Month: May 2023
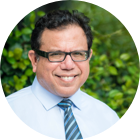
MMS • RSS
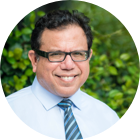
MMS • Sergio De Simone
Article originally posted on InfoQ. Visit InfoQ
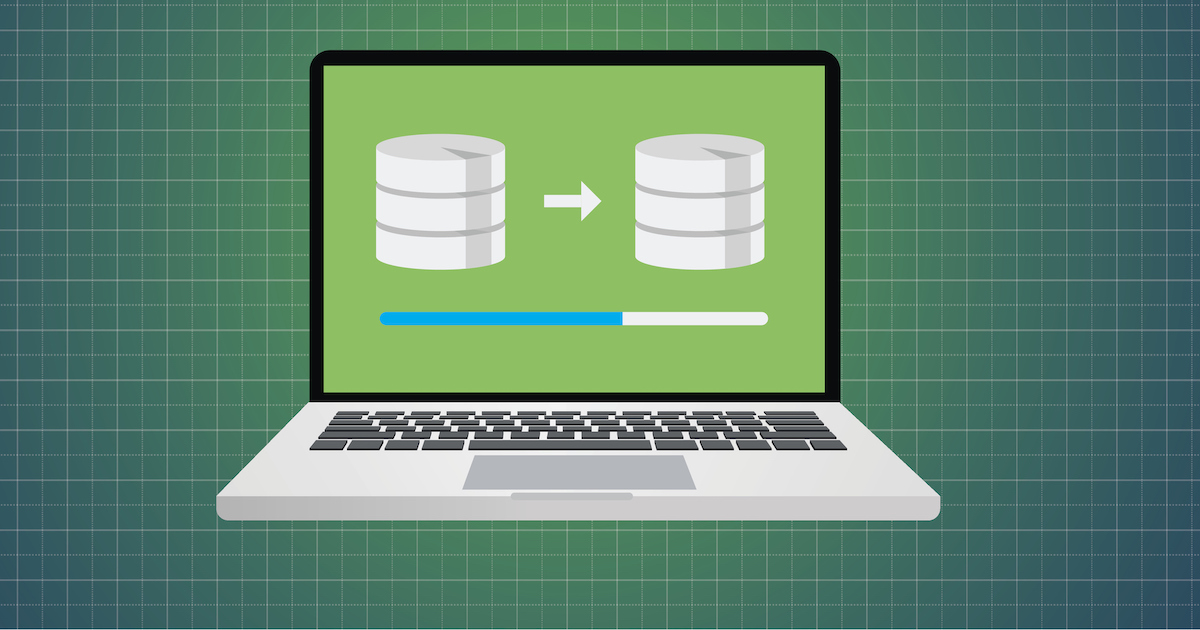
LLMs can be an effective way to generate structured data from semi-structured data, although an expensive one. A team of Stanford and Cornell researchers claim to have found a technique to reduce inference costs by 110x while improving inference quality.
According to Simran Arora, first author of the paper, using LLMs for inference on unstructured documents may get expensive as the corpus grows, with an estimated cost of at least $0.001 per 1K tokens. The strategy she and her colleagues at Stanford propose promises to reduce inference cost by 110 times using a sophisticated code synthesis tool dubbed EVAPORATE.
The basic task that EVAPORATE wants to solve can be described in the following terms: starting from heterogeneous documents, such as HTML files, PDFs, text files, and so on), identify a suitable schema, and extract data to populate a table. Often, traditional approaches to extract structured data from semi-structured data relies on a number of simplifying assumptions, for example regarding the position of tags in HTML documents or the existence of annotations, which necessarily end up reducing the generality of the system. EVAPORATE aims to maintain generality leveraging large language models.
In their paper, the researchers explore two alternative ways to extract data: using an LLM to extract values from the documents and build a tabular representation of the data; or synthesizing code that is later used to process the documents at large scale. The two approaches have different trade-offs in terms of cost and quality. Indeed, while the direct approach shows a very good performance in comparison to traditional techniques, it is very expensive.
LLMs are optimized for interactive, human-in-the-loop applications (e.g. ChatGPT), not high-throughput data processing tasks. The number of tokens processed by an LLM in EVAPORATE-DIRECT grows linearly with the size of the data lake.
On the other hand, the code approach, dubbed EVAPORATE-CODE, uses the LLM only on a small subset of the documents to generate a schema and synthesize a number of functions in a traditional programming language, e.g., Python, to extract the data from the whole set of documents. This approach is clearly less expensive than the former, but synthesized functions tend to be of varying quality, which affect the quality of the output table.
To strike a better balance between quality and cost, the researchers added a new element to their recipe: generating many candidate functions and estimating their quality. The results produced by those functions are then aggregated using weak supervision. This solution helps reducing the variability across generated functions, especially for those working only for a specific subset of documents, as well as the impact of those presenting any syntactic or logical errors.
Based on their evaluation of 16 sets of documents across a range of format, topics, and attribute types, the researchers say the extended approach, named EVAPORATE-CODE+, outperforms the state-of-the-art systems, which make simplifying assumptions, and achieves a 110x reduction in inference cost in comparison to EVAPORATE-CODE.
Our findings demonstrate the promise of function synthesis as a way to mitigate cost when using LLMs. We study the problem of materializing a structured view of an unstructured dataset, but this insight may be applicable in a broader suite of data wrangling tasks.
According to the researchers, there are many opportunities to further develop their system, including the possibilities of generating functions that invoke other AI models, such as those available on Hugging Face or through OpenAI. Another dimension to explore, they say, is iterating function generation so that, in case a sub-optimal or incorrect function is generated, it is fed back to the LLM to generate an improved function.
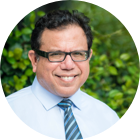
MMS • RSS
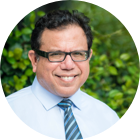
MMS • Irina Scurtu Martin Thwaites Guilherme Ferreira Scott Hansel
Article originally posted on InfoQ. Visit InfoQ
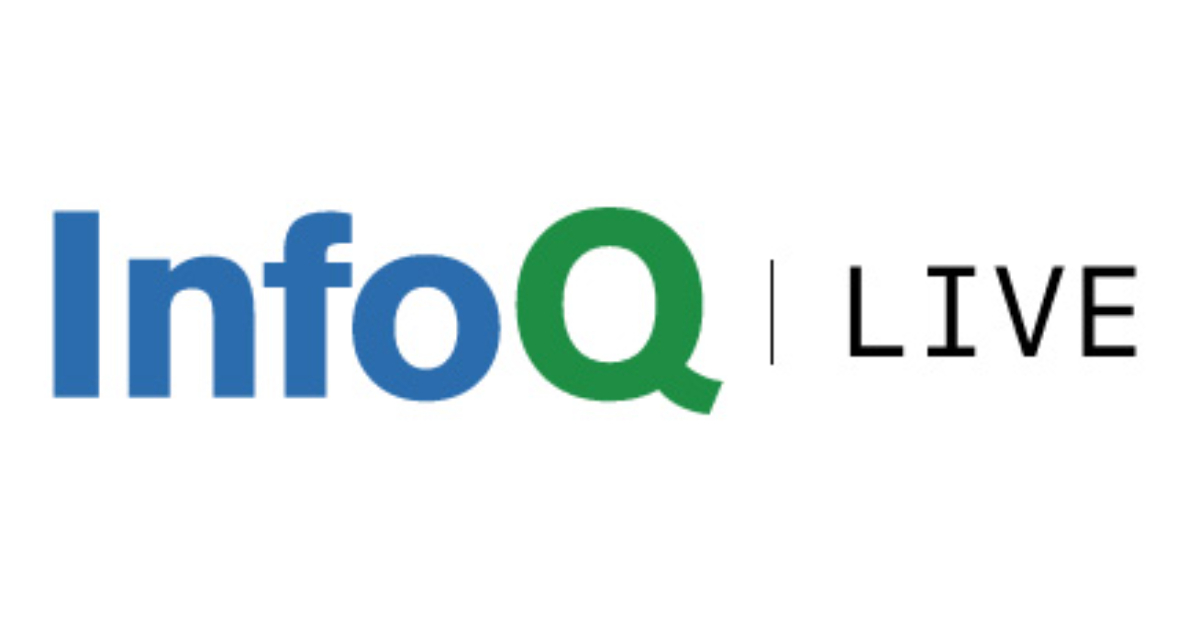
Transcript
Losio: In this session, we are going to be chatting about moving .NET applications to the cloud. I would like just to clarify a couple words about the topic. As organizations are increasingly moving towards cloud computing, there is of course a growing need as well for .NET application, the .NET world to be migrated to the cloud. We are going to discuss which tools, which services a .NET developer can use to be successful in building cloud native application. We’ll discuss benefits as well as challenges and mistakes made, and suggestions, whatever the different options because there’s not just one way to do it, whatever cloud provider you choose. You can use whatever we are using, managed Kubernetes services, serverless platform, EdgeDB based hosting option, the way you’re going to do it, we’ll see during this amazing panel. We now see how we can move .NET application to the cloud.
Background & Experience with .NET and Cloud Tech
My name is Renato Losio. I’m an editor here at InfoQ. I work as a principal cloud architect at Funambol. We are joined by four experts on .NET and cloud technology, coming from very different companies, different countries, different backgrounds. I would like to start giving each one of them the opportunity to introduce themselves, share their experience with .NET, and cloud technology.
Thwaites: I’m Martin Thwaites. I go by MartinDotNet on the Twitter’s, should give you an idea of where my focus has been for the last years on .NET. I’m a principal developer advocate for a company called Honeycomb, who provide observability type solutions. I work a lot on the OpenTelemetry .NET open source libraries.
Scurtu: My name is Irina Scurtu. I’m an independent consultant, Microsoft MVP, and speaker at various conferences. I’ve worked with .NET since I know myself, when I finished the computer science faculty, I leaned toward .NET because I hated Java at the moment. .NET was the alternative and C# was lovely to learn.
Ferreira: Feel free to call me Gui. I’ve been working with .NET since my first job. I have been in the cloud since 2012, I think so. Currently, I’m a developer advocate at FARFETCH and a content creator on YouTube, and all those kinds of things.
Hanselman: My name is Scott Hanselman. I’ve been programming now for 31 years. I have been doing .NET since its inception. Before I worked at Microsoft, I did large scale banking. I was basically putting retail banks online. I have experience in not just doing things on the web and in the cloud, but also doing it securely within the context of government requirements and things like that.
Major Pain Points of a .NET Developer, Dealing with the Cloud
Losio: Let’s start immediately with the challenges. What do you think is the major pain point today for a .NET developer, dealing with cloud technology, moving to the cloud?
Hanselman: I think that sometimes people move to the cloud in a very naive way. They think of the cloud as just hosting, at scale. I think that that’s a very simplistic or naive way to look at that. I use that word naive very specifically, because to be naive is a kind of ignorance, but it doesn’t indicate that it’s not something you can move beyond. You can teach yourself about these things. I feel a lot of people just lift and shift. What they’ll end up doing is they’ll pick up their .NET app, and they’ll move it over there. They’ll say, we’re in the cloud. Maybe they’ll get on a virtual machine, or maybe they’ll do platform as a service. That’s great. I think it is simplistic when there’s so much more elasticity and self-service that they could do. They also tend to spend too much money in the cloud. The amount of headroom that you need on a local machine that you paid for, and the amount of like extra CPU space, extra memory space, you can abuse the cloud, you can treat the cloud like a hotel room or an Airbnb, and you can leave it destroyed. Then let the people clean up after you.
Scurtu: I feel so many teams like using the cloud just because it’s cloud and it’s there and it should be used, and afterwards complaining about the invoices that come at the end. They didn’t know how to tweak things or use things just because they are shining, that they needed those.
Minimizing Lift and Shift During Migration
Losio: Scott raised the point of lift and shift. I was wondering if it’s really that people don’t know, or maybe people are even overwhelmed by the too many options to do one thing, or how many services now cloud providers can offer? What’s the first step a .NET developer should do, to not do, at this point, lift and shift? What do you recommend?
Thwaites: I honestly don’t think it’s .NET developer specific. This is about defining why you’re moving to the cloud. Is it because you want things to be cheaper? Is it because you would like things to be easier to scale? Do you want that elasticity? If you don’t define why you want to go, you can’t decide how you’re going to go. If you want elasticity, just dropping your stateful website that doesn’t support autoscaling in the cloud is not going to give you elasticity. Because you’re still going to have to have that stateful thing in the cloud and it won’t scale. You can maybe buy a bigger server quicker, but you’re still going to have downtime. If you don’t go into moving up, you can’t choose the right platform. Is it choosing App Service? Is it choosing the new Cloud Run stuff? All of that stuff is, I need to know why I’m trying to do it in order to be able to choose the right things.
There’s everything from Container Apps in Azure, and Fargate in AWS. You’ve got Functions. You’ve got Lambda. You’ve got all of those different things. Unless you know why you want to move to the cloud, you’ve got that edict that’s come down from the big people upstairs that say we need to move to the cloud. Like, “Great. Ok, I’ve moved to the cloud.” Why is it seven times more expensive? You didn’t specify that reducing cost was the reason why we’re going up there. If you don’t specify the why, then you are not going to get there. You might say, yes, we’ve got the rubber stamp, the tick box that says we’re in the cloud, but that’s it. I think that’s the biggest problem that people have at the moment. They think that just lifting and shifting an app that exists on-premise, or on that machine under Scott’s desk, that moving that up to the cloud doesn’t inherently make it more scalable, it doesn’t make it cheaper, it doesn’t do any of those things. It will make you tick the checkbox of in the cloud. If that’s the only thing you want to hit, do it.
Lift and Shift: First Step or Incremental Move to the Cloud?
Losio: I was thinking, we’re back really to the lift and shift topic. For example, if you see it more as a, no, very bad approach always, or it’s like, can it be a first step or an incremental move to the cloud? I have my monolith, I move it to the cloud. I start to break it in pieces, or maybe I prefer to start immediately with moving my .NET app to Functions or whatever.
Ferreira: It’s maybe a first step, because everyone remembers what was the first two months of the pandemic, and you have a service in-house, and everyone needs to access it now. How do you do it? If you have just a month to do something, it’s better than nothing. As Martin was saying, I can remember those moments where we were being sold that cloud was just about cost savings. I don’t recall a sales pitch on the potential of cloud. If you go with lift and shift, you can achieve some results. At least you should think about what you’ll be doing next. What are the next steps? There’s a strategy needed there, in my opinion.
The Next Step After a Lift and Shift Migration
Losio: Irina, I was thinking, as he just mentioned, you should think what the next step is. I was thinking, I’m a developer. I did my very first step. I got some credit from Microsoft, or some credits from AWS, I decided to lift and shift my stuff to the cloud. I have maybe a simple app bridging .NET with a SQL server, database, or whatever. Now, what’s the next step? Just wait until I run out of credits and then think, or how can I really go cloud native? What’s the next step for me that you recommend?
Scurtu: As an architect, I would say that it depends. Again, if you’re a developer trying to learn about cloud and what it is and why it should be used, then as a .NET developer, it’s very easy. You have everything there, minimal code changes, you’re up and running in cloud. If you’re trying to think that, I have a whole system that I want it in cloud, the story is, it’s way longer than it depends. It depends on the ulterior reason that you have. You want to be in cloud because it’s shiny, you want to modernize your app, or you want to actually achieve some business checkpoints, rather in costs, rather in scaling your app or serving your customers, or just making sure that you won’t lose business when there is high demand. You need the elasticity of the cloud.
Hanselman: I just think it’s lovely, though, because what we’re acknowledging is that software is meant to solve problems for humans and for businesses. If we go into those things, just looking at the tech for the tech’s sake, we’re going to miss the point. Everyone on the panel has so eloquently said that, why? Why does the cloud exist? Why are you moving to the cloud? Because you’ll see people work for a year for their Cloud Run migration strategy. They’ll go, what did you accomplish? I’ll say, it’s over there now. This one goes to 11.
Approaching .NET Development from Scratch (Serverless vs. Kubernetes)
Losio: Let’s say that I’m in a very lucky scenario, I start from scratch. I don’t have to migrate anything. Now let’s get a bit more in the, what can I really use? I have the world open in front of me, I can choose any cloud provider I want. I want to go to the cloud. How do I develop my app? I start with serverless because it’s cool, because it’s better. No? I start with Kubernetes. I start with whatever. How would I approach my .NET development from scratch?
Thwaites: The first step I would go with is Container Apps or Fargate. It’s middle of the road, which is the reason why I normally recommend it to people because you’ve got control over your stuff. You can run it very efficiently locally. You’re scaling very easily. In using containers, you’re essentially going to be building something that’s stateless, or that’s built to scale. You’re not having to worry about VMs. You’re not having to worry about a lot of things. You’re also not having to worry about hiring 17 Kubernetes administrators to fire up your AKS cluster and manage it, and scale it, and all that stuff. There’s been a while where people are going, “Kubernetes is the future. Everybody should be deploying on Kubernetes.” I don’t agree. I think managed container platforms are the future. Because I don’t want to care about Kubernetes, I want to say go and run my app. I would probably use App Service, if I’m on a .NET and Azure. There’s actually something that AWS have just released for the .NET stuff, which is very similar, which is, here’s my code, go run it. I don’t want to care about where it runs. I don’t want to care about VMs. I don’t want to care about a slider to add more VMs. I just want like, here’s my code, and go and run it.
I also don’t want those costs to scale exponentially, like you would get with serverless. There is too much to consider with serverless. To me, middle of the road is containers. That’s what I always recommend to people, run it in a container, run it on Container Apps, run it in Fargate. Those things are much easier to get started with. Then you can choose which direction do you go. Do I go with something that’s going to be charged by request or execution, or do I go with something where I have consistent scale, so I can actually get a lot more cost by buying VMs up front? If you go middle of the road, you’ve got both ways that you could go with it.
Losio: Gui, do you agree or will you go more on the Kubernetes way?
Ferreira: No, I will say that when you start, if we’re talking about Azure, the easiest way is that for sure you are a web developer, you are more than used to building web applications, deploying to App Services, it’s quite simple. You don’t need to learn a lot of things. It’s an entry point. Once you start getting comfortable about that, maybe you can start thinking about what types of things I can solve with serverless. Because learning serverless when you have been doing web development for your life, it’s a different beast. There’s different concerns that you need to think about. Kubernetes, you most likely don’t need it.
Managed Container Platforms
Losio: If I understand well, you’re all saying that as a single developer, or if you’re not running a huge, large-scale project, probably you’re going too early in Kubernetes. Did I get it right or wrong? Do you see any scenario where it makes sense to think almost immediately to move to Kubernetes?
Hanselman: A couple of years ago, maybe 5 or 10, IIS ran the world, then it was Apache. Then we went to sleep for a minute and we woke up on a Tuesday afternoon, and it was NGINX, and no one could find Apache anywhere. Then a couple of weeks went by, and then it was Kubernetes. It’s always going to be something. Kubernetes is great. It’s lovely. It’s the hotness right now. I have to agree with Martin that I don’t want to see all the knobs and the dials. I don’t want my web application to look like the dashboard of a 747. It’s too much. I think that managed container platforms of which Kubernetes is one, with orchestrators, of which Kubernetes is the first among equals, is the move. I think that we should probably spend some time thinking about what it is about .NET that is special and impressive, and then how it relates to containers.
As an example, I ran my blog and my podcast on Windows 2003 Server on IIS for 15 years. Then with the .NET Core wave and now .NET 5.6.7, compiled it on Linux, put it in a Docker container. Now I can put it anywhere I want to. I can run it on Kubernetes. I can run it on Linux. I can run it on WSL. I can run it on a Raspberry Pi. This is an 18-year-old .NET Windows application that by virtue of .NET’s ecosystem is now a cloud-based container application and happens to be running in App Service for containers on Azure, but could work in ACA or could work in AKS. That’s the magic in my mind of .NET. If I wanted to move it to Linode, I could do it tomorrow. It wouldn’t even have a moment of downtime.
When Azure App Service is not the Best Choice
Losio: Actually, I already heard three people mentioning the benefits of using App Service. Is there any scenario where any of you would recommend not to use, where probably going App Service is probably not the best choice for moving, for example, a .NET of course?
Hanselman: One example I like to give is actually now almost a 20 or 25-year-old example. I used to work at a company called 800.com. We had a deal where we sold three DVDs for a dollar. It was pre-Amazon online system. We had the shopping cart, and we had the product catalog. We all ran it on IIS. Imagine if you’re running it on App Service, and people are browsing, and people are buying stuff. Ninety-seven percent of people were basically browsing, and 3% were buying stuff. Then we said, three DVDs for a dollar to get the internet’s attention, and the internet lost their minds. Then suddenly, 3% of people were browsing, and 97% of people were literally trying to give us a dollar. Because the whole thing was one application in one place, we couldn’t just scale up the shopping cart. In this case, again, 20-plus years ago, we had to change DNS to have shoppingcart.800.com and products.800.com. Effectively partitioning node sets, and then change the scaling model. If you put everything in one single pile in App Service, you have limited scaling abilities, because you don’t have microservices, you don’t have individual things. You could have separate app services, where you’ve got Kubernetes, and you’re running things, you go, “Quick, turn the knob on the shopping cart side and turn down the knob on the product side,” or serverless, and then change the elasticity of those services. App Service would probably be a little more difficult if you had a more complicated architecture like that.
Patching and Security Updates in the Cloud
Losio: It sounds great just to run my code, but how about patching and security updates. I hear that question often about the move to the cloud. How do I think about patching and security updates when I move to the cloud?
Scurtu: The nice thing about cloud is that they just take care of that. Patching and security in your code, it’s your business, like using secure DLLs, libraries and everything related to the code itself. When it comes to infrastructure, whatever you’re using, except virtual machines, they will take care of that. They will remove the burden of you going in, updating, and having downtimes and so on, because basically someone else is running the things in your place. It’s nice. It removes the burden of manually getting into servers and applying updates. For example, when you’re running, you have your computer open and just starts updating. Yes, it’s not nice to happen on the server that’s on-premise.
Thwaites: We talked about running containers. I think one of the things that people miss is your container is your responsibility, not just your code, when you’re doing containers. When you’re doing App Service, which is the reason why I want, here’s my code, just run it, because somebody else manages both the container runtime and what’s installed inside my container, and updating the base images, and all of that stuff. App Service is great, because I can just say, here’s my code, or Functions is great, just give me my code, and you can go run it. If you’re running .NET in the container, you’re going to have to go up a little bit further. You’re going to have to say, I need something that’s going to manage the security of my containers, and know the operating system they’re running on. If I’m running a Debian container, or even if I’m running Alpine, there are security vulnerabilities that are in there. Do keep that in mind, because you’ve still got to do that if you want to run containers. You’re not completely out of the whole thing.
What to be Aware of, When Architecting and Designing for Cloud Native
Losio: When we’re architecting and designing for cloud native, what is a concern for us? What do developers need to be aware of? Basically, how do we keep the 747 cockpit that we mentioned before, hidden from the devs? Any advice?
Ferreira: I have worked at least in two companies where due to the size and all those “Netflix Problems,” we will either run on Kubernetes or in AKS as well. One common problem that I’ve seen is that when you give access to everything, and all the responsibilities are for developers, they will have extra concerns on the day-to-day job. They have more stuff to learn. You will demand more from them. Usually that creates some problems, because not everyone likes to do that Ops part of the job. It’s always a tradeoff. With great power comes great responsibility. That’s the way I see it. Because on those organizations, I’ve always seen by the end, the architecture team, trying to create abstractions on top of those platforms. If you are creating those abstractions, maybe they already exist on the cloud platform that you choose.
Thwaites: I wanted to just bring in the new word that people are going on about, is platform engineering. The idea of the 747 cockpit is what platform engineering build. They build your own internal abstraction on top of Azure. The Kubernetes stuff, they will build their own dashboards, or they’ll build their own cockpits. That is useful to them. That 747 cockpit is what they’re built to look at. That’s their tool. Yes, you hide that away from them but developers need to care about more. They need to care about how they deploy. They need to care about where it’s deployed. They need to care about scale. Don’t abstract too much away from them. It’s a balance. There’s no single answer.
Azure AKS vs. Azure App Service
Losio: How do you decide which service you should use between Azure AKS and Azure App Service? Which of the two domains?
Hanselman: I have a whole talk on this. In the talk I use this analogy that I really like, which is well known, it was called pizza as a service. You’re having a party, and you’re going to have some pizza. One option is you have to bring your own fire, your own gas, your own stove, and friends, conversation, and the stack goes all the way up. Or you could just rent like the actual people, and you could go to a place, they have the pizza. They provide the party room. They could even provide pretend actors to pretend to be your friends. Pizza as a service goes all the way out to software as a service. I could write a fake version of Microsoft Word and run it in a virtual machine, or I could pay 5 bucks a month for Office 365 and everything in between.
To the question, how do you decide between App Service and AKS? You have to ask yourself, do I have an app that I want to scale in a traditional web form way which has knobs going horizontally? I want to have n number of instances, like in a web form, and I want to scale up, those two dimensions? Or do I want something that is more partitioned and chopped up? Where I’ve got my shopping cart and my tax microservice and my products, and all of the different things can scale multi-dimensionally. Do I have something that’s already architected like that? If you have an app right now, like I did, sitting on a machine under your desk, App Service. If you’re doing some Greenfield work, or you have something that’s maybe a little bit partitioned, and you already have container understanding, put it into a managed Kubernetes service. I think you’ll be a lot more successful. It gives you more choice. You can do that in multiple steps.
Reducing Future Maintenance Costs, for a Functions as a Service engineer
Losio: What are the best practices for a Functions as a Service engineer to reduce the future perpetual maintenance costs?
Ferreira: When we are talking about serverless, it’s not a problem of all in or nothing. It’s important to find the correct scopes for functions and for the rest. From my experience, there’s always a place for them, but it’s not always the place that maybe you’re thinking about. Not every single type of problem can be solved with Functions as a Service.
Scurtu: I’ve seen a project where they used to have like huge costs caused by functions, because they basically architected it that way. Each function costs a bit to run it. Each function generated an output, that output we used to trigger a few wheels in the system. At the end of the month, the cost was huge. Most of those things could have been replaced with simple smaller components, just to reduce the costs. In the end, if you’re not doing flowers and painting things on the walls, you might have very predictable costs even with Azure Functions. It depends what you get after you run the function. That might be the actual issue.
Thwaites: I read something recently, where they were talking about the, yes, your Azure function infrastructure looks a mess, because it’s this one column, this one, this one column, this one. However, that’s a much more honest analysis of your system, than doing it in a monolith and saying, there’s one big thing going in, and one big thing going out. Because actually what’s happening is that’s the communication that’s happening between all of your individual functions in your application. People building these big nanoservice type infrastructures, not functions, the nanoservices, they do really not one domain, they do one little thing. It’s like 10 lines of code. When you then architect that entire thing out as Azure Functions, and you get your architects in and they build a big whiteboard thing, it’s a more honest description of what your system is actually doing. I liked that idea that actually, no, you should simplify the system. It’s like that is your system. It may look complicated, because it is.
Hanselman: I totally agree with everything that you just said. I have a good example also, and this is the difference between cloud ready and a cloud native. When you say cloud native, what does that really mean? People usually think cloud native means Kubernetes, but it really means that the app knows the cloud exists, and it knows all the things are available to it. Using Azure Friday, as an example, simple application, it’s an app service, it’s a container. It’s not rocket surgery. However, it has some background services that are doing work, and they’re 10 to 50 lines of code. The Azure Friday app knows that the cloud exists. When a file drops into Azure Storage, triggers fire off, 10 lines of code runs here, background Azure Functions go and run processing in the background. Then it provides microservices to allow for search and cataloguing of the 700 different shows that we have at azurefriday.com. In the old days, that might have been a background thread in asp.net, in a pipeline in App Service. Because I know the cloud’s available, and it’s pennies to run serverless, I have an application that is both a container app but also a cloud native app in that it knows that a serverless provider exists for it. That’s another example where you could move them into the cloud naively, then move to App Service. Then, to Martin’s point, say, this weird background thing that we used to run that way, that really belongs over in an Azure Function or a Lambda.
When Moving to the Cloud Makes no Sense
Losio: Basically, we started this discussion saying, we’re going to discuss how we’re going to move .NET application to the cloud, the different options, whatever. My 20-plus years old application on my server running under my desk, or whatever, my server in my office or wherever that server is. Do I need to move to the cloud? Do you see a scenario where it makes no sense to move to the cloud? If so, which one?
Ferreira: I can remember some cases where I’ve seen companies avoiding it and completely understand why. Usually, it’s because of security or compliance reasons. I have worked for a company where legally they couldn’t do it because that data according to the jurisdiction of their country couldn’t move to another place. For example, when we are in the European Union, it’s quite simple, but then when you start going to other countries, these kinds of problems may happen. On those cases, it doesn’t make sense at all. Besides that, I think that if you are not willing to do the investment of taking advantage of things that cloud can give you, maybe you can keep up taking care of your servers for a while. Because if you go into the lift and shift mode, the cost will be quite high. Then you will get into a point where everyone that took the decision of moving to the cloud will regret about that. If you don’t want to modernize the applications, I don’t see that going well in the long term.
Scurtu: Like COBOL, for example. COBOL, the programming language that appeared 64 years ago, it’s easier for systems that were written in COBOL, and it’s cheaper to just hire new people, train new people to continue to run them, not moving those to cloud. There will be a time, five years in the future, when these companies will have to be on cloud, just because maybe their code that is old becomes deprecated. The security things will not be supported anymore. I’m not seeing those businesses up and running in the future. They will encounter a problem, when modernization will become an issue, maybe not on cloud, per se, but just to modernize them in a way, whatever that means for them.
Is a .NET App in Azure Service Fabric, Running in the Cloud?
Losio: If I had a .NET app running under Azure Fabric, should it be considered running under cloud?
Hanselman: If it’s running in Azure, it’s in the cloud. It could be a virtual machine, it’s in the cloud. If it’s not under your desk, or in someone’s colocated hosting, it’s in the cloud. Yes, absolutely. Is a fabric or a mesh like that? Is it a cloud service? Absolutely. If it’s calling any Azure APIs directly, that’s absolutely considered running under the cloud, 100%.
Thwaites: I’d like to follow up on that and ask, why is there a question? It comes back to what we were saying about the goals of running under the cloud. Because that’s the sort of question that you get an exec asking, saying, are we running in the cloud? It’s like, we’ve got a VM in Azure, we’re in the cloud. Great, tick box on a tender document. Is that what the answer that Billy is looking for is, can I say to my execs that we’re running in the cloud?
Hanselman: I think that is what it is. They want to tell their boss, are we in the cloud? Yes. Again, you could be running a $7 Linux VM in Azure, it’s still running in the cloud.
Disadvantages of Serverless
Losio: Serverless is not all rosy, there is concern of time period of execution, or run to completion is not guaranteed. When we start breaking down too much, the architecture becomes too cluttered. I think this one doesn’t really refer to .NET only. It’s a common question about serverless deployment. If any one of you wants to address the point of disadvantage of serverless approaches.
Thwaites: It’s about how you design them. I think the way that you avoid a lot of this is by being conscious about your choices. Why is that a function? Is there a reason why you’ve made it a function over some of the other choices, some other cloud native choices? Is there a reason why you’ve decided that you want 10 lines of code and a function here, and 10 of them? I consulted for a bank recently, and they were writing nanoservices, literally every function, every HTTP endpoint that they’d created was an individual function app. Because, to Scott’s point earlier, they wanted to be able to scale them differently, and maybe migrate them to another App Service because they’re all fronted by APIM. Then you look at them and go, why did you make that decision? Because we wanted to be serverless, and everything should be serverless. I think it’s that decision making that’s the problem. Why should they do that? What is the reason that they’re choosing to do serverless? I think it comes down to that same question of cloud. It’s because I want to be able to say that I’m serverless. Serverless to me is about elasticity of scale, essentially, infinite scale. I know it’s not infinite-infinite. For most intents and purposes, it is. It’s also about the scalability of cost, which is why, say serverless databases like Cosmos or DynamoDB, where you pay by the request, those are to me what serverless is about, because if I don’t get any hits on my website, I don’t pay anything. If I get lots of hits on my website, then I do pay for things. I think, yes, this idea that serverless isn’t all rosy comes from too many people choosing serverless for a small function that should have probably just been five or six of them dropped into one container app.
Mainframe Apps in the Cloud
Losio: Someone followed up on Irina’s comment on COBOL, saying, there are some frameworks like COBOL .NET, if Microsoft is a big business, they could address them.
Hanselman: There’s a whole mainframe migration department. You can basically run mainframe applications in virtual machines in the cloud, which is really interesting. I actually did a couple episodes of Azure Friday on it, with the idea that you’ve got mainframes mid-range, and then like Solaris and VAX machines running emulated or otherwise, in the cloud. That’s really big business. It’s super interesting.
Factors to Consider when Choosing a Cloud Provider
Losio: How do you choose a provider apart from cost? Usually, from an engineer’s point of view, whatever, I might say you’re an engineer or an architect, I work for a company that often has already made a choice or is already on the cloud, has a deployment already. Usually, I deploy my .NET app wherever. Do you see any specific reason apart from cost that should be taken into account when you make the decision, if you have that chance to actually make a decision yourself. I keep hearing people talking about cost, but as I’m usually working on the cloud technologies, I often find it hard myself to predict cost. People keep saying, you choose one provider or you choose the other one according to cost of a specific serverless. Martin mentioned about serverless or mixed solution is not just a lift and shift. It’s not that easy to predict, if you consider this cluster, if you consider anything else. How do you make a choice? How do you do that first step?
Hanselman: How do you make the decision about what host to go with?
Losio: Yes.
Hanselman: If your needs are simple, and you need to spin up a container for 8 bucks in the cloud, you can do that anywhere. You can pick whatever host makes you happy. There are a lots of people that are ready to spin your $8 container up in the cloud. If you are building an application with some sophistication, and you have requirements, be those requirements, data sovereignty, and you need a cloud in Germany run by Germans, or if you have HIPAA, American health requirements for how your data is treated, then you’re going to want to look at the clouds, the certifications. If you are primarily a Linux house, you’re going to want to make sure that you’ve got the tooling that is available, and everything that runs on Linux. If you all run Ubuntu on the desktop, does Visual Studio Code have the extensions that you want to run on the cloud that you’re going to want to go to? For me, it’s not just the runtime aspect of Azure that makes me happy and why I stay on Azure. It’s the tooling. It’s the Azure command line, and the plugins for both Visual Studio and Visual Studio Code. You need to look at the holistic thing. If you just want to drive, buy a Honda, buy a Toyota, but people who are really thinking about their relationship with that car company are thinking about, where am I going to go for service when the Honda breaks down? How many different Honda dealers are there? Those kinds of things. It’s a little more holistic.
Monitoring a .NET App in the Cloud
Losio: I move my .NET app to the cloud. Next step, monitoring. How do I monitor it? What’s the best way to do it?
Thwaites: Monitoring is becoming more of a dev concern. The whole DevOps movement around bringing people together and allowing devs to care about a lot more of this stuff around how their app scale. That’s where OpenTelemetry comes in. It all comes down to the whole portability debate. Scott said, if you’ve got a container, you can run it anywhere. You’ve also got to think about your monitoring, how exactly you’re going to monitor this thing, make sure it’s up. Does your cloud provider have built-in monitoring? Are you going to choose an external vendor? That’s where OpenTelemetry comes in now, because it’s vendor agnostic, and everybody should be doing it. The otel.net stuff is stable. It is robust, and allows you to push to anywhere, from Azure Monitor, to X-Ray, to vendors like ours. That is, to me, where this future of how do we ensure that developers can see that their app is running. Because if you deploy your app to Azure, and nobody can hit it because it’s down, was there any point in deploying it to Azure in the first place? You need to know these things.
That, I think, is where as a .NET community, we’ve not done as much to enable people to think about this monitoring stuff. They’ve seen it as a concern, where they hand it over to somebody else, and somebody else will do monitoring and observability for them. I think we’re getting to a point now where everybody’s starting to care about it. It makes me really happy that everybody’s starting to care about this stuff. I want to know, tell me, is it running fast? Is it running slow? Is it doing the right things? How did this go through that application? How does it transition through the 17 Azure functions that I’ve written? All of that stuff is really important. I think we’re getting to a stage now where people care about it.
Public Cloud Portability
Losio: Could you share thoughts on public cloud portability, containers and Kubernetes are presenting the least common denominator. Basically, is the concern about moving towards managed service, is somehow offset by concern around platform lock-in. Gui, do you have any feedback when to avoid that?
Ferreira: The good thing is that Docker became so common sense in our industry, that even things like App Service will give you a way to run your Docker containers inside it. That’s the good news. Besides that, what I always say is that the cloud doesn’t remove the job of doing proper work on your code itself. Creating the right abstractions in case you need to move to a different thing, a different SDK, a different something, it’s always important. If you are concerned about that, for example, in things like functions, even in functions or serverless, there’s ways to abstract yourself from the platform where you are running.
Scurtu: Actually, the thing with vendor lock-in, I’ve seen it as a concern from the business people, saying, we have this product but we do not want to be locked-in in Azure or in AWS, we want at the moment in time to just move over. I’ve seen the concern, but I never seen it put in practice. Everyone wants just to be prepared for it, but no one does it ever. I think this is somehow a premature optimization. Like let’s do this, because maybe in the future, we’re going to switch the cloud provider. I’ve never seen it. Because major projects with vendor lock-in problems, I don’t think it’s real.
Thwaites: I’ve never heard anybody say that they changed database provider.
Hanselman: It’s very likely not the runtime that is keeping you there, it’s almost always going to be your data. You’re going to end up with a couple of terabytes in Azure Storage and you want to move them to S3, or you’re going to go with Cosmos and you want to move to Atlas or whatever. It’s not going to be your containers, it’s going to be your data.
Thwaites: I think you also miss out. You miss out on a lot of the optimizations that you get by developing specifically for Cosmos. You get to use a lot of things that are very specific to the way that Cosmos works. Its indexing systems are very different to Dynamo.
Hanselman: People want to put their finger on the chess piece and look around the board and not actually make the move.
Thwaites: You lose scale. It costs you more money, because you end up going lowest common denominator, as the question said. You go with the, what’s the API that both Dynamo and Cosmos support? It’s Mongo. We’ll go with Mongo, then. There’s a lot more that you can do with Cosmos, there’s also a lot more that you can do with Dynamo, and the way that they work, if you really lock yourself in.
Hanselman: Azure Arc is a really cool way that you can have Kubernetes running in multiple places, but then manage it through one pane of glass. I could have Kubernetes and AKS in the cloud, and I could have Kubernetes on my local Raspberry Pi Kubernetes cluster, but it would show up in Azure, which means that I could also have Kubernetes running in Google Cloud, or in AWS, but all manage it on one place, which is cool.
The Journey to .NET Apps in the Cloud
Losio: Thinking, from the point of view of an engineer that attended this roundtable, I did it, I enjoyed it. What can I do tomorrow? What’s your recommendation of one thing I can do tomorrow to start my journey?
Thwaites: Decide why. Decide why you want to go to the cloud. Set your objectives: cost, efficiency, scale, whatever it is. Set some values that you either want to go up or down. Then, you can make some decisions.
Scurtu: Just make an informed decision, look it up before just deciding on a thing or putting your finger on that.
Ferreira: Get comfortable with a platform. When I say to get comfortable is not to go deeper on it, but give me a first step. There’s a different feeling that comes from playing around with things, getting a notion of what it is. Doing a quick tutorial to see how you go from your code to the cloud, can spark a lot of ideas.
Hanselman: Get back to basics. Someone asked me what someone would want to learn in 2023. They thought I was going to say, take a class on this cloud or that cloud. I was actually going to say learn about DNS and HTTP. You would be surprised how many people I’ve seen with 5, 10 years of experience that can’t set up a TXT record, or a CNAME in DNS. Those things aren’t changing, so learn the basics. The cloud is implementation detail.
Thwaites: The problem is always DNS, and the answer is always DNS.
See more presentations with transcripts
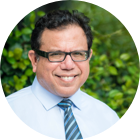
MMS • RSS
Posted on nosqlgooglealerts. Visit nosqlgooglealerts
In this month’s column for The Stack, former divisional CTO at Worldpay and IT veteran David Walker is a little frustrated with SQL myths and a lack of database category salesperson knowledge. Or is it ethics?
Some things in life are complicated: tax; love; the point of Tottenham Hotspur FC. And some things in life are simple. Always choose the steak if it’s on the menu –(your home frying pan can’t reach the same temperature as a commercial kitchen pan, so it will cook better!), no task will ever be completed in the time you estimated, and if you ask a BMW salesman to give you a fair verdict of a Mercedes, they just can’t—the inbuilt bias is just too strong.
You’d think that last one would be obvious, but a few conversations with CIOs have made me fear that some database vendors think they can pull the wool over their eyes by misrepresenting the full capabilities of the other fellow’s wares.
A case in point: vendors of NoSQL solutions telling big customers that SQL-based competitors can’t do x, could never do y—and that whatever your use case, their solution is the best fit.
All’s fair in love and database marketing? Maybe not
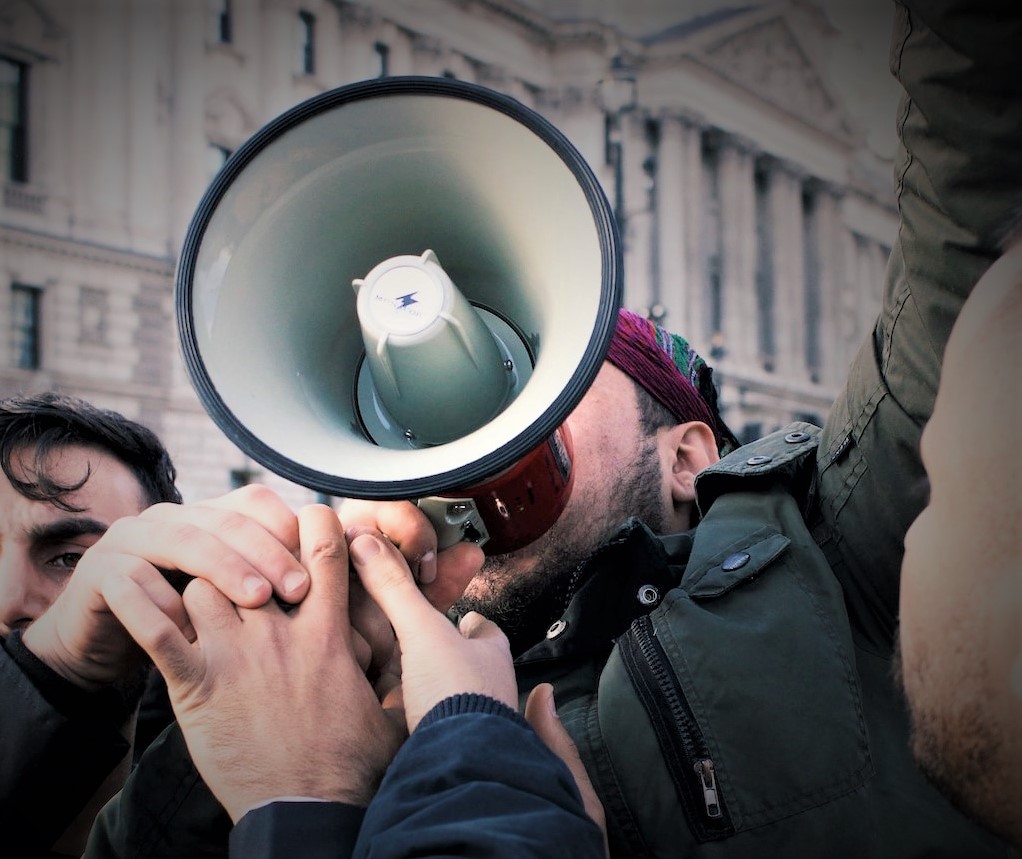
Don’t get me wrong: database companies love slagging each other off. We had so much fun doing it in the 1990s Database Wars that some people never lost the habit. And I’m all for competition, beauty contests and feature battles.
What I’m less keen on is those who provide partial, or inaccurate, or outdated competitive competitor summaries to potential customers. Especially when these customers then make very expensive decisions based on partial, inaccurate, and often outdated, information.
You might score one against the competition this way (ask Spurs—actually, don’t, that’s a very unfamiliar experience for them). But long term, perpetuating the good old IBM FUD (Fear, Uncertainty and Doubt) is bad for the database market and the industry.
It’s not good for CIOs either. If nothing else, it’s a massive time suck. When I was on the other side of the fence and a CTO buyer, I could never trust anything the salespeople said, and I ended up doing double due diligence to work out what was real or not.
And because I couldn’t rely on people to just stick to what they’re good at and leave the opposition to talk about what they’re good at, I ended up duplicating a lot of work. I’d often say, Guys: I’ll buy you next time. For this one, the other mob fit better. We’re all going to win in the end, don’t worry about it.
I’d like to think this carries over into what I do now, which is promoting a tech I really believe in—but which I know is not the only or best solution for every database use case that every possible customer has.
So, I will go out on a limb and say some NoSQL vendors are actively peddling myths about SQL (feeling the heat perhaps?!). This is definitely the case if you hear that you ‘can’t’ use a SQL database for a particular business problem because they’re too ‘rigid’ and they ‘won’t scale’.
I won’t go into it here, but you hear very similar quacking noises from the Graph people, who also claim their brilliant, but very specialised, software is a universal Turing Machine for every problem.
Let’s jump in the database wayback machine
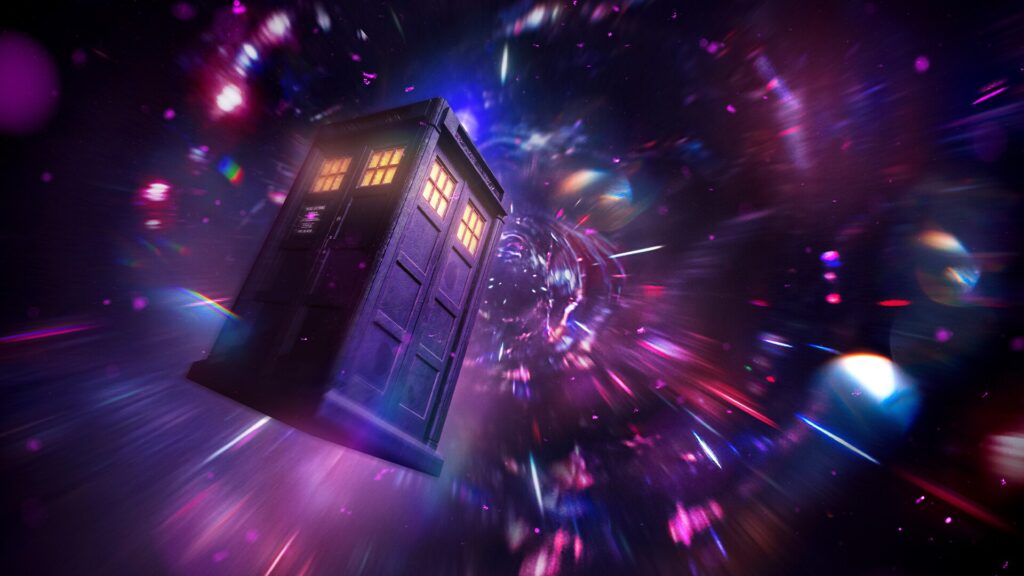
To see why this is a particularly pernicious method of, er, marketing, we need a quick history lesson for context.
Once upon a time, all databases were monolithic, on-prem, and all SQL-based. But, we started to see that they couldn’t handle complex structures—what NoSQL databases called ‘documents.’ This is that sector’s term for a big, hierarchical structure of everything about the customer or product (essentially, any business entity).
When e-commerce was taking off, it turned out that it was a handy thing to have. It meant you had records of everything you knew about the customer, everything they owned, all their orders. Basically, all their history in one handy data structure (a cache) that you could stick in the database, press ‘FIRE,’ and off you went.
Even today, document databases are often the go-to choice. If you want to update information about a user, their preferences, or their wish list, you can do all of that very simply with a document database.
BUT… now you can do it in a SQL database too! A 1990s style SQL database… not so much.
SQL myths and my hairline…
But it’s not the 1990s anymore. (A quick glance at my hairline reminds me of this brutal truth only too well.) SQL has advanced incredibly since then. So, if the document database and other NoSQL people are still trotting this myth out, it must be that they don’t know anything about relational databases, or they are wilfully ignoring huge advances in database technology over the last two decades.
Is this misinformation ignorance or ill intent? I’m sure it’s (mostly) the former.
Saying SQL can’t do 90% of what a NoSQL database can in 2023 is just not true, even for good old monolithic SQL DBs such as MariaDB, MySQL, SQL Server, or even Oracle or PostgreSQL. It’s certainly untrue for Distributed SQL databases such as Cockroach, Spanner, or my company’s YugabyteDB.
Ultimately, NoSQL is great for some things, but not everything. One size does not fit all and when you have a hammer, just because everything looks like a nail does not make it true.
SQL data engines are a helpful solution for a lot of use cases. Any enterprise that is planning a database modernisation project needs to consider more important factors than whether it’s easy to get started on Day 1 (which is why many developers love MongoDB for example). It needs to still be great to work with on Day 365.
They’re also not (and have never been) great with transactional data. If you want to move money from one bank account to another you must be able to update two entities. The account details of customer A and customer B, plus a document approach just doesn’t guarantee that everyone ends up with the correct amount in the end.
So, use NoSQL. It has superb applicability for some things and should be part of your portfolio. Use SQL for other problems. Use graph too. Don’t assume that one approach (or technology) works for everything; for some problems, an abacus is the ideal tool!
BUT always check vendor claims. If they say X can’t do this because of something a bit hand-wavey, push back. That includes claims from my company! Only adopt what makes sense for you, and only ever select the technology most appropriate for the business problem.
As Dr Pepper used to say: What’s the worst that could happen?
Which brings us back to the simple things in life. You’d be mad to listen to a car salesman detail why you shouldn’t buy a competitive car brand. So, why on earth would you give credence to competitive information from a rival database vendor?
At the very least, give both a test drive. You might love the other option… and save a load of money on back-pedalling when it turns out you made the wrong choice.
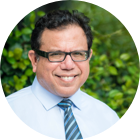
MMS • Anthony Alford
Article originally posted on InfoQ. Visit InfoQ
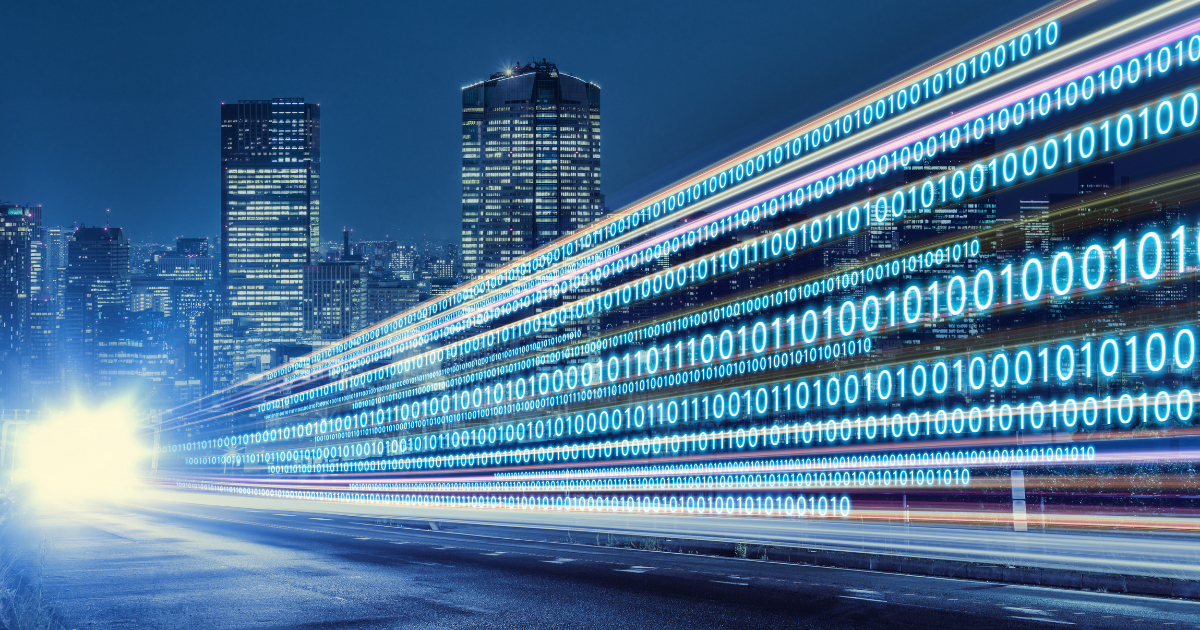
Stability AI released two sets of pre-trained model weights for StableLM, a suite of large language models (LLM). The models are trained on 1.5 trillion text tokens and are licensed for commercial use under CC BY-SA-4.0.
The released models contain 3B and 7B parameters respectively, with larger models soon to come. The new suite of models is a result of Stability’s previous collaboration efforts with EleutherAI; the training dataset is an updated version of EleutherAI’s The Pile dataset, with three times the data used to train EleutherAI’s models. The release also includes versions of the StableLM models that have been fine-tuned on instruction-following and chat datasets, including Stanford’s Alpaca dataset. The fine-tuned models are licensed for non-commercial use only, due to Alpaca’s licensing requirements. According to Stability AI,
With the launch of the StableLM suite of models, Stability AI is continuing to make foundational AI technology accessible to all. Our StableLM models can generate text and code and will power a range of downstream applications. They demonstrate how small and efficient models can deliver high performance with appropriate training…Language models will form the backbone of our digital economy, and we want everyone to have a voice in their design. Models like StableLM demonstrate our commitment to AI technology that is transparent, accessible, and supportive.
The success of generative LLMs such as OpenAI’s GPT-3 spurred the development of smaller open-source models with similar capabilities. In 2022, InfoQ covered the release of EleutherAI’s GPT-NeoX-20B, an open-source 20B parameter LLM; more recently, InfoQ covered Meta’s 7B parameter LLaMA LLM. OpenAI’s release of ChatGPT showed that LLM performance could be improved by fine-tuning them on “instruction-following” datasets, which led to the release of similar models such as Stanford’s Alpaca, a fine-tuned version of LLaMA.
Although only the 3B and 7B parameter StableLM models have been released, Stability AI says that models with 15B, 30B, and 65B parameters are in progress, and a 175B parameter model is planned. The company also says they will be crowd-sourcing an open-source dataset for fine-tuning chatbot assistants, to further the efforts of projects such as OpenAssistant. While Stability AI did not announce any benchmark performance data for the models, they claim “surprisingly high performance in conversational and coding tasks.”
In a Hacker News discussion about the release, one user said:
Selling access to LLMs via remote APIs is the “stage plays on the radio” stage of technological development. It makes no actual sense; it’s just what the business people are accustomed to. It’s not going to last very long. So much more value will be unlocked by running them on device. People are going to look back at this stage and laugh, like paying $5/month to a cell phone carrier for Snake on a feature phone.
Stability’s CEO Emad Mostaque replied to questions about StableLM in an “ask me anything” thread on Twitter. When asked about the hardware used to train the models, he said that they were using “3,000 A100s and 512 TPU v4s.”
Stanislav Fort, LLM lead at Stability, posted a helpful tip on Twitter:
For the early StableLM models, try adding “User: ” to the prompt. Because of the way these models were trained, prepending your evals with “User: ” should make things *much* better.
The code for the StableLM models is available on GitHub. The model weights and a demo chat interface are available on HuggingFace.
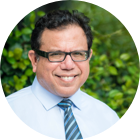
MMS • Leslie Miley
Article originally posted on InfoQ. Visit InfoQ
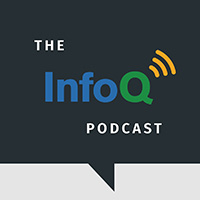
Subscribe on:
Transcript
Introduction [00:44]
Roland Meertens: Welcome everyone to the InfoQ podcast. My name is Roland Meertens, your host for today, and I will be interviewing Leslie Miley. He’s a technical advisor to the CTO at Microsoft and we are talking to each other in person at the QCon London Conference where he gave the keynote on the first day of the conference about AI bias and sustainability. Make sure to watch his presentation as he draws interesting parallels between historical infrastructure projects and modern AI development. During today’s interview, we will dive deeper into the topic of responsible AI. I hope you enjoy it and I hope you can learn something from it.
Welcome, Leslie to the InfoQ podcast. You just gave a keynote talk as QCon London. Could you maybe give a summary of the things you talked about?
Leslie Miley: Thank you for having me, Roland. I’m appreciative of the time and the forum. Wow, that was a really interesting talk. I gave a talk on AI bias and sustainability and how they both are inexorably linked. It’s interesting because when you look at AI and when you look at sustainability, they are both human problems and they can impact humans in many different ways. If we don’t think about the human beings first and human beings that can be impacted more, so marginalized groups, we can really run into problems. Part of the talk was really about showing how the road can be of good intentions. What do they say? The road to hell is paved with good intentions.
The fascinating part of that is we have something in the United States called the interstate highway system that was designed and built in the 1950s and 1960s as 46,000 miles of road that was supposed to connect all of the major cities and create not just a transportation network, but a network for shipments of goods and services. While it did do that, it also cut communities off from each other and it created a huge social problem, huge cultural problem, huge pollution problem. It gave rise to the American car and the American gas guzzler car that increased the amount of CO2 and nitrous oxide and N02 being released, particularly in some of the most disadvantaged communities in the country. I really do think the infrastructure that we’re building for AI and specifically the infrastructure demands for generative AI could be the same thing. We could get a lot of good out of it, but we could also end up impacting vast numbers of people who are already being impacted by the inequities in society.
Trade-offs in AI [03:21]
Roland Meertens: I think that’s really the difficult thing right now, right? All these new technologies, all these new AI technologies are bringing us a couple of good things and a couple of bad things. How would you evaluate this? Do you for yourself have any balance? Do you see some kind of trade-offs which we can take right now?
Leslie Miley: The question I ask, and this goes back to my early days at Twitter when I was running the safety and security group, was how can something be weaponized? Asking that question is not always a straight line answer and sometimes I ask the question and if I come back with I don’t know, then I have to ask the question, who can I ask? I think that’s the question we have to ask ourselves all the time is can this be weaponized? If you don’t know, who would know and how do you get in touch with them and ask them the questions? I think that’s my framework and that’s the rubric I use. I think that open AI, strangely enough, may have done something similar where they understood this in the very beginning and they had a very extensive manual review process for the data that they were collecting and the outputs, so they could tag this.
I think that’s a start, but it wasn’t perfect, but it’s a start. I think that’s what we have to do is as we design these technologies, we ask the harm that they can do before we get really far down the road and then we try to build into the actual development of the technology, the mitigation measures.
Roland Meertens: I think that’s the difficult thing right now with ChatGPT, they built in a couple of safeguards, or at least they tried to build it in. The AI will always say, “I’m a language model and this is not ethical,” but also immediately people started jail-breaking this to try to get it to talk about hijacking a car, trying to get it to give them unethical advice. Do you have any idea of how they should have deployed it then, or how should they have tested or thought about this?
Leslie Miley: Yes, I do actually. I’m not sure if I want to give Sam Altman any free advice. He should be paying me for the advice. I think you have to be inclusive enough in your design from the beginning to understand how it can be used. Of course, people are going to try to jailbreak it. Of course, people are going to try to get it to hallucinate and respond with pithy things or not so pithy things. In the rush to make this technology available, I don’t think that you’ll ever get to a point to where you can’t stop the harm. I’ll use the statement throughout this podcast, which is capitalism is consistent. The rush to market, the desire to be first, the desire to scale first, the desire to derive revenue will consistently overtake any of the mitigation measures that a more measured approach would have.
I think that’s a problem and that’s why I wanted to do this talk because I wanted people to start thinking about what they’re doing and its potential harms even before they begin. I mean, we’ve learned so much in engineering and software development over the last 25 years, and one of the things that we’ve learned, and I’ll try to tie this together, is it’s actually better to test your code as you’re writing it. I mean, think about that. 25 years ago nobody was doing that. Even 20 years ago it was still a very nascent concept and now it’s a standard practice, it’s a best practice and it allows us to write better code, more consistent code, more stable code that more people can use. We need to start thinking along the same lines. What’s the equivalent of writing your test as you write your code to generative AI? I don’t know, but maybe that’s a way to look at it.
Roland Meertens: I think that’s at least one of the things I see in generative AI is that, for example, in the DALL-E interface of open AI, it will come back to you if it detects that you’re trying to generate something it doesn’t like. It also has kind of false positives here, so sometimes it’s let you generate something which is absolutely innocent. But how does one go for the unknown unknowns? Don’t you think that there are always more unethical cases you didn’t think of which you can’t prevent?
Leslie Miley: Yes. Human history is replete with that. I mean, gunpowder was not made to fire bullets. It was made to celebrate. I don’t know where that analogy just came from, but I just pulled it out of the depths of my memory somewhere. I’m sure the people who developed gunpowder were not like, “Ah, how do we keep this safe from people making weapons of mass destruction with it?” I mean, because they were just like, “Ah, this is great. It’s like fireworks.” I don’t think you can, but I think you can make it difficult, and I think you can prompt people, to use a term that’s appropriate here, to subscribe to their better angels. Twitter, before its recent upheaval, and may still have this feature, if you are going to tweet something spicy, it will actually give you an interstitial. I don’t know if you’ve seen that.
I’ve seen it where if I’m responding to a political discourse, and usually my responses aren’t going to be really that far off and that very spicy, but it gave me an interstitial saying, “Hey, are you sure you want to tweet this? It looks like it could be offensive and offend people.” I was just like, “That’s a good prompt for me to maybe get in touch with my better angels as opposed to just rage tweet and put it out there and get engagement.” I really want to kind of come back to that because it’s in response, strangely enough, to the data that shows that negative content has higher engagement on social media.
Roland Meertens: Also, social media started out as something which was fun, where you could meet your friends, you could post whatever you wanted, and it somehow became this echo chamber where people are split up into political groups and really attacking each other.
Leslie Miley: That’s a great analogy. I’d just like to take us to the logical illogical conclusion, perhaps. What happens if what we’re doing with generative AI has a similar societal impact as the siloing of communities, vis-a-vis social media? I mean, that’s actually frightening, because now we just don’t like each other and we go into our echo chambers and every now and then we’ll pop out and we’ll talk about right wing this, left wing that, Nazi this, fascist that. AI can make it a lot worse.
Roland Meertens: You also see that AI could maybe for the same communities help them out a bit. For example, one fear of AI is that it’s possible that the people are a bit poor, their jobs might be taken away. But on the other hand, ChatGPT also allows someone who’s maybe not that highly educated to write a perfect application letter or to get along in a field where previously they would have to … maybe someone who is an immigrant not native with English language and didn’t have that much training can now write perfect English sentences using a tool like ChatGPT. Don’t you think it could also offer them some opportunities?
Leslie Miley: I think so. I’m trying to think of something I just read or listened to. It’s almost like you need a CERN for generative AI to really have something that is for the benefit of humanity. I can see what you say. It’s like, hey, we will help people do this, help people do that. Yes, it has that potential and I would hope that it would do that. I just don’t know if, like I said, we’ll subscribe to our better angels. Perhaps we will, but we also run the risk, and this is part of what my talk was about, was if we deploy this to communities that may be in a different country and have to learn the language or may not have had the best school system, but now have access to basically the sum of knowledge on the entire internet, which I think ChatGPT 4.0 was trained on, the other side of that is if you have a million or five million or 100 million or 200 million people doing that, what’s the carbon cost of that? Where are these data centers going? How much more CO2 are we going to use?
Then you have people, yes, taking advantage of this, hopefully improving their lives, but vis-a-vis their actions, they’re going to be impacting other people’s lives who are also disenfranchised. It’s like, yes, we allow people to own cars and this created mobility and gave people jobs and gave people this, and it pumped a bunch of CO2 into the environment, and we grew these big cities and we grew all this industry and we educated all these people and yes, and we had to build power plants that burn coal to .. it’s like we do this good thing, but then we have this bad thing and then we try to mitigate this bad thing and then we keep repeating this pattern and the mitigating the bad thing never really fixes the original problem.
Responsibility of developers [12:08]
Roland Meertens: Where would you put a responsibility for something like this? For example, in the Netherlands, we always have this discussion about data center for Meta, which all the people in Netherlands are very much against, but at the end of the day, people are still using a lot of Meta products also in the Netherlands, so apparently I think there is a need for a data center if Meta says so, they’re not building it for fun, which you put the responsibility more on the users of the services to reconsider what are they actually using or would you put it on the developers? Where would you put their responsibility to think about this?
Leslie Miley: It’s a collaborative approach. It’s not just Meta and it’s not just your local state or country government. It’s also the community and how do you make sure that everyone’s voice is being heard? That includes if Meta says, “We’re putting this data center in this place and we’re going to use coal to run it”, what about people in the South Pacific whose islands or their homes are going to disappear? How do they get a voice? This is what I mean. The point I was trying to make, I don’t think I really got a chance to make in the talk, is that the choices we make today are no longer confined to our house or our office or our country. They’re worldwide in many cases. What open AI is doing is going to have a worldwide impact, not just in people’s jobs, but also from an environmental standpoint.
The data center buildouts that Meta and Google and Microsoft and everyone else is doing, it’s the same thing, and how do you do that? The reason I’m bringing these questions is because there aren’t answers to them, and I understand that, but there are questions that we, the global we, have to start having a dialogue about because if we don’t, we’ll just continue doing what we’ve always done and then try to fix it on the back end after the harm has been done. I just want us as a species to break that cycle because I don’t think we survive long term as a species unless we do. I know that’s really meta and really kind of far out there, but I fundamentally believe that. It’s a terrible cycle that we see every day and the impact all over the world, and we have to stop it.
Roland Meertens: I think especially breaking out of such a cycle is hard. You mentioned the highway system as something which is great because you can drive all across America, but the downside is, of course, that we destroyed many beautiful cities in the process, and especially America still has many, many problems with massive sprawling suburbs where you can only get by car with a big vehicle emitting CO2. But for example, I know that in many European cities, at some point people learned and they started deconstructing the highways. You also see it, for example, the ring road around San Francisco is not a ring road anymore. Do you have any ideas on how we can learn faster from mistakes we make or how we can see these mistakes coming before they are happening, specifically in this case for AI?
Leslie Miley: I think about that and what comes to mind is the fossil fuel industry and how ridiculous it is that when you have these economic shocks like the illegal invasion of Ukraine by Russia that shoot energy prices through the roof, energy companies make billions in profits, tens of billions, hundreds of billions in profit. I’m like, “Well, there’s a wrong incentive. It is a totally wrong incentive.” What could happen is that you could actually just take the money from them and just say, “You don’t get to profit off of this. You don’t get to profit off of harm. You don’t get to profit off of the necessity that you’ve created and that the people have bought into.” I think that may be what we have to do, and it’s the hardest thing in the world to do is to go to somebody and say, “Yeah, I know you made all this money, but you can’t have it.”
Roland Meertens: How would you apply this then to AI companies? How would you tell them what they can profit from and what they cannot profit from?
Leslie Miley: Well, maybe let’s speed up on Meta or maybe even Twitter at this point. I think Meta is a little bit better. I think you can look at Meta and you can say it is demonstrably provable that your ad-serving radicalizes people, that your ad-serving causes bad behavior and that it causes people to take some horse dewormer instead of getting a COVID vaccine, so we’re just going to start finding you when your platform is used against the public good. Yes, I know the public good is probably subjective, but I also think that at some point we just have to start holding companies responsible for the harm they do. The only way to hold companies responsible for the harm they do is to take their money and not a $100 million fine or 200, just like a five or 10 or $50 billion fine and make it so that companies are incentivized to it at a minimum not do the wrong thing.
Meta knows, their CTO said this. He’s like, “Our job is to connect people. If that means that people die, okay, as long as we connect them. If people use our platform to kill each other, that’s okay as long as they’re connected.” This is a quote from him. I’m like, “Oh, no time out Sparky. You can’t say that,” and as a government we should be like, “No. No, you don’t get to profit from that. You don’t get to profit from amplifying the hatred that causes a genocide in a country. You don’t get to profit off of misinformation that causes people to take action against their own health. You just don’t get to profit from that, and we’re going to take your profit from that.” I almost assure you if you did that, the companies of course would fight it, but if you did that, they would probably change their algorithms.
Balancing short-term and long term incentives [17:41]
Roland Meertens: But how would you, for example, balance this between the short term and the long term? If we look, for example, at ChatGPT, I think in the short term, it seems to help people write better letters, students can learn, so amazing, but we can probably already see coming that there will be a massive influx of spam messages, massive long texts being sent to people. It can be used in an evil way to maybe steer the discourse you were talking about on Twitter between groups to automatically generate more ideas and weird ideas and actually put these ideas on paper. How would you balance the short term goods and the long-term goods between the maybe short term evil and the long term evil?
Leslie Miley: This is where I think open sourcing algorithms help, open sourcing what these models are being trained on. If you deploy a model and it goes kind of up and to the right and you start to be able to realize significant revenue from this, that starts impacting society, and that can be defined as a town, a city, or a country. I think that’s when the discussion starts of what does this mean? I don’t think it’s mutually exclusive. You can have a Twitter without it being a honey pot for assholes. You can have a Meta without it being a radicalization tool for fascist. You can have a DALL-E or a Midjourney that doesn’t create child exploitation images. That’s all possible. When it happens and there’s no back pressure to it, and by back pressure, I mean regulation, companies are going to act in their best interest and changing an algorithm that …
As I said, Meta knows their algorithm, they know what their algorithm does, but they also know that changing it is going to hit their other metrics and so they don’t change it. I think these are the things that we have to push back against. Once again, these companies, and I’ve worked at most of the ones we’re talking about, love to say that they have the smartest people in the world. Well, if you can’t figure out how to continue to make money without child exploitation, maybe you don’t deserve to make money.
Open source AI [19:42]
Roland Meertens: But for example, for the open sourcing, don’t you think there’s also inherent risks and problems where, for example, if I were to be a bad actor, I can’t really use OpenAI’s DALL-E to generate certain images. I could download Stable Diffusion, which has a certain level of security in there where it gives you an image of Rick Astley actually if you try to generate something weird.
Leslie Miley: You get Rickrolled. Wow, that’s great,
Roland Meertens: You get Rickrolled. But the problem is that you have to uncomment one or two lines to bypass the security. So in there you see that some malicious actors actually use the open source of software instead of the larger commercial companies because the open source software allows them to train it on whichever artists or whichever images they want.
Leslie Miley: I would like to think that that will always be the exception and not the rule, and that will always exist, but it won’t scale. That is my hope. I think that we have a lot of evidence that it won’t scale like that. Will people use it to do that? Yes. Do people use 3D printers to print gun parts today? Yes. Do you ban 3D printers? No. Do you try to install firmware in them so that they can’t do that? I don’t think that’s even possible, or somebody will just figure out how to rewrite the microcode. But I think the great part about it is it just doesn’t scale. If it doesn’t scale, then yes, you’ll have it, but it won’t be at a Meta level, it won’t be at a Google level, it won’t be at a Twitter level.
Roland Meertens: You not afraid that at some point some company will create a bad GPT, which will actually answer to all your unethical questions, but at two times the price and people will switch to that whenever they want to create something evil or people will switch to bad GPT just in general because it gives them more answers every time instead of always having a lecture about that it’s just a large language model.
Leslie Miley: I mean, we shut down Silk Road. I mean, it’s like we have a way to go after bad actors, and I think that it’s always a game of cat and mouse. Like I said, being in safety and security at Twitter, I called it we had to play a global game of Whack-a-mole and the only way you could even begin to make a significant difference is that you need an army of trained octopus to play the game. Because they’re just popping up everywhere and we’ll always be behind, because as ubiquitous as these tools become, and as easy as they are to use, anyone can do it. There used to be a higher bar of entry because having a computer, they cost so much, you didn’t do this. Then having fast internet access is a little bit too much. But now it’s like a lot of people can do this development on their iPad or on a tablet or on $150 Chromebook or a $80 Chromebook for that matter.
Then you have labor costs that in some countries is just ridiculously cheap, or just people just sitting there. The internet research agency in Russia really showed us what a troll farm could do, and this is now that troll farm that’s being replicated all over the world. People just throw them in. So my whole point is you will always have bad actors. Bad actors will always find a way to exploit the tools. The point that we have to look at is what type of enforcement do you have that will limit the impact of that? I think that we’ve gotten better at that over the years. Yes, but we’re still going to have the dark web. You’re still going to be able to do things like this if you’re sufficiently motivated. Yes, somebody will figure out, or somebody will release a model that will allow you to create your version of an explosive or your version of something bad, and there will be a lot of naval gazing as to what we should do and the answer to the question, which won’t ever happen, which is you don’t let tools like that out.
Individual responsibilities [23:25]
Roland Meertens: So maybe if we bring it back to the audience of this podcast, the software engineers, what could developers introduce in the routine? Are there a couple of things which we can do as a community to prevent this? Is there something which we can as individuals do against AI bias?
Leslie Miley: This is interesting. I was just saying for startup founders like, “Oh, talk to your customer,” and you got to get in front of your customer and you should talk to your customer on a regular basis. I fundamentally believe that, and I think that’s part of the answer is you talk to your customer. But I think the other answer is talk to the people who could potentially be impacted or who know what that impact feels like and looks like. We got better at mitigating abuse and harmful content on Twitter once we engaged the communities that were being impacted by it. That’s what you do. You have to engage those communities. You have to engage them authentically. You just can’t like send them a form to fill out. You have to sit with them and you have to hear what they have to say, and you have to have empathy for the impact it has.
There are many people who are just like, “Well, you just don’t have to look at it.” It’s like, “Oh, somebody send you a DM you don’t have to look at that.” It’s like, yeah, if somebody … I mean, I’ve gotten bad, terrible tweets. I’ve gotten hate mail. I’ve had people threaten me for some of the things I’ve said online, and it impacts you. If someone’s creating a new product and they talk to people outside of their network who could potentially be negatively impacted, they will learn a lot. I think that’s the part that is sometimes missing. In our rush to scale, in our rush to get a product out, we don’t connect with human beings and we don’t connect with our customers in a human way. We don’t connect to the people who could be potentially impacted. In many ways, and I made this example, people don’t care.
Just this amazing example out of the talk was this guy named Robert Moses, who designed part of the highway system in the United States so that bridges were too low so that buses from neighborhoods with minorities couldn’t go to the beach. I mean, people will be bad actors and they’ll do it at scale if you let them, but if you were to take this dude and he wasn’t a violent racist, but if you were to take other people and say, “Look at the impact that this is going to have”, maybe they just were like, “Hey, yeah, we’re not going to listen to this guy. We’re going to do something different.”
Roland Meertens: Maybe that’s another problem that people are often trying to improve the entire world at once, which then often means the entirety of the rich people in America who can afford your app. But for example, whenever I’m in Silicon Valley, I’m always so disappointed that these people are trying to improve the entire world, but they can’t even solve the homelessness of the person in front of the office.
Leslie Miley: Living in San Francisco for the last decade, that resonates and it’s almost as if the problem of the unhoused in San Francisco portends the downfall of tech, because as the homeless problem has become more acute, tech’s issues have become more acute. I think you’re right. If you can’t take care of your house, how can you take care of the world? And San Francisco’s tech’s house? For somebody who likes it, I’m a native, I mean, this is my home, I’m disgusted. I’m not disappointed, I’m disgusted. I’m disgusted with our political leaders. I’m disgusted with our business leaders who have put the time and effort into extracting money out of a system and wealth out of a system, and really at the expense of the humanitarian crisis that runs on the streets of San Francisco on a daily basis.
I mean, I would love tech to be like, “Hey, let’s work with cities and organizations that are solving this problem, measurably solving this problem to amplify it”, and then maybe the humanity that we’ll get in touch with will help us build better products.
Roland Meertens: I’m just going to repeat the same question again, but are there specific things where you say developers should do this? Should they vote with their time? Should they raise problems?
Leslie Miley: Take a humanities course. Somebody said that. Take a humanities course. What can you do? This is not a technical problem to solve. This is a human problem. I think that it’s like how do you get more in touch with your humanity? When was it ever okay that you had to step over someone to go in your office? But people do that every day in San Francisco, maybe not so much today with San Francisco not going back to the office, but we’re okay with that, and that’s the problem. I just don’t get how we can be okay with saying, “We’re changing the world,” when there are people outside who are in crisis that you have no desire to address. It is hypocritical, there’s a cognitive dissonance there that I can’t get, and I’ll bring this a little closer to home. As I said, I’m a native, but right now I have packed up my place, put all my stuff in storage, and I’m out of San Francisco.
I actually do not have an address in California for the first time in, I don’t know, 20 years. Because I was just like, “I can’t solve this,” and it’s an assault on all of my senses, and I’m paying taxes in a place that is not addressing the problem. I’m watching wealth get extracted by tech for the last 20 years that hasn’t measurably addressed this problem. Some people have. I give Mark Benioff the credit for sponsoring initiative that raises $300 million a year for unhoused services in San Francisco. So that’s part of doing the problem. For whatever you want to say, that’s a huge, huge, huge initiative that he took on to try to make the city that he’s built a big giant tower in more livable for everyone. We need more of that though, because it’s just not Benioff, it’s Bob, it’s just not Bob, it’s Mary. It’s not Mary, it’s everyone who lives there has a say in this.
I don’t want to spend too much time on that, but I think it’s developers and product managers and co-founders have a responsibility to understand the impact of what they’re doing. If they don’t understand, if they don’t think they know, they need to go and learn. ChatGPT could probably help you. Personally, I’d rather you go out and talk with someone. I’d rather you go out and talk with people who are impacted by these types of problems and learn what that impact is and not just, “Hey, can we get product market fit?” Because sometimes product market fit isn’t what you should do. Maybe what you should do is ask what that impact is going to be and build a product that makes it harder for people to be bad actors.
Assholificiation of Technology [29:41]
Roland Meertens: Then last but not least, maybe to put it a bit broader, you also want to say something about how the bigger tech companies are behaving nowadays.
Leslie Miley: Oh, gee, we can just go on and on about this. The Silicon Valley Bank is an interesting story, and it’s an interesting story because here was an entity that almost every co-founder, startup co-founder, would tell you, “This bank had our back. This bank had our back. When we needed funding, we got debt financing, we got bridge funding, we got this, we got …” Silicon Valley was the savior for many, many co-founders and for many startups and Silicon Valley was killed by the very people it helped. I mean just like that’s crazy town. It’s like, “Hold it.”
Any number of Peter Teal backed companies probably got some bridge financing from Silicon Valley Bank. Yes, companies have to do the right thing, and if their money’s there, they think they’re not going to get their money. Maybe they have to do the right thing. But the other side of it is also the collective wealth of the people who were tweeting about Silicon Valley Bank was enough to keep Silicon Valley Bank afloat, which to me is just … it’s once again hypocritical in tech. I think there’s just this … I call it the assholification of tech these days. I think there are people who just are really just in it for themselves and they will burn the house down that they’re in. Or if they’re not burning their own house down, they’ll burn the house down of the people they say they support are in, which is almost as bad.
I don’t know how we got there. It looks to me so much like the financial crisis that hit New York banking in the ’80s or ’90s where people just fell upon themselves like a pack of jackals. Is that what’s happening in Silicon Valley? Is this who we’ve become? I guess the answer is yes, if you’re ready to take down the bank, that may have helped you, which many people did. Then I ask, what’s the consequence of this type of behavior? Or what’s the consequence of Elon Musk lying his way through Twitter. He’s like, “Oh, we’re only going to lay off this many people,” and he lays off more people. Or, “We’re going to do give you less ads,” and well, I haven’t gotten less ads and we’re going to do all these things and he treats people like crap and they just get fired and they don’t know they get fired and he abuses somebody who has mobility issues online and makes fun of him and there doesn’t seem to be any blowback.
I mean, he is valuing his company for $24 billion less than he just paid for it, so maybe that is the blowback. But I wonder if that bad behavior is being mimicked in other ways. People are now kind of flipping the bit and saying, “We need to treat workers like workers, and that means that they need to be in the office. That means that we need to be able to keep track of their time.” Are we just turning into a bunch of assholes? I kind of think we are. I mean, Mark Benioff said this, I’m going to bring him up again, he’s like, “There are a lot of tech CEOs right now who are asking if they should get in touch with their inner Elon Musk.” I’m like, “Ooh, that’s rough,” and I think he’s projecting, right? Because he’s doing a lot of the same thing. He’s telling workers that they have to come back and he’s laying off people and he wants to score people’s productivity.
Then other companies are doing the same thing. Mark Zuckerberg, some of his statements, and you could see it. Early on he was like, “Some of you shouldn’t even be here.” I mean, he said that last year all the way to his year of efficiency. I’m like, “Well, if it’s a year of efficiency, what about that $10 billion you just spent on the Metaverse? That didn’t seem too efficient. But you just are essentially telling people they don’t have a job anymore because of your mistakes and you are unapologetic about it.”
Roland Meertens: It seems to be a great way to end the podcast. Thank you very much, Leslie, for being here today.
Leslie Miley: No, thank you for having me.
Note: Roland Meertens works as a Machine Learning Scientist at Bumble Inc. The views he expresses here are his own, and not those of his employer.
.
From this page you also have access to our recorded show notes. They all have clickable links that will take you directly to that part of the audio.
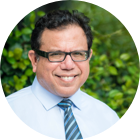
MMS • Steef-Jan Wiggers
Article originally posted on InfoQ. Visit InfoQ
AWS recently announced Amazon OpenSearch Ingestion, a capability of Amazon OpenSearch Service that provides a serverless, auto-scaled, managed data collector that receives, transforms, and delivers data to Amazon OpenSearch Service domains or Amazon OpenSearch Serverless collections.
Muthu Pitchaimani, a Search Specialist with Amazon OpenSearch Service, explains in an AWS Big Data blog post:
OpenSearch Ingestion is powered by Data Prepper, an open-source, streaming ETL (extract, transform, and load) solution that’s part of the OpenSearch project. When you use OpenSearch Ingestion, you don’t need to maintain self-managed data pipelines to ingest logs, traces, metrics, and other data with OpenSearch Service. Amazon OpenSearch Ingestion responds to changing volumes of data, automatically scaling your ingest pipeline.
Source: https://aws.amazon.com/opensearch-service/features/ingestion/
The company claims that OpenSearch Ingestion can be an alternative for managing Logstash or other streaming data pipelines since it removes the complexities of managing a multi-node cluster for data ingestion like choosing the suitable instance types, applying security patches, and adding or removing nodes to optimize for data volume fluctuations.
Besides scaling and not having to manage the infrastructure for data ingestion, the service is also cost-effective; users only pay for the amount of data ingested and stored. In addition, the service provides robust security features, including data encryption at rest and in transit, enabling users to maintain the security of their data. And finally, Amazon OpenSearch Ingestion can easily integrate with other AWS services, such as Amazon S3 and AWS Lambda.
Developers can create a pipeline in the AWS Management Console and define their source, processors, and destination cluster or collection. In addition, they can also start from a blueprint for the most common ingestion use cases.
During creating the pipeline, a developer can specify the capacity value for Ingestion- OpenSearch Compute Units (OCU) and edit the hosts, aws.sts_role_arn, and region fields of the OpenSearch Service sink. A sample trace pipeline can look like this:
version: "2"
entry-pipeline:
source:
otel_trace_source:
path: "/${pipelineName}/v1/traces"
processor:
- trace_peer_forwarder:
sink:
- pipeline:
name: "span-pipeline"
- pipeline:
name: "service-map-pipeline"
span-pipeline:
source:
pipeline:
name: "entry-pipeline"
processor:
- otel_trace_raw:
sink:
- opensearch:
hosts: [ "https://search-mydomain-1a2a3a4a5a6a7a8a9a0a9a8a7a.us-east-1.es.amazonaws.com" ]
aws:
sts_role_arn: "arn:aws:iam::123456789012:role/Example-Role"
region: "us-east-1"
index_type: "trace-analytics-raw"
service-map-pipeline:
source:
pipeline:
name: "entry-pipeline"
processor:
- service_map_stateful:
sink:
- opensearch:
hosts: [ "https://search-mydomain-1a2a3a4a5a6a7a8a9a0a9a8a7a.us-east-1.es.amazonaws.com" ]
aws:
sts_role_arn: "arn:aws:iam::123456789012:role/Example-Role"
region: "us-east-1"
index_type: "trace-analytics-service-map"
Note that there are several competing services in the market that offer similar functionality to Amazon OpenSearch Ingestion such as Google Cloud Dataflow, Microsoft Azure Stream Analytics, Confluent, and Elastic Cloud.
Amazon OpenSearch Ingestion is currently available in several AWS regions globally, and the pricing details can be found on the Amazon OpenSearch pricing page (Ingestion section). In addition, developers can find more guidance through the getting started tutorials.
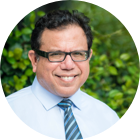
MMS • Dominick Blue
Article originally posted on InfoQ. Visit InfoQ
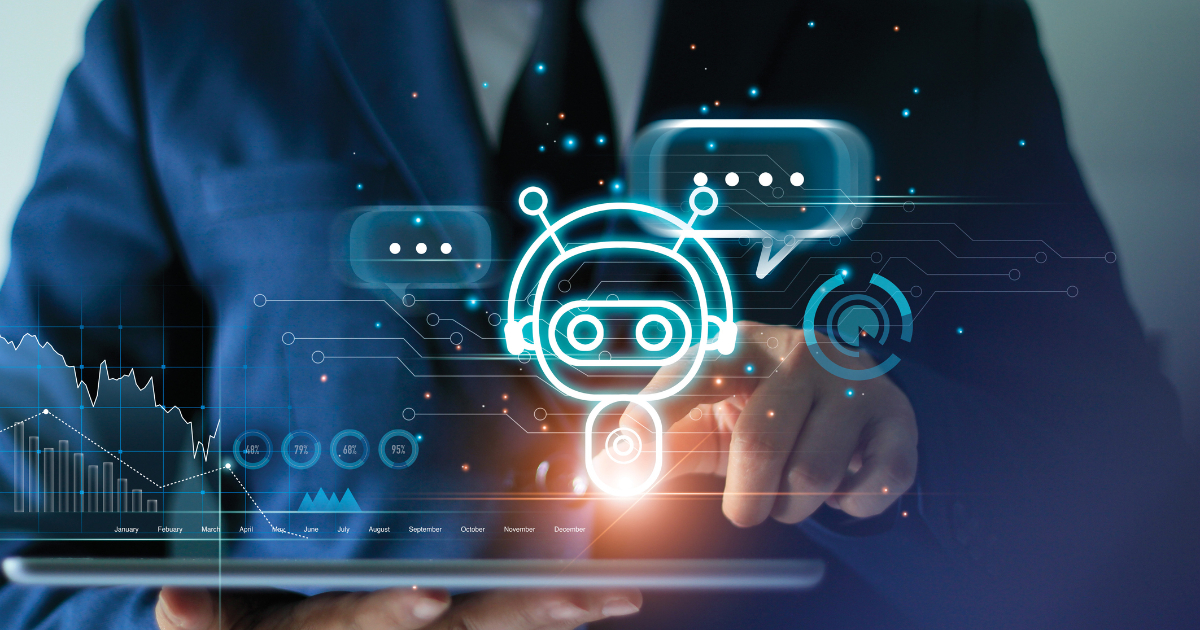
Key Takeaways
- AIOps can simplify and streamline processes which can reduce the mental burden on employees
- Another benefit is improved communication and collaboration between departments leading to more efficient use of resources and reduced budget overhead
- AIOps can simplify implementing measures to minimize downtime, such as improving maintenance schedules or upgrading equipment
- AIOps can improve customer satisfaction and enhance customer trust while reducing service disruptions.
It was in the 20th century when software began eating the world. In today’s 21st-century environment, its appetite has turned to humans.
Whether it is financial systems, governmental software, or business-to-business applications, one thing remains: these systems are critical to revenue, and in some cases, to human safety. They must remain highly available in the face of technological, natural, and human-made adversity. Enter the Site Reliability Engineer or SRE.
The SRE model was born out of Google when Ben Treynor Sloss established the first team in 2003:
Fundamentally, it’s what happens when you ask a software engineer to design an operations function … So SRE is fundamentally doing work that has historically been done by an operations team, but using engineers with software expertise, and banking on the fact that these engineers are inherently both predisposed to, and have the ability to, substitute automation for human labor.[1]
Since its inception, engineering organizations have adopted this model in various ways, yet the fact remains the same. These engineers support revenue and business-critical operations 24x7x365.
It is challenging to locate, hire, and train SREs. In an ever-changing landscape of infrastructure and buzzwords, it begs the question of how to scale these teams sustainably to ensure the well-being of the team and the continuity of operations. Enter AIOps.
AIOps, or artificial intelligence for IT operations, is a set of technologies and practices that use artificial intelligence, machine learning, and big data analytics to improve the reliability of software systems. AIOps enables cognitive stress reduction, increased cross-functional collaboration, decreased downtime, increased customer satisfaction, and reduced cost overhead.
Reducing Cognitive Overload
The on-call engineer’s cognitive stress problem comes in two forms: alert vs. signal noise and information retrieval.
For anyone who has ever held the proverbial pager (we don’t still use real pagers, do we?), the noise versus signal problem immediately comes to mind when considering cognitive stress factors. This problem explores the balance between actionable alerts and alerts that are too sensitive or noisy. This creates a symptom called alert fatigue.[2]
One of the critical benefits of AIOps is cognitive stress reduction. AIOps systems can automatically identify and diagnose issues and can even predict potential problems before they occur. This can reduce the cognitive load on SRE teams, allowing them to focus on more business-aligned project work rather than spending their time troubleshooting issues.
Additionally, AIOps systems can assist with the “front door problem” associated with incident triage. Monitoring systems have millions of data points they collect. The quality of information associated with the alert received is human-dependent. Often, this generates a single question when an SRE begins system triage:
“Where do I begin looking to understand the potential blast radius better?”
AIOps systems can assist with this initial triage measure by analyzing potential anomalies in system state and or telemetry data and providing both potential areas to focus on, as well as complimentary documentation sourced on the organizational intranet.
SREs must begin thinking about how to empower the adoption of AIOps in their organizations. While this is yet another tech stack SREs need to learn, the benefits can have exponentially positive results in reducing their overall cognitive load.
Enhancing the Cross-Team Engagement Model
AIOps (Artificial Intelligence for IT Operations) can significantly improve cross-functional engagement in a business. In traditional IT operations, different teams may work in silos, resulting in communication gaps, misunderstandings, and delays in issue resolution. AIOps can help bridge these gaps and facilitate collaboration between different teams.
One way AIOps improves cross-functional engagement is through its ability to provide real-time insights and analytics into various IT processes. This enables different teams to access the same information, which can help improve communication and reduce misunderstandings. For example, the data provided by AIOps can help IT teams and business stakeholders identify potential issues and proactively take action to prevent them from occurring, leading to better outcomes and higher customer satisfaction.
Another way AIOps improves cross-functional engagement is through its ability to automate various IT processes. By automating routine tasks, AIOps can free up time for IT teams to focus on strategic initiatives, such as improving customer experiences and innovating new solutions. This can lead to improved collaboration between IT teams and business stakeholders. Both groups can work together to identify areas where automation can be implemented to improve efficiency and reduce costs.
Overall, AIOps can improve cross-functional engagement by providing real-time insights and analytics, automating routine tasks, and enabling collaboration between different teams. By breaking down silos and improving communication between IT and business stakeholders, AIOps can help businesses deliver more reliable and efficient IT services, leading to better outcomes and higher customer satisfaction.
Reducing Downtime Throughout the SDLC
Another critical benefit of AIOps is decreased downtime. The nature of diagnosing a system degradation or failure involves the performance of computing systems within a constrained environment. The thousands of data inputs involve humans-in-the-loop (HIL) to design additional systems to alert an engineer based on a given set of metrics. Furthermore, the process extends further when an engineer has to read and interpret the data presented to them after an alert is triggered.
Metrics such as time-to-detection and time-to-resolution are an aggregate evaluation of an engineering team’s effectiveness at receiving, interpreting, triaging, and resolving such incidents. All of this can be drastically improved upon by implementing an AIOps system. In critical environments, it may be necessary to maintain a HIL to decide what actions to take inside a company’s infrastructure. All of this can be drastically improved upon by implementing an AIOps system. An AIOps system can intelligently and diligently analyze the streams of data points it ingests consistently while auto-remediating on less critical issues without the interference of a human, while only alerting for the highest severity issues.
Happy Customers, Happy Life
From a customer perspective, AIOps can have a significant impact on their satisfaction with the services they receive. For example, AIOps can help businesses proactively identify and resolve issues before they impact customers. This means that customers are less likely to experience service disruptions or downtime, resulting in improved availability and reliability of services. Additionally, AIOps can help businesses improve the speed and accuracy of incident resolution, which can help minimize the impact of incidents on customers.
Another benefit of AIOps is that it can help businesses identify and resolve issues more quickly, leading to shorter resolution times. This can be particularly important for customers who are experiencing critical issues or downtime. By resolving these issues faster, businesses can minimize the impact on customers and reduce the risk of customer churn.
Overall, AIOps has the potential to significantly improve customer satisfaction by helping businesses deliver more reliable and available IT services, faster incident resolution times, and shorter resolution times. As a senior software engineer, I believe that AIOps is a powerful approach to IT operations that can help businesses stay ahead of the curve in today’s fast-paced and competitive market.
Patching the Leaky Bucket
AIOps can help automate and optimize various IT processes, including monitoring, event correlation, and incident resolution. By automating these processes, AIOps can reduce the need for manual intervention, which can help reduce labor costs. Additionally, by optimizing these processes, AIOps can help companies reduce the time and resources required to manage IT operations, leading to overall cost savings.
This can help companies reduce the number of service disruptions and outages, which can lead to significant cost savings. Downtime and service disruptions can be costly for businesses, resulting in lost productivity, revenue, and customer satisfaction. By detecting and resolving issues before they impact services, AIOps can help minimize the risk of service disruptions and downtime, leading to cost savings for the business.
Additionally, AIOps can help businesses improve their overall IT infrastructure and application performance. By providing real-time insights into application and infrastructure performance, AIOps can help companies optimize their resources and reduce inefficiencies. This can lead to cost savings by reducing the need for additional hardware and software resources.
A quick internet search will reveal the average salary of a Software Engineer in the United States is $90,000 – $110,000 USD. This roughly equates to $47 – $57 an hour. Imagine, on average, your incidents involve five engineers, and it takes you three hours to resolve an issue. That means your incidents cost you $705 – $855 per incident. Now imagine you have three incidents a month, bringing you to approximately $30,780 a year in costs. This doesn’t include any customer revenue loss or the intangible costs of losing customer trust. There are a few essential questions to ask yourself to get a rough estimate of how much an incident costs your company.
- How much are engineers paid at my company?
- How many incidents do we have in a year?
- How long does it take us to resolve those incidents?
- What are the intangible costs to our company because of incidents?
Once you do this back-of-envelope math, you’ll quickly understand how even a 10% decrease in incidents will save your company an impressive amount of money on the bottom line.
Where to Start
The truth is, adopting AIOps is a long journey for any organization. However, with persistence and focus, a company can realize the benefits discussed earlier in this article. Here are a few considerations to get started on your adoption of AIOps.
- Define your goals: The first step is to determine what you want to achieve with AIOps. This can include reducing downtime, improving incident response times, or optimizing resource utilization.
- Assess your current IT infrastructure: Before implementing AIOps, you need to understand your existing IT infrastructure, including the tools and technologies you currently use. This will help you identify any gaps that AIOps can fill and ensure that your AIOps program integrates smoothly with your existing systems.
- Choose an AIOps platform: There are many AIOps platforms available in the market. Evaluate different options and choose a platform that aligns with your goals and IT infrastructure. Look for features such as automated root cause analysis, anomaly detection, and machine learning algorithms.
- Identify data sources: AIOps platforms require a significant amount of data to operate effectively. Identify the data sources you will need to collect, such as log files, performance metrics, and configuration data.
- Develop a data strategy: Determine how you will collect, store, and manage the data required for AIOps. This includes deciding on data retention policies, data security measures, and data access controls.
- Train your AIOps platform: Once you have set up your AIOps platform and data strategy, you will need to train the platform to recognize patterns and anomalies in your IT infrastructure. This involves feeding historical data into the platform and tweaking the algorithms to optimize performance.
- Integrate with your IT operations: Finally, you will need to integrate your AIOps program with your IT operations. This includes setting up workflows for incident management, change management, and resource allocation.
Conclusion
In conclusion, AIOps is a set of technologies and practices that use artificial intelligence, machine learning, and big data analytics to improve the reliability of software systems. AIOps enables cognitive stress reduction, increased cross-functional collaboration, decreased downtime, increased customer satisfaction, and reduced cost overhead. These benefits can be achieved by automating incident management processes, providing real-time visibility into the performance of software systems, and optimizing resource allocation.
References
- Google Interview
- Want to Solve Over-Monitoring and Alert Fatigue? Create the Right Incentives!” [Kishore Jalleda, Yahoo, USENIX SREcon17]
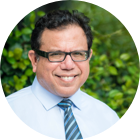
MMS • RSS
Posted on nosqlgooglealerts. Visit nosqlgooglealerts
A System Design Guide
SQL vs. NoSQL: Navigating Database Choices for System Design Interviews.
When it comes to system design interviews, selecting the right type of database is crucial, as it forms the foundation for data storage, retrieval, and manipulation in most applications.
Employers expect candidates to have a good understanding of database technologies and be able to choose the appropriate one for a given scenario. Being able to select and justify the right database can easily distinguish you from other candidates.
This comprehensive blog aims to provide you with a solid understanding of SQL and NoSQL databases, their respective advantages and disadvantages, and how to choose the most suitable one for a given scenario.
Overview of SQL and NoSQL databases
Databases can be broadly classified into two categories: SQL (Structured Query Language) and NoSQL (Not only SQL) databases. SQL databases, also known as relational databases, are based on the relational model, where data is stored in tables with predefined schema and relationships between them. Some popular SQL databases include MySQL, PostgreSQL, Microsoft SQL Server, and Oracle. SQL databases are known for their consistency, reliability, and powerful query capabilities.
On the other hand, NoSQL databases are a diverse group of non-relational databases that prioritize flexibility, scalability, and performance under specific workloads. NoSQL databases can be further categorized into document databases, key-value stores, column-family stores, and graph databases, each with their unique data models and use cases. Some widely-used NoSQL databases are MongoDB, Redis, Apache Cassandra, and Neo4j.
Understanding SQL Databases
A. Definition and key characteristics
SQL (Structured Query Language) databases, also known as relational databases, are built on the relational model, which was first introduced by E.F. Codd in 1970. The relational model represents data in the form of tables, also known as relations, with rows (tuples) and columns (attributes). Each row represents a unique entity, and each column represents an attribute of that entity. The primary advantage of SQL databases is their ability to maintain data consistency and integrity through constraints, relationships, and transactions.
Relational databases use SQL as their primary query language for defining, manipulating, and querying data. SQL is a powerful and expressive language that allows developers to perform complex operations on the data, such as filtering, sorting, grouping, and joining multiple tables based on specified conditions.
B. Common SQL databases
There are several popular SQL databases available, each with its unique features, performance characteristics, and ecosystem. Some of the most widely-used SQL databases include:
- MySQL: An open-source, highly scalable, and widely-used relational database management system (RDBMS). MySQL is known for its ease of use, robustness, and strong community support.
- PostgreSQL: Another open-source RDBMS that focuses on extensibility, standards compliance, and performance. PostgreSQL is well-regarded for its advanced features, such as support for custom data types, full-text search, and spatial data operations.
- Microsoft SQL Server: A commercial RDBMS developed by Microsoft, featuring a comprehensive set of tools and features for enterprise-level applications. SQL Server is known for its tight integration with other Microsoft products, security features, and business intelligence capabilities.
- Oracle: A widely-used commercial RDBMS that offers high performance, advanced features, and scalability. Oracle is popular in large organizations and mission-critical applications due to its robustness, reliability, and comprehensive toolset.
C. Pros and cons of using SQL databases
- ACID properties and consistency: SQL databases adhere to the ACID (Atomicity, Consistency, Isolation, Durability) properties, which ensure the reliability of transactions and the consistency of the data. These properties guarantee that any operation on the data will either be completed in its entirety or not at all, and that the data will always remain in a consistent state.
- Structured schema: SQL databases enforce a predefined schema for the data, which ensures that the data is structured, consistent, and follows specific rules. This structured schema can make it easier to understand and maintain the data model, as well as optimize queries for performance.
- Query language and optimization: SQL is a powerful and expressive query language that allows developers to perform complex operations on the data, such as filtering, sorting, grouping, and joining multiple tables based on specified conditions. SQL databases also include query optimizers, which analyze and optimize queries for improved performance.
- Scalability and performance: SQL databases can be scaled vertically by adding more resources (such as CPU, memory, and storage) to a single server. However, horizontal scaling, or distributing the data across multiple servers, can be more challenging due to the relational nature of the data and the constraints imposed by the ACID properties. This can lead to performance bottlenecks and difficulties in scaling for large-scale applications with high write loads or massive amounts of data.
In conclusion, SQL databases provide a robust, consistent, and powerful solution for data storage and manipulation in many applications. They are particularly well-suited for scenarios where data consistency, relationships, and complex queries are of paramount importance. However, their scalability limitations and rigid schema requirements may make them less suitable for some use cases, such as large-scale applications with high write loads or rapidly-evolving data models. In these
Understanding NoSQL Databases
A. Definition and key characteristics
NoSQL (Not only SQL) databases are a diverse group of non-relational databases designed to address the limitations of traditional SQL databases, particularly in terms of scalability, flexibility, and performance under specific workloads. NoSQL databases do not adhere to the relational model and typically do not use SQL as their primary query language. Instead, they employ various data models and query languages, depending on the specific type of NoSQL database being used.
The key characteristics of NoSQL databases include their schema-less design, which allows for greater flexibility in handling data; horizontal scalability, which makes it easier to distribute data across multiple servers; and their ability to perform well under specific workloads, such as high write loads or large-scale data storage and retrieval.
B. Types of NoSQL databases and their use cases
NoSQL databases can be broadly categorized into four main types, each with its unique data model and use cases:
- Document databases: These databases store data in a semi-structured format, such as JSON or BSON documents. Each document can contain nested fields, arrays, and other complex data structures, providing a high degree of flexibility in representing hierarchical and related data. Document databases are well-suited for applications with diverse and dynamic data models, such as content management systems, user profiles, and event logging. Some popular document databases include MongoDB and CouchDB.
- Key-value stores: Key-value databases store data as key-value pairs, where the key is a unique identifier and the value is the associated data. These databases excel in scenarios requiring high write and read performance for simple data models, such as caching, session management, and real-time analytics. Some widely-used key-value stores are Redis and Amazon DynamoDB.
- Column-family stores: Also known as wide-column stores, these databases store data in columns rather than rows, making them highly efficient for read and write operations on specific columns of data. Column-family stores are particularly well-suited for large-scale, distributed applications with high write loads and sparse or time-series data, such as IoT systems, log analysis, and recommendation engines. Examples of column-family stores include Apache Cassandra and HBase.
- Graph databases: Graph databases store data as nodes and edges in a graph, representing entities and their relationships. These databases are optimized for traversing complex relationships and performing graph-based queries, making them ideal for applications involving social networks, fraud detection, knowledge graphs, and semantic search. Some notable graph databases are Neo4j and Amazon Neptune.
C. Pros and cons of using NoSQL databases
- Flexibility and schema-less design: One of the primary advantages of NoSQL databases is their schema-less design, which allows for greater flexibility in handling diverse and dynamic data models. This makes it easier to adapt to changing requirements and accommodate new data types without the need for extensive schema modifications, as is often the case with SQL databases.
- Horizontal scalability: NoSQL databases are designed to scale horizontally, enabling the distribution of data across multiple servers, often with built-in support for data replication, sharding, and partitioning. This makes NoSQL databases well-suited for large-scale applications with high write loads or massive amounts of data, where traditional SQL databases may struggle to maintain performance and consistency.
- Performance under specific workloads: NoSQL databases can offer superior performance under specific workloads, such as high write loads, large-scale data storage and retrieval, and complex relationships. By choosing a NoSQL database tailored to the needs of a particular application, developers can optimize performance and resource utilization while maintaining an appropriate level of data consistency and reliability.
- CAP theorem and trade-offs: The CAP theorem states that a distributed data store can provide only two of the following three guarantees: Consistency, Availability, and Partition Tolerance. NoSQL databases often prioritize Availability and Partition Tolerance over Consistency, resulting in a trade-off known as “eventual consistency.” While this may be acceptable in some applications, it can lead to challenges in maintaining data integrity and reconciling conflicting updates in scenarios where strong consistency is required.
- Query complexity and expressiveness: While some NoSQL databases offer powerful query languages and capabilities, they may not be as expressive or versatile as SQL when it comes to complex data manipulation and analysis. This can be a limiting factor in applications that require sophisticated querying, joining, or aggregation of data. Additionally, developers may need to learn multiple query languages and paradigms when working with different types of NoSQL databases.
Factors to Consider When Choosing a Database
When it comes to selecting a database, candidates must take into account all the factors involved, such as data model and structure, scalability requirements, consistency and reliability, query complexity and frequency, performance and latency, as well as operational complexity and maintenance. Let’s look into all of these one by one:
A. Data model and structure
One of the primary factors to consider when selecting a database is the data model and structure of the information you plan to store. Understanding the complexity, diversity, and relationships within the data will help you determine the most appropriate database type.
- Tabular data and well-defined relationships: If your data model is primarily tabular with well-defined relationships, an SQL database may be a better fit. The relational model used by SQL databases is particularly suited for structured, tabular data and can maintain data integrity through constraints and relationships.
- Hierarchical, dynamic, or unstructured data: If your data is hierarchical, dynamic, or unstructured, a NoSQL database may offer more flexibility. NoSQL databases often use alternative data models, such as document, key-value, column-family, or graph, which can better accommodate diverse and evolving data structures.
B. Scalability requirements
Evaluating your application’s scalability needs, both in terms of data volume and read/write load, is crucial in choosing the right database.
- Vertical scaling: SQL databases are generally more adept at scaling vertically by adding more resources (such as CPU, memory, and storage) to a single server. This can be sufficient for many applications but may eventually hit performance limitations as resource demands increase.
- Horizontal scaling: NoSQL databases are designed to scale horizontally, enabling the distribution of data across multiple servers, often with built-in support for data replication, sharding, and partitioning. This makes NoSQL databases well-suited for large-scale applications with high write loads or massive amounts of data, where traditional SQL databases may struggle to maintain performance and consistency.
C. Consistency and reliability
The level of consistency and reliability required by your application plays a significant role in determining the most suitable database type.
- ACID properties and strong consistency: If strong consistency and ACID (Atomicity, Consistency, Isolation, Durability) properties are essential, an SQL database may be the better choice. SQL databases enforce these properties to guarantee the reliability of transactions and the consistency of the data.
- Eventual consistency and trade-offs: NoSQL databases often prioritize Availability and Partition Tolerance over Consistency, resulting in a trade-off known as “eventual consistency.” While this may be acceptable in some applications, it can lead to challenges in maintaining data integrity and reconciling conflicting updates in scenarios where strong consistency is required.
D. Query complexity and frequency
Assessing the complexity and frequency of queries your application will perform is essential in selecting the right database.
- Complex querying: SQL databases are known for their powerful query capabilities, making them ideal for applications with complex querying requirements. SQL is an expressive language that allows developers to perform operations like filtering, sorting, grouping, and joining multiple tables based on specified conditions.
- Simple lookups or updates: If your application primarily performs simple lookups or updates, a NoSQL database may offer better performance. NoSQL databases often excel in scenarios requiring high write and read performance for simple data models.
E. Performance and latency
Considering the performance and latency requirements of your application is critical when choosing a database.
- High performance and low latency: If you need high performance and low latency for specific workloads or data access patterns, choose a NoSQL database that is optimized for those scenarios. NoSQL databases can offer superior performance under certain workloads, such as high write loads, large-scale data storage, and complex relationships.
- General-purpose performance: SQL databases can provide robust, general-purpose performance for a wide range of applications. While they may not be optimized for specific workloads or access patterns, they offer a consistent and reliable performance profile for most use cases.
F. Operational complexity and maintenance
Finally, take into account the operational complexity and maintenance requirements of your chosen database. This includes factors such as deployment, monitoring, backup, and recovery. Choose a database that aligns with your team’s expertise, tools, and processes.
- Deployment: Consider the ease of deployment and integration with your existing infrastructure. Some databases may require more complex setup and configuration, while others offer streamlined deployment processes or managed services that handle the operational aspects for you.
- Monitoring: Evaluate the monitoring capabilities of the database, including performance metrics, error tracking, and log analysis. A database with comprehensive monitoring tools can help you identify and address issues proactively, ensuring the smooth operation of your application.
- Backup and recovery: Assess the backup and recovery features of the database, including the ease of creating and restoring backups, as well as the ability to handle disaster recovery scenarios. A robust backup and recovery strategy is essential to protect your data and maintain business continuity in case of unforeseen events.
- Security: Investigate the security features of the database, such as encryption, access control, and auditing. A secure database can help protect your sensitive data from unauthorized access and mitigate potential risks associated with data breaches.
- Community and support: Consider the community and support ecosystem surrounding the database. A vibrant community can provide valuable resources, such as documentation, tutorials, and forums, while a strong support ecosystem can offer professional assistance and guidance when needed.
- Cost: Finally, take into account the cost of using the chosen database, including licensing, hardware, and operational expenses. Depending on your budget and requirements, you may need to weigh the benefits of various databases against their associated costs to make an informed decision.
Real-World Examples and Case Studies: Putting Database Choices into Context
Understanding the theoretical differences between SQL and NoSQL databases is essential, but examining real-world examples and case studies can provide valuable insights into how these databases are used in practice. This section will explore various use cases where SQL and NoSQL databases have been successfully implemented, highlighting their respective strengths and showcasing how they can be employed to address specific application requirements. Additionally, we will discuss hybrid solutions that combine the capabilities of both database types to create robust and versatile systems. By exploring these real-world scenarios, you can gain a deeper understanding of how to select the appropriate database in your system design interview.
A. SQL Databases in Action
- E-commerce platforms: SQL databases are widely used in e-commerce platforms, where structured data and well-defined relationships are the norm. For example, an online store’s database may have tables for customers, products, orders, and shipping details, all with established relationships. SQL databases enable efficient querying and data manipulation, making it easier for e-commerce platforms to manage inventory, customer data, and order processing.
- Financial systems: Financial applications, such as banking and trading platforms, rely on SQL databases to maintain transactional consistency, ensure data integrity, and support complex queries. The ACID properties of SQL databases are crucial in this context, as they guarantee the correct processing of transactions and safeguard against data corruption.
- Content Management Systems (CMS): Many popular CMS platforms, such as WordPress and Joomla, use SQL databases to store content, user data, and configuration information. The structured nature of the data and the powerful query capabilities of SQL databases make them well-suited for managing content and serving dynamic web pages.
B. NoSQL Databases in Action
- Social media platforms: NoSQL databases, particularly graph databases, are ideal for managing complex relationships and interconnected data found in social media platforms. For example, Facebook uses a custom graph database called TAO to store user profiles, friend connections, and other social graph data. This allows Facebook to efficiently query and traverse the massive social graph, providing features like friend recommendations and newsfeed personalization.
- Big data analytics: NoSQL databases, such as Hadoop’s HBase and Apache Cassandra, are commonly used for big data analytics, where large-scale data storage and processing are required. These databases are designed to scale horizontally, enabling them to handle vast amounts of data and high write loads. For example, Netflix uses Apache Cassandra to manage its customer data and viewing history, which helps the streaming service to provide personalized content recommendations to its users.
- Internet of Things (IoT): IoT applications generate massive volumes of data from various devices and sensors, often with varying data structures and formats. NoSQL databases like MongoDB and Amazon DynamoDB are suitable for handling this diverse and dynamic data, providing flexible data modeling and high-performance storage capabilities. For example, Philips Hue, a smart lighting system, uses Amazon DynamoDB to store and manage data generated by its connected light bulbs and devices.
C. Hybrid Solutions
- Gaming industry: In the gaming industry, developers often use a combination of SQL and NoSQL databases to support different aspects of their applications. For instance, an SQL database may be employed to manage user accounts, in-game purchases, and other transactional data, while a NoSQL database like Redis can be used to store real-time game state information and leaderboards.
- E-commerce with personalized recommendations: Some e-commerce platforms combine SQL databases for transactional data and inventory management with NoSQL databases for personalized recommendations. This hybrid approach allows the platform to leverage the strengths of both database types, ensuring efficient data storage, querying, and analysis for various aspects of the application.
Avoiding Common Pitfalls in Database Selection
One of the biggest mistakes candidates make in system design interviews is relying too much on their personal experience or bias when selecting a database. Remember, interviews are not about which database you prefer, but about which one is most suited to the problem you’re facing.
When selecting a database, it’s important to consider the specific needs of your application. What type of data will you be storing? How much data will you be storing? How frequently will you be accessing the data? These are all important questions to ask yourself before making a decision.
Another common pitfall is failing to fully understand the requirements of the application. It’s important to take the time to thoroughly analyze the needs of your application before selecting a database. This includes understanding the expected traffic and usage patterns, as well as any specific performance requirements.
Choosing the wrong database can have serious consequences for your application. For example, if you choose a database that can’t handle the amount of data you need to store, you may run into performance issues or even data loss. On the other hand, if you choose a database that is too complex for your needs, you may end up with a system that is difficult to maintain and scale.
It’s also important to consider the long-term implications of your database selection. As your application grows and evolves, your database needs may change. Choosing a database that is flexible and scalable can help ensure that your application can continue to meet your needs as you grow.
Conclusion
Choosing the right database in system design interviews requires careful consideration of the technical requirements of the application, as well as strategic factors such as cost, maintainability, and team expertise. By understanding the pros and cons of SQL and NoSQL, as well as other database options, candidates can make an informed decision on which technology to use. Beyond selecting a single database, polyglot persistence can combine the strengths of multiple database systems, further boosting an application’s performance and scalability.
➡ Check Grokking System Design Fundamentals for a list of common system design concepts.
➡ Learn more about these questions in “Grokking the System Design Interview” and “Grokking the Advanced System Design Interview.”