How the industrial monitoring systems vendor selected the best database for its real-time machine learning environment.
May 31st, 2023 6:10am by
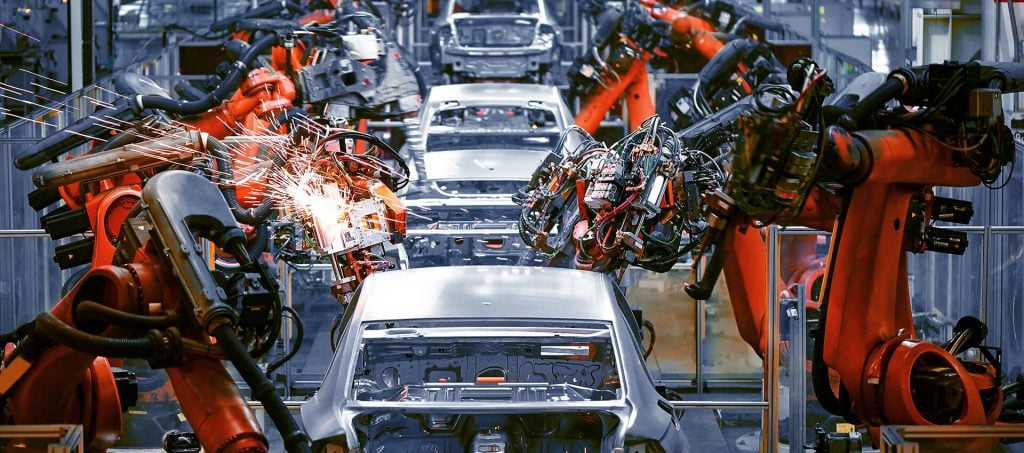
Tractian is a machine intelligence company that provides industrial monitoring systems. Last year, we faced the challenge of upgrading our real-time machine learning (ML) environment and analytical dashboards to support an aggressive increase in our data throughput, as we managed to expand our customers database and data volume by 10 times.
We recognized that to stay ahead in the fast-paced world of real-time machine learning, we needed a data infrastructure that was flexible, scalable and highly performant. We believed that ScyllaDB would provide us with the capabilities we lacked, enabling us to push our product and algorithms to the next level.
But you probably are wondering why ScyllaDB was the best fit. We’d like to show you how we transformed our engineering process to focus on improving our product’s performance. We’ll cover why we decided to use ScyllaDB, the positive outcomes we’ve seen as a result and the obstacles we encountered during the transition.
How We Compared NoSQL Databases
When talking about databases, many options come to mind. However, we started by deciding to focus on those with the largest communities and applications. This left three direct options: two market giants and a newcomer that has been surprising competitors. We looked at four characteristics of those databases — data model, query language, sharding and replication — and used these characteristics as decision criteria for our next steps.
First off, let’s give you a deeper understanding of the three databases using the defined criteria:
MongoDB NoSQL
- Data model: MongoDB uses a document-oriented data model where data is stored in BSON (Binary JSON) format. Documents in a collection can have different fields and structures, providing a high degree of flexibility. The document-oriented model enables basically any data modeling or relationship modeling.
- Query language: MongoDB uses a custom query language called MongoDB Query Language (MQL), which is inspired by SQL but with some differences to match the document-oriented data model. MQL supports a variety of query operations, including filtering, grouping and aggregation.
- Sharding: MongoDB supports sharding, which is the process of dividing a large database into smaller parts and distributing the parts across multiple servers. Sharding is performed at the collection level, allowing for fine-grained control over data placement. MongoDB uses a config server to store metadata about the cluster, including information about the shard key and shard distribution.
- Replication: MongoDB provides automatic replication, allowing for data to be automatically synchronized between multiple servers for high availability and disaster recovery. Replication is performed using a replica set, where one server is designated as the primary member and the others as secondary members. Secondary members can take over as the primary member in case of a failure, providing automatic fail recovery.
ScyllaDB NoSQL
- Data model: ScyllaDB uses a wide column-family data model, which is similar to Apache Cassandra. Data is organized into columns and rows, with each column having its own value. This model is designed to handle large amounts of data with high write and read performance.
- Query language: ScyllaDB uses the Cassandra Query Language (CQL), which is similar to SQL but with some differences to match the wide column-family data model. CQL supports a variety of query operations, including filtering, grouping and aggregation.
- Sharding: ScyllaDB uses sharding, which is the process of dividing a large database into smaller parts and distributing the parts across multiple nodes (and down to individual cores). The sharding is performed automatically, allowing for seamless scaling as the data grows. ScyllaDB uses a consistent hashing algorithm to distribute data across the nodes (and cores), ensuring an even distribution of data and load balancing.
- Replication: ScyllaDB provides automatic replication, allowing for data to be automatically synchronized between multiple nodes for high availability and disaster recovery. Replication is performed using a replicated database cluster, where each node has a copy of the data. The replication factor can be configured, allowing for control over the number of copies of the data stored in the cluster.
PostgreSQL
- Data model: PostgreSQL uses a relational data model, which organizes data into tables with rows and columns. The relational model provides strong support for data consistency and integrity through constraints and transactions.
- Query language: PostgreSQL uses structured query language (SQL), which is the standard language for interacting with relational databases. SQL supports a wide range of query operations, including filtering, grouping and aggregation.
- Sharding: PostgreSQL does not natively support sharding, but it can be achieved through extensions and third-party tools. Sharding in PostgreSQL can be performed at the database, table or even row level, allowing for fine-grained control over data placement.
- Replication: PostgreSQL provides synchronous and asynchronous replication, allowing data to be synchronized between multiple servers for high availability and disaster recovery. Replication can be performed using a variety of methods, including streaming replication, logical replication and file-based replication.
What Were Our Conclusions of the Benchmark?
In terms of performance, ScyllaDB is optimized for high performance and low latency, using a shared-nothing architecture and multithreading to provide high throughput and low latencies.
MongoDB is optimized for ease of use and flexibility, offering a more accessible and developer-friendly experience and has a huge community to help with future issues.
PostgreSQL, on the other hand, is optimized for data integrity and consistency, with a strong emphasis on transactional consistency and ACID (atomicity, consistency, isolation, durability) compliance. It is a popular choice for applications that require strong data reliability and security. It also supports various data types and advanced features such as stored procedures, triggers and views.
When choosing between PostgreSQL, MongoDB and ScyllaDB, it is essential to consider your specific use case and requirements. If you need a powerful and reliable relational database with advanced data management features, then PostgreSQL may be the better choice. However, if you need a flexible and easy-to-use NoSQL database with a large ecosystem, then MongoDB may be the better choice.
But we were looking for something really specific: a highly scalable and high-performance NoSQL database. The answer was simple: ScyllaDB is a better fit for our use case.
MongoDB vs. ScyllaDB vs. PostgreSQL: Comparing Performance
After the research process, our team was skeptical about using just written information to make a decision that would shape the future of our product. We started digging to be sure about our decision in practical terms.
First, we built an environment to replicate our data acquisition pipeline, but we did it aggressively. We created a script to simulate a data flow bigger than the current one. At the time, our throughput was around 16,000 operations per second, and we tested the database with 160,000 operations per second (so basically 10x).
To be sure, we also tested the write and read response times for different formats and data structures; some were similar to the ones we were already using at the time.
You can see our results below with the new optimal configuration using ScyllaDB and the configuration using what we had with MongoDB (our old setup) applying the tests mentioned above:
MongoDB vs. ScyllaDB P90 Latency (Lower Is Better)
MongoDB vs. ScyllaDB Request Rate/Throughput (Higher Is Better)
The results were overwhelming. With similar infrastructure costs, we achieved much better latency and capacity; the decision was clear and validated. We had a massive database migration ahead of us.
Migrating from MongoDB to ScyllaDB NoSQL
As soon as we decided to start the implementation, we faced real-world difficulties. Some things are important to mention.
In this migration, we added new information and formats, which affected all production services that consume this data directly or indirectly. They would have to be refactored by adding adapters in the pipeline or recreating part of the processing and manipulation logic.
During the migration journey, both services and databases had to be duplicated, since it is not possible to use an outage event to swap between old and new versions to validate our pipeline. It’s part of the issues that you have to deal with in critical real-time systems: An outage is never permitted, even if you are fixing or updating the system.
The reconstruction process should go through the data science models, so that they can take advantage of the new format, increasing accuracy and computational performance.
Given these guidelines, we created two groups. One was responsible for administering and maintaining the old database and architecture. The other group performed a massive reprocessing of our data lake and refactored the models and services to handle the new architecture.
The complete process, from designing the structure to the final deployment and swap of the production environment, took six months. During this period, adjustments and significant corrections were necessary. You never know what lessons you’ll learn along the way.
NoSQL Migration Challenges
ScyllaDB can achieve this kind of performance because it is designed to take advantage of high-end hardware and very specific data modeling. The final results were astonishing, but it took some time to achieve them. Hardware has a significant impact on performance. ScyllaDB is optimized for modern multicore processors and uses all available CPU cores to process data. It uses hardware acceleration technologies such as AVX2 (Advanced Vector Extensions 2) and AES-NI (Advanced Encryption Standard New Instructions); it also depends on the type and speed of storage devices, including solid-state disks and NVMe (nonvolatile memory express) drives.
In our early testing, we messed up some hardware configurations, leading to performance degradation. When those problems were fixed, we stumbled upon another problem: data modeling.
ScyllaDB uses the Cassandra data model, which heavily dictates the performance of your queries. If you make incorrect assumptions about the data structures, queries or the data volume, as we did at the beginning, the performance will suffer.
In practice, the first proposed data format ended up exceeding the maximum size recommended for a ScyllaDB partition in some cases, which made the database perform poorly.
Our main difficulty was understanding how to translate our old data modeling to one that would perform on ScyllaDB. We had to restructure the data into multiple tables and partitions, sometimes duplicating data to achieve better performance.
Lessons Learned: Comparing and Migrating NoSQL Databases
In short, we learned three lessons during this process: Some came from our successes and others from our mistakes.
When researching and benchmarking the databases, it became clear that many of the specifications and functionalities present in the different databases have specific applications. Your specific use case will dictate the best database for your application. And that truth is only discovered by carrying out practical tests and simulations of the production environment in stressful situations. We invested a lot of time, and our choice to use the most appropriate database paid off.
When starting a large project, it is crucial to be prepared for a change of route in the middle of the journey. If you developed a project that did not change after its conception, you probably didn’t learn anything during the construction process, or you didn’t care about the unexpected twists. Planning cannot completely predict all real-world problems, so be ready to adjust your decisions and beliefs along the way.
You shouldn’t be afraid of big changes. Many people were against the changes we were proposing due to the risk it brought and the inconvenience it caused to developers (by changing a tool already owned by the team to a new tool that was completely unknown to the team).
Ultimately, the decision was driven based on its impact on our product improvements — not on our engineering team, even though it was one of the most significant engineering changes we have made to date.
It doesn’t matter what architecture or system you are using. The real concern is whether it will be able to take your product into a bright future.
This is, in a nutshell, our journey in building one of the bridges for the future of Tractian’s product. If you have any questions or comments, feel free to contact us.
Created with Sketch.