Month: February 2023
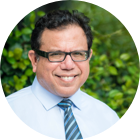
MMS • RSS
Posted on nosqlgooglealerts. Visit nosqlgooglealerts
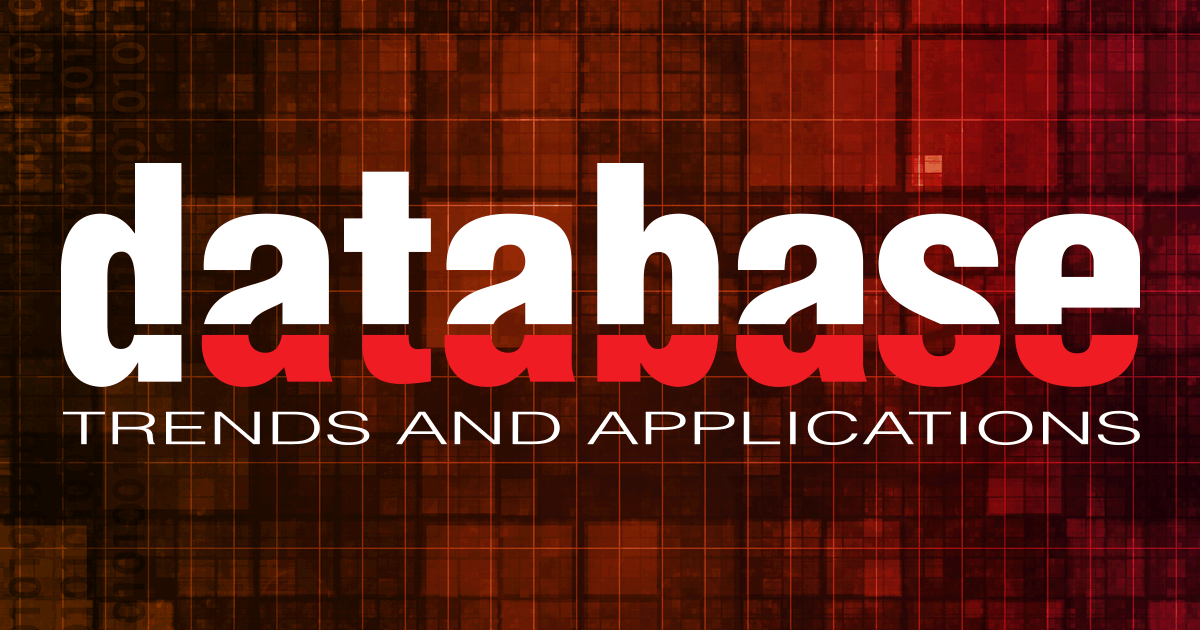
It’s time to submit nominations for the annual Database Trends and Applications Readers’ Choice Awards, a competition in which the winning information management solutions, products, and services are selected by you, the users.
The nominations period is open through March 8, 2023, followed by actual voting on the products nominated by our readers.
Votes can only be cast for nominated products, so be sure to make your nominations now!
Winners will be showcased in a special section on the DBTA website and in the August 2023 edition of Database Trends and Applications magazine.
This year’s nominating categories are as follows:
BEST AI SOLUTION
BEST CDC SOLUTION
BEST CLOUD DATABASE
BEST CLOUD SOLUTION
BEST DATA ANALYTICS SOLUTION
BEST DATA GOVERNANCE SOLUTION
BEST DATA INTEGRATION SOLUTION
BEST DATA LAKEHOUSE SOLUTION
BEST DATA MODELING SOLUTION
BEST DATA PLATFORM
BEST DATA FABRIC SOLUTION
BEST DATA MESH SOLUTION
BEST DATA QUALITY SOLUTION
BEST DATA SECURITY SOLUTION
BEST DATA STORAGE SOLUTION
BEST DATA VIRTUALIZATION SOLUTION
BEST DATA WAREHOUSE SOLUTION
BEST DATABASE BACKUP SOLUTION
BEST DATABASE DEVELOPMENT SOLUTION
BEST DATABASE OVERALL
BEST DATABASE PERFORMANCE SOLUTION
BEST TIME-SERIES DATABASE
BEST DATAOPS SOLUTION
BEST DBA SOLUTION
BEST GRAPH DATABASE
BEST IoT SOLUTION
BEST MULTIVALUE DATABASE
BEST NOSQL DATABASE
BEST STREAMING SOLUTION
To nominate products, go to www.dbta.com/Readers-Choice-Awards.
DataStax launches Astra Block – a new service aimed at helping developers more easily …
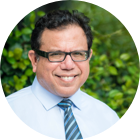
MMS • RSS
Posted on nosqlgooglealerts. Visit nosqlgooglealerts
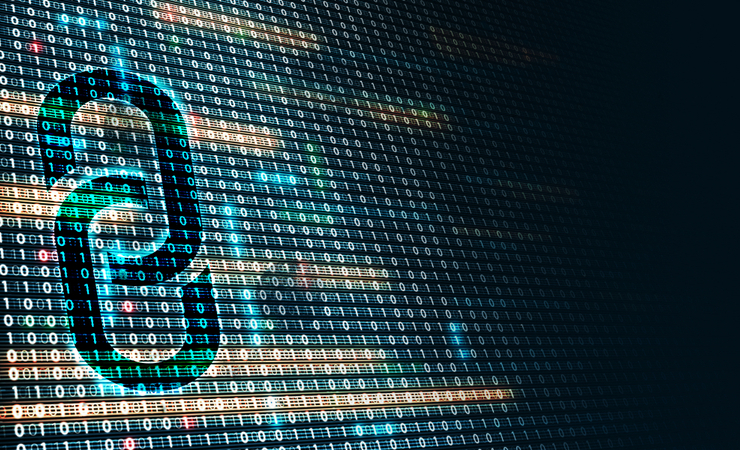
DataStax is expanding its use case of event streaming data, via its Astra Cloud service, into the world of Web3 technologies – where it sees an opportunity to help developers more easily access data in real time from blockchain ledgers. The central premise to Astra Block, the service announced today, is that any change to a ledger is essentially an ‘event’ that could easily be captured via DataStax’s Astra database, which uses Cassandra, to help developers build Web3 applications.
Web3 has been described as the ‘next phase of the Internet’, where distributed ledger technology provides the potential to decentralize data across the web, providing increased data security, scalability and privacy for users. The reality, however, has been somewhat different, as proponents of the technology have been caught up in money-making schemes that include crypto currencies and NFTs.
Not to mention the energy consumption required in ensuring that every node in a blockchain system is informed about every transaction in the chain, whenever a change is made, resulting in an exponential increase in data generation.
However, it’s still early days in the development and there are real benefits in the use of Web3 applications, particularly around securing identity and being able to give provenance of ownership. Simply put, it’s hard to see how this phase of web development will play out – and DataStax sees an opportunity to provide simplified tooling to developers that perhaps are struggling to efficiently get access to data when needed.
DataStax says that Astra Block is making the “previously daunting task” of cloning the entire blockchain dataset possible with the “click of a button, leveraging the real-time speed, massive scale and zero downtime provided by Cassandra”.
It adds that the new service allows advanced querying and real-time analytics to be run at sub-second speeds, enabling developers to build new blockchain-based functionalities into their applications.
For example, developers can build applications with the capability to analyze any transaction from the entire blockchain history, including crypto or NFTs. Astra DB’s CDC and streaming features, DataStax says, ensure that the clone of the chain is updated in real time, as new blocks are mined.
Ed Anuff, DataStax’s Chief Product Officer, explains that what happens in practice for developers currently working with Web3 technologies, is an onerous series of activities. He explains:
What you do right now, if you’re a developer, is you need to firstly operate your own node, which is essentially a server instance. You need at least one of these – that is going and capturing the data from the blockchain as it’s being updated.
Then you need to be able to go and parse that data. Remember, as ‘blockchain’ would imply, these are blocks of data, large blocks of data. But typically, what you’re interested in is a specific transaction within that block. So you would need to go and parse that out. And then you need to load it into a database, in a format that was designed for you to be able to query it. And then you’ve got to continuously update that.
Those basic things end up being challenging. And so what we’ve done is we’ve eliminated the need to do all those steps. You get a constant stream of the data, we operate the blockchain nodes, we’re getting the data updates on the blockchain as they’re happening.
We’re taking that data and parsing it out in a very efficient way, because as a company that operates the database, we know a lot about how to store that data in the most performant way and most efficient way, so that you as a developer querying that data, you’ll get it very, very quickly, with minimal overhead.
The opportunity
DataStax is currently pitching itself as the ‘data streaming platform of choice for the enterprise’, with its Astra Cloud service being central to that. In a recent conversation with CEO Chet Kapoor, he said:
It’s all about real time. People sometimes get into ‘is it about nanoseconds and microseconds?’. No, it’s about real time. Whether it’s web apps or whether it’s mobile apps, it doesn’t matter. It’s about apps and it’s about doing things in real time. And you cannot do real time without streaming.
And this is central to DataStax’s thinking around Web3, in that it sees the updates to distributed ledgers and the data from blockchains needed to fuel web applications as ‘event based data’ (or streaming data). Anuff builds on this and says:
What it means is that if you want to build an application that’s constantly checking the blockchain, to see whether something has happened, which is a very common use case, it’s trivial to do [with Astra Block].
You don’t have to operate your own infrastructure for that, you just simply go and log into Astra, say that you want to track Ethereum, or a specific NFT, and then you set the conditions to go and say, for example, ‘whenever this changes hands, I want to get an event sent to my application, that I, the developer, will do something with’.
We abstract all of that out, so that all the hard work of interfacing with the data is done for us.
On DataStax being positioned to take advantage of this, he continues:
The interesting thing was that we looked at the blockchain ledgers, and the immediate thing that we saw – and it wasn’t a unique insight, but certainly we were uniquely poised to be able to take advantage of it – is that these are real time data streams. They are event based data.
What that meant was that when we looked at how you might use this data, our Cassandra database was uniquely suited for that. And this is something that we actually initially discovered because we had a number of developers and customers coming to us, using our Astra Cloud service.
The challenge of dealing with this data is that you need to be able to store it, you need to be able to index it so that you can query it and search it – but being able to keep up with the real time updates. Because when you look at the activity on these blockchains, for example, on Ethereum, there’s a constant set of updates that’s happening.
It’s a very large data set, it’s being updated in a distributed way by applications and transactions happening all around the world.
And so it was a fairly straight line from that, going and saying, okay, if you’re going to build applications on top of this, it would make sense for us to go and take a look at how to go and capture that data as it happens into Astra and provide the tools for people to be able to go and do very powerful queries against this data.
Anuff says that Astra Block has been under development for a year or so and was created in response to developers telling DataStax that they were using Cassandra to work with their Web3 applications.
However, Anuff does admit that it’s early days for this area of development, and won’t initially be a huge portion of DataStax’s business – but the aim is to get in early and offer the support to developers that are experimenting and building. He says:
There’s lots of things that are happening, some of them good, some of them bad. But what’s been really interesting all along is that it has been the developers that have been building new applications, finding new use cases, and if they do that, it pushes us to find different ways to make this data available. And so that’s where Astra Block comes from.
We have some blockchain customers that are doing significant amounts of our platform. I know at least one of them is spending seven figures with us annually. These projects, blockchain applications, do use a significant amount of data.
Is it going to be a large percentage of our overall business? No, but it has a specific emerging use case that we’re able to extend into and we’re able to, to accelerate, and add some value for developers. It’s going to be a small percentage of our business, but it’s a use case that we’ve seen as being important.
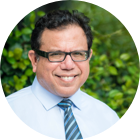
MMS • RSS
Posted on nosqlgooglealerts. Visit nosqlgooglealerts
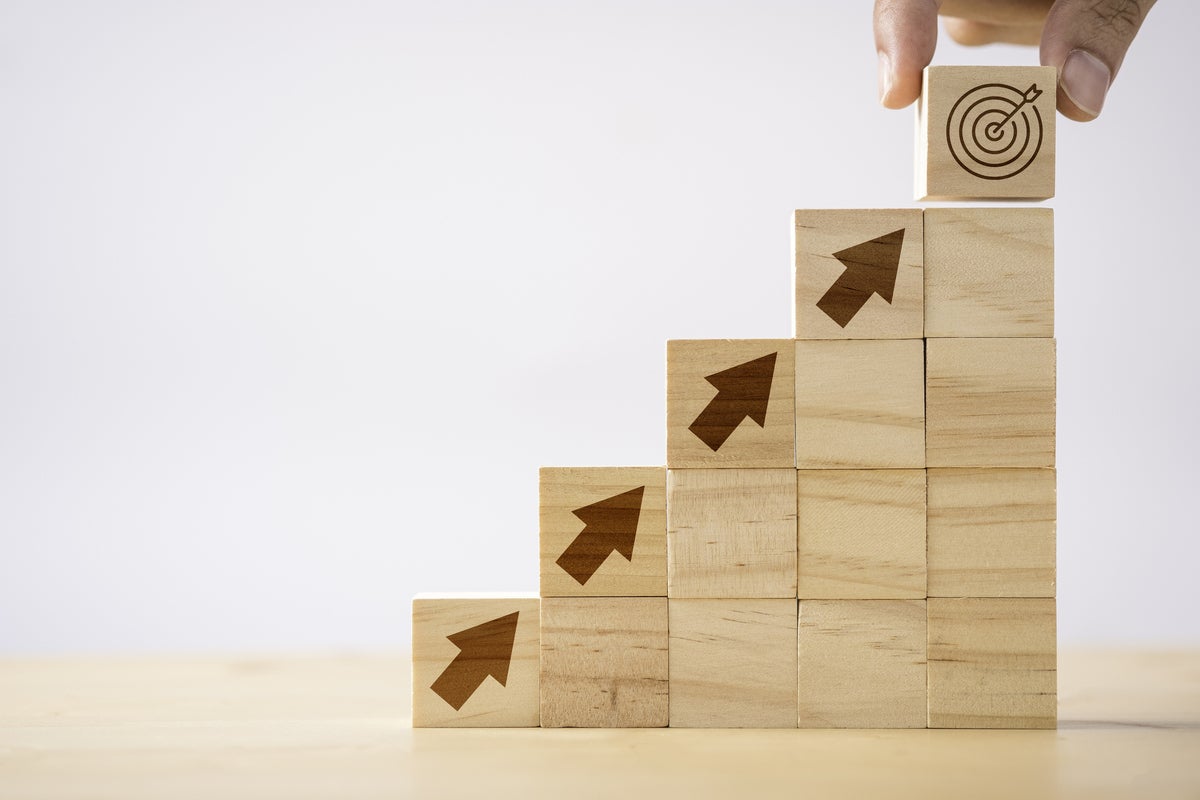
ScyllaDB, the company behind the ScyllaDB database for data-intensive applications that require high performance and low latency, announced that it is achieving staggering performance results on the new AWS I4i instances powered by the 3rd Gen Intel Xeon Scalable processors with an all-core turbo frequency of 3.5 GHz.
Recent benchmarks compared ScyllaDB performance on AWS i4i.16xlarge vs. i3.16xlarge. The results demonstrated up to 2.7x higher throughput per vCPU on the 3rd Gen Intel Xeon Scalable processor I4i instances compared to I3 instances for reads. With an even mix of reads and writes, tests achieved 2.2x higher throughput per vCPU on the 3rd Gen Intel Xeon Scalable processor I4i series, with a 40% reduction in average latency versus I3 instances.
The Intel 3rd Gen Intel Xeon Scalable processor is based on a balanced, efficient architecture that increases core performance, memory, and I/O bandwidth to accelerate diverse workloads from the data center to the intelligent edge. These powerful new processors are the next generation of the Intel Xeon E5-2686 v4 Broadwell processor operating at 2.3 GHz with bursts up to 3 GHz, which are used in i3.metal instances.
ScyllaDB is uniquely designed to capitalize on continuing hardware innovations like the latest Intel processors. Its close-to-the-metal, shared nothing design delivers greater performance for a fraction of the cost of DynamoDB, Apache Cassandra, MongoDB, and Google Bigtable.
While other NoSQL databases are effectively insulated from the underlying hardware, ScyllaDB fully capitalizes on processor, memory, network, and storage innovation to maximize performance and use less infrastructure.
These two companies, both recognized for pushing the limits of innovation, are expanding their technical relationship through the Intel Disruptor Initiative. ScyllaDB joining this program is a significant milestone. Intel supports its members by driving growth through technical enablement, allowing Disruptor Program innovators to accelerate their time-to-market with early access to hardware and software. In addition, Intel engineers work closely with the ScyllaDB team to ensure that future releases reach new levels of optimized performance.
“We architected ScyllaDB from the ground up to squeeze every ounce of power from hardware innovations like the new 3rd Gen Intel Xeon Scalable processor,” said Dor Laor, CEO and co-founder, ScyllaDB. “We’re both obsessed with performance, and we’re eager to work more closely with Intel to reach even higher levels of speed and efficiency.”
“ScyllaDB’s close-to-the-hardware design is uniquely poised to take full advantage of the hardware advancements that Intel is known for. It’s a perfect combination for performance-minded engineers,” said Arijit Bandyopadhyay – CTO Enterprise Analytics and AI, Head of Strategy – Cloud and Enterprise, Data Center Group, Intel Corporation.
To learn more, visit https://www.scylladb.com
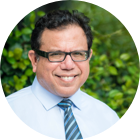
MMS • RSS
Posted on nosqlgooglealerts. Visit nosqlgooglealerts
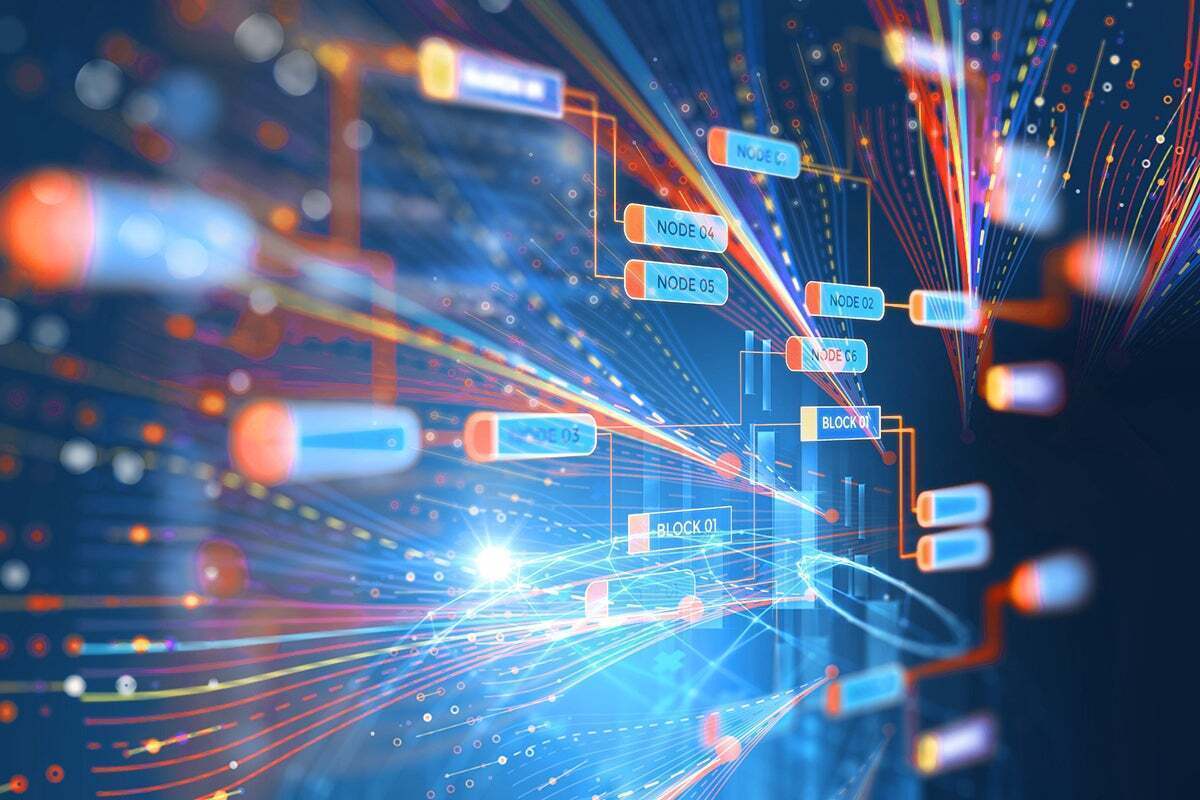
DataStax on Wednesday said that it was launching a new cloud-based service, dubbed Astra Block, to support building Web3 applications.
Web3 is a decentralized version of the internet where content is registered on blockchains, tokenized, or managed and accessed on peer-to-peer distributed networks.
Astra Block, which is based on the Ethereum blockchain that can be used to program smart contracts, will be made available as part of the company’s Astra DB NoSQL database-as-a-service (DBaaS), which is built on Apache Cassandra.
The new service can be used by developers to stream enhanced data from the Ethereum blockchain to build or scale Web3 experiences virtually on Astra DB, the company said.
Use cases include building applications to analyze any transaction within the blockchain history for insights, DataStax added.
Enterprises adoption of blockchain has grown grow over the years and market research firm Gartner estimates that at least 25% of enterprises will interact with customers via Web3 by 2025.
The data within Astra Block that is used to create applications is decoded, enhanced and stored in human-readable format, accessible via standard Cassandra Query Language (CQL) database queries, the company said.
Astra Block can decode and store the data used to create applications in human-readable format, and the data is accessible via standard Cassandra Query Language (CQL) database queries, the company said.
In addition, applications made using Astra Block can take advantage of Astra DB’s change data capture (CDC) and streaming features, DataStax added.
In June last year, the company made its Astra Streaming service generally available in order to help enterprises deal with the challenge of becoming cloud-native and finding efficiencies around their existing infrastructure.
A version of Astra Block that offers a 20GB partial blockchain data set can be accessed through the free tier of Astra DB. The paid tier of Astra DB — based on pay-as-you-go usage and standard Astra DB pricing – includes the ability to clone the entire blockchain, updated as new blocks are added. Depending on user demand, DataStax will expand Astra Block to other blockchains.
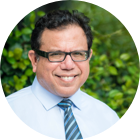
MMS • Sergio De Simone
Article originally posted on InfoQ. Visit InfoQ
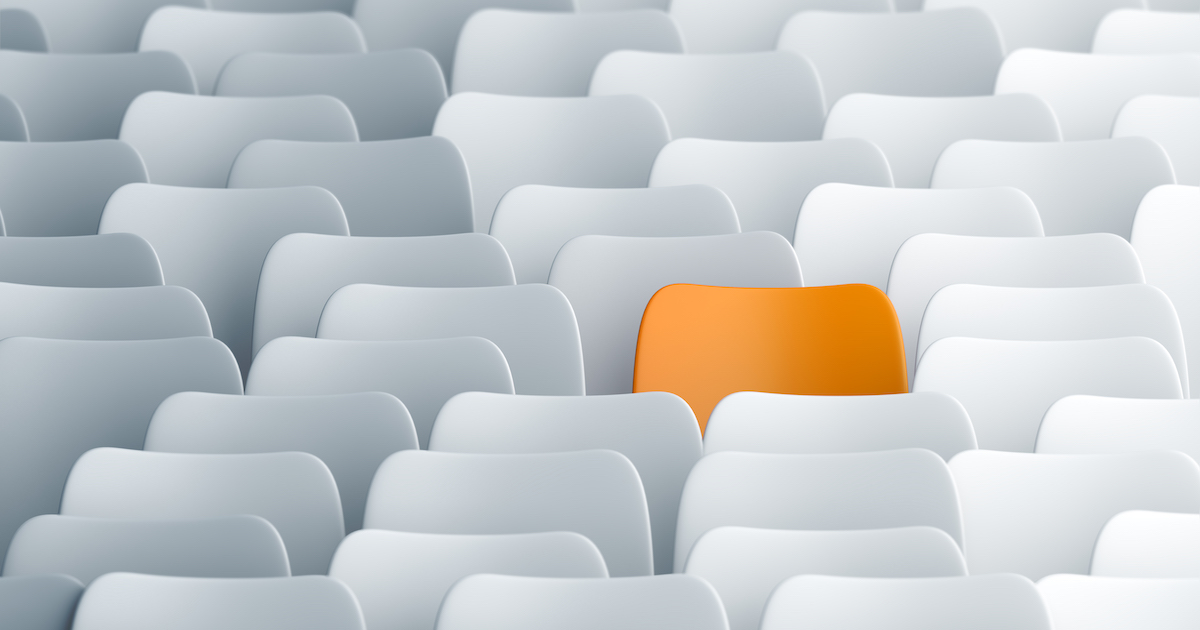
Facebook rewrote its iOS app in 2012 to take advantage of native performance and improve reliability and usability over its previous, HTML5-based cross-platform implementation. In the ten years since the rewrite, the app codebase has evolved non-stop to account for the introduction of new features, to circumvent SDK limitations, and to keep up with changes in the iOS platform, explains Facebook engineer Dustin Shahidehpour.
Two years after its native rewrite, Facebook’s app for iOS started to show reliability issues related to the use of Core Data. Core Data models are intrinsically mutable, says Shahidehpour, and this makes it hard to use them in a multithreaded app.
Ultimately, this design exacerbated the creation of nondeterministic code that was very difficult to debug or reproduce bugs. It was clear that this architecture was not sustainable and it was time to rethink it.
Facebook engineers implemented then ComponentKit, a React-inspired declarative framework to define UIs. ComponentKit used immutable data, which simplified reasoning about the code and provided a 50% performance improvement over the previous implementation. ComponentKit has been hugely successful within Facebook, where it still is the default choice to create iOS UIs.
In 2015, the Facebook app saw what Shahidehpour describes as a “feature explosion”, which had the net effect of degrading launch time up to the point where the app could risk being killed by iOS. This led to the effort of modularizing the codebase using dynamic libraries (dylibs) so that part of the code could be loaded lazily, thus reducing the number of tasks to execute before main
.
While the adoption of dylibs solved the issue with launch time, it introduced a different class of reliability problems, mostly related to the possibility of a runtime error when trying to access some code in a not-yet-loaded dylib. To fix this problem, Facebook engineers decided to leverage the build graph produced by Buck, their own build system.
Each ‘target’ lists all information needed to build it (dependencies, compiler flags, sources, etc.), and when ‘buck build’ is called, it builds all this information into a graph that can be queried.
Using that information, the app was able to create a mapping from classes and functions to dylibs and then automatically generate code to ensure a dylib was loaded into memory when some functions tried to access it.
This further led to the creation of a plugin system that made it possible to detect dependency graph-related errors at build-time instead of runtime.
It was not until 2020 that Facebook started using Swift in their mobile apps, prompted by a growing number of Swift-only API appearing in the iOS SDK. This represented a radical change from the previous stance of accessing SDK functionality only through some kind of wrapper. While motivated by the goal to improve developer efficiency, this approach was made more complicated by the lack of interoperability between Swift and C++. The solution to this was requiring that UI-related code should not contain any C++ so that engineers could use current and future Swift APIs from Apple, while C++ was reserved for infrastructure code.
Overall, the evolution of Facebook’s app for iOS shows a number of strategies that can be useful to overcome platform limitations and to adapt to the changing nature of requirements and of the underlying platform. Do not miss the original article if you are interested in the full detail.
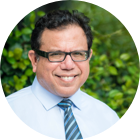
MMS • Steef-Jan Wiggers
Article originally posted on InfoQ. Visit InfoQ
Microsoft recently announced that Azure Durable Functions support for the new storage providers, Netherite and Microsoft SQL Server (MSSQL), is generally available.
With Azure Durable Functions, developers can write long-running, reliable, event-driven, and stateful logic on the serverless Azure Functions platform. In addition, these functions can have several storage providers, also called “backends,” for storing orchestration and entity runtime states. By default, new projects are configured to use the Azure Storage provider; however, a developer can choose another backend, including the now generally available Netherite and MSSQL.
Earlier, the company previewed the storage backends Netherite and MSSQL as new storage options allowing developers to run durable functions at a larger scale, with greater price-performance efficiency and more portability than the default Azure Storage configuration.
Microsoft Research developed the Netherite storage provider and uses Azure Event Hubs and the FASTER database technology on top of Azure Page Blobs to store state information. It provides better throughput performance achieved by representing states differently than Azure Storage – also visible through HelloCities5 benchmark tests the company conducted.
Source: https://microsoft.github.io/durabletask-netherite/#/throughput
The MSSQL provider was designed to fulfill enterprise needs, including the ability to decouple from the Azure cloud, and is compatible with both on-premises and cloud-hosted deployments of SQL Server, including Azure SQL Database, Edge devices, and Linux containers. In addition, the Azure Functions Core Tools and the Azure Arc App Service extension have been updated to support automatically configuring Durable Function apps on a Kubernetes cluster with the MSSQL KEDA scaler for elastic scale-out.
Davide Mauri, a principal product manager of Azure SQL DB at Microsoft, posted on LinkedIn:
#AzureSQL and #SQLServer can now be used to support Azure Durable Functions to scale above and beyond , anywhere you want to run them. On #Azure, On-Prem, or even in other clouds. Amazing!
With the availability of several backends, Microsoft recommends the default Azure storage provider for minimal setup and lowest minimum cost, Netherite for maximum throughput, and MSSQL to run anywhere.
Lastly, more detailed documentation on architecture, scaling, and performance is available on the github.io pages for Netherite and MSSQL.
Article: Hard-Won Lessons from the Trenches: Failure Modes of Platform Engineering — And How to Avoid Them
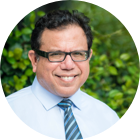
MMS • Aaron Erickson
Article originally posted on InfoQ. Visit InfoQ
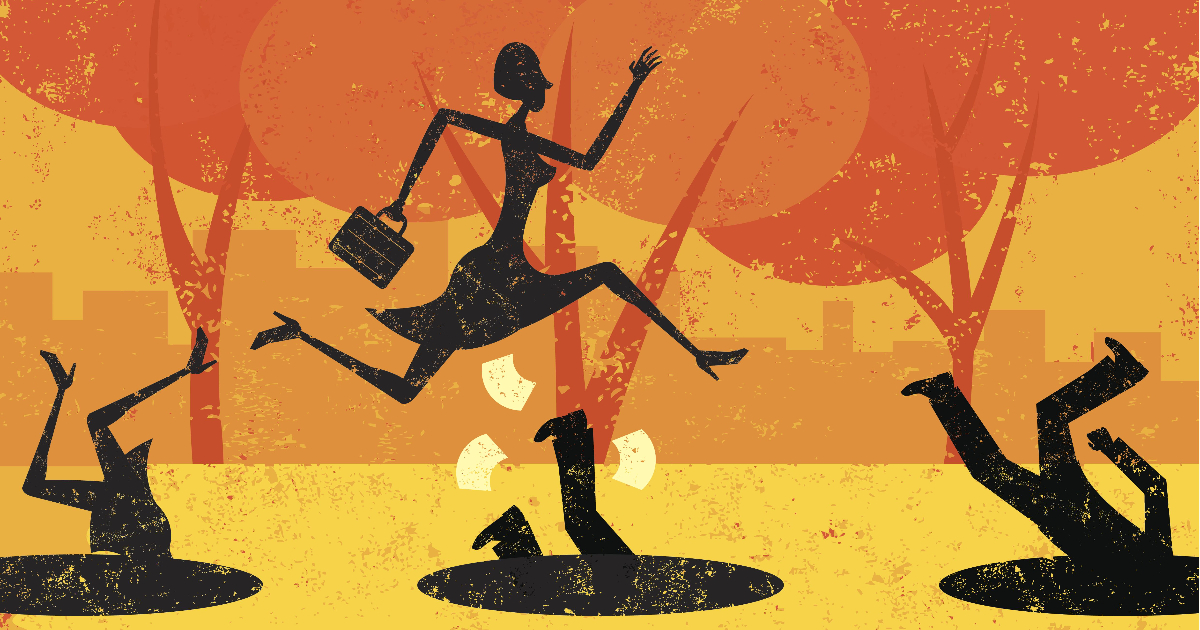
Key Takeaways
- Forcing people to use your platform can lead to “malicious compliance.” Every time the platform fails them, however, they’ll blame it — and your platform team.
- If your IDP forces people to accept unworkable practices, it won’t succeed.
- Don’t sacrifice security to implement your platform faster. When things go wrong, your biggest fans will become your worst detractors.
- A strong focus on the costs and budget of your platform will help ensure the business continues investing the next time budgets are under review.
- Start small and iterate. You probably don’t have the budget and resources to reinvent Heroku.
It’s impossible to dispute the value platform engineering brings businesses as a whole. Not only did Gartner name platform engineering as one of its top 10 tech trends for 2023, but it also included it in its well-known hype cycle, making a strong case for how companies can improve developer experiences by building internal developer platforms (IDPs).
Even more relevant, the platform engineering community is rapidly growing. In other words, this is not just about decisions made in some boardroom. Developers who use internal developer platforms have gained an indisputable appreciation for their advantages on the front line, like increased developer productivity, better product outcomes, better developer experiences, and lower costs.
In today’s world, where everyone is doing something in the cloud and workforces are clamoring for more distributed opportunities, IDPs have gained a reputation as competitive necessities that dramatically improve developer quality of life.
Unfortunately, this also means that expectations are sky-high — so anything short of inventing a new Heroku (but of course without any of its limitations) might be seen as a failure.
It’s critical to double down on anything and everything that increases developer productivity. But before you start, it’s all about knowing what not to do: Understanding where the pitfalls lie makes dodging them easier. So here are some of my takeaways from creating IDPs for Salesforce and other companies, seeing countless efforts crash and burn, and watching platform engineering evolve.
The Failure Modes That Plague Platform Engineering Efforts
There are numerous ways platform engineering projects can fail — including some that’ll hamstring them before ever they reach maturity. Watch out for these wrongheaded modalities as you create your IDP:
The Build It and They Will Come Fallacy
This is a big logical error. Know what you’re doing by assuming people will use your platform just because you’ve built it? Say it with me: You’re setting yourself up for failure.
Sure, your platform may be better than the already-broken systems you’re trying to improve on. That doesn’t mean it won’t have its own pain points, like wasting time or failing to serve developers’ needs.
The best-case outcome in such a scenario is that you’ll foster discontent. Users will just grumble about the new problems you’ve saddled them with instead of complaining about the old ones. This is not an improvement.
What’s more likely is that you’ll engender an environment of “malicious compliance.” People will use the platform because that’s what they’ve been told to do. Every time it fails them, however, they’ll blame it — and your platform team. Way to go, scapegoat!
This does far more than just harm your career. It also prevents future adoption, not to mention poisons the well of your corporate culture.
Fortunately, there’s a straightforward solution. Be a good, dedicated product manager, and get closer to your customer — preferably well before you start building anything.
Projects that focus on what the platform team thinks the end product should include (instead of asking the users what they really need!) get irrecoverably bogged down. In other words, understanding your typical user personas in advance should be your guiding star.
Remember: Improving the developer experience is a crucial goal. Solicit regular feedback so that you understand what to build and how to improve. By adopting a user-centric product management approach, you’ll naturally promote usage by solving important problems.
The One True Way Falsehood
Building IDPs means building golden paths for developers. The problem is that some would-be golden paths don’t quite hold up to scrutiny. There’s no “one true way” to develop software, and if your IDP forces people to accept unworkable practices, it won’t succeed.
At one point or another, devs who use overly opinionated tooling will come up against edge cases you didn’t anticipate — and being good devs, they’ll start looking more closely to devise workarounds. Then, it’s only a matter of time before they’ll realize: “Hey! These are just a bunch of bricks somebody’s painted yellow!”
Your golden paths need to accommodate people’s tendency to go offroad — and be sufficiently adaptive to match. For example, your IDP can still succeed without supporting queueing technologies like managed Kafka or distributed computation frameworks like Hadoop out of the box. But your IDP should be architected in a way that allows all integrations you can think of, so you would be able to extend later. The architecture of the platform must already take into account that developers will want to self-serve future technologies you even haven’t heard about.
The People Pleaser Platform
While the “one true way” antipattern leads to certain failure, so does the opposite — building a “people pleaser” platform. After all, you’ll never make everyone happy, and trying will likely make things worse.
Not every feature request carries equal weight. Imagine one of your teams wanted to use some cutting-edge experimental technology. Integrating said tooling might make them happy, but it could also result in widespread instability — not quite a desirable platform characteristic.
In other cases, you’ll simply oversell what you can do and inevitably end up disappointing. For instance, you may have lots of teams that use diverse tech stacks. By saying “sure, we can support them all, no problem,” you’ve condemned yourself to a kind of Sisyphean torture.
Remember that you can only do so much. More importantly, understand that the thinner you spread your team, the more the quality of your work will suffer. Even if you working with massive amounts of funding and resources, you can’t support every conceivable combination of technologies — let alone do so well!
Instead of trying to please the entire organization, start with an MVP and work closely with a lighthouse team that has an early adopters mindset and is willing to give continuous feedback. By helping you guide improvements and enhance production efficiency, your lighthouse project will let you satisfy needs as they arise. Of course, you’ll still need to prioritize ruthlessly as you go, but that’s a lot easier when you aren’t in the habit of overextending. Once you gained stability, you can think of rolling out the platform to more teams before you expose it to the entire organization.
The House of Cards Architecture
You might be creating a platform in the hopes of helping your organization reach new heights — but it shouldn’t come out looking like a Jenga tower of unstable technologies. There couldn’t be a worse strategy when you’re trying to create a foundation for the products and services that let your company thrive.
So how does this occur? After all, no platform team sets out to create a royal mess — but I’ve seen it happen countless times.
The problem often lies in how teams architect their platforms. Many try to weld together a slew of immature technologies that are still in the early phases of their lifecycles. Even if you can keep up with a few of these fast-moving components, the combined effect compounds exponentially — leaving you in the dust.
Avoid the house of cards antipattern by pacing yourself early. Don’t be hesitant to tackle the tough, unsexy tasks instead of going straight for the flashy stuff — Raise your odds of success by starting with the essentials.
Imagine you were building a house: You’d do things in a specific order. First, you’d pour the foundation, then build the frame and walls, and lastly add the doors, windows, and trim. As I mentioned in a recent blog post, that means you start with designing your IDP’s architecture before you think about building a shiny UI or service catalog.
And sure, I’ll admit that this strategy might not be the most glamorous — but laying the groundwork in the proper order pays off later. On top of increasing your IDP’s stability, constructing a solid base can make other tasks more doable, like integrating those cool technologies that teams have been clamoring for since the beginning.
The Swiss Cheese Platform
Swiss cheese has a lot going for it. For instance, it’s wonderfully aerodynamic, lightweight, and pretty tasty. Like Jenga, however, it’s not a good look for an IDP.
The problem is that you don’t always get to pick where the holes are — and some are deadlier than others. While you might be able to overcome gaps in areas like usability, security is an entirely different ballgame.
Remember: It only takes one vulnerability to cause a breach. If your platform is rife with holes, you dramatically increase the odds of compromising data, exposing sensitive customer information, and transforming your organization from an industry leader into a cautionary tale.
Building a platform that everyone loves is a noble pursuit. If you’ve done so at the expense of security, however, then your biggest fans will become your worst detractors the instant things go belly up.
The solution here is straightforward: Make security a priority from day one of the project — or better, before you even get to the first day of coding.
The only valid role Swiss cheese has in systems engineering is when you’re talking about risk analysis and accident causation, which drives home the point: You need to keep security front of mind at all stages.
The Fatal Cost Spiral
This is a big one. Many teams create platforms that lack inherent cost controls — like AWS provisioning quotas. This tendency to go “full steam ahead, budget be damned,” is no way to build.
The drawbacks of this antipattern should be obvious, but it’s easy to overlook their scope. Every platform engineer understands that it’s bad to exceed their project budgets. Few appreciate the broader impacts cost overruns can have on a company’s unit economics. Many companies that are compute-intensive spend more on the cloud than they do on offices and payroll combined. Bad unit economics for a tech company can literally make the difference between meeting earnings expectations or not.
Unfortunately, this mental disconnect seems to be quite deep-rooted. I’ve been in companies where you couldn’t order the dev team a pizza without a CFO’s approval — yet anyone on the payroll could call an API endpoint that would spin up hundreds of thousands of instances per day!
Getting everyone in your organization on board with cost-conscious DevOps may be a long shot. As a platform architect, however, you can lead the charge.
Consult with your FinOps liaison to keep your DevOps undertakings tightly aligned with your company’s financial architecture. If you don’t control the bottom line, your platform is doomed — no matter how much investment you secure early on!
Vital Takeaways for Smarter Platform Engineering
So what differentiates winning platform engineering efforts from failures? In review, you need to:
- Approach your platform from a product manager’s perspective,
- Sell your platform, but don’t oversell it,
- Treat your platform like a product, and identify who your key customers and stakeholders are,
- Accept that you won’t reinvent Heroku or AWS unless you’ve got hundreds of millions of dollars to spend, and
- Understand and iterate on an MVP that sets you up to win the next round of investment.
Of course, the failure modes listed thus far are just some of the ways to go wrong. Other common traps include mistakes like engineering IDPs to satisfy the loudest voice on the team at the expense of the quietest and sacrificing critical access to underlying technologies solely for the sake of abstraction. No matter which of these hazards proves the most relevant to your situation, however, gaining a broader appreciation for the risks in the early planning stages is a step in the right direction.
Know what to avoid — but also gain an appreciation of what to concentrate on. Focus on the things that make your platform engineering team more productive. Doing so will make it vastly easier to succeed and build something that delivers lasting organizational value.
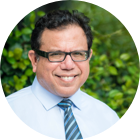
MMS • Daniel Dominguez
Article originally posted on InfoQ. Visit InfoQ
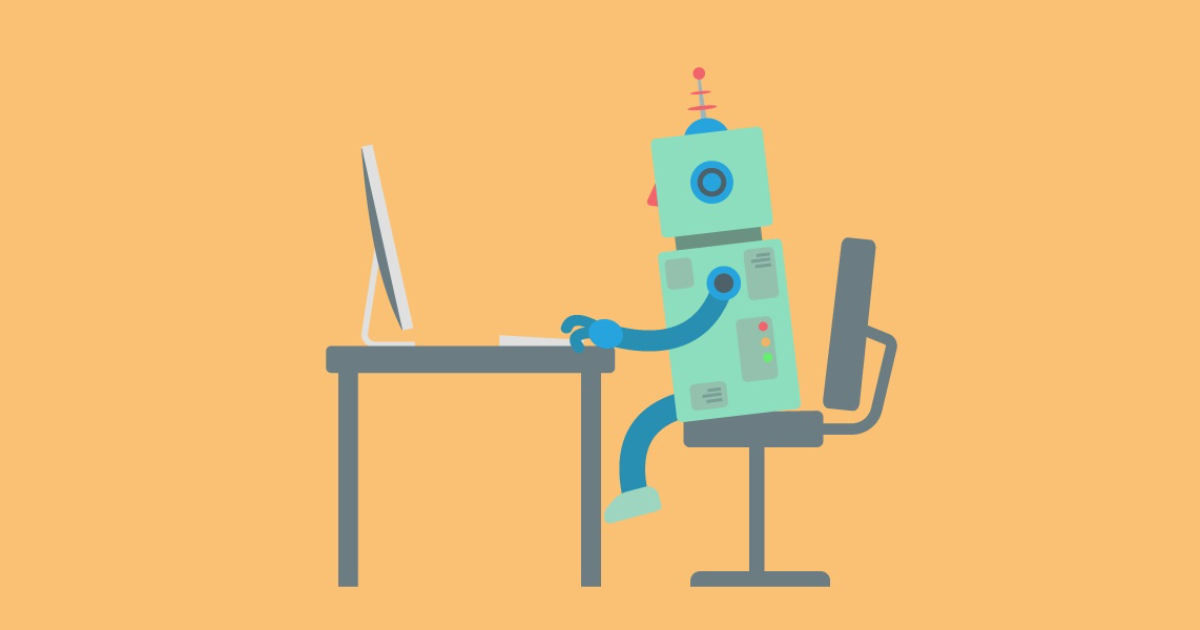
OpenAI is releasing a trained classifier to distinguish between text written by a human and text written by AIs. This classifier comes from a growing need for technologies that can help discern between material authored by people and that written by machines.
According to OpenAI, this new AI classifier is a language model that has been improved on a dataset made up of pairings of texts on related subjects that were both written by humans and generated by AI. The information was gathered from a variety of sources, including pre-training data from InstructGPT and replies to prompts that were thought to have been created by people.
However, the classifier has limitations and shouldn’t be depended upon exclusively, according to OpenAI. When determining the source of a text, it should be utilized in addition to other techniques.
AI text classifiers are constrained due to the nature of language, AI systems may find it challenging to fully comprehend the nuances of human writing, which is intricate, and frequently context-dependent. As a result, there’s a danger that important details and complex facets of the text being studied by AI text classifiers will be overlooked, which might lead to inaccurate predictions.
The importance of detecting AI generated text and its effects are acknowledged since the launch of ChatGPT, the AI system that has received high accolades for its ability to comprehend and react to a variety of questions and prompts with amazing accuracy and speed. It generates human-like prose using deep learning techniques.
In that order, the ability to distinguish between writing written by machines and text authored by people is becoming more and more crucial as AI generated material becomes more and more common. Teachers, students, and suppliers of educational services are among the groups that OpenAI is asking for comments and any useful materials they may have.
As part of its goal, OpenAI seeks to deploy substantial language models in a responsible and safe manner, working together with impacted communities. In addition, OpenAI has produced a resource manual that covers how to use ChatGPT as well as its advantages and disadvantages.
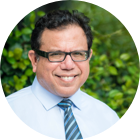
MMS • Johan Janssen
Article originally posted on InfoQ. Visit InfoQ
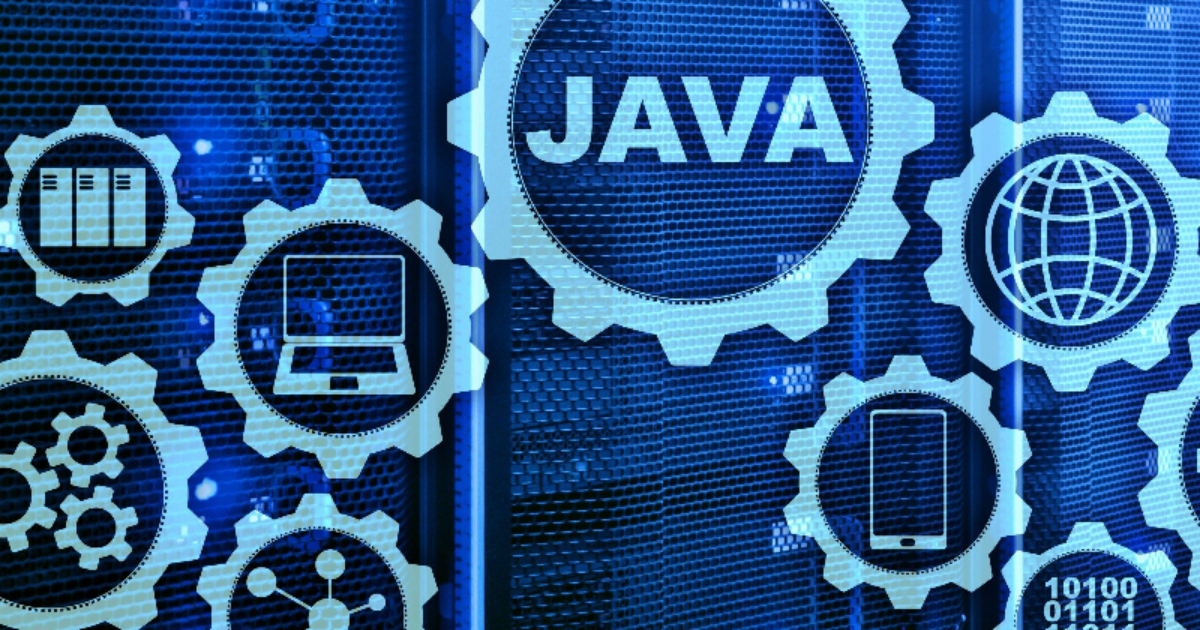
Microsoft announced an experimental feature, still under development, which improves the performance of escape analysis by increasing the opportunities for scalar replacement. Currently the feature is only available for Microsoft Build of OpenJDK, but might become part of OpenJDK in the future.
The feature may be enabled, on Microsoft Build of OpenJDK 11 and 17, by supplying the following JVM flags:
-XX:+UnlockExperimentalVMOptions -XX:+ReduceAllocationMerges
Escape analysis is performed by the Java runtime, or more specifically the JIT compiler, in order to detect objects which are used exclusively inside a method. Normally, each object is created on the heap, but if the object doesn’t escape the method, as it’s not returned by the method and not used by other methods, then the JVM could use something similar to automatic stack allocation of the object instead of allocating it on the heap. This means the memory is freed automatically after the method with the stack allocated object returns and the garbage collector doesn’t have to manage the object.
The HotSpot VM source code file, escape.hpp
, describes how each object is treated:
typedef enum {
UnknownEscape = 0,
NoEscape = 1, // An object does not escape method or thread and it is
// not passed to call. It could be replaced with scalar.
ArgEscape = 2, // An object does not escape method or thread but it is
// passed as argument to call or referenced by argument
// and it does not escape during call.
GlobalEscape = 3 // An object escapes the method or thread.
}
The NoEscape
option is used when the object can be replaced with a scalar. This form of elimination is called scalar replacement. The fields are extracted from the object and stored as some form of local variables inside the method which allocates the object. Afterwards the JIT compiler can store the object fields and local variables inside CPU registers or, if necessary, on the stack.
The experimental feature improves scalar replacement of objects by simplifying object allocation merges. Microsoft Build of JDK 11 contains a simplified version, whereas JDK 17 is capable of performing more scalar replacements.
Microsoft saw an increase of scalar replacements, when enabling the feature on JDK 17, between three and eight percent and a two percent throughput increase with internal benchmarks. Enabling the feature on JDK 11 resulted in a decrease of eight percent for the average P99 latency in memory intensive applications.
Cesar Soares, developer at Microsoft, created a pull request with the implementation on the OpenJDK GitHub repository. The feature is described, discussed and reviewed in the GitHub conversation. The specific changes for JDK 11 and JDK 17 may be viewed as a Git diff
on GitHub.
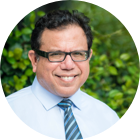
MMS • RSS
Posted on nosqlgooglealerts. Visit nosqlgooglealerts
MongoDB is a widely used NoSQL database that can function well in containers if you know how to set it up. Learn how to connect the Compass GUI here.
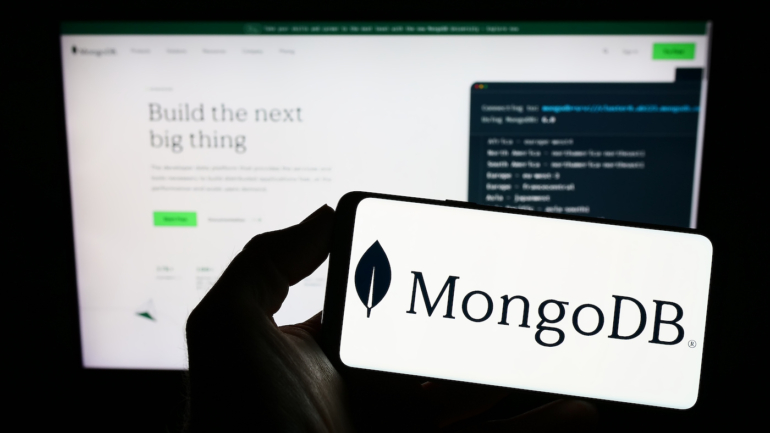
MongoDB is one of the most widely-used open source NoSQL databases on the market. It offers all the features you need to handle and manage massive troves of data and even offers an official desktop application that makes managing those databases a bit easier.
SEE: Hiring Kit: Database engineer (TechRepublic Premium)
You might think connecting the GUI application to a Docker-deployed instance of MongoDB would be pretty challenging, but it’s not nearly as hard as it sounds. In this tutorial, I’ll show you how to deploy the MongoDB container and then connect to it from MongoDB Compass.
Jump to:
What you’ll need to connect MongoDB Compass to a containerized database
To make this connection work, you’ll need a running instance of an operating system that supports both Docker and the MongoDB Compass app. I’ll demonstrate with Ubuntu Linux and show you how to install Docker, deploy the container and then connect Compass to a database. Please note that this process is compatible with a variety of Linux distros.
If you’re more interested in general instructions for installing MongoDB GUI Compass and connecting it to a remote server, this tutorial may be a better place to start.
Connecting to MongoDB hosted via Docker
The first thing to do when connecting to MongoDB through this method is installing Docker. You can add the official Docker GPG key with this command:
curl -fsSL https://download.docker.com/linux/ubuntu/gpg | sudo gpg --dearmor -o /usr/share/keyrings/docker-archive-keyring.gpg
Next, you’ll add the Docker repository:
echo "deb [arch=amd64 signed-by=/usr/share/keyrings/docker-archive-keyring.gpg] https://download.docker.com/linux/ubuntu $(lsb_release -cs) stable" | sudo tee /etc/apt/sources.list.d/docker.list > /dev/null
From there, it’s time to install the necessary dependencies with this command:
sudo apt-get install apt-transport-https ca-certificates curl gnupg lsb-release -y
In order to install the latest version of the Docker engine, you can use the following two commands:
sudo apt-get update
sudo apt-get install docker-ce docker-ce-cli containerd.io -y
Now, add your user to the Docker group with the following:
sudo usermod -aG docker $USER
Log out and log back in so the changes take effect.
Deploying and configuring the MongoDB container
We can now deploy the MongoDB container with the following:
docker run -d -p 27017:27017 --name example-mongo mongo:latest
With the container running, you’ll need to access it with this command:
docker exec -it example-mongo bash
Once inside the container, we need to edit the MongoDB configuration file with this command:
sudo nano /etc/mongod.conf.orig
In that file, locate the following section:
net:
port: 27017
bindIp: 127.0.0.1
Change that section to the following:
net:
port: 27017
bindIp: 0.0.0.0
Once you’ve made those changes, save and close the file. Exit from the container with the exit
command.
From there, restart the container with:
docker restart ID
In that command, ID is the ID of the Mongo container. If you’re not sure of the ID, you can find it with:
docker ps
Note: You might have to deploy the MongoDB container with environmental variables for the username and password, which can be done like so:
docker run -d –name some-mongo -e MONGO_INITDB_ROOT_USERNAME=NAME -e MONGO_INITDB_ROOT_PASSWORD=SECRET mongo
NAME is a username and SECRET is a unique and strong password.
Connecting to Compass
With the MongoDB container running, you can now connect to it with Compass using the same connect command you would use if MongoDB were installed via the traditional package manager and the user credentials you used with the environmental variables.
If you are still unable to connect to the containerized version of MongoDB from a remote instance of Compass, you might have to install Compass on the same machine running the MongoDB container.
Next steps
Congratulations, you now have a well-designed GUI to help make your MongoDB admin tasks a bit easier. You can connect to as many MongoDB servers as you need to from Compass and start creating and managing all the MongoDB collections you need.
Read next: Top data quality tools (TechRepublic)