Month: November 2020
If Data Scientist is the Modern-Day Alchemist, Then Why are We Using Leeches to Monetize Data?
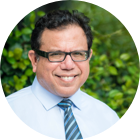
MMS • RSS
Article originally posted on Data Science Central. Visit Data Science Central
Okay, I’m as mad as hell, and I’m not going to take this anymore! If data scientists are the modern-day alchemist who turn data into gold, then why are so many companies using leeches to monetize their data?
When I talk to companies about the concept of a “Chief Data Monetization Officer” role, many of them look at me like I have lobsters crawling out of my ears. “Sorry, we don’t have that role here.” Of course you don’t have that role, and that’s why you SUCK at monetizing data. That’s why you have no idea how to leverage data and analytics to uncover and codify the customer, product, and operational insights that can optimize key business and operational processes, mitigate regulatory and compliance risks, create net-new revenue streams and create a compelling, differentiated customer experience. What, you expect that just having data is sufficient? You expect that if you just have the data, then some sort of “miracle” will occur to monetize that data?
Before I give away the secret to solving the data monetization problem, I think we first need to understand the scope of the problem.
Tragic Mismatch Between Data Acquisition versus Monetization Strategies
Organizations spend 100’s of millions of dollars in acquiring data as they deploy operational systems such as ERP, CRM, SCM, SFA, BFA, eCommerce, social media, mobile and now IoT. Then they spend even more outrageous sums of money to store, manage, backup, govern and maintain all of the data for regulatory, compliance and management reporting purposes. No wonder CIO’s have an almost singular mandate to reduce those data management costs (hello, cloud). Data is a cost to be minimized when the only “value” one gets from that data is from reporting on what happened (see Figure 1).
Figure 1: “Digital Strategy Part I: Creating a Data Strategy that Delivers Value”
As the Harvard Business Review article “Companies Love Big Data But Lack the Strategy To Use It Effectively” stated:
“The problem is that, in many cases, big data is not used well. Companies are better at collecting data – about their customers, about their products, about competitors – than analyzing that data and designing strategy around it.”
Bottom-line: while companies are great at collecting data, they suck at monetizing it. And that’s where the Digital Transformation Value Creation Mapping comes into play.
Mastering the Digital Transformation Value Creation Mapping
The Digital Transformation Value Creation Mapping lays out a roadmap to help organizations identify, codify, and operational the sources of customer, product and operational value within a continuously-learning and adapting culture. Let’s deep dive on each of the 4 steps of the Digital Transformation Value Creation Mapping so that any organization can realize the data monetization dreams…without leeches (see Figure 2).
Figure 2: Digital Transformation Value Creation Mapping
Step 1: Identify the Sources of Value Creation. This is a customer-centric (think Customer Journey Maps and Service Design templates) exercise to identify and value sources and impediments to customer value creation.
- Identify the targeted business initiative and understand its financial ramifications
- Identify the business stakeholders who either impact or are impacted by the targeted business initiative
- Interview the business stakeholders to identify the decisions that they make in support of the business initiative and their current impediments to making those decisions
- Document the customer’s sources, and impediments, to value creation via Customer Journey Maps and Service Design templates
- Across the different stakeholder interviews, cluster the decisions into common subject areas or use cases.
- Conduct an Envisioning workshop with all stakeholders to validate, value and prioritize the use cases while building organizational alignment for the top priority use case(s)
- Create a Hypothesis Development Canvas for the top priority use cases that details the metrics and KPI’s against which use case progress and success will be measured, business entities/assets around which analytics will be built, key decisions and predictions required to address the use case, data and instrumentation requirements, and costs associated with False Positives and False Negatives to reduce AI model unintended consequences.
Figure 3: Hypothesis Development Canvas
Step 2: Codify Sources of Value Creation. This the data science, data engineering and advanced analytics effort to put into math (codify) the customer, product and operational requirements defined in Step 1.
- Establish the advanced analytics environment (using open source tools such as Jupyter Notebooks, TensorFlow AI/ML framework, ML Flow for ML model management, Seldon Core for deploying ML models, Ray distributed ML model execution framework, Python, etc.)
- Ensure access to the required internal and external data sources (ideally via a data lake)
- Use agile sprints and rapid prototyping to prototype and co-create with customers the reusable analytic modules that will drive the optimization of the targeted use case(s)
- Establish Asset Model architecture and technology for the creation of analytic profiles on the key business entities around which the analytics will be applied
- Use AI/ML to create a library of reusable Analytic Modules that will accelerate time-to-value and de-risk future value engineering engagements
Step 3: Operationalize Sources of Value Creation. This step is the heart of the data monetization process – integrating the analytic models and analytic results into the operational systems in order to optimize the organization’s business and operational decisions.
- Integrate the analytic modules and a model serving environment into the organization’s operating environment
- Provide API’s that can deliver the analytic outcomes (propensity scores, recommendations) to the necessary operational systems in order to optimize business and operational decisions
- Instrument the operational systems to track the results from the decisions in order to determine the effectiveness of the decisions (AI model decision effectiveness)
- Implement a model management environment to continuously monitor and adjust for model drift
Step 4: Continuously-learning and adapting to Sources of Value Creation. This step provides the feedback loop to facilitate the continuously-learning and adapting of the analytic modules and assets models (the heart of creating autonomous assets that continuously-learn and adapt with minimal human intervention).
- Leverage AI / Reinforcement Learning capabilities to continuously monitor and adjust AI model performance based upon the actual versus predicted outcomes
- Implement a Data Monetization Governance Process that drives (enforces) data and analytics re-use and the sharing and codification of learnings
- Leverage Explainable AI (XAI) techniques to understand the AI model changes and their potential impact on privacy and compliance laws and regulations
- Revisit the costs of the False Positives and False Negatives that drive the AI models
- Create a culture of continuously learning where the organization learns and adapts with every front-line customer engagement or operational interaction.
Data Monetization Summary
Data Scientists are the modern-day alchemists – they know how to turn data into gold. And the Digital Transformation Value Creation Mapping gives you that roadmap for turning data into gold. Heck, here is a Value Creation cheat sheet to get you started!
Figure 4: Value Creation Mapping Template
So, what are you waiting for…bigger leeches?
Extra Credit Reading. Digital Business Transformation 3-part series:
- It’s Not Digital Transformation; It’s Digital “Business” Transformation! – Part I https://www.linkedin.com/pulse/its-digital-transformation-business-part-i-bill-schmarzo/
- It’s Not Digital Transformation; It’s Digital “Business” Transformation – Part II https://www.linkedin.com/pulse/its-digital-transformation-business-part-ii-bill-schmarzo/
- It’s Not Digital Transformation; It’s Digital “Business” Transformation – Part III https://www.linkedin.com/pulse/its-digital-transformation-business-part-iii-bill-schmarzo/
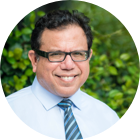
MMS • RSS
Article originally posted on Data Science Central. Visit Data Science Central
A recent Wall Street Journal article, entitled Coronavirus Pandemic Helps Speed More CIOs Toward Business Operations Accountability, observed:
“Large businesses are starting to hold their chief information officers accountable for the results of corporate-wide digital business operations, giving them much wider responsibility within the organization. . . Gartner, which last month released its annual list of top strategic predictions, said that by 2024, a quarter of the CIOs working in large, traditional enterprises will effectively become, as the research and advisory firm calls it, ‘COO by proxy.’”
The Adolescence of Business Technology Management
When I was in Business School, more than a few years ago, I remember reading any number of articles debating the relative prospects of becoming a CEO via different paths. At the time, Sales was probably the most common route, followed by Operations, Marketing, Legal and Engineering. IS, (Information Systems, as it was then known,) didn’t make the list.
Given my focus on integrating business management with technology and systems, I felt that this was short-sighted. It was clear to me then that information was the life blood of business and whoever managed, cared for and fed it could ultimately acquire a position of substantial responsibility. At the time, I didn’t see why an IS Director (since elevated to the lofty title of CIO) couldn’t capitalize on his or her unique perspective to ascend to the top of the pyramid.
Software as a Component of Value Delivery
Some years later, I returned to the school to attend one of its annual technology conferences. I was staggered by a presentation from one of the senior professors who spoke about a Defense Department-sponsored study of software development productivity and quality he had performed, which was prompted by some notable cost overruns and failures on important and expensive projects. His overall assessment of our capabilities was that we really weren’t too good at it—pretty shocking considering that he was from Stanford University and we were at Carnegie-Mellon University, two of the top three Computer Science departments in the country. One of the projects he’d audited as part of the study was the latest US fighter aircraft under development and here’s the striking statistic he mentioned: even accounting for all the exotic materials, cutting-edge fabrication and manufacturing processes and design innovations, 50% of the cost of such aircraft is software! His point was that, given the proportional value of software in the product, deficiencies in our productivity and lapses in quality, if left unaddressed, would have major consequences. I didn’t have the background to fully appreciate all of the nuances, but the need to understand the relative contributions and risks of each of the inputs and capabilities employed to create a product or other outcome of value has remained with me.
Technology and its Application to Business Then and Now
At the outset of my Information Technology career (trust me, there weren’t pterodactyls flying around) IS costs were deemed acceptable if they were constrained to one or two percent of sales. Applications were designed mainly to mimic existing manual business processes, not to induce change in them. In many cases, forms used in manual process execution were replicated in application interfaces to limit or eliminate retraining expenses. Therefore, what we automated was what we were already doing, just faster and, perhaps, at greater volume. The IS Director’s job was focused on balancing project demands, managing operations, controlling costs and keeping the users from complaining to the senior executives about lapses in attention or service levels.
Subsequently, concepts like Decision Support Systems and Business Process Re-engineering appeared. IS was beginning to acquire some of the capabilities that we take for granted now, such as Big Data and Analytics, Machine Learning and Artificial Intelligence and Robotic Process Automation. It was also around this time that the idea of organizing the business to optimize the use and reusability of information gained momentum. The IS Director gained more opportunity to contribute to strategizing and direction setting. The goal at that time for him (and it was almost always a ‘him’ then) was ‘a seat at the table.’
Now, businesses resemble the fighter aircraft more than ever. The relative contribution of information technology to competitiveness has grown and the design of the enterprise is driven by optimizing the ability to exploit it. The relationship between hard and soft assets, including Intellectual Property, know-how and processes has been upended—the fewer physical assets and the smaller the dedicated work force, the more valuable the company may be versus its direct competitors. The role of the IS Director, now the CIO, is more closely tied to business results than it ever was before.
Organizational Structure and Transformation Then and Now
Running an organization has always required a variety of disciplines and balance between centralizing and distributing each of them. Distributing them brings them closer to where they are applied and makes them more sensitive to operational needs and changing environments. Centralizing them promotes consistency and facilitates disseminating policy and practice standards within them. Distribution may require investment in communication and collaboration technology to help achieve consistency. Centralizing them can create economies by simplifying the systems that a discipline may need to operate.
When information systems were more laborious, time-consuming and expensive to create, less malleable and more difficult to integrate they were an impediment to distributed governance and management. This is not to say that matrix management didn’t happen but it was much more burdensome than it is today. All in all, this contributed to a drag on the ability to initiate new business models—to transform. The early IS Director focused much more on cost-containment than innovation. A company’s infrastructure evolved by capital projects, funded in an all-or-nothing fashion during the annual budgeting cycle. There are a number of pathologies associated with this, about which I have written previously.
What Now?
We have reached a major turning point in how we (must) manage enterprises—the number one capability required to survive is business agility—the ability to evolve and react rapidly to respond to changes in the business environment. Information technology is immeasurably more capable than it was, it has become easier to implement in many ways and its price-performance has plummeted. The major concern relating to Technical Debt when systems were so time-consuming and expensive to create was cost; now it is its impact on agility. With businesses lifecycles accelerating, Technical Debt becomes less of a concern. It’s less important now that two product teams may create redundant application components than that their experimentation with evolving business models not be impeded by a desire to minimize Technical Debt.
There are any number of distinguished subject matter experts who have written about how enterprises must be transformed and operated to be competitive today. Two books that I heartily recommend are Designed for Digital-How to Architect Your Business for Sustained Success by Jeanne W. Ross, Cynthia M. Beath and Martin Mocker and Project to Product: How to Survive and Thrive in the Age of Digital Disruption with the Flow Framework by Mik Kersten.
Designed for Digital describes how enterprises should be architected and Project to Product addresses how business operational management must change to be competitive in today’s markets. What can be taken from both books is the need for tight collaboration between product owners and the technologists that enable them. As product-centric management approaches are rolled out, Capital Expenses (CapEx) transition to Operational Expenses (OpEx) and a new layer of concerns and operational responsibilities accrue to the CIO. Ross, Kersten and many others, as well as enterprises of all sizes have come to the realization that business success is inextricably tied to technological success and the CIO is the person to facilitate that.
As we go forward, any number of advancements will make it easier to implement and exploit technology—Low-Code/No-Code programming environments, intelligent automation that generates, optimizes and deploys Machine Learning models with minimal intervention, Agile/DevOps and new Cloud-based software architectures that improve implementation velocity, performance, malleability and maintainability. Technical Debt will come to matter less and less but the impact on an enterprise’s ability to implement, evolve and operate technology-enabled business models will matter more and more.
I agree with the WSJ’s assertion—CIOs’ and COOs’ responsibilities and job descriptions will begin to merge. The question will become whether it is easier to teach technology to the COO or business to the CIO. I believe that it is important that anyone in, or who aspires to, either role focus on filling technological or business gaps in their background. Competitive organizations will be looking for leaders who present the fusion of skills required to oversee a Digital Transformation, lead the way to rapid innovation and deliver digital products and services.
If you’re a CIO with significant business acumen, and there is every reason to believe that if you are a CIO you have plenty already, then it’s a good time.
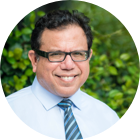
MMS • RSS
Article originally posted on Data Science Central. Visit Data Science Central
Here I share my predictions as well as personal opinion about the pandemic. My thoughts are not derived from running sophisticated models on vast amounts of data. Much of the data available has major issues anyway, something I am also about to discuss. There are some bad news and some good news. This article discusses what I believe are the good news and bad news, as well a some attempt at explaining people behavior and reactions, and resulting consequences. My opinion is very different from what you have read in the news, whatever the political color. Mine has I think, no political color. It offers a different, possibly refreshing perspective to gauge and interpret what is happening.
I will start by mentioning Belgium, one of the countries with the highest death rate. Very recently, it went from 10,000 deaths to 15,000 in the last wave, in a matter of days. They are back in some lock-down, and the situation has dramatically improved in the last few days. But 15,000 deaths out of 10,000,000 people would translate to 500,000 deaths in US. We are far from there yet. Had they not mandated a new lock-down, killing restaurants and other businesses but keeping schools open along the way, they would probably have 20,000 deaths now, probably quickly peaking at 25,000 before things improve. Now we are comparing apples and oranges. In Belgium, everyone believed to have died from covid was listed as having actually died from the virus even if un-tested. Also, the population density is very high compared to US, and use of public transportation is widespread. Areas with lower population density have initially fewer deaths per 100,000 inhabitants, until complacency eventually creates the same spike.
The bad news is that I think we will surpass 500,000 deaths in US by the end of February. But I don’t think we will ever reach 1,000,000 by the end of 2021. A vaccine has been announced for months, but won’t be available to the public at large in time: only to some specific groups of people (hospital workers) in the next few months. By the time it will be widely available, we will all have been contaminated / infected and recovered (99.8% of us) or dead (0.2% of us). The vaccine will therefore be useless to curtail the pandemic, which by then will have died out of its own due to lack of new people to infect. It may still be useful for the future, but not to spare the lives of another 300,000 who will have died between now and end of February.
You may wonder: why not imposing a full lock-down until March? Yes this will save many lives but kill many others in what I think is a zero-sum sinister game. Economic destruction, suicide, drug abuse, crime, riots would follow and would be just as bad. And with surge in unemployment and massive losses in tax revenue, I don’t think any local or state government has the financial ability to do it, it is just financially unsustainable. So I think lock-downs can only last so long, probably about a month or so maximum. What is likely to happen is more and more people not following un-enforced regulations anymore, and those who really need to protect themselves, will stay at home and continue to live in a self-imposed state of lock-down.
Now some good news at least. It is said that for anyone who tests positive, 8 go untested because symptoms are too mild or inexistent to require medical help, and thus are not diagnosed. Me and my whole family and close friends fit in that category: never tested, but fully recovered, with no long-term side effects. Have we been re-infected again? Possibly, but it was even milder the second time, and again none of us were tested. One reason for not being tested / treated is that going to an hospital is much more risky than dining-in in a restaurant (many hospital workers died from covid, much fewer restaurant workers did). Another reason is to not have a potentially worrisome medical record attached to my name. Now you can say we were never infected in the first place, but it’s like saying the virus is not contagious at all. Or you can say we will be re-infected again, but it’s like saying the vaccine, even two doses six months apart, won’t work. Indeed we are very optimistic about our future, as are all the people currently boosting the stock market to incredible highs. What I am saying here is that probably up to half of the population (150 million Americans) are currently at the end of the tunnel by now: recovered for most of us, or dead.
Some people like myself who had a worse-than-average (still mild) case realize that wearing a mask causes difficulty breathing worse than the virus itself. I don’t have time to wash my mask and hands all the time, or buy new masks and so on, when I believe me and my family are done with it. Unwashed, re-used masks are probably full of germs and worse than no mask, once immune. As more and more people recover every day in very large numbers these days (but the media never mention it) you are going to see more and more people who spontaneously return to a normal life. These people are not anti-science, anti-social, or anti-government – quite the contrary, they are acting rationally, not driven by fear. They don’t believe in conspiracy theories, and are from all political affiliations or apolitical. Forcing these people to isolate via mandated lock-downs won’t work: some will have big parties in private homes, a hair-dresser may decide to provide her services privately in the homes of her clients, and be paid under the table. People still want to eat great food with friends and will continue to do so. People still want to date. Even if the city of Los Angeles makes it illegal to meet in your home with members from another household, you can’t stop young (or less young) people from dating, not any more than you can stop the law of gravity no matter how hard you try.
Of course, if all the people acting this way were immune, it would not be an issue. Unfortunately, many people who behave that way today are just careless (or ignorant, maybe not reading the news anymore). But as time goes by, even many of the careless people are going to get infected and then immune; it’s a matter of weeks. So the intensity of this situation may peak in a few weeks and then naturally slow down, as dramatically as it rose.
In conclusion, I believe that by the end of March we will be back to much better times, and covid will be a thing of the past for most of us. Like the Spanish flu. Though it is said that the current yearly flu is just remnants from the 1918 pandemic. The same may apply to covid, but it will be less lethal moving forward, after having killed those who were most susceptible to it. Already the death rate has plummeted. This of course won’t help people who have lost a family member or friend, you can’t make her come back. This is the sad part.
About the author: Vincent Granville is a data science pioneer, mathematician, book author (Wiley), patent owner, former post-doc at Cambridge University, former VC-funded executive, with 20+ years of corporate experience including CNET, NBC, Visa, Wells Fargo, Microsoft, eBay. Vincent also founded and co-founded a few start-ups, including one with a successful exit (Data Science Central acquired by Tech Target). You can access Vincent’s articles and books, here.
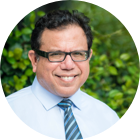
MMS • RSS
Article originally posted on Data Science Central. Visit Data Science Central
Augmented Reality is beginning to push the paradigms of previous expectations as the ability to recognize cards by appearance and orientation opens up the possibility of a new kind of gaming. The use of “magical” proxies to position 3D animations coupled with autonomous agent awareness opens up the possibility of card games with armies attacking castles, chess games going off into n-dimensions, and table top role playing games (RPGs) creating fully simulated mage on warrior battles.
More here.
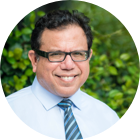
MMS • RSS
Article originally posted on Data Science Central. Visit Data Science Central
The pandemic has shown how vulnerable our supply chains are. A small interruption can bring a supply chain to a halt, which in today’s fast-paced world, is simply not acceptable. Customers are always expecting their product to arrive on time when ordered, and any delay in the supply chain can have significant consequences for organisations.
What today’s supply chains require is speed, agility and flexibility to respond quickly to changes in demand. The pandemic has shown us that demand and requirements can change rapidly, and those organisations that were not able to easily switch their products from geographically distributed stores to centralised warehouses for online orders missed out significantly.
Moving from Doing Digital to Being Digital
To avoid these problems, supply chains have to go digital. This does not mean merely doing digital, as in having your inventory in the cloud, but it means being digital. When you are digital, as an organisation or a supply chain, it offers new opportunities. It provides the speed, agility and flexibility that today’s fast-changing world requires.
Being digital means moving your entire supply chain to the cloud. It means enabling your customers to connect with you via any channel at any time. It also means hiring not only humans but also machines to improve your processes. When you move from doing digital to being digital, you enable human-to-machine collaborations that will bring great results.
This week, I attended Manhattan Exchange 2020, and they shared great stories on how they help organisations turning their supply chain digital. During the completely virtual event, they shared tons of customer stories, including those of L’Oréal, DIESEL and Skechers, showing that their customers can manage complex networks of billions of products moving seamlessly to market using an omnichannel strategy.
Cloud Solutions are the Way Forward for Supply Chains
Intelligent cloud solutions are the way forward, and they can add significant value. It gives organisations and supply chains the required speed, flexibility and scalability. A cloud environment enables greater collaboration as it gives companies the ability to share data more easily compared to non-cloud environments. Product and customer data can be quickly shared across locations and external collaborators.
The Italian retail clothing company DIESEL was able to move its logistics to the cloud. This transformation gave them full control over their eCommerce operations and customer care, offering customers a seamless shopping experience.
Improving Customer Care
An intelligent cloud environment will also result in improved customer care. In today’s world, your customers’ expectations should be leading in how you design your supply chain. Technology can help you achieve a customer-centric supply chain, where each of your customers has a personalised experience when interacting with your products.
Of course, if you sell billions of products per year, this is a major challenge. The French personal care company L’Oréal knows all about how to do this correctly. It is the world’s largest cosmetics company. The company consists of 4 division, 500 brands, of which 36 iconic brands and thousands of products. They have 150 distribution warehouses across the world. With so many products and customers, they need to be able to meet peak demands, especially when the world moved to online shopping earlier this year.
During the event, L’Oréal shared their insights on how they will use Manhattan’s native cloud solution in their distribution centres. One of the benefits of Manhattan’s Active Warehouse Management solution is that it no longer requires updates as it is a version-less product. Therefore, L’Oréal expects to become more flexible and agile by digitalising its supply chain and operate more efficiently in their warehouses, which in the end will result in higher customer satisfaction.
Human-to-Machine Collaboration
While a digital environment helps to make your supply chain more agile and flexible, hiring not only humans but also machines will make your logistical processes more efficient and effective. That is why Skechers, a lifestyle and performance footwear company, heavily focuses on automation using machines. Especially due to the pandemic, the company had to scale up quickly and robots where the best approach to not only meet the demands but also save costs. Thanks to man and machine collaboration in their warehouses, Skechers can meet (peak) demands, urgent orders and ensure continuous customer satisfaction.
Even more, digital tools can also help make your warehouses safer in times of the pandemic. After all, warehouse worker safety should be a priority for any organisation. That is why Manhattan partnered with Zebra Technologies to ensure social distancing in narrow picking alleys within warehouses. Picking routes are adapted continuously to avoid congestions and queues while offering employees proximity alerts—a great way how humans and machines can collaborate to ensure a safe environment.
Conclusion
Retailers around the world have to update and improve their supply chain processes continuously. Those companies that manage to ingest their supply chains with intelligence using native cloud solutions such as developed by Manhattan Associates prepare their business for tomorrow. It will enable them to bring products to market faster across the globe and redirect (return) products quickly when needed. This agility is essential as ‘even when the world stops turning, logistics never stops’, as stated by Sophie Houtmeyers, VP Distribution Operations Wallonia Skechers.
It was a great event, and I am looking forward to next year’s event to see how supply chains have changed due to this pandemic. I think those companies that can transform their logistics into digital platforms will benefit the most and will ensure customer loyalty
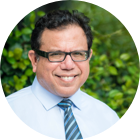
MMS • RSS
Article originally posted on Data Science Central. Visit Data Science Central
Network graphs play a large part in both computing and data science, and they are essential for working with (and visualizing) both semantic graphs and property graphs. Nearly thirty years ago, AT&T produced a set of libraries called graphviz which were designed to generate various types of printed output. Over the years, the library has been adapted for different platforms and roles, and today is still one of the most widely used network graph visualization tools around.
One of the most common libraries associated with graphviz is the DOT library. DOT is a declarative language that let’s users specify the components of a network graph, including the nodes (or dots) that are typically used to represent entities, along with the directed edges that are used to specify relationships or attributes. While there are other things that DOT can be used for, the language is powerful enough to create graphs with hundreds or even thousands of nodes, and serves as the foundation for other visualization libraries when moving out beyond that scale.
At its core, DOT usually specifies directed graphs, or graphs in which each edge has a specific direction of traversal. You can experiment with DOT using the GraphViz Online viewer. One of the simplest such DOT file graphs looks something like this:
The DOT file that describes this graph is about as simple:
digraph G1 { JaneDoe;
Programmer; JaneDoe -> Programmer;
}
The digraph term indicates that this is a directional graph (one of a number of different kinds of graphs), with the G1 indicating the identifier of the graph. Graphs can be used within other graphs, and as such can also be named. The identifiers can either be alphanumeric sequences (along with the underscore character) or can be strings delimited with quotation marks. These identifiers serve as labels if no labels are otherwise given.
Thus, in this example, the graph identifies two nodes (JaneDoe and Programmer) and one edge (JaneDoe -> Programmer) which creates a relationship between the two indicated by an arrow terminated line from one element to another, which is indicated by a right pointing arrow.
If you have a sequence such as JaneDoe -> Programmer -> Javascript, this creates a set of nodes JaneDoe, Programmer and Javascript if they don’t exist already exist, with arrows between them:
digraph G2 {
JaneDoe -> Programmer -> Javascript;
}
For single word graphs, this is probably sufficient, but you can also “decorate” each node and edge, using attributes and labels. Suppose, for instance, that you wanted to include a class indicator with each node, and wanted some kind of relationship between each node. This where labels come in handy:
digraph G3 {
JaneDoe [label="<Person>nJane Doe"];
Programmer [label="<Profession>nProgrammer"];
Javascript [label="<Language>nJavascript"];
JaneDoe -> Programmer [label=" has profession"];
Programmer ->Javascript [label=" has language"];
}
One of the great things about DOT is that you generally do not need to worry about layout, fitting text to different shapes, or managing overlap. In the example for G3, line breaks can be added in with the “n” newline character, letting you create multiline labels. Similarly, the DOT algorithms will place the edge labels to avoid (as much as possible) overlapping with either shapes or other edges.
The attributes section (indicated by square brackets) can also include other formatting metadata. For instance, suppose that you wanted each class to have its own color, wanted the text to be in Arial (or Helvetica) and wanted the text to be white on dark color backgrounds, you can use additional DOT styles that correspond (roughly) to basic CSS.
digraph G4 {
edge [fontcolor=black fontsize="10" fontname=Arial];
node [style="filled" fontcolor=white fontsize="12" fontname=Arial];
JaneDoe [label="<Person>nJane Doe" fillcolor="blue"];
Programmer [label="<Profession>nProgrammer" fillcolor="darkGreen"];
Javascript [label="<Language>nJavascript" fillcolor="green" fontcolor="black"];
JaneDoe -> Programmer [label=" has profession"];
Programmer ->Javascript [label=" has language"];
}
In G4, notice the use of the edge and node descriptors. These provide a way to create a common description for a node or edge that everything in the graph uses. This edge instance, for example, sets the font color (black) font size (10pt) and font name (Arial). The node sets the default color for text (white) and also indicates that the shape in question is filled. Each node entry can then override certain values (such as the font color for Javascript being set to black because the light green is light enough that white text becomes difficult to showcase.
With DOT, you can also create subgraphs, which can both have associated traits and can serve to aggregate groups of content and apply node and edge characteristics at different scopes:
digraph G5 {
edge [fontcolor=black fontsize="10" fontname=Arial];
subgraph referenceNodes {
node [style="filled" fontcolor=white fontsize="12" fontname=Arial];
subgraph person {
node [fillcolor="blue"];
person_JaneDoe [label="<person>nJane Doe"];
person_WendyDoe [label="<person>nWendy Doe"];
person_StacyDoe [label="<person>nStacy Doe"];
}
subgraph profession {
node [fillcolor="darkGreen"];
profession_Programmer [label="<profession>nProgrammer"];
profession_Writer [label="<profession>nWriter"];
}
subgraph vehicle {
node [fillcolor="purple"];
vehicle_Subaru [label="<vehicle>nSubaru"];
vehicle_Ford [label="<vehicle>nFord"];
}
}
subgraph dataNodes {
node [shape=box;style="filled";fillcolor=yellow;fontcolor=black;fontsize="12";fontname=Arial];
value_age24_1 [label="<integer>n24";];
value_age24_2 [label="<integer>n24";];
value_age21_1 [label="<integer>n21";];
}
subgraph JaneDoe {
person_JaneDoe -> person_WendyDoe [label=" has sister";dir=both];
person_JaneDoe -> person_StacyDoe [label=" has sister";dir=both];
person_JaneDoe -> profession_Programmer [label=" is a"];
person_JaneDoe -> vehicle_Subaru [label=" owns a"];
person_JaneDoe -> value_age24_1 [label="has age"];
}
subgraph WendyDoe {
person_WendyDoe -> profession_Writer [label=" is a"];
person_WendyDoe -> vehicle_Subaru [label=" owns a"];
person_WendyDoe -> value_age24_2 [label="has age"];
person_WendyDoe -> person_StacyDoe [label=" has sister";dir=both];
}
subgraph StacyDoe {
person_StacyDoe -> profession_Writer [label="is a"]
person_StacyDoe -> vehicle_Ford [label="owns a"]
person_StacyDoe -> value_age21_1 [label="has age"];
}
}
Here we have the tale of three sisters, Jane, Wendy, and Stacy Doe. Wendy and Stacy are both writers, while Jane is a programmer. Wendy and Jane drive Subarus, while Stacy drives a Ford. Jane and Wendy are both 24, Stacy is 21.
The subgraphs in G5 serve to create logical groupings based upon common type. The hasSister relationship is noteworthy because of its symmetry – there are arrowheads on both sides, set by the dir=both attribute. The has age relationship is also noteworthy in that you have an ellipse (the default) pointing to a box as specified via the shape attribute. There are roughly thirty shapes in the core DOT specification, although different implementations may offer more or fewer. Also notice that atomic values (in the rectangles) are defined with an identifier that is unique per box, (e.g., value_age24_1 vs. value_age24_2. Without this distinction, the arrows for age24 would all point to the same box (Proponents of semantics might argue that they should point to the same box, but this can make for confusing graphs if you have a large number of atomic values).
Note also that, unless otherwise specified, DOT will usually create curved edges when you have topologies that don’t fit neatly into rectangular arrays. DOT, out of the box, attempts to optimize for tension and legibility, though it is possible to change how nodes and edges are laid out that are fodder for a more advanced article. Other capabilities not covered here (and likely will be covered in a subsequent article) include the use of images, an understanding of how to utilize shapes, and the incorporation of HTML content.
DOT (via the command line GraphViz library or through node and related libraries) is capable of producing PNG and SVG versions of graphics, and some implementations include support for TIFF, JPEG, Postscript and other outputs. DOT is (with a few exceptions) consumable by both the vis.js and d3.js node libraries, among others. You can also find up to date documentation on both GraphViz and DOT on the GraphViz Documentation page.
It should also be pointed out that, as you gain proficiency with DOT, patterns for utilizing it to build different types of network graphs programmatically should become more and more evident. These will be explored in a subsequent article as well. Regardless, DOT can be used to build not only network graphs but flowcharts and state diagrams as well, making it a useful tool for any data scientist or programmer who would prefer not to have to recreate the wheel for visualizations.
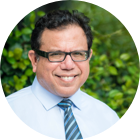
MMS • RSS
Article originally posted on Data Science Central. Visit Data Science Central
Recently, someone (not me) suggested in a tweet to Judea Pearl, let’s have a Kaggle** competition on the topic of Causal AI. I love the idea, so I am trying to promote it with this blog post. Big Pharma could fund such a competition for much less than what they pay for a TV commercial, and the technical insights they might gain from this exercise might prove to be invaluable to the pharmaceutical industry. (Plus it would be good publicity for them, much better than a lame TV commercial.) . It would not be the first time either. For instance, Merck** has hosted Kaggle competitions in the past.
Judea Pearl, who won the Turing Award (often called the “Nobel prize” of computer science) for his work in Bayesian Networks and the closely related subject of Causal AI, might be willing to help design such a contest. Another Turing Award winner, Yoshua Bengio, who has recently been working on CausalWorld, might also be willing to help. The Columbia Uni professor and famous ex-student of Judea Pearl, Elias Bareinboin, might be willing to help too. There is no shortage of experts in this field that might be willing to help design what, there is no doubt in my mind, would be a historic, history changing, seminal, mind bending event.
Causal AI is certainly a worthy topic for Kaggle and Pharma to pursue. So far, Machine Learning is just glorified curve fitting. The next step in mimicking human reasoning is described by Pearl’s Theory of Causality far better and more explicitly than by any other theory I’ve ever seen. And Pharma would benefit greatly by using in the drug discovery process, a theory that harnesses the crucial differences between correlation and causation in a logical, self-consistent, quantitative way, as does Pearl’s Theory of Causality.
**Kaggle (which rhymes with gaggle), is a company that holds machine learning competitions, with prize money. It was founded in 2010 and acquired by Google Alphabet in 2017. By now, Kaggle has hosted hundreds of competitions, and played a significant role in promoting Data Science and Machine learning. For example, a team including the Turing award winner Geoffrey Hinton, won first place in 2012 in a competition hosted by Merck. Here is an excerpt from Wikipedia’s Kaggle entry:
In June 2017, Kaggle announced that it passed 1 million registered users, or Kagglers.[3] The community spans 194 countries. It is the largest and most diverse data community in the world[citation needed], ranging from those just starting out to many of the world’s best known researchers.[4]
Kaggle competitions regularly attract over a thousand teams and individuals. Kaggle’s community has thousands of public datasets and code snippets (called “kernels” on Kaggle). Many of these researchers publish papers in peer-reviewed journals based on their performance in Kaggle competitions.[5]
By March 2017, the Two Sigma Investments fund was running a competition on Kaggle to code a trading algorithm.[6]
Haggling Kaggle of Geese, or Kaggling Gaggle of Geese
When Daphne Koller met Judea Pearl. When Feynman met Dirac. When The Beatles met Bob Dylan.
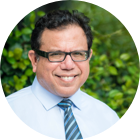
MMS • RSS
Article originally posted on Data Science Central. Visit Data Science Central
Daphne Koller is the leader of a mega-startup (Insitro) that uses Machine Learning (do they use Causal Bayesian Networks???) to do drug research. She also co-founded Coursera with Andrew Ng, and she co-wrote with Nir Friedman a 1200 page book about Probabilistic Graphical Models (e.g., Bayesian Networks)
Judea Pearl won a Turing award (commonly referred to as the Nobel prize of computer science) for his work on Bayesian Networks and Causal AI.
Recently, I posted a Tweet about Daphne Koller to which Judea Pearl responded with a question to Daphne Koller
https://twitter.com/yudapearl/status/1330393994661154817
Both Daphne Koller and Judea Pearl are longtime heroes of mine. I would be so thrilled to be a fly on the wall that could listen to their conversations. All this started me thinking that it would be highly instructive and inspiring, not just to me, but to other fans of theirs and to Bayesian Net/ Causality aficionados all over the world, to hear them converse and ruminate in public (verbally or in writing). Plus, I think it could prove to be highly beneficial to both of them. There is historical precedent where a conversation between two towering scientific or artistic figures, which Daphne Koller and Judea Pearl certainly are, caused the two figures to make important course corrections to their scientific and artistic styles. I end with two examples when this happened, the first example (The Beatles meet Bob Dylan) is probably apocryphal but rather funny, but the second example (Feynman meets Dirac) is well documented.
It’s not uncommon for writers to write stories about conversations between famous historical figures, call them A and B, conversations that changed the world. Sometimes those conversations are obvious fabrications, fun exercises of imagination, because A and B did not overlap temporally.
At others times, the historical record shows conclusively that A and B did meet, but the stories about said meeting sound a bit too perfect to be true. For example, I once read a funny story about the day The Beatles met Bob Dylan. Supposedly, Bob Dylan told the Beatles that their songs were too easy to understand, and advised them to be more cryptic, resulting in a new, more trippy style for the Beatles, the one in the Sgt. Peppers album.
Sometimes, the historical record is much less flimsy and more believable, as happened with A=Richard Feynman (1918-1988) and B=Paul Dirac (1902-1984). Those 2 met in at least 3 scientific conferences in 1946 (Princeton Univ. ), in 1948 (Poconos), in 1963 (Warsaw), so their conversations spanned a period of 17 years. The story of those 3 encounters is beautifully told in the article given below. Dirac was notoriously eccentric and terse, whereas Feynman was ebullient and charismatic. So their encounters were hilarious.
But a more serious take-away is that in their first encounter, Dirac and a bunch of other famous physicists were very critical of Feynman’s theory of quantum electrodynamics. They thought it was too hand-wavy and violated many important basic principles of physics. Feynman’s pride was badly hurt, so over the next 3 years, he buckled down, and wrote the 4 papers that put his hand-wavy theory on firmer footing and ultimately won him the Nobel Prize in Physics, in 1965, together with Tomonaga and Schwinger.
“When Feynman Met Dirac” by Jørgen Veisdal (link to Medium blog post)
If you don’t suscribe to Medium, you might be able to access it via an incognito tab in your browser, or via this twitter tweet:
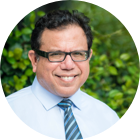
MMS • RSS
Article originally posted on Data Science Central. Visit Data Science Central
Humans don’t start their thinking from scratch every second. As you read this essay, you understand each word based on your understanding of previous words. You don’t throw everything away and start thinking from scratch again. Your thoughts have persistence.
Traditional neural networks can’t do this, and it seems like a major shortcoming. For example, imagine you want to classify what kind of event is happening at every point in a movie. It’s unclear how a traditional neural network could use its reasoning about previous events in the film to inform later ones.
Recurrent neural networks address this issue. They are networks with loops in them, allowing information to persist.
Recurrent Neural Networks have loops.
In the above diagram, a chunk of neural network, A, looks at some input Xt and outputs a value ht. A loop allows information to be passed from one step of the network to the next.
These loops make recurrent neural networks seem kind of mysterious. However, if you think a bit more, it turns out that they aren’t all that different than a normal neural network. A recurrent neural network can be thought of as multiple copies of the same network, each passing a message to a successor. Consider what happens if we unroll the loop:
An unrolled recurrent neural network.
This chain-like nature reveals that recurrent neural networks are intimately related to sequences and lists. They’re the natural architecture of neural network to use for such data.
And they certainly are used! In the last few years, there have been incredible success applying RNNs to a variety of problems: speech recognition, language modeling, translation, image captioning… The list goes on. I’ll leave discussion of the amazing feats one can achieve with RNNs to Andrej Karpathy’s excellent blog post, The Unreasonable Effectiveness of Recurrent Neural Networks. But they really are pretty amazing.
Essential to these successes is the use of “LSTMs,” a very special kind of recurrent neural network which works, for many tasks, much better than the standard version. Almost all exciting results based on recurrent neural networks are achieved with them. It’s these LSTMs that this essay will explore.
The Problem of Long-Term Dependencies
One of the appeals of RNNs is the idea that they might be able to connect previous information to the present task, such as using previous video frames might inform the understanding of the present frame. If RNNs could do this, they’d be extremely useful. But can they? It depends.
Sometimes, we only need to look at recent information to perform the present task. For example, consider a language model trying to predict the next word based on the previous ones. If we are trying to predict the last word in “the clouds are in the sky,” we don’t need any further context — it’s pretty obvious the next word is going to be sky. In such cases, where the gap between the relevant information and the place that it’s needed is small, RNNs can learn to use the past information.
But there are also cases where we need more context. Consider trying to predict the last word in the text “I grew up in France… I speak fluent French.” Recent information suggests that the next word is probably the name of a language, but if we want to narrow down which language, we need the context of France, from further back. It’s entirely possible for the gap between the relevant information and the point where it is needed to become very large.
Unfortunately, as that gap grows, RNNs become unable to learn to connect the information.
In theory, RNNs are absolutely capable of handling such “long-term dependencies.” A human could carefully pick parameters for them to solve toy problems of this form. Sadly, in practice, RNNs don’t seem to be able to learn them. The problem was explored in depth by Hochreiter (1991) and Bengio (1994), who found some pretty fundamental reasons why it might be difficult.
Thankfully, LSTMs don’t have this problem!
LSTM Networks
Long Short Term Memory networks — usually just called “LSTMs” — are a special kind of RNN, capable of learning long-term dependencies. They were introduced by Hochreiter & Schmidhuber (1997), and were refined and popularized by many people in the following work.1 They work tremendously well on a large variety of problems and are now widely used.
LSTMs are explicitly designed to avoid the long-term dependency problem. Remembering information for long periods of time is practically their default behavior, not something they struggle to learn!
All recurrent neural networks have the form of a chain of repeating modules of neural network. In standard RNNs, this repeating module will have a very simple structure, such as a single tanh layer.
The repeating module in a standard RNN contains a single layer.
LSTMs also have this chain like structure, but the repeating module has a different structure. Instead of having a single neural network layer, there are four, interacting in a very special way.

In the above diagram, each line carries an entire vector, from the output of one node to the inputs of others. The pink circles represent pointwise operations, like vector addition, while the yellow boxes are learned neural network layers. Lines merging denote concatenation, while a line forking denote its content being copied and the copies going to different locations.
The Core Idea Behind LSTMs
The key to LSTMs is the cell state, the horizontal line running through the top of the diagram.
The cell state is kind of like a conveyor belt. It runs straight down the entire chain, with only some minor linear interactions. It’s very easy for information to just flow along it unchanged
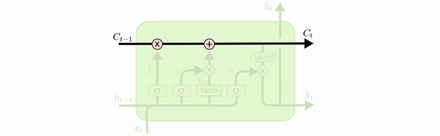
The LSTM does have the ability to remove or add information to the cell state, carefully regulated by structures called gates.
Gates are a way to optionally let information through. They are composed out of a sigmoid neural net layer and a pointwise multiplication operation.
The sigmoid layer outputs numbers between zero and one, describing how much of each component should be let through. A value of zero means “let nothing through,” while a value of one means “let everything through!”
An LSTM has three of these gates, to protect and control the cell state.
LSTMs (or long-short term memory networks) allow for analysis of sequential or ordered data with long-term dependencies present. Traditional neural networks fall short when it comes to this task, and in this regard an LSTM will be used to predict electricity consumption patterns in this instance.
One particular advantage of LSTMs compared to models such as ARIMA, is that the data does not necessarily need to be stationary (constant mean, variance, and autocorrelation), in order for LSTM to analyse the same — even if doing so might result in an increase in performance.
What Are Citizen Data Scientists and Can They Improve the Quality of Business Intelligence?
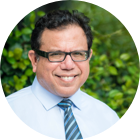
MMS • RSS
Article originally posted on Data Science Central. Visit Data Science Central
Citizen Data Scientists represent a new breed of business analysts – a group of individuals with diverse business responsibilities and training, who wield sophisticated analytical tools, and employ complex methods of analysis to improve business results – all without the training or assistance of data scientists or IT team members. Citizen Data Scientists are business users who leverage the knowledge and skill they have developed through professional training and business roles to navigate business intelligence and advanced analytical tools to answer business questions, identify trends and patterns and develop new competitive strategies to support business decisions.
Citizen Data Scientists work in a self-serve, easy-to-use business intelligence model wherein they can share data, produce reports and test hypotheses and theories. Citizen Data Scientists are not trained as analysts or technology experts. They come from every corner of the organization, from sales and marketing to finance, HR, production and purchasing and they use advanced data analytics to make decisions in support of their business team, division or unit.
Can Citizen Data Scientists Improve the Quality of Business Intelligence?
When an organization integrates data sources and enables self-serve, Advanced Data Discovery and analysis, the business can cascade knowledge and skill and make it easier for every business user to complete tasks, make accurate decisions and perform with agility in a fast-paced business environment. In a self-serve business intelligence environment, every team member is empowered to find and use clear, accurate data.
The Gartner report entitled, ‘Citizen Data Science Augments Data Discovery and Simplifies Data Science,’ states that, ‘the number of Citizen Data Scientists will grow five times faster than the number of highly skilled data scientists.’
By providing these crucial self-serve analytical tools to each team member, the business ensures that every location, business unit, division and team will work with consistent, uniform, clear data to make decisions, forecast future results and plan for market entry, new products and competitive initiatives.
Citizen Data Scientists use drag and drop tools, and features like Assisted Predictive Modelling, auto-suggestion and auto-recommendations to create, share and use predictive models to get data insights and use predictive analytics to their day to day Use Cases. These tools allow for sophisticated analysis without the assistance of precious IT and analyst resources and ensure that problems are solved quickly and that the organization can identify opportunities, and optimize resources at every level.
Citizen Data Scientists can go beyond data monitoring to discover those all-important nuggets of information that will make a real difference in business success.
Giving business users the right tools and a simple way to manage and analyze data and information encourages the adoption of business intelligence and analytical tools and assures that every team member an asset to your organization.
Original Post : What Are Citizen Data Scientists and Can They Improve the Quality of Business Intelligence?