Month: April 2022
Uncategorized
On Go's Generics Implementation and Performance
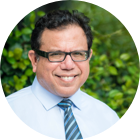
MMS • Sergio De Simone

On the heels of Go generics becoming stable in Go 1.18, PlanetScale performance engineer Vicent Martí dissected how they work and highlighted some performance limitations of their actual implementation. He also provided a few handy suggestions about their usage.
One key characteristics of Go generics implementation is they only partially use monomorphization, a technique used in languages like C++, D, or Rust to compile generic code. In a nutshell, monomorphization consists in replicating a function’s implementation to specialize it for distinct types. Monomorphization is usually faster than inheritance-based polymorphic code, at the expense of compilation time and binary size. In fact, monomorphization ensures zero-overhead calls, while inheritance-based polymorphism requires the use of indirect pointers through virtual dispatch tables. Additionally, monomorphized code can be specifically optimized and/or inlined by the compiler.
In Go, says Martí, monomorphization is only partially applied using a technique called “GCShape stenciling with Dictionaries”. The main effect of this is that all arguments of pointer types or interfaces are treated as belonging to the same underlying type, which means only one monomorphic version of that function is generated. Contrast this with functions taking arithmetic arguments, such as int32
and float64
, each of which gets its own specialized function version.
There’s no incentive to convert a pure function that takes an interface to use Generics in 1.18. It’ll only make it slower, as the Go compiler cannot currently generate a function shape where methods are called through a pointer.
Martí uses PlanetScale’s Vitess open-source database to test out how introducing generics may impact a complex, real-life application. He goes down to assembly-level to study how the code generated by the Go compiler looks and make his argument. Additionally, he carries some micro-benchmarking through to measure exactly how slower generics can be in a best-case scenario. In a more realistic scenario, though, things can get worse.
In an actual production service there is cache contention, and the global itabTable can contain from hundreds to millions of entries […]. This means that Generic method call overhead in your Go programs will degrade with the complexity of your codebase.
Do not miss Martí’s highly-detailed and argumented analysis if you are interested in the fine details of his reasoning.
At the end of his analysis, Martí provides a few suggestions about the use of generics in Go 1.18. Specifically, he discourages attempting to de-virtualize or inline method calls, passing an interface to a generic function, and rewriting interface-based APIs to use generics. On the contrary, generics seem promising to de-duplicate methods that take string
and []byte
, with generic data structures, and with callback arguments.
However, there is nothing in Go generics specification that prevents all of this from being improved in future versions with a different choice of trade-offs. Only you might want to know where the potential risks lie and apply generics incrementally to your codebase without forgetting to measure your performance.