Month: June 2023
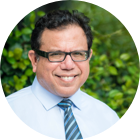
MMS • RSS
Posted on mongodb google news. Visit mongodb google news
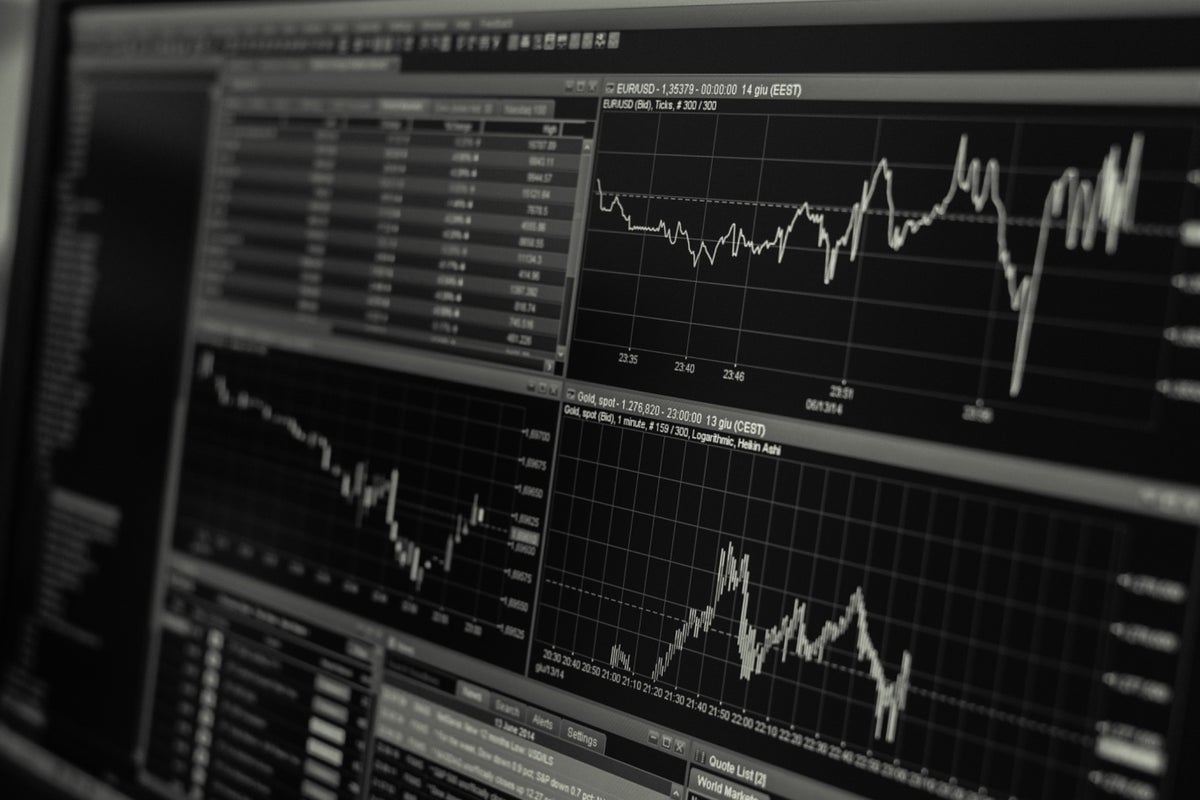
Over the past 3 months, 24 analysts have published their opinion on MongoDB MDB stock. These analysts are typically employed by large Wall Street banks and tasked with understanding a company’s business to predict how a stock will trade over the upcoming year.
Bullish | Somewhat Bullish | Indifferent | Somewhat Bearish | Bearish | |
---|---|---|---|---|---|
Total Ratings | 10 | 12 | 1 | 0 | 1 |
Last 30D | 1 | 1 | 0 | 0 | 0 |
1M Ago | 7 | 8 | 1 | 0 | 1 |
2M Ago | 1 | 1 | 0 | 0 | 0 |
3M Ago | 1 | 2 | 0 | 0 | 0 |
These 24 analysts have an average price target of $363.75 versus the current price of MongoDB at $388.36, implying downside.
Below is a summary of how these 24 analysts rated MongoDB over the past 3 months. The greater the number of bullish ratings, the more positive analysts are on the stock and the greater the number of bearish ratings, the more negative analysts are on the stock
This average price target has increased by 42.36% over the past month.
Stay up to date on MongoDB analyst ratings.
Benzinga tracks 150 analyst firms and reports on their stock expectations. Analysts typically arrive at their conclusions by predicting how much money a company will make in the future, usually the upcoming five years, and how risky or predictable that company’s revenue streams are.
Analysts attend company conference calls and meetings, research company financial statements, and communicate with insiders to publish their ratings on stocks. Analysts typically rate each stock once per quarter or whenever the company has a major update.
Some analysts will also offer forecasts for metrics like growth estimates, earnings, and revenue to provide further guidance on stocks. Investors who use analyst ratings should note that this specialized advice comes from humans and may be subject to error.
This article was generated by Benzinga’s automated content engine and reviewed by an editor.
Article originally posted on mongodb google news. Visit mongodb google news
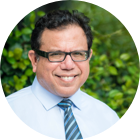
MMS • RSS
Posted on mongodb google news. Visit mongodb google news
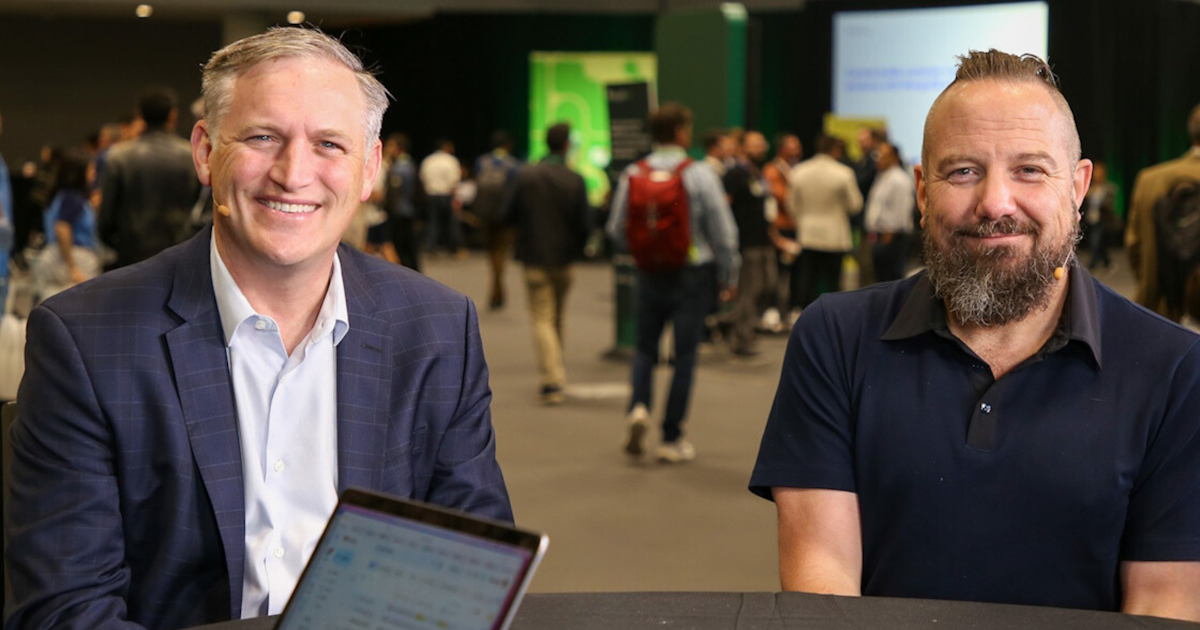
The landscape of software development is constantly evolving, driven by the relentless pursuit of innovation and the needs of developers worldwide.
In this era of rapid technological advancements, the collaboration between MongoDB Inc. and Amazon Web Services Inc. is forging new frontiers in developer empowerment. Their integration through the AWS Marketplace is revolutionizing the way developers procure, build and scale their applications, according to Chris Grusz (pictured, left), managing director of technology partnerships at AWS.
“AWS loves developers, and so Marketplace is one of the ways that we’re delivering third-party products to the developer community,” he said Chris Grusz.
Grusz and Peder Ulander (right), chief marketing officer of MongoDB, spoke with theCUBE industry analyst John Furrier at the MongoDB .local NYC event, during an exclusive broadcast on theCUBE, SiliconANGLE Media’s livestreaming studio. They discussed the benefits the integration between MongoDB and AWS offers to developers and enterprises and analyzed the future of developer empowerment in an ever-expanding digital landscape. (* Disclosure below.)
The power of AWS Marketplace
The AWS Marketplace is significant as a platform for procuring and developing technology. With over a decade of existence, the Marketplace has gained significant momentum and has become a vital resource for developers, providing a self-service engine that enables customers to easily access technologies, offering options for free trials, pay-as-you-go models and larger subscriptions, according to Grusz.
The integration with MongoDB has brought additional benefits, such as a seamless landing capability, management governance features and automated processes that make third-party products look like first-party services within AWS.
“We’ve got a great self-service engine that we’ve been working with MongoDB on so that customers can just come in and get their hands on the technology,” Grusz said.
Empowering developers and enterprises
Both MongoDB and AWS have always prioritized the needs of developers, regardless of their scale or industry. MongoDB’s expansion into various verticals, such as financial services, healthcare and media, has allowed the company to provide compelling solutions tailored to specific industries.
On the other side of the coin, the AWS Marketplace has been instrumental in supporting developers throughout their journey, enabling them to buy, provision, build and run MongoDB services at scale without the need for a traditional sales team.
“We were an early partner with AWS on that movement,” Ulander said. “It wasn’t just the work that we’re doing with the Marketplace. There’s integrations in the console, in the management and governance services. What those integrations do is, we take the hard work of stitching these pieces together that are required for developers on the platform and give it to them day one. I think that is such an important thing to do to get them running as quickly as possible at their code.”
The future of developer empowerment
The integration between AWS Marketplace and MongoDB represents a shift in the software business toward a builder culture, empowering developers to innovate without the burden of extensive sales efforts.
The AWS Marketplace continues to evolve, incorporating innovations such as built-in templates for security and vendor assessment components. Additionally, AWS and MongoDB are working together to simplify the procurement process through channel partner functionality and the TriParty program. These initiatives remove friction and heavy lifting, providing customers with a frictionless experience and enabling them to leverage the full potential of MongoDB’s data platform.
“This is the dream of every enterprise and the benefit of a developer-oriented or bottoms-up motion … the flywheel that gets created with that product-created growth … you can just get moving so quickly,” Ulander said.
Here’s the complete video interview, part of SiliconANGLE’s and theCUBE’s coverage of the MongoDB .local NYC event:
(* Disclosure: TheCUBE is a paid media partner for the MongoDB .local NYC event. Neither MongoDB Inc., the sponsor of theCUBE’s event coverage, nor other sponsors have editorial control over content on theCUBE or SiliconANGLE.)
Photo: SiliconANGLE
Your vote of support is important to us and it helps us keep the content FREE.
One-click below supports our mission to provide free, deep and relevant content.
Join our community on YouTube
Join the community that includes more than 15,000 #CubeAlumni experts, including Amazon.com CEO Andy Jassy, Dell Technologies founder and CEO Michael Dell, Intel CEO Pat Gelsinger and many more luminaries and experts.
THANK YOU
Article originally posted on mongodb google news. Visit mongodb google news
MongoDB Launches AI Initiative with Google Cloud to Help Developers Build AI Powered …
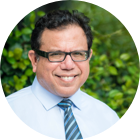
MMS • RSS
Posted on mongodb google news. Visit mongodb google news
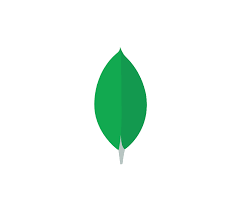
- Expanded partnership between MongoDB and Google Cloud enables developers to use state-of-the-art AI foundation models from Google to build new classes of generative AI applications with expert assistance and professional services
MongoDB, Inc. (NASDAQ: MDB) today at its developer conference MongoDB.local NYC announced a new initiative in partnership with Google Cloud to help developers accelerate the use of generative AI and build new classes of applications. MongoDB Atlas is the multi-cloud developer data platform used by tens of thousands of customers and millions of developers globally to quickly build and scale applications using a single platform. With an integrated operational data store at its core, MongoDB Atlas is uniquely positioned to help organizations of all shapes and sizes build applications powered by generative AI faster and with less complexity. Developers can now take advantage of MongoDB Atlas and industry-leading partner integrations with Google Cloud’s Vertex AI large language models (LLMs) and new quick-start architecture reviews with MongoDB and Google Cloud professional services to accelerate software development. To learn more about MongoDB Atlas, visit mongodb.com/products/platform/atlas-cloud-providers/google-cloud
Recent advancements in generative AI technology like LLMs present opportunities to reimagine how end users interact with applications. Developers want to take advantage of generative AI to unlock their creativity and build new classes of applications, but many current solutions require piecing together several different technologies and components or bolting on solutions to existing technology stacks, making software development cumbersome, complex, and expensive. MongoDB and Google Cloud are helping address these challenges by providing a growing set of solutions and integrations to meet developers where they are and enable them to quickly get started building applications that take advantage of new AI technologies:
- Seamlessly use Google Cloud’s Vertex AI foundation models with MongoDB Atlas Vector Search: MongoDB and Google Cloud have partnered since 2018 and have helped thousands of joint customers—including Keller Williams, Rent the Runway, and Ulta—adopt cloud-native data strategies. Earlier this year, that partnership was expanded to include deeper product integrations. Now, developers can use MongoDB Atlas Vector Search with Vertex AI to seamlessly build applications with AI-powered capabilities for highly personalized and engaging end-user experiences. Vertex AI provides the text embedding API to generate embeddings from customer data stored in MongoDB Atlas, combined with the PaLM text models to create advanced functionality like semantic search, classification, outlier detection, AI-powered chatbots, and text summarization. To learn more about building applications on MongoDB Atlas with Vertex AI, visit mongodb.folloze.com/google-cloud-ai
- Build AI applications faster with hands-on assistance from experts: From ideation to execution, the MongoDB and Google Cloud professional services teams can help rapidly prototype applications by providing expertise on data schema and indexing design, query structuring, and fine-tuning AI models to build a strong foundation for applications. The Vertex AI platform caters to the full range of AI use cases from advanced AI and data science practitioners with end-to-end AI/ML pipelines to business users who can create out-of-the-box experiences leveraging the foundational models to generate content for language, image, speech, and code. Developers can also tune models to further improve the performance of the model for specific tasks. Google Cloud and MongoDB are working closely together under the Built with Google Cloud AI program to make these experiences even more seamless with Google’s Generative AI capabilities built right into MongoDB Atlas. When applications are ready for production, the MongoDB and Google Cloud professional services teams can optimize applications for performance and help solve future problems through quick iteration to get new features into production more quickly. To get started, visit https://www.mongodb.com/products/consulting
“With the shift in technology powered by generative AI taking place today, the future of software and data is now, and we’re making it more evenly distributed for developers with MongoDB Atlas,” said Alan Chhabra, Executive Vice President of Worldwide Partnerships at MongoDB. “This shift begins with developers, and we want to democratize access to game-changing technology so all developers can build the next big thing. With MongoDB Atlas and our strategic partnership with Google Cloud, it’s now easier for organizations of all shapes and sizes to incorporate AI into their applications and embrace the future.”
“Generative AI represents a significant opportunity for developers to create new applications and experiences and to add real business value for customers,” said Kevin Ichhpurani, Corporate Vice President, Global Ecosystem and Channels at Google Cloud. “This new initiative from Google Cloud and MongoDB will bring more capabilities, support, and resources to developers building the next generation of generative AI applications.”
One AI enables businesses to deploy the world’s best language AI capabilities, tuned to their unique needs, in days. “We needed a platform that allowed us to build agile, data-driven, and scalable software, and MongoDB Atlas on Google Cloud was the obvious choice,” said Amit Ben, Founder & CEO at One AI. “The flexibility and scalable document data model MongoDB Atlas provides enabled us to do hundreds of small incremental changes and additions to our platform in the last year alone, allowing rapid development and quick adaptation to shifting and growing needs with zero effort and no need for any migration or compatibility issues. We are looking forward to the additional support MongoDB and Google Cloud will provide as we grow and scale our company.”
***
MongoDB Developer Data Platform
MongoDB Atlas is the leading multi-cloud developer data platform that accelerates and simplifies building with data. MongoDB Atlas provides an integrated set of data and application services in a unified environment to enable developer teams to quickly build with the capabilities, performance, and scale modern applications require.
About MongoDB
Headquartered in New York, MongoDB’s mission is to empower innovators to create, transform, and disrupt industries by unleashing the power of software and data. Built by developers, for developers, our developer data platform is a database with an integrated set of related services that allow development teams to address the growing requirements for today’s wide variety of modern applications, all in a unified and consistent user experience. MongoDB has tens of thousands of customers in over 100 countries. The MongoDB database platform has been downloaded hundreds of millions of times since 2007, and there have been millions of builders trained through MongoDB University courses. To learn more, visit mongodb.com.
Article originally posted on mongodb google news. Visit mongodb google news
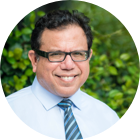
MMS • Michael Redlich
Article originally posted on InfoQ. Visit InfoQ
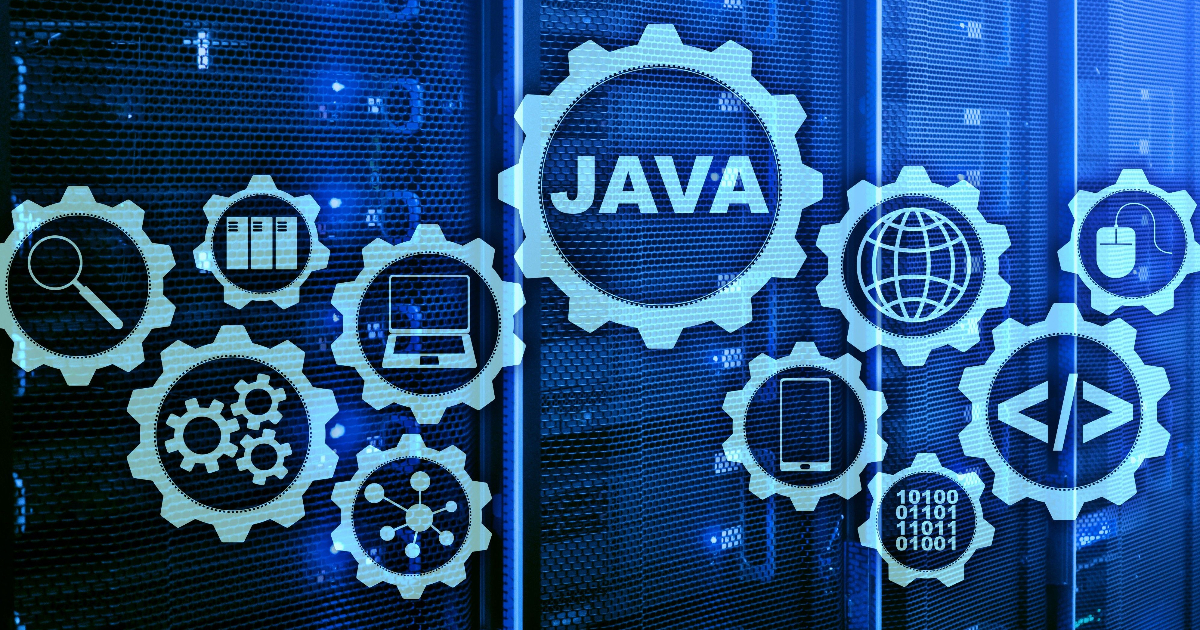
Day Three of the 9th annual QCon New York conference was held on June 15th, 2023 at the New York Marriott at the Brooklyn Bridge in Brooklyn, New York. This three-day event is organized by C4Media, a software media company focused on unbiased content and information in the enterprise development community and creators of InfoQ and QCon. It included a keynote address by Suhail Patel and presentations from these four tracks:
Morgan Casey, Program Manager at C4Media, and Danny Latimer, Content Product Manager at C4Media, kicked off the day three activities by welcoming the attendees. They introduced the Program Committee, namely: Aysylu Greenberg, Frank Greco, Sarah Wells, Hien Luu, Michelle Brush, Ian Thomas and Werner Schuster; and acknowledged the QCon New York staff and volunteers. The aforementioned track leads for Day Three introduced themselves and described the presentations in their respective tracks.
Keynote Address: The Joy of Building Large Scale Systems
Suhail Patel, Staff Engineer at Monzo, presented a keynote entitled, The Joy of Building Large Scale Systems. On his opening slide, which Patel stated was also his conclusion, asked why the following is true:
Many of the systems (databases, caches, queues, etc.) that we rely on are grounded on quite poor assumptions for the hardware of today.
He characterized his keynote as a retrospective of where we have been in the industry. As the title suggests, Patel stated that developers have “the joy of building large scale systems, but the pain of operating them.” After showing a behind-the-scenes view of the required microservices for an application in which a Monzo customer uses their debit card, he introduced: binary trees, a tree data structure where each node has at most two children; and a comparison of latency numbers, as assembled by Jonas Bonér, Founder and CTO of Lightbend, that every developer should know. Examples of latency data included: disk seek, main memory reference and L1/L2 cache references. Patel then described how to search a binary tree, insert nodes and rebalance a binary tree as necessary. After a discussion of traditional hard drives, defragmentation and comparisons of random and sequential I/O as outlined in the blog post by Adam Jacobs, Chief Scientist at 1010data, he provided analytical data of how disks, CPUs and networks have been evolving and getting faster. “Faster hardware == more throughput,” Patel maintained. However, despite the advances in CPUs and networks, “The free lunch is over,” he said, referring to a March 2005 technical article by Herb Sutter, Software Architect at Microsoft and Chair of the ISO C++ Standards Committee, that discussed the slowing down of Moore’s Law and how the drastic increases in CPU clock speed were coming to an end. Sutter maintained:
No matter how fast processors get, software consistently finds new ways to eat up the extra speed. Make a CPU ten times as fast, and software will usually find ten times as much to do (or, in some cases, will feel at liberty to do it ten times less efficiently).
Since 2005, there has been a revolution in the era of cloud computing. As Patel explained:
We have become accustomed to the world of really infinite compute and we have taken advantage of it by writing scalable and dist software, but often focused on ever scaling upwards and outwards without a ton of regard for perf per unit of compute that we are utilizing.
Sutter predicted back then that the next frontier would be in software optimization with concurrency. Patel discussed the impact of the thread per core architecture and the synchronization challenge where he compared: the shared everything architecture in which multiple CPU cores access the same data in memory; versus the shared nothing architecture in which the multiple CPU cores access their own dedicated memory space. A 2019 white paper by Pekka Enberg, Founder and CTO at ChiselStrike, Ashwin Rao, Researcher at University of Helsinki, and Sasu Tarkoma, Campus Dean at University of Helsinki found a 71% reduction in application tail latency using the shared nothing architecture.
Patel then introduced solutions to help developers in this area. These include: Seastar, an open-source C++ framework for high-performance server applications on modern hardware; io_uring, an asynchronous interface to the Linux kernel that can potentially benefit networking; and the emergence of programming languages, such as Rust and Zig; a faster CPython with the recent release of version 3.11; and eBPF, a toolkit for creating efficient kernel tracing and manipulation programs.
As an analogy of human and machine coming together, Patel used as an example, Sir Jackie Stewart, who coined the term mechanical sympathy as caring and deeply understanding the machine to extract the best possible performance.
He maintained there has been a cultural shift in writing software to take advantage of the improved hardware. Developers can start with profilers to locate bottlenecks. Patel is particularly fond of Generational ZGC, a Java garbage collector that will be included in the upcoming GA release of JDK 21.
Patel returned to his opening statement and, as an addendum, added:
Software can keep pace, but there’s some work we need do to yield huge results, power new kinds of systems and reduce compute costs
Optimizations are staring at us in the face and Patel “longs for the day that we never have to look at the spinner.”
Highlighted Presentations: Living on the Edge, Developing Above the Cloud, Local-First Technologies
Living on the Edge by Erica Pisani, Sr. Software Engineer at Netlify. Availability zones are defined as one or more data centers located in dedicated geographic regions provided by organizations such as AWS, Google Cloud or Microsoft Azure. Pisani further defined: the edge as data centers that live outside of an availability zone; an edge function as a function that is executed in one of these data centers; and data on the edge as data that is cached/stored/accessed at one of these data centers. This provides improved performance especially if a user is the farthest away from a particular availability zone.
After showing global maps of AWS availability zones and edge locations, she then provided an overview on the communication between a user, edge location and origin server. For example, when a user makes a request via a browser or application, the request first arrives at the nearest edge location. In the best case, the edge location responds to the request. However, if the cache at the edge location is outdated or somehow invalidated, the edge location must communicate with the origin server to obtain the latest cache information before responding to the user. While there is an overhead cost for this scenario, subsequent users will benefit.
Pisani discussed various problems and corresponding solutions for web application functionality on the edge using edge functions. These were related to: high traffic pages that need to serve localized content; user session validation taking too much time in the request; and routing a third-party integration request to the correct region. She provided an extreme example of communication between a far away user relative to two origin servers for authentication. Installing an edge server close to the remote user eliminated the initial latency.
There is an overall assumption that there is reliable Internet access. However, that isn’t always true. Pisani then introduced the AWS Snowball Edge Device, a physical device that provides cloud computing available for places with unreliable and/or non-existent Internet access or as a way of migrating data to the cloud. She wrapped up her presentation by enumerating some of the limitations of edge computing: lower available CPU time; advantages may be lost when network request is made; limited integration with other cloud services; and smaller caches.
Developing Above the Cloud by Paul Biggar, Founder and CEO at Darklang. Biggar kicked off his presentation with an enumeration of how computing has evolved over the years in which a simple program has become complex when persistence, Internet, reliability, continuous delivery, and scalability are all added to the original simple program. He said that “programming used to be fun.” He then discussed other complexities that are inherent in Docker, front ends and the growing number of specialized engineers.
With regards to complexity, “simple, understandable tools that interact well with our existing tools” are the way developers should build software along with the UNIX philosophy of “do one thing, and do it well.” However, Biggar claims that building simple tools that interact well is just a fallacy and is the problem because doing so leads to the complexity that is in software development today with “one simple, understandable tool at a time.”
He discussed incentives for companies in which engineers don’t want to build new greenfield projects that solve all the complexity. Instead, they are incentivized to add small new things to existing projects that solve problems. Therefore, Biggar maintained that “do one thing, and do it well” is also the problem. This is why the “batteries included” approach, provided by languages such as Python and Rust, deliver all the tools in one package. “We should be building holistic tools,” Biggar said, leading up to the main theme of his presentation on developing above the cloud.
Three types of complexity: infra complexity; deployment complexity; and tooling complexity, should be removed for an improved developer experience. Infra complexity includes the use of tools such as: Kubernetes, ORM, connection pools, health checks, provisioning, cold starts, logging, containers and artifact registries. Biggar characterized deployment complexity with a quote from Jorge Ortiz in which the “speed of developer iteration is the single most important factor in how quickly a technology company can move.” There is no reason that deployment should take a significant amount of time. Tooling complexity was explained by demos of the Darklang IDE in which creating things like REST endpoints or persistence, for example, can be quickly moved to production by simply adding data in a dialog box. There was no need to worry about things such as: server configuration, pushing to production or a CI/CD pipeline. Application creation is reduced down to the abstraction.
At this time, there is no automated testing in this environment and adoption of Darklang is currently in “hundreds of active users.”
Offline and Thriving: Building Resilient Applications With Local-First Techniques by Carl Sverre, Entrepreneur in Residence at Amplify Partners. Sverre kicked off his presentation with a demonstration of the infamous “loading spinner” as a necessary evil to inform the user that something was happening in the background. This kind of latency doesn’t need to exist as he defined offline-first as:
(of an application or system) designed and prioritized to function fully and effectively without an internet connection, with the capability to sync and update data once a connection is established.
Most users don’t realize their phone apps, such as WhatsApp, email apps and calendar apps, are just some examples of offline-apps and how they have improved over the years.
Sverre explained his reasons for developing offline-first (or local-first) applications. Latency can be solved by optimistic mutations and local storage techniques. Because the Internet can be unreliable with issues such as dropped packets, latency spikes and routing errors, Reliability is crucial to applications. Adding features for Collaboration leverage offline-first techniques and data models for this purpose. He said that developers “gain the reliability of offline-first without sacrificing the usability of real time.” Development velocity can be accomplished by removing the complexity of software development.
Case studies included: WhatsApp, the cross-platform, centralized instant messaging and voice-over-IP service, uses techniques such as end-to-end encryption, on-device messages and media, message drafts and background synchronization; Figma, a collaborative interface design tool, uses techniques such as real-time collaborative editing, a Conflict-Free Replicated Data Type (CRDT) based data model, and offline editing; and Linear, an alternative to JIRA, uses techniques such as faster development velocity, offline editing and real-time synchronization.
Sverre then demonstrated the stages for converting a normal application to an offline-first application. However, trade-offs to consider for offline-first application development include conflict resolution, eventual consistency, device storage, access control and application upgrades. He provided solutions to these issues and maintained that, despite these tradeoffs, this is better than an application displaying the “loading spinner.”
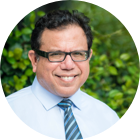
MMS • RSS
Posted on mongodb google news. Visit mongodb google news

Yasmin Boland
Aries Daily Horoscope – 23 June 2023
The moon moves into Virgo shifting your focus onto work and your daily routine and despite it being Friday, the wind-down day, if you’re lucky, you might still feel all fired up and ready to take on the world, by storm, in your own way. You also seem to be a lot more detail-oriented by […] The post Aries Daily Horoscope – 23 June 2023 appeared first on Yasmin Boland.
Article originally posted on mongodb google news. Visit mongodb google news
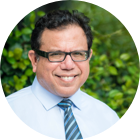
MMS • Frank Yu
Article originally posted on InfoQ. Visit InfoQ
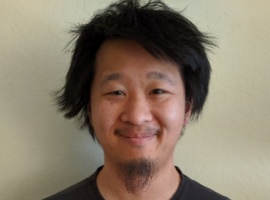
Transcript
Yu: We’ll be talking about leveraging determinism, or in more words, trusting deterministic execution to stabilize, scale, and simplify systems. My name is Frank. I’m on the engineering team that built and runs the Coinbase Derivatives Exchange. I’ve got two things I’d like you to come away with. First, I’ll try to make the case that if you’ve got important logic, make it deterministic. You’ll be happy you did. If you’ve already got deterministic logic, don’t be afraid to rerun it anywhere in your stack, in production, even, for efficiency and profit. As a disclaimer, we’ll largely be going over practices directly from our experience running financial exchanges. We don’t rigorously prove any of the concepts we present here. Any references to the canon or any prior art we’re bringing together is for inspiration, and as a case study for what we’ve done. Just remember, n equals 1 here, it’s all anecdotal.
About Us and Our Problems
Let’s talk us and our problems. We built and run what is now the Coinbase Derivatives Exchange, formerly a startup called FairX, which built a CFTC government regulated trading exchange for folks to trade financial contracts with each other. You can submit us an order to buy or sell something for some price, and we’ll let you and the market know asynchronously when you submit that order, and when you trade, and what price you get. Think of exchanges as financial infrastructure. Everyone who participates in finance relies on us to provide the real prices of things traded on our platform, and relies on us to fairly assign trades between folks who want to enter into financial transactions. First thing to think about when running an exchange is mission critical code. A bug on our system could result in catastrophic problems for both ourselves and all of the clients in our platform, because we’re dealing directly with up to millions of dollars of client funds. Potential losses we risk are multiple orders of magnitude more than any revenue we might make from a transaction. In a previous life running a currency exchange, our clients would trust us with millions of dollars for each request, and maybe we’d be happy to earn 0.00001%, give or take a zero on that transaction. Business logic has got to be rock solid. Exchanges absolutely have to be correct.
The second thing about running a financial exchange is that many of our partners expect us to operate as close to instantaneously as possible, and also to be as consistent as possible. If we’re the ground that folks stand on for financial transactions, we’ve got to be predictable and fast. Specifically, here, we expect our 99th percentile response time latencies to stay comfortably under a millisecond. Finally, we got to keep records. We need to allow regulators, customers, debuggers like me to reconstruct the state of the market or the system exactly as it was at any given microsecond instant, for the last seven years. All of this adds up to a super high bar of quality on our core business logic. You might ask here, if risks are so high, correctness bugs are catastrophic, and performance bugs get instantly noticed, how do we actually add features to the system in any reasonable timeframes? We’ve got to make sure our core logic stays simple. In principle, we want to minimize the number of arrows and boxes in our architecture diagram. We don’t want a lot of services that can fail independently in a transaction and result in a partially failed system. When we handle financial transactions, we handle them in an atomic and transactional manner. We also avoid concurrency at all costs in our logic. In the last 10 to 20 years, multiprocessor systems have offered programs an interesting deal with the devil. Run stuff in parallel and you get some more throughput and performance. You’ve got multiple CPUs, why not assign work to all of them at the same time? You just have to make sure you don’t have any race conditions. Your logic is correct for every possible interleaving of every line of code in your concurrent system. Then, if you’re trying to reproduce a bug by running code over again, you can’t count on it to happen, because you’ve left things up to the OS scheduler. Here, I claim that simplicity through deterministic execution, it’ll get you simplicity, but it can also get you more performance and throughput than concurrent logic.
Deterministic Execution
Let’s describe deterministic execution a bit. You can largely consider a traditional program with a variable set to various values in the program counter set to some line of code as a state machine. The idea is this, inputs come into the system and contact with the state of your system and may change it. Inputs also come into your system and may result in some new data or output getting created. In practice, we’ll talk about inputs as requests into the system, API calls, and the change state given a request as the new state of the system. For example, if Alice deposits $1,000 into her account, then the new state of the system would reflect an additional $1,000 in her balance. We also talk about the output of a system as events. This is when Alice submits an order, and we have to asynchronously send a push notification to the rest of the market, informing them of the new bid for a given thing at some given price. You’ll hear me use inputs and requests, and then outputs and events interchangeably here. We’re drawing out that equivalency. A system is deterministic now if given an exact set of inputs, in the same order, we get the exact same state and outputs. In practice, if you take all of your requests, and you sequence them all into a log, and then you apply determinism here, [inaudible 00:07:01], we’ll effectively get replicated state and output events. Again, if we sequence Alice first depositing $1,000, then submitting an order to buy something using that $1,000, our deterministic system should never have Alice using money for an order before she deposited it, even if I rerun my service over again, 1000 times at any point.
Benefits of Determinism
For some of you, replicated state machines might ring a bell, and you might think about consensus. This is a segue to some of the benefits for having a replicated state machine through deterministic execution. The first one here is high availability. We all have high availability concerns these days. Let’s say I’ve got my service running in production, and then some hardware failure crashes my service. Now I’m concerned. I’ve got an outage. I’m getting paged in the middle of the night. I’ve got to start stuff and recover lost data. It’s no fun. It wasn’t even my code that necessarily caused that hardware failure. If I have a deterministic system, I can actually replicate my log of incoming requests to other services running the same logic, and they’ll have the exact same state. If invariably, my service goes down, through no fault of my code, I’m still fine. I can pick a new primary service. Good thing, we have redundancy through determinism. That said, replicating every request into our system is a ton of work. The Raft algorithm says, don’t even start changing your system until you’re sure that your state machine is replicated to most of your machines. If we’re going to operate at microsecond timescales, we’ll need an implementation of consensus that’s really fast. Thanks to Todd Montgomery, and Martin Thompson of Real Logic, we’ve got a very fast open source Raft cluster that we can put our deterministic logic on and get all these nice high availability properties with negligible performance overhead. Hopefully, the civility benefits to determinism are clear.
Let’s talk performance. Another thing that’s nice when your logic is deterministic and serialized, is you can actually run everything in one thread and make use of the consistency and throughput you get from single threaded execution. One barrier to that consistent performance is actually giving up control to the operating system scheduler and hoping that you won’t get context switch out when you start handling a client request. Again, in the vein of keeping things simple, let’s tell that operating system to pin our business logic thread directly to the physical core or a hyper threaded core and not have our logic be preempted for anything else, and remove the complication of having to think about OS scheduling when developing for performance.
Changing tack, it should go without saying that a deterministic program should be easier to test than a non-deterministic program. Certainly, in cases of concurrency where the spaces of tests you need to write expands by n times m of the interleavings of the lines of code running in parallel. There’s some other nice things you can do if you’ve got one deterministic monolith that handles every request as a transaction to test. Each of your user stories basically turns into a test case. You can make use of time travel to forward your system to a very specific date so you don’t crash or have issues every leap year or something like that. Despite all that testing, when something still seems weird with the system, let’s say I’m in prod again, or staging, or anywhere, and something weird starts happening on my service. If I’m in prod, I’m concerned. I don’t know what’s going on. I wish I added log lines for this case. I hope this doesn’t impact too many people. If I’ve got deterministic logic now, when the weird behavior starts, I can go ahead and copy the request log, run it on my laptop. When the problem comes up, maybe I was running the system in a debugger. Now I know exactly why my program got into that weird state, because I’m inspecting all the states in memory and I’m figuring out the problem. I’ve still got some weird behavior in prod, but at least I maybe have a plan for how to deal with it. This idea of being able to take a running system in production, and rerun patch with log lines on your development environment, it’s a superpower. It also can’t be overstated how useful it is for maybe finding some pathological performance situations or tracing bugs. You rarely say, it works on my machine, again, for logic bugs, if your system is deterministic like this.
Business Logic
All this stuff. Great, sold. We’ve built this. What you’re seeing on the screen here is our business logic running in Raft consensus that’s in blue, surrounded by gateways or API endpoints, just like an HTTP JSON endpoint, or special message endpoints that you send finance messages to, those are all in green. Here’s a simple message flow to illustrate our system. Clients are going to send us requests through our gateways, like Alice wants to buy 2 nano-Bitcoin contracts for $20,000. That request gets forwarded onto our business logic service, the Raft monolith, and we go ahead and output events via multicast here to clients that connect to our bare metal data center. This is where the latency sensitive nature of our system impacts our architecture. For the non-latency sensitive use case, or the high throughput use case, or the cold storage use cases, we’re going to go ahead and send events over some transport to the cloud. Then forward them to regulators sitting behind the gateway or a database, so we can actually keep track of what happened long term. This big, red pipe, in other stacks, you might think of them like Kafka or a message queue. In general, you’ve got requests coming in to your business object in blue, and then events coming out of the system, and sometimes over a big transport to another region.
Here’s one thing we noticed. At this point in time, we’re in production. We’re a startup at this point, and we noticed something interesting about our requests. That request, the tuple of Alice buying 2 Bitcoin contracts, it’s 5 pieces of data, really. It’s about maybe 100 bytes coming in, but what comes out after we handle that API call might be huge. What if Alice at this point successfully buys 2 Bitcoin, and let’s say she trades with 2 different people. What are we sending over that big, red pipe? First, we’re sending the event that we successfully handled Alice’s or his order. Then, let’s say she trades 1 Bitcoin with Bob. We tell Alice that she bought a Bitcoin from Bob. We tell Bob that he sold a Bitcoin contract to Alice. If Alice also trades with Charlie in that request, we got to tell Alice that she traded with Charlie, and we got to tell Charlie that she sold to Alice. One request to buy 2 Bitcoin contracts suddenly becomes a mouthful of events once it goes through a logic. Here’s the kicker. We’re sending events from our bare metal data center over to our AWS data center. We’re finding out that AWS’s compute charges are fairly reasonable. Then, network ingress and network egress costs were nuts, we were being charged for our data bandwidth. At the time we were a starving startup, at least burn rate conscious, we weren’t super happy about lighting money on fire sending all this data. Yes, big question here, can we optimize? What’s our problem?
What we’re saying here is that in our situation, and in many situations, in general, business logic is fast and cheap, but reading and writing the data is what’s actually slow and expensive. How does the fact that our business logic is deterministic help here? Here’s a proposal. How about if when handling requests, we still go ahead, and instead of sending a ton of events over this expensive network connection, and lighting money on fire, we replicate only that request over the network. Then we continue on, we only replicate that request to our downstream services. That doesn’t really work, our downstream services want to see the impacts of that request, not the request itself. Let’s just rerun our business logic on the same machines as our downstream services, and send our red big blasts of events over IPC, or interprocess communication. That effectively means that we’ve now lighted all the network costs of egress events: way less network usage, less burning money.
Let’s catch our breath here. For the first time, we had to blink a few times. We’re saying, we can go from sending fat events everywhere, from one little request message, and we can go to just sending the requests everywhere? How does this work? It works because everything is deterministic. Our monolith is just a function that turns requests into their output events. It does the same thing every time as long as the ordering of requests are the same. If we didn’t have a multicast in the data center, if our data center on the left here was actually just in the cloud, we could apply that same trick. We could replicate requests to a monolith that is localized to our gateways, and send all that through IPC. What this has opened up is a whole axis of tradeoffs you can make, where you can now replicate compute instead of data and cases.
Replay Logic to Scale and Stabilize
Why does this work? Here’s what we noticed about inputs and requests. They’re often smaller than outputs. When we receive an order or an API call, maybe one of the first things we do about it when we handle it, is we actually stick an ID on it, and we want to identify the state of that request. We’re sticking a bunch of state on it. We’re contextualizing that request with our own services’ business context. Here’s another interesting thing we noticed. Inputs can probably be more consistent than our output events. You all know about 99 percentile latencies. How often do we think about our 99th percentile network load given some request? Here’s an example. Let’s say Alice sends us one request to sell 1000 Bitcoin contracts at just any price, because she just wants to cash out. Those 100 bytes coming to your system, they’re going to cause possibly thousands of events to happen, because she’s going to trade with the entire market. What you get here is a ton of events coming out of one request. If you’ve got an event driven system, you’ve got a positive feedback loop in here somewhere where you’re creating more events from handling events. This is how you get what’s called thundering herd. This is how massive autoscaling systems in data centers and in the cloud, can go down. Your network buffers completely fill up, because you’re blasting tons of events everywhere. Your services time out, and we’re not able to keep health checks up, or things like that.
Why are we saying here that these input requests can be more predictable, and outputs can’t be? The size of an input, a sequence into your log and the rate of them, they can be validated and rejected. Alice sending one API request is actually not a lot of network usage. If a client had a bug and was just spamming us with tons of requests, we can actually validate their input rate with rate limits, throttles. Your API gateways, your load balancers actually should be doing it for you. When you talk about the size of inputs, if there’s malformed data, you can validate that before it gets into your business logic. It’s not quite so easy with outputs and events. An event, in some sense of fact, it’s a change in your system because of some request. You don’t reject an effect of a request. You can, but it’s pretty difficult. Also, it’s often unclear when a request might cause just a landslide of events to come out. Those are what you would basically call pathological cases. You’ve got to analyze them to be able to predict them and mitigate them. In this case, if your entire network utilization is just your input rate that is validated by all of your external endpoints, then you’ll actually get some pretty consistent network utilization for all of your replication here. You can protect from thundering herd here by not sending blasts of events. If you’re replaying your logs in production, that’s how you’ll stabilize your system. Then, because it’s less network utilization, it’ll also help you scale.
Here’s a third benefit. When you replicate compute, you can also simplify your downstream code. All those blue boxes you saw on that diagram, monolith, monolith, monolith, it’s all the same code. It’s deduplicated. What that means is, you don’t have a lot of endpoints where you might be reimplementing your business logic just so you can materialize it, or have a better view of it. That said, deduplication is optimization here, it’s not architecture. We’re not offering up our firstborn at the altar of DRY, but it’s still pretty cool. Let’s take a look at it in action. Again, here’s my system, with monoliths everywhere. A common use case for any given system is you might want to accept user queries. If Alice wants to get her active orders, she’ll send the request through one of our endpoints. Traditionally, you would forward that query to your datastore. In our case, that’s the Raft monolith. The datastore responds with the data that you’re asking for, so blue coming in, red coming out, and that’s why these arrows are purple.
These requests might come in from all over the world. You might have clients in different regions. That might be another gateway talking to your datastore to go fetch data. We try to solve some of the scaling problems with this by maybe introducing caches in the middle. What that means is you still have to keep that cache up to date. What are you doing? Are you sending data to that cache? Are you doing some a lazy LRU type thing? It’s all just a lot of complication here. There’s a fairly obvious optimization that can be made, given that we’ve got monoliths everywhere. Instead of going to our main piece of business logic, our main datastore, we can just make an IPC call to the local monolith running alongside your endpoints and get the data back super quickly, and get fantastic query performance in this case, because you’re totally localized. We can remove a box from our architecture diagram.
What if we have no choice? We’ve built this great system, we got monoliths everywhere, and then a lot of new features keep getting added: new query opportunities, new gateways, new endpoints, new functionality. Alice wants to go get her balances, and a bunch of other things. You’ve got all these requests coming in from the outside. To scale, we might need a cache again. A really great cache, in this case, is actually our monolith. What we can do is, instead of replicating events, or updating maps, key-value stores, we can actually just replicate that same sequence log of requests. Now that monolith can actually answer queries over the network to all of these gateways who don’t necessarily need to be running the monolith in the same machine. Here’s an example of maybe going a little more in the middle of the spectrum between replicating all the compute and replicating none of the compute.
Challenges and Considerations
What are some things we got to think about when we’ve built a system like this? Here’s a listicle. One, we’ve got to make sure to replicate well tested code, or else your bugs will replicate too. This architecture assumes that you’ve got some battle tested, hardened logic that’s really important to your business, and that you’ll actually test and make sure that it is stable. Because if you don’t, you’ll just have bugs everywhere. Here’s the other thing. When you’ve got a deterministic system, you got to make sure that all of your replaying services are actually deterministic, they cannot drift. A common source of drift is when you change your business logic. One point you’ve got to keep track of is you’ve got to respect old behavior when replaying inputs. What that means is, let’s say on Thursday, you had some undesirable behavior on your system, and you handled some requests, and then you fixed it on Friday, and you deployed it. If your downstream monoliths are replaying logs from Thursday, then they need to make sure to do that incorrect, undesirable behavior before Friday comes and we actually enable that new behavior. What this means is deploying code should not change the behavior of your system, you’ve got to decouple that and enable behavior changes with an API request to your business logic.
Four, we often need randomness in systems. It gives us uniform distributions, and fairness, load balancing, maybe some security benefits. As it turns out, you can do randomness deterministically. There’s tons of deterministic pseudo algorithms out there for you to pass the seed in. Then, all of your systems that are replicating the input log are going to get the same nicely distributed, random looking output, but it’ll all be exactly the same. Five, this one’s fairly subtle. If you’ve got a single threaded deterministic system, then any long task is going to block every other task in your input log. This is called the convoy effect. If you’ve got requests, especially that maybe will explode into more work and then do a bunch more work in a transaction, consider breaking up that computation into multiple stages and allow more request messages to come in, while you’re tracking the state of that computation. This is a tradeoff, of course, between better consistent performance dealing with the convoy effect, and the complexity of having to manage additional state because now you’ve just split up your computation into multiple stages.
Also, if you want a fast Raft service, everything that you’re persisting between requests, you must fit in memory. That said, there’s a lot of commodity RAM out there now, you’d be surprised. You can fit quite a lot of customer data into hundreds of gigabytes of RAM, especially if you’re being maybe judicious about how you’re storing that. If you’re storing data in fixed length fields, and you’re avoiding arbitrarily nested and variable length data, you can easily store millions of accounts worth of information in a megabyte times the number of 64-bit integers you’re using to actually persist that info. Also, you’d be very surprised what fast single threaded code running on one core can get done. If you’re not concerned about writing to disk with each message and you’re not IO blocked, you can easily keep your processing times of your API calls maybe even under a microsecond. That gets you a throughput of a million messages per second all on one box. Really think about that if you come to the decision where you might want to shard, because sharding introduces tons of complexity into your system.
Nine, keep your 99s and 99.9s down. You’ve got that single threaded service, your response rate for everything is impacted by your slowest requests. When running in this manner, and if you want consistent performance, you’ve really got to apply some craft to make sure you avoid pathological performance cases, and make sure you’re not slowing down all of the other requests by iterating through too many things or something like that. Last, our inputs are more consistent if we validate them to a band of acceptable size. You’ve got to make sure that your log, your monolith is protected from any client that maybe suddenly goes nuts, because you can now easily denial of service your entire system if a client goes haywire, and you’re not applying rate throttles.
Simplicity
All this determinism is motivated by a call to simplicity. In general, we say that simplicity is going to get you stability. It’s going to get you performance and development speed. I’m sure you’ve all seen plenty of talks and had engineering mentors talk about the virtues of keep it simple. Hopefully, I don’t need to sell simplicity to you all. What I’m hoping I got across is that deterministic execution help keeps things simple. Again, you’ve got some important logic, make it deterministic. If you’ve got deterministic logic, trust it, replay it, run it anywhere in production, on your laptop, all over the place.
Questions and Answers
Montgomery: In order for this to work, you need the same state everywhere, that is, no external state. How do you do that? I suspect having all the market data at hand with prices changing very frequently is too large to hold in your local data storage.
Yu: If you’re going to run everything basically in a Raft monolith, you are relying on the fact that your performance and everything is basically all in memory, and you rely on a persisted append only log of the requests to give you all that state. What that means is any external state, you want to be persistent, and you want to have access to in between your messages. They’ve got to fit in memory. I think we addressed this one in the listicle. Basically, if you avoid variable length data, so avoiding strings, using lots of fixed length fields, keep on to registers and primitives, you can store quite a lot of data in memory on one process. The question is, when do you shard? The complexity with sharding, and setting up basically some broker to demultiplex, and all that, is a pretty huge decision. In general, cloud systems and all of that tend to go to that shard mechanic looking for shard axes maybe a little too quickly, because having fewer boxes really does help a lot. Yes, this does not work if all of your market data at hand does not fit in memory, but your market data should ideally be maybe three, four numbers, so that’s 32 bytes, 64 bytes. You can fit quite a lot of market data in your systems.
Montgomery: I think also, too, there’s one difference that I know, it’s very common between a lot of the financial systems that operate in this space and a lot of more consumer facing systems. That is things like you’re not storing names, you’re not storing a whole bunch of other static data in those systems. Because you’re just not actually operating on them. How often does someone change their address? Does your business logic at that point need to know address, for example? There is a lot more that you can get in terms of useful information into a system into memory, when you take a look at it and go, “I don’t care about some of this external stuff, because it’s never going to actually change my business logic,” for example. There is an assumption that some of that information that’s normal for other systems to just have available is usually not available, because it’s pared down.
How do you keep determinism when executing the same input run at different times when replicating? On a stateful system, the market changes over time.
Yu: This piggybacks off an important piece of architecture, called the sequencer architecture, where you basically rely on one leader, the leader of your Raft monolith to timestamp and provide the order of the requests that come in. The problem is that having a gigantic consensus pool, where you’re expecting the shard outward to get you easy reads can quickly backpressure your leader. This idea of basically rerunning monoliths outside of Raft consensus, basically, as an optimization, it’s basically separating the writes, which is the writes to the sequencer, and the reads, which are queries of your state machine. The timestamps stay the same. What happens is Alice’s request to come in is timestamped at Monday, 11:59 p.m. That request that is replicated over to a monolith, maybe it’s replaying some historical stuff, like maybe it’s replaying it days later on Wednesday, but that message still has that timestamp of Monday, 11:59.
Montgomery: In other words, you can say that time is actually injected into the log, you’re not grabbing time some other way. It’s just a function of the log itself.
Yu: Agree. If you are comfortable relying on that one clock, you also avoid things like a clock skew. Fundamentally, if you have clocks running on multiple machines, that’s another distributed consensus problem. Ideally, you try to rely on your solution to the consensus problem to give you the things you need to keep things simple.
Montgomery: This can only work if there is no external state, that is, services are purely compute reliant and nothing else. That’s really how services exist, services rely on external state. Is there a version of this that works with services with external state?
Yu: Just refining what we mean by external state, I think of that as maybe a source of truth, or something that is basically feeding in information into your state machine. What this means is, let’s say, we’ve got some clients who have programs that are interacting with our system, strictly the state of their programs is external to our system. Then, how does that state that they’ve got in their system come to us? They come to us in the form of requests. What that means is, if you want any state to end up in your state machine, you make that an explicit part of the state machine’s API. Therefore, the state comes in. When you need to reconcile that application-level protocol, it’s not data reconciliation protocol. It’s fundamentally business logic that needs to reconcile, you end up actually opening APIs to actually support, say, I need to maybe pass in a snapshot of the old state, or maybe please replay me the stuff that came before. You’ve already got that, because we’re replaying. The world is actually this way, and that state comes into a state machine through the external API of the state machine. The state machine itself is a source of truth for all things that it is keeping track of, and taking snapshots of. Obviously, systems don’t exist in a vacuum, they only have any meaning with regards to their interaction with external systems. If you’ve got some external state that is updating, you will go ahead and pass that into your state machine via API calls. You got to expect that your state machine is fast enough to basically handle all of those changes in external state.
Montgomery: To go back to the idea of an account address, maybe your service does accept changing an address. Could it in a way, execute that by sending that off to something else, and then it comes back in through the log of events that says, this has been updated? In other words, it doesn’t really do it itself, it passes it off to something else, and then it comes back in through the input sequence. Would that work?
Yu: Yes, this works for everything that isn’t secret. I think static data can pass through a system. The throughput of data coming in that’s not really being read that just pass back through is some function of your network card and your disk storage or whatnot. Secrets, I think, don’t really fit. We try not to put secrets into our Raft monolith. What that means is, ok, when a secret changes, the business domain impact of that change should be expressed in some feature. Like if a password changed and has been invalidated, if we’ve invalidated some API key, what has been passed in to the Raft monolith is not the new password or anything, but rather, this thing is now invalidated. If your business logic says validate for a valid API key or something, it should then reject requests that are keyed off that API key. I think this model computation isn’t well suited for being the source of truth on just any secrets or things that you don’t want to basically be spreading everywhere.
Montgomery: Is it safe to say then that those things are really our external services, and whether they influence the logic or not, they influence it in such a way that you can send something into the service to change their behavior, like you were saying, invalidating a key. You’re just invalidating that to give its current impact into the state logic. It means what you need to do then is to make sure that you don’t have a lot of leakage of those kinds of things.
Yu: Absolutely.
Montgomery: Is there other places that you have thought about, since you made the presentation that you could possibly be pushing your logic that is not obvious, since you found this pushing this logic into other places, are you looking at certain problems a little differently now?
Yu: I think in general you want to separate out functional logic that is mission critical. In general, I think the reason we’ve spent a lot of time on trying to make a big portion of our logic mission critical is that running exchanges, almost all of it is. This is quite a big hammer, and you don’t want everything to look like a nail here. When going back to any system, like say, if you’re running something that keeps track of customer funds, or is keeping track of an important case where you have highly concurrent access, that determinism is incredibly useful, like scheduling, anything like that. We try to say we should localize this particular high throughput, low latency approach to only things that you intend to have very good test coverage for, and would really benefit from an explicit ordering of all events that come in. When you have massively parallel systems that are highly independent from each other, you don’t really get as much benefit from having one sequence deterministic stream of requests.
See more presentations with transcripts
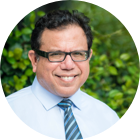
MMS • RSS
Posted on mongodb google news. Visit mongodb google news
MongoDB yesterday added new capabilities to its MongoDB Atlas developer data platform, including generative AI, stream processing and more. The details were announced at the company’s developer conference in New York City.
MongoDB Atlas Vector Search now uses generative AI to create highly relevant data retrieval and personalization into applications for improved customer experiences, the company said in its announcement.
The company also said it enhanced MongoDB Search Nodes to provide a dedicated infrastructure for search use cases, enabling customers to scale the infrastructure independent of the database. This helps them more flexibly and efficiently manage unforeseen spikes and high-throughput workloads.
MongoDB Atlas Stream Processing helps developers build event-driven applications by unifying how they handle data in motion and at rest; MongoDB Time Series collections now have significant scaling and efficiency improvements; and MongoDB Atlas Data Federation and Online Archive have new features for querying data and isolating workloads on Microsoft Azure as well as AWS, the company announced.
Dev Ittycheria, president and CEO at MongoDB, said, “With the new features we’re launching today, we’re further supporting not only customers who are just getting started, but also customers who have the most demanding requirements for functionality, performance, scale, and flexibility so they can unleash the power of software and data to build advanced applications to transform their businesses.”
To learn more about MongoDB Atlas, visit mongodb.com/atlas.
Article originally posted on mongodb google news. Visit mongodb google news
MongoDB’s Expanded Monetizable Opportunities: Atlas Stream Processing and Vector Search
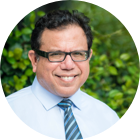
MMS • RSS
Posted on mongodb google news. Visit mongodb google news
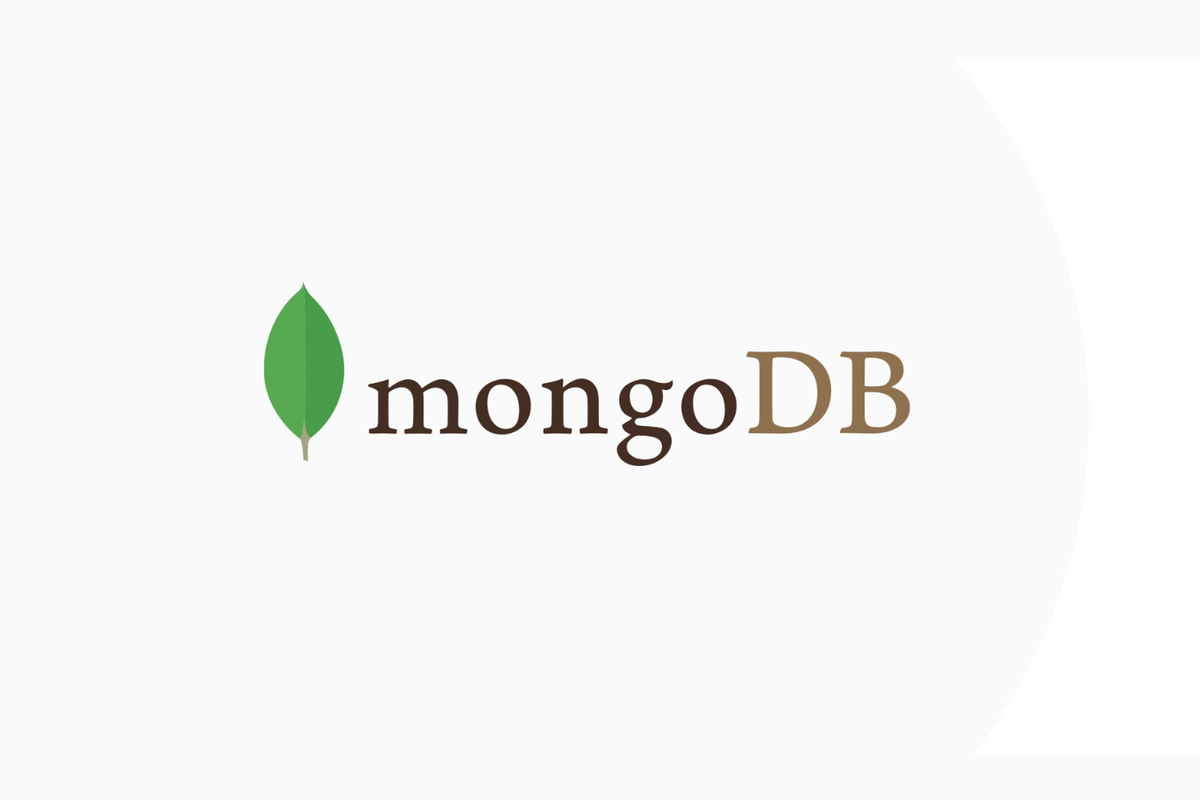
Truist Securities analyst Miller Jump reiterated a Buy rating on the shares of MongoDB, Inc. MDB, raising the price target to $420.00 from $365.
The analyst views the rollout of Atlas Stream Processing and Vector Search as two areas that will expand the monetizable opportunity for the company mid-term.
MongoDB rolled out the Atlas Stream Processing at their NYC local user conference, which will boost the company’s TAM into more real-time use cases.
Jump also believes MongoDB’s solid foothold in the AI ecosystem will support “durable growth” through the remainder of the decade.
The analyst applauds MongoDB’s sales productivity, which continues to increase per rep as the sales force matures while also gaining leverage from technical and sales partnerships.
Jump considers MDB’s margin performance to exceed current Street expectations, serving as a “tailwind for shares.”
On their most recent earnings call, with the guidance bar reset in the 2H23, MongoDB continued its momentum into FY24 with an impressive beat and raise on both the top and bottom lines driven by consumption levels that exceeded their outlook, the analyst added.
Price Action: MDB shares are trading higher by 1.54% to $394.33 on the last check Friday.
Article originally posted on mongodb google news. Visit mongodb google news
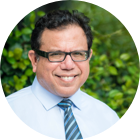
MMS • Mykyta Protsenko
Article originally posted on InfoQ. Visit InfoQ
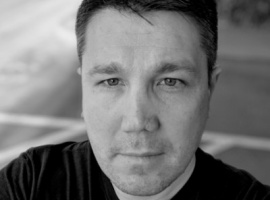
Transcript
Protsenko: My name is Mykyta. I work at Netflix. My job is basically making sure that other developers don’t have to stay at work late. I call it a win when they can leave at 5 p.m., and still be productive. I work in the platform organization, namely in productivity engineering, where we try to abstract toil away for the rest of engineers. Where we try to make sure that the engineers can focus on solving business problems instead of dealing with the same boring technical issues over again.
Let me ask you a few questions. How many of you work in companies that practice, you build it, you run it philosophy? How many of you are happy that you don’t have any gatekeepers between you and production, that you can deliver features and fixes faster? How many of you have ever faced a situation when you’re dealing with a production incident, and you’re wordless what to do, and you wished it would better be somebody else’s problem? Let’s be honest, because I’ve been there quite a few times myself. Yet, I don’t want to go back to the times of having a big old wall between development, QA, and operations, where you write the code and you throw it over the wall to QA, who find bugs. Then they throw it back to you, and so on, until you both agree, it’s good enough. Then you throw the code over another wall to operations, and then you expose it to users. Then your users find bugs. This whole process repeats, usually, being really painful.
Accelerate: State of DevOps 2021
It’s not just my opinion, the State of DevOps survey highlights year after year that companies with a higher degree of DevOps adoption, they perform better. They have a better change failure rate. Basically, their deployments fail less often, and that happens even though they’re doing much more deployments in general. However, this achievement, this win comes with a price, because operating your own code in production means that you have to wear a pager. You need to be ready to respond to incidents. You may need to actually communicate with real customers who may provide valuable feedback, but they also require time and attention. As a result, you can definitely ship code faster. Your code quality improves. The burden of operational duties, it’s non-negligible on top of that. As the shifting left movement is getting traction, we see even more load being added to the developers’ product.
Shifting Left
How does it happen? Let’s review it on the example of shifting left the testing. The traditional software development lifecycle has testing activity as one of the very last steps. Majority of the testing happens after the code is written. By using things like test-driven development, by automating our tests, we can shift the majority of testing activities to the earlier phases, and that reduces the number and the impact of the bugs. Because the earlier we can catch a bug, the less expensive it is. A bug that is caught at the development phase, it means that no users have to suffer from it. A bug that is caught in the planning phase, it means that we don’t even have to waste time and resources writing a code that is faulty, defective by the very design.
Of course, it’s not free. To achieve that, developers have to learn things like testing frameworks, testing methodologies, things like JUnit test driven development, Jenkins’ pipeline DSL, GitHub Actions. They’re just a few things that now become an everyday part of developers’ work. Yet shifting left doesn’t stop with shifting left the testing, the next step may be shifting left security because, yes, on one hand, we need to care about security, especially as we provide developers freedom to design, deploy, and operate on code in production. On the other hand, it means that developers and security specialists now have to work together and developers have to learn and take care of security related things like static code analysis, vulnerability scanning. Shifting left doesn’t even stop there, more processes are being shifted left as we speak, for example, things like data governance. We have a problem, on one hand DevOps as a concept introduced shifting left to all these different practices. It removes barriers. It let us deliver code faster with more confidence. On the other hand, we’ve got ourselves a whole new set of headaches. We need to navigate between two extremes now. We don’t want to introduce a process so rigid that it gets in the way of development and shipping code, yet we cannot avoid the price that we pay to ensure this constant flow of changes. We can and we should try to minimize this price, make it more affordable. Let’s talk about how we can do it. What can we do to make the complexity of DevOps, complexity of shifting left more manageable? What are the problems that we may face along the way?
Looking At History
First, let’s take a look at the history of the problem to see if we can identify the patterns, to see if we can come up with solutions. I’m going to pay attention to several things when I will be reviewing each step of our DevOps journey. I’m going to take a look at communication, basically, how does the problems that we face affect the way we interact? Who’s interacting with who in the first place? I’m going to take a look at tooling. What tools work best for each step of the journey? How do we even set up those tools? I’m going to look at cognitive load that happens during bootstrapping, during creation of the new projects, and during migration. Basically, I’m going to see how easy it is to set things up and how easy are the changes. I’ll also try to illustrate the journey with some examples from my day-to-day work where I can, to make sure that the things I’m talking about are not just hand wavy stuff.
Ad-Hoc DevOps
How does DevOps adoption start? You may have a small company, or a startup, or maybe a separate team in a larger company where people realize they want to ship things fast, instead of building artificial barriers. They want to identify, automate, and eliminate repetitive work, whatever this work is in their case. Now we may have a cross-functional team that works together on a handful of the services. The members of this team, they exchange information mostly in informal conversations. Even if they have documentation, even if this documentation exists, it’s usually pretty straightforward. They may have a Google Doc or a Confluence page that people can just state it as needed. Tooling the automation is also pretty much ad hoc.
Here’s a quick example. We might start with setting up a few GitHub repositories to store the code. We can create an instance of Jenkins service to monitor the changes in those repositories and run the build jobs to create Docker images, and publish them to Artifactory. We may have another set of Jenkins Jobs that we can set up to test the code and another set to deploy them to our Kubernetes cluster. At this stage, we can totally get away with managing those Jenkins Jobs and Kubernetes clusters, manual or semi manual, because it’s not a big effort. It’s literally a matter of copy pasting a few lines of code or settings here and there. At this point, the goal is more about finding the pain points and automating the toil, removing this manual work from the software development process. Those few scripts and jobs that you set up, they provide an obvious win. You have an automated build and deployment process, which is so much better than deploying things manually. Moreover, putting more effort into managing Jenkins and Kubernetes automatically may require more time compared to those small manual changes. After all, at this point is just a few projects and a few people working together. When something is deployed, everybody knows what happened. It’s not like you have hundreds of projects to watch and investing a lot of time into automating something experimental, something that you’re not even sure it’s going to work for you in the long run, it may not be worth it at all.
What about making changes about migration? Sure, let’s spice things up. What if we decide that regular Kubernetes deployments are not enough? What if we want advanced canary analysis and decide to use Spinnaker instead of Jenkins? Because Spinnaker provides canary support out of the box, so let’s go ahead and use it. It’s a great tool. It’s still pretty straightforward. We can decide to do an experiment. We can choose one of the projects and we can keep tinkering until we get everything right, then we can just proceed with the rest of the services. We can keep disabling Jenkins Jobs and enabling Spinnaker pipelines, project by project, because the risk of something going wrong, the risk of something being left in a broken or incomplete state is low. Because you literally can count the number of places where you need to make changes on one hand and you can do this change in one seat. If you have five microservices, and if you need to upgrade a pipeline for each of them, it’s not too hard to find the owners. It may be just a couple of people in your team or company. As for the tooling, basically anything that works for you, if it solves a problem. It basically doesn’t matter yet how you set it up. Yes, there is some additional work for all involved parties but it’s not overwhelming yet because the size of the effort itself is limited. There is an immediate noticeable win. The developers don’t have to worry about manual steps, about repetitive tasks, they can concentrate on coding. That approach reduces cognitive load for developers at this stage where you just have a handful of services.
Here’s a real-life example. Even in Netflix, with all the best practices, with all the automation, there is still room for solutions that are managed manually, for example, this particular application has some caches, and those caches have to be warmed up because the application has to make sure that it has warm caches before it can start accepting production traffic. If we don’t do that, its performance drops dramatically. This pipeline was just created manually by the team who owns this application, and its mind features these custom delays before starting to accept live traffic. Since this is a single pipeline that serves a single application, maintenance and support is not a huge deal, because updating or tuning this pipeline once a quarter or less is not a huge deal. Since the team that manages this pipeline is small, it’s not hard to find the people who are responsible for maintaining it. Updating it is also pretty straightforward, because the pipeline is pretty much self-contained. It doesn’t have any external dependencies that people need to worry about. They can just make whatever changes they need. This manually maintained pipeline provides an immediate benefit. It solves the performance issues that are caused by cold caches, and it removes the cognitive load created by shifting left those operational duties.
What’s the Next Step?
However, as the number of applications keep growing, as they become more complex, we face new challenges because it’s now harder to keep track of what users want. We may have a few applications that are written in Ruby and the rest of applications written in Java. Some of the Java applications may be on Java 11. Some of those Java applications may be on Java 17 already. On top of that, you have more people managing those applications. Even with bootstrapping, when you have new developers who want to create a new service, it’s not easy for those new developers to find the right example, whatever right means in their particular context. Even finding the right person to talk to about this may be challenging.
The maintenance of applications become more problematic as well, because if I have an improvement that I need to share across the whole fleet, I need to track down all their services, all their owners. That’s not easy anymore. For example, if we take our migration scenario, where we set up Spinnaker pipelines with canary deployments, and we keep disabling all Jenkins Jobs, it looks doable on the first glance. However, when we start scaling this approach, we quickly run into our next issue. Once you’re trying to migrate dozens or hundreds of applications, you cannot just keep doing this manually, because you have too many things to keep in mind, too many things that can just go wrong. When you have hundreds of applications, somebody will forget to do something. Somebody will mess up this setting. They will start the migrations, get distracted, will leave an incomplete, broken state. They will forget about that. Imagine what if the production incident happens and you need to figure out what state your application infrastructure is in? People will be just overwhelmed with this amount of work. Just tracking all the changes, coordinating all the efforts is not an easy problem anymore. That’s exactly the problem we started with. We have created more cognitive load for the developers by shifting left those operational duties, and that cognitive load doesn’t bring any immediate benefits.
The Paved Path
Here we come to the concept of paved path. It is known under different names. It’s called paved path in one company. It’s called golden path in other companies, but the result is the same. The paved path is an attempt to find a balance between old school gatekeeping and a free-for-all approach. Usually, once it’s clear that a free-for-all approach produces too much chaos, creates too much of a cognitive load, people will start looking for alternative, for guidance. They start looking for a better solution, and for more centralized solutions. People start to feel a need for experts that can just solve problems for them. Those experts may be just a few volunteers originally or they may compose a separate platform team. Those experts can provide the rest of development with curated solutions, selecting those solutions like curators select art pieces for an exhibition. Here we start to see a separation of responsibilities. Experts choose solutions that work out of the box. They guarantee a certain level of support. Developers are free either to use those solutions, or to experiment with other tools, as long as they understand the consequence of those experiments, as long as they take the responsibility for those experiments.
For example, if Cassandra is provided as a curated solution, and if there is something wrong with your Cassandra clusters, developers may just rely on experts to have a set of metrics, set of alerts to warn about the problem. Developers may rely on experts to diagnose and fix those Cassandra specific problems. Basically, developers don’t have to worry about becoming Cassandra experts themselves. Yet, developers are still free to use any non-standard solution. If they feel like they need to use Neo4j for their particular application, they can do it as long as they are willing to take care of it themselves. Basically, we see the change in interaction, this stage introduces a clear separation of responsibilities. It doesn’t matter yet if this separation is formal, as long as everybody has their understanding, clear expectations set up between those two different roles.
What about the tooling at this stage? Let’s start with bootstrapping. When it comes to bootstrapping, creating a new service, developers don’t want to worry about rediscovering how to bootstrap an application, how to create a new infrastructure each time they create a new service. Having a single point of entry helps here. If you have a developer portal or a command line tool that you just use every day to find your applications, operate them, and create them, it makes that so much easier to find this paved path. You just use this tool or portal every day. You build your muscle memory. Common sense tells us that developers usually have less experience creating new services than maintaining or operating existing ones. If both creation and operating the services uses the same entry point, developers don’t have to remember how to do this less frequent thing that is creating a service, setting up all the related infrastructure. Yes, developers may not remember all the steps. At least they remember that there is a big but, and cold create a new service, and they know where to find it. Or if you have a command line tool, developers can know how to run a help command and find the actual command they need to run.
Let’s talk about the next step. We can use this single point of entry to create whatever infrastructure, whatever resources are needed for the project bootstrapping. What is done exactly? To do it properly, we need to treat our infrastructure as code. Not just operational infrastructure like Kubernetes clusters, load balancers, databases, we need to take care of all infrastructure. Including infrastructure that covers different aspects of software development, different things that are shifted left, like build and deploy, like testing, like security and all other aspects of your software development. We can definitely do it now. We have multiple tools. We have things like GitHub Actions, Jenkins pipeline DSL, other tools that we can just configure as code and use those solutions to address our problems. Why is it important? Why do we want to have that code? Because this way, we can reuse this same code for different projects. Code can and should take inputs, and we can abstract implementation details away. We can let developers answer high level questions, and the code can use those answers as inputs to create the infrastructure we need, eliminating the need to do these repetitive tasks by hand again. It removes the cognitive load that exists, then infrastructure is managed manually.
What code works best here? In my experience, imperative code may not be a good fit. Because, yes, you can write a script to create a new deployment pipeline, for example. Yet it also means that in your imperative code, you have to account for each and every edge case because, basically, you’re saying, please change the current state of the world this particular way. If the current state is not something that you expect, you might face an error because what if the deployment pipeline you’re trying to create, what if it already exists? What if it exists, but has a different configuration, what do you do then? How do you reconcile those states? You need to account for them. I think that declarative approach works so much better for infrastructure. With a declarative approach you can describe infrastructure resource, any resources, basically, by saying, I want the state of the world to be like that. Then your tools figure out whatever changes are necessary to achieve that particular goal. Declarative tools, they give you a benefit of idempotency, because no matter how many times you run them, they will leave your world in the same state each time you run them. You don’t have to create unique deployment scripts. You don’t need to adjust those scripts each time you change something. If you need to make a change, you change the declaration itself, and the declarative tools will take care of whatever steps are needed to make it happen. If you need to provision a pipeline and it doesn’t exist, with declarative approach, it will be created. If it exists already, nothing will happen. If it exists, but if there is a configuration discrepancy, it will be reconciled, and you don’t have to do anything special, the process is the same in all the cases.
Let’s apply this approach to our paved path where we have a separation of responsibilities, where we have experts who work on solutions that developers can use. Now developers can define our paved path using declarative tools. They can define Jenkins Jobs using declarative pipeline DSL, for example. They can describe those jobs as code. They can set up automation that commits this code to the repositories that decide to use the paved path, and developers don’t have to do anything about it. It just works. As for migrations, we can go back to our examples when experts decide to introduce canary deployments, when they decide to migrate to Spinnaker instead of Jenkins. They can set up different declarative code that describes those Spinnaker pipelines, and they can keep using the same approach. Basically, they can keep delivering zero effort migrations to the developers. They just change the declaration and it modifies the infrastructure transparently. Yes, we added a bit of work that a handful of experts have to deal with to reduce a lot of serious cognitive load that unstructured manual solutions create, and scale for everybody, first and foremost, for the developers.
Let’s try to make this example more concrete. If we go back to the concept of single point of entry. In Netflix, we use a command line tool called Newt. We use it for development, because it provides entry points for most common actions for different kinds of projects. Projects like Java projects, Node.js projects, you can still use Newt to build them, or to run and debug them locally. It’s easy to find a command to bootstrap to create a new project. Even if you don’t remember that, you can always call Newt help. When a new project is created, Newt asks a bunch of human friendly questions, human readable questions, and then converts them into the infrastructure we talked about, like Jenkins Jobs, for example. Behind the scenes, it integrates with another in-house declarative tool that in turn takes care of talking to Jenkins, Spinnaker, other solutions. Now experts can codify and templatize those resources that they need to create, like Jenkins Jobs and Spinnaker pipelines. Tooling can help developers configure Jenkins Jobs individually for each particular app. Some settings like application name, or Git repository URL can just be set up automatically in the newly created jobs. On top of that, whole blocks, steps of the process, parts of the jobs can be configured conditionally using the inputs provided by developers. That’s just a small part of the templated engine. Then those templates are rendered to provide whatever output is needed by the system they talk to, like Jenkins. They can use XML to describe Jenkins Jobs. They can use JSON to describe Spinnaker pipelines, and so on.
Then, that declarative tool can take care of creation or updating the resources as needed. Here we have a piece of templatized XML that can take inputs and can produce a proper Jenkins Job. That just solves the problem of bootstrapping. On top of that, it solves the problem of migration too. If experts need to make the change to infrastructure, they can just make the change to this particular template. It can be something as serious as replacing a Jenkins Job with your Spinnaker pipeline, like in our theoretical example, or it can be something much more targeted, like replacing a Jenkins plugin in a specific Jenkins Jobs that we do here. Or it can address security issues in AWS metadata service like in this particular example. When you manage fleets of applications, you know that such changes are regular, they happen often. They happen every week, if not every day. If you have to do them manually, they just turn into death by a thousand cuts. If you can automate this change, if you can just update the declaration and roll it out to the whole fleet, you can save yourself days or weeks of herding the cats, of trying to pull away developers from their regular work, from coding, from solving business problems. You don’t have to force them now to do infrastructure migrations that are not in their duties anymore. When you have dozens of applications, that’s already a significant win, and when you have hundreds or more, this approach is a lifesaver.
One Paved Path?
However, as the company grows, one paved path may not be enough, because for more homogeneous companies, for more homogeneous teams, it may be ok if all developers are working on the same set of problems, it totally makes sense to stick to the same set of solutions. Yet, if your company is big enough, it may no longer be the case. For example, in Netflix, developers who work on streaming projects, they have to deal with high load, high availability systems with clear traffic peaks, because a lot of people come home after work and start watching movies starting at 6 p.m., Eastern time. On the other hand, developers who work on studio automation, those developers solve problems that are no less important. Those problems have totally different aspects, different traffic patterns, different availability requirements, different latency requirements, and so on. What works for studio may not work for streaming and the other way around. For example, canary deployments, where you roll out a new version of your service to just a few percent of your user base. It works fine in streaming, but it may not provide a good reliable signal in studio, just because the size of the user base is so different, it may be different by a few orders of magnitude.
Paved Paths at Scale
A single shared path no longer works in this situation. To make the situation even more complicated, the paths of different paved paths may intersect, for studio, if streaming prefer Java for their backend, it makes sense to implement that part of the paved path only once and then compose those parts needed. What’s the best way to do it? How do we approach composable paved paths? What do we need to implement to enable it? First of all, a single set of nodal experts is not going to work anymore, because, yes, definitely some of these experts can and should work on the pieces that can be reused across the board. Multiple composable paved paths, they actually require specialized knowledge. They require experts who are familiar with a particular domain area, with the problems of a specific organization. We’re going to see an additional separation of duties here. Now we have organization level experts that can work on solutions to be used in their particular team, particular organization. In turn, those experts, they can rely on common platform solutions that are provided by company level experts like a platform organization. If we go back to our example of streaming versus studio, we may have streaming experts who solve problems that are specific to streaming, like, what does it take to safely redeploy a massive fleet of servers while handling regular or daily traffic spikes? Platform experts can provide building blocks that can be reused by both streaming and studio. For example, they can provide managed database solutions or managed security solutions.
Second, when it comes to actual implementation, we’re going to have paths that are identical, like running unit tests, and paths that are different, like using canary deployments, which work much better for high traffic applications. Implementing each path separately means that we are creating a risk of implementing identical things twice, which increase the maintenance burden because things have to be implemented twice at the very beginning. The change also has to be duplicated. In the standard paved path approach, we can just codify the best practices using declarative tools. However, when we have multiple paved paths, when they share certain practices, we need to split the code as well to separate those different practices to keep them apart. For example, if we have a paved path that has a distinct step like build and deploy, then each step should be codified and versioned separately. If those steps depend on each other, the dependencies should be set explicitly like that, because deploy depends on the build, not the other way around.
By doing this, we are going to get a quick win. Because if we know which version of infrastructure is used for which app, we can avoid the mess, like I don’t know what’s happening within app x. We can avoid strict gatekeeping, like it has to be this way because it’s written like that, no other way is possible. We can separate different paved paths and provide different ways of doing things. What’s most important, we can avoid big bad migrations, like let’s migrate everything first because this is the way things should be done, because we only have one paved path and we cannot do anything about it. Instead, we can try new solutions, we can try them with a few select apps for us to see if we have any issues. If there are issues, we can safely go to the previous good version since we’re using a declarative approach. We’re saying, I want the state of the world to be like that. We’re not doing imperative migrations, where we say, do action z with my existing resources to change their state from x to y, because this imperative approach might require another imperative migration to go back to the previous state in case something is wrong. That can be especially tricky if the original rollout was just partially successful, and the actual state is something between x and y.
Let’s see how this approach plays out with our original migration example where we migrate deployment pipelines from Jenkins to Spinnaker. We can have two big infrastructure blocks like build and deploy. We can codify them separately. We can version them separately. We can define version requirements. Since we’re making a breaking change, we can even increment a major version of our deploy step and roll it out first to just a small subset of our applications. Then when we make sure that it doesn’t break anything, we can roll it out to the whole fleet. This way, we can minimize the blast radius, we can maximize our confidence because versioning each part of our infrastructure code separately gives us better visibility, better control. If we decide to keep splitting our paved path into two or more, we can keep deduplicating the common paths and we can keep separate paths separate. Basically, we apply the standard coding practices, versioning, code reuse, controlled rollouts with our paved paths being represented as code. This approach is not limited to updating deployment pipelines. Any solution that you can codify declaratively can be managed this way, be it security, be it testing, be it something else. The result is reduction of cognitive load during migrations for both developers and the experts. Organization level experts don’t have to reinvent the wheel for each separate path. They can reuse common building blocks and they can focus on solving problems, providing curated solutions that are common to a particular team, particular part of their organization or company.
Example: gRPC vs. GraphQL
One example I can mention from my Netflix experience is, splitting regular gRPC applications that are mostly used in streaming and GraphQL applications that are common in studio into two different paths. This way, organization level experts are able to focus on solving their specific task without worrying about the single paved path becoming too bloated, too complex to maintain and manage. Those paths have significant differences. I’m only showing those significantly different paths here. For example, gRPC applications publish the protobuf, the gRPC scanner, the gRPC contract as a part of the client publishing and promotion process. It’s actually included in the client’s jar, maybe a candidate jar or a release jar. Since those applications usually have more predictable traffic, they rely more on canaries. On the other hand, GraphQL application deployment process is more simple. Those applications, they need to rely on a special pipeline stage to publish their GraphQL schemas, the GraphQL contract because they are not published as jar, they are published as standalone GraphQL registry. If we kept all the solutions as part of one spaghetti-like paved path, we don’t have configurations options, this approach would be next to impossible. Separating those paths, teasing them apart, it allowed experts from different organizations to work independently without stepping on each other’s toes.
Things to Watch Out For
You might ask me, it all sounds great, but what are the downsides? What can possibly go wrong here? That’s a great question. That’s a loaded question, because any model looks great in theory, but in practice there’s always that catches you off guard. One of the most common problems in software engineering is solving an interesting problem instead of solving a problem you actually have. Because many people want to do things like Google does, or like Netflix does, and very often it’s the right thing to do. A lot of times, other companies, smaller world companies maybe don’t even have these same kinds of problems that larger companies suffer from. When it comes to DevOps, when it comes to choosing the right tool and then the right approach, it’s the same. We have been talking about different stages of DevOps journey. The tools you’re going to need are different too. It definitely makes sense to first see where you are by just starting, you only have a handful of services to manage. Maybe you already have hundreds of services. It also makes sense to check the dynamics. If you’re going through explosive growth, and you think it’s very likely that 15 of your services will turn into 200 pretty soon, then it may make sense to start planning for the next step, to make sure that you don’t commit to the solution that will hold you back in the near future.
Also, you want to find the right level of abstraction, because too much abstraction may not be a good thing. As we hide things away, you may end up hiding things that may not be essential for day-to-day operation, and you think it’s a great idea, it is going to reduce cognitive load. Those things might be critical when you’re dealing with a production incident. When you’re investigating it. When you’re looking at a broken service. When you’re trying to find out what is wrong. As my colleague Lorin, once noted on Twitter, it’s impossible to find an abstraction that is going to work for everybody. You can make it possible for people to navigate through layers of abstraction, to understand what actually happens. How do you navigate abstractions? Two things are important there. First, you need to understand the effects of your change. You need to understand, what is going to happen if I make this change? Which systems are going to be affected? Second, you need to understand the cause of the change that has already happened. I need to understand things like, if I see my system in this particular state, what was the change to cause it? If your abstractions can help you with that, then you have the right level of abstractions. I just wanted to highlight that ensuring observability in your abstractions may actually improve cognitive load in both day-to-day operations and during production incidents, during the investigation.
Where Do I Start?
You may ask, where do I start? That’s another good question. I’m not going to advocate for any specific tools. I can give you a few examples that you can start with, you can research them. You can see if their competitors are a good fit for you. You can start with Terraform. It’s a well-known player when it comes to infrastructure as code. Its plugin system is extensible. If you need to add custom solutions, it’s easy to do with its plugin system. Its modularization, its versioning approach is worth looking into. It can provide the way to version your infrastructure to manage your paved path. Another declarative tool that I wanted to highlight is Spinnaker’s managed delivery. It focuses on continuous delivery and related infrastructure. I believe that it’s certainly worth looking into. Of course, there are things like GitHub Actions, or Jenkins pipeline DSL. Those things are more generic. They may require other tools to solve your particular problem. They also provide a good degree of flexibility. You can start there. You can research those tools, you can research their competitors, and you can see what is the best fit for your particular situation.
Am I Going the Right Way?
You also may ask, I have started working on my solutions, on my infrastructure, build, deploy, test, and security, whatever problems you’re trying to solve. You want to say I’m working on improving my developers’ experience and reducing cognitive load. How can I say if I’m going the right way? How do I know if my efforts are actually useful? There are metrics that you can use to see if your efforts are producing the right results. Most of your set of metrics is the change rate and the change failure rate. Basically, you can check how fast your deploy changes. How often do deployments fail? Those are proxy metrics. They are affected by other factors, but they also measure a really important thing, like they measure your ability to deliver quality code. Another set of metrics may be time to create, to bootstrap a service and the time to reconfigure the service. You may measure the time it takes to update the infrastructure, time to perform a migration. You may measure it across a single service and across the whole fleet of services if you choose the right abstractions. If you actually reduce the cognitive load, you should see the reduction of these times as well and vice versa. If it takes longer to make a change, then your abstractions might need some tuning.
Questions and Answers
Tucker: Is there a path at Netflix from a custom solution that used to be an experiment to becoming a curated solution? Is there a concept of a grassroots curated solution?
Protsenko: There are two sides to this problem. Definitely, I can think of examples where developers at Netflix started working on some solutions, and they realized it works for their teams, and it was scaled. At Netflix, we actually have a notion of local central teams, of teams and developers that are responsible for helping the developers in their particular organizations, like local organizational level experts that have the expertise. That does correlate to the grassroot problem to scaling grassroot solutions. One thing that wasn’t in the question that I’d like to elaborate on, yes, if you have the tooling in place, if you can define your infrastructure, if you can scale it, basically, you may be free to scale your homebrewed solution. There is a caveat, it’s not just about scaling a particular technical solution and approach, because these solutions have to be maintained. The developer will have to wear an expert’s hat, and they will have to be responsible for doing customer support, performing migrations, and that can be a barrier.
Unfortunately, there is no magic way to get rid of the barrier. We can try to lower the threshold, especially when we’re dealing with support at scale. When we have dedicated support teams helping those developers/experts to scale the approach, but it’s something that you have to be aware of before you go into this territory. Another thing that I saw examples of it being used, and being encoded, incorporated as a paved path solution, is when you have an escape hatch in your paved path. When your paved path defines some custom hooks, some custom plugins that other people can lean into. When experts see these Jenkins Jobs being defined and called by other customers during this custom hook, and there is a lot of need for that, maybe we should take the burden off and incorporate and maintain it themselves. It’s less about the tooling. It’s more about the communication and the support burden. That’s the stuff I wanted to emphasize here.
Tucker: Can you share an example of a real-world migration that you’ve simplified or enabled using the building blocks you described? How did that go? What was the impact of that?
Protsenko: With AWS metadata service introducing a new security option that had to be enabled across the whole fleet, just flipping this flag to true across the hundreds and thousands of applications, is a task that takes probably months to perform manually. When those applications are codified, just changing this flag, and switching it, things like introducing IPv6 for services, or enabling specific types of canaries when you have to replace a certain stage inside a Spinnaker pipeline is another good example. The change by itself is not hard, like there is a new canary type being rolled out. Canaries are already incorporated. We’re just doing the replacement. Sending the email to the developers and asking them to do it will lead to huge delays. They have other better stuff to do. When you have everything that is codified, we just make the change, roll it out to a set of applications to make sure nothing is broken. Then we propagate the change to the rest of the applications using this paved path.
Tucker: Do you think of your paved path as a product where a team is accountable? I want to elaborate on that a little bit. Could you walk us through for your use cases like the streaming, and the studio use case, who was responsible for curating that paved path? How did that go?
Protsenko: With both examples, we have separate teams responsible for maintaining their respective paved paths. I’d like to focus on the product part, because I already mentioned wearing a product manager hat. I have to admit, as a part of the team that is actually responsible for curating streaming solutions, we don’t always have an access to dedicated product managers. We did have to wear that hat. We went and we interviewed the customers. We actually created the framework, trying to assess the importance of different problems. We used the dimension as the impact, like, how important a specific problem is. The blast radius, the reach, how many customers are affected by any specific problem, and looking through these dimensions? We just tried to sort whatever the main pain points are. Because there are more problems that we can solve at any given time. Just sorting and prioritizing them is important before you start actually incorporating them into the paved path. That is the biggest challenge when you’re approached by paths of product.
See more presentations with transcripts
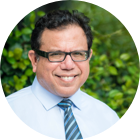
MMS • RSS
Posted on mongodb google news. Visit mongodb google news
NEW YORK, June 23, 2023 — MongoDB, Inc. has announced MongoDB Atlas for Industries, a new program to help organizations accelerate cloud adoption and modernization by leveraging industry-specific expertise, programs, partnerships, and integrated solutions. With MongoDB Atlas for Industries, customers have access to expert-led architectural design reviews, technology partnerships that deliver enhanced solutions for industry-specific challenges, and industry-specific knowledge accelerators to provide highly relevant technology training paths for development teams.
MongoDB Atlas for Industries is launching its first set of vertical solutions for financial services, an industry that is changing rapidly with shifts in automation and technology advancements. With MongoDB Atlas, financial institutions can improve customer experiences by modernizing legacy functionality on existing in-house banking systems and building composable architectures—architectures that make it easy to integrate industry-leading software tools—to get ideas to market faster with the performance and scale they need. MongoDB Atlas for Industries programs for manufacturing and automotive, insurance, healthcare, retail and other industries will follow over the course of the year. To get started, visit mongodb.com/industries.
Tens of thousands of customers rely on MongoDB to power their applications every day, but each industry has its own unique set of challenges and needs. Many industries are also at a crossroads between being restricted by the capabilities of their legacy environments and the opportunity that is being presented by next-generation applications, data analytics, and generative AI. However, additional pressures like cost optimization can make it seem like transformation efforts are being stifled. Despite all of this, many organizations still feel a sense of urgency to modernize workloads for their most mission-critical applications and innovate quickly to remain competitive and meet customer demands. When providers can offer experts who understand the nuances and have deep experience working in a specific industry along with their technology solutions, it delivers the extra support organizations need to get started.
MongoDB Atlas for Industries provides:
- Industry Innovation Workshops: dedicated executive engagement with industry experts from MongoDB and our partners to discuss client-specific solutions using best practices developed through proven industry experience
- Industry Access Pass: access to MongoDB’s industry-specific partner integrations and toolchains to evaluate how to accelerate innovation and modernization projects
- Industry Jumpstart: building on the innovation workshops, organizations can engage with the MongoDB professional services team to accelerate a project from concept towards the production of a minimum viable product
- Industry Knowledge Accelerators: tailored MongoDB University courses and learning materials, including unlimited access to curated webinars and solutions sessions, to enable developer success
MongoDB Atlas for Financial Services
With changes in customer demands, regulatory compliance, and new players challenging incumbents, the financial services industry, which has traditionally been conservative in its adoption of new technology, is under increasing pressure to modernize applications and innovate consumer experiences. MongoDB Atlas is ideally built to help with this modernization, as well as enable organizations to build next-generation applications that take advantage of new technologies like generative AI. MongoDB Atlas also provides the resilience, scale, and highest levels of data privacy and regulatory compliance that MongoDB financial customers require.
As part of the MongoDB Atlas for Financial Services launch, MongoDB has achieved Amazon Web Services (AWS) Financial Services Competency. To obtain this competency, MongoDB was tested against strict security, operational, and reliability requirements, validating that MongoDB and AWS can help financial institutions get ideas to market faster, while reducing cost and enhancing business agility. This builds on MongoDB’s long standing relationship with AWS, including MongoDB Atlas’ availability in the AWS Marketplace. Additionally, MongoDB has become a critical foundation of the Temenos Banking Cloud, with a recent benchmark proving that together, Temenos and MongoDB can support the needs of even the largest global banks with exceptionally high performance while providing transparent data access through the JSON document model.
“Increasingly organizations in different industries are moving away from one-size-fits-all technology solutions to improve their competitive advantage and better serve customers. At MongoDB, we’ve built a team of experts with deep industry knowledge, many of whom used to be customers, so we are not only building better products with the right third party integrations, but our team can help customers get up and running faster,” said Boris Bialek, Managing Director of Industry Solutions at MongoDB. “Nineteen out of the top 20 banks in North America already use MongoDB in their infrastructure to solve some of their most critical problems. Launching Atlas for Industries is our commitment to continuing this level of dedication across financial services and other key industries.”
About MongoDB
Headquartered in New York, MongoDB’s mission is to empower innovators to create, transform, and disrupt industries by unleashing the power of software and data. Built by developers, for developers, our developer data platform is a database with an integrated set of related services that allow development teams to address the growing requirements for today’s wide variety of modern applications, all in a unified and consistent user experience. MongoDB has tens of thousands of customers in over 100 countries. The MongoDB database platform has been downloaded hundreds of millions of times since 2007, and there have been millions of builders trained through MongoDB University courses. To learn more, visit mongodb.com.
Source: MongoDB
Article originally posted on mongodb google news. Visit mongodb google news