Month: December 2022
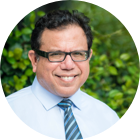
MMS • Ben Linders
Article originally posted on InfoQ. Visit InfoQ
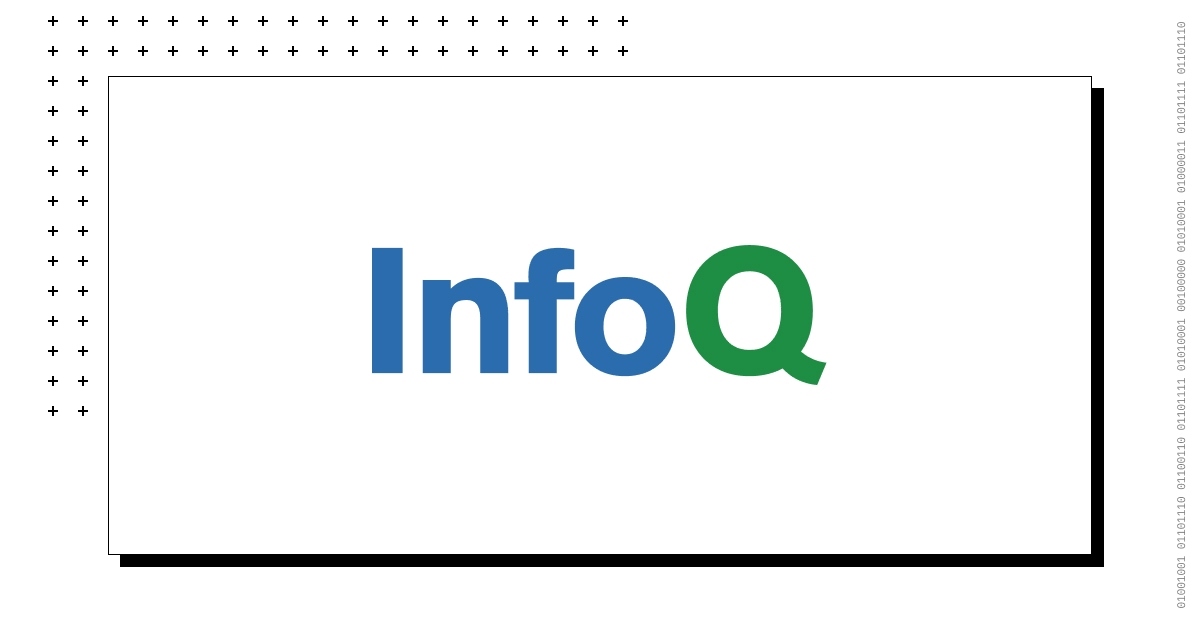
The quality practices assessment model (QPAM) explores quality aspects that help teams to deliver in an agile way. The model covers both social and technical aspects of quality; it is used to assess the quality of the team’s processes and also touches on product quality. With an assessment, teams can look at where their practices lie within the quality aspects and decide on what they want to improve.
Janet Gregory spoke about assessing quality using this model at Agile Testing Days 2022.
The quality practices assessment model has ten quality aspects. Each quality aspect has several practices to be considered, although Gregory mentioned they are not specific agile practices.
The quality aspects are:
- Feedback loops
- Culture
- Learning and improvement
- Development approach
- Quality and test ownership
- Testing breadth
- Code quality and technical debt
- Test automation and tools
- Deployment pipeline
- Defect management
The first three are social aspects, how people work within the quality system, Gregory explained. The following two are a combination of social and technical, and the last five are purely technical aspects of the system.
Gregory mentioned that the behaviour exhibited by teams for each quality aspect, falls into one of four dimensions: Beginning, Unifying, Practicing and Innovating. This does not mean that every quality aspect for a team falls into the same dimension, she said.
Exploring the first quality aspect of feedback loops, the practices looked at are communication and feedback cycles within the delivery team, between the customers, stakeholders, and the delivery team, and also between leadership and the delivery team.
Gregory explained the role that feedback loops can play in quality:
We list feedback as the most important quality aspect, which can take many forms. Teams use feedback to determine whether they are on the correct path to create a valuable solution for both customers and the business. Communication among team members is also a form of feedback to understand their current state and how to achieve their goals together.
The faster the feedback loop, the easier it is to make adjustments as needed, Gregory concluded.
The quality practices assessment model is described in the book Assessing Agile Quality Practices with QPAM which Gregory co-authored with Selena Delesie and is listed on Gregory’s publications page.
InfoQ interviewed Janet Gregory about the quality practices assessment model.
InfoQ: Looking at the 7th quality aspect, what can teams do to increase code quality and reduce technical debt?
Janet Gregory: Technical debt is anything that needs fixing, improvement, restructuring, maintenance, or even further testing later. It exists because the team took a shortcut to deliver the software “today”. Unfortunately, some shortcuts never get addressed.
Addressing technical debt is first about recognizing it exists, then planning how to address that hidden backlog. Outstanding defects are a good indication that technical debt exists.
Start by creating simple coding standards that all programmers can live with and hold peer reviews on any new or changed code going forward. This helps eliminate “new” technical debt.
Create an impediment backlog listing the areas that have been compromised, including areas with large concentration of defects. Plan to tackle one of those areas regularly – perhaps one every iteration. With every new story, look at the code and incorporate the standards as needed, building in testability and adding appropriate automation for fast feedback.
Teams who prioritize continuously addressing technical debt can safely modify existing code, create new features, and reliably deliver high quality and high value to their customers.
InfoQ: How can teams use the model to improve their quality practices?
Gregory: Once an assessment has been completed, teams can look at where their practices lie within the quality aspects. Once a team knows where they are, and reflects on what they want to improve, they can look at the rest of the model to see what might be possible. Teams may decide that their level of a practice/aspect is where they want to be, and that is ok.
The model is meant for teams to dig deep and reflect on the quality of their products and the quality of their agile practices. It may extend to multiple teams to reflect on the entire organization.
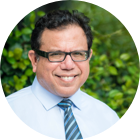
MMS • Steef-Jan Wiggers
Article originally posted on InfoQ. Visit InfoQ
Microsoft recently announced the open-source release of Azure DeepStream Accelerator (ADA) in collaboration with Neal Analytics and NVIDIA, allowing developers to build Edge AI solutions with native Azure Services integration quickly.
Specifically, the Azure DeepStream Accelerator provides a simplified developer experience for deploying accelerated computer vision workloads at the edge. Microsoft aims to provide developers with the ability to create NVIDIA DeepStream AI-based solutions and integrate them with a multitude of Azure services, such as Blob Storage and Monitor.
The Azure DeepStream Accelerator consists of several components:
- An AI Pipeline Container, ingesting USB or RTSP camera streams and applying AI models to the video frames. The outputs of the models are multiplexed together and then sent out to the Business Logic Container for user logic to handle.
- A Controller Module responsible for configuring the whole system.
- A Business Logic Container (BLC) where a user’s application logic resides and is configured through the Controller Module.
- A Video Uploader Container responsible for taking inferences and MP4 video snippets and uploading them to the cloud.
- An Azure DeepStream Accelerator Player is a JavaScript widget that provides a convenient way to view video snippets found in the user’s connected Blob Storage.
Tony Sampige, a principal program manager at Microsoft, explains in a Tech Community blog post:
The open-source project includes tools to ease the developer journey, including a region of interest widget and supplementary developer tools that developers can leverage to build, manage, and deploy their AI solutions to NVIDIA’s AGX Orin edge devices and more. Additionally, ADA provides support for 30+ pre-built AI models out of the box (Nvidia, ONNX, TF, Caffee, Pytorch, and Triton models) and the ability to bring your own Model/Container for deployment to IoT edge devices.
In addition, Imran Bashir, an IoT, AI, and computer vision engineer, commented on a LinkedIn post:
ADA will give your team a jump start and can save your company tons of time and money. ADA provides a low-code environment and allows any developer to build complex video analytics applications without mastering all the underlying technologies.
More details on DeepStream are available on Nvidia’s documentation and DeepStream SDK | NVIDIA Developer. Furthermore, Microsoft provides a learning module on DeepStream development.
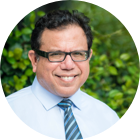
MMS • Sergio De Simone
Article originally posted on InfoQ. Visit InfoQ
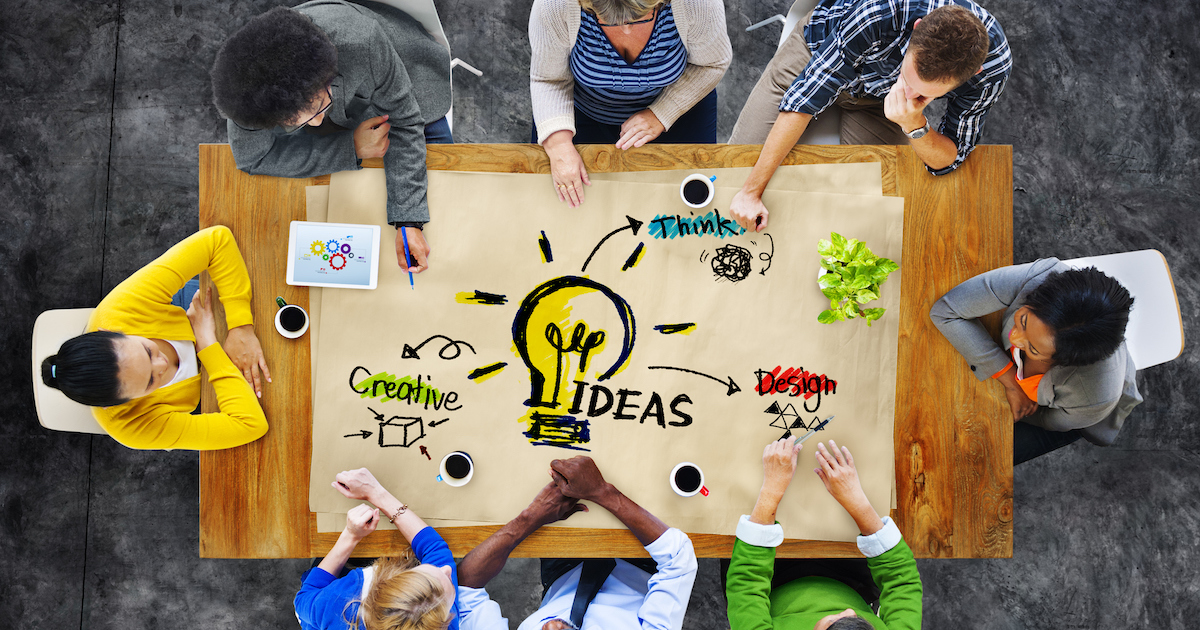
Engineers at Uber created their own remote development environment to improve developer experience and productivity by fixing a number of issues brought about by their adoption of a code monorepo.
Uber’s remote development environment (RDE), named Devpod, follows the same philosophy as other recently introduced RDEs such as GitHub Codespaces, JetBrains Space, and AWS CodeCatalyst. This includes the possibility of setting up a project in a few seconds, centralizing security, and improving performance thanks to the huge processing power available in the Cloud:
Moving from laptop to cloud enabled us to experiment with machines that have 90+ cores and hundreds of Gigabytes of RAM.
One of the driving factors behind Uber’s decision to adopt a remote development environment was the inherent complexity of their huge monorepo, which consolidated over 4000 services, 500 Web apps and many tools across thousands of repositories.
Our move to monorepo provided several important benefits including better dependency management; consistent and universal library versions in production; single, centralized build platform (Bazel); easier support for a standard set of tools and processes; and improved code visibility, collaboration, and code sharing.
As Uber engineer Leo Horie explained on Hacker News, concrete examples of the advantages brought by the monorepo is fixing log4j vulnerabilities in a centralized way, simplifying npm auditing, and so on. He also stresses that the main problem a monorepo is trying to solve is not technological, rather it is a people problem:
For example, say you cron CI job fails. Then what? Someone needs to look at it. It’s easier for someone to fix things they currently have context for (e.g. if I upgrade Python or update some security-related config and something breaks, I can reason it was my change that broke it), vs an unsuspecting contractor getting around to some backlog task 6 months after the fact with no context.
Unfortunately, the monorepo made builds slower, especially on laptop and when working remotely, with cloning and setting up a project taking hours.
With their current Devpod implementation, though, Uber engineer have been able to speed up a number of important metrics, including git status
and Go build times, by a factor ranging between 1.8 and 2.5. It’s hard to say if this is a good result, considering the amount of effort that Uber must have put into Devops, but Devpod adoption inside of the organization at over 60% of the engineering workforce, seems to indicate this was the right move for Uber.
To create Devpod, Uber chose to go with Debian-based Docker images and Kubernetes as a deployment platform. Kubernetes clusters are deployed in several locations to be closer to engineers and reduce latency. To make management easier, they also created a centralized Web app and provided mechanisms to keep all environments up to date.
Uber engineers are still hard at work on Devpod to make it faster to clone a project, to introduce ephemeral environments, improve IDE experience, and allow for team-specific configuration.
It is not clear yet if Uber will ever open source Devpod, but their decision to migrate to a remote development environment could be a sign of a growing interest towards RDEs, as attested by Slack and GitHub cases, too.
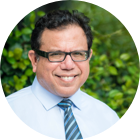
MMS • Edin Kapic
Article originally posted on InfoQ. Visit InfoQ
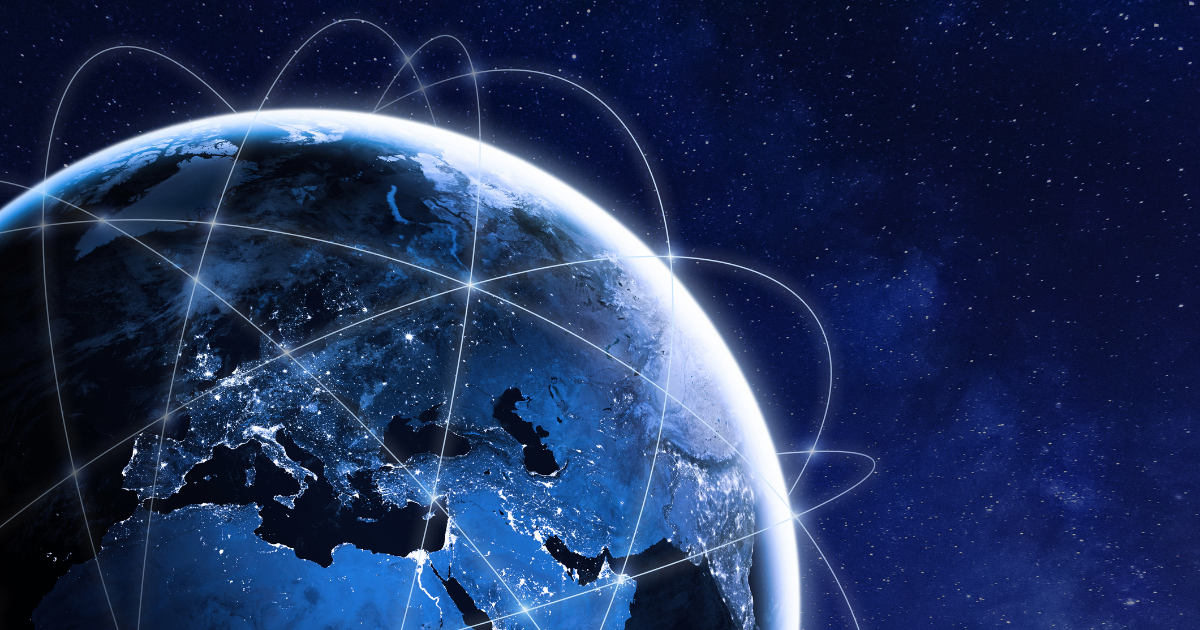
On November 8th, 2022, Microsoft announced the general availability of their .NET multiplatform framework MAUI for the recently launched .NET 7 major release. MAUI is now supported on both .NET 6 and .NET 7, running on Windows, macOS, iOS, Android and Tizen. The open-source MAUI Community Toolkit has also been updated in coordination.
MAUI is an acronym that stands for Multiplatform Application UI. According to Microsoft, it’s an evolution of Xamarin and Xamarin Forms frameworks, unifying separate target libraries and projects into a single project for multiple devices. Currently, MAUI supports writing applications that run on Android 5+, iOS 11+, macOS 10.15+, Samsung Tizen, Windows 10 version 1809+, or Windows 11.
The version 7 of MAUI brings the support for .NET 7, as the version released for Visual Studio 17.3 was only supporting .NET 6 upon launch in . The Visual Studio update 17.4 now supports full MAUI workload on .NET 7. To upgrade from .NET 6, only the target framework moniker (TFM) in the project file has to be changed.
Together with the support for .NET 7, the MAUI update also brings the support for Tizen, the mobile operating system from Samsung. Another important highlight from the new release is the native MAUI map control, lifted and updated from Xamarin.
Later in November, after the release, Microsoft added .NET MAUI with .NET 7 as a supported workload for Visual Studio on Mac.
.NET MAUI is now supported on .NET 6 until May of 2023 and on .NET 7 until May of 2024. This is consistent with .NET concurrent versions support.
.NET MAUI Community Toolkit (NMCT) is one of Microsoft’s .NET community toolkits hosted on GitHub. Their purpose is to let the community contribute useful code missing in the official frameworks. The community toolkits are released as open-source software and they encourage the developers to submit their contributions.
An updated version of NMCT was released the day after the .NET 7 official launch. The version 3.0.0 of the toolkit brings support for .NET 7, as expected, together with the support for Tizen.
There are three new components available in the NMCT 3.0.0:
- Expander view allows for simple content reveal when the header is clicked.
- DockLayout brings the capability to dock views to the sides of the layout container.
- StateContainer allows for multiple views of the content, based on the state, such as no content state, errored state, normal state and loading state.
The .NET MAUI Community Toolkit is versioned in three different releases, allowing for the use of the toolkit by both .NET 6 and .NET 7 developers:
- Version 1.4.0 includes the new components on .NET 6
- Version 2.0.0 brings Tizen support on .NET 6
- Version 3.0.0 includes the support for .NET 7
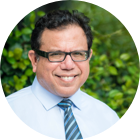
MMS • Michael Redlich
Article originally posted on InfoQ. Visit InfoQ
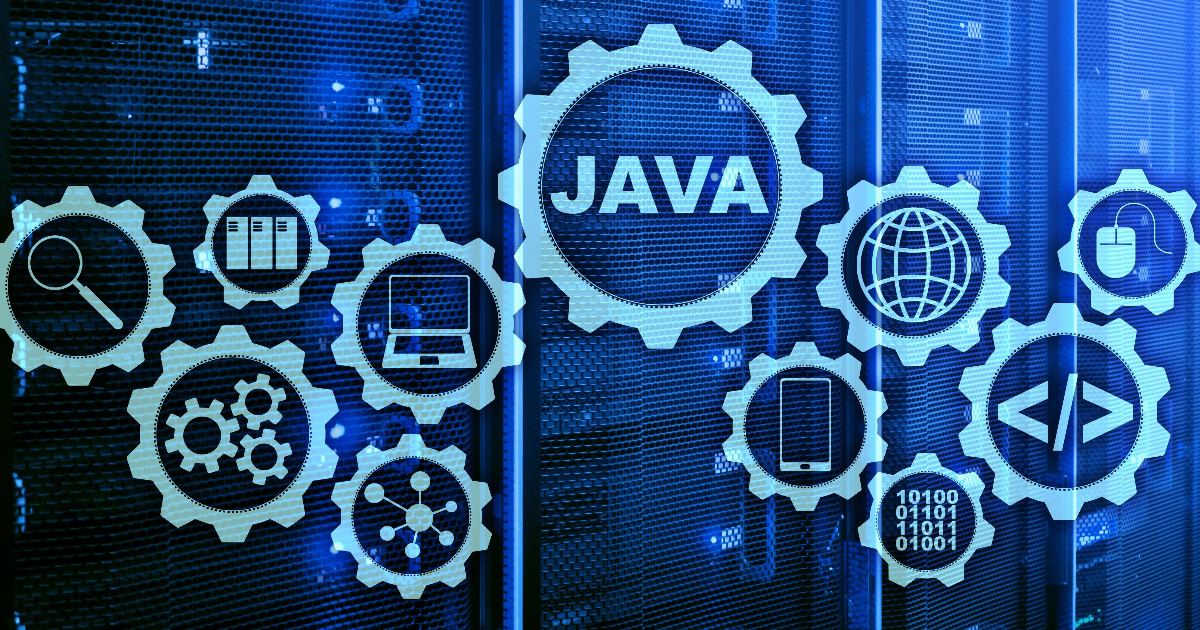
This week’s Java roundup for December 19th, 2022 features news from OpenJDK, JDK 20, JDK 21, Spring Cloud 2022.0.0, point releases for other Spring projects, Open Liberty 22.0.0.13, Quarkus 2.15.1, Micronaut 3.7.5, Helidon 3.1.0, Hibernate 6.2.CR1, Eclipse Vert.x 4.3.7, point releases for Groovy, Camel 3.20, MicroStream joins Eclipse, Kotlin 1.8-RC2, and introducing SourceBuddy, Jarviz and Just.
OpenJDK
Ioi Lam, consulting member of technical staff at Oracle, has proposed improved support for archived Java heap objects in class data sharing (CDS) because Project Leyden will, more-than-likely, make extensive use of archived Java heap objects.
Due to a number of defined limitations in the G1, SerialGC and ParallelGC garbage collection algorithms related to CDS, the goals in this proposal are: uniform support of CDS for all collectors; and minimize the code needed for each collector to support CDS.
Recently submitted and subsequently updated issues already related to proposal are:
InfoQ will monitor progress on this proposal.
JDK 20
Build 29 of the JDK 20 early-access builds was made available this past week, featuring updates from Build 28 that include fixes to various issues. More details on this build may be found in the release notes.
JDK 21
Build 3 of the JDK 21 early-access builds was also made available this past week featuring updates from Build 2 that include fixes to various issues.
For JDK 20 and JDK 21, developers are encouraged to report bugs via the Java Bug Database.
Spring Framework
The release of Spring Cloud 2022.0.0, codenamed Kilburn, delivers GA updates to Spring Cloud sub-projects such as: Spring Cloud OpenFeign 4.0.0, Spring Cloud Commons 4.0.0, Spring Cloud Function 4.0.0 and Spring Cloud Starter Build 2022.0.0. There are, however, breaking changes with the removal of sub-projects: Spring Cloud CLI, Spring Cloud for Cloud Foundry and Spring Cloud Sleuth. Spring Cloud 2022.0.0 builds upon Spring Framework 6.x and Spring Boot 3.x and includes compatibility with Jakarta EE and requires a Java 17 baseline. Further details on this release may be found in the release notes and InfoQ will follow up with a more detailed news story.
Versions 6.0.1, 5.8.1, 5.7.6 and 5.6.10 of Spring Security have been released featuring bug fixes and improvements in documentation. In all four releases, the deprecated set-state and set-output commands defined in GitHub Actions have been replaced, presumably with the recommended $GITHUB_STATE
and $GITHUB_OUTPUT
environment files. More details on these releases may be found in the release notes for version 6.0.1, version 5.8.1, version 5.7.6 and version 5.6.10.
Spring Boot 3.0.1 has been released featuring 54 bug fixes, improvements in documentation and dependency upgrades such as: Spring Security 6.0.1, Spring Integration 6.0.1, Reactor 2022.0.1, Tomcat 10.1.4 and Jetty 11.0.13. Further details on this release may be found in the release notes.
Spring Boot 2.7.7 has also been released featuring 24 bug fixes, improvements in documentation and dependency upgrades such as: Spring Security 5.7.6, Spring Integration 5.5.16, Reactor 2020.0.26, Tomcat 9.0.70 and Jetty 9.4.50.v20221201. More details on this release may be found in the release notes.
The release of Spring for GraphQL 1.1.1 ships with new features such as: new builder customizers to customize the QuerydslDataFetcher
and QueryByExampleDataFetcher
repository classes by allowing these repositories to implement customizer interfaces; and relaxing the generic type check in @Argument Map
as it was determined that this was too strict. There were also dependency upgrades to: Micrometer 1.10.2, Reactor 2022.0.1, Spring Framework 6.0.3 and Spring Security 6.0.1. Further details on this release may be found in the release notes.
Spring Modulith 0.2 has been released that delivers: a new @ApplicationModuleListener
annotation to ease the declaration of asynchronous, transactional event listeners that run in a transaction; the application module dependency structure is now also exposed as Spring Boot actuator; and the Postgres event publication registry schema now follows Postgres best practices. More details on this release may be found in the release notes.
Open Liberty
IBM has released Open Liberty 22.0.0.13 featuring: the ability to configure the maximum age of their First Failure Data Capture (FFDC) application data collection system; and fixes for CVE-2022-3509 and CVE-2022-3171, both of which have parsing issues with text data and binary data, respectively, in the Protocol Buffers Java core and lite versions prior to 3.21.7, 3.20.3, 3.19.6 and 3.16.3 that can lead to a denial of service attack.
Quarkus
Red Hat has released Quarkus 2.15.1.Final that ships with bug fixes, improvements in documentation and dependency upgrades to Vert.x 4.3.6, Infinispan 14.0.3.Final, Dekorate 3.1.3 and mongo-client.version
4.8.1. Further details on this release may be found in the changelog.
Micronaut
The Micronaut Foundation has released Micronaut 3.7.5 featuring bug fixes and improvements such as: a change in the resulting Health status output from “Health monitor failed check with status {}” to “Health monitor check with status {}” since Health status only reports UP
or DOWN
; and an instance of the TextStreamCodec
class now requires a bean of type ByteBufferFactory
to fix a loading issue. More details on this release may be found in the release notes.
Helidon
Oracle has released Helidon 3.1.0 featuring: new Exponential
and Fibonacci
strategies defined in the Retry
interface; support for the MicroProfile Config specification via a new MPConfigSourceProvider
interface; a new relativeUris
property in the OidcConfig
class to allow an OIDC web client to use a relative path on the request URI; and dependency upgrades such as GraalVM 22.3.0, Netty 4.1.86.Final and PostgreSQL JDBC driver 42.4.3.
Hibernate
The first release candidate of Hibernate ORM 6.2 provides: support for Java records, mapping composite/struct types, and the Jakarta Persistence 3.1 specification; UUID support for MariaDB 10.7 and SQL Server 2008; allow the @SqlInsert
, @SqlUpdate
, @SqlDelete
annotations to refer to a SecondaryTable
class; and a new @PartitionKey
annotation to identify a field of an entity that holds the partition key of a table.
Eclipse Vert.x
In response to a number of reported bugs found in version 4.3.6, Eclipse Vert.x 4.3.7 has been released featuring a dependency upgrade to Netty 4.1.86 to address CVE-2022-41881, HAProxyMessageDecoder Stack Exhaustion DoS, and CVE-2022-41915, HTTP Response Splitting from Assigning Header Value Iterator. Further details on this release may be found in the release notes.
Apache Software Foundation
A maintenance release of Apache Groovy 3.0.14 features bug fixes, dependency upgrades and two improvements: the static type checker does not recognize closure input parameter when implementing an interface with a map; and the evaluateExpression()
method defined in the StaticTypeCheckingSupport
class can now provide lightweight evaluation for simple expressions. More details on this release may be found in the release notes.
Apache Groovy 2.5.20, also a maintenance release, features bug fixes, dependency upgrades and the same type checker improvement as described in version 3.0.14. Further details on this release may be found in the release notes.
The release of Apache Camel 3.20.0 delivers over 200 bug fixes, improvements, dependency upgrades and new features such as: provide completion for positional file path parameters (camel-jbang
component); added support for Event Resources (camel-kubernetes
component); provide a prefixId
to the route model such that generated IDs of the route is prefixed (camel-core
component); and a new camel-etcd
component. More details on this release may be found in the release notes.
MicroStream
MicroStream has announced that they have joined the Eclipse Foundation as a member. With MicroStream already integrated with Helidon and Micronaut, their goal is to closely collaborate with the Eclipse community and actively contribute to Eclipse projects. InfoQ will follow up with a more detailed news story.
Kotlin
The second releases candidate of Kotlin 1.8.0 delivers fixes such as: an IllegalStateException
upon reading a class that delegates to a Java class with a definitely non-nullable type with a flexible upper bound; an argument for the UseSerializers
class does not implement an instance of the KSerializer
interface or does not provide serializer for a concrete type; and no mapping for symbol, VALUE_PARAMETER SCRIPT_IMPLICIT_RECEIVER
, on the JVM IR backend.
SourceBuddy
SourceBuddy, a new utility that compiles Java source code dynamically created in a Java application, was introduced by Peter Verhas, architect at EPAM Systems, this past week and has also quickly released version 2.0 featuring: a major restructuring of the class loading structure; hidden and non-hidden classes may now be mixed; and removal of the loadHidden()
method in favor of the hidden()
method for each added source separately. As the latter feature is a breaking change, it was necessary to provide a major release within the short timeframe. InfoQ will follow up with a more detailed news story.
Jarviz
Jarviz, a new JAR file analyzer utility, has been introduced by Andres Almiray to the Java community. Version 0.1.0 delivers: GAV support to resolve JARs; streamline manifest commands; and resolve the output directory before invoking URL-based processors. Further details on this release may be found in the release notes and InfoQ will follow up with a more detailed news story.
Just
Just, a command Line toolkit for developing Spring Boot applications, was introduced by Maciej Walkowiak, freelance architect & developer, earlier this month. Version 0.12.0, released this past week, features: a significant improvement to the just kill
command such that it stops/kills a running Docker container, instead of killing the Docker process, when it learns that there is a Docker container running on a chosen port; two new configuration properties, just.build.extra-args
and just.build.maven.profiles
, for the just build
command to simplify the build configuration; and the value, SPRING_BOOT
, can be set to the just.framework
property if the framework autodetection fails. InfoQ will follow up with a more detailed news story.
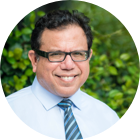
MMS • Anthony Alford
Article originally posted on InfoQ. Visit InfoQ
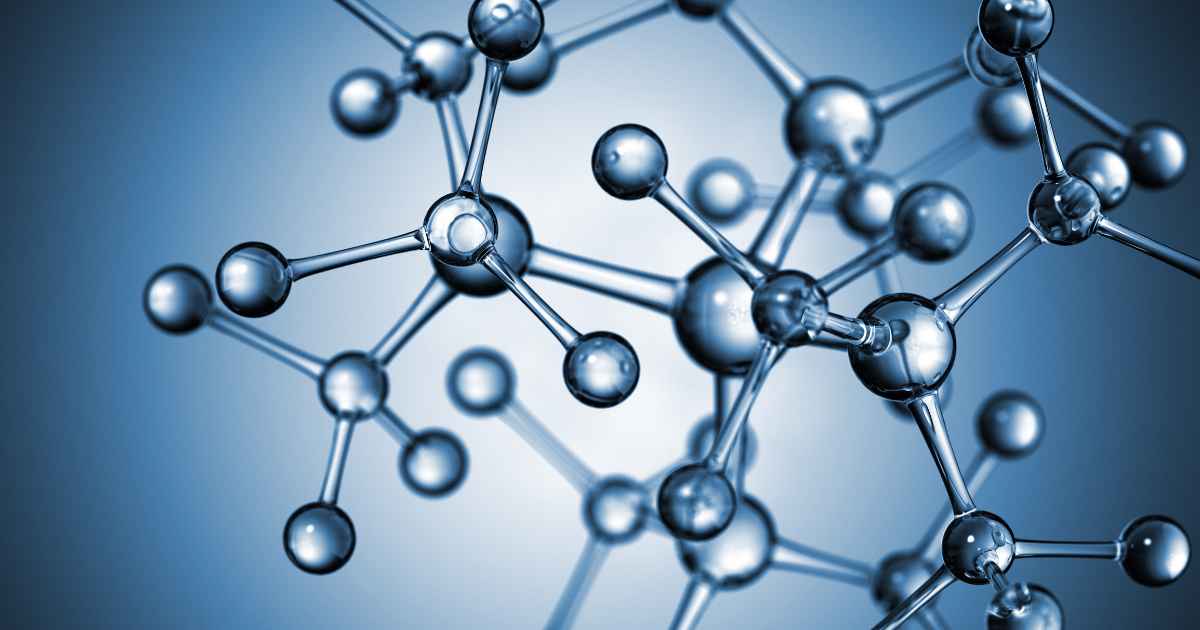
Microsoft announced the release of ML.NET 2.0, the open-source machine learning framework for .NET. The release contains several updated natural language processing (NLP) APIs, including Tokenizers, Text Classification, and Sentence Similarity, as well as improved automated ML (AutoML) features.
Program manager Luis Quintanilla announced the release at the recent .NET Conf 2022. The updated NLP APIs are powered by TorchSharp, a .NET wrapper for the popular PyTorch deep learning framework. The release includes the EnglishRoberta tokenization model and a TorchSharp implementation of NAS-BERT, which is used by the Text Classification and Sentence Similarity APIs. Updates to AutoML include an API for automated data pre-processing and a set of APIs for running experiments to find the best models and hyperparameters. Quintanilla also announced a new release of the Model Builder tool for Visual Studio, which includes the new text classification scenario and advanced training options.
The Text Classification API, which was previewed earlier this year, is based on the NAS-BERT model published by Microsoft Research in 2021. This model was developed using neural architecture search (NAS), resulting in smaller models than the standard BERT model, while maintaining accuracy. Users can fine-tune the pre-trained NAS-BERT model with their own data, to fit their custom use cases. The Sentence Similarity API uses the same pre-trained model, but instead of being fine-tuned to classify an input string, the model takes two strings as input and outputs a score indicating the similarity of the meaning of the two inputs.
The AutoML APIs are based on Microsoft’s Fast Library for Automated Machine Learning & Tuning (FLAML). While the Featurizer API is designed for pre-processing, the rest of the APIs work together to search for the best set of hyperparameters. The Experiment API coordinates the optimization of a Sweepable pipeline over a Search Space using a Tuner. Devs can use the Sweepable API to define the training pipeline for hyperparameter optimization of their models; the Search Space API for configuring the range of the hyperparameter search space for that pipeline; and the Tuner API to choose a search algorithm for that space. The release includes several tuner algorithms, including basic grid and random searches as well as Bayesian and Frugal optimizers.
Quintanilla also gave viewers a preview of the ML.NET roadmap. Future plans for deep learning features include new scenarios and APIs for question answering, named-entity recognition, and object detection. There are also plans for TorchSharp integrations for custom scenarios and improvements to the ONNX integration. Other plans include upgrades to the LightGBM implementation and to the implementation of the IDataView interface, as well as improvements to the AutoML API.
At the end of his presentation, Quintanilla answered questions from the audience. One viewer asked about support for different vendors’ GPUs and accelerator libraries, and Quintanilla noted that currently only NVIDIA’s CUDA accelerator is supported. When another viewer asked whether ML.NET’s object detection algorithms would run fast enough to support a live video stream, Quintanilla replied:
We want to focus on performance. We’re introducing new deep learning scenarios and we realized that performance is key there, so performance is a focus for us going forward.
The ML.NET source code is available on GitHub.
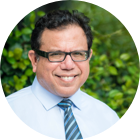
MMS • Steef-Jan Wiggers
Article originally posted on InfoQ. Visit InfoQ
Recently AWS announced it would make two changes to Amazon Simple Storage Service (Amazon S3): all buckets in a region will have S3 Block Public Access enabled and access control lists (ACLs) disabled by default. These changes will take effect in April 2023 and will be rolled out by the company in all AWS Regions within weeks.
Amazon S3 is a managed object storage service on AWS, and its S3 buckets and objects have always been private by default. The company added Block Public Access in 2018 and the ability to disable ACLs in 2021 to provide customers more control. In addition, customers can also leverage AWS Identity and Access Management (IAM) policies to manage access.
Both S3 Block Public Access enabled and access control lists (ACLs) disabled were default settings in the console. As of April 2023, they will become the default for buckets created using the S3 API, S3 CLI, the AWS SDKs, or AWS CloudFormation templates.
Source: https://aws.amazon.com/blogs/aws/heads-up-amazon-s3-security-changes-are-coming-in-april-of-2023/
Through these new defaults, customers who do require applications to have their buckets publicly accessible or use ACLs must deliberately configure their buckets to be public or use ACLs. To configure these settings, they must update automation scripts, AWS CloudFormation templates, or other infrastructure configuration tools.
Other public cloud providers Microsoft and Google also offer managed storage services with security defaults. For instance, Azure Storage accounts, by default, do not allow public access to containers. However, the default configuration for an Azure Resource Manager storage account permits a user with appropriate permissions to configure public access to containers and blobs in a storage account. Similarly, public access to Google Cloud Storage buckets can be prevented.
An IT & Infosec Consultant, regarding the defaults, tweeted:
Make it easy to do the usually right thing and harder to do the usually wrong thing.
In addition, a respondent on a Reddit thread commented:
Good security to make it the default. I hope a lot of lab blogs get their instructions updated for this, or there will be a lot of new AWS users confused as they are learning. I’ve seen so many labs use public buckets.
Lastly, more details on the changes are available on the FAQ page.
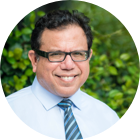
MMS • Daniel Dominguez
Article originally posted on InfoQ. Visit InfoQ
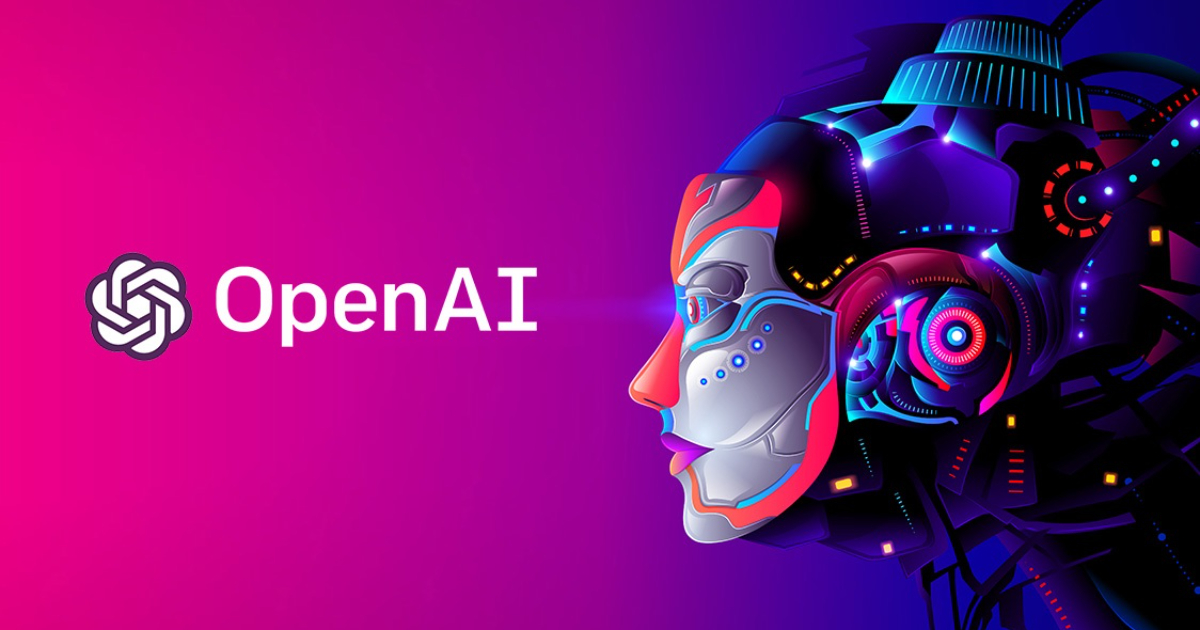
OpenAI is introducing text-embedding-ada-002, a cutting-edge embedding model that combines the capabilities of five previous models for text search, text similarity, and code search. This new model outperforms the previous most capable model, Davinci, on most tasks, while being significantly more cost-effective at 99.8% lower pricing. In addition, text-embedding-ada-002 is easier to use, making it a more convenient option for users.
Embeddings are numerical representations of concepts that allow computers to understand the relationships between those concepts. They are often used in tasks such as searching, clustering, recommendation, anomaly detection, diversity measurement, and classification. Embeddings consist of vectors of real or complex integers with floating-point arithmetic, and the distance between two vectors indicates the strength of their relationship. Generally, closer distances indicate a stronger connection, while farther distances indicate a weaker one.
There are seventeen different embedding models available through OpenAI, including one from the second generation and sixteen from the first generation. The chosen approach for OpenAI is text-embedding-ada-002. Compared to alternatives, it is more practical, affordable, and efficient.
In addition to its capabilities and cost effectiveness, text-embedding-ada-002 is also simpler to use than previous models. This makes it a convenient choice for those who want to save time and effort when implementing embedding solutions.
Since the initial release of the OpenAI embeddings endpoint, several applications have adopted embeddings to tailor, suggest, and search information. One example of improved models is the new embedding model, which is a more effective tool for NLP and other coding-related tasks.
According to OpenAI, the new embedding model is a far more potent tool for jobs involving code and natural language processing. Nevertheless, embedding models may be unreliable or pose social risks in certain cases, and may cause harm in the absence of mitigations.
With the introduction of text-embedding-ada-002, embedding technology has advanced significantly. It is an invaluable tool for a variety of applications and users due to its potent combination of efficiency, affordability, and usability.
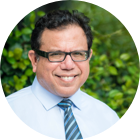
MMS • Nafees Butt
Article originally posted on InfoQ. Visit InfoQ
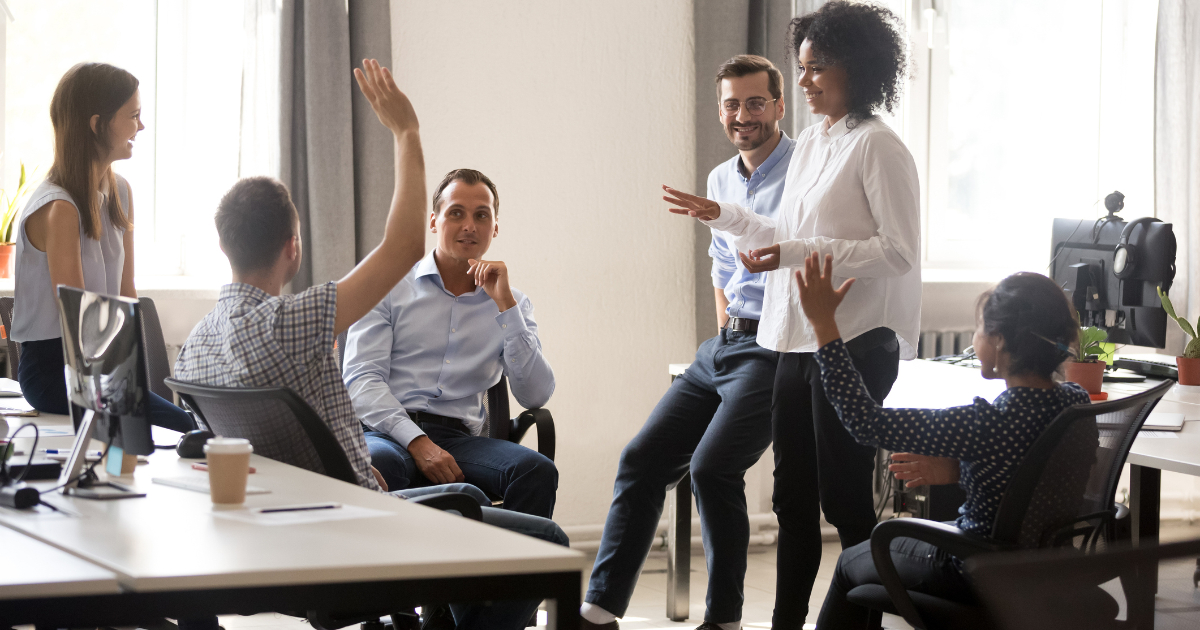
Key Takeaways
- The most common modality in coaching today is individual coaching. Group coaching, i.e. a coaching relationship between these individuals, is an approach that hasn’t become mainstream as of yet.
- Group coaching uses relationship system intelligence, that builds on top of emotional intelligence and social intelligence, to help groups/teams realise their untapped potential.
- Focusing on relationship instead of the individual, trusting the group’s ability to self-heal and a heightened psychological safety needs are some of the building blocks of group coaching.
- Coaches need to acquire additional skills and experiences to be able to effectively tap into the benefits of group coaching.
- Helping teams grow into productive and amazing teams via group coaching, by focusing on the relationship between the individuals, is the next frontier of coaching.
There are so many groups and teams out there that could be much more productive if they tapped into the relationship side of things and worked on becoming a better team before working on the problems they are solving. Group coaching is a skill growing in popularity over the last few years. It is getting a lot of traction for coaching senior executive leadership teams or for coaching situations that pay attention to relationships and interconnection among the members of a group.
In this article I provide an introduction to group coaching and explain how it is different from individual coaching. I shed light on the benefits of using group coaching, skills that coaches would need and the challenges they would face, based on my experience. I briefly touch on an example scenario using one of the group coaching techniques and describe the context in which such a technique can be used.
With this article on group coaching, I aim to pique your interest and attract more coaches; aspiring to join the coaching ranks or already well versed in individual coaching, to venture into the world of group coaching. It is my hope that as a collective, we can extend the growth opportunity from individuals to groups/teams and help them grow into productive and amazing teams, making this world a better place.
Group vs Individual Coaching
Let’s start by looking at group coaching in comparison to individual coaching. The most important difference between individual and group coaching is what they focus on. Coaching individuals is powerful in its own right and has its place, though in complex relationships and systems there is a lot that is not specific to one individual, but how the individuals interact with each other and the intangible relationships between the individuals. Group coaching focuses on that intangible relationship between the individuals.
Take the example of a deterministics mechanical system where a group of components are combined together to perform a function bigger than what each component can perform. When something is not right with the mechanical system, the problem can be tracked down to how each individual component is working or at the exact interface between two components. The components don’t have emotions; they don’t behave differently based on their cultural upbringing, or what side of the bed they woke up that morning. There is no ego, no hidden agendas, no career ambitions, no hierarchy of roles, gender/religious/other stereotypes.
Humans and the systems/relationships humans form in organisations or in personal life have all of these and more. Humans are not deterministic, i.e., given the same input they don’t produce the same outputs. The interaction between them is affected by all of the factors mentioned above. Practically speaking this creates another entity between the individuals that is unique to that group. An entity that is intangible but it is embodiment of the relationship between the individuals of that group. The group coaching focuses on that intangible entity between the individuals.
Building Blocks
The basis of group coaching, to be able to comprehend and to practise it, lies on a few building blocks. First, as a coach since our focus is on the relationship and interactions between the individuals, we don’t coach individuals in separate sessions. Instead, we bring them together as the group/team that they are part of and coach the entire group. Anything said by one member of the team is heard by everyone right there and then.
The second building block is holding the mirror to the intangible entity mentioned above. To be accurate, holding the mirror is not a new skill for proponents of individual coaching, but it takes a significantly different approach in group coaching and has a more pronounced impact here. Holding the mirror here means picking up the intangibles and making the implicit explicit, for example, sensing the mood in the room, or reading the body language, drop/increase in energy, head nods, smiles, drop in shoulders, emotions etc. and playing back to the room your observation (sans judgement obviously). Making the intangibles explicit is an important step in group coaching – name it to tame it, if you will.
The third building block is the believing and trusting in the group system that it is intelligent and self-healing. It builds on the second building block mentioned above that once the intangibles are made explicit, we trust in the ability of the group system to use that information to heal itself and move/grow from that position to another. As coaches, we don’t steer that in a direction of our liking, but trust that whatever direction that it takes is the right next natural progression for the third entity.
The fourth and last building block is the heightened psychological safety needs. While having psychological safety is an important ingredient in individual coaching, its significance shoots through the roof in group coaching. Since there are multiple people in the group being coached and each individual brings their sensitivity to roles, hierarchies and stereotypes etc, it naturally may not lead to a psychologically safe environment. Group coaching, therefore, requires extra care to not only establish a working agreement at the start to create a safe environment for all participants, but also to maintain it throughout the coaching engagement as well. This building block is foundational in nature, as other building blocks rely on this to be present for them to function.
Challenges
Learning how to coach groups isn’t a trivial matter. The first challenge that coaches would face, from my experience, is defining the boundaries of what constitutes a system (and who the members in that system are). Take an example of a 50-person strong marketing department. Since decisions are made by the three senior leadership team members of the department, are those three people the system itself? Or should a dozen-odd handpicked people from the department be considered the system? How about coaching all 50 people together as a system? Unfortunately, there is no one correct answer here. It depends on the coaching problem at hand and the context. Even though coaching 50 people (or more) as a group together seems scary and unrealistic, there are techniques and skills of group coaching that make it possible, and in fact very worthwhile.
Another important thing to keep in mind is that as a coach, group coaching demands increased self-awareness and the ability to work with ambiguity, letting go and trusting the system’s ability of self-healing more than ever before. An individual human is complex in itself; bringing a group of individuals together increases the complexity exponentially. The possible permutations of what can happen in such interactions is hardly predictable. Creating and keeping the space psychologically safe makes things even more interesting. As a coach, getting comfortable with the complexity and ambiguity to the point that these thoughts are not distracting you from focusing on the coachees, comes with experience and practice. I recommend practising with coaching dojos and setting up a community of practice around it with fellow practitioners. If you are already working with a team, try one of the techniques from group coaching (e.g. LandsWork) with that team with their consent and build your coaching muscle and confidence along the way.
Skills Needed
Though these challenges are real and the learning curve is steep, it’s not an insurmountable journey. Before diving deep into relationship or group coaching, my recommendation is to ensure proficiency in coaching competencies (for example from ICF Core Competencies) as a first port of call. Oftentimes I have seen people use “coaching” to describe mentoring and/or teaching. The Agile Coach Competency Framework from Lyssa Adkins and Michael Spayd is also worth looking at if you are in the world of agile coaching. With prerequisites covered, the additional skill required by coaches to work with teams and relationships is Relationship System Intelligence (RSI), which is different from emotional and social intelligence.
Emotional Intelligence (EQ) teaches us self-awareness and an understanding of our emotional state/response etc. Social Intelligence (SI), on the other hand, teaches us the ability to understand and act on the feelings, thoughts, and behaviours of other people. The RSI, the final frontier if you will, builds on top of EQ and SI and adds the ability to understand the emotional experience of the intangible entity that I have mentioned earlier. Having the RSI means that as a coach, you have the ability to see past the individuals and see the intangible entity, the system itself. You pay attention to all voices in the system, even the unpopular ones. You are able to read the emotional climate of the system/team and are able to play it back without judgement. There is a lot more that can be added here, but the above is a very decent start and would get you going with coaching groups, teams and relationships.
Benefits
As briefly alluded to earlier, individual coaching has its benefits and its place, but group coaching offers benefits above and beyond individual coaching. There are two main drivers here.
Firstly, humans behave differently in different systems/teams. A person labelled as laid-back (read lazy) at work can be the driving force in another context. An extremely obsessed person at work can be the most easy going person among their mates. Therefore, it is important that apart from the individuals, enough attention is paid to the interactions between the individuals as well. This is the intangible entity between the individuals that I mentioned earlier.
Secondly, many of us have experienced being in an amazing team and we know that none of those teams were that amazing the day they were formed. Rather, these teams have worked hard to become amazing.
Let’s combine these two drivers together and we are looking at teams/groups full of unrealised potential. These teams can get a lot better in terms of how they are working together as a system and how productive they are. In a world of increasing complexity and high innovation demands, there is little additional reason we need to suggest that working on teams as a whole has its benefits. Please note that the team here can be the senior executive leadership team of an organisation, a team of developers working on a product, siblings in a family business or any other group of people forming a system. The more complex the relationship, the more likely it is dependent on the interactions between the individuals then the individuals themselves.
Group Coaching Scenario: Lack of Alignment
Let’s look at a group coaching scenario -a lack of alignment. As odd as it sounds, it is a common symptom in many groups/teams. Alignment is one of the fundamental aspects of a team and a lack of alignment will affect all facets of a team’s working; from individual mindsets to team culture, and from individual actions to team policies and structures. In such teams, even trivial things can become a multi–way tug of war, where each team member is rooting for their own goals and has their own reasons.
The problem is only aggravated if we zoom out of the individual teams and look at teams at the organisation level, e.g. team of teams, business units as teams, executive leadership teams, etc. A quick look at the state of agile report would tell us that even though “lack of alignment” isn’t quoted as a one of the challenges directly, it is a factor in more than a few of the challenges mentioned. Clashing cultures, inconsistent processes and resistance to change are the top three challenges in the report, and all three unfortunately stem from lack of alignment. The untapped potential and low rate of successes, either at the team level or the organisation level, is what makes pursuing alignment so important and coaching groups for alignment the tool that you need as a coach in this day and age.
Group Coaching Technique: Landswork
LandsWork is a group coaching technique that helps teams (product team, executive leadership team, etc.) create alignment when there are different opinions and perspectives on any topic. It comes from the organisation and relationship system coaching (ORSC) body of knowledge. The idea of the LandsWork is to use physical space, travelling metaphor and principles of relationship system intelligence to raise the awareness of the participants on the topic that the team isn’t aligned on. The system that emerges, from the raised level of awareness of all the participants, builds the alignment needed. It is a facilitated workshop that can be run with a team/system of any kind either in person or remotely. Examples are, a product delivery team of 7 people discussing a pair-programming approach, a 30-member strong middle management of an organisation discussing best investment amidst recent budget cuts, or the 12-person executive leadership team discussing life after the merger of the two organisations.
I ran a simulation workshop based on LandsWork at Lean Agile Scotland 2022 to demonstrate the technique in practice. In this workshop, the participants role played one of the three roles: change agents, sponsors, and members of the organisation. I started with creating a social agreement to ensure a psychologically safe environment and as an enabler to hear all opinions, no matter how unusual.
First, I divided the floor space into three sections, one for each role and asked participants to go to their section and imagine this is the land that they belong to. I extended the land metaphor by asking people to think about the climate, terrain and traditions of the land and then share that with everyone. Next, I asked each group to visit the other land as a visitor and experience the climate, terrain and traditions of the land they are visiting. I asked them to travel without any baggage from their own land so that they could truly experience the land that they were visiting without preconceived notions holding them back. Once everyone visited everyone else’s land, I asked all participants to move to a new area in the room and create a new, shared, land for all of them. I asked them to use the experiences of visiting all the individual lands to enrich the new shared land with empathy and understanding of others’ point of view. Lastly, from that shared land, participants were able to create action items to bridge the gap in alignment.
The participants saw the use of social agreement, physical space, the use of metaphors and facilitation as helping them see the perspective of other people in the system. Since they participated in the role play, they experienced the effectiveness first hand and left the room eager to explore more about group coaching.
Further Information
For those of us more geared towards reading books, the work of Amy Mindell and Arnold Mindell is worth looking at if you want to explore the topic further. The resource section of the CRR website is full of useful content as well and should be bookmarked. Additionally, look for a meetup group (for example the ORSC Australia Community meetup group) that runs simulations, dojos or discusses/practices topics around group coaching. Lastly, if you are interested in dipping your toes into it all guns blazing, in-person training options are also available from CRR in your region.
In today’s complex world, coaching has become a mainstream approach for individuals to seek growth. The group coaching has expanded the vistas of possibilities and has provided us, the coaches, with the ability to realise the unfulfilled potential in groups. Despite the additional challenges, complexity and the need to acquire knowledge & experience to excel at group coaching, the benefits far outweigh the effort and is a journey worth taking. I find group coaching fascinating and being a journeyman myself, I would recommend all the coaches out there to explore it. If you do, please reach out, share your experiences and let’s learn from each other and do our part in helping this world create awesome and productive teams/groups.
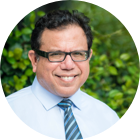
MMS • Sergio De Simone
Article originally posted on InfoQ. Visit InfoQ
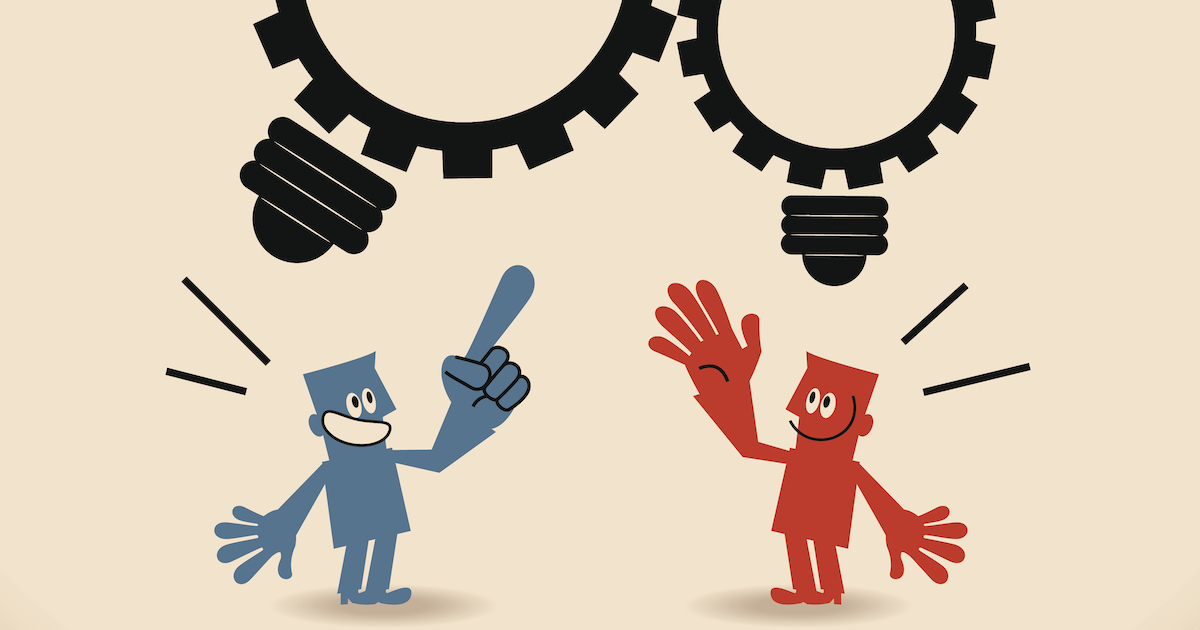
Several years in the making, OCaml 5 introduces runtime support for shared memory parallelism and effect handlers, which are the basis for exception handling, concurrency, async I/O, and more.
Algebraic effect handlers are a first-class abstraction aimed to represent and manipulate control flow in a program. In its most immediate form, effect handlers provide a restartable exception mechanism that can be used to recover from errors. Thanks to their flexibility, they provide also the basis for other abstractions such as generators, async I/O, concurrency, and more.
Similar to exceptions, effects are (typed) values that you instantiate through their constructors. Unlike exceptions, they can be performed and return a value. This is how you would declare a Conversion_failure
algebraic effect that takes a string as a parameter and returns an integer upon execution:
type _ Effect.t += Conversion_failure : string -> int Effect.t
Effects are associated with effect handlers, which are records with three fields: a retc
function that takes the result of a completed computation; an exnc
function that is called when an exception is thrown; and an effc
generic function that handles the effect. For example, for the previously declared Conversion_failure
effect, we could write the following handler that intercepts the exception, prints some debugging information, then let the program continue (alternatively, it could discontinue it):
match_with sum_up r
{ effc = (fun (type c) (eff: c Effect.t) ->
match eff with
| Conversion_failure s -> Some (fun (k: (c,_) continuation) ->
Printf.fprintf stderr "Conversion failure "%s"n%!" s;
continue k 0)
| _ -> None
)
}
As mentioned, handling exceptions is only one possible use of effects, but since effects can be stored as values in a program to be used at some later moment, they also enable the implementation of generators, async/await, coroutines, and so on. For example, this is a simplified view of how effects can be used to create coroutines:
type _ Effect.t += Async : (unit -> 'a) -> unit Effect.t
| Yield : unit Effect.t
let rec run : 'a. (unit -> 'a) -> unit =
fun main ->
match_with main ()
{ retc = (fun _ -> dequeue ());
exnc = (fun e -> raise e);
effc = (fun (type b) (eff: b Effect.t) ->
match eff with
| Async f -> Some (fun (k: (b, _) continuation) ->
enqueue (continue k);
run f
)
| Yield -> Some (fun k ->
enqueue (continue k);
dequeue ()
)
| _ -> None
)}
When a task is completed, retc
is executed, which dequeues it and lets thus the next task ready for execution. If an Async f
effect is returned, the task is enqueued and f
is performed. Finally, for a Yield
effect, the next task is enqueued and another is dequeued from the scheduler.
While algebraic effects are useful for concurrency, domains
are at the heart of Multicore OCaml, an OCaml extension with native support for parallelism across multiple cores using shared-memory. A domain is in fact the basic unit of parallelism, providing two fundamental primitives, spawn
and join
:
let square n = n * n
let x = 5
let y = 10
let _ =
let d = Domain.spawn (fun _ -> square x) in
let sy = square y in
let sx = Domain.join d in
Printf.printf "x = %d, y = %dn" sx sy
Multicore OCaml builds more advanced abstractions on top of spawn
and join
, such as tasks and channels, implemented through the Domainslib library.
Tasks are essentially a way to parallelize work by only spawning one single domain. Since spawning and joining a domain is an expensive operation, using tasks is a more effective way to scale computation across multiple cores, where you can execute multiple tasks in parallel with async
and then wait for their completion using await
.
Channels, on the other hand, are a mechanism to allow domains to communicate with one another, as their name implies. Channels are blocking, meaning that if a domain attempts to receive a message from a channel but the message is not ready, the domain may block. Likewise, a send operation may also cause a domain to block until another domain will receive that message if the channel has reached the maximum number of messages it can hold.
Currently, one limitation of the OCaml 5 compiler is it only supports x86-64 and arm64 architectures on Linux, BSD, macOS, and Windows/migw64. The OCaml team is working to restore support for the rest of traditionally supported architectures during 2023.
Support for concurrency and parallelism are not the only new features in OCaml 5, which also includes improvements to the runtime system, the standard library, and several optimizations. Do not miss the official release notes for the full detail.