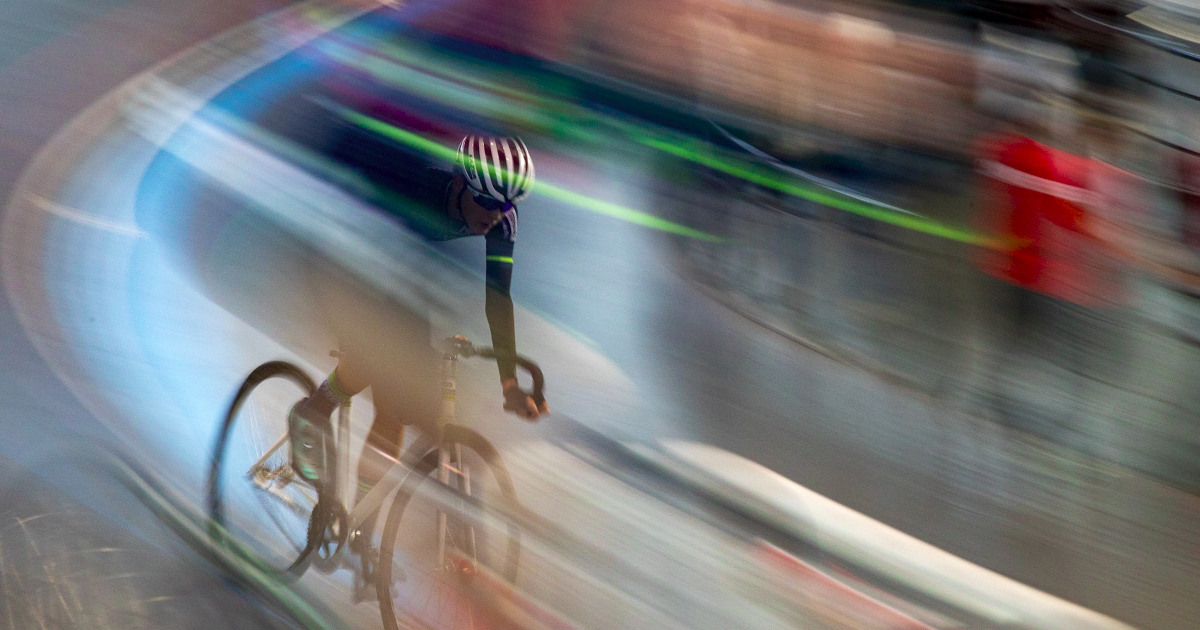
AWS recently announced the general availability of the R6a instances, EC2 designed for memory-intensive workloads like SQL and NoSQL databases.. The new instances are built on the AWS Nitro System and are powered by AMD Milan processors.
With sizes from r6a.large to r6a.48xlarge, the new instances provide up to 192 vCPU and 1536 GiB of memory, twice the limit of the r5a, for vertical scaling of databases and in-memory workloads. Channy Yun, principal developer advocate at AWS, explains:
R6a instances, powered by 3rd Gen AMD EPYC processors are well suited for memory-intensive applications such as high-performance databases (relational databases, noSQL databases), distributed web scale in-memory caches (such as memcached, Redis), in-memory databases such as real-time big data analytics (such as Hadoop, Spark clusters), and other enterprise applications.
As covered recently on InfoQ, the Cockroach Labs’ 2022 cloud report reported that AMD-based instances running Milan processors are the price-for-performance leaders on the major cloud providers and a better choice than Intel ones. According to AWS, the new AMD memory-optimized instances provide up to 35% better compute price performance compared to the previous generation instances and cost 10% less than x86-based R6i instances.
Differently from the previous generation r5a, the r6a instances are SAP-certified for memory-intensive enterprise databases like SAP Business Suite. Mario de Felipe, global director at Syntax, notes:
The not great news is they are not HANA certified. Despite the R family at AWS being focused on DB workloads, and the R family is a great host of SAP databases (Oracle, SQLserver, DB2, or HANA), the HANA database runs on Intel (…) This makes the r6a family the best option available for SAP AnyDB option (excluding HANA).
R6a instances support 40 Gbps bandwidth to EBS in the largest size, more than doubling the R5a limit, and up to 50 Gbps networking. On the largest size, customers can also enable the Elastic Fabric Adapter, the network interface designed for running HPC and ML applications at scale relying on high levels of inter-node communications.
Supporting new AVX2 instructions for accelerating encryption and decryption algorithms, the AMD Milan processor provides Secure Encrypted Virtualization (SEV). Khawaja Shams, co-founder at momento, tweets:
So excited to see always-on memory encryption in the new R6a instances! Glad to see this support go beyond Graviton2/3 & the M6i instances.
The new instances are currently available in a subset of AWS regions: Ohio, Northern Virginia, Oregon, Mumbai, Frankfurt, and Ireland. The on-demand hourly rate goes from 0.1134 USD (r6a.large) to 10.8864 USD (r6a.48xlarge) in the US East regions.
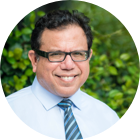
MMS • Sergio De Simone
Article originally posted on InfoQ. Visit InfoQ
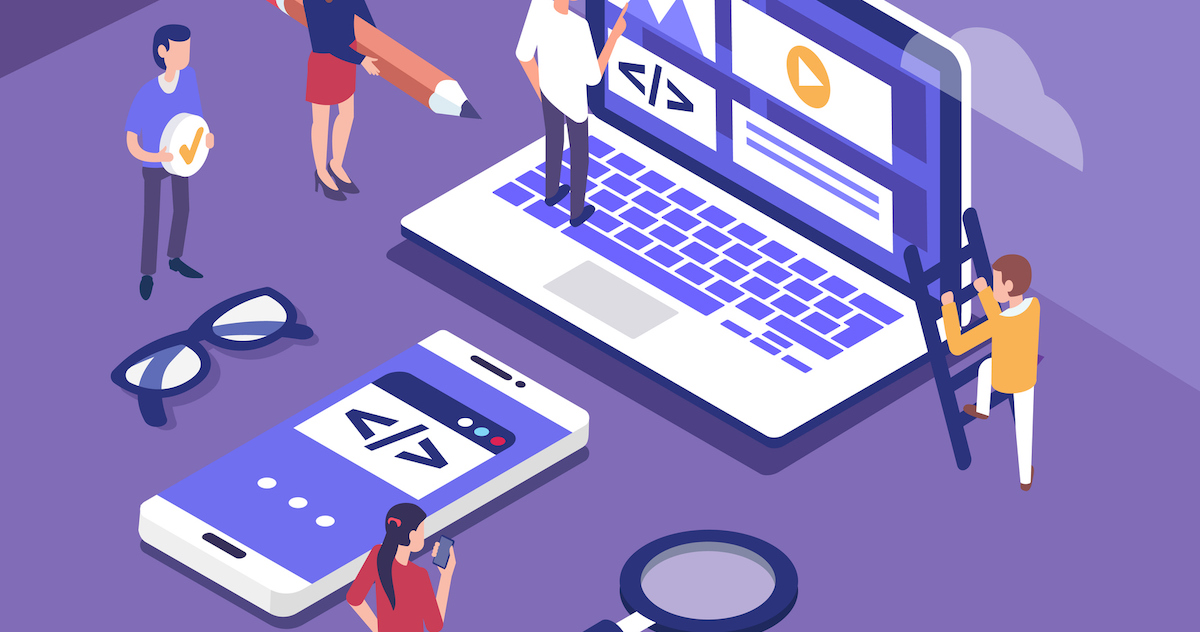
Jetpack Compose 1.2 stabilizes a number of features, including lazy grids, nested scroll, easing curve for animations, and more. In addition, it brings several new experimental features, like custom layouts and downloadable fonts, and fixes many issues.
Lazy grids can be built using the LazyHorizontalGrid and LazyVerticalGrid APIs. Their behaviour is optimized so only visible items are actually presented, meaning only columns or rows that are visible are rendered in memory. The following partial snippet shows how you can define a vertical grid with several items:
LazyVerticalGrid(
columns = GridCells.Fixed(3)
) {
items(itemsList) {
Text("Item is $it", itemModifier)
}
item {
Text("Single item", itemModifier)
}
}
Easing curves extends animation speed control, specifically to speed up or down the animating value either at the start or end of the animation. Easing can help to create smoother and more realistic animations. Beside the usual ease in and out curves, many other curves are available, e.g., to emphasize the animation at the end, to accelerate or decelerate it, and so on. The framework also allows to define custom easing curves, like in the following example:
val CustomEasing = Easing { fraction -> fraction * fraction }
@Composable
fun EasingUsage() {
val value by animateFloatAsState(
targetValue = 1f,
animationSpec = tween(
durationMillis = 300,
easing = CustomEasing
)
)
// ...
}
Nested scroll enables embedding a scrollable view within another scrollable view. Nested scroll is usually tricky to get right and Compose provides a nestedScroll
modifier that allows to define a scrolling hierarchy so scroll deltas are propagated from inner views to outer views when you reach scroll start or end bounds.
On the front of new experimental features, Compose 1.2 introduces lazy layouts, which could be seen as a generalization of lazy grids. As with lazy grids, lazy layouts only render those items that are actually visible to improve performance and increase efficiency.
Additionally, you can now use Google Fonts in your Android app using the GoogleFont
class, which you can instantiate by providing a font name and then use to create a FontRequest
.
As mentioned, Compose 1.2 fixes a number of bugs and implements many features requested by the community. For example, it now allows to disable scrolling of lazy layouts, unifies behaviour of TextField
and EditText
back button, ensures Compose animations honor the animation setting in developer options, and more.
As a final note, it is worth mentioning that updating Compose 1.2 requires Kotlin 1.7.0.
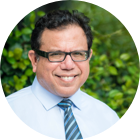
MMS • Todd Montgomery
Article originally posted on InfoQ. Visit InfoQ
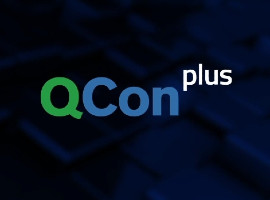
Transcript
Montgomery: I’m Todd Montgomery. This is unblocked by design. I’ve been around doing network protocols and networking for a very long time, exceedingly long, since the early ’90s. Most of my work recently has been involved in the trading community in exchanges, and brokerages, trading firms, things like that, so in finance. In general, I’m more of a high-performance type of person. I tend to look at systems that have to, because of their SLAs, be incredibly performant. That’s not all, other things as well.
Outline
What we’re going to talk about is synchronous and asynchronous designs. The idea of having sequential operation and how that impacts things like performance, and things like that, and some, hopefully have a few takeaways of things, if you’re looking to improve performance in this category, things you can do. We’ll talk about the illusion of sequentiality. All of our systems provide this illusion of the sequential nature of how they work. I think it all boils down to exactly what do you do while waiting, and hopefully have some takeaways.
Wording
First, a little bit about the wording here. When we talk about sequential or synchronous or blocking, we’re talking about the idea that you do some operation. You cannot continue to do things until something has finished or things like that. This is more exaggerated when you go across an asynchronous binary boundary. It could be a network. It could be sending data from one thread to another thread, or a number of different things. A lot of these things make it more obvious, as opposed to asynchronous or non-blocking types of designs where you do something and then you go off and do something else. Then you come back and can process the result or the response, or something like that.
What Is Sync?
I’ll just use as an example throughout this, because it’s easy to talk about, the idea of a request and a response. With sync or synchronous, you would send a request, there’ll be some processing of it. Optionally, you might have a response. Even if the response is simply just to acknowledge that it has completed. It doesn’t always have to involve having a response, but there might be some blocking operation that happens until it is completed. A normal function call is normally like this. If it’s sequential operation, and there’s not really anything else to do at that time, that’s perfectly fine. If there are other things that need to be done now, or it needs to be done on something else, that’s a lost opportunity.
What Is Async?
Async is more about the idea of initiating an operation, having some processing of it, and you’re waiting then for a response. This could be across threads, cores, nodes, storage, all kinds of different things where there is this opportunity to do things while you’re waiting for the next step, or that to complete or something like that. The idea of async is really, what do you do while waiting? It’s a very big part of this. Just as an aside, when we talk about event driven, we’re talking about actually the idea of on the processing side, you will see a request come in. We’ll denote that as OnRequest. On the requesting side, when a response comes in, you would have OnResponse, or OnComplete, or something like that. We’ll use these terms a couple times throughout this.
Illusion of Sequentiality
All of our systems provide this illusion of sequentiality, this program order of operation that we really hang our hat on as developers. We look at this and we can simplify our lives by this illusion, but be prepared, it is an illusion. That’s because a compiler can reorder, runtimes can reorder, CPUs can reorder. Everything is happening in parallel, not just concurrently, but in parallel on all different parts of a system, operating systems as well as other things. It may not be the fastest way to just do step one, step two, step three. It may be faster to do steps one and two at the same time or to do step two before one because of other things that can be optimized. By imposing order on that we can make some assumptions about the state of things as we move along. Ordering has to be imposed. This is done by things in the CPU such as the load/store buffers, providing you with this ability to go ahead and store things to memory, or to load them asynchronously. Our CPUs are all asynchronous.
Storages are exactly the same way, different levels of caching give us this ability for multiple things to be optimized along that path. OSs with virtual memory and caches do the same thing. Even our libraries do this with the ideas of promises and futures. The key is to wait. All of this provides us with this illusion that it’s ok to wait. It can be, but that can also have a price, because the operating system can de-schedule. When you’re waiting for something, and you’re not doing any other work, the operating system is going to take your time slice. It’s also lost opportunity to do work that is not reliant on what you’re waiting for. In some application, that’s perfectly fine, in others it’s not. By having locks and signaling in that path, they do not come for free, they do impose some constraints.
Locks and Signaling
Let’s talk a little bit about that. Locks and signaling introduce serialization into a speed-up. If you look at Amdahl’s law, what it’s really saying is, the amount of serialization that you have in your system is going to dictate how much speed-up you get by throwing machines or processors at it. As you can tell from the graph, if you’re not familiar with Amdahl’s law, which I hope you would be, but it does, it limits your scaling, and even just a simple thing such as 5% serialization within a process. That’s a small percent compared to most systems, can reduce that scaling dramatically, so that you don’t gain much as you keep throwing processors at it and scaling.
That’s only part of the issue. It also introduces a coherence penalty. If you want to see a coherence penalty in action, think of a meeting where you have five people in it, and how hard it is to get everyone to agree and understand each other, and make sure that everyone knows what is being talked about. This is coherence. It is a penalty that is attached to getting every entity on the same page and understanding everything. When you add in a coherence penalty and do something like that, it turns out that Amdahl was an optimist. That it actually starts to decrease the speed-up that you get, because the coherence penalty starts to add up, so that becomes a dominant factor, in fact. It’s not simply that you have to reduce the amount of serialization, but you also have to realize that there’s a coherence. Locks and signaling have a lot of coherence, and so this limits scaling. One thing to realize is that by adding locks and having signaling, you are, in effect, limiting your scaling to some degree. It goes even further than that. More threads, more contention, more coherence, less efficient operation. This isn’t always the case, but it often is.
Synchronous Requests and Responses
There is actually more to think about. The reason why I’m going through a lot of this is so that you have some background in terms of thinking about this from a slightly different perspective. I’ve had a lot of time to think about it, and as systems that I’ve worked on, I’ve distilled down some things. I always have to set the stage by saying here’s some of the things that limit and here’s how bad it is, but there are things we can do. Let’s take a look here. First, the synchronous requests and responses. You have three different requests. You send one, you wait for the response, you send another, and you send a third, and you wait for the response. That may be how your logic has to work. Just realize the throughput of how many requests you can do is limited by that round-trip time. Not by the processing, it’s limited by how fast you can actually send a request and get a response.
If you want to take a look at how our technology has grown, response time in systems does not get faster very quickly. In fact, we’ve very much stagnated on that response. You can take a look at clock speed, for example, in CPUs. If you look at network bandwidth, storage capacity, memory capacity, and somewhat the CPU cores, although that hasn’t grown as much, as the accumulated improvements have grown over time, they’ve grown more than improvements in response time, for example. From a throughput perspective, we are limited. If you take a look at it from a networking perspective, and look at it through throughput, just in trying to get data across, this stop and wait operation of sending a piece of data, waiting for a response, sending another piece of data, waiting for a response, is limited by the round-trip time. You can definitely calculate it. You take the length of your data, you divide it by the round-trip time. That’s it. That’s as fast as you’re going to go. Notice that you can only increase the data length, or you can decrease the round-trip time. That’s it. You have nothing else to play with.
You’d rather have something which was a little bit faster. This is a good example, a fire hydrant. The fire hydrant has a certain diameter that has a relationship to how much water it can push out, as opposed to a garden hose. Our networks are exactly the same thing. It doesn’t matter if it’s network. It doesn’t matter if it’s the bandwidth on a single chip between cores, all of them have the same thing, which is the bandwidth delay product. The bandwidth is how much you can put in at a point of time. That’s how big that pipe is. The delay is how long that pipe is. In other words, the time it takes to traverse. The bandwidth and delay product is the amount of bytes that can be in transit at one time. Notice, you have a couple different things to play with here. To maximize that you have to not only have a quick request-response, request-response, but you also have to have multiple pieces of data outstanding at a time, that N right there. How big is N? It’s a whole different conversation we can have, and there’s some good stuff in there. Just realize that you’d want N to be more than just 1. When it’s 1, you’re waiting on round trips.
More Requests
The key here is while something is processing or you’re waiting, is to do something, and that’s one of the takeaways I want you to think of. It’s a lost opportunity. What can you do while waiting and make that more efficient? The short answer is, while waiting, do other work. Having the ability to actually do other stuff is great. The first thing is sending more requests, as we saw. The sequence here is, how do you distinguish between the requests? The relationship here is you have to correlate them. You have to be able to basically identify each individual request and individual response. That correlation gives rise to having things which are a little bit more interesting. The ordering of them starts to become very relevant. You need to figure out things like how to handle things that are not in order. You can reorder them. You’re just really looking at the relationship between a request and a response and matching them up. It can be reordered in any way you want, to make things simple. It does provide an interesting question of, what happens if you get something that you can’t make sense of. Is it invalid? Do you drop it? Do you ignore it? In this case, you’ve sent request 0, and you’ve got a response for 1. In this point, you’re not sure exactly what the response for 1 is. That’s handling the unexpected.
When you introduce async into a system where you’re doing things and you’re going off and doing other stuff, you have to figure out how to handle the unexpected because that’s what actually makes a lot of things like network protocols. How you handle them is very important. There’s lots of things we can talk about here. I want to just mention that errors are events. There’s no real difference. An event, can be a success, it can also be an error. You should think about errors and handling the unexpected as if they were events that just crop up in your system.
Units of Work
The second thing to think about is the unit of work. When we think about this from a normal threads perspective, we’re just doing sequential processing of data, we’re doing work, and it’s between the system calls that we do work. If you take that same example I talked about, like a request, and then a response, if you think about it from getting a request in, doing some work, and then sending a response, it’s really the work done between system calls. System call to receive data. System call to send data. The time between these system calls may have a high variance. On the server side, this isn’t so that complicated. When you start to think about it from the other side, where it’s, I do some work, I then wait. Then I get a response. Now it’s highly varying in terms of the time between them, which may or may not be a concern, but it is something to realize.
Async Duty Cycle
When you turn it around and you say something like from an asynchronous perspective, the first thing you should think about is, ok, what is the work that I can do between these? Now it’s not simply just between system calls. It’s easier to think about this as a duty cycle. In other words, a single cycle of work. That should be your first class concern. I think the easiest way to think about any of this is to look at an example in pseudocode. This is an async duty cycle. This looks like a lot of the duty cycles that I have written, and I’ve seen written and helped write, which is, you’re basically sitting in a loop while you’re running. You usually have some mechanism to terminate it. You usually poll inputs. By polling, I definitely mean going to see if there’s anything to do, and if not, you simply return and go to the next step. You poll if there’s input. You check timeouts. You process pending actions. The more complicated work is less in the polling of the inputs and handling them, it’s more in the checking for timeouts, processing pending actions, those types of things. Those are a little bit more complex. Then at the end, you might idle waiting for something to do. Or you might just say, ok, I’m going to sleep for a millisecond, and you come right back. You do have a little bit of flexibility here in terms of idling, waiting for something to do.
Making Progress
The key here is, you should always think about it as, your units of work should always be making progress towards your goal. Once you break things down, which is where all the complexity comes into play, you start to realize that the idea of making progress and thinking about things as steps like you would normally do in just listing out logic is the same. The difference here is that you have to think about it as more discrete, as opposed to just being wrapped up. To give an example of this, I’ve taken just a very simple example. Let’s say you’re on a server, and you get a request in. The first step you need to do is to validate a user. Then if that’s successful, you then process the request. If it’s not successful, then you would send an error back. Then once you’re done, you send a response. That response may be something as simple as, ok, I processed your request. It could be that you generate a response. If you turn that into the asynchronous side, you can think about the requests as being an event, like OnRequest. The next thing you would do is you would request that validate. I’ve made this deliberately a little bit more complicated. The validating of the user in a lot of sequential logic is another blocking operation. That’s the actual operation we want to look at from an asynchronous perspective.
Let’s say that we have to request that validation externally. You have to send away for it to be validated and come back. This might be from a Secure Enclave, or it could be from another library, or it could be something else. It could be from a totally separate system. The key is that you have to wait at that point. You go off and you process other things. Other requests that don’t depend on this step can be processed, other pending actions. There could be additional input for other requests, more requests, other OnRequests that come in. At some point, you’re going to get a response from that validation: it might be positive, it might be negative. Let’s assume that it’s positive here for a moment. You would then process the request at that point. That could spawn other stuff and send the response. What I wanted to point out here is, that’s the lost opportunity. If you simply just did get a request, validate user, and then you just go to sleep, that’s less efficient. It’s lost opportunity. You want to see how you would break it down. That’s where having a duty cycle comes into play. That’s where that duty cycle helps you to basically look at this and to do other stuff, and so breaking it down into states and steps. At the first time, you actually had an implicit set in the sequential version on the left, of states that something went through, like request received, request being validated, request validated ok, processing request, sending a response. Those states are now explicit in a lot of cases on the right. Think about it from that perspective. You’ve got those states, it’s just how you’ve managed it.
Retry and Errors as Events
One of the more complicated things to handle here is the idea of, ok, that didn’t work and I have to try it again. Retrying logic is one of the things that makes some of these asynchronous duty cycles much more complicated. Things like transient errors, backpressure, and load are just some of the things that you might look at as transient conditions that you then can try again. If we look at that now from a little bit different perspective, we take and expand this a little bit. On a request, request to validate. You wait, and it’s ok. You process a request, you send a response. That’s the happy path. The not so happy path is not the case where you get an error on the validate, you say, no, can’t do that. It’s where you get an error that basically says, ok, retry.
This does not add that much complexity if you’re tracking it as a set of state changes, because you would get the request. If we look at this, we’ll see this on the right there, you will request to validate, you wait. The same as before. On validate, if the OnValidateError indicates that it’s not a permanent error, like somebody put in bad credentials, let’s say, that the system for validation was overloaded, please wait and retry. You would wait some period of time. That is not any more complex than waiting for that response. You’re simply just waiting for a timeout. The key here is that you would then request validate again. You can add things like a number of retries, and things like that. It doesn’t really make things more complicated. Something may hide just underneath of you for the sequential case, but it’s just lost opportunity. This is what I mean by making progress. This is making progress at some form every step of the way. Again, one size does not fit all. I don’t want to get you to think that one size fits all here. It does not.
Takeaways
Takeaways here are the opportunity when you’re waiting for something external to happen, or things like that. If you think about it from an asynchronous perspective, we may think that a lot of times it’s complicated, but it’s not. It’s, what do you do when waiting? Sometimes it’s easy a question to answer, and leads us down interesting paths. Sometimes it doesn’t. Not all systems need to be async, but there’s a lot of systems that could really benefit from being asynchronous and thinking about it that way.
Questions and Answers
Printezis: You did talk about the duty cycle and how you would write it. In reality, how much a developer would actually write that, but instead use a framework that will do most of the work for them?
Montgomery: I think most of the time, developers use frameworks and patterns that are already in existence, they don’t do the duty cycle. I think that’s perfectly fine. I think that also makes it so that it’s easy to miss the idea of what a unit of work is. To tie that to one of the questions that was asked about actor model, reactive programming, patterns and antipatterns, what I’ve seen repeatedly is, when using any of those, the idea of a unit of work is lost. What creeps in is the fact that in that unit of work, now you have additional, basically blocking operations. Validation is one that I used here, because I’ve seen multiple times where the idea of, I got a piece of work in. The first thing I’m going to do is go and block waiting for a validation externally, but I’m using the actor model in this framework. It’s efficient, but I can’t figure out why it’s slow. I think the frameworks do a really good job of providing a good model, but you still have to have that concern about, what is the unit of work? Does that unit of work have additional steps that can be broken down in that framework? There’s nothing wrong with using those frameworks, but to get the most out of them, you still have to come back to the idea of, what is this unit of work? Break it down further. It’s hard. None of this is easy. I just want to get that across. I’m not trying to wave my hand and say this all is easy, or we should look at it, be more diligent or rigorous. It’s difficult. Programming is difficult in general. This just makes it a little bit harder.
Printezis: I agree. At Twitter, we use Finagle, which is an asynchronous RPC that most of our services use to communicate. Sometimes the Finagle team have to go and very carefully tell other developers, you should not really do blocking calls inside the critical parts of Finagle. That’s not the point. You schedule them using Finagle, you don’t block. Because if you block all the Finagle threads, that’s not a good idea. We haven’t eliminated most of those. None of this stuff is easy.
Any recommendations out of this actor model, patterns, antipatterns, would you like to elaborate more?
Montgomery: I am a fan of the actor model. Again, if you look at me, the systems that I have out in open source and have worked on, using queues a lot, using the idea of communication, and then having processing that is done. I don’t want to say it’s the actor model. I think that model is easier, at least for me to think about. That might be because of my background with protocols and packets on the wire, and units of work are baked into that a lot. I have very much an affinity for things that make the concept of a unit of work, already to be something that is very front and center. The actor model does that. Having said that, things like the reactive programming, especially with the RX style, I think have a lot of benefit from the composition side. I always encourage people to look at that, whether it makes sense to them or not, as you have to look at various things and see what works for you. I think reactive programming has a lot. That’s why I was involved in things like RSocket, reactive socket, and stuff like that. I think that those have a lot of very good things in them.
Beyond that, I mean, patterns and antipatterns, I think, learning queuing theory, which may sound intimidating, but it’s not. Most of it is fairly easy to absorb at a high enough level that you can see far enough to help systems. It is one of those things that I think pays for itself. Just like learning basic data structures, we should teach a little bit more about queuing theory and things behind it. Getting an intuition for how queues work and some of the theory behind them goes a huge way, when looking at real life systems. At least it has for me, but I do encourage people to look at that. Beyond that, technologies frameworks, I think by spending your time more looking at what is behind a framework. In other words, the concepts, you do much better than just looking at how to use a framework. That may be front and center, because that’s what you want to do, but go deeper. Go deeper into, what is it built on? Why does it work this way? Why doesn’t it work this other way? Asking those questions, I think you’ll learn a tremendous amount.
Printezis: The networking part of the industry has solved these with TCP, UDP, HTTP/3, what has prevented us from solving this in an industry-wide manner at an application level? How much time do we have?
Montgomery: The way that I think of it, because I’m coming from that. I spent so much time early on in my career learning protocols and learning how protocols were designed, and designing protocols. From my perspective, it is a lesson I learned early. It had a big influence on me. When I look back, and why haven’t we applied a lot of that to applications, it’s because just like CPUs provide you with program order, and compilers reorder, but with the idea that none of your critical path that you look through in your program in your mind is still going to function step one, step two, step three. By giving this illusion of sequentiality that we can base our mental models on, it’s given us the idea that it’s ok to just not be concerned about it. While at the networking level, you don’t have any way to not be concerned about it, especially if you want to make it efficient. I think as things like performance become a little bit more important because of in effect climate change. We’re starting to see that performance is something that people take into consideration for other reasons than just the trading community, for example. We’ll start to see some of this revisited, because there’s good lessons, they just need to be brought into more of the application space. At least that’s my thought.
Printezis: Any preference for an actor model framework, Erlang, Elixir, or Akka, something else?
Montgomery: Personally, I actually like Erlang and Elixir from the standpoint of the mental model. Some of that has to do with the fact that as I was learning Erlang, I got to talk to Joe Armstrong, and got to really sit down and have some good conversations with him. It was not surprising to me. After reading his dissertation, and a lot of the other work, it was something that was clearly so close to where I came from, from the networking perspective, and everything else. There was so much good that was there that I find, when I get to use some Erlang. I haven’t actually used Elixir any more than just playing around with it, but Erlang, I’ve written a few things in, especially recently. I really do like the idioms of Erlang. From an aesthetic perspective, and I know it’s odd, I do prefer that.
Akka is something I’m also familiar with, but I haven’t used it in any bigger system. I’ve used Go and Rust and a few others that have pieces of the same things. I think it is really nice to see those. It’s very much more of a personal choice. The Erlang or Elixir thing is simply just something that I’ve had the opportunity to use heavily off and on, last several years, and really do like, but it’s not for everyone. I think that’s just a personal preference. I think, keeping an open mind, trying things out on your own, is very valuable. If possible, I suggest looking at what speaks to you. Whenever you use a framework or a language, there’s always that thing of, this works but it’s a little clunky. Then, this isn’t great. Everything is pretty much bad all over. It doesn’t matter. I find if you like something you haven’t probably used it enough. For me, I do encourage people to take a look at Erlang, but that doesn’t necessarily mean that you should do that and avoid other stuff. You should try everything out, see what speaks to you.
Printezis: I’ve always been fascinated by Erlang. I don’t want to spend more time on this. I’ve always been fascinated because I’m a garbage collection person, and it has a very interesting memory management model. The fact that thread-local GC in the language, basically, the language assures it, the way it structures the objects. That’s been fascinating for me.
Project Loom basically is supposed to introduce fibers in Java, which are very lightweight threads. The idea is that you can run thousands of them, and they’re not going to fill up your memory, because not all over we’re going to have a full stack. Any thoughts on Java fibers? The idea is that they’re very lightweight, and then you can run thousands of them, and then you get the best values, but if one of them starts doing some synchronous I/O, another one will be scheduled very quickly. Any thoughts on that?
Montgomery: Yes, I do. I’m hopeful. I’ve been down this road a couple times where the idea of let’s just have lighter weight threads has come up a few times. What tends to happen is we think, this is hidden from me and so I won’t take care of it, or I won’t think about it until it becomes an issue. I don’t think that’s really where we should spend some of that time. I don’t see it as a panacea, and then all of a sudden the coherence penalty and the serialization will go away, which are inherent in a lot of those designs. It would be very interesting to see how this gets applied to some systems that I’ve seen. I’ve seen some systems with 600 threads running on 2 cores, and they’re just painful. It’s not because of the application design, except for the fact that they’re just interfering with one another. Lightweight threads don’t help that. They can make it worse. It’ll be interesting to see how things go. I’m holding my breath, in essence, to see how that stuff comes out.
Some of the things that have come out of Project Loom that have impacted the JVM are great, though, because there are certain things that I and others have looked at for many years and thought, this should just be better, because this is clearly just bad, looking at them. They have improved a number of those things. I think that’s great. That’s awesome. I’m not exactly sold on the direction.
Printezis: I’m also fascinated to see where it’s going to find usage and where it’s going to improve things.
One of the most challenging aspects of doing something like an asynchronous design where you send requests, and then you get them later, is actually error reporting and error tracking. If you have a linear piece of code, you know like, ok, it failed here, so I know what’s going on. If you have an exception in the middle of this request, sometimes it’s challenging to associate it with what was going on. Any thoughts on that?
Montgomery: A lot of code that I’ve seen that has a big block and a try and a catch, and then it has like I/O exception, and there’s a whole bunch of I/O that happen, some of the sequential logic has the same problem. I think, in my mind, it’s context. It’s really, what was the operation? If it’s an event that comes in, you can handle it just like an event. You might think about state change. I think that is an easier way to deal with some exceptions in big blocks as well, is to break it down, and to look at it in a different way. In my mind, I think that it makes sense to think of them as events, which is something I’ve harped on for a number of years now. When you look at systems, you should think of them as errors should be higher level and handled a little bit better in context. It doesn’t mean you handle them locally, it means you handle them with the context that they share. It is hard. One of the things that does make them a little bit easier, in my mind, are things like RX and other patterns that an error happens as an event that you can deal with slightly separately, which forces you to have a little bit more context for them.
See more presentations with transcripts
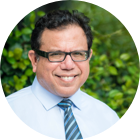
MMS • Harry Zhang Ramya Krishnan Ashley Kasim
Article originally posted on InfoQ. Visit InfoQ
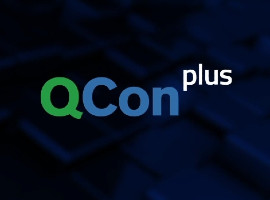
Transcript
Watson: I’m Coburn Watson. I’m Head of Infrastructure and Site Reliability at Pinterest. I’m the track host for the cloud operating model. Each of the panelists has experienced running Kubernetes large scale on the cloud.
We have three panelists from three companies. We have Ashley Kasim from Lyft. We have Ramya Krishnan from Airbnb. We have Harry Zhang from Pinterest. Obviously, you’ve had a journey at your company, and your career to Kubernetes running at large scale on the cloud. If you could make a phone call back to your former self when you started that journey, and say, “You should really take this into account, and it’s going to make your trip there easier.” What would be the one piece of advice you’d give your former self. Feel free to take a little time and introduce yourself as well and talk about what you do at your company.
Kasim: I’m Ashley. I’m the Tech Lead of the compute team at Lyft. Compute at Lyft is basically everything from Kubernetes in an operator webhook, all the things that run on Kubernetes wire, all the way down to AWS and Kernel. It’s a space that I’m involved with. Looking back, what started as a Kubernetes journey back in I think circa 2018, with the planning starting in 2017. I think that the one thing to consider carefully is this huge transition for us it was from just like Amazon EC2 VM based to containerizing and move to Kubernetes. Just to think about when you have orchestrating infrastructure, it’s different than building this large deployment of Kubernetes from scratch. Think carefully about like what legacy concepts, or other parts of unrelated infrastructure too that you’re planning on bridging, what you’re planning on reimplementing, fork better in Kubernetes, and what you’re going to punt down the line. Because, spoiler alert, the things that get punted, it sticks with you. Then also, like when you decided that, we’re just going to adapt something, you can quickly get to this realm of diminishing returns, where you’re just spending too much time on this adaptation. I think how you build that bridge is as important as the end state of the infrastructure that you’re building.
Krishnan: I’m Ramya. I’m an SRE with Airbnb. I’ve been with Airbnb for about four years. We started our Kubernetes journey about three years ago. Before that, we were just launching instances straight up on EC2. I was back then managing internal tools that just launch instances. Then about three years ago, we started migrating everything into Kubernetes. What would I say to my past self? Initially, we put everything in a single cluster the first one year, then we started thinking about multi-clusters. We were a little late in that, so think that you are going to launch about 10 clusters and then automate everything that you’re going to do for launching an instance. Terraform, or any other infrastructure as code tool, use them efficiently. Do not do manual launches and use ad hoc startup scripts because they are going to break. Think about how you’re going to split deployments across availability zones and clusters. Customers are going to be ok about splitting deployments across three availability zones. These are the two things I would tell myself about three years ago.
Zhang: I’m Harry Zhang from Pinterest. Currently, I am the Tech Lead for the cloud runtime team. My team builds a compute platform leveraging Kubernetes, which serves a variety of workloads across Pinterest, different organizations. My team solves problems related to container orchestration, resource management, scheduling, infrastructure management and automation, and of course, compute related APIs, and problems related in this area. I’m personally a Kubernetes contributor. Prior to joining Pinterest, I worked at LinkedIn data infrastructure and focused on cluster management as well.
Pinterest started the Kubernetes journey also about three years ago in 2018. If I have something to tell my past self about it, I would say that Kubernetes is a very powerful container orchestration framework. Power really means opportunities to you, as well as responsibility to you as well. Letting things grow wildly may lead to a fast start, but will slow you down very soon. Instead, I would suggest to my past self to take an extra step to really think carefully about how you architect your system. What are the interfaces? What are the capabilities you want to provide within the systems and to the rest of your businesses? What is the bridge you’re going to be building between your business and the cloud provider? If you use Kubernetes wisely, you’re going to be surprised to see how significant a value it can bring to your businesses.
How to Manage Multi-Cluster Deployment and Disaster Recovery
Watson: How do you manage multi-cluster deployment and disaster recovery?
Kasim: On the whole, multi-cluster somewhat gets into like cluster layout as well. For us, for our main clusters, which are like all the stateless services, we have them like one cluster per AZ, and they’re supposed to be entirely independent and redundant. The theory is that we should be able to lose one AZ, and everything will be fine. We use Envoy as a service mesh so that customers just drop out of the mesh, and the other clusters will scale up to pick up that slack. We haven’t really experienced AZ outage instances, but we have in staging had bad deployments or something that go to a single staging cluster and take it out. We’ve found that because we have these independent and redundant clusters, we can just basically go so far as to like blow away the cluster that gets broken or whatever, and just re-bootstrap it. I think keeping your failure domain very simple, logically has worked well for us, and having this redundancy so that you can afford to lose one.
Zhang: I can share some stories. We run multi-cluster architecture in Pinterest as well. Starting in 2020, we also get into those single cluster scaling problems, so we started a multi-cluster architecture with automated cluster federation. Just like what Ashley talked about, we also do single zonal clusters, and we have zonal clusters, and we have our regional API entry point for people to launch their workloads. The Zonal cluster brings very good and very easy ways for people to isolate the failures, and the blast radius to zonal or sub-zonal domain. We have zonal clusters and one or more clusters in each zone. Our federation control plane is going to be taking the incoming workloads and split them out into different zones, and to take the zonal health, zonal load into its smart scheduling decisions. If a zone goes into a crappy state or something, we have human operational or automated ways to cordon the entire zone and to load balance the traffic to the others and healthy zones. We do see a very big of a value for the cross-zone multi-cluster architecture that we can bring to the platform with a better scalability and easier operations, and many more.
Krishnan: Right now we don’t do zonal deployments. That’s something that we’re going to strive next quarter.
How to Split Kubernetes Clusters
How do you split the Kubernetes clusters? We don’t split it by team. When a particular cluster reaches a particular number for us, we’ve picked an arbitrary number of 1000 nodes, we mark the cluster as full and not available for new scheduling, new deployments. We stamp out Terraform changes to create a new cluster. It’s not split by team, the cost attribution happens by CPU utilization and other stuff and not by the cluster. Cost attribution does not happen at the cluster level. That way the teams are split across multiple clusters.
Watson: Do you have anything else you want to add on that one of how you break apart Kube Cluster workloads?
Kasim: Let’s tease it a bit differently, where it’s not like we have this cutoff and then on to the next, we instead roughly chunk out clusters based on their intended purpose. For stateless services, and it’s all around like interruptibility, since we find that that is like, how well does a Kubernetes delimiter? We have stateless services on our redundant core clusters that are per AZ, and each one of them is a replica of each other that basically deploys to five targets instead of one. Then we have a stateful cluster, which is a little bit more high touch because it’s for stateful services that don’t Kubernetes very well. Then we have dedicated clusters for some of the ML or the ETL long running jobs stuff, so it’s kind of different interruption policies. We just found that the thing that we split on is just like interruptibility, which works well for batching things into different maintenance schedules.
Zhang: In Pinterest, we provide Kubernetes related environment in multi-tenanted setups. We run them mixed off the long running and stateless workloads, and also batch workloads, such as workflow and pipeline executions, machine learning, batch processing, and distributed training and all the things to that. We treat the Kubernetes environment as an entire we call federated environment, which is a regional entry point for the workload submissions and executions, including new workload launches, and workloads updates. We totally hide the details of the zonal clusters, which we call the member clusters for executing the workloads away from our user. We’ve built a layer of federation sitting on top of all the Kubernetes clusters that is responsible for workload executions, and there’s smart scheduling decisions and dispatching logics and updates to the workload executions. Also, of course, the zonal cluster workload execution statuses will be aggregated back to our regional API endpoint, and people can know how their workloads are executed, or what are the status they have from the compute perspective?
Spot and Preemptible Workloads
Watson: I know internally at Pinterest, we’ve dealt with this. At a previous company I did where basis, let’s make it efficient, use Spot. I know capacity management is one of the challenges with Kubernetes. Let’s say you’re on Amazon, you basically become the EC2 team. Everybody wants it when they want it. I’m interested to answer that question particularly because efficiency is always a concern. Does anybody have any experience with running using either Spot or preemptible workloads internally?
Kasim: Spot has been something that we’ve been very interested in. Currently, pretty much most of staging, it’s all Spot because interruption was a concern. This is again, something that works very well for stateless service and doesn’t work so well for stateful services, or like long running jobs. One of the things we’ve looked at is with interruption of batch using like the minimum lifetime, where you guarantee the runtime of like one hour or something. Then just using annotations to run lower priority jobs or jobs that we expect to be done in an hour on those things. I think it’s less of a limitation of Kubernetes for Spot, but more like, what do your workloads look like? In general, Kubernetes favors interruptibility, so the more interruptible you can make things, and using things like checkpointing, the more Spot friendly your clusters will be.
Krishnan: We are also trying to introduce Spot for staging and dev clusters. We’ll probably launch that by the end of this year.
Zhang: In Pinterest, we also have a great interest about the Spot. Not only Spot, to me we call it opportunistic compute. We have teams plan their capacities for sure, every once a while, but businesses can grow out of the bound, or people just want to have things to execute it opportunistically. Opportunistic computing to me has two categories. One is those provided from the cloud provider, which is Spot Instances directly. The other part is like all the reserved capacity from the company that is not actually currently being used. Those capacities can be aggregated as a whole pool of resources for those workloads who can afford opportunistic computing, that can tolerate interruptions and retries, such as your dev, your staging workloads, or any other batch processing that is not very high tiered. Currently, we are exploring the possibilities of both categories. We do have some experimental results inside and we try to move forward with those.
Watson: When I was at a previous job at Netflix, and we had a container runtime, Kubernetes was not there yet. We actually had to use the term internal Spot to get people to adopt that concept of, we have hundreds of thousands of instances, 97% are covered under reservations. Don’t worry about it, like launch it, and we’ll make sure that you’re running in some compute area. Because that nondeterministic behavior of Spot became a pain. At Pinterest, we create blended cost rates, so we roll together on-demand and RIs to normalize cost for people, because we find that most individuals can’t even control their on-demand very well on a large shared account.
Service Mesh for Traffic Management
Do you run Istio or another mesh in your clusters for traffic management?
Zhang: In Pinterest, we have our traffic teams who have built very internal mesh systems based on Envoy, we don’t use Istio, but we have our Pinterest specific mesh systems to connect all the traffic.
Krishnan: Here at Airbnb we have our traffic team work with Istio. We are just migrating from our internal legacy traffic service discovery into Istio based mesh system.
Kasim: Envoy was born at Lyft, so we of course use Envoy. We don’t use Istio, we have our own custom control plane. Actually, we had an older control plane before we moved to Kubernetes. That was, for the longest time, probably the most basic thing out there. We actually had to rewrite and redesign it for Kubernetes to keep up with the rate of change of infrastructure in Kubernetes. We use Envoy as a service mesh, and we are very happy with it.
Starting with Mesh from Day One on Kubernetes
Watson: Thumbs up if you think people should start with mesh from day one on Kubernetes regardless of the mesh solution, or it’s too much overhead.
Kasim: It depends on where you are coming from. We started with mesh beforehand, so would have been hard to rip out that stack. Then for us, it’s very important to not run any overlay networks. We needed something to do that [inaudible 00:19:00].
Krishnan: Definitely don’t run overlay network. Setting up Istio and mesh requires a considerable investment from your traffic team. If they are ready to undertake that as you migrate to Kubernetes might be a lot to ask from your traffic team. It depends on how much time and investment bandwidth you have on you.
Zhang: I would echo what Ramya said as well because mesh could be a complicated system, and it really depends on what is the scale of your businesses and how complex your service architecture is. If you only have a very simple web server plus some backends plus some storage, probably like mesh is too much overkill. If you have a lot of microservices and want to communicate with each other, and like Ramya said, your traffic team is able to take the big responsibilities with all the communication and traffic, probably there’s more values in mesh.
Watson: I know at least in Pinterest we have mesh. Like you said, Ramya, if you have a traffic team you can put in the cycles, that’s really important. Given our huge architecture outside of Kubernetes, it’s like trying to replace someone’s skeleton. It’s pretty painful. What I’ve seen people do is there’s a capability of a mesh you get, maybe you use mTLS, and you have secure traffic communication, so you try to find that carrot that out the gate, they get that. Yes, going back to people and saying, in all your spare time, why don’t you adopt mesh on everything? It’s a painful composition.
Kasim: For us, it’s the other way out where we already had that skeleton of the service mesh, and was putting the Kubernetes on top of that.
Managing the Kube Cluster for Multiple Teams
Watson: How do you manage the choice to have Kube cluster for one or multiple teams when you see that a team needs more services than the number of their apps? Does anybody have a perspective on that?
Krishnan: If a team has more services, just split them across clusters. I’m a strong believer of don’t put too many eggs in a single cluster. If a team has too many services, or too many important services, don’t put all your level zero, subzero services in a single cluster.
Zhang: To us, currently for the dedicated cluster use cases, we evaluate very carefully. Because we are putting this investment into a federated environment, we want to build on one single environment that is very horizontally scalable, and easy management. We try to push people onto this big compute platform we built. However, within the compute platforms we do have those different tiers of resources, different tiers of quality of services we provide to our users, so if people really want a level of isolation. When people talk to you about the isolations, we usually ask them, what is the exact thing that you’re really looking for? Are you looking for the runtime level isolations? Are you looking for control plane isolations, or you simply want to get your clusters because you want to control? More clusters and sporadic clusters across the company may bring you extra burdens into supporting them, because Kubernetes provides you with very low level abstractions, and it can be used in very creative or a diverged way. This could be hard for people to support in the end. Currently, in Pinterest, the direction we want to push people is to guide people to the multi-tenanted and the big platform we are using. As we clean up those low hanging fruit and moving forward, I do see the potential that some people really need the level of isolations. Currently, it’s not the case here.
How to Select Node Machines by Spec
Watson: How do you select the node machines by spec? In your clusters, do all the nodes, are they the same machine type? I assume in this case, we’re talking about like EC2 instance type, or so.
Krishnan: All this while, we had a single instance type C5, 9xlarge. Now we are going multi-instance type this second half. Now we have moved to larger instances. Now we have added GPU instances. Now we have added memory instances all in a single cluster. We have Cluster Autoscaler that scales up different autoscaling groups with different node types. It works most of the time.
Zhang: In Pinterest, we also have very diverse instance profiles, but we try to limit the number of instances, which means like user cannot arbitrarily pick the instance types. We do have a variety of different combinations like compute intense, GPU intense, or those that have local SSDs, or different GPU categories. We do have those different instance types. We try to guide our user to a way to think about the resources that, what exactly is the resources you’re using? Because sometimes when we bound the workloads to the instance types, we can suffer from the cloud provider outages, but if you try to get your workloads away from the particular instance types, there are more flexibilities at the platform side for the dynamic scheduling to ensure the availabilities. There are our workloads that wants the level of isolations, or their workload is tuned to particular resource categories or types and they pick their instances, or they tune their workload sizes to exactly that instance type so they get scheduled onto that instance. In Kubernetes, we do manage a variety of instance types, and we leverage those open source tools, like Kubernetes itself can manage those different instance types scheduling, and autoscaler as well to pick whatever instance groups to scale up as well.
Kasim: A limiting factor, I think, [inaudible 00:25:44] is the Cluster Autoscaler. It only allows node pools with homogeneous resources. The way that we get around this, because we do want to hedge against things like AWS availability, and like being a fallback to other instance types in the launch template. Because we just have formal pools that are just sewn together via just like the same labels on them so that the same workloads can schedule there, and they don’t really know where they’re going to end up. This allows us do interesting things that are maybe beyond the scope of what autoscaler is able to do. For example, we were interested in introducing AWS Graviton instances, which is the Arm instances now being supplied by AWS, and Cluster Autoscaler doesn’t handle this whole architecture concept very gracefully. We ended up just using AWS launch templates to have multi-architecture launch templates. Then the autoscaler just doesn’t know the difference, and just like boots nodes to either arch, and so we prefer Arm because the price is better. We can fall back to Intel still if we run out of Arm since demand is high and so we don’t want to get squeezed out of instances.
Watson: Amazon just keeps releasing instances, so it’s something we’ll deal with.
Preferred Kubernetes Platforms
There are many Kubernetes platforms like Rancher, OpenShift, which one do you prefer and what makes them more special when compared to others?
Kasim: We use just like vanilla Kubernetes, that we self-manage ourselves on top of AWS. I know that cloud providers all have their own special offering out there like EKS, GKE. Part of this is historical concern. When Lyft started the Kubernetes journey, EKS was not really as mature as it is now. We weren’t comfortable running production workloads on it. Probably if we’re doing the same decision process today, we might take a closer look at some of the providers. I think the key thing is all about the backplane. If you manage your own, run your own backplane, you have a lot of control, but also a lot of responsibility. Debugging SED is not fun. There are many ways to completely hose your cluster, if you do something, cut SED the wrong way. There is a tradeoff where if you don’t want to deal with operating the backplane, upgrading the backplane, then looking at a managed provider even just for backplanes may make sense. On the other hand, if you need to run a lot of very custom codes, particularly like patching a GET server code, then probably you want to host your own just because you can’t really do that with most providers. A consideration for Lyft in the beginning is that we wanted that debuggability of being able to actually get on to that API server and tailor logs and run commands, that you just weren’t comfortable having to file a ticket with some provider somewhere. That’s just consideration as well.
Watson: In Pinterest, we have a similar journey of evaluating things, and we’re constantly evaluating the question about what layers of abstractions we would like to offload to the cloud providers. For Kubernetes, particularly, the majority of the work we’ve been spending on is to integrate this Kubernetes ecosystem with the rest of the Pinterest. We have our own security. We have our own traffic. We have our own deploy systems. There are a lot of things that we need to integrate, and also metrics and logging, all the things we need to integrate with the existing Pinterest platform. At the end, the overhead of provisioning clusters, as well as operating clusters compared with all the other work is not that significant. Also, like to have our own clusters, we have more control over the low level components. We can quickly turn around with the problem, just like Ashley described before, to provide our engineers with a more confident environment of running their applications. Currently, up to now, we’re still sticking with our own managed Kubernetes clusters.
Krishnan: We have a similar story. We started our Kubernetes journey about three years ago. At that time, EKS was under wraps, and we evaluated it, and it did not meet any of our requirements. Particularly, we wanted to enable beta flags, feature flags, for example, port topology spreaders and a beta flag at 1.17, and we have enabled it in all our clusters. We cannot enable such flags on EKS. We also run to a patched scheduler and patched a API server, we cherry picked bug fixes from future version and patched our current version API server and scheduler, and ran it for a couple of months. We feel that if we just use EKS, we may not be able to look at logs and patch things as we find problems because we cannot do all this. We are little bit hesitant about going to EKS. If we have to reevaluate everything right now we may make a different decision.
Watson: I’ll just double down on what Harry said. We had conversations a few months back with the EKS team, because much like the question of, why are we not using EKS? If you take your current customer, you say, here’s the Amazon Console, go use EKS. They say, where’s my logs? Where’s my dashboards? Where’s my alerts? Where’s my security policies? It’s like, no, that’s our PaaS. That’s about what 80% of our team does is integrate our environment to our container runtime. When we talk to the EKS team, we’re like, how do you guys solve and focus on user debuggability and interaction? They say, here’s a bunch of great third party tools, use these. I think it’s that tough tradeoff of the integration.
Managing Deployment of Workloads on Kubernetes Clusters
How do you manage deployment of workloads on Kubernetes clusters? Do you use Helm, Kube Control, or the Kubernetes API?
Krishnan: We use a little bit of Helm to stand up our Kubernetes clusters itself. For deployments of workloads, customer workloads, we use internal tools that generate YAML files for deployment and replica sets. Then we just apply those during runtime. We just straight up call the API server and apply these YAML files. That’s what we do.
Zhang: In Pinterest, we have a similar way of abstracting the underlying compute platforms away from the rest of the developers. After all, Kubernetes is one of the compute platforms sitting inside of Pinterest that the workloads can deploy to. We have a layer of compute blended APIs sitting on top of Kubernetes, which abstracts all the details away, and our deploy pipelines just call that layers of API to deploy things.
Kasim: Yes, similar at Lyft. Hardly any developers actually touch any Kubernetes YAMLs. There is an escape hatch provided for people who want to do custom or off-the-shelf open source stuff, but for the most part, service owners manage their code. Then there’s this manifest file that has a YAML description of general characteristics of their service. Then that feeds to our deploy system. Our deploy system generates Kubernetes objects based on those characteristics. It applies those to like what clusters it should be deployed to based on if it’s a stateless service, in a Kubernetes cluster. I think that helped smooth our transition as well, where developers didn’t actually have to learn Kubernetes. It just preserved this abstraction for us. I think another way of looking at this is like this is somewhat CRD-like, not necessarily yet. It’s a similar concept where there’s just one layer above all of it that can raise objects for developers to interact with.
StatefulSets for Stateful Services on Kubernetes
Watson: StatefulSets are great for stateful services on K8s, do you find that that’s the case, or is there some other mechanism used to support stateful services?
Kasim: StatefulSets work well for small stateful components, the big problem that we have for developers is if you have StatefulSets that have hundreds of pods in them, it’ll take a day for you to roll your application. Developers using them will be like, “Developer velocity. I can’t be spending a day to roll out a change. Yet, it’s not really safer for me to roll faster, because I can’t handle more than one replica being done at a time.” We’ve looked at a variety of things. There’s been many misuse of StatefulSets, meaning people who just didn’t want things to change ever, but actually were in danger of data loss or something, in case of a replica going down. Just making sure everybody who’s using a StatefulSet really needs to be using a StatefulSet.
Then looking at StatefulSet management strategies like sharding, or looking at third party components. We have some clusters running the cruise controller here, which runs some extensions on the built-in StatefulSet object, like clone sets, and advanced StatefulSets, which just are basically StatefulSets with more deployment strategies. They’re basically breaking down StatefulSets into shards, and these shards can all roll together so that you can roll faster. That has helped address some of the concerns. There’s also a lot of issues there. Bugs with Nitro and EBS, and just volumes failing to mount, which could go smoother and [inaudible 00:36:56], actually ends up taking your node and cordoning it, and then you have to go and uncordon it. Yes, stateful I think is one of the frontiers on Kubernetes, where I think a lot more can be done to make it work really smoothly at scale.
Krishnan: For our stateful there are very little StatefulSets in our infrastructure, Kafka and everything else is still outside of Kubernetes. We are right now trying to move some of our ML infrastructure into StatefulSets, into Kubernetes. The problem is we cannot rotate instances in 14 days. They are very against killing pods and restarting pods. We are still in conversations about how to manage StatefulSets and still do Kubernetes upgrades and instance maintenance. It is a big ask if you’re just starting out.
Zhang: Pinterest does not run StatefulSets on Kubernetes. I was working at a previous company in LinkedIn, I worked in data infra, and my job is to do the cluster management for all the online databases. A couple very big challenges at a high level to upgrade StatefulSet, one is about the churn. How do you gracefully shut down a node? How do you pick the optimal data node started to upgrade them? How do you move the data around? How do you ensure that all the data shards have their masters on? If you wanted to do leadership election and to do a leadership transfer for different replicas of the shard, how do you do that efficiently and gracefully? This would involve a lot of deep integrations with the application’s internal logics to achieve that. Also, just like Ramya said, to update the StatefulSets, we needed to do very smooth and in-place upgrades of the things, and if you put other side cars inside the pod, if you just shut down the pod and bring it up, it will finally converge, but it’s about the warm-up overhead. It’s about the bigger risk that the top-line success rate of all the data systems is going to be having a dip during the upgrade. There are a lot of challenges that’s not very easily resolvable.
See more presentations with transcripts
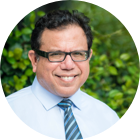
MMS • RSS
Posted on mongodb google news. Visit mongodb google news
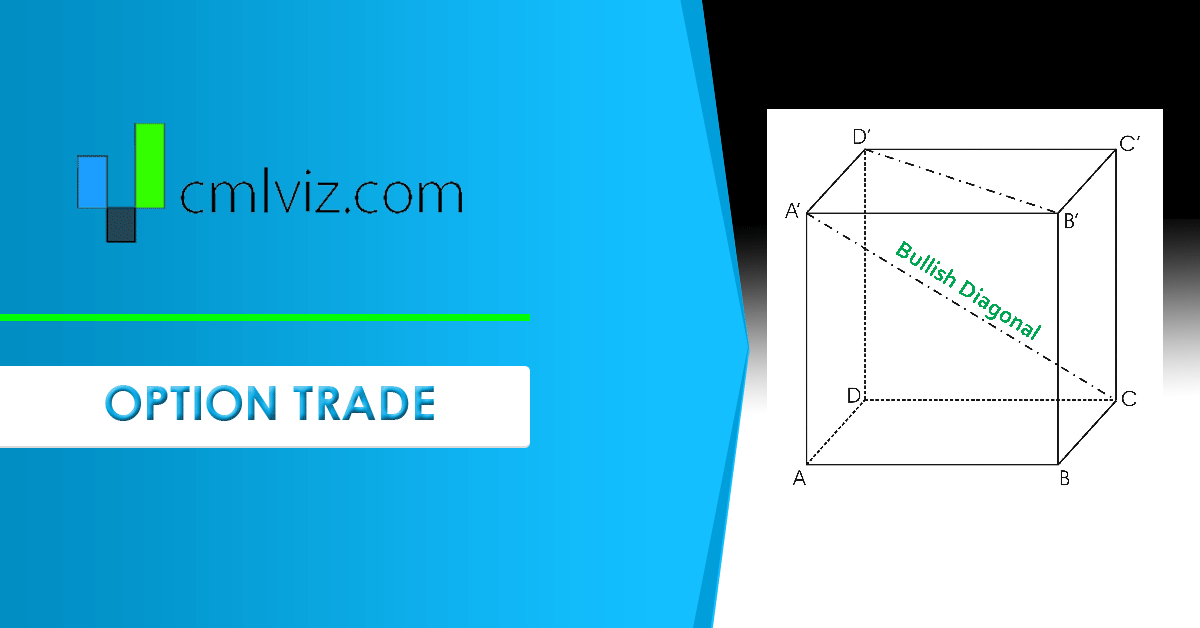
Disclaimer
The results here are provided for general informational purposes from the CMLviz Trade Machine Stock Option Backtester as a convenience to the readers. The materials are not a substitute for obtaining professional advice from a qualified person, firm or corporation.
Article originally posted on mongodb google news. Visit mongodb google news
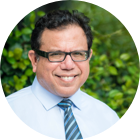
MMS • RSS
Posted on mongodb google news. Visit mongodb google news
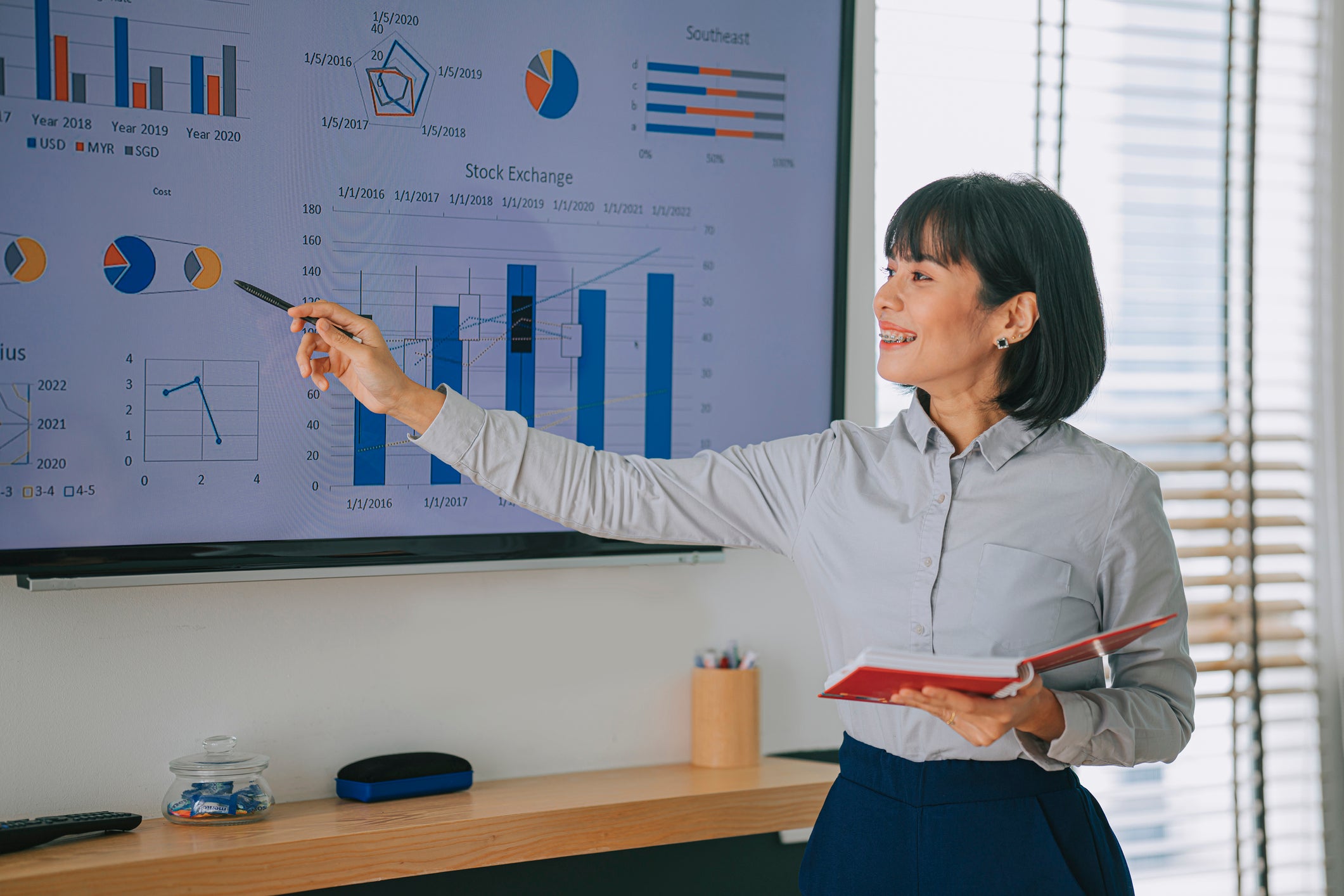
If you think you spend too much time glued to your phone or computer — checking social media feeds, shopping, paying bills, or learning a new skill — you’re not alone. Worldwide, people with internet access, on average, spent about seven hours online every day in 2021.
While growing digitization is bringing the world to our fingertips, it is also producing massive amounts of new data every day. That large amount of data needs to be stored, logically connected, and analyzed in a timely manner so enterprises can make business-critical decisions. Two companies that are playing a pivotal role in helping businesses manage this data are MongoDB (MDB 5.21%) and Snowflake (SNOW 4.63%). Let’s review why investing in these two companies can produce stellar results for long-term investors.
MongoDB: Central to the operation of modern digital enterprises
Every interaction on the internet is enabled behind the scenes by a collection of software applications. And at the heart of those applications is a database like MongoDB that keeps track of real-time data such as consumer profiles, product orders, etc. In a digital-first world, MongoDB plays an essential role for businesses.
MongoDB’s relatively modern “NoSQL” design paradigm — significantly more flexible and scalable relative to the traditional “relational”, or tabular, databases — simplifies the overall app development process, allowing the developers to adopt the ever-evolving requirement of businesses. The bottom line is faster speed and lower cost to develop and enhance software.
MongoDB is also cloud-agnostic: Clients can set up MongoDB databases in a cloud environment of their choice, such as Amazon‘s Amazon Web Services or Alphabet‘s Google Cloud Platform. With MongoDB’s full-service cloud offering, Atlas, the company is eliminating the operational complexity for its clients so they can focus on what’s truly important for their business rather than having to spend time managing databases.
MongoDB has grown in popularity, and its revenue in the recently reported first quarter of fiscal 2023 (ending on April 30, 2022), reached $285.4 million, growing 57% year over year. And that was higher than the past two quarters when revenue jumped 56% and 50%. From fiscal 2017 through fiscal 2022, MongoDB has grown its revenue over sevenfold, from $115 million to $874 million. And the revenue contributed by Atlas — the primary driver of the business at this point — continues to rise at a very impressive rate. Atlas revenue in the recent quarter surged 82% and now makes up 60% of total revenue, which bodes really well for MongoDB.
NoSQL databases are becoming increasingly popular, and MongoDB is a top choice for developers. With growing digitization and the need to effectively manage the increasing amounts of data, IDC estimates the database software market to grow from $85 billion in 2022 to $138 billion in 2026. With management forecasting revenue of about $1.18 billion for fiscal 2023, MongoDB has a significant runway in front of it. It’s probably not an exaggeration to think MongoDB could be a multi-bagger over the long run.
Snowflake: Making businesses smarter with its one-stop-shop data platform
While MongoDB is primarily helping businesses to manage real-time data, Snowflake’s data cloud is predominantly used to assemble large amounts of current and historical data sets from disparate sources into a singular cohesive view to analyze business performance and gain insights to make future decisions. A task that enterprises have historically struggled in a big way to accomplish.
Like MongoDB, Snowflake can be set up in any cloud environment of the customer’s choice. And besides that flexibility, Snowflake is also highly scalable. Customers don’t have to worry about slowdowns in performance when they add more users — the platform automatically assigns more computational resources instantaneously to support the increased workload.
Furthermore, with Snowflake’s usage-based pricing, customers pay only for the database and computational resources they actually use, allowing them to manage costs effectively. Finally, in addition to a large suite of its own data tools, Snowflake’s platform easily integrates with products from over 425 technology companies, so customers can mine, analyze, and visualize data with the tools they’re most comfortable with.
Customers are loving Snowflake’s platform, and it showed in the company’s exceptional net retention rate — the measure of how much existing clients spent over the previous year — of 174%, reported for the first quarter of fiscal 2023 (ending on April 30, 2022). And this wasn’t an unusual occurrence for the company. Over the past four fiscal years, Snowflake has averaged an annual net retention rate of over 168%, which is a testament to its superior product and execution. The company grew its revenue by 84% over a year ago in the recent quarter and has increased revenue by a whopping 132% compound annual growth rate (CAGR) from fiscal 2019 through fiscal 2022. The company is also steadily improving profitability and turned free-cash-flow positive in fiscal 2022.
In a world where enterprises are struggling to make sense of the mountains of data produced every day, Snowflake is uniquely positioned to offer just the right solution. The big demand, a highly coveted product, and outstanding execution set up Snowflake really well for long-term success. For patient investors, now may be a great time to make Snowflake a part of their portfolio.
Suzanne Frey, an executive at Alphabet, is a member of The Motley Fool’s board of directors. John Mackey, CEO of Whole Foods Market, an Amazon subsidiary, is a member of The Motley Fool’s board of directors. Kaustubh Deshmukh (KD) has positions in Alphabet (A shares), Alphabet (C shares), Amazon, MongoDB, and Snowflake Inc. The Motley Fool has positions in and recommends Alphabet (A shares), Alphabet (C shares), Amazon, MongoDB, and Snowflake Inc. The Motley Fool has a disclosure policy.
Article originally posted on mongodb google news. Visit mongodb google news
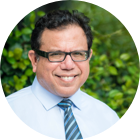
MMS • Steef-Jan Wiggers
Article originally posted on InfoQ. Visit InfoQ
Amazon Detective is a security service in AWS that allows customers to analyze, investigate, and quickly identify the root cause of potential security issues or suspicious activities. Recently, AWS announced the expansion of Amazon Detective towards Kubernetes workloads on Amazon’s Elastic Kubernetes Service (EKS).
The announcement was made during the annual AWS re:Inforce conference, where the company updates the world and its attendees on the developments in cloud security and related topics. The company first introduced the service in March 2020 – a service that continuously looks at things such as login attempts, API calls, and network traffic from Amazon GuardDuty, AWS CloudTrail, and Amazon Virtual Private Cloud (Amazon VPC) Flow Logs.
After its initial release, the company updated the service with features such as AWS IAM Role session analysis, enhanced IP address analytics, Splunk integration, Amazon S3 and DNS finding types, and the support of AWS Organizations. The service’s latest update is a new feature to expand security investigation coverage for Kubernetes workloads running on Amazon EKS.
Channy Yun, a Principal Developer Advocate for AWS, explains in an AWS news blog post:
When you enable this new feature, Amazon Detective automatically starts ingesting EKS audit logs to capture chronological API activity from users, applications, and the control plane in Amazon EKS for clusters, pods, container images, and Kubernetes subjects (Kubernetes users and service accounts).
When potential threats or suspicious activity are found on Amazon EKS clusters, Amazon Detective creates findings and layers them on top of the entity profiles using Amazon GuardDuty Kubernetes Protection. Subsequently, the new Detective feature can help quickly find the answers to queries like which Kubernetes API methods were used by a Kubernetes user account that was detected as compromised, which pods are hosted in an Amazon Elastic Compute Cloud (Amazon EC2) instance that was discovered by Amazon GuardDuty, or which containers were created from a potentially malicious container image.
The support for Kubernetes workloads on Amazon EKS in the Detective service was one of the updates AWS announced at re:Inforce around Cloud Security next others like a new Amazon GuardDuty feature Malware Protection, AWS Wickr, and AWS Marketplace Vendor Insights.
Currently, Amazon Detective for EKS is available in all AWS regions where Amazon Detective is available, and pricing will be based on the volume of audit logs analyzed. Furthermore, there is a free 30-day trial when EKS coverage is enabled, allowing customers to ensure that the capabilities meet their security needs and get an estimate of the service’s monthly cost before committing to paid usage.
Daily Brief TMT/Internet: Advantest Corp, Russell 2000 Index, Softbank Group, Wemade Co …
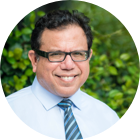
MMS • RSS
Posted on mongodb google news. Visit mongodb google news
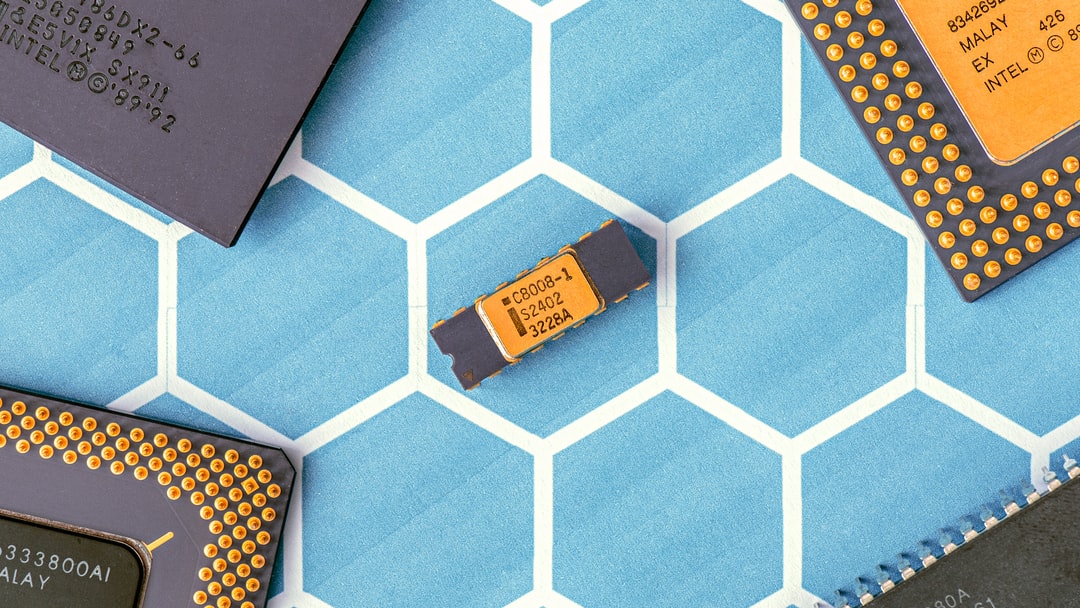
💡 Before it’s here, it’s on Smartkarma
Sign Up for Free
The Smartkarma Preview Pass is your entry to the Independent Investment Research Network
- ✓ Unlimited Research Summaries
- ✓ Personalised Alerts
- ✓ Custom Watchlists
- ✓ Company Data and News
- ✓ Events & Webinars
Article originally posted on mongodb google news. Visit mongodb google news