Month: December 2023
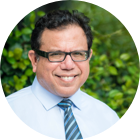
MMS • Renato Losio
Article originally posted on InfoQ. Visit InfoQ
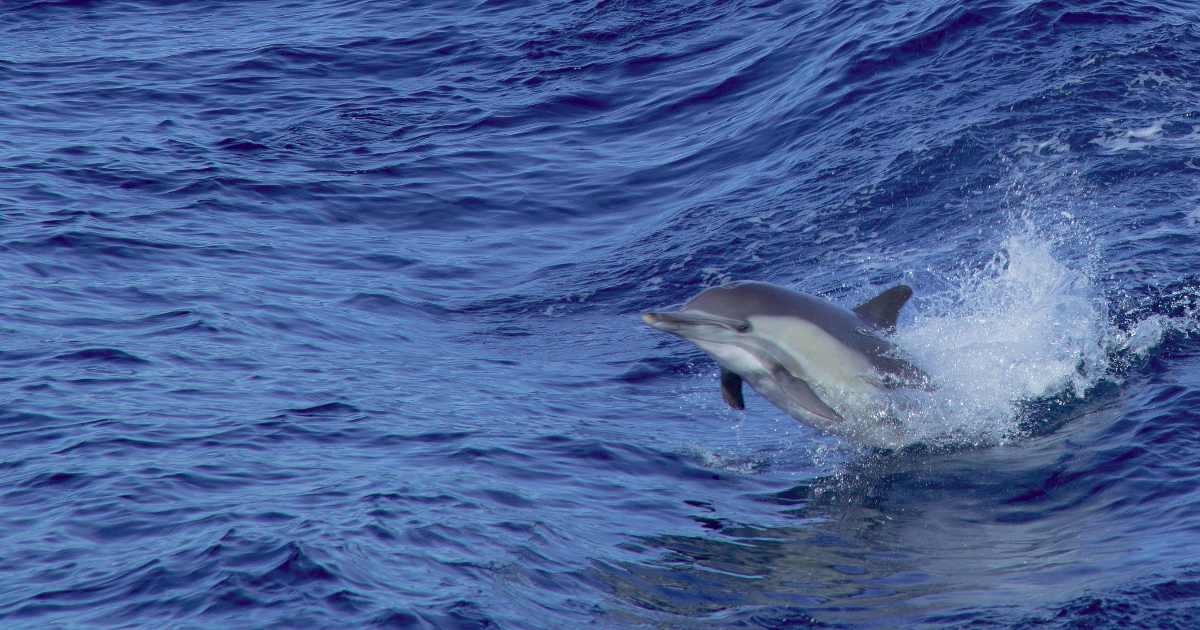
Oracle recently announced that the MySQL database server now supports JavaScript functions and procedures. JavaScript for stored routines is currently in preview and only available in the MySQL Enterprise Edition and MySQL Heatwave.
The introduction of JavaScript support enables developers to implement advanced data processing logic within the database. By minimizing data movement between the database server and client applications, stored functions and procedures can reduce latencies, network overhead, and egress costs. Øystein Grøvlen, senior principal software engineer at Oracle, and Farhan Tauheed, consulting member of technical staff, write:
The support for JavaScript stored programs, will not only improve developer productivity by leveraging the large ecosystem; more developers will now possess the necessary skills to write stored programs. In other words, organizations may now tap into a broader range of developer talent by utilizing the widely accessible JavaScript skill set for backend development.
Among the common use cases for the new feature, Oracle highlights data extraction, data formatting, approximate search, data validation, compression, encoding, and data transformation. Favorably received by the community, the announcement provides an example of a function where the JavaScript code is embedded directly in the SQL definition:
CREATE FUNCTION gcd_js (a INT, b INT) RETURNS INT
LANGUAGE JAVASCRIPT AS $$
let [x, y] = [Math.abs(a), Math.abs(b)];
while(y) [x, y] = [y, x % y];
return x;
$$;
Source: Oracle blog
When the function is invoked using the traditional CALL statement, an implicit type conversion occurs between SQL types and JavaScript types. As per the documentation, JavaScript support is based on the ECMAScript 2021 standard, and all variations of integers, floating point, and CHAR/VARCHAR types are supported. Grøvlen and Tauheed add:
MySQL-JavaScript integration uses a custom-built VM for its specific use case to allow the best end-to-end performance. This customization is based on GraalVM’s ahead-of-time (AOT) compilation where the language implementation is compiled down into a native binary representation for fast processing. GraalVM has its own JavaScript implementation based on the ECMAScript 2021 standard. The language implementation is competitive in terms of performance although it is implemented using GraalVM’s Polyglot framework.
The GraalVM run time includes JDK, language implementations (JavaScript, R, Python, Ruby, and Java), and a managed virtual machine with sandboxing capability and tooling support. While MySQL-JavaScript is available in the MySQL Enterprise Edition and MySQL Heatwave cloud service on OCI, AWS, and Azure, there is no support in the MySQL Community Edition.
MySQL is not the first open-source relational database supporting Javascript in stored routines, with PLV8 being the most popular Javascript language extension for PostgreSQL. PLV8 is supported by all current releases of PostgreSQL, including managed services like Amazon RDS, and can be used for stored routines and triggers.
Oracle has released three MySQL HeatWave videos on YouTube to demonstrate how to run the Mustache library, validate web form inputs, or process web URLs using stored programs in JavaScript.
Amazon Route 53 Resolver Introduces DNS over HTTPS Support for Enhanced Security and Compliance
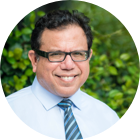
MMS • Steef-Jan Wiggers
Article originally posted on InfoQ. Visit InfoQ
AWS recently announced that Amazon Route 53 Resolver will support using the Domain Name System (DNS) over HTTPS (DoH) protocol for both inbound and outbound Resolver endpoints.
Amazon Route 53 Resolver is a comprehensive set of tools for resolving DNS queries across AWS, the internet, and on-premises networks, ensuring secure control over the DNS of your Amazon Virtual Private Cloud (VPC). Earlier, the company announced the availability of the service on AWS Outposts Rack. Now, another enhancement is added with the support of the DoH protocol – data exchanged for DNS resolutions is encrypted. It enhances privacy and security by preventing eavesdropping and manipulation of DNS data during transmission between a DoH client and the DNS resolver based on DoH.
Furthermore, enabling DoH on Resolver endpoints aids customers in fulfilling regulatory and business compliance requirements, aligning with standards outlined in the US Office of Management and Budget memorandum.
Customers can utilize Amazon Route 53 Resolver to address DNS queries in hybrid cloud environments. For instance, AWS services can respond to DNS requests from any location within the hybrid network by setting up inbound and outbound resolver endpoints. Upon configuring the Resolver endpoints, customers will have the option to establish rules specifying the domains’ names for forwarding DNS queries from their VPC to an on-premises DNS resolver (outbound) and vice versa, from on-premises to their VPC (inbound).
Danilo Poccia, a Chief Evangelist at AWS, writes:
When you create or update an inbound or outbound Resolver endpoint, you can specify which protocols to use:
- DNS over port 53 (Do53), which is using either UDP or TCP to send the packets.
- DNS over HTTPS (DoH), which is using TLS to encrypt the data.
- Both, depending on which one is used by the DNS client.
- For FIPS compliance, there is a specific implementation (DoH-FIPS) for inbound endpoints.
In the Route 53 console, users can choose Inbound endpoints or Outbound endpoints from the Resolver section of the navigation pane.
Inbound endpoint Amazon Route 53 Resolver (Source: AWS News blog post)
In a research report on to what extent DoH prevents on-path devices from eavesdropping and interfering with DNS requests, Frank Nijeboer concluded:
We have shown in this research that, while eavesdropping of individual queries has not been evaluated, it is probably possible to deduce a visit to a specific website by looking at patterns in DoH packet sizes. Furthermore, interfering with DoH traffic by manipulating responses might not be possible, but detecting DoH resolvers and thereby blocking DoH is possible. As a consequence, the promised privacy protection of DoH is debatable, and the advantage of DoH against DoT (DNS over TLS) is getting smaller.
Other public cloud providers like Google offer DNS services like Cloud DNS, which also has DoH support. Furthermore, Cloudflare DNS and Infoblox provide DoH support with their Cloud DNS offerings.
Currently, DNS over HTTPS support for Amazon Route 53 Resolver is available in all AWS Regions where Route 53 Resolver is offered, including GovCloud Regions and Regions based in China. In addition, according to the company, there is no additional cost for using DNS over HTTPS with Resolver endpoints. The pricing details of Amazon 53 Resolver are available on the pricing page.
Microsoft.CodeCoverage v17.8 Released with New dotnet-coverage Tool and Other Improvements
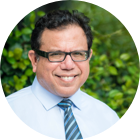
MMS • Almir Vuk
Article originally posted on InfoQ. Visit InfoQ
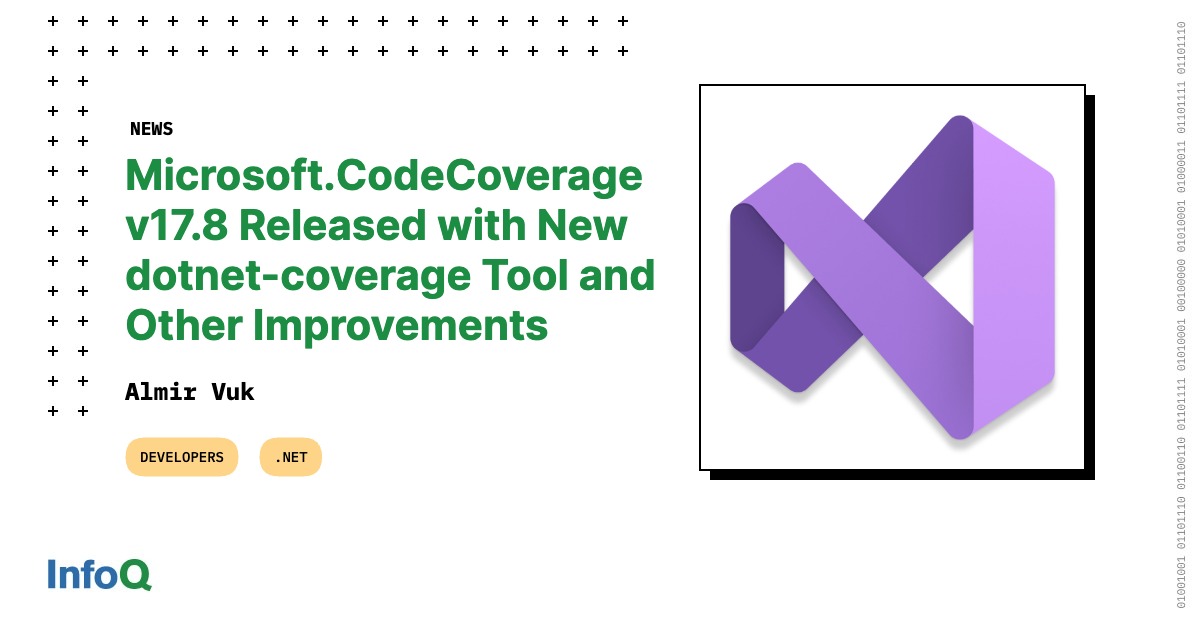
Microsoft recently announced an upgraded version of its developer tools, version 17.8.0, introducing significant improvements to Microsoft.CodeCoverage tools. Notably, the update includes the introduction of the dotnet-coverage tool. Additional enhancements comprise new report formats, an auto-merge tool, performance upgrades, and improved documentation.
A notable addition to the set of tools is dotnet-coverage, which carries various roles, including collecting code coverage for console and web applications, merging coverage reports, instrumenting binaries, and calculating code coverage for individual tests. This new tool significantly contributes to a more complete and efficient code coverage analysis of Microsoft.CodeCoverage.
One notable improvement is the expanded platform compatibility of the tools, achieved through the incorporation of static instrumentation. Regarding that, Microsoft’s code coverage tools initially relied on dynamic instrumentation for the Windows platform.
Later versions introduced static code coverage collection, pre-instrumenting libraries on disk for universal .NET platform support. Dynamic instrumentation remains the default, but on non-Windows systems, static is activated, avoiding redundant dynamic instrumentation. Developers control the method through configuration flags.
Furthermore, the code coverage report formats have experienced a comprehensive overhaul to integrate with auxiliary tools such as ReportGenerator. While the default .coverage format remains unchanged, several new formats have been introduced, including Binary (Default) in .coverage format for Visual Studio Enterprise, Cobertura in .cobertura.xml format compatible with Visual Studio Enterprise and text editors, and XML in .xml format for use with Visual Studio Enterprise and other text editors.
A new feature introduced in this update is Auto-Merge for solutions. Executing the command dotnet test --collect 'Code Coverage'
at the solution level now automatically consolidates code coverage data from all associated test projects. This process simplifies the management of code coverage across complex solutions.
Addressing a performance concern before the 16.5 release, there has been a notable improvement in the efficiency of code coverage report collection. Previously, this process substantially restrained test execution speed.
With the identified issue rectified, developers can now benefit from an impressive 80% increase in performance, making the code coverage analysis more efficient and less intrusive. The readers can take a look at benchmark results which are available at the official announcement post.
To get started with the most recent features and optimise build processes, developers need to incorporate the latest stable packages into test projects.
With the note that solutions without C++ code, developers can enhance speed and reliability by deactivating native code coverage using the specified flags in the runsettings configuration.
False
False
Lastly, the latest documentation improvements can be found in the updated GitHub repository. The repository provides users with a comprehensive information resource and samples for an improved documentation experience.
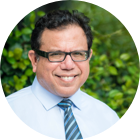
MMS • Renato Losio
Article originally posted on InfoQ. Visit InfoQ
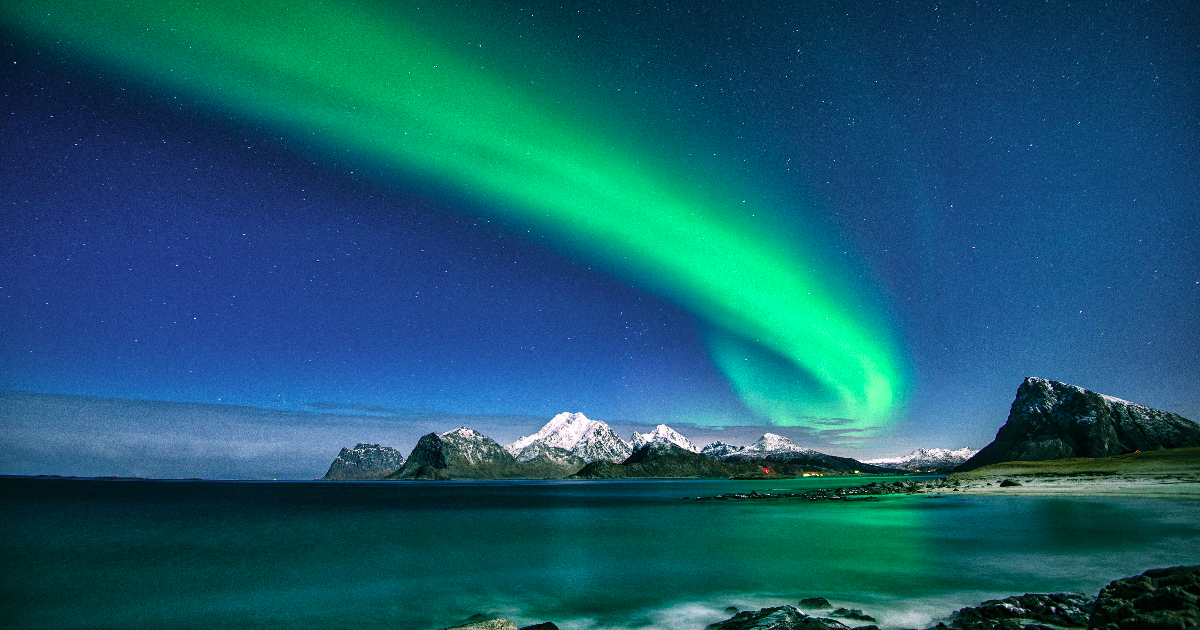
Recently, AWS introduced a new Data API for Aurora Serverless v2 and Aurora provisioned database instances. Currently available for PostgreSQL clusters only, the Data API doesn’t require persistent connections to a database cluster, addressing a long-awaited need among developers to simplify serverless deployments.
By utilizing the Data API, developers can access Aurora clusters through an HTTPS endpoint and execute SQL statements without the need to manage connections or database drivers. While Aurora Serverless v1 introduced a Data API several years ago, the absence of a Data API on Aurora Serverless v2 has proven to be a significant limitation for serverless workloads running on AWS.
Steve Abraham, principal database specialist solutions architect at AWS, writes:
AWS has rebuilt the Data API for Aurora Serverless v2 and Aurora provisioned to operate at the scale and high availability levels required by our biggest customers. (…) Because the Data API now works with both Aurora Serverless v2 and provisioned instances, database failover is supported to provide high availability.
The serverless community has positively received the new feature but Joshua Moore comments on X (formerly Twitter):
It’s a good step but one of its limitations is it only supports the write cluster and not read clusters though! Reads are where most apps are heavily hit!
The Data API is not yet available on MySQL clusters and burstable general-purpose classes. Furthermore, Performance Insights cannot monitor queries made using the Data API.
According to the documentation, the latest RDS Data API is backward compatible with the old one for Aurora Serverless v1. Still, there are significant differences between the two versions, with the cloud provider encouraging customers to migrate to the newest version. Abraham adds:
We have removed the 1,000 requests per second limit. The only factor that limits requests per second with the Data API for Aurora Serverless v2 and Aurora provisioned is the size of the database instance and therefore the available resources.
In a popular Reddit thread, user beelzebroth writes:
This is huge if you like Aurora Serverless. The data API is a big reason I was holding onto some v1 clusters.
User Nater5000 adds:
Damn, this is great. The lack of the Data API with Serverless v2 was very disappointing. I figured it wasn’t coming back.
The Data API has a size limit of 64 KB per row in the result set returned to the client. The new feature is supported in AWS AppSync, allowing developers to create GraphQL APIs that connect to Aurora databases, using AppSync JavaScript resolvers to run SQL statements.
Data API supports Aurora PostgreSQL 15.3+, 14.8+, and 13.11+ clusters in Northern Virginia, Oregon, Frankfurt, and Tokyo. According to the announcement, the Data API for MySQL clusters should be available soon.
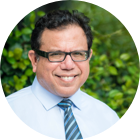
MMS • Sergio De Simone
Article originally posted on InfoQ. Visit InfoQ
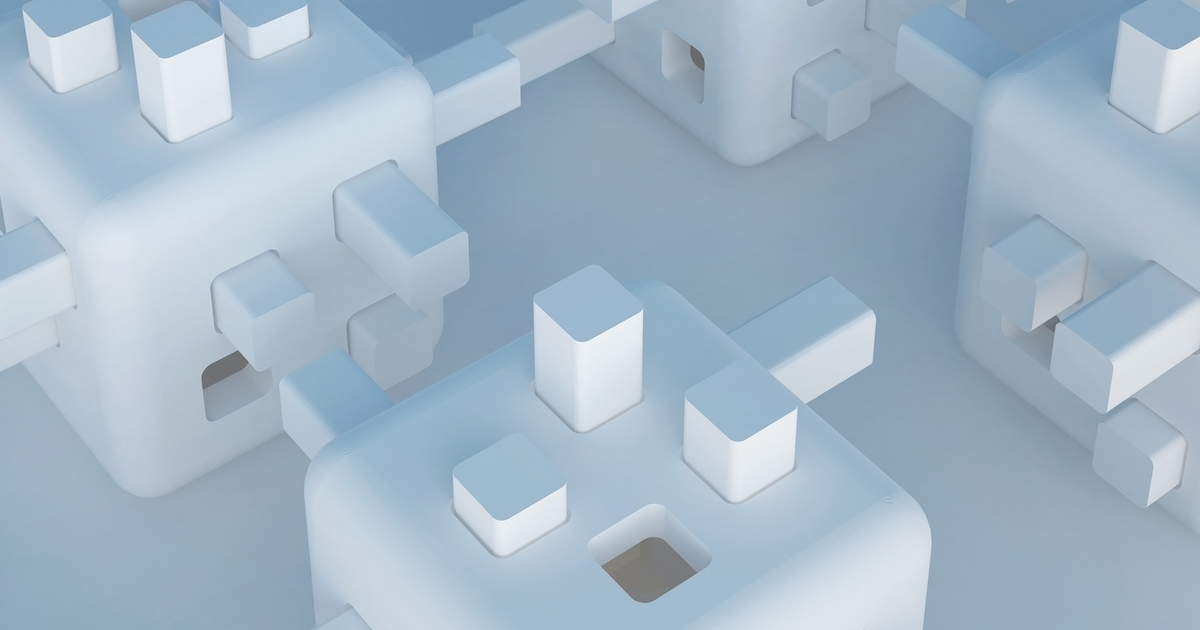
Recently announced at BazelCon 23, Bazel 7 materializes several new features that have been in development for multiple years, including the new modular external dependency management system Bzlmod, a new optimizing “Build without the Bytes” mode, improved multi-target build performance thanks to Project Skymeld, and more.
Bzlmod is a new modular external dependency management system replacing the old WORKSPACE
mechanism. A Bazel module is a project that can have multiple versions, with each version depending on a set of dependencies, as shown in the following snippet:
module(name = "my-module", version = "1.0")
bazel_dep(name = "rules_cc", version = "0.0.1")
bazel_dep(name = "protobuf", version = "3.19.0")
Bzlmod, the new external dependency subsystem, does not directly work with repo definitions. Instead, it builds a dependency graph from modules, runs extensions on top of the graph, and defines repos accordingly.
Bzlmod is now enabled by default, which means that if a project does not have a MODULE.bazel
file, Bazel will create an empty one. Bazel 7 will still work with the previous system for compatibility, but developers should migrate to Bzlmod before Bazel 8 is released.
Build without the Bytes (BwoB) reduces the amount of data that Bazel transfers for remote builds by only downloading a subset of intermediate files.
In the past, Bazel’s default behaviour was to download every output file of an action after it had executed the action remotely, or hit a remote cache. However, the sum of all output files in a large build will often be in the tens or even hundreds of gigabytes. The benefits of remote execution and caching may be outweighed by the costs of this download, especially if the network connection is poor.
While it has been available for a long time, BwoB is now stable and has been made reliable for edge cases so it can be used as the new default.
Skymeld is another feature aimed at boosting productivity, specifically for builds with multiple top-level targets. It brings an evaluation mode that eliminates the barrier between analysis and execution phases and enables targets to be independently executed as soon as their analysis finishes to improve build speeds.
As a final note, Bazel 7 now enables by default platform-based toolchain resolution for its Android and C++ rules. This change aims at streamlining the toolchain resolution API across all rulesets, thus obviating the need for language-specific flags such as --android_cpu
and --crosstool_top
.
Android projects will need to stop passing the legacy flag
--fat_apk_cpu
, and instead use--android_platforms
using platforms defined with the@platforms//os:android
constraint.
There is much more to Bazel 7 than can be covered here, so do not miss the official release notes for the full details.
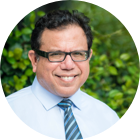
MMS • RSS
Posted on mongodb google news. Visit mongodb google news
Chipmaker Nvidia (NVDA) isn’t the only AI play investors should be favoring. Macquarie Head of U.S. AI & Software Research Fred Havemeyer joins Yahoo Finance Live the assortment of tech and software developers AI investors should consider in 2024, including Microsoft (MSFT), ServiceNow (NOW), and CrowdStrike (CRWD) among others.
“I believe that in 2024, we’re going to begin to see the first really significant and material signs of enterprise adoption of generative AI, that going into also calendar year 2025… we’ll be seeing significant adoption trends occurring,” Havemeyer says. “With now products like Microsoft 365 Copilot rolling out, enterprises will actually have the opportunity to adopt and purchase these products.”
Click here to watch the full interview on the Yahoo Finance YouTube page or you can watch this full episode of Yahoo Finance Live here.
Video Transcript
BRIAN SOZZI: All morning long, we’re sitting here really all week long, I should say, really talking about all things NVIDIA. What might be in store for them? But you’re here to say I think there are other names besides NVIDIA investors should be looking at. What are those names?
FRED HAVEMEYER: Yeah. Thank you. We put together a basket of names that we think provide exposure to the theme of generative AI just across a number of different sectors of software because even on our end, we thought for a while that Microsoft was one of the primary names to find exposure to significant and we think profitable generative AI growth.
So we put together a basket of stocks that we believe have solid exposure to generative AI, either directly or indirectly. And that includes, of course, Microsoft ServiceNow, CrowdStrike, MongoDB, HubSpot, Salesforce, and PowerSchool.
So we’re looking at names all up and down the value chain here. We’re looking at companies that we think will be monetizing products built with generative AI directly, like your ServiceNow, is your Salesforce, is HubSpot’s, even PowerSchool, as well as those that are in the pick and shovel side of things like a MongoDB on the database side. And those that are exposed we think too what will be trends that are supportive of cybersecurity adoption related to generative AI with CrowdStrike pick
BRAD SMITH: So you think about some of the largest forces that have driven even investors’ attention towards cybersecurity AI over the course of this year. What type of long tail are you seeing on those trades?
FRED HAVEMEYER: I think that this year was the year that we started seeing in 2023, it was the rise of ChatGPT. We all now suddenly know and can see a really commercialized use case that became extremely popular among consumers and even businesses of generative AI. I think going into 2024 and beyond, I believe that in 2024, we’re going to begin to see the first really significant and material signs of enterprise adoption of generative AI.
That going into also calendar year of 2025, I think we’ll be seeing really significant adoption trends occurring. I think with now products like Microsoft 365 Copilot rolling out, enterprises will actually have the opportunity to adopt and purchase these products, which were not previously available except on early access bases.
So going into 2024, we’re finally going to see pricing emerge for many of these products. And as a result and on the other side of this, I think we’re going to see a significant trend of adoption on enterprise generative AI. We’re starting to see it with ServiceNow. We’re certainly seeing it with Microsoft. And as companies roll out more products, I’m looking forward to seeing how the pricing and competition emerges. But I think 2024 is going to be the year of enterprise adoption of generative AI.
Article originally posted on mongodb google news. Visit mongodb google news
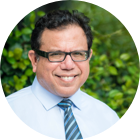
MMS • Aditya Kulkarni
Article originally posted on InfoQ. Visit InfoQ
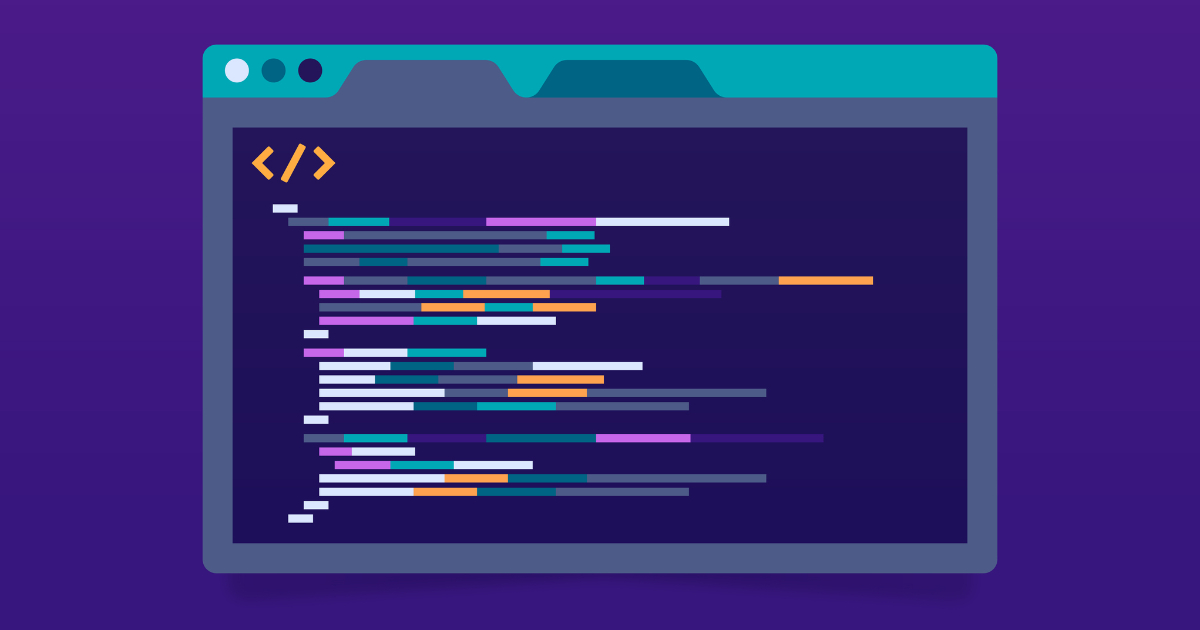
GitHub recently introduced trace2receiver, an open-source tool that integrates with OpenTelemetry to analyze Git performance data. This tool allows users to identify performance issues, detect early signs of trouble, and highlight areas where Git itself can be enhanced.
In a blog post, Jeff Hostetler, a Staff Software Engineer at GitHub, detailed the process of collecting Git performance data. As enterprises develop larger monorepos, the demand on Git for high performance, regardless of repository size, has increased. Effective monitoring tools are essential to assess Git’s performance in real-world scenarios, beyond just simulated tests. Trace2 offers detailed performance data, but interpreting this complex information often requires additional visualization.
The core of OpenTelemetry includes a customizable collector service daemon, supporting various modules for data collection, processing, and export. To integrate Git performance data, the Git team developed an open-source component, trace2receiver. This component allows custom collectors to gather Git’s Trace2 data, convert it to a standard format (like OTLP), and send it to visualization tools for analysis.
The trace2receiver tool serves two main functions in collecting Git telemetry data. First, it allows for an in-depth analysis of individual Git commands, tracking the time spent on each step, including any nested child commands in what is termed a “distributed trace” by OpenTelemetry. Second, it facilitates the aggregation of data across various users and machines over time. This enables the calculation of summary metrics, like average command times, providing a comprehensive view of Git’s performance on a larger scale. This data is crucial for identifying areas for improvement and understanding user frustrations with Git.
The tech community is responding positively to advancements in handling large repositories. This is reflected in the reactions on a related Hacker News post, where user ankit01-oss praised the adoption of OpenTelemetry by large firms. Additionally, Microsoft’s introduction of Git Partial Clone in Azure DevOps shows a trend toward more efficient handling of large codebases.
Hostetler noted certain limitations and caveats, such as the potential for laptops to sleep during Git command execution, which can skew performance data and the fact that Git hooks do not emit Trace2 telemetry events. Interactive Git commands, like git commit
or git fetch
, often pauses for user input, making them appear to run longer than they do. Similarly, commands git log
may trigger a pager, further delaying command completion. To understand these delays, the trace2receiver tool uses child(...)
spans to track the time spent on each subprocess, whether it’s a shell script or a helper Git command. This helps in identifying the actual duration of Git commands, distinguishing real processing time from waiting for user interaction.
In conclusion, Hostetler encourages readers to begin tracking performance data for their repositories to identify and analyze any Git-related issues. For those interested in learning more, he suggests reviewing the project documentation or consulting the contribution guide to understand how to contribute to the project.
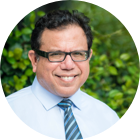
MMS • RSS
Posted on mongodb google news. Visit mongodb google news
Investors interested in Computer and Technology stocks should always be looking to find the best-performing companies in the group. Is MongoDB (MDB) one of those stocks right now? By taking a look at the stock’s year-to-date performance in comparison to its Computer and Technology peers, we might be able to answer that question.
MongoDB is one of 622 companies in the Computer and Technology group. The Computer and Technology group currently sits at #4 within the Zacks Sector Rank. The Zacks Sector Rank considers 16 different groups, measuring the average Zacks Rank of the individual stocks within the sector to gauge the strength of each group.
The Zacks Rank is a proven system that emphasizes earnings estimates and estimate revisions, highlighting a variety of stocks that are displaying the right characteristics to beat the market over the next one to three months. MongoDB is currently sporting a Zacks Rank of #2 (Buy).
Within the past quarter, the Zacks Consensus Estimate for MDB’s full-year earnings has moved 24.4% higher. This shows that analyst sentiment has improved and the company’s earnings outlook is stronger.
Our latest available data shows that MDB has returned about 111.9% since the start of the calendar year. In comparison, Computer and Technology companies have returned an average of 54%. This means that MongoDB is performing better than its sector in terms of year-to-date returns.
Alphabet (GOOGL) is another Computer and Technology stock that has outperformed the sector so far this year. Since the beginning of the year, the stock has returned 58.9%.
The consensus estimate for Alphabet’s current year EPS has increased 2.8% over the past three months. The stock currently has a Zacks Rank #2 (Buy).
Breaking things down more, MongoDB is a member of the Internet – Software industry, which includes 147 individual companies and currently sits at #29 in the Zacks Industry Rank. This group has gained an average of 73.4% so far this year, so MDB is performing better in this area.
In contrast, Alphabet falls under the Internet – Services industry. Currently, this industry has 39 stocks and is ranked #36. Since the beginning of the year, the industry has moved +60.2%.
Going forward, investors interested in Computer and Technology stocks should continue to pay close attention to MongoDB and Alphabet as they could maintain their solid performance.
Want the latest recommendations from Zacks Investment Research? Today, you can download 7 Best Stocks for the Next 30 Days. Click to get this free report
MongoDB, Inc. (MDB) : Free Stock Analysis Report
Alphabet Inc. (GOOGL) : Free Stock Analysis Report
To read this article on Zacks.com click here.
Article originally posted on mongodb google news. Visit mongodb google news
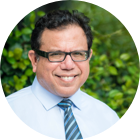
MMS • Mehrnoosh Sameki
Article originally posted on InfoQ. Visit InfoQ
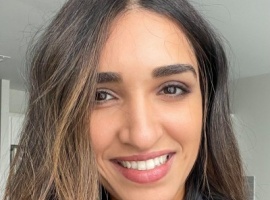
Transcript
Sameki: My name is Mehrnoosh Sameki. I’m a Principal Product Lead at Microsoft. I’m part of a team who is on a mission to help you operationalize this buzzword of responsible AI in practice. I’m going to talk to you about taking responsible AI from principles and best practices to actual practice in your ML lifecycle. First, let’s answer, why responsible AI? This quote from a book called “Tools and Weapons,” which was published by Brad Smith and Carol Ann Browne in September 2019, sums up this point really well. “When your technology changes the world, you bear a responsibility to help address the world that you have helped create.” That all comes down to AI is now everywhere. It’s here. It’s all around us, helping us to make our lives more convenient, productive, and even entertaining. It’s finding its way into some of the most important systems that affect us as individuals across our lives, from healthcare, finance, education, all the way to employment, and many other sectors and domains. Organizations have recognized that AI is poised to transform business and society. The advancements in AI along with the accelerated adoptions are being met with evolving societal expectations, though, and so there are a lot of growing regulations in this particular space in response to that AI growth. AI is a complex topic because it is not a single thing. It is a constellation of technologies that can be put to many different uses, with vastly different consequences. AI has unique challenges that we need to respond to. To take steps towards a better future, we truly need to define new rules, norms, practices, and tools.
Societal Expectations, and AI Regulation
On a weekly basis, there are new headlines that are highlighting the concerns regarding the use or misuse of AI. Societal expectations are growing. More members of society are considering whether AI is trustworthy, and whether companies who are innovating, keep these concerns top of mind. Also, regulations are coming. I’m pretty sure you all have heard about the proposal for AI Act from Europe. As I was reading through it, more and more, it’s talking about impact assessment and trustworthiness, and security and privacy, and interpretability, intelligibility, and transparency. We are going to see a lot more regulation in this space. We need to be 100% ready to still innovate, but be able to respond to those regulations.
Responsible AI Principles
Let me talk a little bit about the industry approach. The purpose of this particular slide is to showcase the six core ethical recommendations in the book called “The Future Computed” that represents Microsoft’s view on it. Note that there are a lot of different companies, they also have their own principles. At this point, they are a lot similar. When you look at them, there are principles of fairness, reliability and safety, privacy and security, inclusiveness, underpinned by two more foundational principles of transparency and accountability. The first principle is fairness. For AI, this means that AI systems should treat everyone fairly and avoid affecting similarly situated groups of people in different ways. The second principle is reliability and safety. To build trust, it’s very important that AI systems operate reliably, safely, and consistently under normal circumstances, and in unexpected situations and conditions. Then we have privacy and security, it’s also crucial to develop a system that can protect private information and resist attacks. As AI becomes more prevalent, protecting privacy and security of important personal and business information is obviously becoming more critical, but also more complex.
Then we have inclusiveness. For the 1 billion people with disability around the world, AI technologies can be a game changer. AI can improve access to education, government services, employment information, and a wide range of other opportunities. Inclusive design practices can help systems develop, understand, and address potential barriers in a product environment that could unintentionally exclude people. By addressing these barriers, we create opportunities to innovate and design better experiences that benefit all of us. We then have transparency. When AI systems are used to help inform decisions that have tremendous impacts on people’s lives, it’s critical that people understand how these decisions were made. A crucial part of transparency is what we refer to as intelligibility, or the user explanation of the behavior of AI and their components. Finally, accountability. We believe that people who design and deploy AI systems must be accountable for how their systems operate. This is perhaps the most important of all these principles. Ultimately, one of the biggest questions of our generation as the first generation that is bringing all of these AI into the society is how to ensure that AI will remain accountable to people, and how to ensure that the people who design, build, and deploy AI remain accountable to all other people, to everyone else.
The Standard’s Goals at a Glance
We take these six principles and break them down into 17 unique goals. Each of these goals have a series of requirements that sets out procedural steps that must be taken, mapped to the tools and practices that we have available. You can see under each section, we have for instance, impact assessment, data governance, fit for purpose, human oversight. Under transparency, there is interpretability and intelligibility, communication to stakeholders, disclosure of AI interaction. Under fairness, we have how we can address a lot of different harms like quality of service, allocation, stereotyping, demeaning, erasing different groups. Under reliability and safety, there are failures and remediation, safety guidelines, ongoing monitoring and evaluation. Privacy and security and inclusiveness are more mature areas and they get a lot of benefit from previous standards and compliance that have been developed internally.
Ultimately, the challenge is, this requires a really multifaceted approach in order to operationalize all those principles and goals at scale in practice. We’re focusing on four different key areas. At the very foundation, bottom, you see the governance structure to enable progress and accountability. Then we need rules to standardize our responsible AI requirements. On top of that, we need training and practices to promote a human centered mindset. Finally, tools and processes for implementation of such goals and best practices. Today, I’m mostly focusing on tools. Out of all the elements of the responsible AI standard, I’m double clicking on transparency, fairness, reliability, and safety because these are areas that truly help ML professionals better understand their models and the entire ML lifecycle. Also, they help them understand the harms, the impact of those models on humans.
Open Source Tools
We have already provided a lot of different open source tools, some of them are known as Fairlearn, InterpretML, Error Analysis, and now we have a modern dashboard called Responsible AI dashboard, which brings them together and also adds a lot of different functionalities. I’ll talk about them one by one, by first going through fairness and the philosophy behind it and how we develop tools. I will cover interpretability. I will cover error analysis. I will talk about how we bring them under one roof, and created Responsible AI dashboard. I’ll talk about some of our recent releases, the Responsible AI Tracker and Responsible AI Mitigation, and I’ll show you demos as well.
AI Fairness
One of the first areas that we worked on was on the area of AI fairness. Broadly speaking, based on the taxonomy developed by Crawford et al., at Microsoft Research, there are five different types of harms that can occur in a machine learning system. While I have all of the definitions of them available for you on the screen, I want to double click on the first two, harm of allocation, which is the harm that can occur when AI systems extend or withhold opportunities, resources, and information to specific groups of people. Then we have harm of quality of service, whether a system works as well for one person as it does for another. I want to give you examples of these two types of harms. On the left-hand side, you see the quality-of-service harm, where we have this voice recognition system that might fail, for instance, on women voices compared to men or non-binary. Or for instance, you can think of it as the voice recognition system is failing to recognize the voice of non-native speakers compared to native speakers. That’s another angle you can look at it from. Then on the right-hand side we have the harm of allocation, where there is an example of a loan screening or a job screening AI that might be better at picking candidates amongst white men compared to other groups. Our goal was truly to help you understand and measure such harms if they occur in your AI system, and resolve them, mitigate them to the best of your knowledge.
We provided assessments and mitigation. On the assessment side, we give you a lot of different evaluations. Essentially giving you an ability where you can bring a protected attribute, say gender, ethnicity, age, whatever that might be for you. Then you can specify one of many fairness metrics that we have. We support two categories of fairness metrics, what I would like to name as, essentially group fairness, so how different groups of people are getting treatments. These are metrics that could help measure those. One category of metrics that we provide is disparity in performance. You can say how model accuracy or model false positive rate, or false negative rate, or F1 score, or whatever else that might be differs across different buckets of sensitive groups, say female versus male versus non-binary. Then we have disparity in selection rate, which is how the model predictions differ across different buckets of a sensitive feature. Meaning, if we have female, male, non-binary, how do we select them in order to get the loan? To what percentage of them are getting the favorable outcome for a job, for a loan? You just look at your favorable outcome, or your prediction distribution across these buckets and you see how that differs.
On the mitigation side, we first enable you to provide a fairness criteria that you would like to essentially have that criteria guide the mitigation algorithm. Then we do have a couple of different classes of mitigation algorithms. For instance, for fairness criteria, two of many that we support is demographic parity, which is a criteria that reinforces that applicants of each protected group have the same odds of getting approval under loans. Loan approval decision, for instance, is independent of what group you belong to in terms of protected attributes. Then another fairness criteria that we support is equalized odds, which has a different approach in that sense that it looks at qualified applicants have the same odds of getting approval under a loan, regardless of their race, gender, whatever protected attribute you care about. Unqualified applicants have the same odds of getting approval under loans as well, again, regardless of their race, gender. We also have some other fairness criteria that you can learn more about on our website.
Now that you specify a fairness criteria, then you can call one of the mitigation algorithms. For instance, one of our techniques, which is state of the art from Microsoft Research, is called a reduction approach, with the goal of finding either a classifier, a regressor that minimizes error, which is the goal of all objective functions of our AI systems, subject to a fairness constraint. What it does is it takes a standard machine learning algorithm as a black-box, and it iteratively calls that black-box and reweight and possibly sometimes relabel the training data. Each time it comes up with one model, and you now get a tradeoff between the performance versus fairness of that model, and you choose the one that is more appropriate to you.
Interpretability
Next, we have interpretability. The tool that we put out there is called InterpretML. That is a tool that provides you with functionality to understand how your model is making its predictions. Focusing on just the concept of interpretability, we provide a lot of what we know as glassbox models or opaque box explainers. What that means, for glassbox models, these are models that are intrinsically interpretable, so they can help you understand your model’s prediction, understand how that model is coming up with its prediction. You train this model, and then they’re see-through. You can understand exactly how they make decisions based on features. Obviously, you might know of decision trees rule is linear models, but we also support a state-of-the-art technique or model called explainable boosting machine, which is very powerful in terms of performance, but also, it’s very transparent. If you are in a very regulated domain, I really recommend looking at EBM or explainable boosting machine. Not just that, we also do black-box explainability. We provide all these techniques that are out there under one roof for you to bring your model, pass it to one of these explainers. These explainers often don’t care about what is inside your model. They use a lot of different heuristics. For instance, in terms of SHAP, it’s a game theory technique that it uses to infer how your inputs have been mapped to your predictions and it provides you with explanations. We provide capabilities like, overall, model explanation. Overall, how the model is making its prediction. Also, individual predictions or explanations, like say for Mehrnoosh, what are the top key important factors impacting the model predictions of rejection for her?
Not just that, but inside InterpretML, we also have a package called DiCE, which stands for Diverse Counterfactual Explanations. The way that it works is it not only allows you to do freeform perturbation, and what if analysis where you can perturb my features and see how the model has changed its predictions, if any. You can also take a look at each data point and you say, what are the closest data points to this original data point for which the model is producing an opposite prediction? Say Mehrnoosh’s loan has got rejected, what are the closest data points that are providing a different prediction? In other words, you can think about it as what is the bare minimum change you need to apply to Mehrnoosh’s features in order for her to get an opposite outcome from the AI? The answer might be, keep all of Mehrnoosh’s features constant, but only increase her income by 10k, or increase income by 5k and have her to build one more year of credit history. That’s where the model is going to approve her loan. This is a very powerful information to have because, first, that’s a great debugging tool if the answer is, if Mehrnoosh had a different gender, or ethnicity, then the AI would have approved the loan. Then, obviously, you know that that’s a fairness issue. Also, it’s a really great tool, if you want to provide answer to humans as they might come and ask, what can I do next time to get approval from your loan AI? You can say, ok, so if you are able to increase your income by 10k in the next year, given all your other features stay constant or improve, then you’re going to get approval from our AI.
Error Analysis
After interpretability, we worked on another tool called Error Analysis. Essentially, Error Analysis, the idea of it came to our mind because, how many times really and realistically, have you seen articles in the press that mention that a model is 89% accurate, using therefore a single score accuracy number to describe performance on a whole new benchmark. Obviously, these one scores are great proxies. They’re great aggregate performance metrics in order to talk about whether you need to build that initial trust with your AI system or not. Often, when you dive deeper, you realize that errors are not uniformly distributed across your benchmark data. There are some maybe pockets of data in your benchmark with way higher error discrepancies or higher error rate. This error discrepancy can be problematic, because there is essentially, in this case, a blind spot, 42%. There is a cohort that is only having 42% accuracy. If you miss that information, that could lead to so many different things. That could lead to reliability and safety issues. That could lead to lack of trust for the people who belong to that cohort. The challenge is, you cannot sit down and combine all your possible features, create all possible cohorts, and then try to understand these error discrepancies. That’s what our error analysis tool is doing.
Responsible AI Toolbox
I just want to mention that before Responsible AI dashboard, which I’m about to introduce to you, we had these three individual tools, InterpretML, Fairlearn, and Error Analysis. What we realized is people want to use these together because they want to gain a 360 overview of their model health. That’s how we, first of all, introduced a new open source repository called Responsible AI Toolbox, which is an open source framework for accelerating and operationalizing responsible AI via a set of interoperable tools, libraries, and also customizable dashboards. The first dashboard underneath this Responsible AI Toolbox was called Responsible AI dashboard, which now brings all of these tools that I mentioned to you and more tools under one roof. If you think about it, often when you want to go through your machine learning debugging, you first want to identify what is going wrong in your AI system. That’s where error analysis could come into the scenario and help you identify erroneous cohorts of data that have a way higher error rate compared to some other subgroups. Then, fairness assessment can come also into the scenario because they can identify some of the fairness issues.
Then you want to move on to the diagnosis part, where you would like to look at the model explanations. How the model is making predictions. You maybe even want to diagnose the issue by perturbing some of the features or looking at counterfactuals. Or even, you might want to do exploratory data analysis in order to diagnose whether the issue is rooted into some data misrepresentation, lack of representation. Then you want to move on to the mitigate, because now that you’ve diagnosed, you can do targeted mitigation. You can now use unfairness mitigation algorithms I talked about. I will also talk about data enhancements. Finally, you might want to make decisions. You might want to provide users with what could they do next time, in order to get a better outcome from your AI, that’s where counterfactual analysis come into the scenario. Or you might want to just look at historic data, forget about a model, just look at historic data, and see whether there are any factors that have a causal impact on a real-world outcome, and provide your stakeholders with that causal relationship. We brought all of these tools under one roof called Responsible AI dashboard, which is one of the tools in Responsible AI Toolbox.
Demo (Responsible AI Dashboard)
Let me show you a demo of the Responsible AI dashboard so that we can bring all this messaging home. Then I’ll continue with two new additional tools under this family of Responsible AI Toolbox. I have here a machine learning model that can predict whether a house will sell for more than median price or not, and provide the seller with some advice on how best to price it. Of course, I would like to avoid underestimating the actual price, as an inaccurate price could impact seller profits and the ability to access finance from a bank. I turn into the Responsible AI dashboard to look closely at this model. Here is the dashboard. I can do first error analysis to find issues in my model. You can see it has automatically separated the cohorts with error accounts. I found out that bigger old houses have a much higher error rate of 25% almost in comparison with large new houses that have error rate of only 6%. This is an issue. Let’s investigate that further. First, let me save these two cohorts. I save them as new and old houses, and I go to the model statistics for further exploration. I can take a look at the accuracy, false positive rate, false negative rate across these two different cohorts. I can also observe the prediction probability distribution, and observe that older houses have higher probability of getting predictions less than median.
I can further go to the data explorer, and explore the ground truth values behind those cohorts. Let me set that to look at the ground truth values. First, I will start from my new houses cohort. As you can see here, most of the newer homes sell for higher price than median. It’s easy for the model to predict that and get a higher accuracy for that. If I switch to the older houses, as you can see, I don’t have enough data representing expensive old houses. One possible action for me is to collect more of this data and retrain the model. Let’s now look at the model explanations and understand how the model has made its predictions. I can see that the overall finish quality, above ground living room area, and total basement square footage are the top three important factors that impact my model’s prediction. I can further click on any of these, like over finish quality, and understand that a lower finish quality impacts the price prediction negatively. This is a great sanity check that the model is doing the right thing. I can further go to the individual feature importance, click on one or a handful of data points and see how the model has made predictions for them.
Further on, I come to the what if counterfactual. What I am seeing here is, for any of these houses, I can understand what is the minimum change I can apply to, for instance, this particular house, which has actually a high probability of getting the prediction of less than median, so that the model predicts the opposite outcome. Looking at the counterfactuals for this one, only if their house had a higher overall quality from 6 to 10, then the model would predict that this house would sell for more than median. To conclude, I learned that my model is making predictions based on the factors that made sense to me as an expert, and I need to augment my data on the expensive old house category and even potentially bring in more descriptive features that help the model learn about an expensive old house.
Now that we understood the model better, let’s provide house owners with insights as to what to improve in these houses to get a better price ask in the market. We only need some historic data of the housing market to do so. Now I go to the causal inference capabilities of this dashboard to achieve that. There are two different functionalities that could be quite helpful here. First, the aggregate causal effect, which shows how changing a particular factor like garages, or fireplaces, or overall condition would impact the overall house price in this dataset on average. I can further go to the treatment policy to see the best future intervention, say switching it to screen porch. For instance, here I can see for some houses, if I want to invest in transforming a screen porch for some houses, I need to shrink it or remove it. For some houses, it’s recommending me to expand on it. Finally, there is also an individual causal effect capability that tells me how this works for a particular data point. This is a certain house. First, I can see how each factor would impact the actual price of the house in the market. I can even do causal what if analysis, which is something like if I change the overall condition to a higher value, what boost I’m going to see in the housing price of this in the market.
Responsible AI Mitigations, and Responsible AI Tracker
Now that we saw the demo, I just want to introduce two other tools as well. We released two new tools as a part of the Responsible AI Toolbox. One of them is Responsible AI Mitigations. It is a Python library for implementing and exploring mitigations for responsible AI on tabular data. The tool fills the gap on the mitigation end, and is intended to be used in a programmatic way. I’ll introduce that to you in a demo as well. Also, another tool, Responsible AI Tracker. It is essentially a JupyterLab extension for tracking, managing, and comparing Responsible AI mitigations and experiments. In some way, the tool is also intended to serve as the glue that connects all these pieces together. Both of these new releases support tabular data, Responsible AI dashboard supports tabular data and support for computer vision and NLP scenarios, starting from text classification, image classification, object detection, and question answering scenarios. The new developments have two new differentiations. First, they allow you to do targeted model debugging and improvement, which in other words means understand before you decide where and how to mitigate. Second, they are interplay between code, data, model, visualizations. While there exist many data science and machine learning tools out there, as a ML professional team, we believe that one can get the full benefits in this space only if we know how to manage, serve, and learn from the interplay of all these four pillars in the data science, which are code, data, model, and visualization.
Debugging
Here you see the same pillars I talked about identify, diagnose, mitigate. Now you have this track, compare, and validate. The Responsible AI dashboard as is could cover identify and diagnose because it has tools like error analysis, fairness analysis, interpretability counterfactual, and what if perturbation, for both identify and diagnose. With Responsible AI mitigations, you could mitigate a lot of issues that are rooted in the data. Also, with Responsible AI Tracker, you can track, compare, validate, and experiment with which model is the best for your use case. This is different from general techniques in ML, which merely measure the overall error and only add more data or more compute. In a lot of cases, you’re like, let me just go and collect a lot more data. It’s obviously very expensive. This blanket approach is ok for bootstrapping models initially, but when it comes to really carefully mitigating errors related to particular cohorts or issues of interest that are particular to underrepresenting, overrepresenting to a certain group of people, it becomes too costly to add just generic data. It might not tackle the issue from the get-go. In fact, sometimes adding more data is not even doing much and is hurting particular other cohorts because the data noise is going to be increased or there are some unpredictable shifts that are going to be happening. This mitigation part, it is very targeted. It essentially allows you to take a look at exactly where the issue is happening and then tackle that from the core.
Demo: Responsible AI (Dashboard + Tracker + Mitigations)
Now let’s see a demo together to make sure we understand how these three offerings work together. Responsible AI Tracker is an open source extension to the JupyterLab framework, and helps data scientists with tracking and comparing different iterations or experiments on model improvement. JupyterLab itself is the latest web based interactive development environment for notebooks, code, and data for Project Jupyter. In comparison to Jupyter Notebooks, JupyterLab gives practitioners the opportunity to work with more than one notebook at the same time to better organize their work. Responsible AI Tracker takes this to the next step by bringing together notebooks, models, and visualization reports on model comparison, all within the same interface. Responsible AI Tracker is also part of the Responsible AI Toolbox, a larger open source effort at Microsoft for bringing together tools for accelerating and operationalizing responsible AI. During this tour, you will learn how to use tracker to compare and validate different model improvement experiments. You will also learn how to use the extension in combination with other tools in the toolbox, such as Responsible AI dashboard, and the Responsible AI Mitigations library. Let me show you how this works.
Here I have installed the Responsible AI Tracker extension, but I have not created a project yet. Let’s create a project together. Our project is going to use the UCI income dataset. This is a classification task for predicting whether an individual earns more or less than 50k. It is also possible to bring in a notebook where perhaps you may have created some code to build a model or to clean up some data. That’s what I’m doing right now. Let’s take a look at the project that was just created and the notebook that we just imported. Here we’re training on a split of the UCI income dataset. We are building a model with five estimators. It’s a gradient boosted model. We’re also doing some basic feature imputation and encoding.
After training the model, we can then register the model to the notebook, so that in the future we can perhaps remember which code was used to generate the model on the first place. We just pick the model file. We’re going to select the machine learning platform, in this case, sklearn, and the test dataset where we want to evaluate on. Next, we’re giving a few more information items related to the formatting and the class label. Then we’re going to register the model. We see that the overall model accuracy is around 78.9%. This doesn’t give us yet enough information to understand where most errors are concentrated on. To perform this aggregated model evaluation, we are going to use the Responsible AI dashboard. This is also part of the Responsible AI Toolbox. It’s a dashboard that consists of several visual components on error analysis, interpretability, data exploration, and fairness assessment. The first component that we see here is error analysis. This visualization is telling us that the overall error rate is 21%, which coincides to the accuracy number that we saw on tracker. Next, it’s also showing us that there exist certain cohorts in the data such as for example, this one, where the relationship is husband or wife, meaning that the individual is married for which the error rate increases to 38.9%. At the same time for individuals who are not married on the other side of the visualization, we see that the error rate is only 6.4%. We also see other more problematic cohorts such as for instance individuals who are married and have a number of education years higher than 11 years, for which the error rate is 58%.
To understand better what is going on with these cohorts, we are going to look at the data analysis and the class distribution for all these cohorts. In overall, for the whole data, we see that there exists a skew towards the negative label. There exist more individuals that earn less than 50k. When we look at exactly the same visualization for the married cohort, we see that the story is actually more balanced here. For the not married cohort, the balance looks more similar than the prior on the overall data. In particular, for the cohort that has a very high error rate at 58% married and number of education years higher than 11, we see that the prior completely flips on the other end. There exist more individuals in this cohort that earn more than 50k. Based on this piece of information, we are going to go back to tracker to see if we can mitigate some of these issues and also compare them.
Let’s now import yet another notebook that performs a general data balancing approach. We are going to import this from our tour files in the open source repository. These data balancing techniques, basically what it’s doing is that it is generating the same number of samples from both classes, the positive and the negative one. If we see how the data looks like after rebalancing, we can see that the full data frame is perfectly balanced. However, more positive labels have been sampled from the married cohort. That is probably because the married cohort initially had more positive examples to start with. After training this model, we can then register the model that was generated by this particular notebook. Here, we can bring in the model. We can register exactly the same test dataset so that we can have a one-to-one comparison. We give in the class and see how these two models compare. We can see that, overall, data balancing has helped, the accuracy has improved, but we want to understand more. We want to understand how the tradeoff between accuracy and precision plays off. Indeed, we can see here that even though the overall accuracy has improved by 3.7%, precision has dropped by a large margin.
When we look at the married and not married cohorts, which are the ones that we started earlier with the Responsible AI dashboard, we can see that indeed most of the improvement comes from improvements in the married cohort. However, we also see that a lot of the precision has declined in the married cohort, which brings up the question of whether this type of balancing that is more like a blanket and general approach has hurt the precision for the married cohort, mostly because most of the positive data was sampled from this one. Then the question is, can we do this in a more custom way? Can we perhaps isolate the changes in data balancing between the two cohorts so that we can get the best of both worlds? There are two ideas that perhaps come in mind here, one of them could be, we can perfectly balance the two cohorts separately. The other idea would be perhaps to balance the married cohort, but since the not married cohort has good accuracy from the start, maybe it would be better to not make any changes. We will try both here. Both of these notebooks are available in our open source repository. I’m going to upload both of these in the project now, the one that balances both cohorts, and the one that leaves the not married cohort, unbalanced. We are going to talk about this as the targeted mitigation approach.
First, let’s see how we have implemented both of these mitigation techniques using the RAI Mitigations Library. In particular, we are going to use the data balancing functionalities in the library. Here, we see that we have created the cohorts. The first cohort is the married cohort. The other one is the complement of that. In this case, this coincides with the not married cohort. We have defined two pipelines, one for each cohort. Through the cohort manager class, we can assign which pipeline needs to be run in an isolated way for each of the cohorts. We see that basically these are doing the same thing. They are sampling the data so that in the end, we have equal frequencies of each of the classes. This is how the data looks like after the rebalancing. The overall data is perfectly balanced, and so are the two other cohorts. The other mitigation technique that we are going to explore here is to apply rebalancing only for the married cohort, because this is the one that had higher errors on the first place. For the second one, we are giving an empty pipeline. This is how the data looks like after this mitigation. The married cohort is perfectly balanced, but we have not touched the distribution for the rest of the data.
After this, we’re going to compare all of these models together. We’re going to compare them across different metrics, but also across the cohorts of interest. After registering the new models that we just trained with the two new mitigation techniques, we can then go back to the model comparison table and see how the model has improved in all these cases. First, let’s compare the strategy where we balance both cohorts separately. We see that this type of mitigation technique is at least as good as the baseline. However, most of the improvement is focused on the married cohort, and we see a sudden drop in performance for the not married cohort. Often, we refer to these cases as backward incompatibility issues in machine learning, where we see new errors being introduced with model updates. These are particularly important for real-world deployments because there may have been end users that are accustomed to trusting the model for certain cohorts, in this particular for the not married cohorts. By seeing these performance drops, this may lead to loss of trust in the user base. This is the story for the mitigation technique that balances both cohorts.
For the next mitigation technique, where we saw that we could target the data balancing only for the married cohort, and leave the rest of the data untouched, we see that in overall there is a 6% improvement, which is the highest that we see in this set of notebooks. At the same time, we see that there are no performance drops for the not married cohort, which is a positive outcome. Of course, there is good improvement in the cohort that we were set to improve in the first place for the married cohort. The precision is higher than in the blanket approach where we just balance the whole data. In this way, we can get a good picture of what has improved and what has not improved across all models, across different cohorts, and across different metrics. We can create more cohorts by using the interface. For example, earlier, we saw that the performance drops were mostly focused in the cohort of married individuals who had a number of education years that is higher than 11. We’re trying to bring in that cohort now and see what happened to this one. Here, we are adding the married relationship as a filter. Then we are going to add another filter that is related to the number of education years. We want this to be higher than 11. Let’s save this cohort and see what happened here. We saw that, initially, the accuracy for this cohort was only 41.9%. Through the different mitigation techniques, we are able to reach up to 72% or 73% by targeting the mitigation to the types of problems that we saw in the first place.
See more presentations with transcripts
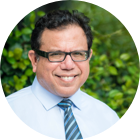
MMS • Rebecca Parsons Rafiq Gemmail Craig Smith Shaaron A Alvares
Article originally posted on InfoQ. Visit InfoQ
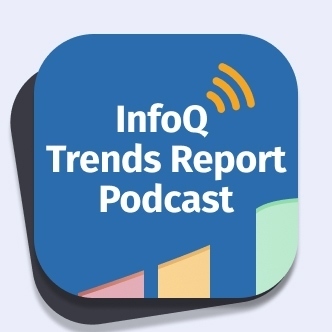
Subscribe on:
Transcript
Shane Hastie: Good day folks. This is the whole InfoQ culture team and special guest for recording our podcast for the Trend Report for 2023. I’m Shane Hastie, I’m the lead editor for Culture & Methods on InfoQ. I’m going to shepherd our conversation today, but we’ll start and I’ll go around our virtual room and ask people to introduce themselves. We’ll start with our special guest, Rebecca Parsons.
Rebecca, thank you so much for joining us. Would you mind just telling us very briefly who’s Rebecca?
Introductions [00:36]
Rebecca Parsons: Well. Thank you Shane for having me. As you said, my name is Rebecca Parsons. I’m the chief technology officer for Thoughtworks and we’re a software consulting company that we basically write software for other people.
Shane Hastie: Welcome.
Rebecca Parsons: Thank you.
Shane Hastie: Craig Smith.
Craig Smith: I’m the business agility practice lead for SoftEd, and I work with you as well Shane. Glad to be a part of the team.
Shane Hastie: And Raf Gemmail.
Raf Gemmail: Hi. Yes, I’m Raf. I am an engineering manager with Marigold in the MarTech space and many years technical coaching, Agile teams.
Shane Hastie: Thanks Raf. Last but certainly not least, Shaaron.
Shaaron A Alvares: Thank you Shane for having me. Very excited to be here and to be with all of you. Shaaron Alveres, I’m located in Seattle, Washington and I am director of GT Agile Transformation and Delivery at Salesforce. I’m working closely with executives to help them get better. I’m supporting of them and their teams in operation and engineering at GT.
Shane Hastie: Welcome folks. This is our motley crew of editors, reporters, and contributors for the InfoQ Culture and Method Space. It’s wonderful to get us all together in one virtual space for a change. It doesn’t happen often, but what’s happening with the Culture and Methods trends for 2023? We were having a bit of a chat earlier and I think the big elephant in the room is the economy and the layoffs and everything that we’ve seen in that space.
What do people think? Shaaron, can we start with you?
The economy and layoffs in tech companies [02:11]
Shaaron A Alvares: Yeah, I’m happy to kick us off. You’re right, Shane, with this new recession, there’s a lot of changes happening in organization, priorities are shifting. Executives are more concerned at the moment about financial results, which led to a number of layoffs globally.
We’ve seen a lot of layoffs globally, in the US as well. Then that led to prioritizing high performance team, productivity, and efficiency. A huge impact I want to say on the culture aspect of organizations, on delivery as well, but a huge impact on culture and maybe a lost understanding how psychological safety is important for organization and teams.
Shane Hastie: Well, we’ve just come through two years where we’ve been focused on wellness and suddenly we’ve shifted. What’s happened?
Rebecca, what have you seen?
Rebecca Parsons: Well, I think when the boom was happening, the tech talent was very clearly in the driver’s seat. You add that to the pressures coming from the war in Ukraine, the global pandemic, increased visibility of these extreme weather events, the earthquake. All of these things have been contributing to a tremendous amount of stress. I think the response from many of the employers, both of the technology companies themselves, but also the enterprises who are trying to build up their own technology competency is they knew they had to fight to get the talent that they wanted.
Thinking about people-care was an opportunity to appeal to the needs of some of the people that they were trying to hire. I think as the macro economic pressures have changed, and we’ve heard this from some of the enterprises that we talk to that are not digital natives or not the technology companies, I don’t have to fight with Facebook anymore for talent, because Facebook’s just laid off all of these people or insert name of technology company here.
I think one of the interesting things that is particularly relevant from a culture and methods perspective is, okay, yes, you’ve got these people who are on the market, but are you going to actually be able to hire them as an enterprise? Even if you can hire them, are you going to be able to retain them when the market does turn around?
I’m reminded of a talk that Adrian Cockcroft gave several years ago where somebody from a more traditional enterprise said, “Well, yes, you can do all these things because you’ve got these wonderful people. And it’s like, but I hired those people from you. I just gave them an environment within which they could be productive.”
I do think we are going to see much more of a focus on how do we actually create an environment where we can get efficiencies and effectiveness, but we don’t impact time to market, because I don’t think we’re going to go back to that era around the turn of the century, where so many places still considered IT as a cost center and that meant you had to stabilize and you had to standardize and you had to lock things down. Consumers are not going to accept that, even in this downturn situation.
Shane Hastie: Thank you. Craig, what are your thoughts on this?
Craig Smith: Firstly, to anyone’s whose listening who’s been through that, I think we share their pain and things that they’re going through, but I think what I’m seeing from the outside is this is a little bit for a lot of organizations actually are a right-sizing that they needed to do that actually there’s a lot of waste that we see in organizations. Whilst all those people were effectively working on things, the size of the organization probably couldn’t sustain that.
What worries me though is that in this, were the leaders responsible for doing this, did they actually take the people from the right places? In other words, rather than just chopping off a whole side of a place here and moving it along, what I’m seeing in some of the organizations that I’ve been working with is that they’ll just say, “Look, we’re just going to get rid of these six teams over here,” but they weren’t the right places to get rid of.
The actual waste was in the actual mechanics of the organization. I think there’s also going to be a realization of that moving through, but you can argue for a lot of those tech companies, particularly in Silicon Valley and the like, maybe they were just too big for what they needed. I think the way we’re seeing some of these things play out in the media, whilst the way … as Ben was talking about … the way that’s played out and the real culture of the company may start to shine through, it’s also going to be interesting to see how this actually plays out in the end.
Do some of those organizations and now there’s a much more radicalized size they have, was that actually what they should have been in the first place? Was there just too many people doing too many things for the organization anyway?
Shane Hastie: Raf, your thoughts.
Raf Gemmail: Listening to them about the culture coming out and the discrepancy in styles of letting people go. One thing I’ve been reflecting on is I remember back to mid 2000s, I was in the environment of US banks and there would be culls every now and then, where people would just pop, pop, pop away from their desks. We went through a transformation after that I saw, where there was a lot more humanism in the workplace. Looking at what’s happened externally and to the people I know, the change management in letting people go in a more respectful fashion for some reason seems to be disappearing.
Yes, there may be an element of right sizing in all of this, but I completely agree with Craig, because when you are doing things with such haste, and maybe I’m projecting, inferring here, but when you’re doing things at such haste, the knock on impact, which I have seen, is that you are left with teams which may not reflect the capabilities and the topologies and communication channels you’d intentionally try to create in the first place. You will have a consequence of that.
I’m looking at it from the perspective of we’ve been through one big shift with COVID and maybe it took us in a particular direction, which I think was potentially positive. Now there’s this fear which is dollar driven, this financial fear, realizable fear. Maybe to some extent that is compromising a humanism as organizations, which have been trying to become more humanistic generally. That disturbs me a little bit.
Shane Hastie: Rebecca, can I put you on the spot? In your role at Thoughtworks, I’m assuming that you’ve had these conversations internally, have we got the right people and so forth? How do you keep that humanistic perspective at the fore?
Keeping workplaces humanistic when faced with pressure [08:29]
Rebecca Parsons: Well, I definitely agree it is one of those fundamental cultural aspects of companies. From the beginning, we have looked at our people as the most important asset that we have. If you truly believe that, and a lot of companies say it, but if you truly believe that, then you know that you have to look at things like capability and capacity and policies from that more humanistic perspective. I often get into debates with people when they’re looking at diversity programs and they want to go immediately to the business case.
Yes, there’s a very strong business case for this. There is a strong business case for having a strong employee value proposition, because turnover is expensive and replacing people is expensive. If you treat people well, you can make all of these wonderful business case arguments, but you can also look at it as it’s just the right thing to do. If you truly believe that your people are your most valuable asset, what do you do with valuable assets?
You invest in maintaining them and nurturing them and helping them grow. You have to look at it from that humanistic perspective. Even now, particularly given the options that are available to technologists, treating somebody poorly in a layoff. It sounds like that is something that is happening in many of the organizations right now, based on the froth that you hear in the social media realm. You’re just dooming yourself, in terms of your ability to hire, because there’s only so much you can get back in terms of that credibility when push comes to shove, okay, we have to do a layoff. Then you’ve got all of these people going off on Twitter and LinkedIn about just how wrong and how cruel, needlessly cruel the process was.
Shane Hastie: An interesting article. It was a Wall Street Journal article I saw I think was talking about yes, there have been these massive layoffs, but also most of these people who are laid off are being re-employed very quickly. We’re not seeing a lack of employment and a lack of demand. Is it just perhaps the people moving around. As Craig said, somewhat a bit of right-sizing? How do people feel about that?
Downsizing or rightsizing? [10:49]
Raf Gemmail: I think we’re at the start of the curve. We’ve seen a big spike, lots of people in the market, but what I’m seeing is that trickling to other places. We’re in New Zealand and I’ve recently heard of a local company in New Zealand, which is letting go of many people. It had grown rapidly to hundreds of people, same sort of deal, and people are being let go of.
These things often start somewhere and they spread. While they’re starting to spread, they may be still later demand in the market. I’m hiring right now, but we are on that curve and so we’ll see where we go. I think I’m confident that if a company manages and looks after its people well that you can create a good culture, but in the industry at large there are always waves. I think we’re starting on that wave.
Shaaron Alveres: About this article. I didn’t read it but I’m going to read it, so thank you for the reference,Shane, but what I think there are jobs, it’s true that there’s been an increase in job posting. I read that, but I think it’s in small and medium size companies and mostly retail and service type of companies. I don’t think it’s in technology, software.
What I want to say is during the pandemic, those technology companies have hired massively, because we had to move to online, to the cloud, and there’s been massive hires. Companies like Amazon, my company, Microsoft, they hired people they probably hired in two years close to between I think between 50,000 to a 100,000 people. Sometimes, in those large companies. Now, what had happened is there wasn’t always a good onboarding process for those engineers. We focused on hiring and we expected them to deliver right away, but there wasn’t a great onboarding plan, especially when we hire so many people and we set up brand new teams. I think that has created maybe a perceived lack of productivity and efficiencies.
Other companies, what I want to add is some companies chose to lay off massively, but very few executive chose to actually decrease their bonus or their compensation and not lay off those employees. They had a choice as well. We saw a lot of articles around executive who chose to not lay off their employees, because they had onboarding them. They already have acquired a lot of knowledge. That’s good to see.
I’m trying to look at the silver lining around those layoffs. One thing that happened recently is I think companies organization, as they lay out their FY 24 plan, objectives, they started focusing a lot more on priorities. There’s a ruthless prioritization that is happening actually within organizations to make sure that we focus on the right thing and all of our resources are really focusing on maybe doing less but focusing on the right thing. That’s a silver lining. That’s good.
Raf Gemmail: There’s a counterpoint to focusing on the right thing. I came across a term recently … I’m not prolific like the others, but … the last piece I wrote was something a lady called Sandy Mamoli who was with us last year and she was quoting a guy from New Zealand who used the word “quarter fall”. This idea of upfront planning, sometimes if those priorities are guiding where you go too stringently, that ability to discover, to create, to pivot may be compromised somewhat. I’m not always a fan of the ruthless prioritization sometimes, because I think innovation comes in the gaps in between.
Shaaron A Alvares: It has to be both prioritizing … and when I talk about annual planning, I think most organization, I hope so, they’re doing both. We set up goals for the year, but we review those goals on a quarterly basis. But I agree with you Raf, there’s also pitfalls with less prioritization.
Shane Hastie: hink that gives us a nice segue to what could be our next topic. What is happening? What are the innovations that our listeners, our readers should be preparing for addressing?
One of the big ones I want to throw up there is ChatGPT. We can’t have a conversation without somebody bringing in, “I type this into ChatGPT and here’s what it says…” Just taking that as one example, what’s happening?
The impact of ChatGPT and AI [14:57]
Craig Smith: Well, obviously the world of AI was always going to catch up with us in some way. I’m sure we’ve all read the articles about how ChatGPT is going to take away our roles and how, I think the one I just read the other day was that Agile coaches and scrum masters would be no longer needed, because you could just ask ChatGPT your questions. But I think going past just one particular tool, we’re on the very start of the hockey stick in relation to AI and machine learning and all those types of things.
We’ve been collecting all this data, we talk about it, but now actually starting to turn it into tools like what we’re starting to see, not only with that but also with image processing and all sorts of other things. How those tools can be used for useful purposes as opposed to, I guess some of the negative reporting we get now, which is how they use for evil, but also then trying to bring in things like copyright.
We read articles about how schools are banning tools like that, which worries me greatly because it’s like when I went to school … showing my age … you couldn’t use a calculator, you had to add up in your head. I can’t remember how many times I’ve tried to do functions and equations in my head without a calculator or something in real life. Embrace the tools that help us do that, but don’t copy the answers. Teach people about using these tools effectively.
It’s going to be a real challenge across all of our industries about how we embrace these tools and use them to move forward as opposed to trying to block them or push them away.
Raf Gemmail: There’s a security and privacy aspect to that as well, Craig, around what you’re putting into these third party tools. I’ve been using it, experimenting is probably the word to use at this phase, but I had to give some feedback to someone who was interviewed for some other documentation. I’ve used the text side of it, which it’s amazing at, but I’m very conscious with these things not to put real names in, not to disclose more than you would want to put into a Google search.
I was in a conversation yesterday which covered this sort of thing, that you don’t want to put too much IP into these things. You want to have some guidelines. I think we’re in that wild west where we’re still trying to figure out how to use it, but there’s definitely an element of how much do you put into those systems? How much do you edit it? I edit it after the event. I’m like, okay, this is what it said, now fit it to my context, because A, I don’t talk like this. And B, I want this to be more personal and reflect the person I’m talking to, and what I know about them.
Craig Smith: While we were doing this, I just typed to the ChatGPT, “What are some of the innovations in culture and methods?” The answer was “agile culture, Lean Startup, design thinking, DevOps, holacracy and agile HR.”
There you go. Conversation done.
Shane Hastie: Interestingly, that many of those are the trends that we have on our graph.
Rebecca, what are you seeing with ChatGPT and AI?
Rebecca Parsons: Well, we’re doing a fair amount of experimentation both with the text capabilities of ChatGPT as well as the code-generation of both co-pilot and ChatGPT. The big concern and the big questions that we get and are uncovering ChatGPT in particular, is very confident of every answer it gives, whether it’s utter nonsense or not. Sometimes, it really is absolutely spot on. Okay, yeah, be confident, you’re right. Then it will confidently assert that the name of a country that is four letters that starts and ends with the same letter is “Chad”.
Again, very confidently asserting, but it’s utter nonsense. It does not know what it does not know. It knows areas it’s not supposed to talk about, because it’s got the guardrails in there, but it doesn’t know what it doesn’t know. That means from a code generation perspective, very often the mistakes are subtle. Occasionally, it will be blindingly obvious because it’s generating some java code that won’t compile or whatever, but very often it’s things, off by one errors or something subtle like that, where if you don’t actually know the result that you want, you won’t actually know that the result it gave you is wrong.
Now, even with the level of quality that it has right now within a specific context, it can generate some really impressive code. One of our people in China put it through an exercise in typescript to basically generate a CRUD application, connecting to a Postgress database and oh, what do I have to do now? Now, how do I get this thing containerized? Took it through the step. One of the really powerful things about even the current instance is the ability for the model to get context, so that you’re not always starting from scratch.
That means you can say, “Oh well that was close, but use this library instead,” and it will keep the context of what it had before and take your new prompt into account. We’re seeing people who are claiming though 60% increase in developer productivity. Well, I can imagine if you’re doing something for which a canned algorithm exists out there on the net, that yeah you could probably generate it pretty easily, but to make a general claim about more broad software development activities, you have to take into account how much time do you actually spend typing versus thinking versus executing?
It’s only going to be able basically to help you with the typing. Maybe if you’re trying to learn a new language, an experienced programmer, we’ve got somebody who’s actually trying to do that, trying to learn a new language by using ChatGPT. But again, it works best if you know the answer that you want.
Shane Hastie: What’s happening in responsible tech? Rebecca, I know that you have a particular focus there.
Movements in responsible tech [20:46]
Rebecca Parsons: Well, people are starting to take it more seriously in the first place. They’re starting to recognize the negative brand implications of breaches or other unmitigated consequences. Their investors are taking note of it, although there is some backlash in various places against ESG reporting, but investors are looking more broadly at how our companies are approaching their technology development.
If you leave hackers aside for the moment, none of us sets out to do bad things to people or communities. We don’t do it intentionally. We’re trying to do the right thing and sometimes the unintended consequences of what we do are good and there are additional benefits that we did not anticipate, but more often than not those unintended consequences are in fact bad. This whole responsible tech movement is around can’t we as technologists be more intentional about trying to see what we wouldn’t otherwise see?
The way I like to think about it, we are problem solvers as technologists. We see a problem, here’s a stakeholder group, here’s the pain point that they have, this is how I’m going to solve it. We have blinders on. Metaphorically, what we need to do is we need to lift our head up and look around the problem. Okay, what are the other stakeholders and communities that are being impacted by this and how? How do they relate to whoever my target audience is?
There are all kinds of wonderful facilitation techniques out there to help us as technologists see outside of our own head. One of my particular favorites is called the tarot cards of tech. They have tarot cards, but they’re posing questions and posing scenarios that will prompt you to think about how someone else might react to your solution or what other impacts might these things have.
I’m quite encouraged at the fact that more organizations are taking this seriously, even if some of it does come from the perspective of compliance. We’re seeing more and more regimes like GDPR, we have accessibility standards, and they’re more or less enforced in different places. Some of it is coming from a compliance aspect, but some of that I think is also coming from this branding aspect. As more of the Gen Z’s come into the talent market, they are expressing, “I don’t want to work for a company that doesn’t actually match my values and live out that particular value statement.”
Responsible tech is the big part of that as well. So I am encouraged.
Shane Hastie: Craig, together we’ve been doing, but you’ve been very much doing a lot of work on ethics. How does that play in here?
Craig Smith: Yeah, I think a couple of things. Slightly segueing back to what Rebecca was talking about, one of the things I’m noticing a lot in organizations is that with all this compliance, a lot of organizations just have their head in the sand. It’s just we have to maintain compliance and we particularly see this in regulated industries like finance and health, where when you talk to a lot of their technical teams, 90% sometimes what they work is just how do we keep the compliance in check?
I think the real challenges for the leadership of those organizations and the organizations in general is how do you then take those things and then innovate within them? Because if you stand back and go, well these particular laws around privacy and security or financial constraints, whatever it is, have been set up for a reason. Whether you agree or disagree, the ones that then innovate around that and go and think about how do I then satisfy the customer? Is the important part as opposed to just how do I satisfy the regulation that comes along? Work within the boundaries that you have being innovative.
In relation into ethical standards that you mentioned there before. For many of us on this call, Rebecca, you’ve been around since the start of this new work with us on the Agile Alliance, as well Shane and I. One of the challenges that you and I were seeing for many years is that there’s been an influx of people who haven’t gone on the same journey as many of us have, whether that be in technical or whether that be in agility. The older of us on the call here, we made the mistakes and righted them. As the industry starts to get older, we don’t remember why we did certain things. The new programmers have never had to go through potentially writing things down to the machine language and understanding what happens at the base, because the compiler or the no-code just takes care of it for them.
In agility, they haven’t been through the why did we go through a whole journey of things like story points, for example? It was because we were trying to solve a problem from way before, but now we’ve moved past things like story points. We’re onto the next step, but they’ve missed the links about why we were there in the first place. When we start talking about ethical type things, it’s how can we make sure that we still understand our base, that we still have this core skill set, but we are operating in an ethical way?
That’s a challenge for lots of organizations, whether it be the work that now you and I have been doing Shane on coaching ethics, or indeed whether you’re talking about programming, testing or any of those other parts of the puzzle that you might be filling.
Shane Hastie: What is the state of play in product management? We’ve had the project to product movement around for a while. We’re certainly seeing it moving to the right on our trends graph, but what is the state of product management?
The state of product management – project to product gaining traction [26:09]
Sharon, maybe you’ve got some thoughts there.
Shaaron A Alvares: Yeah, I think it’s still very slow. There’s a lot of great practices that are getting traction. To draw on what you said about asking the team, I think that’s not happening enough. That’s still not happening downstream. It’s happening upstream, when the product management hand off the requirements, but we still see too much of a silo between product management and then the product owner and the team. Not enough involvement of UX, developers, equality downstream actually. That’s the key challenge I’m seeing that’s still happening now.
There’s a lot of great conversation happening though around discovery. I forgot how it’s called, the three amigos, so it’s getting a little bit of traction but still not enough.
Shane Hastie: Rebecca, what are you seeing in the product management and project to product thinking?
Rebecca Parsons: We’re actually seeing it get more traction definitely. It has not crossed the chasm yet, but people are seeing the value in taking that more user-centered approach and thinking about it more from the perspective of how can I continue to deliver value? As opposed to can I meet this project plan and come in “on time and on budget”?
In fact, why are we building this? If you can’t really answer the question of what the value is of this thing, it doesn’t matter if it was on the project plan, we shouldn’t be building it, if we don’t know what value we’re going to get out of it. I think it’s as much as anything this focus on, we are looking at something that is a product and we are wanting to see how we can increase the value that our end users get from our product.
Value focus is causing this re-evaluation of how do I look at this effort that is now going to go in to create this product? That if I consider it a project, it has an end and it has a scope, whereas a product doesn’t in the same way have that defined scope. It evolves as the value needs of the users evolve or as new users are brought into the fold. I think that in many ways the value mindset is part of what is driving, okay, I need to think about what I am delivering differently. This is easier to talk about in the context of a product life cycle than it is in the context of a project life cycle.
Shaaron A Alvares: In fact, we are seeing a more interest for a value stream. I think organizations are looking more at value stream. I think it ties into the mindset shift between project management, waterfall, and product management. It’s great to see that.
Shane Hastie: That touches on team topologies, team patterns. Raf, I know that this is an area you’re interested in.
Team topologies in rapidly changing environments [29:07]
Raf Gemmail: Going over the topics that we’ve just covered. We’re in this environment where there is this financial pressure, there’s this shift in what teams look like, teams becoming smaller, organizations restructuring on the fly. I think there’s been some intentionality over the last few years to try and set up orgs, where the communications structures enable teams to focus on their value lines.
The fear I have right now is we’re getting into a state where potentially the investment that has been there to try and create longer term efficiencies to enable teams to focus on that product may be compromised by how we restructure organizations. Another thing in there, just commenting on a point that Shaaron made there, was around value streams. Of the last year, I’ve been in a startup, I’m in a very large enterprise now. I could have been right in front of your customer, which I love. Then the customer goes through proxies before the team sees them.
I’ve been trying to get a team to get closer to their customers and it’s really effective, but I found the value streams really helpful both in both contexts actually. To actually draw to teams here, this is why we’re working on this, this is the why. It trickles down to us. When we try and ship software and we continue to monitor in production, this is why it matters and how it feeds back into the loop. Even though we don’t have that closeness that you may have in a startup, you still can try and build some of that empathy. I think that’s really important.
But again, going back to those earlier points, I don’t have that challenge, but I’ve heard of other organizations condensing and bringing teams together as they make mass redundancies. I do wonder what’s going to happen with the team that has way too much cognitive load, that ends up having to manage too many systems. Yeah, we were nicely structured before, we had ownership of these microservices. Now, we’re coming together and we need to know about 20 different things.
I have no data points, so I’ll probably stop there, but there is a level of apprehension as we go through change. How do we preserve these structures that we’ve intentionally put in, to enable us to have good architectures, to have happy teams, to deliver value?
Shane Hastie: Rebecca, reconciling architecture with teams was a point that you raised before we started recording. Do you want to explore that with us a little bit?
Reconciling architecture with teams [31:13]
Rebecca Parsons: When you look at the way, particularly in large enterprises, enterprise architects used to work, it was architecture by decree. We have a very small group that was viewed as overhead and so we had to keep them as small as possible, because that’s what you do with overhead, you try to shrink it and they rule by decree. Part of what I started to try to do, I had a hypothesis actually set many years ago that turned out to be wrong.
My initial hypothesis was that enterprise architecture groups in my experience at that time were generally ineffective. My hypothesis for why was that they didn’t really have a shared understanding about what success looked like. I was giving a workshop at an international software conference, not an Agile conference. This was definitely not an Agile-friendly crowd. There were 53 enterprise architects from around the world. I asked them if they thought they understood what being successful looked like and that that was a shared understanding with everybody.
Everybody put their hand up. It’s like, okay, well that shoots down that hypothesis. I didn’t even get one person to agree with me, but then I asked the follow on question is how many of them felt they were successful? Three people put their hand up. These are clearly not people who were failing at their job, because their companies paid to send them to an international conference. Yet, only three people felt like they were being successful, which told me that the fundamental working model was wrong.
What I started to try to pick apart is what are the things that Agile teams can learn from architects and what are the things that architects need to learn from the teams? I think one of the most important things that the teams need to learn from the architects are what are the things that they’re actually worrying about? Because a software delivery team has one objective. Get business functionality out as quickly and efficiently as possible to delight the users of that software. That’s what they’re there for.
The enterprise architects are there for protecting the overall long-term asset value of the IT estate. Completely different objectives, so it’s not at all surprising that they don’t often see eye to eye. Trying to bridge that gap. One important thing … this was pre-DevOps movement, so this would be a little easier now … but very often the teams didn’t care if their log messages were effective. They didn’t care how easy it was to recover a system or troubleshoot a production problem. That was somebody else’s job. They didn’t know what the enterprise architect was worried about.
Similarly, the enterprise architects, because they’re so vastly outnumbered, because they’re seen as overhead and therefore must be squeezed, they really didn’t understand the reality on the ground. They didn’t know how to get their concerns inserted into that process. That was really where that work started from is how can we get awareness in both directions? What are they actually worrying about and why?
Because at the end of the day, the architects shouldn’t win all the time, but then the development team shouldn’t win all the time either. But you can’t have a conversation, you can’t have that trade off conversation unless there’s shared context. There was not that shared context. That was really the first step.
Shane Hastie: How do we maintain and increase that shared context?
Rebecca Parsons: To me, a lot of it is you have to get the enterprise architects in a position where they are seen as value generating, instead of cost savings, because as soon as you are associated with top line value, you don’t have the same resource squeeze that you do if you’re considered a utility function. Once you can start to get that, you can begin to have the levels of conversation.
I also think by the way, it takes almost a shadow architecture organization, because in a large enterprise with 50,000 developers, you’re not going to be able to have a 1 architect to 10 or 20 developer ratio. There’s still has to be other scaling mechanisms in there, but to me the first thing is to get organizational recognition of the value that you can derive from an enterprise architecture.
In many cases, that means being able to articulate what are the outcomes that I’m trying to achieve with the architectural decisions that I am making? If you focus those things on outcomes that are readily understandable by “the business”, then they are going to start to recognize the value.
In remote environments process and documentation matter more [<a href="javascript:void(0);" onclick="seekTo(35,5335:53<]
Raf Gemmail: I’m keen to share something I’ve seen recently in that space, Rebecca, which is I’ve worked in enterprises which had the enterprise architecture function and seen that mismatch between teams focused on the now and being the person between trying to shepherd them towards the architecture. I’m a big believer in your work and the notion of the emergent architectures, but you’ve got that contention. I’m in an organization now where there’s a really lovely distributed function around democratized function, around looking at that broader architecture.
We’re a family of products which is global. We’re fully remote. This is quite impressive. It’s works and I think it’s works partly because it’s remote, but we move that thinking into more asynchronous processes, RFC processes which are proven and defined most of our standards. Collaborative processes, people providing feedback. Process is probably the word here. One thing I’ve become comfortable with is we’ve always said, “It’s just over,” when people interactions over processes and tools. We said “It’s over,” it’s still important, but there was a bias towards the people interactions.
What I’ve found since I’ve gone remote is that as we set up processes, we set up documentation, people work at their own pace, you set the boundaries that you don’t need to always consciously shepherd. Then people innovate within those boundaries. What I’m seeing, are great architectural decisions, evolution of historic architecture, really powerful conversations that allow teams to get involved, as well as senior engineers resulting in technical outcomes that actually hit backlogs.
It’s an engineering focused culture with a good focus on trying to deliver for product, but I’ve not seen that to this day. It’s working really, really well. I think there may be more evolution to things becoming more asynchronous and slow. I’ve been looking at GitLab and GitLab is very focused around documentation driven cultures, allowing this async to happen. If remote continues the way it has, maybe we’ll move into some of these functions, which are seen as overhead, perhaps being more democratized and shared across teams and across the stakeholders are actually impacted.
Shane Hastie: That’s a really nice segue into what is happening in terms of hybrid, remote, in person. We’re seeing the mix and certainly one of the trends we’ve been looking at is that shift to more asynchronous work.
What do people feel about that?
Hybrid & remote work is now the de facto standard, and it’s still hard [38:12]
Craig Smith: It is now just the de facto standard. I don’t want to say “new normal,” but the thought where everyone throws around. We were moving that way I think the last couple of years anyway, it’s just moved it forward. Again now, it comes back to a segue and a call back to some of the things you talked about earlier, is it starts to show the culture of organizations as to whether they force people back into the office or whether people come together, because it’s the right thing.
Now there are some things, I’m sure we all know where talking face-to-face really does make a difference when you’re really trying to make tough decisions or trying to do things quickly. Yet we didn’t have to get on an airplane to do a recording like this, which even maybe five or six years ago, would’ve been done around a microphone and not in Tools like the ones that we’re using today.
My biggest concern that I’m seeing in my role, though, is that organizations still haven’t truly made this hybrid working option as good as it can be. What worries me is that if we can’t do it through one vendor, and I’ll call Microsoft out here, that if you can’t do it in Teams/Whiteboard/SharePoint, then you can’t do it. There is not one vendor yet that actually has the solution nailed, whether in that market domain or another one.
Organizations still, I believe haven’t all made the switch to tools that really help people work remotely. Rebecca, you were talking about the tools like co-pilot and other things that help engineers. The reality is that once you get outside of the true tech companies, that the people who are just trying to sling code for your bank or insurance company or a small to medium size organization often don’t have the luxuries of those tools, whatever they might be. They’re trying to pull things together.
As a result, they’re the people who are also riding the code that drives our cars and runs our traffic lights and all those kinds of things, which does worry me. Investing in those and making the right decisions is one of the big challenges, whilst also laying on top of that all the challenges that we have in technology, like cybersecurity and other things that go along with it.
You don’t want to have too many tools. The argument that tools like say Miro or Mural, those type of sharing tools is that most organizations don’t want to turn those on, because you can upload a file and drop it onto a board. When you block those things out, it makes those tools less usable, because I need to share an image with Shane in order to make this thing happen.
We’ve still got challenges around how we do this. Again, it comes back a bit to the ethical thing, trusting people to do the work whilst at the same time making sure that we don’t give away all the corporate secrets and throw them into it all the tools.
Shane Hastie: Sharon, you’re in a mega organization that is tackling all of these things. What are you seeing?
Shaaron Alveres: Yeah, speaking about my organization, I want to say that surprisingly we introduced a lot of great practices right at the beginning of the pandemic. We design and rolled out a program that’s called Success from Anywhere. We revised the program, we talked about reviewing priorities, a plan and so on. We called it a “connect from anywhere” when we were in the middle of the pandemic because we realized that working remotely introduced a lot of disconnect between people.
We moved from “success from anywhere” to “connect from anywhere”. We developed a lot of great content to help teams stay connected and also new hires onboard and feel connected with their teams but also with their managers. We also focused a lot on the team working agreement. I would say it’s a small activity, but in this scheme of software development, it’s just a team working agreement, but we realize how important that activity is anytime, but especially when we go through a pandemic. How do we want to connect? How do we want to work together? Because we are completely remote, so we started being 100% remote, but then progressively we introduce some hybrid ways of working. How do we transition from a remote to hybrid? How will we ensure that our teammates who are located, who are not in the US or who are remote still feel connected, included?
I have to say that we’ve done a lot of great work actually. Now, I see a lot of organizations moving to hybrid or 100% back to the office. It’s interesting, because I was making the comparison between what we saw … the bad hybrid and bad return to the office, was which led the great resignation. Now, we are talking about the great layoffs. It’s interesting how events leads to reactions from people, organizations.
Ways of working hybrid and remote is a really, really important topic to solve. I hope that companies are going to keep investing in that because it’s really, really important for the health of the team, for their ability to work together, connect, but also innovate.
Tools can help, but they’re not a panacea [42:52]
Raf Gemmail: I might share from our perspective. I’m in a globally remote team as I said, but my local team, my immediate peers, my first team, based in the same time zone. I’m hiring now and an interesting consideration as I do this is we do async fairly well, but does one open up the local team? That small team trying to keep within Dunbar’s number, does that one keep that communication loop in the same time zone or does one allow that to seep into other time zones?
For now, the focus is teams which are localized, collaborating asynchronously, interfacing each other. I don’t know how that may work for others. I’ve heard of other teams trying to do global remote, but I think you end up with a lot of autonomous people. The collaboration may potentially be compromised, the real time collaboration. We are asynchronously able to communicate with others because … I think we use Slack reasonably well. The things that you can do. I remember Manuel Pias did a talk from some years ago around being intentional about your topologies, your conventions in something like Slack, making the channels discoverable, making sure you didn’t have too much noise.
We have fairly good access to people via Slack. We have the processes, as I said previously that allow us to function asynchronously. Our standups, we have standups currently, four standups which are on a call. One and it may be more later, which is fully async. The async one works really well. People continue with stuff, we talk, but when we have interaction points later in the day, you don’t feel that sense of loss. Some of my teammates, I hadn’t met for months after I joined this context. I felt like I knew them by the time we met in person.
I’ve always been like, “We should get together.” I remember I went to an Alistair Cockburn talk years ago, where I had a distributed team and asked, “How do I get this team over here and this team over here to get together?” He used the word “trust budgets”, for years, I parroted, “We need a trust budget.” It was actually really valuable bringing people together. I still think it is, but our tools have evolved to the stage where it feels first class to have an interaction digitally and to be able to call it and stop.
Obviously, you’ve got the Zoom fatigue and you don’t want to go over, but if you can keep those boundaries right, we can work really effectively remotely. Documentation, source code, pull requests, jumping on calls, swarming where we need to, mobbing where we need to, pairing where we need to, but being intentional and using the tools to our benefit rather than anything else.
Another point, just to Craig’s thing earlier about using these tools, these third party tools, I see tools like Loom, which are really powerful and people use them to share content. Sometimes you’re like, “Oh, has that got something sensitive in it?” It’s a hashed URL, it’s not within the org scope. I think one thing that needs to happen there is being intentional again about what tools that in that organizational context we’re using and managing them intentionally, because security and privacy always is the afterthought. With these, if we’re using them, let’s sign up for licenses. Let’s make them part of the org thing. Let’s put SSO in front of it, let’s stick guidance in front of those.
Shane Hastie: Rebecca, how is Thoughtworks handling this? You’re massive, global and you’re dealing with thousands of different customers as well.
There is no one-size-fits-all approach and you can’t get away from time zones [45:55]
Rebecca Parsons: Yeah, and it varies by country, it varies by client. In places like North America and Australia and Germany, our clients are not saying, “You need to start coming back into our offices five days a week.” In fact, ironically we have more of our individual client people working in our offices, because we do have all of our offices open now. We are not requiring people to come into the office. Certain countries are trying to encourage that. Other countries have said, “We’re going to accept that we have people who will always be fully remote.”
We have, what I would say is lots of different experiments going on across the company on how to do things. One thing, and this is just probably because I spend so much of my time working with global groups, I’m exposed to it more. The one thing that you can’t get away from is time zones. That’s why I think this emphasis on can we look at some of these processes and make them more asynchronous is so important. But for those things that really do require that co-creation that was spoken of earlier, you can’t get away from time zones.
I’ve had people say to me, “There will never again be a global meeting.” And I said, “Well, actually it’s dangerous to use the ‘N word’ anytime. But no, you can’t get away from time zones.” There are going to be things. I think a lot of what we’re going through at the moment is identifying these are the kinds of activities that we can effectively do asynchronously. These are the kinds of activities that should be synchronous, but can still be remote first. These are things that you really have to not only do synchronously, but you’ve got to be co-located, because the exchange needs to be that rich. I think we’re looking at many of our different activities and this is what it takes to do this and this is what it takes to do that.
One final thing that I found interesting, we did experiments again across our development community on remote pairing tools and there wasn’t a winner. Different people would swear, “This is the most wonderful tool available.” Another group would swear, “No, this is the most wonderful tool available and that thing is horrible.”
I think one of the things that we are going to also have to grapple with is, it’s one thing to tell somebody, “Okay, I’m pairing with you Shane, and we’re sitting next to each other and we need to be able to swap keyboards.” There’s a good compelling reason to make sure there’s standardization. As we get further and further away, the needs for, okay, everybody needs to use the same too,. well, the computation is different in that context. I think we’re going to be seeing a lot of innovation in our ways of working as we sort out how to do these different activities.
Raf Gemmail: I’m hoping Rebecca, at some stage I get to pair with people in virtual reality. I’ve got a way of exporting monitors into here, a virtual setup. I can be floating in space and working. I did a demo for some people at work, but I think we’re still in that stage, where there’s a lot of skepticism. And Meta gets a lot of bad rep, because the images that are shown look rudimentary, but I’ve tried out their Work Spaces product. When you’re in, it’s a collaborative thing. The whiteboards, they’re other things I’ve used, but I’m still hopeful we’ll get to the stage where some of the collaboration is a bit more immersive.
Rebecca Parsons: But you still can’t get away from time zones.
Raf Gemmail: You can’t get away from time zones. Oh, one more thing to thread though is that we talked about trust in the team’s building cohesion. As a remote person, it’s really easy to feel or to be worried that you’re going to feel excluded, but we’re doing things like painting events remotely. Sibling team did a cooking event. The games and all sorts of things which have really, I’d say for people are trying to do the remote, make sure you do the social aspects as well, because it builds cohesion, it’s fun. I’ve received in the post canvases and paints.
Awesome! I can’t paint, but by the end of it, I had something and I did it with my team.
Shane Hastie: We have come close to the end of our time box, so we’ll do a quick whip around. Hopes and wishes for 2023 and beyond. Shaaron, can I start with you?
Hopes and wishes for 2023 and beyond [49:59]
Shaaron A Alvares: I really hope if I had a magic wand, I would want all organization to support our teams, our Agile projects, our development teams through the recession, to the priority of productivity and high performance. I think it’s not just about productivity and high performance. I hope we’re not going to stop looking at wellness, mental strength, and also psychological safety. That would be my wish.
Shane Hastie: Raf, your turn.
Raf Gemmail: My hope for this year is that, to echo Shaaron, that we see a rebalancing in how we work. After this right sizing that’s going on in the industry. It settles, my hope is that humanism is still valued and that we work together and we value working together as people. The dollar target will always be there, but how we create the teams that are going to achieve that and deliver to our org and stay within our org, remains the focus.
My hope is that we remain responsible in how we use tools. We were talking about ChatGPT, and it’s going to come regardless. I’m hoping as we embrace this new technology, we’re responsible as to how we use it, how it impacts our teams, how it impacts our planning. I’m hoping we see innovation beyond looking to a few solutions that have come from a few models and we see innovation from other companies trying to localize and train and create models from corpus’ of data for their organizations and context.
I’m hopeful we get to the end of the year after a few rough years (we just had a cyclone in New Zealand) which I’m getting out of and we see a pathway to 2024, which is good for the whole planet actually. A good thing I’ve shared to finish is I saw recently a friend of mine got a technical certification. Now, there’s so many of them out there, but it was a technical certification around green ethical development. I thought that was brilliant to see. I’m hoping we have that more as a focus going forward.
Shane Hastie: Craig.
Craig Smith: Well, Shane, I asked ChatGPT what my hopes are for Culture and Methods were in 2023. It told me that as an AI language model, it doesn’t have access to my personal hopes and aspirations. That’s a good start, but what I hope not to see is that as we have these challenges Ben was just talking about and then everyone was just talking about, is that we resist the pull of the past. That there’s been a lot of good work over the last few years for individuals and organizations to embrace new ways of working and moving technology forward. When things start to become unstable, we tend to often batten down the hatches. I’m seeing that in organizations that we talked about product before. Yeah, it’s like, no, let’s tighten up purse strings and now do a lot of upfront analysis and go back to old project methods.
You could use a lot of different things like that, the way we structure teams, because we’re talking about all the layoffs and things is just let’s just chop off the left arm and remove innovation and all those things. I think the individuals and teams that are going to win are the ones that continue to push forward and continue to innovate. That’s my hope for our listeners, as we move forward is that we still have to chip away the basics.
When you work with organizations like Thoughtworks and things that you can be lulled sometimes in a false sense that all developers are great and they’re all using lots of tools and that DevOps is ruling the world. That isn’t in a lot of places, but when you actually get to the rats and mice of things like government and large corporates, we’re still doing things like we were doing them 40 years ago. We’re making slow inroads, but we still have to chip away at all of those basics, because those organizations have to keep at the same pace as everybody else. If not, we all get dragged backwards. I hope that the people in organizations continue to innovate.
Shane Hastie: And Rebecca.
Rebecca Parsons: A couple of unrelated things. One thing I hope for is that we can continue the move towards more user-centered development, that we can continue to focus on what is the value that we’re actually delivering? Use that to guide our decision making, as opposed to re-trenching too far into, oh, I’ve got to go back into cost cutting. I’ve got to go back into lockdown of my systems, because expense. I think there are some forces in the world that are pushing that direction, because the changes to those expectations of our users are not going to slow down.
Organizations are going to have to continue to respond. I have some hope on that. A second, although this is a pipe dream, but I really wish that our industry could get away from the belief in the one true way or the silver bullet, or, oh yes, if I apply this, a miracle will occur. We still, as an industry have not accepted that there is a lot of actual complexity in the systems that we are building. Yes, we have accidental complexity and we want to continue to chip away at that, but there is essential complexity in there as well. We have to accept that. There isn’t going to be this magic package that you can throw at a complex problem and have it all go away.
Finally, I hope we can continue to raise awareness across the industry of the importance of, in particular, security and privacy. Because there’s still so much low hanging fruit out there for the hackers to exploit and we’re all human beings. We are going to make mistakes, but if we can continue to raise the awareness, find new tools to automate, particular kinds of scans, to reduce exposure, whatever we have to do, but as an industry, I believe we need to take that more seriously. I hope we continue to advance on that journey.
Shane Hastie: For myself, I would echo pretty much everything that you have said and add the wonderful opportunity that it is to work with such a great group of people in the culture and method space. Thank you all so much for the contributions over the last many years.
Rebecca, thank you for joining us as our special guest today.
Rebecca Parsons: Thank you for having me.
EDITORIAL NOTE:
When we recorded this podcast we were also joined by Ben Linders, however a technical glitch meant the file with his voice recording did not get saved so we lost his contribution. Watch for a future podcast where Ben and Shane discuss their views on the current and future state of culture and methods.
.
From this page you also have access to our recorded show notes. They all have clickable links that will take you directly to that part of the audio.