Month: February 2023
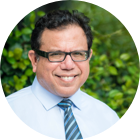
MMS • Daniel Dominguez
Article originally posted on InfoQ. Visit InfoQ
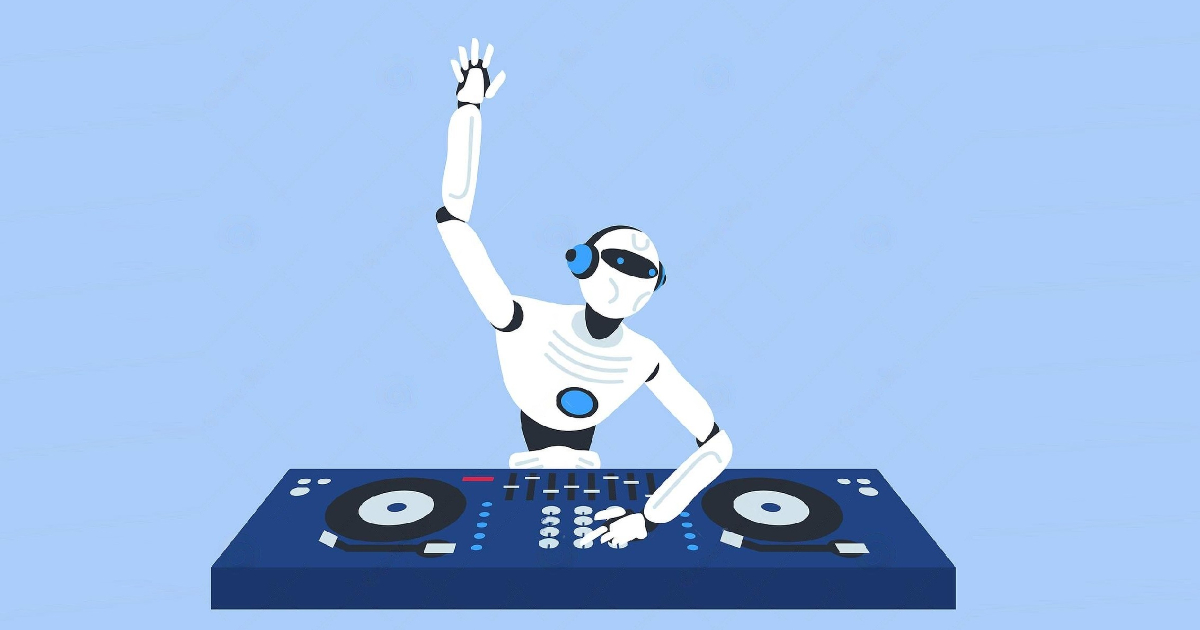
Google researchers have introduced MusicLM, an AI model that can generate high-fidelity music from text. MusicLM creates music at a constant 24 kHz throughout a number of minutes by modeling the conditional music generating process as a hierarchical sequence-to-sequence modeling problem.
According to the research paper, MusicLM was trained on a dataset of 280,000 hours of music to produce songs that make sense for complex descriptions. The researchers also claim their model outperforms previous systems both in audio quality and adherence to the text description.
MusicLM samples, includes five-minute pieces produced from only one or two words like melodic techno, as well as 30-second samples that sound like entire songs and are formed from paragraph-long descriptions that prescribe a genre, vibe, and even specific instruments.
MusicLM is also capable of transforming a collection of sequentially written descriptions into a musical story or narrative built on existing melodies, whether they are whistled, hummed, sung, or played on an instrument.
AI-generated music has a long history and has been credited with writing hit songs, and enhancing live performances. In a more recent version, written prompts are converted into spectrograms and music using the AI picture generating engine Stable Diffusion.
Contrary to text-to-image machine learning, where it is claimed that large datasets have contributed significantly to recent advancements, there are hurdles for AI music related to the absence of coupled audio and text data. For instance, Stable Diffusion and OpenAI’s DALL-E tool have both sparked a surge in interest from the general public. Also the fact that music is structured along a temporal dimension presents another difficulty in AI music generation. Consequently, compared to using a description for a still image, it is far more difficult to convey the intention of a music track using simple text.
Google is being more cautious with MusicLM than some of its competitors may be with comparable technology, as it has been with prior excursions into this form of AI. The article ends with the statement, “We have no plans to disclose models at this point”.
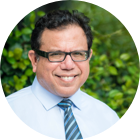
MMS • Johan Janssen
Article originally posted on InfoQ. Visit InfoQ
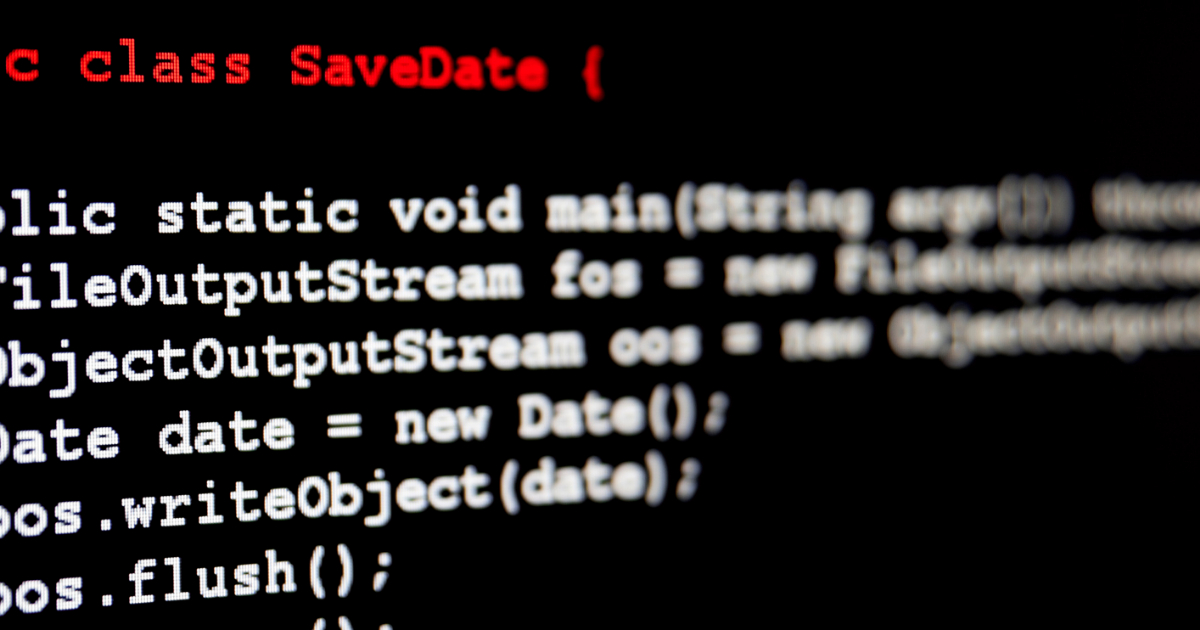
The fifth generation of the Reduced Instruction Set Computer (RISC) architecture, called RISC-V, was introduced in 2010. RISC is an open standard instruction set for processors, available for free, under open source licenses. GraalVM may now be used to compile and run native images on RISC-V machines.
GraalVM, by default, uses the Graal compiler to create machine code. The hardest part of supporting new architectures is implementing the Graal compiler for those new architectures. Alternatively, the LLVM backend may be used, which allows users to support CPU architectures, currently unsupported by the GraalVM Native Image. LLVM contains several compiler and toolchain technologies which may be used as a backend for any instruction set architecture. LLVM is written in C++ and supports various instruction sets such as x86-64, ARM, WebAssembly and RISC-V.
The LLVM backend for GraalVM is a separate component for native images and may be installed with the GraalVM updater:
gu install native-image-llvm-backend
The LLVM backend may be enabled by supplying the -H:CompilerBackend=llvm
argument to the native-image
command:
$JAVA_HOME/bin/native-image -H:CompilerBackend=llvm
Now the LLVM backend uses the LLVM compiler together with the LLVM intermediate representation to create native executables.
Application binaries may be built directly on a RISC-V machine:
$JAVA_HOME/bin/native-image MyFirstRISCVApplication -H:CompilerBackend=llvm
--add-exports=jdk.internal.vm.ci/jdk.vm.ci.riscv64=org.graalvm.nativeimage.builder
Alternatively, the binary may be cross-built on another architecture as Linux runs relatively slow on RISC-V machines. Sacha Coppey, working on GraalVM for Oracle Labs, provided instructions on how to set up the environment with the RISC-V Toolchain, CAPCaches and Static Libraries, in order to cross build the application on another architecture.
An example Micronaut application was used to measure the performance difference between running on a JVM and running as a Native Image on RISC-V. The startup time on the JVM was about 9300 milliseconds, compared to about 300 milliseconds for a Native Image.
The Native Image LLVM backend for RISC-V based on Linux may be found in the GraalVM CE dev builds. The LLVM backend supports most features of the other backends, but lacks support for macOS and other operating systems. There’s also some room for future optimizations by using the RISC-V specific features. The implementation for RISC-V took just six months, which led the team to consider evaluating other environments such as WebAssembly.
GraalVM published the complete article online and feedback may be provided via Twitter, Mastodon, GitHub or Slack.