Month: January 2020
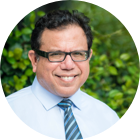
MMS • Guy Nesher
Article originally posted on InfoQ. Visit InfoQ
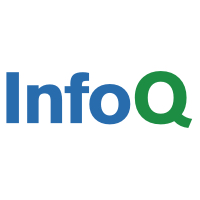
Raspberry Pi is a series of small single-board computers that are sold at a fraction of the price of a standard desktop computer and are widely used as media centers, retro gaming machines, low desktops, etc.
However, the Raspberry Pi also comes with a set of GPIO or ‘General Purpose Input Output’ pins that enables developers to connect and control external electronic devices such as sensors, motors, etc.
The exact number and role of the pins changes between individual models but is generally divided into power, ground, and general-purpose pins.
The power and ground pins are not programmable. The power pin supplies a constant 3.3V/5V power to the circuit while the ground pin is used to connect to the cathode of the circuit.
On the other hand, the general-purpose pins are programmable and can be used in either an output or input mode. When set to output mode, the pins provide a constant 3.3V power that can be turned on or off. When set to input mode, the pin reads the current supplied by the circuit and returns a Boolean value indicating if it receives 3.3V power or not.
Of course, these capabilities aren’t new and have been widely available to developers through microcontrollers such as Arduino or NodeMCU. However, these devices generally came with limited memory and computing power and required the use of particular programming languages.
The Raspberry Pi, on the other hand, supports a more robust CPU that is capable of running Linux and supports NodeJS, allowing JavaScript developers to use their existing skillset and build sophisticated devices with relative ease.
To interact with the GPIO pins, we use a NodeJS module called onoff that provides simple access to the individual pins.
The equivalent of a ‘hello world’ demo in the world of microcontrollers is a blinking led. Most of the code in the example below should already be familiar to JavaScript developers.
const Gpio = require('../onoff').Gpio;
const led = new Gpio(17, 'out');
After requiring the module, we define the roles of the pins we wish to interact with. The number identifies the pin on the board, followed by determining if the pin is used to read (‘in’) or write (‘out’).
In this example, we defined a pin called led, assigned it to physical pin 17 and set it to write mode (‘out’)
const blinkInterval = setInterval(blinkLED, 500);
function blinkLED() {
if (led.readSync() === 0) {
led.writeSync(1);
} else {
led.writeSync(0);
}
}
Now all that’s left is to make our led blink by creating a 500ms interval and turning the led on/off based on its current state.
setTimeout(() => {
clearInterval(blinkInterval);
led.writeSync(0);
led.unexport();
}, 5000);
Assuming we do not wish to continue blinking the LED indefinitely, we would also need to clean up at the end. In this example, we will wait 5 seconds before clearing our interval, turning off the led, and releasing its resource.
Of course, the Raspberry Pi can do more than just blinking LEDs. Developers have built anything from drones to Raspberry Pi skateboards. While not specifically written for JavaScript, you can find many ideas for exciting projects of all levels at the Raspberry Pi website
Uncategorized
Thomas Bull Sells 415 Shares of Mongodb Inc (NASDAQ:MDB) Stock
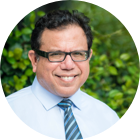
MMS • RSS
Posted on mongodb google news. Visit mongodb google news
Article originally posted on mongodb google news. Visit mongodb google news
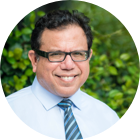
MMS • Greg Law
Article originally posted on InfoQ. Visit InfoQ
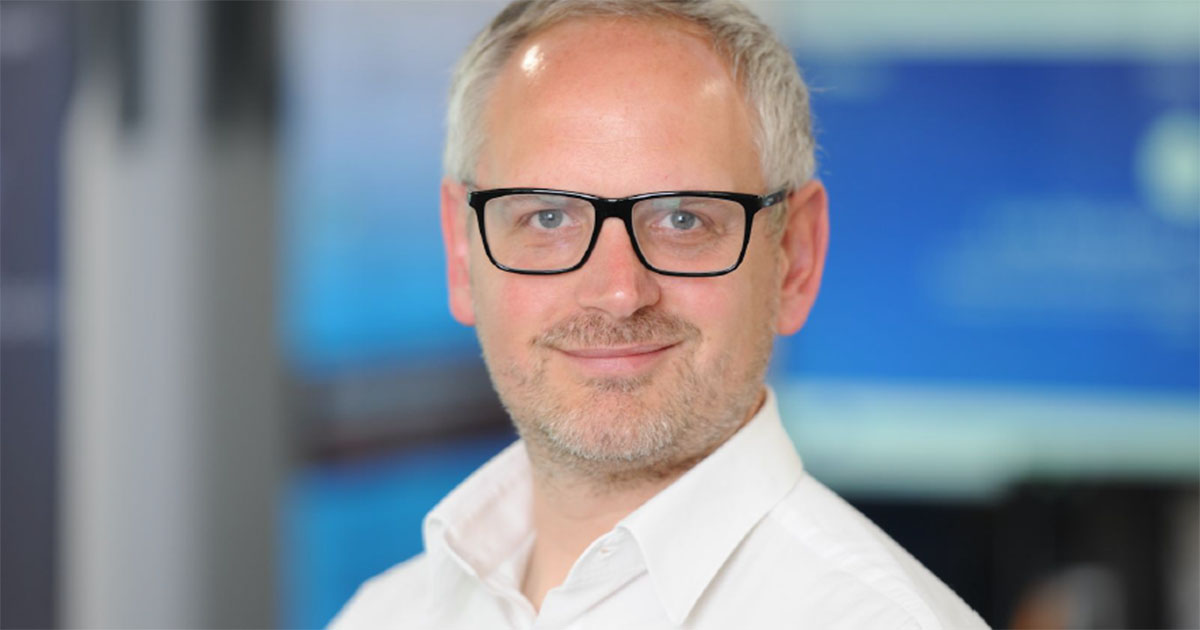
In this podcast, Daniel Bryant sat down with Greg Law, CTO at Undo. Topics discussed included: the challenges with debugging modern software systems, the need for “hyper-observability” and the benefit of being able to record and replay exact application execution; and the challenges with implementing the capture of nondeterministic system data in Undo’s LiveRecorder product for JVM-based languages that are Just-In-Time (JIT) compiled.
Key Takeaways
- Understanding modern software systems can be very challenging, especially when the system is not doing what is expected. When debugging an issue, being able to observe a system and look at logging output is valuable, but it doesn’t always provide all of the information a developer needs. Instead we may need “hyper observability”; the ability to “zoom into” bugs and replay an exact execution.
- Being able to record all nondeterministic stimuli to an application — such as user input, network traffic, interprocess signals, and threading operations — allows for the replay of an exact execution of an application for debugging purposes. Execution can be paused, rewound, and replayed, and additional logging data can be added ad hoc.
- Undo’s LiveRecorder allows for the capture of this nondeterministic data, and this can be exported and shared among development teams. The UndoDB debugger, which is based on the GNU Project Debugger, supports the loading of this data and the execution and debugging in forwards and reverse execution of the application. There is also support for other debuggers, such as that included within IntelliJ IDEA.
- Advanced techniques like multi-process correlation reveal the order in which processes and threads alter data structures in shared memory, and thread fuzzing randomizes thread execution to reveal race conditions and other multi-threading defects.
- The challenges of using this type of technology when debugging (micro)service-based application lies within the user experience i.e. how should the multiple process debugging experience be presented to a developer?
- Live Recorder currently supports C/C++, Go, Rust, Ada applications on Linux x86 and x86_64, with Java support available in alpha. Supporting the capture and replay of data associated with JVM language execution, which contain extra abstractions and are often Just-In-Time (JIT) compiled, presented extra challenges.
Subscribe on:
Show Notes
Can you introduce yourself and what you do? –
- 01:20 I’m Greg Law, co-founder and CTO at undo.io, and we have technology to record application execution so that developers can see what the program did.
Can you provide an overview of live recorder product, and what problems it solves? –
- 01:55 The problem is one of observability; modern applications are complex, executing billions of instruction per second.
- 02:15 If you add multiple threads, multiple processes and multiple nodes, the complexity is staggering.
- 02:20 When anything goes ‘wrong’ – anything you weren’t expecting or hoping for – like an unhandled exception, or a suboptimal customer experience – understanding what’s happened is borderline impossible.
- 02:40 It’s the ultimate needle in a haystack exercise to figure out what’s happened.
- 02:50 The approach we take with live recorder is to say: let’s not try to guess what bits of information we need are, let’s record everything.
- 03:00 If we record everything down to the machine level, you can after the event decide which are the interesting bits you want to look at.
- 03:05 When debugging, or investigating program behaviour, what you nearly always do is turn to the logs.
- 03:15 Maybe you’ll have something through old-fashioned printf-style logs, or you might have a fancier approach available these days.
- 03:30 If you ask the question: how often, when you turned to the logs, do you have all the information that you need to root-cause and diagnose the problem?
- 03:40 Sometimes – but that’s a good day, right? Nearly always, you will have something that gives you a clue of something that doesn’t look right.
- 03:50 You have to pull on that thread, and find another clue – and go on a cycle of the software failing many times – typically more than ten or hundreds in times before you solve it.
- 04:15 Live recorder takes a different approach: record the application once, and then spend as long as you need looking at the recording pulling out the information piece by piece.
What type of data is recorded? –
- 04:35 What we offer is the ability to wind back to any machine instruction that was executed, and see any piece of state; register value, variable in the program – from machine level to program.
- 04:50 Clearly, there’s billions of instructions executed every second, and it’s not practical to store all of the information.
- 05:00 This idea of replay or time travel debugging have academic papers going back to the 1970s trying to do this.
- 05:15 Up until recently, they would try to record everything – which would work for a ‘hello world’ but wouldn’t work on a real world application.
- 05:30 The trick is to record the minimum that you need in order to be able to recompute any previous state, rather than store everything.
- 05:35 You need to be able to record all the non-deterministic stimuli to your program.
- 05:40 Computers are deterministic – if you run a program multiple times, and you give it exactly the same starting state each time, it will always do the same thing.
- 05:50 This is why random numbers are difficult to generate.
- 05:55 We can use that to our advantage; computers are deterministic – until they are not.
- 06:00 There are non-deterministic inputs into a program’s state: the simplest might be some user input, if they type in text into a field – so we have to capture that, along with networking, thread scheduling.
- 06:30 While there’s a lot of that stuff, it’s a tiny fraction of what the computer is doing.
- 06:35 99.99% of the instructions that execute at the machine level are completely deterministic.
- 06:45 If I add two numbers together, then I should get the same result each time – otherwise I’m in trouble.
- 06:50 We went through some JIT binary translation of the machine code as it is running, and we’re able to snoop any of those non-deterministic things that happen, and save it into a log.
- 07:00 The result is, you can capture a recording of your program on one machine, take it to a different machine with a different OS version, and guarantee that it’s going to exactly the same thing.
- 07:30 This allows you to go through a test and try again debug cycle which you typically do in a development cycle much tighter – and in addition, some tools to navigate that information.
- 07:40 For example, if you have state – a variable whose value is wrong – I can put a watchpoint on “a”, and go back in time until that value is changed.
- 08:00 You can then determine why “a” has been changed to an invalid value and find the line of code where that happened.
- 08:15 We literally have cases of customers that have been struggling with nasty bugs for months, if not years, and they can be nailed with this tech in a few hours.
- 08:30 In a sense, that’s a big things that gets the headlines – but the bigger things is that most of the software development process to those tedious afternoon debug sessions.
- 08:50 If you could get each one of those done in 10 minutes, then it would be a huge boost to productivity, developer velocity, software reliability and quality.
So how does the undo debugger work? –
- 09:10 To go into the details of it, we have a technology that will capture the execution of a Linux application at the binary level – so we don’t care what the original language was.
- 09:25 Then, we can take that recording, rewind, step through it – but you need a means of displaying that recording.
- 09:35 The way we typically (but not exclusively) do that is by stepping through a source-level debugger that the developers understand.
- 09:45 There’s a GDB interface on top of this – other debuggers are available for Fortran, COBOL – it turns out that there’s lots of COBOL code out there, but the original developers are no longer working in the field.
- 10:10 We have just released an alpha version of our Java debugger, with IntelliJ support, with the release early in 2020.
- 10:15 What we’re trying to do is to not produce a new debugger, but provide debuggers with the ability to view these recordings in a useful way.
- 10:30 We give you features that allow you to step back a line, or rewind to a certain point or a watchpoint when a value was changed.
- 10:40 Also coming out in Q1 2020, we have something coming out called postmortem logging.
- 10:45 It’s the ability to take a recording, and add some log statements, to find out how often things happen.
- 11:00 You can then replay with the logging information to see what it would have looked like, or if you could have caught it earlier.
- 11:05 Sometimes the debugger is the right thing, but sometimes logs are a better depending on what problems you are trying to solve.
- 11:15 You shouldn’t think it as a fancy new debugger, but rather as a way of getting hyper observability into program execution, and one of the interfaces onto that is a debugger.
What is the developer workflow to finding a bug in production? –
- 11:40 The first thing you’ve got to do is capture it; you’ve got to record it in the act.
- 11:45 This isn’t meant to be something that you turn on recording all the time, just in case something goes wrong – it’s a bit heavyweight for that.
- 11:55 The mode is: I’ve got a problem, it’s happening multiple times a week – so then I need to enable the recording.
- 12:10 We’ve got multiple ways of doing that – either an agent which can record a process on the machine, or you can link against a library that we supply and have an API onto that.
- 12:20 Let me give you a concrete example of a customer that we’re working with at the moment doing just this.
- 12:25 Mentorgraphics have design and simulation software, supplied to the big chip manufacturers.
- 12:35 This is cutting edge – people doing 7nm designs, that kind of thing.
- 12:45 When a customer – typically a chip design firm – has a problem, that they can reproduce on their system – they can go into their software, and click a checkbox to start recording.
- 13:05 The Mentorgraphics program can then spit out a recording, which can be sent to a Mentorgraphics engineer for further information.
- 13:10 It’s in production, not just in a system that you control, but as a product which is running on a customer system, and it still works there.
- 13:20 To take another example, in test and CI, the premise is that you’re running these tests and they’re always running green.
- 13:35 The theory is that when something goes red or non-deterministically fails.
- 14:00 A lot of our customers will have their flaky, sporadic, or intermittent tests failures (people call them different things) and that subset are running with recording all the time.
- 14:05 Now when you get a test failure, with your artefacts in your CI system you will have not only the logs that you usually have, but one of these recordings as well.
- 14:20 This allows you to replay the failure seen in CI without having to reproduce it exactly.
What languages are supported? –
- 14:45 Right now, it’s C, C++, Go as the main languages, though there are Fortran, COBOL (which are slightly niche) – and Java will be in beta in early 2020.
- 15:00 The tech is fairly machine agnostic, but people don’t want to debug in x86 instructions, they want some source level debugging adapter.
- 15:15 Expect to see JavaScript, Scala, Kotlin etc. as time goes on.
Were there any challenges with recording the JVM? –
- 15:30 The reason that we did the compiled languages C, C++ and Go, first is because the adapter that we needed for the source level debugging is much closer.
- 15:40 When you’re debugging C code, you’re much closer to x86.
- 15:45 Java is definitely the worse in that regard, not just because it’s abstracted away in the JVM, but there’s a whole bunch of assumptions in the JVM around what debugging looks like.
- 15:55 In particular, when running Java code, you can run it in interpreted mode or in compiled JIT-ted mode with C1 or C2 or using Graal.
- 16:05 Mapping back from JITted Java code to source line information is something that no-one seems to have thought about.
- 16:10 If you’re inside IntelliJ, and you put a breakpoint on a line, that method will always be run in interpreted mode – so it won’t be in the JIT.
- 16:30 It’s reasonable and understand why the JVM architects decided that was the right design, because you know what lines of code have breakpoints on them.
- 16:40 Here, you don’t – you are going to put the breakpoint on a line of code when the code executed in the past – maybe an hour, maybe a month ago.
- 16:50 When the code executes, you don’t know if a breakpoint is needed on this line or not.
- 17:00 That was a challenge to work around, and provide some hooks which would allow us to solve that problem.
- 17:05 It was tough – but it was the kind of challenges that we’re used to, as opposed to the core technology of capturing all this non-deterministic information and replaying it perfectly.
- 17:20 It wasn’t the biggest challenge that we faced, but it did have its own special challenges.
Isn’t the JIT code generation non-deterministic itself? –
- 17:35 That bit is OK for us, because we provide completely faithful replay of your application.
- 17:45 So whatever data the JVM relied on to make that decision of whether or not to JIT the thing, that’s going to look the same on the replay and the JVM is going to make the same decision.
- 17:55 Ultimately it’s all just x86 (or ARM) instructions, down at the bottom.
- 18:00 The JVM – to us – is just an x86 application, and we replay that completely deterministically and faithfully.
- 18:05 The problem is when you’re trying to get that observability into a process that was a JVM and application on top of it – extracting out that process that the developer is interested in.
- 18:35 We don’t have to do all of it – the regular Java debuggers are good at giving you information when you’ve got layers of Java code.
- 18:45 It’s when you get to the layers below the Java code, and you have to translate between the two, that it gets confusing.
- 18:50 Having done this, it gives you nice properties – if you have JNI code linked in to your application, guess what – we’ve captured that as well, and you can debug this in your C++ debugger.
What use cases are multi-process correlation and thread fuzzing used for? –
- 19:20 These days it’s unusual for an application to be completely monolithic, single threaded, running a bunch of statements outputting an answer.
- 19:30 For example, a compiler will typically run like that, but that’s mostly the exception to the rule.
- 19:40 In the vast majority of applications, there’s lots of things going on – within most processes, there’s multiple threads, and within the application there may be multiple processes.
- 19:50 It might be a full-on microservices type architecture, or it might be something less parallel than that – but there’s almost always some parallelism in or between processes.
- 20:00 They are some really hard challenges to track down – race conditions are challenging, and in microservices each component on its own is perfect, but integrating them is fragile.
- 20:25 In a sense, you’ve now shifted the task from debugging a system to debugging a set of services.
- 20:30 Thread fuzzing is about the multi-threaded process case.
- 20:40 One of the most common questions I get when I talk about this is “Heisenbug” effects, when observing it changes its behaviour.
- 20:55 The answer is to some degree, it’s true – we’ve not broken the laws of Physics here.
- 21:00 Often, there’s some kind of rare race condition bug – and it’s just as likely to recur as fix the problem.
- 21:10 You can have something that happens 1 in 1000 times outside of live recorder, but happens 1 in 10 times inside live recorder.
- 21:20 There are other times, when 1 in 1000 is running natively, and when running in live recorder it just doesn’t show up.
- 21:30 What thread fuzzing does is to deliberately schedule the thread’s applications in a particular way to make those race conditions more likely to appear.
- 21:40 We can see at the machine level when the code is running locked machine instructions, for example.
- 21:45 That’s a hint that there’s some kind of critical section which could be important.
- 22:00 We can see what’s happening with shared memory – that’s one of the most difficult things for us to deal with, where memory is shared between multiple processes, or kernel or device.
- 22:05 We have to track all of those to get into the weeds.
- 22:10 Most memory has the property that when you read from a location of memory is that you read what was most recently written to that memory.
- 22:15 When your memory is shared between you and someone else, that’s no longer the case, so we have to capture those, which is a source of non-determinism that we know about.
- 22:30 To cut a long story short, we need to know about these bits of non-determinism that might only fall over 1 in 1000 – so we can hook those points and perturb those scheduling to make it more likely.
- 22:45 It’s not a data fuzzing, but a thread ordering fuzzing.
- 22:50 The idea is that it makes it more likely to fail, and we can make things that fail rarely or never, such that they usually or always fail with thread fuzzing.
- 22:55 Or if you’ve got something that fails so rarely, or you have no information as to why it’s failed, then turn on thread fuzzing, and these intermittent race conditions become trivial.
- 23:15 A customer quote from a month ago: “once a recording has been captured, it will be fixed the same day”
- 23:30 The catch is: can you capture it in a recording? – sometime’s it’s easy, sometimes not so much.
- 22:35 Thread fuzzing makes it easier to reproduce, and then fix.
- 23:45 Compute time is cheap: human time is expensive, so you can leave it running all week and then diagnose it in minutes or hours.
- 24:00 Multi-process correlation is for dealing with issues between multiple processes.
- 24:10 If you have multiple processes communicating over sockets or shared memory, you can record some subset or all processes.
- 24:25 You can then replay those recordings in a way you can trace the dependencies through the network of processes.
- 24:40 We have multi-process correlation for shared memory – it’s more niche than doing something over the network.
- 24:45 The problems are severe, which is why we decided to go there first – actually, we got encouraged to do so by some of our biggest enterprise customers.
- 24:55 You’ve got multiple processes, sharing memory, and one of the processes does something bad.
- 25:00 This is the worse kind of debugging experience – you’ve got a problem with the process having crashed or a failed assertion, or something wrong, and you know one of the other processes may have scribbled on your shared memory structure.
- 25:20 When you’ve got multi-process correlation, you can find out what process wrote to that shared memory location, and it will tell you.
- 25:30 If you want, you can go to the recording that find out when the bad thing occurred and what it did, so you can follow the multi-process flow back to the offending line.
- 25:45 This makes things that are borderline impossible to solve very easy to fix.
- 25:50 We plan to follow up with multi-process correlation for distributed networking, with sockets.
- 26:00 It’s a bit like the language support; we’ll provide the support for those.
- 26:05 Imagine reverse stepping through the Java remote procedure call back to the call site on another system and find out exactly why that called you in the weird way that it did.
- 26:20 That’s going to come along a little later in 2020.
Is it challenging to do the correlation between different languages and systems? –
- 26:40 From an intellectual, hard computer science point of view, not really – we’ve done the hard bit; we have all the information we need to do that.
- 26:50 The challenge is from the user interface point of view – figuring out how to present that complex web.
- 27:00 We’re already snooping everything that’s going in and out of the process – shared memory, socket etc.
- 27:10 If you have two microservices communicating, at some point there’s going to be IO between them – HTTP, or whatever.
- 27:15 We’ve already got that communication.
- 27:20 As an industry, we’re just getting our heads around what this all means from a user interface and user experience perspective.
- 27:25 We have existing traceability things and observability things already.
- 27:30 Where this gets valuable is when we can marry this technology with existing tracing tools.
- 27:45 Logging and tracing are all forms of observability, and we’re just a different form of observability.
- 27:50 Logging and tracing give the developer a good high-level narrative or linear story of what was happening.
- 28:05 That’s typically the place to start – you’ll get the high-level 10,000 foot view; but the times that story gives you enough information to root cause it happens a few times a year.
- 28:25 Usually you might find a smoking gun, and have some idea that something is wrong, but you’re going to need more information to fix it.
- 28:35 You’ll need the next level of observability, and for us the challenge is what is the right way to fit in and complement the other forms of observability.
- 28:45 You can think of this as being a zoom-in technology for the problem.
What are you personally looking forward to in 2020? –
- 29:05 The things we were talking about are what’s in my mind.
- 29:15 As an industry, we’re figuring out what observability of microservices means, there’s lots of things like testing or debugging in production that will be interesting.
- 29:30 How all of these things gel together is something I’m looking forward to finding out.
If people want to follow you, what’s the best way of doing that? –
- 29:40 For me personally, Twitter is the best way – https://twitter.com/gregthelaw but for company stuff https://undo.io or https://twitter.com/undo_io
- 29:45 If there’s any C or C++ developers out there, I’ve created several 10 minute how-to videos on using GDB over the years. I’ve learnt a lot from using GDB
- 30:05 GDB is one of those things that’s very powerful, but its lousy for discoverability, so I’m putting together these screencasts to expose things that are there but which people don’t know about.
About QCon
QCon is a practitioner-driven conference designed for technical team leads, architects, and project managers who influence software innovation in their teams. QCon takes place 8 times per year in London, New York, Munich, San Francisco, Sao Paolo, Beijing, Guangzhou & Shanghai. QCon London is at its 14th Edition and will take place Mar 2-5, 2020. 140+ expert practitioner speakers, 1600+ attendees and 18 tracks will cover topics driving the evolution of software development today. Visit qconlondon.com to get more details.
More about our podcasts
You can keep up-to-date with the podcasts via our RSS Feed, and they are available via SoundCloud, Apple Podcasts, Spotify, Overcast and the Google Podcast. From this page you also have access to our recorded show notes. They all have clickable links that will take you directly to that part of the audio.
Previous podcasts
Amazon Updates the Elastic File System Service with New Features: IAM Authorization and Access Point
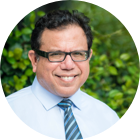
MMS • Steef-Jan Wiggers
Article originally posted on InfoQ. Visit InfoQ
Amazon’s Elastic File System (EFS) Service (EFS) offers a simple, scalable, fully managed elastic NFS file system for use with AWS Cloud services and on-premises resources. Recently Amazon announced updates for this service by adding two new features, namely Identity and Access Management (IAM) authorization for Network File System (NFS) and EFS Access Points.
By using EFS, customers get:
- strong file system consistency across three Availability Zones,
- a performance that scales with the amount of data stored,
- and the option to provision the throughput.
Last year, the team responsible for the EFS service had a focus on cost reduction. It introduced the EFS Infrequent Access (IA) storage class – which allows customers to reduce costs by setting up Lifecycle management policies to move the files which haven’t been accessed for a certain amount of days to EFS IA .
Now the has team added:
- Identity and Access Management (IAM) authorization for Network File System (NFS) to identify clients and use IAM policies to manage client-specific permissions
- and EFS Access Points to enforce the use of an operating system user and group, optionally restricting access to a directory in the file system.
Scott Francis, a solution architect at AWS, said in a tweet:
If you’re using EFS, the addition of these features (which map roughly to traditional NFS usermap.cfg and mount-level and file-level restrictions) should make EFS much more closely approximate to the management feature set of conventional NFS servers.
With IAM, users can set up file system policies when creating or updating ESF, which are applicable for all NFS clients connecting to a file system. During the setup process, users can choose a combination of predefined policy statements, set the policy and review the JSON. Moreover, the user can even alter the JSON format to fit more complex scenarios and, for example, give individual accounts or IAM roles more privileges.
Source: https://aws.amazon.com/blogs/aws/new-for-amazon-efs-iam-authorization-and-access-points/
Lastly, every time an IAM permission is checked, the AWS CloudTrail console logs an appropriate event, making the process auditable.
The other new feature, access points, is a feature with a similar purpose as IAM, providing enterprise system administrators more control when allowing applications file system access. Furthermore, these admins can specify which POSIX user and group to use when accessing the file system and restricting access to a directory within a file system.
Source: https://docs.aws.amazon.com/efs/latest/ug/create-access-point.html
In the Amazon blog post on the EFS updates, Danilo Poccia, principal evangelist at AWS, mentions the benefits of access points:
- Container-based environments, where developers build and deploy their own containers
- Data science applications that require read-only access to production data
- Sharing a specific directory in your file system with other AWS accounts
Both IAM authorizations for NFS clients and EFS access points are available in all regions where EFS is available, and there is no additional cost for using them. Furthermore, details about using EFS with IAM and access points are available in the documentation.
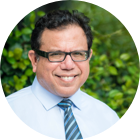
MMS • Helen Beal
Article originally posted on InfoQ. Visit InfoQ
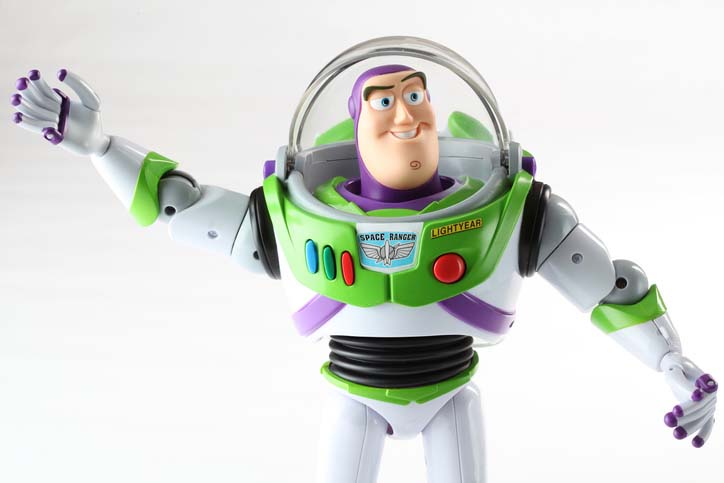
Business directory and crowd-sourced review service, Yelp, has open-sourced their in-house developed testing framework, fuzz-lightyear, that identifies Insecure Direct Object Reference (IDOR) vulnerabilities.
Fuzz-lightyear uses stateful Swagger fuzzing and has been designed to support enterprise microservices architectures can and be integrated with continuous integration pipelines. Yelp identified IDOR vulnerabilities as not only high-risk but also particularly difficult to prevent and detect. Swagger is an open-source software development framework for RESTful web services. It allows APIs to describe their own structure then asks the API to return a YAML or JSON file that contains a detailed description of the entire API. Being able to read an API’s structure means documentation can be automatically built, multi-lingual client libraries can be generated and it can be leveraged for automated testing.
Fuzzing is a testing technique that can be used to discover security vulnerabilities. It inputs large amounts of random data, called fuzz, to the test subject in a detection attempt.
Application security engineering manager at Yelp, Aaron Loo, explains in his blog post announcing the availability of fuzz-lightyear that a common industry recommendation to prevent IDOR vulnerabilities is to use a mapping, such as a random string, to make it harder to enumerate values as an attacker. However, maintaining a mapping will likely lead to either cache management issues that impact SEO or challenges around inconsistent internal reference methods. Loo explores the problem with this attack vector further in his article:
Another common industry recommendation is to merely perform access control checks before manipulating resources. While this is easier to do, it’s more suitable for spot-fixing, as it’s a painfully manual process to enforce via code audits. Furthermore, it requires all developers to know when and where to implement these access control checks.
Yelp’s view on detection strategies is that they aren’t any better than the prevention strategies: penetration or vulnerability testing is hard to scale and can be expensive, and SAST tools create ‘noise’. The team also considered API fuzzing:
The issue with traditional fuzzing is that it seeks to break an application with the assumption that failures allude to vulnerabilities. However, this is not necessarily true. As a security team, we care less about errors that attackers may or may not receive. Rather, we want to identify when a malicious action succeeds, which will be completely ignored by traditional fuzzing.
Loo refers to a research paper published by Microsoft in February 2019 that described how stateful Swagger fuzzing was able to detect common vulnerabilities, including IDOR, in REST APIs by having a user session execute a sequence of requests and then having an attacker’s session execute the same sequence of requests to ensure that the user and the attacker are able to reach the same state. For the last request in the sequence, having the attacker’s session execute the user’s request and producing a successful result detects a potential vulnerability.
This research inspired the team to consider a Swagger fuzzing solution for their IDOR vulnerability problem since it allowed them to consider testing scenarios not about whether an application breaks for a specific user but about successful requests in situations where they should have failed. Loo explains how:
Swagger, as a standardised API specification, is fantastic for programmatically defining the rules of engagement for the fuzzing engine. Furthermore, by making it stateful, we can simulate user behaviour through proper API requests/responses which keep state between each response. This state can then be used to fuzz future request parameters so that a single request sequence is able to accurately simulate a user’s session, enabling chaos engineering testing.
Whilst the Yelp team encountered some issues with infrastructure dependencies and incomplete CRUD resource lifecycles, they solved them using sand-boxed Docker Compose acceptance testing and providing developers with the ability to define factory fixtures to be used while fuzzing. An additional problem with expected direct object references was addressed with an endpoint whitelisting system. As a result, they were able to produce fuzz-lightyear; a testing framework that allows developers to configure dynamic IDOR vulnerability tests and integrate them into a continuous integration pipeline.
You can access fuzz-lightyear here on GitHub.
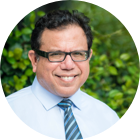
MMS • Bruno Couriol
Article originally posted on InfoQ. Visit InfoQ
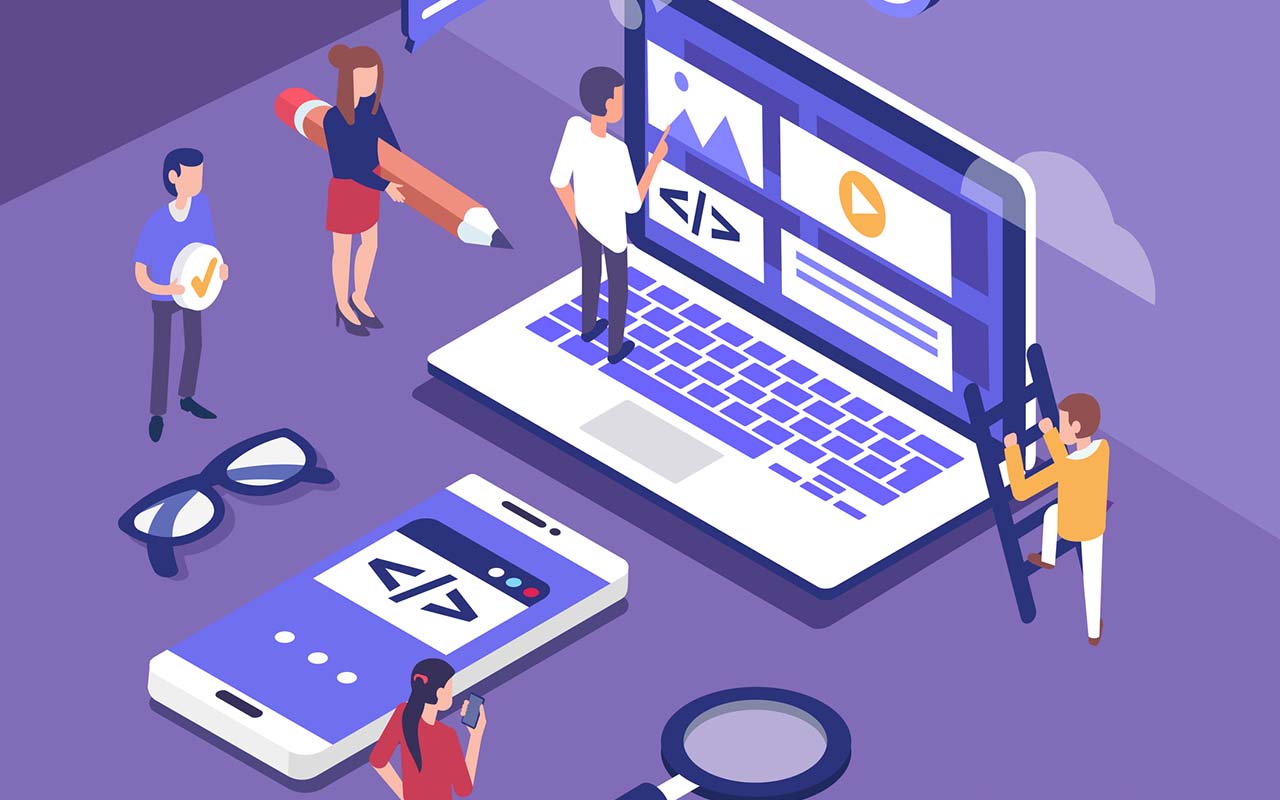
At its Node+JS Interactive conference in Montreal, the OpenJS Foundation announced welcoming into its midst Electron, a cross-platform desktop application development tool based on Node.js and Chromium.
Electron joins the foundation at the incubator level, but as a mature project already in use in widely known applications, such as Visual Studio Code, Microsoft Teams, Skype, Discord, Slack, or Trello. The pooling of resources and a better governance model appear to be the rationale behind the move to the OpenJS Foundation. As the foundation explains:
OpenJS Foundation support includes organizing community events like Node+JS Interactive, providing marketing and community management support to projects and working groups, and coordinating financial investments across projects. In addition, the combined governance structure enables projects of all sizes to benefit from experienced mentors as they progress through the project lifecycle, and benefit from foundation-wide marketing activity.
Electron was first developed by GitHub in 2013 to allow JavaScript developers to build desktop apps that would run on Windows, Mac, and Linux computers. While the project began under GitHub’s guidance, Robin Ginn, executive director of the OpenJS Foundation, addressed the change in the progressive in the governance model:
[Electron] truly has moved to a kind of project that’s broadly maintained by a number of developers. It started moving last year to an open governance. (…) This really helps them formalize decision making and make it so it isn’t just a project owned by a single entity. Moving into the foundation was sort of a natural step for them.
The flurry of open-source alternatives for cross-platform JavaScript development (including defunct TideSDK), seems to have dried up. NW.js, formerly known as Node Webkit, is currently the most popular alternative to Electron for cross-platform desktop JavaScript application development. While NW.js was created in the Intel Open Source Technology Center in 2011, as of today, it seems that NW has not seen the same amount of adoption as Electron. Interestingly, just like Microsoft (new GitHub’s hence Electron’s owner), Intel is a member of the OpenJS Foundation.
The OpenJS Foundation aims to be the central place to support critical open-source JavaScript projects and web technologies. The OpenJS Foundation is committed to providing a neutral organization to host and maintain projects and to fund the benefit of the entire community. The foundation consists of 32 open-source JavaScript projects, including jQuery, Node.js, and Webpack, and is supported by 30 companies including Google, IBM, Intel, and Microsoft.
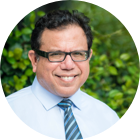
MMS • Dylan Schiemann
Article originally posted on InfoQ. Visit InfoQ
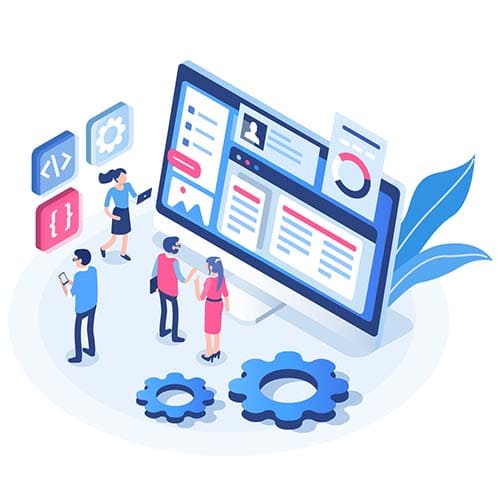
Playwright is an open-source Node.js library started by Microsoft for automating browsers based on Chromium, Firefox, and WebKit through a single API. The primary goal of Playwright is improving automated UI testing.
Playwright is similar in mission to Puppeteer, though Puppeteer only supports Chromium-based browsers. As explained by the Playwright team:
We are the same team that originally built Puppeteer at Google, but has since then moved on. Puppeteer proved that there is a lot of interest in the new generation of ever-green, capable, and reliable automation drivers. With Playwright, we’d like to take it one step further and offer the same functionality for all the popular rendering engines. We’d like to see Playwright vendor-neutral and shared governed.
The Playwright team strives to create more testing-friendly APIs by learning from lessons and challenges with Puppeteer.
Playwright also aims at being cloud-native through its BrowserContext
abstraction, allowing BrowserContext
s to either get created locally or provided as a service.
The Playwright team believes that due to the similarity of the core concepts and the APIs, migration between the Puppeteer and Playwright should be straightforward.
Playwright is also an alternative to WebDriver, the current W3C standard for web automation and testing. The Playwright team notes that Puppeteer influenced the WebDriver standard by steering WebDriver towards a bi-directional communication channel. The Playwright team hopes to shape future WebDriver standards to support numerous Progressive Web App (PWA) features, such as support for additional browser capabilities, more ergonomic APIs and increased testing reliability.
Playwright supports upstream versions of Chromium, and plan to synchronize their npm
release cycle with the Chromium stable channel releases.
To support WebKit, Playwright makes modifications to WebCore and WebKit2 to extend WebKit’s remote debugging capabilities and Playwright APIs. The team hopes to land these changes in WebKit to rely instead on the upstream version of WebKit.
For Firefox, Playwright also makes modifications to Firefox for features such as content script debugging, workers, CSP, emulation, network interception, and more. Similar to WebKit, the Playwright team hopes to land these changes in Firefox soon.
Playwright supports each browser engine across Windows, macOS, and Linux. Headless mode supported is available for all supported browsers and platforms.
Playwright is currently in a 0.9.x release, with a stable 1.0 version expected in 2020. Is Playwright Ready shares the current status of Playwright across the supported browser engines.
Playwright is open source software available under the Apache 2 license. Contributions are welcome via the Playwright contribution guidelines, following the Microsoft code of conduct.
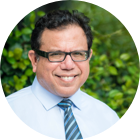
MMS • Sergio De Simone
Article originally posted on InfoQ. Visit InfoQ
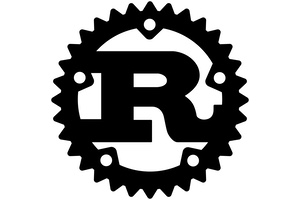
Rust Analyzer is an emerging endeavour within the Rust ecosystem aimed to bring a great IDE experience with Rust.
Compiler performance has always been a major focus of Rust tooling development and compile times have been steadily improving across releases. However, as Igor Matuszewski explained in his Rust Belt Rust Conference talk, Rust IDE support is an area of active work:
Despite the landscape shifting a lot during these last 3 years, including a proliferation of new tools and improved integration between tools, it feels like the Rust IDE story is not yet complete.
This work is being carried through under the guidance of the RLS 2.0 working group and includes among its main components Rust Analyzer. InfoQ has taken the chance to speak with Aleksey Kladov, main contributor to Rust Analyzer, and Rust Core Team member Steve Klabnik to learn more about it.
Rust has been gathering lots of interest recently, and the language has been evolving and growing its ecosystem/tooling at a great pace. What ia Rust current maturity status and what can we expect in the next few years?
Klabnik: “Maturity” can mean many things. For me, the metric is companies using Rust in a real product. Today, that looks like:
- Amazon
- Microsoft
and more. Three out of five FAANGs ain’t bad.
In general, Rust is slowing down, and getting less new features, and more refinement to existing stuff. Now that async/await has shipped, for example, more work is being put into things like diagnostics. Rust will get a few more big features in the next few years, but similarly to how async/await is mostly good for networked applications, they’re features that are extremely valuable in a particular niche, but may not be huge to all Rust programmers. For example, “const generics” let you write code that’s generic over integers, rather than just types, and this is going to be very good for numerics libraries. But in general, these features are being added more slowly than previous major ones.
Can you briefly explain what limitations the current Rust compiler has when it comes to IDE integration? What are the goals of the Rust Analyzer project?
Kladov: The limitations here are not specific to the Rust language, but a general for command line vs IDE compiler.
The main thing is that the command line (or batch) compiler is primarily optimized for throughput (compiling N thousands lines of code per second), while an IDE compiler is optimized for latency (showing correct completion variants in M milliseconds after user typed new fragment of code). As usual with throughput vs latency, these two goals require pretty different optimizations (and even high-level architectures). In general, it’s hard to retrofit low latency requirement on a compiler which was developed solely with big throughput in mind.
Another thing is difference in handling invalid code. A traditional compiler front-end is usually organized as a progression of phases, where each phase takes an unstructured input, checks the input for validity, and, if it is indeed valid, adds more structure on top. Specifically, an error in an early phase (like parsing) usually means that the latter phase (like type checking) is not run for this bit of code at all. In other words, “correct code” is a happy case, and everything else can be treated as an error condition. In contrast, in IDE code is always broken, because the user constantly modifies it. As soon as the code is valid, the job of IDE ends and the job of the batch compiler begins. So, an IDE-oriented compiler should accommodate an incomplete and broken code, and provide IDE features, like completion, for such code.
The overarching goal of rust-analyzer project is to provide a single Rust compiler with excellent scores along both the latency and the throughput dimensions. The road towards this goal is long though, so currently we are in the phase where there are effectively two front-ends:
- rustc, which is a very mature batch compiler.
- rust-analyzer, which is a very-much experiment IDE/latency oriented compiler.
This front ends share a tiny bit of code at the moment, and the immediate tactical goal is to share more of the easily shareable code between them.
Does this project supersedes Rust LSP implementation?
Kladov: Not at the moment, rust-analyzer is an experiment, and we are not quite ready to recommend it as the official LSP implementation. However, the current tentative plan is indeed that rust-analyzer supersedes RLS in the near-ish future.
Can you share some detail about which direction the compiler refactor will take?
Kladov: The main idea is to make the compiler more lazy. The single most important trick an IDE can use to achieve low latency is avoiding as much work as possible. For example, to provide code completion, you generally need to analyze code on the screen and its immediate dependencies, you don’t care what’s written in the other five millions lines of code in your project. The idea is simple, but to make a compiler to not look at the extra code is actually pretty tricky, and that’s where the bulk of work will go. Some more specific things we plan to do are:
- Transitioning to a full-fidelity syntax tree representation, which includes white space and comments.
- Adding “multi-crate” mode, where a single compiler instance can process several compilation units at once.
- Making the compiler process persistent and adding ability to send diffs of the input files to the compiler.
All those things are already implemented in rust-analyzer, but in a rather proof-of-concept manner. The tricky bit is to moving them all into the production compiler without breaking user’s code in the process.
Rust Analyzer is still alpha quality and requires building from source:
$ git clone https://github.com/rust-analyzer/rust-analyzer
$ cd rust-analyzer
$ cargo xtask install
Also, do not forget to check out its manual if you are interested in trying it out.
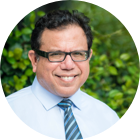
MMS • Ben Linders
Article originally posted on InfoQ. Visit InfoQ
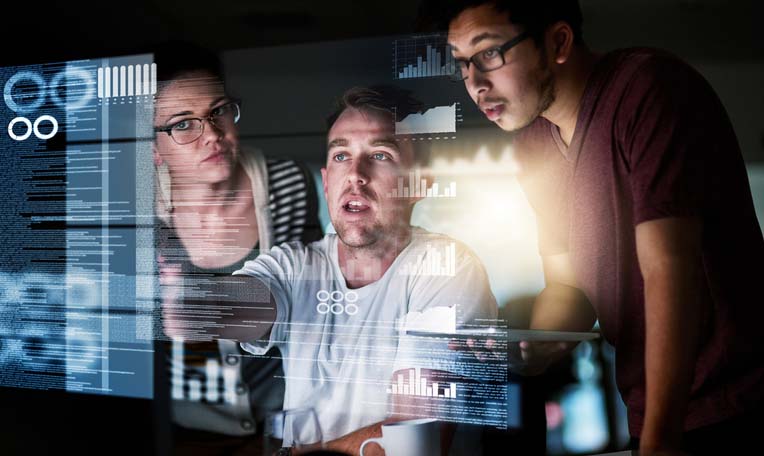
Simulation can be used to compare agile strategies and increase understanding of their strengths and weaknesses in different organisational and project contexts. The Lazy Stopping Model derived from the idea that we often fail to gather sufficient information to get an optimal result. Often we can’t avoid this; we learn it as we go, or when working towards moving targets. Agile strategies can then be simulated in the model as more or less effective defences against this “lazy stopping.”
Adam Timlett is researching the simulation of agile strategies with King’s College, London. In his research, he will simulate different strategies for software development or capital projects using the idea of “lazy stopping”. The simulations can be used either as an educational tool or as a way to test a set of proposed project strategies against different scenarios.
Timlett will be speaking about simulating agile strategies using the lazy stopping model at Aginext 2020, to be held in London on March 19 – 20.
InfoQ interviewed Adam Timlett about how the Lazy Stopping Model works, hypothesising different agility strategies, and the advantages of simulation.
InfoQ: How does the Lazy Stopping Model work?
Adam Timlett: The “Lazy Stopping Model” derives its name from the idea of “optimal stopping” or “early stopping theory,” (see Knowing When to Stop) but whereas those subjects are about how to find the minimum amount of information you need to make a very good decision, the idea of “lazy stopping” (which is my proposal) is derived from the idea that we often fail to gather sufficient information to get an optimal result. This idea is also inspired by Hoffman’s Interface Theory of Perception which argues that perception is “sub-optimal,” as evolution only dictates that we gather enough information to survive rather than to accurately perceive our environment. This is “viability” over “optimality”.
The “Lazy Stopping Model” therefore just reflects the idea that we choose how much information to gather before taking an action. If we gather less than we “should”, for some reason, then we can say that the agent (a simulated person or organisation) has stopped gathering info and is taking action before it should. But in practice, it may be impossible to avoid “lazy stopping,” which is where agile strategies come in.
Agility is mainly a defensive strategy against your own ignorance. It’s about dealing with the costs of previous decisions by either failing fast and thereby learning quickly, and/or by lowering the costs of adjustments and re-working them when you learn that what you had built or deployed at first is not quite right. This includes creating an environment and office culture where that is OK and expected, as long as you also learn quickly. In contrast, to maximise efficiency, a more offensive strategy would need to be used when you are confident you have enough information to act quickly in order to maximise your advantage over competitors. These defensive and offensive strategies can look similar in practice, but in reality, the rationale is quite different.
InfoQ: What different agile strategies do you plan to hypothesise in the model?
Timlett: The strategies that I plan to simulate in the model are variations on the defensive strategies theme, and I want to cover a wide range of different styles of agility which emphasise different things. There is, for example, “Continuous Improvement,” which is more about modular systems and fast deployment, “Working Right to Left” which is more about culture, and “Lean Ux” which is more about learning fast. I also want to model “Waterfall,” which is still very much in use but not agile at all because it mainly bets on agents not stopping “lazily,” but actually having gathered enough information before deciding to move on to the next stage of the project.
I expect the model to show that each style of agility and even Waterfall is strongest in a different organisational and project context, and that each has its own strengths and weaknesses depending on where the uncertainty or “traps” actually are in the project or organisational context. (The “traps” are where it is almost impossible to avoid “lazy stopping”). It is this ability to compare very different strategies that will, I hope, be the strong point of the modelling approach.
InfoQ: How will you do the simulations?
Timlett: The simulation method is known as an “agent-based model,” which is a type of computer simulation approach that is used in biological sciences and the social sciences as a way to model systems more “holistically” by relying on many computations, rather than a small number of equations. The “agents” in the model could be cells, or they could be individuals, companies or units of an organisation. The agents interact with one another and often the modellers talk about the “emergent properties” of the system. These result from many discrete local interactions between the agents that add up to a new system state.
A simple example of this “emergence” is the way that traffic jams result from lots of individual drivers making small local decisions to respond to the cars ahead of them. By taking discrete amounts of time to respond to other cars by braking and accelerating after a short delay, these small delays in response can add up to a system traffic jam if the weight of traffic is already heavy and then someone ahead of them suddenly slows down further to look at something that is happening on the other side of the road.
For modelling software projects, the local interactions of the agents is the information available for making decisions, and then the actions the agents take affect other agents downstream, just like in traffic. But it is more like a task flow, with each agent handing over the task in a different state than the one it was in previously. The principles of agent-based modelling still apply though: you define agents, their dispositions, policies, their “driving style”, if you like, and what information they can see before they act and what state of the world they inherit before they themselves can act.
InfoQ: What are the advantages of simulation with the Lazy Stopping Model?
Timlett: The main advantage of the Lazy Stopping Model is the conceptual development that it entails, which takes us from discussing agile strategies using only our own personal experiences and anecdotes, to a conversation about agility in which we are better informed and are capable of being more precise and considerate than before. It also holds on to the possibility of encoding many experts’ knowledge and adding them together so that the Lazy Stopping Model library becomes a repository for expert knowledge about agile project management techniques and the traps that exist out there.
But mainly, the advantage is in us humans learning how to discuss agility using the simulation as a means of advancing our concepts and elevating our own discussion with one another. It is not necessarily about the output of the simulation itself, because at least initially, we may not be entirely confident about its predictive capacity. This is especially because, whilst the simulation can model scenarios, without knowing these scenarios’ base rates of probabilities, we can’t really say what is optimal in a given context.
InfoQ: Where can InfoQ readers go if they want to learn more about simulating agile strategies?
Timlett: There are pioneering complex systems scientists in this area who are involved in very exciting research which I try to follow as closely as I can. Some of them actually work in systems biology rather than organisational economics, but there is actually a lot of potential crossover. For example, the review article cellular noise and information transmission (paid content) by Levchenko and Nemenman provides a good overview of the systems biology topic, from the perspective of the measurement of noise and information transmission in biological systems. In organisational economics, it is also worth checking out Michael Fyall’s thesis from Stanford, 2002, which makes use of an existing simulation of organisations to test out some new ideas. For an overview of some foundational ideas in complexity science, I recommend Yaneer Bar-Yam “Making Things Work”.
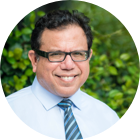
MMS • Arthur Casals
Article originally posted on InfoQ. Visit InfoQ
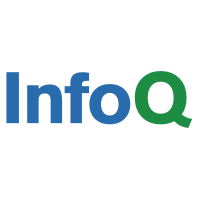
Earlier this week, Microsoft announced experimental support for gRPC-Web with .NET Core. The new addition allows Blazor WebAssembly clients to call gRPC applications directly from the browser, enabling gRPC features such as server streaming to be used by browser-based applications.
Originally developed and used by Google, gRPC is a remote procedure call system officially recommended by Microsoft as a replacement to WCF in .NET Core. Although the .NET support is still experimental, gRPC-Web is not a new technology: released in 2018 as a JavaScript client library, it allows web applications to communicate directly with gRPC services without using an HTTP server as a proxy.
Similarly to gRPC, gRPC-Web uses pre-defined contracts between the (web) client and backend gRPC services. It allows the creation of an end-to-end gRPC pipeline compatible with HTTP/1.1 and HTTP/2 – which is particularly relevant since browser APIs can’t call gRPC HTTP/2.
The new experimental features allow ASP.NET Core gRPC applications to be called from the browser by both .NET Blazor WebAssembly applications and JavaScript SPAs. They also offer an alternative to hosting ASP.NET Core gRPC applications in IIS and Azure App Service, since neither server can currently host gRPC services. The new features include support for server streaming, using Protobuf messages, and strongly-typed coded-generated clients.
While the new features open new opportunities for using gRPC with web applications, however, Microsoft classifies gRPC-Web for .NET as “experimental.” That means there is no commitment from Microsoft to develop it into a product. According to James Newton-King, a principal software engineer in the ASP.NET development team:
We want to test that our approach to implementing gRPC-Web works, and get feedback on whether this approach is useful to .NET developers compared to the traditional way of setting up gRPC-Web via a proxy.
It is also important to notice that not all gRPC features are supported (i.e., client streaming and bi-directional streaming). Using gRPC-Web for .NET requires .NET Core 3.1. The experimental packages are available on NuGet (here and here), and a tutorial on how to use gRPC-Web with .NET Core can be found here.