Month: March 2023
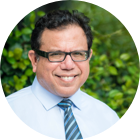
MMS • Peter Pilgrim
Article originally posted on InfoQ. Visit InfoQ
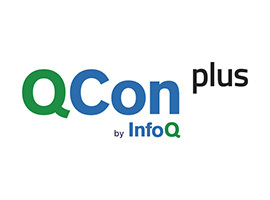
Transcript
Pilgrim: My name is Peter Pilgrim. I’m going to be talking to you about this, software engineering, sustainable empathic capacities, an excursion on the lived experience to minor creative genius. I am a Java champion, a senior manager at Cognizant. Previously, I was an independent contractor. I had this expansive view of software engineering. The first school of thought is that I would give an autobiographic view of my lived experience in software engineering. The second school of thought is that we are journeying, at least in my humble opinion, to empathy, to express empathy within our discipline.
Remote Sacking/Firing
First a corporate statement. The company has made a decision that the future of its vehicles going forward will be crewed by a third-party crew provider. Therefore, I’m sorry to say that this need to inform and employ you is terminated with immediate effect upon granted redundancy. Your final day of employment is today. Remote sackings and firings have happened so recently. British people wouldn’t have heard of better.com, but Americans, and Canadians, and North Americans have definitely heard of Vishal Garg, who fired over 900 employees over Zoom, in the 7th of December. How heartless and lacking in empathy was this, in the month of December? Yes, that’s it. Then in 17th of March, 2022, in Britain, on these shores, P&O Ferries dismissed 800 members of its shipping staff primarily from the Port of Dover, and that the British government didn’t take too well with this and the ramifications are still happening now.
What Is Empathy?
What is this thing that we are talking about? What is empathy? Where does it come from? This is a picture of the Homo sapien brain. It turns out, it’s a concoction. It’s from a German word, Einfühlung, which is a German psychological term, literally meaning feeling-in, inner, inside, intrinsic, the inner feeling. Germans are well known, famous for putting and inventing words from two separate words, so combining Ein, which is inner, and fühlung, which is feeling, to have this feeling in, or what we translate now, thanks to two scientists at Cambridge and Cornell University in 1908, as Empathos. Em is for in. Pathos is for feeling. That has been the early translation. This is why we have the word empathy in the English language. Thanks to Susan Lanzoni of Cambridge University Press.
Now for the technical details, or rather, the medical details. What seems to happen is we have an organ deep inside our brain, called the amygdala. This thing that looks like a grape or a squash grape, and we actually have two of this, because this picture is a cross section of the brain. We have our famous gray matter here, or the lobes, the frontal and parietal lobe, and the occipital lobe here, and the famous cerebellum, where we do a lot of our processing at the back of our brain. What seems to happen is that we have five sensitives, and I will concentrate just on one, the visual sense, when a photon hits the retina, a signal is transferred straight through our brain from left to right. Reach in and passing through the hypothalamus, which is this orange-yellow bit that looks like a bug’s head, looks like a squash potato, reaches the back of the occipital lobe here. Enters the cerebellum, and then magically, these signals are transferred via the hippocampus to the amygdala.
What happened recently, it’s been known since the 1960s, is that these signals hit the amygdala very quickly, so quickly they’re known as microexpressions or microsignals. They are the result of, I suppose, voluntary and involuntary emotional response conflicting with another human being. If you think about it, this is the very essence of communication. Here’s another human being, is threatening me, is he friendly, is he foe? What is it? We have to develop these social behaviors. This is because in the purple section here, we have an ancient part of our brain when we first cross-evolved through the millennia, or millions of years from amphibians that first called out of sea. We had lizard brains. What has happened through evolutions, we’ve kept on extending this brain structure, which we need.
It seems the amygdala is the heart of fear and flight anxiety. It’s part of our lizard brain, the limbic system. It’s responsible for connections, emotional learning, and memory regulation. That’s where the cerebellum comes in. It’s very complicated, and scientists are still trying to work out what is going on there. Essentially, emotions and empathy, and any chance of empathy comes from this little organ that looked like a grape or two grapes. Because we have one in the left and one on the right side of our brainstem, in our brains. We have two of them, just like two kidneys.
This is Labi Siffre, the famous singer, songwriter. I was listening to him a couple of days ago in fact, on a radio program, an interview he gave. I just quoted him here. “Humans tend to hate nuance.” I wonder why, especially in 2022. “We want to put things in a box, we want to compartmentalize. However, the reality is that there’s nothing simple about anything we do with human beings.” I love this next part. “We are complexity upon complexity upon paradox on toast.” That describes us. We have toast, the amygdala, and it’s pure. Because we have these lobes and this interpretation, we mix it all up, together. This is from the brilliant Labi Siffre talking about his musical performance, and what he thinks about musical performance. That is from the BBC, Colin Murray show, Midnight Meets, from the 4th of May, 2022.
I love this quote from Vex King. “It is your subconscious mind that is responsible for your beliefs. All that you perceive is a result of what you accept as true in the conscious mind.” You can choose to bury certain facts about the world, or have a total different independent view of the world for most human beings and what is deemed as reality. I would get vexed by Vex King, but you must live in a real world. How many people have had that said to them? The real world is what you actually believe to be true. You never suffer bullies or people when they force or coerce you into accepting something else that you know is true. That is not the way to live.
For me, as I was talking in the QCon London conference there, I had this observation in my life that, a change of contradictions, a series of disruptions that invalidates the assumptions or surroundings is a rug pull. I learned from my physics teacher who said, any news of the world that breaks the illusion, a system in a steady state condition is a whirlpool. My old physics teacher is proven right time over time. This isn’t me doing a somersault or the backflip, but it’s the start of my lived in experience. I went to a secondary school called Spencer Park in South London. Just like Harry Potter, we wore Blazers to school, in a secondary school. I have this coat of arms, Dieu defend le droit, defend the right, yours with God, defends the right, on my blazer here.
Feeling for Another’s Suffering/Vulnerability
I think at 13 years old, I finally figured out what I wanted to be in life. The first thing that happened is that I discovered Research Machines 380Z in the 1980s, because I joined the electronics club, [inaudible 00:10:55] and I, and Pete went to the computer club, and learned BASIC. What got me into computer club was there was a friendly face here, an empathic face. A face that I could ask, how does a computer work? I went to a parents’ evening where you could get the kids into extra-curricular subjects and you can stay behind after school and try certain things. I happened to talk to Mark and ask him, he was also in my school year, how does this computer work? He showed me. From that friendly face, this is why I’m talking to you now. This is proof beyond doubt that empathy does work, at least for me. Eventually, I persuaded my mum to get me an Acorn Electron. What I really wanted was the top right here, the BBC Micro Model B, but that cost £400. I ended up with the lower left, Acorn Electron. In the end, because the Acorn Electron is a cut-down BBC Micro, I was still able to program primitive games, learn assembly language, and write code like this that showed graphics. I honed my chops, just like a guitarist really, or a musician. I started coding. I really wanted to know more about it. I knew at 13 years old I was going to be something to do with computing. I didn’t know if it was going to be a software engineer or architect, I knew this was my vocation. I didn’t know how I was going to get there.
What I’m talking about here, I’m talking about the feelings for another, which is the seventh empathic capacity, which is, we express vulnerability when we’re learning. It takes somebody with compassion, and maybe even pity, and somebody who is formed enough to give us guidance. Mark could have told me to go just bugger off, or he could have been in a bad mood. Because he was there on that parent’s evening. Because I can imagine, I can play this game called perceptual positions, and imagine standing in Mark’s shoes, and a young black kid comes up to him and says, show me how this computer works. Maybe I was rude. I could have been good. I could have asked him. I cannot remember. I owe it to Mark because he set me on the road.
Why Are You Here?
Fast forward. This would have happened to you as well. If you perform and close your eyes, you will know how you found this mentor yourself. Once you always know, because you’re here watching this program, you can make it work for you always, just by using your cerebellum and imagining that you could get back to that moment when someone shows you your first code, your first project, your first laptop, your first ideas, or pointed you in a direction to become who you are or will be tomorrow.
Dealing with Restructuring
Let’s move on with a story. Graduated, ended up working in Germany. Came back to Britain in the mid-1990s just in time for Blur versus Oasis, discovered that. In fact, instead of working in the outskirts of London, the heart of the matter, or the technology was in central London in investment banking. At the same time, I knew about Bjarne Stroustrup and C++. I wanted to be just like the next Stan Lippman. I already became a Linux system admin here, almost did. Then, I happened to chance on to and stumble onto Java. I had stumbled onto Java a year before, but it didn’t click with me. When I joined banking, I said, this is Java? Then my life had changed. I was still interested in C++ a bit, but then Java definitely took over, by the millennium.
In the turn of millennium, Deutsche Bank, which is my first investment bank that I worked for. This is the office environment that I worked on, like hundreds of people with the old cathode ray tube monitors, maybe a few flat screens in this. This is before the financial bubble burst. Then, everything was hunky-dory, just like you’re using the best technology in the summer of the year 2000, and that lasted for another year to 2001. There was Java. There was all these Dotcoms, fabulous technologies. Java 2 was just about to come out. We still didn’t have broadband yet, if I remember, still had a bit of dialogue to get through. I was happy to hack on the bank’s computers there because they had the most up to date technologies there. It’s easy to be trapped in that environment. This is not less about empathy, but you can get trapped in a moment, the rewards of the environments that exist now, that means you can forget about tomorrow. September 11th happened, and the Dotcom bubble burst. I wish I was back at 1988. Life was a little bit simpler.
This is the biography continuing. Ten months later, my job was gone. There was Summer in the City. I became very lazy and very despondent. Ended up watching the football world cup. This is a guy called David Beckham, who’d take fabulous free kicks. Years later, I discovered agility and retrospectives, nearly 10 years later. If I could write or mark this on the board right then in the summer of 2001 and 2002 with a purple marker, this would be my positivity and how I felt. Because restructuring took it all out of me really. I was feeling really chipper, because I got money in compensation. Then everything trended down from there. You might wonder, what has this got to do with empathy? This is George Clooney and Kendrick, and if I’m Up in the Air, the movie, which does talk about restructuring and job loss. When you hit a downturn, you will become depressed, unless you’re one of these very lucky people who managed one week later to be rescued or find the next gig immediately. Banks then, especially Deutsche Bank, they paid for outplacement, a decent compensation, half a year’s salary, and help to kickstart a new job search and a new CV. A very good movie to watch, if you’re going through this.
Then, years later, I realized that everybody is sharing this shared pain, so feeling as another does in the shared circumstance. It’s birth, marriage, bereavement, war, famine, hunger, success, winning the world cup. Those are shared circumstances that you can share, that others can share with you. That is an empathy connection, which is useful in teams, for those of you who are managing, or thinking of managing. The first rule about restructuring, my advice, no matter, because it will happen to you. Your job is made redundant, you are never redundant. Say that again and say it well, your job is made redundant, you are never redundant. If you remember that phrase when it does eventually happen to you, because nobody ever escapes.
What do you have control of? You have control over you, your influence, and your reach. If you set fire to the school, with pupils in, you are influencing and harming students, as well as the teachers and the caretakers, so you have control and influence, and you have reach. The reach is to connect people to the wider community. It’s a very negative example, I know. Then let’s be Afrika Bambaataa about this in hip hop, turn that negative into a positive. That’s what I did with myself. I needed to feel restructuring. I needed that disappointment to lose the semifinal, or quarterfinal, if not the final itself in the tournament. Because I then asked questions about myself like a sportsperson. Where’s my community? What do I want to do? Should I stay with computing or leave it? I had empathetic thoughts. I was feeling these feelings but couldn’t coin a name for them, or couldn’t put my finger on to what I was feeling. Then, I happened to be contracting. I fell into contracting really. Then technology in the times changed, Java EE became big, Spring framework came out, financial services still had that rebound until 2008. Then it crashed again.
Imagining Another’s Thoughts and Feelings
Imagining another’s thoughts and feelings. I had dreams. What about other people in London, and also, working on technology? They had dreams, desires. I wanted to communicate. I wanted to learn and better myself. It just so happened that I finally did it. I didn’t destroy the world or blow up the world, I created this thing called the Java web user group, a user group because I asked a question, are there other independent engineers who are willing to travel with me to JavaOne 2004? It turns out not only I had the money because I was a contractor, but because I started this mini-community, I got good at the MPV parts of it as facilitating, connecting, organizing speakers, getting in contact with Wendy Devolder of Skills Matter. Making presentations, as well as at QCon, and eventually at JavaOne. Getting involved with other people in the wider community to do with Scala, and Groovy, Spring Boot, Hibernate, HTML5, and even JavaFX. There are 20 other technologies that I could list including OSGi, that I could throw in here. Eventually, in 2007, I became a Java champion. It’s because I was a contractor, I had money. I was able to travel and afford to see the greater and wider community that I became one myself. It’s not something that you force yourself or apply to join, other people have to look at your contributions to the wider Java community. This Java Champion program, that was created by Aaron Houston, once of Sun Microsystems, he was the global outreach for Sun Microsystems. He realized that this technology is just not a panacea. It is driven by the people who believe passionately that Java and technologies around Java can help in the world. That’s a huge lesson. He’s still out there somewhere.
At the same time, I was reading Malcolm Gladwell, about connectors are people you seem to know who seem to know everybody. You find connectors in every walk of like. They’re sociable, gregarious, naturally skilled at making and keeping in contact with friends and acquaintances. What Malcolm was talking about was the first genuine influencers, not the influencers that broadcast, but the influencers that facilitate, a facilitating influencer instead of taking money. In order to inspire interest, though, as a user group leader, and I tried to be as fair as I could. Be that connector between groups of diverse people and the audience. Remember to facilitate the conversation. I tried to do that and I did that well for much of my time as well in that user group.
Adopting Another’s Posture for Achievement
What I really did is seeing these disparate speakers and how they presented at not only the user group, the Java web user group, but also at conferences. I learned by watching Neal Gafter, and Joshua Bloch in Java, or Mark Reinhold, or Brian Goetz in Java, by adopting and learning and modeling their accents, and the way they attenuated their tone. They spoke. They informed. They were passionate. They drilled down. They were respectful. They had empathy. That’s who I wanted to be. I wanted to be like them. It’s like Gianfranco Zola, the Italian footballer. He was lucky enough to play with Diego Armando Maradona at Napoli. He always goes on. Zola always goes on, “I was so lucky to see Maradona train.” That’s a sporting, I suppose symbology works in technology too. If you can model excellence in your heroes and heroines, it is a good way to be. In order to do that, you need to understand empathy, which is what this talk is about.
Switching Careers
Hunky-dory came, you know what comes next. The huge financial crisis of Lehman Brothers. Eventually, contracting ended for me. I became full time. I survived Lehman Brothers financial meltdown. I knew many people at Lehman Brothers who were fantastic. On a Friday, sometime in September, everything’s hunky-dory, and over the whole weekend, a bank suddenly, an investment bank, Lehman Brothers suddenly gone. Incredible. This was a big meltdown. I don’t think we’ve had the boom time since 2008. Have you had boom times for the general population? Maybe Elon Musk had some boom times. We haven’t seen it, the ordinary folk in the trough. I stayed with investment banking. I tried up into 2012, I think, Olympic year. Then I’d had enough. Sometimes you do have to switch rails. My partner says, change it, leave it, or accept it. She is an NLP Master Practitioner. She has advised me time and time again, and other people have said the same thing. If you can’t change your organization then change the organization. In order to change the organization, you need empathy. That has to come from you. That’s the secret to that quote.
We get into the penultimate stage here. I left banking. This is the biographical part continuing, I became digital. That was the new way of earning money as a contractor. I stumbled into and I accepted digital web agency development. My head was blown. I heard about this user research and user centric web design, where you have call to action, the serious stuff, copywriting, content generation, structure of English, strategy, content strategy, all these new words. Here it is, including responsive website design. Suddenly, the frontend was taking off. Full stack hadn’t come quite in by 2013. I think full stack is a horrible term, it’s a lack of empathy, because people who are full stack, have a weakness. They may be good at frontend, but they may not be good at the backend, or in the Java side. I’ve never known someone to know all of the platform stack, including DevOps. It’s impossible. Computing subject matter is too big. If you want to get things done, you’re going to have to have empathy. You’ll find out next.
It is because at the same time, the governments were realizing that its digital websites were old, they were antiquated. They were broken, disparate. This lady here, Dame Martha Lane-Fox submitted a paper to Prime Minister David Cameron in 2010, to form gov.uk. This is basically to adopt agile and become saving space. Here, code is a communication vessel of the external intent and behavior, between two or more different human beings. Code itself had changed to be unmaintainable, to definitely maintainable. Somebody has to look at your code, and be able to compile it, or to transpile it, years from now. We have to be agile, because now things were changing.
I’m so ashamed that my first experience of XP was, I think, 2014, or 2015, where I actually paired with a lead developer who was better than I, in terms of the web, JavaScript side. I had to learn pairing with him and her. Then I stumbled into Santander and started programming, become a Spring Boot developer. What I discovered in the Santander project, which was 100% pairing in 2017, is that it really requires empathy. I didn’t have all the times then, but you have to be inclusive. I know this, people are racially inclusive. When you’re coding with a junior, that you have to allow juniors to make mistakes. Likewise, if you’re coding with someone better than you, then you are in the vulnerable state. The other thing about pairing, every two or three days, we would swap pairs, we would follow the Extreme or the Pivotal way of coding. No pair worked on the same story for two or three days, we will all swap a pair. Then we’d get knowledge sharing. That increases knowledge within the team. You’ll see that you quickly need to know each other’s thoughts and feelings for the win in order to get that agile performance and the team. That’s why people were using pair program, and finally I got it.
What I discovered is that in the industry, we do have this negative gamification, which is contempt. It’s a feeling of superiority over people, putting people down. What is required for high performance teams, and if you want to interview for a great team, you need compassion. It is required for effective agility. Then, when I dug deeper into the agile manifesto, one day, I was shocked to read that they’ve been talking about this in 2002. This type of situation goes on every day in marketing, management, external customers, internal customers, and yes, even developers don’t want to make hard tradeoff decisions, so they impose irrational demands for the imposition of corporate power structures. This isn’t merely about software development problem, it runs through Dilbertesque organization. Whoever wrote that as text was suffering because of lack of empathy. They were annoyed at what goes on in their organization. This is in 2001.
Interconnected, Empathetic Software Engineering
I joined that digital development. I had proper daily standups since January 2013. We are in 2020, and the world changed. I enjoyed my contracting days, but suddenly things changed. We had remote communication, remote repositories, remote deployment from being in remote laptops or positions, virtual private network issues. It was a whole smorgasbord, and then some. What’s my opinion of high-quality software engineering? A healthy codebase, systems and application looks like a model of communication between people who exude compassion, and empathy. For interconnected, empathetic software engineering, there are two sides to it. There’s the technical side, the maintainable side where we want less bit rot. We want testable code. We want adaptable code. We want code that is reusable, maybe, that is exchangeable, is that really required? Resilience? This is where we have the non-technical side. We want ethics, teamwork. We want to be open and seen. We want to be inclusive. We definitely need psychological safety, to ensure we also have fairness in the workplace. Because if people aren’t treated fairly, then you’re going to get attrition. We pair in a high-performance team, a high caliber team. Definitely, the people that I’ve worked with in those teams, they have synchronized and composed relationships. They get on. They have that trust and support. They collaborate. Sometimes that leads to innovation. If you want reusable fairness and innovation, get those things right.
Empathetic Toolset
What you need is this empathetic toolset, which first starts with actively listening. Then this is about the culture of content or addressing it. You definitely want to be actively listening, observing, and having that kinesthetic, that feeling. That is touching on the amygdala, listening. There’s three sides to every story. Everybody has strengths and weaknesses. We want to show that we comprehend others and other people. We want to avoid indignation. We want to show people are included. We can do that in the same way we date other people or interview. We mirror. We have rapport. We don’t want platitudes. We want to show that we are self-aware. We are sentient. We are nonjudgement. We also cultivate. We encourage. We collaborate. Then, if we are really lucky, and this is impossible, because even I have a big head, try to be egoless as much as possible. I know Ken Beck and the Scrum XP people proclaim this a lot.
High-Performance Teams
Evidence of high-performance teams. This comes from the Mind Gym, and less so about empathy, but really, is about it. Because the last bit of the sentence, the mixed gender groups consistently outperformed the single gender groups. This was a study about diversity, which is relevant. What’s even more relevant is Kelsey Hightower, who is a Kubernetes [inaudible 00:38:53], Developer Relations at Google. He talks about running a customer empathy session, where he puts Kubernetes engineers through the same challenges that his and her customers face. He’s still having fun. If you think you can avoid empathy, it’s there in the FAANG baby, in those FAANG companies, at least in one of them. It’s coming to a software developing engineering workshop and store that you are in right now. There is no escape, at least.
Empathic Capacities
Daniel Batson, who came up with the empathic capacities, American social psychologist, well known for the empathy-altruism hypothesis. Number one is knowing another’s thoughts and feelings. That is the feeling that we have almost when an event in a circumstance is happening, or when we think, just like the other person. Imagining another’s thoughts and feelings. This is slightly substantively different in that we are in a different plane. We are thinking of what it’s like to occupy that person’s corner of the universe. Adopting the posture of another person, the kind of method acting here. This is really useful for coaching. I believe this is true. Because if you want to learn from the best, then work with the best and model excellence. Feeling as another person does. This is the circumstances shared where you are in that same circumstance. You might be in Ukraine, in Mariupol right now, or luckily, you aren’t, you’re here with us, and you can definitely feel what is going on there.
Number five, imagining how one would feel or fit, or think in another’s place. Again, slightly related to number two and one, but this how is projecting into the future, this one here. If you cause somebody’s harm, or you put ice down that girl’s back, as you did as a child, what stops you doing that? That is not a nice feeling to do, because maybe that happened to you, someone squirted you with water, and you understand pleasure as well as pain. You can imagine how one would feel in another’s place. Feeling distress at another’s suffering. This is an easy one. Bereavement, somebody’s pet has died, family member receives medical bad news. Somebody has taken sick at work. The announcement of terminal cancer is a really severe one, and you can treat it with platitude. If you have a similar distress, or you know a family member that’s had that similar distress, you will know number six. Feeling for another’s suffering, which is the charitable way. That is more to do with pity and compassion. That means you’re not actively involved, but you can feel. It is closely related to five, really, I think. You can feel without actually taking aboard all their emotions. Number eight is the classic projecting oneself into another situation, the actor. Imagine yourself emoting, being in that same person’s shoes, as one says.
Conclusion
An inclusive environment is necessary to reap the benefits of employing a diverse group of people. I’m going to leave you with empathic leadership tips here. For those of you who are leaders, or want to become leaders, you want this seat right now that I’m sitting in, you definitely want to look at and study the things in yellow boxes, which is the blame culture, the unblocking of encumbrances, the sanctuary, and the flexible communication. I’m going to leave you with Bob Odenkirk from Breaking Bad, and Better Call Saul. He says you’re not owed a career just because you want one. How true is that? Empathy. We continuously practice empathy even when we don’t have a lot of it to start with. We share in everyone else’s lived-experiences in order to build that better future for all of us, so say we all.
See more presentations with transcripts
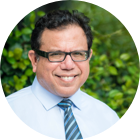
MMS • Mehrnoosh Sameki
Article originally posted on InfoQ. Visit InfoQ
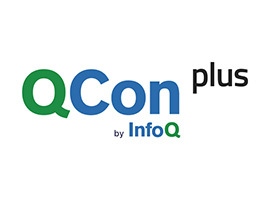
Transcript
Sameki: My name is Mehrnoosh Sameki. I’m a senior program manager and technical lead of the Responsible AI tooling team at Azure Machine Learning, Microsoft. I’m joining you to talk about operationalizing Responsible AI in practice. First, I would like to start by debunking the idea that responsible AI is an afterthought, or responsible AI is a nice-to-have. The reality is, we are all on a mission to make responsible AI the new AI. If you are putting an AI there, and it’s impacting people’s lives in a variety of different ways, you have the responsibility to ensure the world that you have created is not causing harm to humans. Responsible AI is a must-have.
Machine Learning in Real Life
The reason why it matters a lot is, besides the very fact that in a traditional machine learning lifecycle, you have data, then you pass it to a learning phase where that learning algorithm takes out patterns from your data. Then that creates a model entity for you. Then you use techniques like statistical cross-validation, accuracy, to validate and evaluate that model and improve it, leading to a model that then spits out information such as approval versus rejection for loan scenarios. Despite the fact that this lifecycle matters, and you want to make sure that you’re doing it as reliably as you could, there are lots of human personas in the loop that need to be informed in every single stage of this lifecycle. One persona, or many of you, ML professionals, or data scientists, they would like to know what is happening in their AI systems, because they would like to understand if their model is any good, whether they can improve their model, what features of their models should they use in order to make reliable decisions for humans? The other persona are business or product leaders. Those are people who would like to approve the model, should we put it out there? Is it going to put us on the first page of the news another day? They ask a lot of questions from data scientists regarding, is this model racist? Is this biased? Should I let it be deployed? Are these predictions matching some domain experts’ insights that I’ve got from surgeons, doctors, financial experts, insurance experts?
The other persona is end-users, or solution providers. By that I mean either the banking person who works at a bank and is providing people with that end result of approved versus rejected on their loan, or a doctor who is looking at the AI results and is providing some diagnosis or insights to the end user or patient, in this case. Those are people who deal with the end user. Or they might be the end user themselves. They might ask, why did the model say this about me, or about my patient or my client? Can I trust these predictions? Can I make some actionable movements based on that or not? One persona that I’m not showing here, but is overseeing the whole process, are the regulators. We all have heard about the recent European regulations and GDPR’s right to explanation, or California act. They’re all adding lots of great lenses to the whole equation. There are risk officers, regulators who want to make sure that your AI is following the regulations as it should.
Microsoft’s AI Principles
With all of these great personas in the loop, it is important to ensure that your AI is being developed and deployed responsibly. However, even if you are a systematic data scientist or machine learning developer and really care about this area, truth to be told is the path to deploying responsible and reliable machine learning is still unpaved. Often, I see people using lots of different fragmented tools, or a spaghetti of visualizations or visualization primitives together in order to evaluate their models responsibly. That’s our team mission to help you operationalize responsible AI in practice. Microsoft have these six principles in order to inform your AI development and deployment. Those are fairness, reliability and safety, privacy and security, inclusiveness, underpinned by two more foundational ones: transparency and accountability. Our team specifically works on the items that are shown in blue, which are fairness, reliability and safety, inclusiveness, and transparency. The reason why we work on them is because they have a theme. All of these are supposed to help you understand your model better, whether through the lens of fairness or through the lens of how it’s making its prediction, or through the lens of reliability and safety and its errors, or whether it’s inclusive to everyone. Hopefully, help you build trust, improve it, debug it further, and make actionable insights.
Azure Machine Learning – Responsible AI Tools
Let’s go through that ecosystem. In order to guide you through this set of tools, I would like to first start by a framing. Whenever you are having a machine learning lifecycle, or even just data, you would like to go through this cycle. First, you would like to take your model and identify all the issues, aka, fairness issues, errors, that are happening inside that. Without identification stage, you don’t know exactly what is going wrong. Next, another important step is to diagnose why that thing is going wrong. The diagnosis piece might look like that, now I understand that there are some issues or errors in my data. Now I diagnose that the imbalance in my data is causing it. The diagnosis stage is quite important, because that discovers the root cause of the issue. That’s how you can take more efficient, targeted mitigations in order to improve your model. Naturally, then you move to the mitigation stage where, thanks to your identification and diagnosis skills, now you can mitigate those issues that are happening. One last step that I would like to highlight is take action, sometimes you would like to inform a customer or a patient or a financial loan applicant about, for instance, what can they do, so next time they get a better outcome. Or, you want to inform your business stakeholders as what can you give some of the clients in order to boost sales. Sometimes you want to take real-world actions, some of them are model driven, some of them are data driven.
Identification Phase
Let’s start with identify and the set of open source tools and Azure ML integrated tools that we provide for you to identify your model issues. Those two tools are error analysis and fairness tools. First, starting with error analysis, the whole motivation behind us putting this tool out there is the fact that we see people often use one metric to talk about their model’s goodness, like they say, my model is 73% accurate. While that is a great proxy into identifying the model goodness and model health, it often hides this important information, that error is not uniformly distributed in your data. There might be the case that there are some erroneous packets of data, like this packet of data that is only 42% accurate. Versus, in contrast, this packet of data is getting all of the right predictions. If you go with one number, you’re losing this very important information that my model has some erroneous packets, and I need to investigate why that cohort is getting more errors. We released a toolkit called error analysis, which is helping you to validate different cohorts, understand and observe how the error has been distributed across your dataset, and basically see a heat map of your errors as well.
Next, we worked on another tool called Fairlearn, which is also open source, it is to help you understand your model fairness issues. It is focusing on two different types of harms that AI often give rise to. One is harm of quality of service, where AI is providing different quality of service to different groups of people. The other one is harm of allocation where AI is allocating information opportunities or resources differently across different groups of people. An example for harm of quality of service is a voice detection system that might not work as well for say females versus males or non-binary people. An example of harm of allocation is a loan allocation AI or a job screening AI that might be better at picking candidates among white men compared to other groups. The whole hope behind our tool is to ensure that you are looking at the fairness metrics with the lens of group fairness, so how different groups of people are getting this treatment. We provide a variety of different fairness and performance metrics and rich visualizations, in order for you to observe the fairness issues as they occur in your model.
Both of these support a variety of different model formats, Python model using scikit predict convention, Scikit, or TensorFlow, PyTorch, Keras models. They also support both classification and regression. An example of a company putting our fairness tool into production is Philips Healthcare. They put fairness in production into their ICU models. They wanted to make sure that their ICU models that they have out there is performing uniformly across different patients with different ethnicities, gender identities. Another example is Ernst & Young in a financial scenario where they use this tool in order to understand how their loan allocation AI is providing this opportunity of getting a loan across different genders and different ethnicities. They were able to also use our mitigation techniques.
Diagnosis Phase
After the identification phase, now you know where the errors are occurring, and you know your fairness issues. You move on to the diagnosis piece. I cover two of the most important diagnosis capabilities, interpretability and perturbations and counterfactuals. One more to just like momentarily touch on is, we’re also in the process of releasing a data exploration and data mitigation library. The diagnosis piece right now entails the more basic data explorer. I will show that to you in a demo. It also includes interpretability, that’s the module we provide to you, which basically tells you what are the top key important factors impacting your model predictions. How your model is making its predictions. It covers both global explanation and local explanation. How overall the model is making its prediction, and how individual data points for them, how the model has made its predictions.
We do have different packages under Interpret ML capabilities that we have. It’s a collection of black box interpretability techniques that can literally cover any model that you bring to us, no matter if it’s Python, or Scikit, or TensorFlow, PyTorch, Keras. We also have a collection of glassbox models that are intrinsically interpretable models, if you have the flexibility of basically changing your model and training an interpretable model from scratch. An example of that is Scandinavian Airlines. They basically used our interpretability capabilities via Azure Machine Learning to build trust with their fraud detection model of their loyalty program. Of course, you can imagine that in such cases, you want to reduce and minimize and remove mistakes, because you don’t want to tell a very loyal customer that they’ve done some fraudulent activity, or flag their activity by mistake. That is a very bad customer experience. They wanted to understand how their fraud detection model is making their predictions, and so they used interpretability capabilities to understand that.
Another important diagnosis piece is counterfactual and perturbations. You can do lots of freeform perturbations, do what-if analysis, change features of a data point, and see how the model predictions change for that. Also, you can look at counterfactuals and that is simply telling you what is the bare minimum changes to a data point’s feature values that could lead into a different prediction. Say, Mehrnoosh’s loan is getting rejected, what is the bare minimum change that I can apply to her features so that the AI predicts approved next time?
Mitigate, and Take Action Phase
Finally, we go to the mitigation stage, and also take action stage. We do cover a class of unfairness mitigation algorithms that could literally encompass any model. They have different flexibilities. Some of them are just post-processing methods and could adjust your model predictions in order to improve it. Some of them are more like reductions method, combination of pre-processing and in-processing. They can update your model objective function in order to retrain your model and not just minimize error, but also put control on a fairness criteria that you specify. We also do have pre-processing methods that will readjust your data in terms of better balancing it and better representing the underrepresented groups. Then, hopefully, the model that is trained on that augmented data is going to be a fairer model. Last, we realized that a lot of people are using our model, Responsible AI insights, for decision making in the real world. We all know models sometimes take on correlations rather than causation. We wanted to provide you with a tool that works on your data, just historic data, and uses a technique called double machine learning in order to understand whether there are any causal effects of a certain feature on the real-world phenomenon. Say, if I provide promotion to a customer, would that really increase the sales that that customer will generate for me? Causal inference is another capability we just released.
Looking forward, one thing that I want to mention is, while I went through different parts of this identify, diagnose, mitigate, we have brought every single tool I just represented under one roof, and that is called Responsible AI dashboard. The Responsible AI dashboard is a single pane of glass, bringing together a variety of these tools under one roof, same set of API, a customizable dashboard. You can do both model debugging and also responsible decision making with that, depending on how you’re customizing and what you pass to it. Our next steps would be to expand the portfolio of Responsible AI tools to non-tabular data, enable Responsible AI reports for non-technical stakeholders. We do have some exciting work on PDF reports you can share with your regulators, risk officers, business stakeholders. We are working on enabling model monitoring at scoring time just to bring all these capabilities beyond evaluation time and bring it to scoring time, and make sure that as the model is seeing the unseen data, it can still detect some of these fairness issues, reliability issues, interpretability issues. We’re also working on a compliance infrastructure, because we all know that there are nowadays so many stakeholders involved in development, deployment, and testing and approval of an AI system. We want to provide the whole ecosystem to you.
Demo
We believe in the potential of AI for improving and transforming our lives. We also know there is a need for tools to assist data scientists, developers, and decision makers to understand and improve their models to ensure AI is benefiting all of us. That’s why we have created a variety of tools to help operationalize Responsible AI in practice. Data scientists tend to use these tools together in order to holistically evaluate their models. We are now introducing the Responsible AI dashboard, which is a single pane of glass, bringing together a number of Responsible AI tools. With this dashboard, you can identify model errors, diagnose why those errors are happening, and mitigate them. Then, provide actionable insights to your stakeholders and customers. Let’s see this in action.
First, I have here a machine learning model that can predict whether a house will sell for more than median price or not, and provide the seller with some advice on how best to price it. Of course, I would like to avoid underestimating the actual price as an inaccurate price could impact seller profits and the ability to access finance from a bank. I turned into the Responsible AI dashboard to look closely at this model. Here is the dashboard. I can do, first, error analysis to find issues in my model. You can see it has automatically separated the cohorts with error counts. I found out that bigger old houses have a much higher error rate of 25% almost in comparison with large new houses that have error rates of only 6%. This is an issue. Let’s investigate that further. First, let me save these two cohorts. I save them as new and old houses, and I go to the model statistics for further exploration. I can take a look at the accuracy, false positive rates, false negative rate across these two different cohorts. I can also observe the prediction probability distribution and observe that older houses have higher probability of getting predictions less than median. I can further go to the Data Explorer and explore the ground truth values behind those cohorts. Let me set that to look at the ground truth values. First, I will start from my new houses cohort. As you can see here, most of the newer homes sell for higher price than median. It’s easy for the model to predict that and get a higher accuracy for that. If I switch to the older houses, as you can see, I don’t have enough data representing expensive old houses. One possible action for me is to collect more of this data and retrain the model.
Let’s now look at the model explanations and understand how the model has made its predictions. I can see that the overall finish quality, above ground living room area, and total basement square footage are the top three important factors that impact my model’s prediction. I can further click on any of these like overall finish quality, and understand that a lower finish quality impacts the price prediction negatively. This is a great sanity check that the model is doing the right thing. I can further go to the individual feature importance, click on one or a handful of data points and see how the model has made predictions for them. Further, when I come to the what-if counterfactual, what I am seeing here is for any of these houses, I can understand what is the minimum change I can apply to, for instance, this particular house? Which has actually a high probability of getting the prediction of less than median, so that the model predicts the opposite outcome. Looking at the counterfactuals for this one, only if the house had a higher overall quality from 6 to 10, then the model would predict that this house would sell for more than median. To conclude, I learned that my model is making predictions based on the factors that made sense to me as an expert, and I need to augment my data on the expensive old house category, and even potentially bring in more descriptive features that help the model learn about an expensive old house.
Now that we understood the model better, let’s provide house owners with insights as to what to improve in these houses to get a better price ask in the market. We only need some historic data of the housing market to do so. Now I go to the causal inference capabilities of this dashboard to achieve that. There are two different functionalities that could be quite helpful here. First, the aggregate causal effect which shows how changing a particular factor like garages, or fireplaces, or overall condition would impact the overall house price in this dataset on average. I can further go to the treatment policy to see the best future intervention, say switching it to screen porch. For instance, here I can see for some houses, if I want to invest in transforming a screen porch, for some houses, I need to shrink it or remove it. For some houses, it’s recommending me to expand on it. Finally, there’s also an individual causal effect capability that tells me how this works for a particular data point. This is a certain house. First, I can see how each factor would impact the actual price of the house in the market. I can even do causal what-if analysis, which is something like if I change the overall condition to a higher value, what boost I’m going to see in the housing price of this in the market.
Summary
We looked at how these tools help you identify and diagnose error in a house price prediction model and make effective data-driven decisions. Imagine if this was a model that predicted the cost of healthcare procedures or a model to detect potential money laundering behavior, identifying, diagnosing, or making effective data-driven decisions would have even higher consequences on people’s lives there. Learn more about the tool on aka.ms/responsibleaidashboard, and try it on Azure Machine Learning to boost trust in your AI driven solutions.
Questions and Answers
Breviu: Ethics in AI is something I’m very passionate about. There’s so much harm that can be done if it’s not thought about. I think that showing the different tools and the different kinds of thought processes that you have to go through in order to make sure that you’re making models that are going to not only predict well for accuracy, but also that they’re not going to cause harm.
Sameki: That is absolutely true. I feel like the technology is not going to slow down. We’re just starting with AI and we’re expanding on its capabilities and including it in more aspects of our lives, from financial scenarios, to healthcare scenarios, to even retail, our shopping experience and everything. It’s even more important to have technology that is accompanying that fast growth of AI and is taking care of all those harms in terms of understanding them, providing solutions or mitigations to them. I’m quite excited to build on these tools and help different companies operationalize this super complicated buzzword in practice, really.
Breviu: That’s true. In so many companies, they might want to do it, but they don’t really know how. I think it’s cool that you showed some of the different tools that are out there. There was that short link that you provided that was to go look at some of the different tools. You also mentioned some new tooling that is coming out, some data tooling.
Sameki: There are a couple of capabilities. One is, we completely realized that the model story is incomplete without the right data tools, or data story. Data is always a huge part, probably the most important part of a machine learning lifecycle. We are also accompanying this with more sophisticated data exploration and data mitigation library, which is going to land under the same Responsible AI toolbox. That will help you understand your data balances, and that also provides lots of APIs that can rebalance and resample parts of your data that are underrepresented. Besides this, at Microsoft Build, we’re going to release a variety of different capabilities of this dashboard integrated inside our Azure Machine Learning. If your team is on Azure Machine Learning, you will get easy access, not just to this Responsible AI dashboard and its platform, but also a scorecard, which is a report PDF, summarizing the insights of this dashboard for non-technical stakeholders. It was quite important for us to also work on that scorecard because there are tons of stakeholders involved in an end-to-end ML lifecycle. Many of those are not super data science savvy or super technical. There might be surgeons. There might be financial experts. There might be business managers. It was quite important for us to also create that scorecard to bridge the gap between super technical stakeholders and non-technical stakeholders in an ML lifecycle.
Breviu: That’s a really good point. You have the people that understand the data and how to build the model, but they might not understand the business application side of it. You have all these different people that need to be able to communicate and understand how their model is being understood. It’s cool that these tools can do that.
You talked about imbalanced data as well. What are some of the main contributing factors to ethical issues within models?
Sameki: Definitely, imbalanced data is one of them. That could mean many different things. You are completely underrepresenting a certain group in your data, or you are representing that group, but that group in the training data is associated with unfavorable outcomes. For instance, you have a certain ethnicity in your loan allocation AI dataset, however, all of the data points that you have from that ethnicity happen to have rejection on their loans. The model creates that association between that rejection and belonging to that ethnicity. Either not representing a certain group at all, or representing them but not checking whether they are represented well in terms of the outcome that is affiliated with them.
There are some other interesting things as well, after the data, which is probably the most important issue. Then there is the issue of problem definition. Sometimes you’re rushing to train a machine learning model on a problem, and so you’re using the wrong proxies as a predictor for something else. To give you a tangible example, to make it understandable, imagine you do have a particular model that you’re training in order to assign different risk scores to neighborhoods, like security scores. Then you realize that, how is that model trained? That model is trained on a data that is coming from arrest records of the police, imagine. Just using arrest records as a proxy into the security score of a neighborhood is a very wrong assumption to make because we all know that policing practices at least in the U.S. is quite unfair. It might be the case that there are more police officers deployed to certain areas that have certain ethnicities, and way less police officers to some other areas where there are some other ethnicities residing. Just because there are more police officers there, there might be more reporting of certain even like misdemeanors, or something that that police officer didn’t like, or whatever. That will bump up the number of arrest records. Using that purely for proxying to the safety score of that neighborhood, has that dangerous outcome of affiliation between the certain race residing in that neighborhood and the security of that neighborhood.
Breviu: When those kinds of questions come up, I think about, are we building a model that even should be built? Because there’s two kinds of questions when it comes to ethics in AI. It’s, is my model ethical? Then there’s the opposite, is it ethical to build my model? When you’re talking about arrest records, and that kind of thing, and using that, I start worrying about, what is that model going to actually do? What are they going to use that model for? Is there even a fair way to build the model on that type of data?
Sameki: I absolutely agree. A while ago, there was this project from Stanford, it was called Gaydar. It was a project, which was training machine learning models on top of bunch of photos that they had recorded and captured from the internet and from different public datasets. The outcome was to predict whether the person is belonging to the LGBTQ community or not, or gay or not. At that time, when I saw that I was like, who is supposed to use this and for what reason? I think that started getting a lot of attention in the media that, we know that maybe AI could do things like that, questionable, but maybe. What is the point of this model? Who is going to use it? How are we going to guarantee that this model is not going to be used to basically perpetuate biases, stuff like that, against the LGBTQ community that are historically marginalized? There are tons of deep questions that we have to ask that whether machine learning is an appropriate thing to do for a problem, and what type of consequences it could have. If we do have a legit case for AI, could be helpful to make processes more efficient, could be more helpful to expedite certain super lengthy processes. Then we have to accompany it with enough checks and balances, scorecards, and also terms and services as how people use that model. Make sure that we do have a means of hearing other people’s feedback in case they observe this model being misused in bad scenarios.
Breviu: That’s a really good example of one that just shouldn’t have happened. It always tends to be the marginalized, or the oppressed society, or parts of society that are hurt the most, and oftentimes aren’t necessarily the ones that are even involved in building it as well, which is one of the reasons why having a diverse set of engineering for these types of models. Because I guarantee you, if you had somebody that was part of that community building that model, they probably would have said, this is really offensive.
Sameki: They would catch it. I always knew about the focus of the companies on the concept of diversity and inclusion before I joined this Responsible AI effort, but now I understand it from a different point of view that, it matters, that we have representation from people who are impacted by that AI in the room to be able to catch these harms. This is an area where growth mindset is the most important. I am quite sure that even if we are systematic engineers that truly care about this area and put all of these checks and balances, stuff happens still. Because this is a very sociotechnical area where we cannot fully claim that we are debiasing a model. This is a concept that has been studied by philosophers and social scientists for centuries. We can’t come up suddenly out of the tech world and say, we’ve found a solution for it. I think progress could be made to figure out these harms, catching it early on, diagnosing why those happen. Mitigating them based on your knowledge, and documenting what you could not resolve and put some diverse groups of people in the decision making to catch some of those mistakes. Then, have a very beautiful feedback loop where you capture some thoughts from the audience and you are able to act fast and also very solid monitoring lifecycle.
Breviu: That’s actually a good point, because it’s not only just the ideation of it, should I do this? Ok, I should. Now I’m building it, now, make sure that it’s ethical. Then there’s the data drift and models getting stale and needing to monitor what’s happening in [inaudible 00:35:32], so make sure that it continues to be able to predict well, and do that.
Any of these AI tools that you’ve been showing, are they able to be used in a monitoring format as well?
Sameki: Yes. Most of these tools could be, for instance, the interpretability. We do have support of scoring time interpretability, which basically allows you to call the deployed model, get the model predictions, and then call the deployed explainer and get the model explanations for that prediction at runtime. The fairness error analysis pieces are a little trickier. Fairness, basically, you can also specify the favorable outcome, and you can keep monitoring that favorable outcome distribution across different ethnicities, different genders, different sensitive groups, whatever that means to you. For the rest of fairness metrics, or error analysis, and things like that, you might require, periodically upload some labeled data based on your new data, take a piece, maybe use crowdsourcing or human labelers to label that and then parse it. General answer is yes. There are some caveats. We’re also working on a very strong monitoring story that goes around these caveats and helps you monitor that during runtime.
Breviu: Another example, I think of ones where I’ve seen that make me uncomfortable, and this happens, like machine learning models as part of the interview process. It’s one that actually happens a lot. There’s already so many microaggressions and unconscious biases, that using a model like this in the interview process, and I’ve read so many stories about it as well, where having, just even on resumes, how quickly it actually is biased. How do you feel about that particular type of use case? Do you think these tools can work on that type of problem? Do you think we could solve it enough to where it would be ethical to use it in the interviewing process?
Sameki: I have seen both with some external companies, they’re using AI in candidate screening, and they have been interested in using the Responsible AI tools. LinkedIn is now also part of Microsoft family. I know LinkedIn is also very careful about how these models are trained, tested. I actually think these models could be great initial proxies to figure out some better candidates. However, it’s quite important that if you want to trust the top ranked candidates, it’s super important to understand how the model has picked that, and so look at the model explainability, because often, there has been this case of associations.
There are two examples that I can give you. I remember that once there was this public case study from LinkedIn, they had trained a model for job recommendations, how you go to LinkedIn and it says, apply for this and this. Then they realized early on that one of the ways that LinkedIn algorithm was using the profiles in order to match them with the job opportunities was the fact that the person was providing enough description about what they are doing, what are they passionate about? How you have a bio section and then you have your current position, which you can add text to. Then there was a follow-up study by LinkedIn which was mentioning that women tend to have less details shared there, so in a way women tend to market themselves in a less savvy way compared to men. That’s why men were getting better quality recommendations and a lot more matches compared to women or females, non-male, basically: females, non-binary. That was a very great wake-up call for LinkedIn, that, ok, this algorithm is doing this matching, we have to change it in order to not put too much emphasis. It’s great that they have this extra commentary and whatever. First of all, we have to maybe provide some recommendations to people who have not filled those sections as your profile is this much complete, how they give you signals as go and add more context.
Also, we have to revisit our algorithms to really look at the bare minimum stuff, like the latest position posted, experiences. Even then, women go on maternity leave and family care leaves all the time. I still feel like when we have these companies receiving so many candidates and resumes, there is some role that AI could play to bring some candidates up. However, before deploying it in production, we have to look at the examples. We have to also have a little team of diverse stakeholders in the loop to get those predictions and try to take a look at that from the point of view of diversity and inclusion, from the point of view of explainability of the AI, and interfere with some human rules in order to make sure it’s not unfair to some underrepresented candidates.
Breviu: That talks to the interesting thing, I think that you said, one of the beginning things is how the errors are not evenly distributed throughout the data. This is an example where, your model might get a really great accuracy but it was looking at the holistic approach, and realizing that on the non-male, female non-binary side that it was at a very high error rate. That’s like a really good example of that point that you made in the beginning, which I found really interesting. Because many times when we’re building these models, we’re looking at our overall accuracy rate and our validation and loss score. Those are looking at it as a holistic thing, not necessarily on an individual basis.
Sameki: It’s very interesting, because many people use platforms like Kaggle to learn about applied machine learning. Even in those platforms, we often see scoreboards where one factor is used to pick the winner, like accuracy of the model, area under curve, whatever that might be. That implicitly gives out that impression that, ok, there are a couple of proxies, if it’s good, great, go ahead and deploy. I think that’s the mindset that we would love to change in the market through this type of presentations that it’s great to look at your model goodness, accuracy, false positive rate, all those metrics that we’re familiar with for different types of problems. However, they’re not sufficient to tell you about the nuances of how that model is truly impacting the underrepresented groups. Or any blind spots, they’re not going to give you the blind spots. It’s not even always about fairness. Imagine, you realize that your model is 89% accurate or 95% accurate, but you realize that those 5% errors happen to happen for every single time we have this autonomous car AI, and the weather is foggy and dark and is rainy, and the pedestrian is a darker skin tone wearing dark clothes. Ninety-nine percent of the time the pedestrian is missed. That’s a huge safety and reliability issue that your model has. That’s a huge blind spot that is potentially killing people. If you go with one score about the goodness of the model, you’re missing that important information that your model has these blind spots.
Breviu: I think your point about Kaggle too in the ethics thing, kind of just shows where this ethics was an afterthought in a lot of this, and that’s why these popular platforms don’t really necessarily have those tools built in, as Azure Machine Learning does. I think also as we progress and people realize more just about like data privacy as well, I think as data scientists, we’ve always understood the importance of data privacy. I think now it’s becoming more mainstream. I think that part, and then understanding ethics more, I think it really will change how and the way that people build models and think about building models. I think AI is going to keep moving forward exponentially, in my opinion. It needs to move forward in an ethical, fully thought out way.
Sameki: We build all of these tools in the open source first, to help everyone explore these tools, augment it with us, build on it, and bring their own capabilities and components, and put it inside that Responsible AI dashboard. If you’re interested, check out our open source offering and send us a GitHub issue, send us your request. We are quite active on GitHub, and we’d love to hear your thoughts.
See more presentations with transcripts
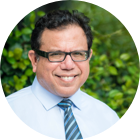
MMS • Rebecca Parsons Rafiq Gemmail Craig Smith Shaaron A Alvares
Article originally posted on InfoQ. Visit InfoQ
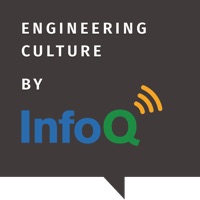
Subscribe on:
Transcript
Shane Hastie: Good day folks. This is the whole InfoQ culture team and special guest for recording our podcast for the Trend Report for 2023. I’m Shane Hastie, I’m the lead editor for Culture & Methods on InfoQ. I’m going to shepherd our conversation today, but we’ll start and I’ll go around our virtual room and ask people to introduce themselves. We’ll start with our special guest, Rebecca Parsons.
Rebecca, thank you so much for joining us. Would you mind just telling us very briefly who’s Rebecca?
Introductions [00:36]
Rebecca Parsons: Well. Thank you Shane for having me. As you said, my name is Rebecca Parsons. I’m the chief technology officer for Thoughtworks and we’re a software consulting company that we basically write software for other people.
Shane Hastie: Welcome.
Rebecca Parsons: Thank you.
Shane Hastie: Craig Smith.
Craig Smith: I’m the business agility practice lead for SoftEd, and I work with you as well Shane. Glad to be a part of the team.
Shane Hastie: And Raf Gemmail.
Raf Gemmail: Hi. Yes, I’m Raf. I am an engineering manager with Marigold in the MarTech space and many years technical coaching, Agile teams.
Shane Hastie: Thanks Raf. Last but certainly not least, Shaaron.
Shaaron A Alvares: Thank you Shane for having me. Very excited to be here and to be with all of you. Shaaron Alveres, I’m located in Seattle, Washington and I am director of GT Agile Transformation and Delivery at Salesforce. I’m working closely with executives to help them get better. I’m supporting of them and their teams in operation and engineering at GT.
Shane Hastie: Welcome folks. This is our motley crew of editors, reporters, and contributors for the InfoQ Culture and Method Space. It’s wonderful to get us all together in one virtual space for a change. It doesn’t happen often, but what’s happening with the Culture and Methods trends for 2023? We were having a bit of a chat earlier and I think the big elephant in the room is the economy and the layoffs and everything that we’ve seen in that space.
What do people think? Shaaron, can we start with you?
The economy and layoffs in tech companies [02:11]
Shaaron A Alvares: Yeah, I’m happy to kick us off. You’re right, Shane, with this new recession, there’s a lot of changes happening in organization, priorities are shifting. Executives are more concerned at the moment about financial results, which led to a number of layoffs globally.
We’ve seen a lot of layoffs globally, in the US as well. Then that led to prioritizing high performance team, productivity, and efficiency. A huge impact I want to say on the culture aspect of organizations, on delivery as well, but a huge impact on culture and maybe a lost understanding how psychological safety is important for organization and teams.
Shane Hastie: Well, we’ve just come through two years where we’ve been focused on wellness and suddenly we’ve shifted. What’s happened?
Rebecca, what have you seen?
Rebecca Parsons: Well, I think when the boom was happening, the tech talent was very clearly in the driver’s seat. You add that to the pressures coming from the war in Ukraine, the global pandemic, increased visibility of these extreme weather events, the earthquake. All of these things have been contributing to a tremendous amount of stress. I think the response from many of the employers, both of the technology companies themselves, but also the enterprises who are trying to build up their own technology competency is they knew they had to fight to get the talent that they wanted.
Thinking about people-care was an opportunity to appeal to the needs of some of the people that they were trying to hire. I think as the macro economic pressures have changed, and we’ve heard this from some of the enterprises that we talk to that are not digital natives or not the technology companies, I don’t have to fight with Facebook anymore for talent, because Facebook’s just laid off all of these people or insert name of technology company here.
I think one of the interesting things that is particularly relevant from a culture and methods perspective is, okay, yes, you’ve got these people who are on the market, but are you going to actually be able to hire them as an enterprise? Even if you can hire them, are you going to be able to retain them when the market does turn around?
I’m reminded of a talk that Adrian Cockcroft gave several years ago where somebody from a more traditional enterprise said, “Well, yes, you can do all these things because you’ve got these wonderful people. And it’s like, but I hired those people from you. I just gave them an environment within which they could be productive.”
I do think we are going to see much more of a focus on how do we actually create an environment where we can get efficiencies and effectiveness, but we don’t impact time to market, because I don’t think we’re going to go back to that era around the turn of the century, where so many places still considered IT as a cost center and that meant you had to stabilize and you had to standardize and you had to lock things down. Consumers are not going to accept that, even in this downturn situation.
Shane Hastie: Thank you. Craig, what are your thoughts on this?
Craig Smith: Firstly, to anyone’s whose listening who’s been through that, I think we share their pain and things that they’re going through, but I think what I’m seeing from the outside is this is a little bit for a lot of organizations actually are a right-sizing that they needed to do that actually there’s a lot of waste that we see in organizations. Whilst all those people were effectively working on things, the size of the organization probably couldn’t sustain that.
What worries me though is that in this, were the leaders responsible for doing this, did they actually take the people from the right places? In other words, rather than just chopping off a whole side of a place here and moving it along, what I’m seeing in some of the organizations that I’ve been working with is that they’ll just say, “Look, we’re just going to get rid of these six teams over here,” but they weren’t the right places to get rid of.
The actual waste was in the actual mechanics of the organization. I think there’s also going to be a realization of that moving through, but you can argue for a lot of those tech companies, particularly in Silicon Valley and the like, maybe they were just too big for what they needed. I think the way we’re seeing some of these things play out in the media, whilst the way … as Ben was talking about … the way that’s played out and the real culture of the company may start to shine through, it’s also going to be interesting to see how this actually plays out in the end.
Do some of those organizations and now there’s a much more radicalized size they have, was that actually what they should have been in the first place? Was there just too many people doing too many things for the organization anyway?
Shane Hastie: Raf, your thoughts.
Raf Gemmail: Listening to them about the culture coming out and the discrepancy in styles of letting people go. One thing I’ve been reflecting on is I remember back to mid 2000s, I was in the environment of US banks and there would be culls every now and then, where people would just pop, pop, pop away from their desks. We went through a transformation after that I saw, where there was a lot more humanism in the workplace. Looking at what’s happened externally and to the people I know, the change management in letting people go in a more respectful fashion for some reason seems to be disappearing.
Yes, there may be an element of right sizing in all of this, but I completely agree with Craig, because when you are doing things with such haste, and maybe I’m projecting, inferring here, but when you’re doing things at such haste, the knock on impact, which I have seen, is that you are left with teams which may not reflect the capabilities and the topologies and communication channels you’d intentionally try to create in the first place. You will have a consequence of that.
I’m looking at it from the perspective of we’ve been through one big shift with COVID and maybe it took us in a particular direction, which I think was potentially positive. Now there’s this fear which is dollar driven, this financial fear, realizable fear. Maybe to some extent that is compromising a humanism as organizations, which have been trying to become more humanistic generally. That disturbs me a little bit.
Shane Hastie: Rebecca, can I put you on the spot? In your role at Thoughtworks, I’m assuming that you’ve had these conversations internally, have we got the right people and so forth? How do you keep that humanistic perspective at the fore?
Keeping workplaces humanistic when faced with pressure [08:29]
Rebecca Parsons: Well, I definitely agree it is one of those fundamental cultural aspects of companies. From the beginning, we have looked at our people as the most important asset that we have. If you truly believe that, and a lot of companies say it, but if you truly believe that, then you know that you have to look at things like capability and capacity and policies from that more humanistic perspective. I often get into debates with people when they’re looking at diversity programs and they want to go immediately to the business case.
Yes, there’s a very strong business case for this. There is a strong business case for having a strong employee value proposition, because turnover is expensive and replacing people is expensive. If you treat people well, you can make all of these wonderful business case arguments, but you can also look at it as it’s just the right thing to do. If you truly believe that your people are your most valuable asset, what do you do with valuable assets?
You invest in maintaining them and nurturing them and helping them grow. You have to look at it from that humanistic perspective. Even now, particularly given the options that are available to technologists, treating somebody poorly in a layoff. It sounds like that is something that is happening in many of the organizations right now, based on the froth that you hear in the social media realm. You’re just dooming yourself, in terms of your ability to hire, because there’s only so much you can get back in terms of that credibility when push comes to shove, okay, we have to do a layoff. Then you’ve got all of these people going off on Twitter and LinkedIn about just how wrong and how cruel, needlessly cruel the process was.
Shane Hastie: An interesting article. It was a Wall Street Journal article I saw I think was talking about yes, there have been these massive layoffs, but also most of these people who are laid off are being re-employed very quickly. We’re not seeing a lack of employment and a lack of demand. Is it just perhaps the people moving around. As Craig said, somewhat a bit of right-sizing? How do people feel about that?
Downsizing or rightsizing? [10:49]
Raf Gemmail: I think we’re at the start of the curve. We’ve seen a big spike, lots of people in the market, but what I’m seeing is that trickling to other places. We’re in New Zealand and I’ve recently heard of a local company in New Zealand, which is letting go of many people. It had grown rapidly to hundreds of people, same sort of deal, and people are being let go of.
These things often start somewhere and they spread. While they’re starting to spread, they may be still later demand in the market. I’m hiring right now, but we are on that curve and so we’ll see where we go. I think I’m confident that if a company manages and looks after its people well that you can create a good culture, but in the industry at large there are always waves. I think we’re starting on that wave.
Shaaron Alveres: About this article. I didn’t read it but I’m going to read it, so thank you for the reference,Shane, but what I think there are jobs, it’s true that there’s been an increase in job posting. I read that, but I think it’s in small and medium size companies and mostly retail and service type of companies. I don’t think it’s in technology, software.
What I want to say is during the pandemic, those technology companies have hired massively, because we had to move to online, to the cloud, and there’s been massive hires. Companies like Amazon, my company, Microsoft, they hired people they probably hired in two years close to between I think between 50,000 to a 100,000 people. Sometimes, in those large companies. Now, what had happened is there wasn’t always a good onboarding process for those engineers. We focused on hiring and we expected them to deliver right away, but there wasn’t a great onboarding plan, especially when we hire so many people and we set up brand new teams. I think that has created maybe a perceived lack of productivity and efficiencies.
Other companies, what I want to add is some companies chose to lay off massively, but very few executive chose to actually decrease their bonus or their compensation and not lay off those employees. They had a choice as well. We saw a lot of articles around executive who chose to not lay off their employees, because they had onboarding them. They already have acquired a lot of knowledge. That’s good to see.
I’m trying to look at the silver lining around those layoffs. One thing that happened recently is I think companies organization, as they lay out their FY 24 plan, objectives, they started focusing a lot more on priorities. There’s a ruthless prioritization that is happening actually within organizations to make sure that we focus on the right thing and all of our resources are really focusing on maybe doing less but focusing on the right thing. That’s a silver lining. That’s good.
Raf Gemmail: There’s a counterpoint to focusing on the right thing. I came across a term recently … I’m not prolific like the others, but … the last piece I wrote was something a lady called Sandy Mamoli who was with us last year and she was quoting a guy from New Zealand who used the word “quarter fall”. This idea of upfront planning, sometimes if those priorities are guiding where you go too stringently, that ability to discover, to create, to pivot may be compromised somewhat. I’m not always a fan of the ruthless prioritization sometimes, because I think innovation comes in the gaps in between.
Shaaron A Alvares: It has to be both prioritizing … and when I talk about annual planning, I think most organization, I hope so, they’re doing both. We set up goals for the year, but we review those goals on a quarterly basis. But I agree with you Raf, there’s also pitfalls with less prioritization.
Shane Hastie: hink that gives us a nice segue to what could be our next topic. What is happening? What are the innovations that our listeners, our readers should be preparing for addressing?
One of the big ones I want to throw up there is ChatGPT. We can’t have a conversation without somebody bringing in, “I type this into ChatGPT and here’s what it says…” Just taking that as one example, what’s happening?
The impact of ChatGPT and AI [14:57]
Craig Smith: Well, obviously the world of AI was always going to catch up with us in some way. I’m sure we’ve all read the articles about how ChatGPT is going to take away our roles and how, I think the one I just read the other day was that Agile coaches and scrum masters would be no longer needed, because you could just ask ChatGPT your questions. But I think going past just one particular tool, we’re on the very start of the hockey stick in relation to AI and machine learning and all those types of things.
We’ve been collecting all this data, we talk about it, but now actually starting to turn it into tools like what we’re starting to see, not only with that but also with image processing and all sorts of other things. How those tools can be used for useful purposes as opposed to, I guess some of the negative reporting we get now, which is how they use for evil, but also then trying to bring in things like copyright.
We read articles about how schools are banning tools like that, which worries me greatly because it’s like when I went to school … showing my age … you couldn’t use a calculator, you had to add up in your head. I can’t remember how many times I’ve tried to do functions and equations in my head without a calculator or something in real life. Embrace the tools that help us do that, but don’t copy the answers. Teach people about using these tools effectively.
It’s going to be a real challenge across all of our industries about how we embrace these tools and use them to move forward as opposed to trying to block them or push them away.
Raf Gemmail: There’s a security and privacy aspect to that as well, Craig, around what you’re putting into these third party tools. I’ve been using it, experimenting is probably the word to use at this phase, but I had to give some feedback to someone who was interviewed for some other documentation. I’ve used the text side of it, which it’s amazing at, but I’m very conscious with these things not to put real names in, not to disclose more than you would want to put into a Google search.
I was in a conversation yesterday which covered this sort of thing, that you don’t want to put too much IP into these things. You want to have some guidelines. I think we’re in that wild west where we’re still trying to figure out how to use it, but there’s definitely an element of how much do you put into those systems? How much do you edit it? I edit it after the event. I’m like, okay, this is what it said, now fit it to my context, because A, I don’t talk like this. And B, I want this to be more personal and reflect the person I’m talking to, and what I know about them.
Craig Smith: While we were doing this, I just typed to the ChatGPT, “What are some of the innovations in culture and methods?” The answer was “agile culture, Lean Startup, design thinking, DevOps, holacracy and agile HR.”
There you go. Conversation done.
Shane Hastie: Interestingly, that many of those are the trends that we have on our graph.
Rebecca, what are you seeing with ChatGPT and AI?
Rebecca Parsons: Well, we’re doing a fair amount of experimentation both with the text capabilities of ChatGPT as well as the code-generation of both co-pilot and ChatGPT. The big concern and the big questions that we get and are uncovering ChatGPT in particular, is very confident of every answer it gives, whether it’s utter nonsense or not. Sometimes, it really is absolutely spot on. Okay, yeah, be confident, you’re right. Then it will confidently assert that the name of a country that is four letters that starts and ends with the same letter is “Chad”.
Again, very confidently asserting, but it’s utter nonsense. It does not know what it does not know. It knows areas it’s not supposed to talk about, because it’s got the guardrails in there, but it doesn’t know what it doesn’t know. That means from a code generation perspective, very often the mistakes are subtle. Occasionally, it will be blindingly obvious because it’s generating some java code that won’t compile or whatever, but very often it’s things, off by one errors or something subtle like that, where if you don’t actually know the result that you want, you won’t actually know that the result it gave you is wrong.
Now, even with the level of quality that it has right now within a specific context, it can generate some really impressive code. One of our people in China put it through an exercise in typescript to basically generate a CRUD application, connecting to a Postgress database and oh, what do I have to do now? Now, how do I get this thing containerized? Took it through the step. One of the really powerful things about even the current instance is the ability for the model to get context, so that you’re not always starting from scratch.
That means you can say, “Oh well that was close, but use this library instead,” and it will keep the context of what it had before and take your new prompt into account. We’re seeing people who are claiming though 60% increase in developer productivity. Well, I can imagine if you’re doing something for which a canned algorithm exists out there on the net, that yeah you could probably generate it pretty easily, but to make a general claim about more broad software development activities, you have to take into account how much time do you actually spend typing versus thinking versus executing?
It’s only going to be able basically to help you with the typing. Maybe if you’re trying to learn a new language, an experienced programmer, we’ve got somebody who’s actually trying to do that, trying to learn a new language by using ChatGPT. But again, it works best if you know the answer that you want.
Shane Hastie: What’s happening in responsible tech? Rebecca, I know that you have a particular focus there.
Movements in responsible tech [20:46]
Rebecca Parsons: Well, people are starting to take it more seriously in the first place. They’re starting to recognize the negative brand implications of breaches or other unmitigated consequences. Their investors are taking note of it, although there is some backlash in various places against ESG reporting, but investors are looking more broadly at how our companies are approaching their technology development.
If you leave hackers aside for the moment, none of us sets out to do bad things to people or communities. We don’t do it intentionally. We’re trying to do the right thing and sometimes the unintended consequences of what we do are good and there are additional benefits that we did not anticipate, but more often than not those unintended consequences are in fact bad. This whole responsible tech movement is around can’t we as technologists be more intentional about trying to see what we wouldn’t otherwise see?
The way I like to think about it, we are problem solvers as technologists. We see a problem, here’s a stakeholder group, here’s the pain point that they have, this is how I’m going to solve it. We have blinders on. Metaphorically, what we need to do is we need to lift our head up and look around the problem. Okay, what are the other stakeholders and communities that are being impacted by this and how? How do they relate to whoever my target audience is?
There are all kinds of wonderful facilitation techniques out there to help us as technologists see outside of our own head. One of my particular favorites is called the tarot cards of tech. They have tarot cards, but they’re posing questions and posing scenarios that will prompt you to think about how someone else might react to your solution or what other impacts might these things have.
I’m quite encouraged at the fact that more organizations are taking this seriously, even if some of it does come from the perspective of compliance. We’re seeing more and more regimes like GDPR, we have accessibility standards, and they’re more or less enforced in different places. Some of it is coming from a compliance aspect, but some of that I think is also coming from this branding aspect. As more of the Gen Z’s come into the talent market, they are expressing, “I don’t want to work for a company that doesn’t actually match my values and live out that particular value statement.”
Responsible tech is the big part of that as well. So I am encouraged.
Shane Hastie: Craig, together we’ve been doing, but you’ve been very much doing a lot of work on ethics. How does that play in here?
Craig Smith: Yeah, I think a couple of things. Slightly segueing back to what Rebecca was talking about, one of the things I’m noticing a lot in organizations is that with all this compliance, a lot of organizations just have their head in the sand. It’s just we have to maintain compliance and we particularly see this in regulated industries like finance and health, where when you talk to a lot of their technical teams, 90% sometimes what they work is just how do we keep the compliance in check?
I think the real challenges for the leadership of those organizations and the organizations in general is how do you then take those things and then innovate within them? Because if you stand back and go, well these particular laws around privacy and security or financial constraints, whatever it is, have been set up for a reason. Whether you agree or disagree, the ones that then innovate around that and go and think about how do I then satisfy the customer? Is the important part as opposed to just how do I satisfy the regulation that comes along? Work within the boundaries that you have being innovative.
In relation into ethical standards that you mentioned there before. For many of us on this call, Rebecca, you’ve been around since the start of this new work with us on the Agile Alliance, as well Shane and I. One of the challenges that you and I were seeing for many years is that there’s been an influx of people who haven’t gone on the same journey as many of us have, whether that be in technical or whether that be in agility. The older of us on the call here, we made the mistakes and righted them. As the industry starts to get older, we don’t remember why we did certain things. The new programmers have never had to go through potentially writing things down to the machine language and understanding what happens at the base, because the compiler or the no-code just takes care of it for them.
In agility, they haven’t been through the why did we go through a whole journey of things like story points, for example? It was because we were trying to solve a problem from way before, but now we’ve moved past things like story points. We’re onto the next step, but they’ve missed the links about why we were there in the first place. When we start talking about ethical type things, it’s how can we make sure that we still understand our base, that we still have this core skill set, but we are operating in an ethical way?
That’s a challenge for lots of organizations, whether it be the work that now you and I have been doing Shane on coaching ethics, or indeed whether you’re talking about programming, testing or any of those other parts of the puzzle that you might be filling.
Shane Hastie: What is the state of play in product management? We’ve had the project to product movement around for a while. We’re certainly seeing it moving to the right on our trends graph, but what is the state of product management?
The state of product management – project to product gaining traction [26:09]
Sharon, maybe you’ve got some thoughts there.
Shaaron A Alvares: Yeah, I think it’s still very slow. There’s a lot of great practices that are getting traction. To draw on what you said about asking the team, I think that’s not happening enough. That’s still not happening downstream. It’s happening upstream, when the product management hand off the requirements, but we still see too much of a silo between product management and then the product owner and the team. Not enough involvement of UX, developers, equality downstream actually. That’s the key challenge I’m seeing that’s still happening now.
There’s a lot of great conversation happening though around discovery. I forgot how it’s called, the three amigos, so it’s getting a little bit of traction but still not enough.
Shane Hastie: Rebecca, what are you seeing in the product management and project to product thinking?
Rebecca Parsons: We’re actually seeing it get more traction definitely. It has not crossed the chasm yet, but people are seeing the value in taking that more user-centered approach and thinking about it more from the perspective of how can I continue to deliver value? As opposed to can I meet this project plan and come in “on time and on budget”?
In fact, why are we building this? If you can’t really answer the question of what the value is of this thing, it doesn’t matter if it was on the project plan, we shouldn’t be building it, if we don’t know what value we’re going to get out of it. I think it’s as much as anything this focus on, we are looking at something that is a product and we are wanting to see how we can increase the value that our end users get from our product.
Value focus is causing this re-evaluation of how do I look at this effort that is now going to go in to create this product? That if I consider it a project, it has an end and it has a scope, whereas a product doesn’t in the same way have that defined scope. It evolves as the value needs of the users evolve or as new users are brought into the fold. I think that in many ways the value mindset is part of what is driving, okay, I need to think about what I am delivering differently. This is easier to talk about in the context of a product life cycle than it is in the context of a project life cycle.
Shaaron A Alvares: In fact, we are seeing a more interest for a value stream. I think organizations are looking more at value stream. I think it ties into the mindset shift between project management, waterfall, and product management. It’s great to see that.
Shane Hastie: That touches on team topologies, team patterns. Raf, I know that this is an area you’re interested in.
Team topologies in rapidly changing environments [29:07]
Raf Gemmail: Going over the topics that we’ve just covered. We’re in this environment where there is this financial pressure, there’s this shift in what teams look like, teams becoming smaller, organizations restructuring on the fly. I think there’s been some intentionality over the last few years to try and set up orgs, where the communications structures enable teams to focus on their value lines.
The fear I have right now is we’re getting into a state where potentially the investment that has been there to try and create longer term efficiencies to enable teams to focus on that product may be compromised by how we restructure organizations. Another thing in there, just commenting on a point that Shaaron made there, was around value streams. Of the last year, I’ve been in a startup, I’m in a very large enterprise now. I could have been right in front of your customer, which I love. Then the customer goes through proxies before the team sees them.
I’ve been trying to get a team to get closer to their customers and it’s really effective, but I found the value streams really helpful both in both contexts actually. To actually draw to teams here, this is why we’re working on this, this is the why. It trickles down to us. When we try and ship software and we continue to monitor in production, this is why it matters and how it feeds back into the loop. Even though we don’t have that closeness that you may have in a startup, you still can try and build some of that empathy. I think that’s really important.
But again, going back to those earlier points, I don’t have that challenge, but I’ve heard of other organizations condensing and bringing teams together as they make mass redundancies. I do wonder what’s going to happen with the team that has way too much cognitive load, that ends up having to manage too many systems. Yeah, we were nicely structured before, we had ownership of these microservices. Now, we’re coming together and we need to know about 20 different things.
I have no data points, so I’ll probably stop there, but there is a level of apprehension as we go through change. How do we preserve these structures that we’ve intentionally put in, to enable us to have good architectures, to have happy teams, to deliver value?
Shane Hastie: Rebecca, reconciling architecture with teams was a point that you raised before we started recording. Do you want to explore that with us a little bit?
Reconciling architecture with teams [31:13]
Rebecca Parsons: When you look at the way, particularly in large enterprises, enterprise architects used to work, it was architecture by decree. We have a very small group that was viewed as overhead and so we had to keep them as small as possible, because that’s what you do with overhead, you try to shrink it and they rule by decree. Part of what I started to try to do, I had a hypothesis actually set many years ago that turned out to be wrong.
My initial hypothesis was that enterprise architecture groups in my experience at that time were generally ineffective. My hypothesis for why was that they didn’t really have a shared understanding about what success looked like. I was giving a workshop at an international software conference, not an Agile conference. This was definitely not an Agile-friendly crowd. There were 53 enterprise architects from around the world. I asked them if they thought they understood what being successful looked like and that that was a shared understanding with everybody.
Everybody put their hand up. It’s like, okay, well that shoots down that hypothesis. I didn’t even get one person to agree with me, but then I asked the follow on question is how many of them felt they were successful? Three people put their hand up. These are clearly not people who were failing at their job, because their companies paid to send them to an international conference. Yet, only three people felt like they were being successful, which told me that the fundamental working model was wrong.
What I started to try to pick apart is what are the things that Agile teams can learn from architects and what are the things that architects need to learn from the teams? I think one of the most important things that the teams need to learn from the architects are what are the things that they’re actually worrying about? Because a software delivery team has one objective. Get business functionality out as quickly and efficiently as possible to delight the users of that software. That’s what they’re there for.
The enterprise architects are there for protecting the overall long-term asset value of the IT estate. Completely different objectives, so it’s not at all surprising that they don’t often see eye to eye. Trying to bridge that gap. One important thing … this was pre-DevOps movement, so this would be a little easier now … but very often the teams didn’t care if their log messages were effective. They didn’t care how easy it was to recover a system or troubleshoot a production problem. That was somebody else’s job. They didn’t know what the enterprise architect was worried about.
Similarly, the enterprise architects, because they’re so vastly outnumbered, because they’re seen as overhead and therefore must be squeezed, they really didn’t understand the reality on the ground. They didn’t know how to get their concerns inserted into that process. That was really where that work started from is how can we get awareness in both directions? What are they actually worrying about and why?
Because at the end of the day, the architects shouldn’t win all the time, but then the development team shouldn’t win all the time either. But you can’t have a conversation, you can’t have that trade off conversation unless there’s shared context. There was not that shared context. That was really the first step.
Shane Hastie: How do we maintain and increase that shared context?
Rebecca Parsons: To me, a lot of it is you have to get the enterprise architects in a position where they are seen as value generating, instead of cost savings, because as soon as you are associated with top line value, you don’t have the same resource squeeze that you do if you’re considered a utility function. Once you can start to get that, you can begin to have the levels of conversation.
I also think by the way, it takes almost a shadow architecture organization, because in a large enterprise with 50,000 developers, you’re not going to be able to have a 1 architect to 10 or 20 developer ratio. There’s still has to be other scaling mechanisms in there, but to me the first thing is to get organizational recognition of the value that you can derive from an enterprise architecture.
In many cases, that means being able to articulate what are the outcomes that I’m trying to achieve with the architectural decisions that I am making? If you focus those things on outcomes that are readily understandable by “the business”, then they are going to start to recognize the value.
In remote environments process and documentation matter more [<a href="javascript:void(0);" onclick="seekTo(35,5335:53<]
Raf Gemmail: I’m keen to share something I’ve seen recently in that space, Rebecca, which is I’ve worked in enterprises which had the enterprise architecture function and seen that mismatch between teams focused on the now and being the person between trying to shepherd them towards the architecture. I’m a big believer in your work and the notion of the emergent architectures, but you’ve got that contention. I’m in an organization now where there’s a really lovely distributed function around democratized function, around looking at that broader architecture.
We’re a family of products which is global. We’re fully remote. This is quite impressive. It’s works and I think it’s works partly because it’s remote, but we move that thinking into more asynchronous processes, RFC processes which are proven and defined most of our standards. Collaborative processes, people providing feedback. Process is probably the word here. One thing I’ve become comfortable with is we’ve always said, “It’s just over,” when people interactions over processes and tools. We said “It’s over,” it’s still important, but there was a bias towards the people interactions.
What I’ve found since I’ve gone remote is that as we set up processes, we set up documentation, people work at their own pace, you set the boundaries that you don’t need to always consciously shepherd. Then people innovate within those boundaries. What I’m seeing, are great architectural decisions, evolution of historic architecture, really powerful conversations that allow teams to get involved, as well as senior engineers resulting in technical outcomes that actually hit backlogs.
It’s an engineering focused culture with a good focus on trying to deliver for product, but I’ve not seen that to this day. It’s working really, really well. I think there may be more evolution to things becoming more asynchronous and slow. I’ve been looking at GitLab and GitLab is very focused around documentation driven cultures, allowing this async to happen. If remote continues the way it has, maybe we’ll move into some of these functions, which are seen as overhead, perhaps being more democratized and shared across teams and across the stakeholders are actually impacted.
Shane Hastie: That’s a really nice segue into what is happening in terms of hybrid, remote, in person. We’re seeing the mix and certainly one of the trends we’ve been looking at is that shift to more asynchronous work.
What do people feel about that?
Hybrid & remote work is now the de facto standard, and it’s still hard [38:12]
Craig Smith: It is now just the de facto standard. I don’t want to say “new normal,” but the thought where everyone throws around. We were moving that way I think the last couple of years anyway, it’s just moved it forward. Again now, it comes back to a segue and a call back to some of the things you talked about earlier, is it starts to show the culture of organizations as to whether they force people back into the office or whether people come together, because it’s the right thing.
Now there are some things, I’m sure we all know where talking face-to-face really does make a difference when you’re really trying to make tough decisions or trying to do things quickly. Yet we didn’t have to get on an airplane to do a recording like this, which even maybe five or six years ago, would’ve been done around a microphone and not in Tools like the ones that we’re using today.
My biggest concern that I’m seeing in my role, though, is that organizations still haven’t truly made this hybrid working option as good as it can be. What worries me is that if we can’t do it through one vendor, and I’ll call Microsoft out here, that if you can’t do it in Teams/Whiteboard/SharePoint, then you can’t do it. There is not one vendor yet that actually has the solution nailed, whether in that market domain or another one.
Organizations still, I believe haven’t all made the switch to tools that really help people work remotely. Rebecca, you were talking about the tools like co-pilot and other things that help engineers. The reality is that once you get outside of the true tech companies, that the people who are just trying to sling code for your bank or insurance company or a small to medium size organization often don’t have the luxuries of those tools, whatever they might be. They’re trying to pull things together.
As a result, they’re the people who are also riding the code that drives our cars and runs our traffic lights and all those kinds of things, which does worry me. Investing in those and making the right decisions is one of the big challenges, whilst also laying on top of that all the challenges that we have in technology, like cybersecurity and other things that go along with it.
You don’t want to have too many tools. The argument that tools like say Miro or Mural, those type of sharing tools is that most organizations don’t want to turn those on, because you can upload a file and drop it onto a board. When you block those things out, it makes those tools less usable, because I need to share an image with Shane in order to make this thing happen.
We’ve still got challenges around how we do this. Again, it comes back a bit to the ethical thing, trusting people to do the work whilst at the same time making sure that we don’t give away all the corporate secrets and throw them into it all the tools.
Shane Hastie: Sharon, you’re in a mega organization that is tackling all of these things. What are you seeing?
Shaaron Alveres: Yeah, speaking about my organization, I want to say that surprisingly we introduced a lot of great practices right at the beginning of the pandemic. We design and rolled out a program that’s called Success from Anywhere. We revised the program, we talked about reviewing priorities, a plan and so on. We called it a “connect from anywhere” when we were in the middle of the pandemic because we realized that working remotely introduced a lot of disconnect between people.
We moved from “success from anywhere” to “connect from anywhere”. We developed a lot of great content to help teams stay connected and also new hires onboard and feel connected with their teams but also with their managers. We also focused a lot on the team working agreement. I would say it’s a small activity, but in this scheme of software development, it’s just a team working agreement, but we realize how important that activity is anytime, but especially when we go through a pandemic. How do we want to connect? How do we want to work together? Because we are completely remote, so we started being 100% remote, but then progressively we introduce some hybrid ways of working. How do we transition from a remote to hybrid? How will we ensure that our teammates who are located, who are not in the US or who are remote still feel connected, included?
I have to say that we’ve done a lot of great work actually. Now, I see a lot of organizations moving to hybrid or 100% back to the office. It’s interesting, because I was making the comparison between what we saw … the bad hybrid and bad return to the office, was which led the great resignation. Now, we are talking about the great layoffs. It’s interesting how events leads to reactions from people, organizations.
Ways of working hybrid and remote is a really, really important topic to solve. I hope that companies are going to keep investing in that because it’s really, really important for the health of the team, for their ability to work together, connect, but also innovate.
Tools can help, but they’re not a panacea [42:52]
Raf Gemmail: I might share from our perspective. I’m in a globally remote team as I said, but my local team, my immediate peers, my first team, based in the same time zone. I’m hiring now and an interesting consideration as I do this is we do async fairly well, but does one open up the local team? That small team trying to keep within Dunbar’s number, does that one keep that communication loop in the same time zone or does one allow that to seep into other time zones?
For now, the focus is teams which are localized, collaborating asynchronously, interfacing each other. I don’t know how that may work for others. I’ve heard of other teams trying to do global remote, but I think you end up with a lot of autonomous people. The collaboration may potentially be compromised, the real time collaboration. We are asynchronously able to communicate with others because … I think we use Slack reasonably well. The things that you can do. I remember Manuel Pias did a talk from some years ago around being intentional about your topologies, your conventions in something like Slack, making the channels discoverable, making sure you didn’t have too much noise.
We have fairly good access to people via Slack. We have the processes, as I said previously that allow us to function asynchronously. Our standups, we have standups currently, four standups which are on a call. One and it may be more later, which is fully async. The async one works really well. People continue with stuff, we talk, but when we have interaction points later in the day, you don’t feel that sense of loss. Some of my teammates, I hadn’t met for months after I joined this context. I felt like I knew them by the time we met in person.
I’ve always been like, “We should get together.” I remember I went to an Alistair Cockburn talk years ago, where I had a distributed team and asked, “How do I get this team over here and this team over here to get together?” He used the word “trust budgets”, for years, I parroted, “We need a trust budget.” It was actually really valuable bringing people together. I still think it is, but our tools have evolved to the stage where it feels first class to have an interaction digitally and to be able to call it and stop.
Obviously, you’ve got the Zoom fatigue and you don’t want to go over, but if you can keep those boundaries right, we can work really effectively remotely. Documentation, source code, pull requests, jumping on calls, swarming where we need to, mobbing where we need to, pairing where we need to, but being intentional and using the tools to our benefit rather than anything else.
Another point, just to Craig’s thing earlier about using these tools, these third party tools, I see tools like Loom, which are really powerful and people use them to share content. Sometimes you’re like, “Oh, has that got something sensitive in it?” It’s a hashed URL, it’s not within the org scope. I think one thing that needs to happen there is being intentional again about what tools that in that organizational context we’re using and managing them intentionally, because security and privacy always is the afterthought. With these, if we’re using them, let’s sign up for licenses. Let’s make them part of the org thing. Let’s put SSO in front of it, let’s stick guidance in front of those.
Shane Hastie: Rebecca, how is Thoughtworks handling this? You’re massive, global and you’re dealing with thousands of different customers as well.
There is no one-size-fits-all approach and you can’t get away from time zones [45:55]
Rebecca Parsons: Yeah, and it varies by country, it varies by client. In places like North America and Australia and Germany, our clients are not saying, “You need to start coming back into our offices five days a week.” In fact, ironically we have more of our individual client people working in our offices, because we do have all of our offices open now. We are not requiring people to come into the office. Certain countries are trying to encourage that. Other countries have said, “We’re going to accept that we have people who will always be fully remote.”
We have, what I would say is lots of different experiments going on across the company on how to do things. One thing, and this is just probably because I spend so much of my time working with global groups, I’m exposed to it more. The one thing that you can’t get away from is time zones. That’s why I think this emphasis on can we look at some of these processes and make them more asynchronous is so important. But for those things that really do require that co-creation that was spoken of earlier, you can’t get away from time zones.
I’ve had people say to me, “There will never again be a global meeting.” And I said, “Well, actually it’s dangerous to use the ‘N word’ anytime. But no, you can’t get away from time zones.” There are going to be things. I think a lot of what we’re going through at the moment is identifying these are the kinds of activities that we can effectively do asynchronously. These are the kinds of activities that should be synchronous, but can still be remote first. These are things that you really have to not only do synchronously, but you’ve got to be co-located, because the exchange needs to be that rich. I think we’re looking at many of our different activities and this is what it takes to do this and this is what it takes to do that.
One final thing that I found interesting, we did experiments again across our development community on remote pairing tools and there wasn’t a winner. Different people would swear, “This is the most wonderful tool available.” Another group would swear, “No, this is the most wonderful tool available and that thing is horrible.”
I think one of the things that we are going to also have to grapple with is, it’s one thing to tell somebody, “Okay, I’m pairing with you Shane, and we’re sitting next to each other and we need to be able to swap keyboards.” There’s a good compelling reason to make sure there’s standardization. As we get further and further away, the needs for, okay, everybody needs to use the same too,. well, the computation is different in that context. I think we’re going to be seeing a lot of innovation in our ways of working as we sort out how to do these different activities.
Raf Gemmail: I’m hoping Rebecca, at some stage I get to pair with people in virtual reality. I’ve got a way of exporting monitors into here, a virtual setup. I can be floating in space and working. I did a demo for some people at work, but I think we’re still in that stage, where there’s a lot of skepticism. And Meta gets a lot of bad rep, because the images that are shown look rudimentary, but I’ve tried out their Work Spaces product. When you’re in, it’s a collaborative thing. The whiteboards, they’re other things I’ve used, but I’m still hopeful we’ll get to the stage where some of the collaboration is a bit more immersive.
Rebecca Parsons: But you still can’t get away from time zones.
Raf Gemmail: You can’t get away from time zones. Oh, one more thing to thread though is that we talked about trust in the team’s building cohesion. As a remote person, it’s really easy to feel or to be worried that you’re going to feel excluded, but we’re doing things like painting events remotely. Sibling team did a cooking event. The games and all sorts of things which have really, I’d say for people are trying to do the remote, make sure you do the social aspects as well, because it builds cohesion, it’s fun. I’ve received in the post canvases and paints.
Awesome! I can’t paint, but by the end of it, I had something and I did it with my team.
Shane Hastie: We have come close to the end of our time box, so we’ll do a quick whip around. Hopes and wishes for 2023 and beyond. Shaaron, can I start with you?
Hopes and wishes for 2023 and beyond [49:59]
Shaaron A Alvares: I really hope if I had a magic wand, I would want all organization to support our teams, our Agile projects, our development teams through the recession, to the priority of productivity and high performance. I think it’s not just about productivity and high performance. I hope we’re not going to stop looking at wellness, mental strength, and also psychological safety. That would be my wish.
Shane Hastie: Raf, your turn.
Raf Gemmail: My hope for this year is that, to echo Shaaron, that we see a rebalancing in how we work. After this right sizing that’s going on in the industry. It settles, my hope is that humanism is still valued and that we work together and we value working together as people. The dollar target will always be there, but how we create the teams that are going to achieve that and deliver to our org and stay within our org, remains the focus.
My hope is that we remain responsible in how we use tools. We were talking about ChatGPT, and it’s going to come regardless. I’m hoping as we embrace this new technology, we’re responsible as to how we use it, how it impacts our teams, how it impacts our planning. I’m hoping we see innovation beyond looking to a few solutions that have come from a few models and we see innovation from other companies trying to localize and train and create models from corpus’ of data for their organizations and context.
I’m hopeful we get to the end of the year after a few rough years (we just had a cyclone in New Zealand) which I’m getting out of and we see a pathway to 2024, which is good for the whole planet actually. A good thing I’ve shared to finish is I saw recently a friend of mine got a technical certification. Now, there’s so many of them out there, but it was a technical certification around green ethical development. I thought that was brilliant to see. I’m hoping we have that more as a focus going forward.
Shane Hastie: Craig.
Craig Smith: Well, Shane, I asked ChatGPT what my hopes are for Culture and Methods were in 2023. It told me that as an AI language model, it doesn’t have access to my personal hopes and aspirations. That’s a good start, but what I hope not to see is that as we have these challenges Ben was just talking about and then everyone was just talking about, is that we resist the pull of the past. That there’s been a lot of good work over the last few years for individuals and organizations to embrace new ways of working and moving technology forward. When things start to become unstable, we tend to often batten down the hatches. I’m seeing that in organizations that we talked about product before. Yeah, it’s like, no, let’s tighten up purse strings and now do a lot of upfront analysis and go back to old project methods.
You could use a lot of different things like that, the way we structure teams, because we’re talking about all the layoffs and things is just let’s just chop off the left arm and remove innovation and all those things. I think the individuals and teams that are going to win are the ones that continue to push forward and continue to innovate. That’s my hope for our listeners, as we move forward is that we still have to chip away the basics.
When you work with organizations like Thoughtworks and things that you can be lulled sometimes in a false sense that all developers are great and they’re all using lots of tools and that DevOps is ruling the world. That isn’t in a lot of places, but when you actually get to the rats and mice of things like government and large corporates, we’re still doing things like we were doing them 40 years ago. We’re making slow inroads, but we still have to chip away at all of those basics, because those organizations have to keep at the same pace as everybody else. If not, we all get dragged backwards. I hope that the people in organizations continue to innovate.
Shane Hastie: And Rebecca.
Rebecca Parsons: A couple of unrelated things. One thing I hope for is that we can continue the move towards more user-centered development, that we can continue to focus on what is the value that we’re actually delivering? Use that to guide our decision making, as opposed to re-trenching too far into, oh, I’ve got to go back into cost cutting. I’ve got to go back into lockdown of my systems, because expense. I think there are some forces in the world that are pushing that direction, because the changes to those expectations of our users are not going to slow down.
Organizations are going to have to continue to respond. I have some hope on that. A second, although this is a pipe dream, but I really wish that our industry could get away from the belief in the one true way or the silver bullet, or, oh yes, if I apply this, a miracle will occur. We still, as an industry have not accepted that there is a lot of actual complexity in the systems that we are building. Yes, we have accidental complexity and we want to continue to chip away at that, but there is essential complexity in there as well. We have to accept that. There isn’t going to be this magic package that you can throw at a complex problem and have it all go away.
Finally, I hope we can continue to raise awareness across the industry of the importance of, in particular, security and privacy. Because there’s still so much low hanging fruit out there for the hackers to exploit and we’re all human beings. We are going to make mistakes, but if we can continue to raise the awareness, find new tools to automate, particular kinds of scans, to reduce exposure, whatever we have to do, but as an industry, I believe we need to take that more seriously. I hope we continue to advance on that journey.
Shane Hastie: For myself, I would echo pretty much everything that you have said and add the wonderful opportunity that it is to work with such a great group of people in the culture and method space. Thank you all so much for the contributions over the last many years.
Rebecca, thank you for joining us as our special guest today.
Rebecca Parsons: Thank you for having me.
EDITORIAL NOTE:
When we recorded this podcast we were also joined by Ben Linders, however a technical glitch meant the file with his voice recording did not get saved so we lost his contribution. Watch for a future podcast where Ben and Shane discuss their views on the current and future state of culture and methods.
.
From this page you also have access to our recorded show notes. They all have clickable links that will take you directly to that part of the audio.
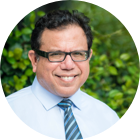
MMS • RSS
Posted on mongodb google news. Visit mongodb google news
PRESS RELEASE
Published March 31, 2023
“market intelligence data That Adds Flavour To Your Success”
The Database as a Service (DBaaS) Provider Market Report 2023 report provides the latest industry data and future industry trends. The forecast market information, SWOT analysis, market scenario, and feasibility study are the vital aspects analyzed in this report.
The worldwide market for Database-as-a-Service (DBaaS), assessed at US$13.5 billion in 2022, is expected to reach an updated size of US$43.1 billion by 2029, expanding at a CAGR of 15.9% over the study period 2023-2029 in the altered post-COVID-19 business environment. The study projects that one of its categories, retail, will grow at a 15.5% CAGR and hit US$14.2 billion by the end of the analysis period. With the current post-pandemic recovery taken into consideration, the BFSI segment’s development is readjusted to a revised 10.3% CAGR for the following 8 years.
Download Full PDF Sample Copy of Database as a Service (DBaaS) Provider Report @
Some of the key players profiled in the study are IBM, AWS, MongoDB Atlas, Ninox, Aiven, Azure, Oracle, Zoho Creator, Kintone, Beats, Google Cloud Bigtable, DataStax, Caspio, SAP, Fusioo and other Prominent players.
Competition is an important issue in any market research analysis. With the help of the competitive analysis provided in the report, players can easily study the key strategies employed by leading players in the Database as a Service (DBaaS) Provider market. The major and emerging players of the Database as a Service (DBaaS) Provider Market are closely studied considering their market share, production, sales, revenue growth, gross margin, product portfolio, and other important factors. This will help players familiarize themselves with the movements of their toughest competitors in the Database as a Service (DBaaS) Provider market.
The segmental analysis section of the report includes a thorough research study on key type and application segments of the Database as a Service (DBaaS) Provider market.
Database as a Service (DBaaS) Provider Market (By Type)
Cloud-based
On-premise
Database as a Service (DBaaS) Provider Market (By Application)
Large Enterprises
SMEs
Purchase Of This Report @
https://www.marketintelligencedata.com/report/purchase/7986401?mode=su?Mode=D211
Important years considered in the Database as a Service (DBaaS) Provider study:
Historical year – 2017-2023; Base year – 2023; Forecast period** – 2023 to 2029 [** unless otherwise stated]
The U.S. Market is Estimated at $3.9 Billion, While China is Forecast to Grow at 14.5% CAGR
The Database-as-a-Service (DBaaS) market in the U.S. is estimated at US$3.9 Billion in the year 2022. China, the world’s second largest economy, is forecast to reach a projected market size of US$7.2 Billion by the year 2030 trailing a CAGR of 14.6% over the analysis period 2022 to 2030. Among the other noteworthy geographic markets are Japan and Canada, each forecast to grow at 14.2% and 13.4% respectively over the 2022-2030 period. Within Europe, Germany is forecast to grow at approximately 11.1% CAGR.
If opting for the Global version of Database as a Service (DBaaS) Provider Market; then the below country analysis would be included:
– North America (the USA, Canada, and Mexico)
– Europe (Germany, France, the United Kingdom, Netherlands, Italy, Nordic Nations, Spain, Switzerland, and the Rest of Europe)
– Asia-Pacific (China, Japan, Australia, New Zealand, South Korea, India, Southeast Asia, and the Rest of APAC)
– South America (Brazil, Argentina, Chile, Colombia, the Rest of the countries, etc.)
– the Middle East and Africa (Saudi Arabia, United Arab Emirates, Israel, Egypt, Turkey, Nigeria, South Africa, Rest of MEA)
Reasons to Procure this Report:
(A) The research would help top administration/policymakers/professionals/product advancements/sales managers and stakeholders in this market in the following ways.
(B) The report provides Database as a Service (DBaaS) Provider market revenues at the worldwide, regional, and country levels with a complete analysis to 2029 permitting companies to analyze their market share and analyze projections, and find new markets to aim for.
(C) The research includes the Database as a Service (DBaaS) Provider market split by different types, applications, technologies, and end-uses. This segmentation helps leaders plan their products and finances based on the upcoming development rates of each segment.
(D) Database as a Service (DBaaS) Provider market analysis benefits investors by knowing the scope and position of the market giving them information on key drivers, challenges, restraints, and expansion chances of the market and moderate threats.
(E) This report would help to understand competition better with a detailed analysis and key strategies of their competitors and plan their position in the business.
(F) The study helps evaluate Database as a Service (DBaaS) Provider business predictions by region, key countries, and top companies’ information to channel their investments.
For More Information or Query, Visit @
What is new in 2023?
– Major developments that can change the business landscape as well as market forecasts.
– Addition/refinement in segmentation–Increase in depth or width of segmentation of the market.
– Coverage of new market players and change in the market share of existing players of the Database as a Service (DBaaS) Provider market.
– Updated financial information and product portfolios of players operating in the Database as a Service (DBaaS) Provider market.
– Updated market developments of the profiled players.
– Any new data points/analysis (frameworks) which was not present in the previous version of the report
The new edition of the report consists of trends/disruptions on customer’s business, tariff and regulatory landscape, pricing analysis, and a market ecosystem map to enable a better understanding of the market dynamics for Database as a Service (DBaaS) Provider.
20% Customization of this Report:
-20% customization.
-Five Countries can be added as per your choice.
-Five Companies can added as per your choice.
-customization upto 40 hours.
-Post-sales support for 1 year from the date of delivery.
inquiry:
Irfan Tamboli (Head of Sales)
Phone: + 1704 266 3234
This Press Release has been written with the intention of providing accurate market information which will enable our readers to make informed strategic investment decisions. If you notice any problem with this content, please feel free to reach us on [email protected]
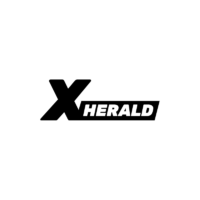
Xherald
Xherald is passionate about offering extensive coverage of global business news. Dedicated to creating every participant’s notification, it reaches its own reader. Daily our experts offer fresh relevant information regarding what is actually occurring in Markets as well as Business. Our headlines are actually swiftly paced and extensive. Our distributor directory connects you with brand new products or services and its market situation from the most effective companies around the planet. Xherald is driven by its 500+ contributors coming from around the world. Xherald provides local as well as global market intelligence information coverage in sectors ranging from healthcare, IOT, chemicals and 17 more sectors. Our updates are quickly paced and detailed. The news we present is actually a mix of authentic content, community contributions and curated headlines announcements. All of the updates our company supplies must pass a strict criterion of genuineness, relevance and significance to our readers.
Article originally posted on mongodb google news. Visit mongodb google news
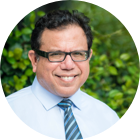
MMS • Steef-Jan Wiggers
Article originally posted on InfoQ. Visit InfoQ
Microsoft recently announced the preview of Azure Cosmos DB for MongoDB vCore, a fully managed MongoDB-compatible database service that provides developers with a familiar architecture for building modern applications with native Azure integrations and a low total cost of ownership.
With the additional Azure Cosmos DB for MongoDB vCore, developers can now choose between two architectures for running their data workloads on Azure Cosmos DB – the original throughput Request Unit (RU) and the newly designed vCore architecture.
According to the Microsoft documentation, the RU-based option works well for building new cloud-native MongoDB apps or refactoring existing apps. In contrast, the vCore-based option is better suited for lifting and shifting existing MongoDB apps to run as-is on a fully supported managed service. In addition, the RU-based option offers up to 99.999% availability with multi-region deployments and limitless horizontal scalability, while the vCore-based option offers competitive SLA (once generally available) and high-capacity vertical and horizontal scaling with vCore-based cluster tier options.
Furthermore, the RU-based option is better suited for workloads with more point reads, fewer long-running queries, and complex aggregation pipeline operations. On the other hand, the vCore-based option can handle workloads with any operation types, including long-running queries, complex aggregation pipelines, distributed transactions, joins, and so on.
Developers can create an Azure Cosmos DB for MongoDB vCore cluster that includes all the MongoDB resources (databases, collections, and documents) and a unique endpoint for various tools and SDKs for connectivity by using the Azure portal or bicep template. Furthermore, some everyday use cases can be storing and querying user-generated content (UGC) for web, mobile, and social media applications, such as chat sessions, tweets, blog posts, ratings, and comments.
Nikisha Reyes-Grange, Director of Product Marketing, Azure Data, told InfoQ:
Empowering MongoDB developers and removing the friction they encounter in the development process of intelligent applications has never been more important. Azure Cosmos DB for MongoDB vCore offers integrations with many Azure services, a familiar vCore architecture, predictable pricing, and enhanced querying to support apps such as chatbots.
Lastly, there are variable fees for RUs and consumed storage with the RU-based option, while with the vCore-based option, customers will pay for the number of vCores provisioned. More details on pricing and availability are available on the pricing page.
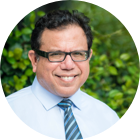
MMS • Sergio De Simone
Article originally posted on InfoQ. Visit InfoQ
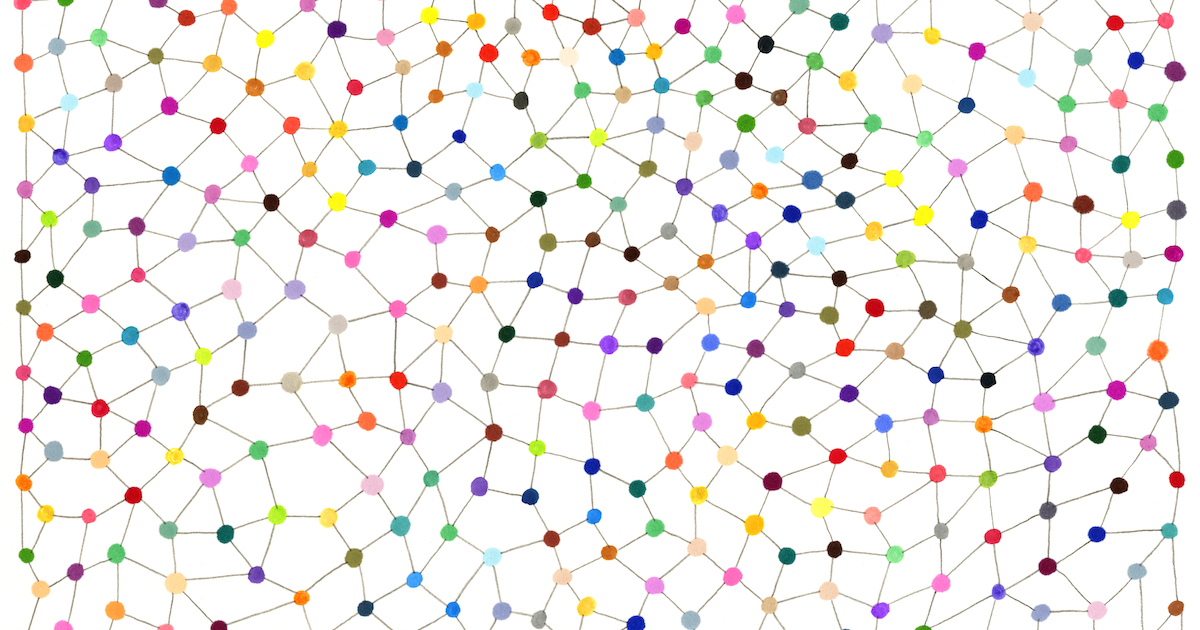
Microsoft has open sourced Semantic Kernel (SK), a lightweight SDK enabling the integration of large language models (LLMs) with conventional programs which can leverage prompt templating, vectorized memory, intelligent planning, and other capabilities.
Semantic Kernel is designed to support and encapsulate several design patterns from the latest in AI research, such that developers can infuse their applications with complex skills like prompt chaining, recursive reasoning, summarization, zero/few-shot learning, contextual memory, long-term memory, embeddings, semantic indexing, planning, and accessing external knowledge stores as well as your own data.
Prompt templating is a mechanism used to define and compose AI functions using plain text. To build prompts, developers can embed expressions within prompts using curly braces. For example, this is how you can call an external function passing an argument to it:
The weather today in {{$city}} is {{weather.getForecast $city}}.
Embeddings make it possible to map each word in a vocabulary to a point in a high-dimensional space that represents its meaning and the relationships between words. This is essential to solutions such as sentiment analysis, document classification, and recommendation systems.
In SK, embeddings are used to create a semantic memory, which mimics how the human brain stores and retrieves knowledge about the world based on the distance existing between concepts. Developers can either use pre-trained embedding models, or train their own using methods such as Word2Vec, GloVe, and FastText.
Another key capability SK provides out of the box is intelligent planning, which consists in working backwards from a user-provided goal. The planner builds thus a reverse sequence of steps, at each step leveraging a pre-defined skill available in a skill library. In case a required skill is not present, the planner can suggest a developer to create that skill or even help them writing the missing skill.
At a lower level of abstraction, a skill is simply a container for a function or a set of functions. In SK, there are three categories of skills: core skills, semantic skills (LLM prompts), and native skills, which are implemented by native code. Microsoft is also providing a number of sample skills for developers to learn how to create one, including chat, coding, classification, summarizing skills and others.
If you are interested to try out Microsoft Semantic Kernel, a good place to start is any of the sample apps that it includes as well as the official Getting Started notebook that will introduce you to the basic syntax to create semantic functions, embeddings, and so on.
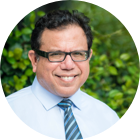
MMS • RSS
Posted on mongodb google news. Visit mongodb google news
Maryland State Retirement & Pension System acquired a new stake in shares of MongoDB, Inc. (NASDAQ:MDB – Get Rating) during the fourth quarter, according to its most recent disclosure with the Securities & Exchange Commission. The fund acquired 5,214 shares of the company’s stock, valued at approximately $1,026,000.
Other institutional investors and hedge funds have also recently added to or reduced their stakes in the company. Sentry Investment Management LLC acquired a new stake in MongoDB in the third quarter valued at approximately $33,000. Alta Advisers Ltd acquired a new stake in MongoDB in the third quarter valued at approximately $40,000. Huntington National Bank lifted its stake in MongoDB by 1,468.8% in the third quarter. Huntington National Bank now owns 251 shares of the company’s stock valued at $50,000 after acquiring an additional 235 shares during the last quarter. Allworth Financial LP lifted its stake in MongoDB by 12.9% in the fourth quarter. Allworth Financial LP now owns 508 shares of the company’s stock valued at $100,000 after acquiring an additional 58 shares during the last quarter. Finally, Quadrant Capital Group LLC lifted its stake in MongoDB by 37.8% in the second quarter. Quadrant Capital Group LLC now owns 419 shares of the company’s stock valued at $109,000 after acquiring an additional 115 shares during the last quarter. 84.86% of the stock is owned by hedge funds and other institutional investors.
MongoDB Trading Up 1.6 %
Shares of MongoDB stock opened at $217.40 on Thursday. The firm has a market capitalization of $15.23 billion, a PE ratio of -43.13 and a beta of 1.00. The company has a debt-to-equity ratio of 1.54, a current ratio of 3.80 and a quick ratio of 3.80. The company’s fifty day moving average price is $214.23 and its two-hundred day moving average price is $195.67. MongoDB, Inc. has a 52-week low of $135.15 and a 52-week high of $471.96.
MongoDB (NASDAQ:MDB – Get Rating) last announced its quarterly earnings results on Wednesday, March 8th. The company reported ($0.98) earnings per share (EPS) for the quarter, beating the consensus estimate of ($1.18) by $0.20. The firm had revenue of $361.31 million for the quarter, compared to analyst estimates of $335.84 million. MongoDB had a negative net margin of 26.90% and a negative return on equity of 48.38%. On average, analysts expect that MongoDB, Inc. will post -4.04 EPS for the current year.
Insider Buying and Selling at MongoDB
In other news, CRO Cedric Pech sold 328 shares of the company’s stock in a transaction dated Tuesday, January 3rd. The shares were sold at an average price of $199.31, for a total value of $65,373.68. Following the sale, the executive now owns 33,829 shares of the company’s stock, valued at approximately $6,742,457.99. The transaction was disclosed in a legal filing with the SEC, which can be accessed through the SEC website. In other news, CEO Dev Ittycheria sold 39,382 shares of the company’s stock in a transaction dated Tuesday, January 3rd. The shares were sold at an average price of $199.96, for a total value of $7,874,824.72. Following the sale, the chief executive officer now owns 190,264 shares of the company’s stock, valued at approximately $38,045,189.44. The transaction was disclosed in a legal filing with the SEC, which can be accessed through the SEC website. Also, CRO Cedric Pech sold 328 shares of the company’s stock in a transaction dated Tuesday, January 3rd. The stock was sold at an average price of $199.31, for a total transaction of $65,373.68. Following the completion of the sale, the executive now directly owns 33,829 shares in the company, valued at approximately $6,742,457.99. The disclosure for this sale can be found here. Insiders have sold 110,994 shares of company stock valued at $22,590,843 in the last 90 days. 5.70% of the stock is currently owned by company insiders.
Analyst Ratings Changes
A number of analysts have issued reports on MDB shares. Mizuho reduced their target price on shares of MongoDB from $220.00 to $180.00 and set a “neutral” rating for the company in a research note on Thursday, March 9th. Citigroup reduced their price objective on shares of MongoDB from $300.00 to $295.00 and set a “buy” rating for the company in a research note on Tuesday, March 7th. Tigress Financial reduced their price objective on shares of MongoDB from $575.00 to $365.00 and set a “buy” rating for the company in a research note on Thursday, December 15th. Stifel Nicolaus reduced their price objective on shares of MongoDB from $256.00 to $240.00 in a research note on Thursday, February 23rd. Finally, Credit Suisse Group reduced their price objective on shares of MongoDB from $305.00 to $250.00 and set an “outperform” rating for the company in a research note on Friday, March 10th. Four equities research analysts have rated the stock with a hold rating and twenty have assigned a buy rating to the company. According to data from MarketBeat, MongoDB currently has an average rating of “Moderate Buy” and a consensus target price of $253.87.
MongoDB Company Profile
MongoDB, Inc engages in the development and provision of a general-purpose database platform. The firm’s products include MongoDB Enterprise Advanced, MongoDB Atlas and Community Server. It also offers professional services including consulting and training. The company was founded by Eliot Horowitz, Dwight A.
Read More
Want to see what other hedge funds are holding MDB? Visit HoldingsChannel.com to get the latest 13F filings and insider trades for MongoDB, Inc. (NASDAQ:MDB – Get Rating).
Receive News & Ratings for MongoDB Daily – Enter your email address below to receive a concise daily summary of the latest news and analysts’ ratings for MongoDB and related companies with MarketBeat.com’s FREE daily email newsletter.
Article originally posted on mongodb google news. Visit mongodb google news
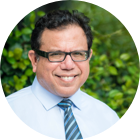
MMS • Ben Linders
Article originally posted on InfoQ. Visit InfoQ
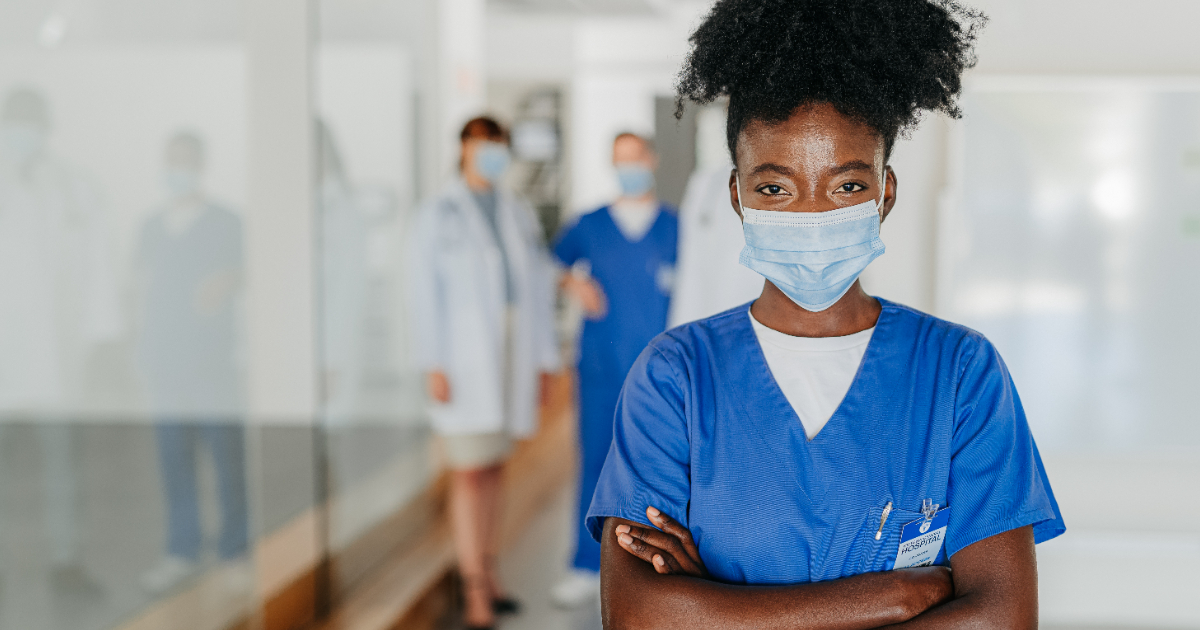
Asking people how they feel about taking certain types of risks can give insight into the level of psychological safety and help uncover issues. Discussing the answers can strengthen the level of safety of more mature teams and help less mature teams to understand how they could improve.
Jitesh Gosai shared his experience with psychological safety in his talk at Lean Agile Scotland 2022.
Measuring psychological safety levels in teams is challenging as it’s hard to judge if someone feels like they are taking an interpersonal risk. Most people don’t think about risk-taking this way, and usually take a chance based on their situation and feelings, Gosai said.
One way to do it is to use a proxy measure of how people think about taking certain types of risks and if they feel supported to do so in their team:
While this still doesn’t give you a definitive scale of psychological safety in a team, or even if people will take interpersonal risks, it can at least indicate if you have any issues that would prevent people from doing so.
Using this scale is a very simple mechanism for understanding where a team is with psychological safety, Gosai mentioned. How people interpret questions can vary and how they respond can depend on how they feel that day.
Gosai mentioned two risks that could come from trying to measure psychological safety in teams. One is that if the responses are not anonymous, an individual could be singled out for pulling down the team average. This could make them feel they are being scrutinised for speaking up, potentially making them less likely to do so in the future. Another consequence of this could be that if others see this happening, they too may hold back, Gosai said.
The other risk Gosai mentioned is if these ratings are used to compare teams and their leads, rather than used to offer help to teams. Then they become just another metric to try and game, giving an even more skewed view of the team.
Gosai mentioned that most teams, instead of trying to measure psychological safety, would benefit from just starting to build a shared understanding of what it is first:
It could seem wasteful for teams with high levels, but they still stand to benefit by strengthening what they have. In contrast, those teams with low levels have everything to gain.
InfoQ interviewed Jitesh Gosai about measuring psychological safety.
InfoQ: How do you measure psychological safety?
Jitesh Gosai: Amy Edmundson provided a set of sample questions in her book The Fearless Organisation that team members can answer using a seven-point Likert scale which can be used as a starting point to gauge where teams are with psychological safety.
Based on how they respond, leaders can see if they have low, medium or high levels of psychological safety. For instance, if they score on the low end of the scale, then that could be seen as low levels of psychological safety, and leaders should take immediate action.
InfoQ: What did you learn from measuring the level of psychological safety?
Gosai: An ideal situation would be that questions related to psychological safety are incorporated into regular team health check questionnaires so that teams can see if there is an overall trend.
If they are trending down, then the team can look to see what has happened recently to cause this, but also, if they are trending up, they can see what they improved to do so. This way, the team can see the benefit of answering the questions as accurately as possible, rather than just another tick-box exercise.
When working with leaders, I find they know how their behaviours affect teams, but they often haven’t made that link to psychological safety. So helping them make that connection could immediately impact psychological safety in teams.
In Learnings from Applying Psychological Safety Across Teams, Gosai explained how they applied ideas from psychological safety to make it safe for people to take interpersonal risks. Creating Environments High in Psychological Safety with a Combined Top-Down and Bottom-Up Approach describes how complementing leadership with team workshops in communication skills can enable people to speak up and feel safe to fail.
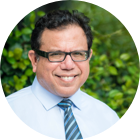
MMS • RSS
Posted on nosqlgooglealerts. Visit nosqlgooglealerts
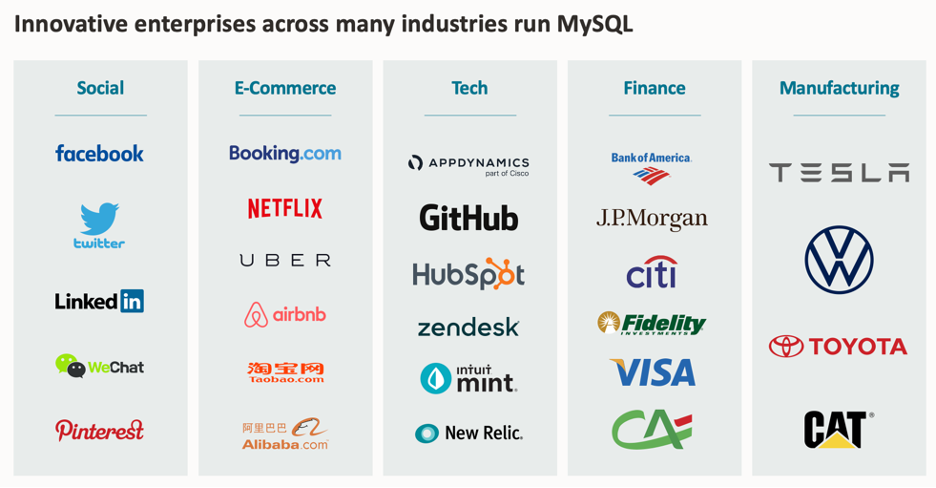
Since Oracle launched its MySQL HeatWave service in December 2020, it has continuously driven differentiation in the database-as-a-service market. While competing against some of the biggest names in the cloud space, the company has shown what appears to be “too good to be true” price-for-performance against competitors such as Snowflake.
With the latest update to MySQL HeatWave, Oracle shows no signs of slowing down. And I think this service may be the “killer app” for Oracle Cloud Infrastructure (OCI)—or even Oracle as a whole. I’ll explain my reasoning in this article.
MySQL HeatWave changed the analytics game
Most IT folks who have spent time around databases understand the pervasiveness of MySQL in the enterprise. Since it was released under the GNU General Public License (GPL) in 2000, the database platform has exploded in popularity among companies of all sizes, from smaller organizations that want a SQL database without the considerable cost, to enterprise organizations deploying large departmental databases. My first exposure to the platform came in 2003, when I worked as an IT executive in a large state government organization. We used MySQL everywhere possible to lower costs without sacrificing performance and functionality.
Over the years, MySQL became the world’s most popular open-source database and the second-most popular database overall (after Oracle Database). Yes, while a lot of the buzz is for the likes of MongoDB and other “cool” NoSQL platforms, the top two database distributions—by far—are owned by Oracle. And they are both SQL-based.
The challenge I saw when running a state IT organization is the same challenge that IT orgs have been facing ever since: data silos and the fact that MySQL is not optimized for analytics. When a business has hundreds or even thousands of MySQL instances, integrating all of that data and gleaning insights from it via analytics is painful. All too often, business users must rely on IT to perform time-consuming and costly—yet error-prone! —extract, load and transform (ETL) processes to bring all the data to one central location for analysis. Or brave business users might attempt this themselves, then reach out to IT a few weeks later when they’ve given up trying. By that time, they’re analyzing old data.
The MySQL development team at Oracle recognized this challenge impacting customers, and MySQL HeatWave was born. The idea was simple: deploy a cloud service whereby customers of all sizes could run real-time analytics on all of their data—both transactional and historical—and enable it with “point-and-click” simplicity, and without needing the dreaded ETL.The challenge I saw when running a state IT organization is the same challenge that IT orgs have been facing ever since: data silos and the fact that MySQL is not optimized for analytics. When a business has hundreds or even thousands of MySQL instances, integrating all of that data and gleaning insights from it via analytics is painful. All too often, business users must rely on IT to perform time-consuming and costly—yet error-prone! —extract, load and transform (ETL) processes to bring all the data to one central location for analysis. Or brave business users might attempt this themselves, then reach out to IT a few weeks later when they’ve given up trying. By that time, they’re analyzing old data.
The MySQL development team at Oracle recognized this challenge impacting customers, and MySQL HeatWave was born. The idea was simple: deploy a cloud service whereby customers of all sizes could run real-time analytics on all of their data—both transactional and historical—and enable it with “point-and-click” simplicity, and without needing the dreaded ETL.
MySQL delivers extreme analytics performance
Oracle
When I look at MySQL HeatWave, I consider two things—the richness of features and the ability of an average user to take advantage of these capabilities. From both perspectives, I’m impressed. As touched on above, the performance numbers are almost too good to be true. More than that, using this service is simple: no refactoring or rearchitecting of applications, no new analytics or visualization tools to learn. Just point the tool in the right direction, click a couple of times, and you have a database environment that supports online transactional processing (OLTP) and analytics.
MySQL Autopilot drives real automated operations
Some product manager once said, “You never know how your product is going to perform until it’s in the hands of paying customers.” Maybe that product manager was me in a previous part of my career. In any case, this truism is obvious to anyone who has ever launched a product.
When Oracle launched its first update to the MySQL HeatWave service in mid-2021, it focused on automating the data management lifecycle using machine learning (ML). In fact, MySQL HeatWave Autopilot was the first service I saw that automated many DBA functions that would previously consume hours a week.
MySQL Autopilot automated many database operations in 2021
Oracle
Database tuning is an art form requiring both technical depth and something like clairvoyance. Deploying and provisioning databases is hard enough but tuning them is a never-ending process—one that can consume database professionals. To alleviate this, MySQL Autopilot combined deep analytics and finely tuned ML models to drive a continually optimized and always resilient MySQL database environment.
ML-driven data management lifecycle
Oracle
This update of MySQL HeatWave is also where I started to pay attention to Oracle’s competitive performance comparisons. Once again, the numbers initially seemed too good to be true. Suffice it to say that HeatWave outperformed every major cloud provider in analytics.
Of special note was its performance relative to the very popular Snowflake. When running TPC-H (a decision support benchmark), MySQL HeatWave showed an incredible 35x price/performance advantage over Snowflake. In terms of raw performance, HeatWave had a 6.8x advantage, and in terms of price, a 5.2x advantage. Pretty compelling, right? Benchmarks can be manipulated—or so I would think. Except that Oracle publishes its test harness on GitHub so customers can see for themselves. That shows real confidence in their capabilities.
HeatWave AutoML and multi-cloud—a natural next step
After introducing MySQL Autopilot, Oracle’s next big act with HeatWave was the integration of ML into MySQL HeatWave for model training purposes—aptly named HeatWave AutoML. This gave HeatWave the ability to automate the building, training, tuning, and explaining of ML models in real-time based on the data residing in MySQL. Again, HeatWave is democratizing machine learning by enabling this functionality for all, so that companies that don’t have teams of data scientists or TensorFlow developers can gain the same kinds of insights and automation usually reserved for larger organizations.
Additionally, Oracle embraced the notion of multi-cloud by releasing MySQL HeatWave on AWS. That means the entire MySQL HeatWave environment—core capabilities, Autopilot and ML—is available for customers to use on AWS. Oracle did this to bring the value of MySQL HeatWave to customers already using AWS who might otherwise be priced out of the service because of data egress fees. (To use HeatWave in-memory analytics, you would have to move all your data from AWS into OCI.) So, rather than force this difficult decision, Oracle stood up the HeatWave service natively—including the control plane, data plane, and console— to run on AWS. Is performance as good as it would be on OCI? No. But it’s still really good. And there is no sense that Oracle is delivering an underpowered product to ultimately convince customers to move to OCI.
If your data resides on Microsoft Azure instead, life is equally easy for you. Because Oracle and Microsoft have deployed a low-latency layer-2 network connection, you can simply use HeatWave on OCI while your applications still reside in Azure. No latency hit, no cost-prohibitive ingress and egress fees.
As an analyst who was also an Oracle customer for some time, I feel like I’m witnessing a new company with a new attitude. In the past, Oracle was not known for its emphasis on making life easy for customers. In comparison to that, its trajectory with MySQL HeatWave is a breath of fresh air.
MySQL HeatWave’s latest release: more ML goodness
In the latest update to HeatWave, the team at Oracle has doubled down on ML by driving usability and automation. The democratization of ML only really happens when ML functions are practically available to all—meaning that they don’t require a team of developers and data scientists. And Oracle has delivered even further on that promise with its latest release.
Fully automated training and explanations with HeatWave AutoML
Oracle
The result of Oracle’s work is automated machine learning that is highly performant and fully automated. And here’s what’s interesting: because of the highly parallelized architecture of MySQL HeatWave AutoML, these models are running on commodity CPUs. This drives down costs considerably—savings that are passed on to customers. So that price performance advantage I discussed earlier? Now it’s even better.
In addition to focusing on usability, Oracle has delivered three new ML capabilities in this latest update that are worth quickly highlighting.
- Unsupervised anomaly detection. This feature identifies events deviating from the norm using a single algorithm. Banks looking to detect fraud, operational technology organizations looking for IoT sensor outliers, and cybersecurity teams focused on intrusion detection are all use cases that would benefit from this ability. HeatWave is the only solution to perform this detection at the local, global, and cluster levels with a single algorithm and a fully automated process. This makes it faster and more accurate than manually selecting, testing, and tuning multiple individual algorithms.
- Recommender system availability. This capability automates the use of recommender systems—which recommend movies to watch and products to buy—in HeatWave, driving significantly faster training time coupled with a lower error rate (as compared with a public-domain solution). By comparison, other services only recommend algorithms, putting the burden on users to select the most appropriate one, and then manually tune it.
- Multivariate time series forecasting. This feature automates the process for companies to accurately predict time series observations based on a number of variables. This would apply, for instance, if a power company is trying to determine seasonal electricity demands when considering other energy sources, weather, and so on. What once would require a team of data scientists is now just a few mouse clicks away.
Additionally, Oracle has made enhancements to MySQL Autopilot that further improve ML automation in a workload-aware way. Whether an organization is using HeatWave for OLTP, OLAP, or data lakehouse purposes, Autopilot removes mundane and tedious chores from the hands of administrators.
Oracle beefs up Autopilot to support new use cases and capabilities
Oracle
If the above graphic looks familiar, it is an update covering available Autopilot capabilities. The MySQL team at Oracle has added considerable functionality as MySQL Autopilot has evolved into a data management platform.
MySQL moves to a Lakehouse
Last October at CloudWorld 2022 Oracle announced MySQL HeatWave Lakehouse (currently in beta), continuing to extend HeatWave’s database capabilities. It enables customers to process and query hundreds of terabytes of data in object store in a variety of file formats, such as CSV and Parquet, as well as Aurora and Redshift export files.
Keeping with tradition, MySQL HeatWave Lakehouse delivers significantly better performance than competitive cloud database services for running queries (17X faster than Snowflake) and loading data (2.7X faster than Snowflake) on a 400TB TPC-H benchmark.
In addition, in a single query, customers can query transactional data in the MySQL database and combine it with data in the object store using standard MySQL syntax. New MySQL Autopilot capabilities that improve performance and make MySQL HeatWave Lakehouse easy to use also became available, increasing administrators’ productivity.
Is MySQL HeatWave really Oracle’s killer app?
Maybe I was being a little hyperbolic with my blog title. But MySQL HeatWave was revolutionary from the moment Oracle released it in late 2020. And it continues to separate itself from competing, but single-focus, database cloud solutions as Oracle adds functionality. MySQL HeatWave is evolving from an OLTP + in-memory analytics tool into something much bigger—if not a data management platform, then a fully featured OLTP, data analytics, machine learning, and lakehouse platform within one integrated database.
My only question is, what’s next? Considering how quickly Oracle has been innovating with HeatWave, I don’t think it will take long to find out.
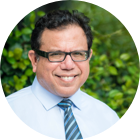
MMS • RSS
Posted on mongodb google news. Visit mongodb google news
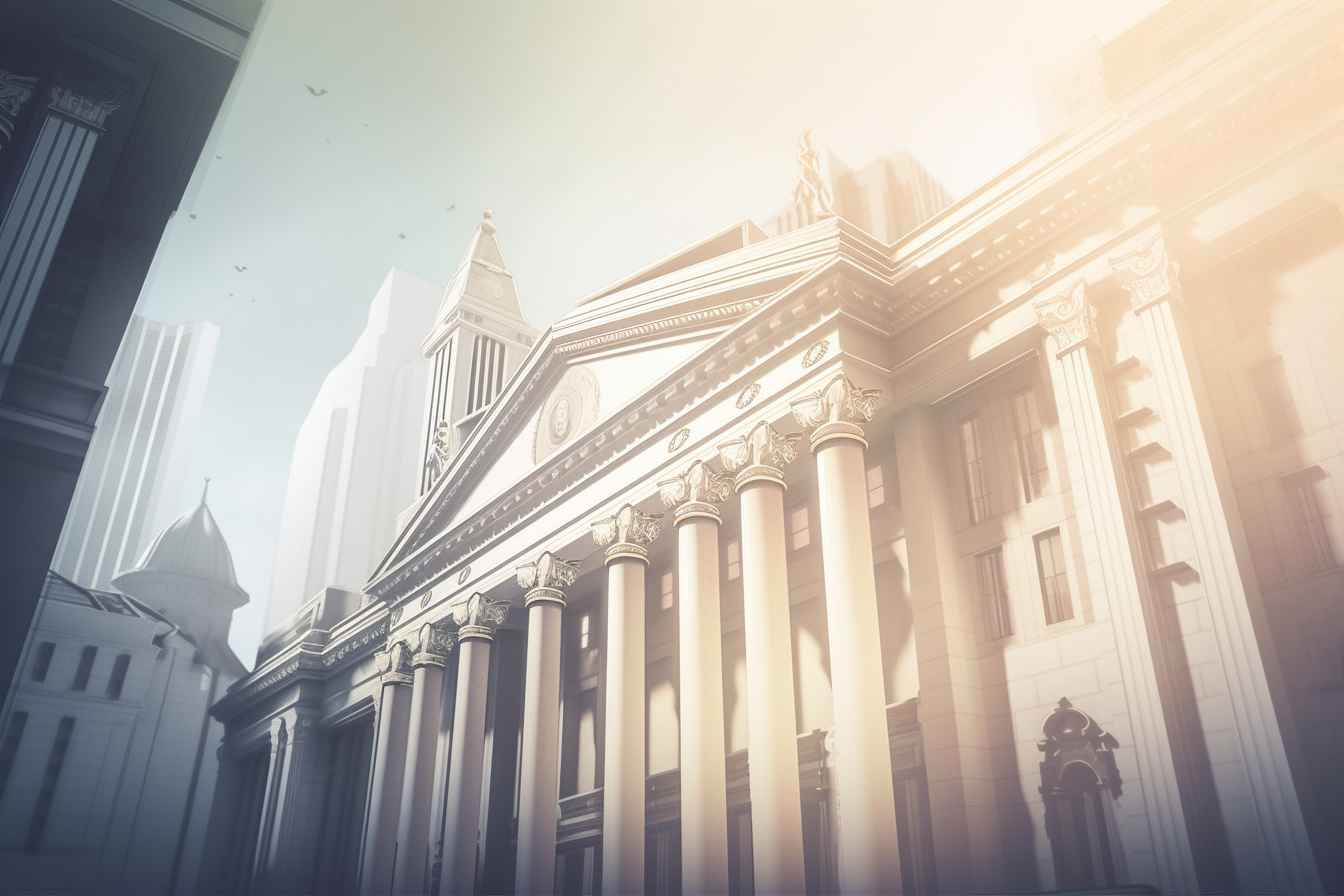
As per recent reports by TradingView News, a big-time investor has adopted a pessimistic stance on MongoDB, the article highlights that almost 68% of investors have opened trades with a bearish outlook, while only 31% have taken a bullish view. The report also sheds light on the specific details of some of the works made by investors, including putting businesses with a bearish outlook and call transactions with a bearish outlook. Some put trades include a $350.00 strike price with an expiration date of January 19, 2024, valued at $404.7K, and another put trade with the same strike price and expiration date valued at $202.4K. A put sweep trade with a strike price of $145.00 expiring on January 20, 2023, valued at $129.0K, was also made. Lastly, a call trade with a $135.00 strike price expiring on December 16, 2022, valued at $93.0K was placed.
MDB Stock Performance on May 14, 2021: Positive Trend with Strong Financial Performance
The stock performance of MDB (MongoDB Inc.) on May 14, 2021, shows a positive trend. The previous close of MDB was $213.83, and it opened today at $216.02. The day’s range was between $215.46 and $219.49, with a volume of 26,725. The average volume of the last three months was 1,935,606, and the market cap was $15.2B.
MDB’s earnings growth for last year was -5.89%, but it has shown a positive trend this year with a growth rate of +26.99%. The expected earnings growth for the next five years is +8.00%. The revenue growth rate for last year was +46.95%, indicating a solid financial performance.
MDB’s P/E ratio is NM, indicating that it has no earnings or negative earnings per share. The price/sales ratio is 11.45, and the price/book ratio is 20.45, meaning the stock trades at a premium compared to its peers.
MDB’s stock performance is favorable compared to other technology services companies. The stock price of SPLK (Splunk Inc.) increased by +1.98%, PTC (PTC Inc.) increased by +0.65%, and ZS (Zscaler Inc.) increased by +0.62%.
MDB’s next reporting date is June 1, 2023, and the EPS forecast for this quarter is $0.20. The annual revenue for last year was $1.3B, but the company reported a loss of -$345.4M. The net profit margin was -26.90%, indicating that the company’s expenses exceeded its revenue.
MDB is a packaged software company in the technology services sector. The corporate headquarters is located in New York, New York. However, there are no executives to display.
In conclusion, MDB’s stock performance on May 14, 2021, shows a positive trend with increased stock price and volume. The company’s financial performance has been positive this year but reported a loss last year. Investors should monitor the company’s financial performance and future earnings reports to make informed investment decisions.
MongoDB Inc: A Promising Investment Opportunity in the Tech Sector
, 2021
MongoDB Inc (MDB) is a popular database software provider making waves in the tech industry. The company’s stock has been performing well, with a steady increase in price over the past year. The 22 analysts offering 12-month price forecasts for MDB have a median target of 247.50, with a high estimate of 290.00 and a low estimate of 180.00. This indicates that the stock is expected to continue its upward trend in the coming months.
The consensus among 27 polled investment analysts is to buy stock in MongoDB Inc. This rating had held steady since March, when it was unchanged from a buy rating. This suggests that investors are optimistic about the company’s prospects and are confident in its ability to deliver strong returns.
Looking at the company’s recent financial performance, MongoDB Inc reported earnings per share of $0.20 and sales of $348.0M for the current quarter, with a reporting date of June 01, 2021. These impressive figures indicate that the company is on track to achieve its financial targets.
Overall, the outlook for MDB stock is positive, with analysts predicting a continued increase in price over the next 12 months. The company’s strong financial performance and positive investor sentiment suggest it is a solid investment opportunity for those looking to invest in the tech sector. However, as with any investment, conducting thorough research and analysis is essential before making decisions.
Article originally posted on mongodb google news. Visit mongodb google news