Month: June 2023
Microsoft Empowers Government Agencies with Secure Access to Generative AI Capabilities
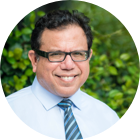
MMS • Steef-Jan Wiggers
Article originally posted on InfoQ. Visit InfoQ
Microsoft continues to prioritize the development of cloud services that align with US regulatory standards and cater to government requirements for security and compliance. The latest addition to their tools is the integration of generative AI capabilities through Microsoft Azure OpenAI Service, which aims to enhance government agencies’ efficiency, productivity, and data insights.
Microsoft recognizes that government agencies handle sensitive data and have stringent security needs. To address this, Microsoft offers Microsoft Azure Government a cloud solution that adheres to strict security and compliance standards, ensuring that sensitive government data is appropriately protected.
Traditionally, large language models powering generative AI tools were available exclusively in the commercial cloud. However, Microsoft has designed a new architecture that enables government agencies to access these language models from Azure Government securely. This ensures that users can maintain the security required for government cloud operations.
Azure Government customers, including US federal, state, and local government agencies and their partners, can now leverage the Microsoft Azure OpenAI Service. Purpose-built, AI-optimized infrastructure provides access to advanced generative models developed by OpenAI, such as GPT-4, GPT-3, and Embeddings. These models can be adapted to specific tasks, including content generation, summarization, semantic search, and natural language-to-code translation.
Access to the Azure OpenAI Service can be achieved through REST APIs, the Python SDK, or the web-based interface in the Azure AI Studio. With Azure OpenAI Service, government customers, and partners can scale up and operationalize advanced AI models and algorithms. Developers can leverage pre-trained GPT models to build and deploy AI-enabled applications quickly and with minimal effort.
Azure Government network connectivity directly peers with the commercial Azure network, providing routing and transport capabilities to the internet and the Microsoft Corporate network. Azure Government applies extra protections and communication capabilities to limit its exposed surface area. All Azure traffic within or between regions is encrypted using AES-128 block cipher and remains within the Microsoft global network backbone without entering the public internet.
Source: https://azure.microsoft.com/en-us/blog/unlock-new-insights-with-azure-openai-service-for-government/
The capabilities offered by Azure OpenAI Service can significantly benefit government customers. These include accelerating content generation, streamlining content summarization, optimizing semantic search, and simplifying code generation. Government agencies can improve their operational efficiency and decision-making processes by automating responses, generating summaries, enhancing information discovery, and using natural language queries.
In addition, Aydin Aslaner, a senior regional sales director & cybersecurity business head at Microsoft, listed some examples for government agencies in a Linked In post:
- In #healthcare: GPT-4 can help with triaging patients, answering common questions, and providing personalized advice based on medical records and symptoms.
- In #education: GPT-4 can assist with student counseling, career guidance, and content creation for personalized learning.
- In #justice: GPT-4 can aid with legal research, document analysis, and case management, improving efficiency and accuracy.
For customers who meet specific criteria and use cases, Microsoft allows modifying Azure OpenAI content management features, including data logging. If approved, no prompts and completions associated with the configured Azure subscription are stored in Azure commercial.
Microsoft Azure Government maintains strict compliance standards to protect data, privacy, and security and provides an approval process to modify content filters and data logging. By completing this process, customers can ensure no logging data exists in Azure commercial. Microsoft’s Data, privacy, and Security for Azure OpenAI Service website provides detailed instructions and examples on modifying data logging settings.
Lastly, more details on Azure OpenAI Service are available on the documentation landing page and FAQs.
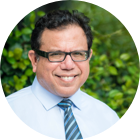
MMS • RSS
Posted on mongodb google news. Visit mongodb google news
MongoDB, Inc. (NASDAQ:MDB – Free Report) was the recipient of some unusual options trading activity on Wednesday. Traders purchased 23,831 put options on the company. This is an increase of approximately 2,157% compared to the average daily volume of 1,056 put options.
MongoDB Trading Up 1.2 %
Shares of MDB stock opened at $402.99 on Friday. The business’s 50 day moving average is $308.79 and its two-hundred day moving average is $244.13. MongoDB has a twelve month low of $135.15 and a twelve month high of $414.48. The company has a market capitalization of $28.22 billion, a P/E ratio of -86.29 and a beta of 1.04. The company has a current ratio of 4.19, a quick ratio of 4.19 and a debt-to-equity ratio of 1.44.
MongoDB (NASDAQ:MDB – Free Report) last posted its quarterly earnings results on Thursday, June 1st. The company reported $0.56 earnings per share for the quarter, beating analysts’ consensus estimates of $0.18 by $0.38. MongoDB had a negative return on equity of 43.25% and a negative net margin of 23.58%. The firm had revenue of $368.28 million for the quarter, compared to the consensus estimate of $347.77 million. During the same period in the previous year, the business earned ($1.15) earnings per share. The company’s revenue for the quarter was up 29.0% compared to the same quarter last year. Equities analysts forecast that MongoDB will post -2.85 EPS for the current year.
Analyst Upgrades and Downgrades
Several analysts have recently commented on the stock. Mizuho increased their target price on shares of MongoDB from $220.00 to $240.00 in a report on Friday, June 23rd. Robert W. Baird increased their target price on shares of MongoDB from $390.00 to $430.00 in a research report on Friday, June 23rd. JMP Securities increased their target price on shares of MongoDB from $245.00 to $370.00 in a research report on Friday, June 2nd. Guggenheim lowered shares of MongoDB from a “neutral” rating to a “sell” rating and raised their price objective for the company from $205.00 to $210.00 in a research report on Thursday, May 25th. They noted that the move was a valuation call. Finally, KeyCorp raised their price objective on shares of MongoDB from $229.00 to $264.00 and gave the company an “overweight” rating in a research report on Thursday, April 20th. One equities research analyst has rated the stock with a sell rating, three have assigned a hold rating and twenty-one have assigned a buy rating to the company. Based on data from MarketBeat, the company currently has an average rating of “Moderate Buy” and an average price target of $366.30.
Insider Buying and Selling at MongoDB
In other news, CEO Dev Ittycheria sold 49,249 shares of the business’s stock in a transaction that occurred on Monday, April 3rd. The shares were sold at an average price of $227.55, for a total value of $11,206,609.95. Following the completion of the transaction, the chief executive officer now directly owns 222,311 shares in the company, valued at approximately $50,586,868.05. The sale was disclosed in a filing with the Securities & Exchange Commission, which is available through this link. In other MongoDB news, Director Hope F. Cochran sold 2,174 shares of the company’s stock in a transaction that occurred on Thursday, June 15th. The shares were sold at an average price of $373.19, for a total value of $811,315.06. Following the completion of the transaction, the director now directly owns 8,200 shares in the company, valued at approximately $3,060,158. The sale was disclosed in a filing with the SEC, which is available at this hyperlink. Also, CEO Dev Ittycheria sold 49,249 shares of the company’s stock in a transaction on Monday, April 3rd. The shares were sold at an average price of $227.55, for a total transaction of $11,206,609.95. Following the completion of the sale, the chief executive officer now directly owns 222,311 shares in the company, valued at $50,586,868.05. The disclosure for this sale can be found here. Insiders sold 108,856 shares of company stock valued at $27,327,511 in the last quarter. Company insiders own 4.80% of the company’s stock.
Hedge Funds Weigh In On MongoDB
Several institutional investors have recently added to or reduced their stakes in the business. 1832 Asset Management L.P. raised its position in shares of MongoDB by 3,283,771.0% in the 4th quarter. 1832 Asset Management L.P. now owns 1,018,000 shares of the company’s stock valued at $200,383,000 after purchasing an additional 1,017,969 shares in the last quarter. Price T Rowe Associates Inc. MD raised its stake in MongoDB by 13.4% during the first quarter. Price T Rowe Associates Inc. MD now owns 7,593,996 shares of the company’s stock valued at $1,770,313,000 after buying an additional 897,911 shares in the last quarter. Renaissance Technologies LLC lifted its holdings in MongoDB by 493.2% during the 4th quarter. Renaissance Technologies LLC now owns 918,200 shares of the company’s stock worth $180,738,000 after buying an additional 763,400 shares during the last quarter. Norges Bank bought a new position in MongoDB during the 4th quarter worth approximately $147,735,000. Finally, Champlain Investment Partners LLC bought a new position in MongoDB during the 1st quarter worth approximately $89,157,000. 89.22% of the stock is currently owned by institutional investors and hedge funds.
MongoDB Company Profile
MongoDB, Inc provides general purpose database platform worldwide. The company offers MongoDB Atlas, a hosted multi-cloud database-as-a-service solution; MongoDB Enterprise Advanced, a commercial database server for enterprise customers to run in the cloud, on-premise, or in a hybrid environment; and Community Server, a free-to-download version of its database, which includes the functionality that developers need to get started with MongoDB.
Featured Articles
Receive News & Ratings for MongoDB Daily – Enter your email address below to receive a concise daily summary of the latest news and analysts’ ratings for MongoDB and related companies with MarketBeat.com’s FREE daily email newsletter.
Article originally posted on mongodb google news. Visit mongodb google news
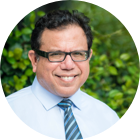
MMS • Karsten Silz
Article originally posted on InfoQ. Visit InfoQ
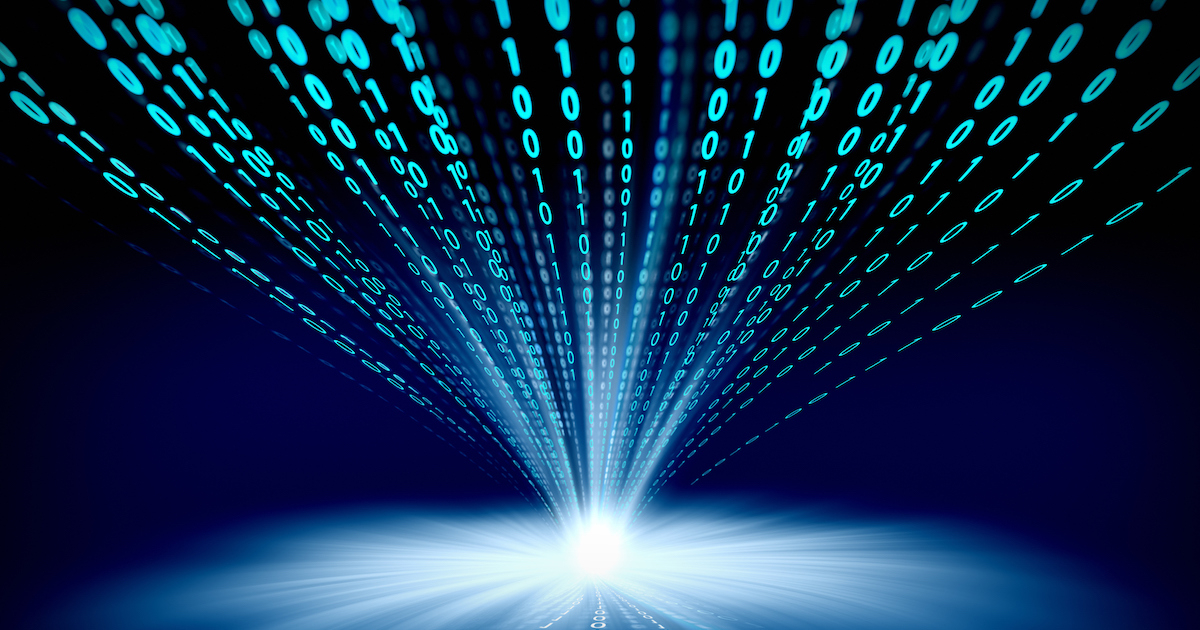
The OpenJDK project CRaC (Coordinated Restore at Checkpoint) drastically reduces the startup time of a Java application and its Time to Peak performance. It does so by taking a memory snapshot at runtime and restoring it in later runs. Azul, the creator of CRaC, now ships an OpenJDK 17 distribution with built-in support for CRaC. Micronaut and Quarkus already support CRaC, and Spring Framework will do so in November 2023.
Azul provided benchmark numbers from 2022 for the Time To First operation of Java applications. A Miconaut application dropped from 1s to 46ms with CRaC, a Quarkus application from 1s to 53ms, and a Spring Boot application from 3.9s to 38ms.
CRaC exists because of the “long-term pain points of Java’s slow startup time, slow Time to Peak performance, and large footprint”, as Java Language Architect Mark Reinhold stated in April 2020. CRaC solves the slow startup time and the slow Time to Peak performance. The GraalVM Native Image Ahead-of-Time (AOT) compiler solves all three pain points but at the price of more constraints and a potentially more expensive troubleshooting process.
It’s rather apparent that loading a snapshot of a Java application will cut down its startup time: Everything is already initialized, and all necessary objects are already created. What is less obvious is that this can also solve the slow Time to Peak: The JIT compiler also stores profiling data and machine code in Java objects. So, taking a snapshot after the JIT compiler created the optimal machine code (e.g., after a couple of hours or even days) means immediately starting with optimized performance when restoring that snapshot.
CRaC only works on the Linux operating system at this time because it relies on the Linux feature Checkpoint/Restore In Userspace (CRIU). On other operating systems, CRaC has a no-op implementation for creating and loading snapshots.
CRaC requires all files and network connections to be closed before taking a snapshot and then re-opened after restoring it. That‘s why CRaC requires support in the Java runtime and the framework. The CRaC API also allows Java applications to act before a snapshot is taken and after it’s restored. Applications can take a snapshot through a CRaC API call or with the Java utility jcmd
. The Azul OpenJDK loads a snapshot with the -XX:CRaCRestoreFrom
command-line option.
The Spring team has a sample Spring Boot project with CRaC using the first milestone release of Spring Framework 6.1. The CRaC team created a sample Quarkus project with CRaC.
Simon Ritter, deputy CTO at Azul, was kind enough to answer questions about CRaC.
InfoQ: You have worked on CRaC for several years. How do you feel now that CRaC ships in an Azul OpenJDK distribution and is also embraced by Java frameworks?
Simon Ritter: Yes, this has been a project Azul has been working on for some time. It was great when it was accepted as a project under OpenJDK. We are very happy that we have now produced a distribution that includes CRaC, which is production-ready and supported, rather than just being a proof-of-concept.
InfoQ: What are Azul’s plans for supporting CRaC in other Java versions than 17, such as the upcoming Java 21 LTS release?
Ritter: Since we have done the work for JDK 17, moving to newer JDKs will be straightforward. We anticipate having this available soon after the release of JDK 21 (although we do not have a confirmed date for this).
InfoQ: To your knowledge, what other Java distributions plan to ship CRaC support?
Ritter: We are not aware of any other distributions with plans to support CRaC at this time.
InfoQ: AWS uses CRaC to speed up Java code in its Lambda serverless offering (“AWS Snap Start”). What other planned uses of CRaC in cloud platforms are you aware of?
Ritter: We are not aware of any other cloud-specific offerings at the moment.
InfoQ: How do you define success for CRaC? And how do you measure it?
Ritter: CRaC is one solution to the issue of startup times for JVM-based applications. Success for this project will certainly be where people use it in production to improve performance. We can measure this by how many people are downloading our Azul Zulu builds of OpenJDK with CRaC included. Personally, I would see the ultimate success for CRaC to be included in the mainstream OpenJDK project. We’re some way off from that, though!
InfoQ: Let’s say an application is run in various configurations – different parameters, heap sizes, garbage collectors, or even processor architectures (such as x64 vs. ARM). How portable is the snapshot file between these configurations?
Ritter: Since CRaC takes a snapshot of a running application, portability is very narrow. You need to restore the snapshot on the same architecture, so trying to restore an x64 image on an ARM-based machine will fail. Even using x86, you will need to make sure that the microarchitecture is compatible. For example, a checkpoint made on a Haswell x64 processor will not run on an older Sandy Bridge processor but should run on a newer Ice Lake processor.
When you restore from an image, you do not specify any command line parameters for things like GC, heap size, etc., as these are already included in the checkpoint. The only way to change these parameters is after the restore using tools like
jcmd
.
InfoQ: How portable is a snapshot file between different versions of an application in an identical runtime configuration?
Ritter: A checkpoint is made of a running application. So restoring it simply restarts the same application. There is no ability to change the application version, and CRaC is not intended for this.
InfoQ: What are the best practices for creating and managing snapshot files?
Ritter: We haven’t really developed any specific best practices for this yet.
InfoQ: CRaC only works on Linux. But most developers either use Windows or macOS. What are your CRaC debugging and troubleshooting recommendations to these developers?
Ritter: The simplest way to use CRaC on either Windows or Mac (at least for development) is to use something like a Docker image or a virtual Linux machine.
InfoQ: CRaC is an OpenJDK project. What are the prospects of including CRaC in a future Java version?
Ritter: We are definitely some way from this. CRaC has been designed to be independent of the underlying OS, but, at the moment, we are using Linux CRIU to simplify persisting the JVM state. To run on Windows or Mac, equivalent functionality would need to be developed, which is a non-trivial task.
Even if we had CRaC running cross-platform, we would still need to convince the OpenJDK architects to add it to the mainstream project. As this is quite a significant feature, that will take both time and effort. There are other projects, like Leyden, that are evaluating alternative strategies for solving this problem.
InfoQ: What’s one thing most people don’t know about CRaC but should?
Ritter: Maybe it’s not that they don’t know, but it’s worth reiterating: When you restore an image, you get the same level of performance you had before, as all JIT-compiled code is included in the snapshot.
InfoQ: You sometimes mention that perhaps CRaC should get a new name, as it shares its pronunciation with a dangerous drug. It seems that ship has sailed – or has it?
Ritter: I think the name will stick. I tend to use the issue more as a joke when I do these presentations. I don’t think anyone will really get confused between our JVM technology and a drug.
:-)
InfoQ: Simon, thank you for this interview!
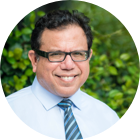
MMS • RSS
Posted on mongodb google news. Visit mongodb google news
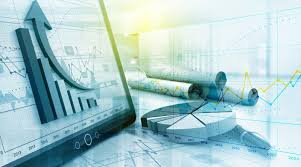
MongoDB Inc. (NASDAQ:MDB) price on Thursday, June 29, rose 1.32% above its previous day’s close as an upside momentum from buyers pushed the stock’s value to $403.26.
A look at the stock’s price movement, the level at last check in today’s session was $398.02, moving within a range at $387.01 and $414.48. The beta value (5-Year monthly) was 1.04. Turning to its 52-week performance, $414.48 and $135.15 were the 52-week high and 52-week low respectively. Overall, MDB moved 37.93% over the past month.
25-cent Stock Takes $11T Commodities Sector Digital
One brilliantly-run technology firm has successfully partnered with some of the largest players in the industry to bring a first-of-its-kind digital solution to the global commodities supply chain sector. Best of all, this upstart technology firm is currently trading undiscovered — below 25-cents per share — so very, very few investors know about it yet! For investors… it’s an early-stage opportunity in a company that’s bringing the US$11T global commodities sector straight into the 21st century.
All the details are in the FREE online report you can get here.
Sponsored
MongoDB Inc.’s market cap currently stands at around $26.98 billion, with investors looking forward to this quarter’s earnings report slated for Aug 29, 2023 – Sep 04, 2023. Analysts project the company’s earnings per share (EPS) to be $0.46, which has seen fiscal year 2024 EPS growth forecast to increase to $1.53 and about $2.11 for fiscal year 2025. Per the data, EPS growth is expected to be 88.90% for 2023 and 37.90% for the next financial year.
Analysts have a consensus estimate of $392.13 million for the company’s revenue for the quarter, with a low and high estimate of $388 million and $410 million respectively. The average forecast suggests up to a 29.10% growth in sales growth compared to quarterly growth in the same period last fiscal year. Wall Street analysts have also projected the company’s year-on-year revenue for 2024 to grow to $1.55 billion, representing a 20.50% jump on that reported in the last financial year.
Revisions could be used as tool to get short term price movement insight, and for the company that in the past seven days was 1 upward and no downward review(s). Turning to the stock’s technical picture we see that short term indicators suggest on average that MDB is a 100% Buy. On the other hand, the stock is on average a 100% Buy as suggested by medium term indicators while long term indicators are putting the stock in 100% Buy category.
27 analyst(s) have given their forecast ratings for the stock on a scale of 1.00-5.00 for a strong buy to strong sell recommendation. A total of 5 analyst(s) rate the stock as a Hold, 17 recommend MDB as a Buy and 4 give it an Overweight rating. Meanwhile, 0 analyst(s) rate the stock as Underweight and 1 say it is a Sell. As such, the average rating for the stock is Overweight which could provide an opportunity for investors keen on increasing their holdings of the company’s stock.
MDB’s current price about 8.10% and 33.89% off the 20-day and 50-day simple moving averages respectively. The Relative Strength Index (RSI, 14) currently prints 71.61, while 7-day volatility ratio is 5.76% and 4.66% in the 30-day chart. Further, MongoDB Inc. (MDB) has a beta value of 1.07, and an average true range (ATR) of 17.43. Analysts have given the company’s stock an average 52-week price target of $401.50, forecast between a low of $210.00 and high of $445.00. Looking at the price targets, the low is 47.92% off recent price level in today’s trading while to achieve the yearly target high, it has to move -10.35%. Nonetheless, investors will most likely welcome a -4.28% jump to $420.50 which is the analysts’ median price.
In the market, a comparison of MongoDB Inc. (MDB) and its peers suggest the former has performed considerably stronger. Data shows MDB’s intraday price has changed 1.32% today and 44.88% over the past year. Comparatively, Progress Software Corporation (PRGS) has moved 1.94% so far today and only 11.97% in the past 12 months. Looking at another peer, we see that Pixelworks Inc. (PXLW) price has dipped -0.29% on the day. However, the stock is -7.75% off its price today a year ago. Elsewhere, the overall performance for the S&P 500 and Dow Jones Industrial shows that the indexes are up 0.32% and 0.64% respectively on the day as seen in early trades.
If we refocus on MongoDB Inc. (NASDAQ:MDB), historical trading data shows that trading volumes averaged 2.1 million over the past 10 days and 1.88 million over the past 3 months. The company’s latest data on shares outstanding shows there are 70.18 million shares.
The 2.60% of MongoDB Inc.’s shares are in the hands of company insiders while institutional holders own 92.40% of the company’s shares. Also important is the data on short interest which shows that short shares stood at 3.76 million on Jun 14, 2023, giving us a short ratio of 1.64. The data shows that as of Jun 14, 2023 short interest in MongoDB Inc. (MDB) stood at 5.33% of shares outstanding, with shares short falling to 3.78 million registered in May 14, 2023. Current price change has pushed the stock 104.87% YTD, which shows the potential for further growth is there. It is this reason that could see investor optimism for the MDB stock continues to rise going into the next quarter.
Article originally posted on mongodb google news. Visit mongodb google news
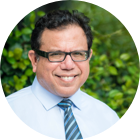
MMS • RSS
Posted on mongodb google news. Visit mongodb google news
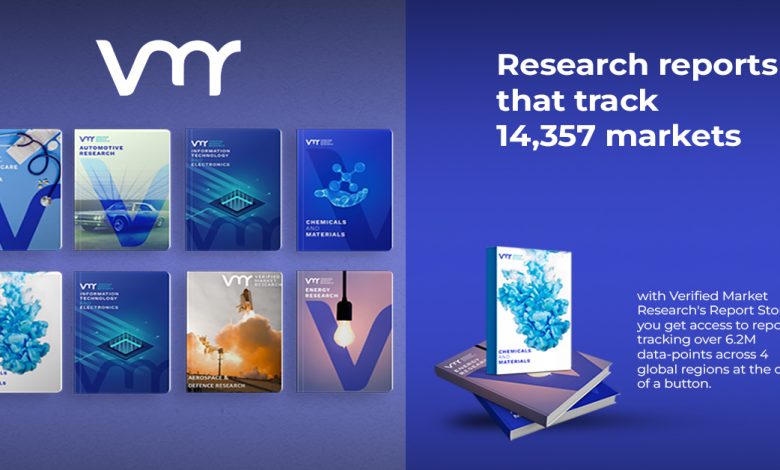
New Jersey, United States – The market research report offers an elaborate study of the Global NoSQL Software Market to help players prepare themselves well to tackle future growth challenges and ensure continued business expansion. With flawless analysis, in-depth research, and accurate forecasts, it provides easy-to-understand and reliable studies on the Global NoSQL Software market backed by statistics and calculations that have been finalized using a rigorous validation procedure. The report comes out as a comprehensive, all-embracing, and meticulously prepared resource that provides unique and deep information and data on the Global NoSQL Software market. The authors of the report have shed light on unexplored and significant market dynamics, including growth factors, restraints, trends, and opportunities.
The vendor landscape and competitive scenarios of the Global NoSQL Software market are broadly analyzed to help market players gain competitive advantage over their competitors. Readers are provided with detailed analysis of important competitive trends of the Global NoSQL Software market. Market players can use the analysis to prepare themselves for any future challenges well in advance. They will also be able to identify opportunities to attain a position of strength in the Global NoSQL Software market. Furthermore, the analysis will help them to effectively channelize their strategies, strengths, and resources to gain maximum advantage in the Global NoSQL Software market.
Get Full PDF Sample Copy of Report: (Including Full TOC, List of Tables & Figures, Chart) @ https://www.verifiedmarketresearch.com/download-sample/?rid=153255
Key Players Mentioned in the Global NoSQL Software Market Research Report:
Amazon, Couchbase, MongoDB Inc., Microsoft, Marklogic, OrientDB, ArangoDB, Redis, CouchDB, DataStax.
Global NoSQL Software Market Segmentation:
NoSQL Software Market, By Type
• Document Databases
• Key-vale Databases
• Wide-column Store
• Graph Databases
• Others
NoSQL Market, By Application
• Social Networking
• Web Applications
• E-Commerce
• Data Analytics
• Data Storage
• Others
The report comes out as an accurate and highly detailed resource for gaining significant insights into the growth of different product and application segments of the Global NoSQL Software market. Each segment covered in the report is exhaustively researched about on the basis of market share, growth potential, drivers, and other crucial factors. The segmental analysis provided in the report will help market players to know when and where to invest in the Global NoSQL Software market. Moreover, it will help them to identify key growth pockets of the Global NoSQL Software market.
The geographical analysis of the Global NoSQL Software market provided in the report is just the right tool that competitors can use to discover untapped sales and business expansion opportunities in different regions and countries. Each regional and country-wise Global NoSQL Software market considered for research and analysis has been thoroughly studied based on market share, future growth potential, CAGR, market size, and other important parameters. Every regional market has a different trend or not all regional markets are impacted by the same trend. Taking this into consideration, the analysts authoring the report have provided an exhaustive analysis of specific trends of each regional Global NoSQL Software market.
Inquire for a Discount on this Premium Report @ https://www.verifiedmarketresearch.com/ask-for-discount/?rid=153255
What to Expect in Our Report?
(1) A complete section of the Global NoSQL Software market report is dedicated for market dynamics, which include influence factors, market drivers, challenges, opportunities, and trends.
(2) Another broad section of the research study is reserved for regional analysis of the Global NoSQL Software market where important regions and countries are assessed for their growth potential, consumption, market share, and other vital factors indicating their market growth.
(3) Players can use the competitive analysis provided in the report to build new strategies or fine-tune their existing ones to rise above market challenges and increase their share of the Global NoSQL Software market.
(4) The report also discusses competitive situation and trends and sheds light on company expansions and merger and acquisition taking place in the Global NoSQL Software market. Moreover, it brings to light the market concentration rate and market shares of top three and five players.
(5) Readers are provided with findings and conclusion of the research study provided in the Global NoSQL Software Market report.
Key Questions Answered in the Report:
(1) What are the growth opportunities for the new entrants in the Global NoSQL Software industry?
(2) Who are the leading players functioning in the Global NoSQL Software marketplace?
(3) What are the key strategies participants are likely to adopt to increase their share in the Global NoSQL Software industry?
(4) What is the competitive situation in the Global NoSQL Software market?
(5) What are the emerging trends that may influence the Global NoSQL Software market growth?
(6) Which product type segment will exhibit high CAGR in future?
(7) Which application segment will grab a handsome share in the Global NoSQL Software industry?
(8) Which region is lucrative for the manufacturers?
For More Information or Query or Customization Before Buying, Visit @ https://www.verifiedmarketresearch.com/product/nosql-software-market/
About Us: Verified Market Research®
Verified Market Research® is a leading Global Research and Consulting firm that has been providing advanced analytical research solutions, custom consulting and in-depth data analysis for 10+ years to individuals and companies alike that are looking for accurate, reliable and up to date research data and technical consulting. We offer insights into strategic and growth analyses, Data necessary to achieve corporate goals and help make critical revenue decisions.
Our research studies help our clients make superior data-driven decisions, understand market forecast, capitalize on future opportunities and optimize efficiency by working as their partner to deliver accurate and valuable information. The industries we cover span over a large spectrum including Technology, Chemicals, Manufacturing, Energy, Food and Beverages, Automotive, Robotics, Packaging, Construction, Mining & Gas. Etc.
We, at Verified Market Research, assist in understanding holistic market indicating factors and most current and future market trends. Our analysts, with their high expertise in data gathering and governance, utilize industry techniques to collate and examine data at all stages. They are trained to combine modern data collection techniques, superior research methodology, subject expertise and years of collective experience to produce informative and accurate research.
Having serviced over 5000+ clients, we have provided reliable market research services to more than 100 Global Fortune 500 companies such as Amazon, Dell, IBM, Shell, Exxon Mobil, General Electric, Siemens, Microsoft, Sony and Hitachi. We have co-consulted with some of the world’s leading consulting firms like McKinsey & Company, Boston Consulting Group, Bain and Company for custom research and consulting projects for businesses worldwide.
Contact us:
Mr. Edwyne Fernandes
Verified Market Research®
US: +1 (650)-781-4080
UK: +44 (753)-715-0008
APAC: +61 (488)-85-9400
US Toll-Free: +1 (800)-782-1768
Email: sales@verifiedmarketresearch.com
Website:- https://www.verifiedmarketresearch.com/
Article originally posted on mongodb google news. Visit mongodb google news
Global NoSQL Database Market Size and Forecast | Objectivity Inc, Neo Technology Inc …
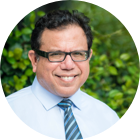
MMS • RSS
Posted on nosqlgooglealerts. Visit nosqlgooglealerts
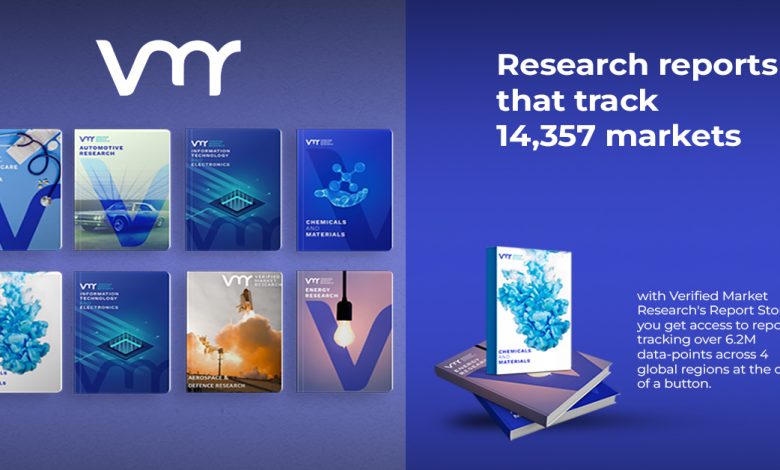
New Jersey, United States – The market research report offers an elaborate study of the Global NoSQL Database Market to help players prepare themselves well to tackle future growth challenges and ensure continued business expansion. With flawless analysis, in-depth research, and accurate forecasts, it provides easy-to-understand and reliable studies on the Global NoSQL Database market backed by statistics and calculations that have been finalized using a rigorous validation procedure. The report comes out as a comprehensive, all-embracing, and meticulously prepared resource that provides unique and deep information and data on the Global NoSQL Database market. The authors of the report have shed light on unexplored and significant market dynamics, including growth factors, restraints, trends, and opportunities.
The vendor landscape and competitive scenarios of the Global NoSQL Database market are broadly analyzed to help market players gain competitive advantage over their competitors. Readers are provided with detailed analysis of important competitive trends of the Global NoSQL Database market. Market players can use the analysis to prepare themselves for any future challenges well in advance. They will also be able to identify opportunities to attain a position of strength in the Global NoSQL Database market. Furthermore, the analysis will help them to effectively channelize their strategies, strengths, and resources to gain maximum advantage in the Global NoSQL Database market.
Get Full PDF Sample Copy of Report: (Including Full TOC, List of Tables & Figures, Chart) @ https://www.verifiedmarketresearch.com/download-sample/?rid=129411
Key Players Mentioned in the Global NoSQL Database Market Research Report:
Objectivity Inc, Neo Technology Inc, MongoDB Inc, MarkLogic Corporation, Google LLC, Couchbase Inc, Microsoft Corporation, DataStax Inc, Amazon Web Services Inc & Aerospike Inc.
Global NoSQL Database Market Segmentation:
NoSQL Database Market, By Type
• Graph Database
• Column Based Store
• Document Database
• Key-Value Store
NoSQL Database Market, By Application
• Web Apps
• Data Analytics
• Mobile Apps
• Metadata Store
• Cache Memory
• Others
NoSQL Database Market, By Industry Vertical
• Retail
• Gaming
• IT
• Others
The report comes out as an accurate and highly detailed resource for gaining significant insights into the growth of different product and application segments of the Global NoSQL Database market. Each segment covered in the report is exhaustively researched about on the basis of market share, growth potential, drivers, and other crucial factors. The segmental analysis provided in the report will help market players to know when and where to invest in the Global NoSQL Database market. Moreover, it will help them to identify key growth pockets of the Global NoSQL Database market.
The geographical analysis of the Global NoSQL Database market provided in the report is just the right tool that competitors can use to discover untapped sales and business expansion opportunities in different regions and countries. Each regional and country-wise Global NoSQL Database market considered for research and analysis has been thoroughly studied based on market share, future growth potential, CAGR, market size, and other important parameters. Every regional market has a different trend or not all regional markets are impacted by the same trend. Taking this into consideration, the analysts authoring the report have provided an exhaustive analysis of specific trends of each regional Global NoSQL Database market.
Inquire for a Discount on this Premium Report @ https://www.verifiedmarketresearch.com/ask-for-discount/?rid=129411
What to Expect in Our Report?
(1) A complete section of the Global NoSQL Database market report is dedicated for market dynamics, which include influence factors, market drivers, challenges, opportunities, and trends.
(2) Another broad section of the research study is reserved for regional analysis of the Global NoSQL Database market where important regions and countries are assessed for their growth potential, consumption, market share, and other vital factors indicating their market growth.
(3) Players can use the competitive analysis provided in the report to build new strategies or fine-tune their existing ones to rise above market challenges and increase their share of the Global NoSQL Database market.
(4) The report also discusses competitive situation and trends and sheds light on company expansions and merger and acquisition taking place in the Global NoSQL Database market. Moreover, it brings to light the market concentration rate and market shares of top three and five players.
(5) Readers are provided with findings and conclusion of the research study provided in the Global NoSQL Database Market report.
Key Questions Answered in the Report:
(1) What are the growth opportunities for the new entrants in the Global NoSQL Database industry?
(2) Who are the leading players functioning in the Global NoSQL Database marketplace?
(3) What are the key strategies participants are likely to adopt to increase their share in the Global NoSQL Database industry?
(4) What is the competitive situation in the Global NoSQL Database market?
(5) What are the emerging trends that may influence the Global NoSQL Database market growth?
(6) Which product type segment will exhibit high CAGR in future?
(7) Which application segment will grab a handsome share in the Global NoSQL Database industry?
(8) Which region is lucrative for the manufacturers?
For More Information or Query or Customization Before Buying, Visit @ https://www.verifiedmarketresearch.com/product/nosql-database-market/
About Us: Verified Market Research®
Verified Market Research® is a leading Global Research and Consulting firm that has been providing advanced analytical research solutions, custom consulting and in-depth data analysis for 10+ years to individuals and companies alike that are looking for accurate, reliable and up to date research data and technical consulting. We offer insights into strategic and growth analyses, Data necessary to achieve corporate goals and help make critical revenue decisions.
Our research studies help our clients make superior data-driven decisions, understand market forecast, capitalize on future opportunities and optimize efficiency by working as their partner to deliver accurate and valuable information. The industries we cover span over a large spectrum including Technology, Chemicals, Manufacturing, Energy, Food and Beverages, Automotive, Robotics, Packaging, Construction, Mining & Gas. Etc.
We, at Verified Market Research, assist in understanding holistic market indicating factors and most current and future market trends. Our analysts, with their high expertise in data gathering and governance, utilize industry techniques to collate and examine data at all stages. They are trained to combine modern data collection techniques, superior research methodology, subject expertise and years of collective experience to produce informative and accurate research.
Having serviced over 5000+ clients, we have provided reliable market research services to more than 100 Global Fortune 500 companies such as Amazon, Dell, IBM, Shell, Exxon Mobil, General Electric, Siemens, Microsoft, Sony and Hitachi. We have co-consulted with some of the world’s leading consulting firms like McKinsey & Company, Boston Consulting Group, Bain and Company for custom research and consulting projects for businesses worldwide.
Contact us:
Mr. Edwyne Fernandes
Verified Market Research®
US: +1 (650)-781-4080
UK: +44 (753)-715-0008
APAC: +61 (488)-85-9400
US Toll-Free: +1 (800)-782-1768
Email: sales@verifiedmarketresearch.com
Website:- https://www.verifiedmarketresearch.com/
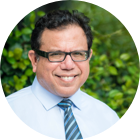
MMS • RSS
Posted on mongodb google news. Visit mongodb google news
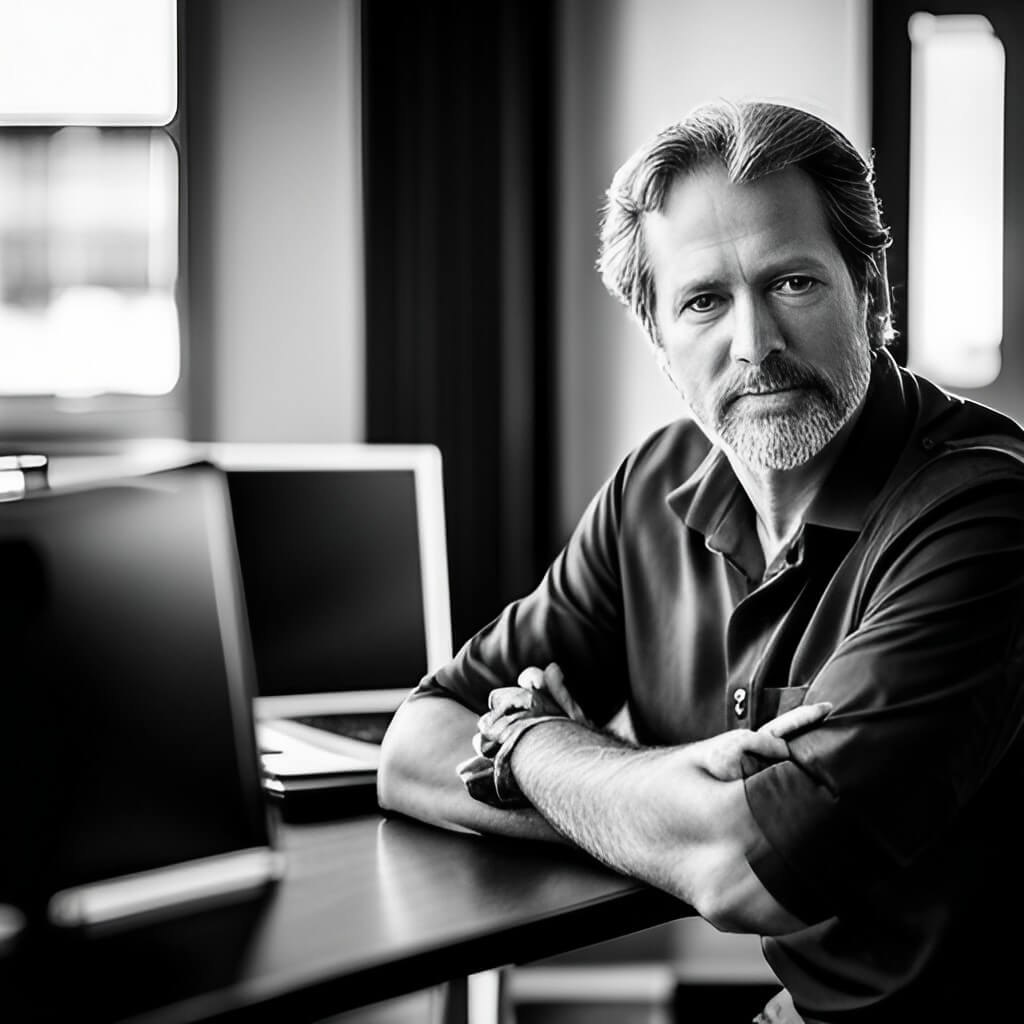
Description
Artificial intelligence (AI) is poised to transform the landscape of software development, empowering developers to write code with unprecedented speed, quality, and completeness. Mark Porter, the Chief Technologist of MongoDB, a leading document database maker, asserts that AI, particularly generative AI like OpenAI’s ChatGPT, will provide a significant advantage to developers, revolutionizing their productivity and … Read more
Artificial intelligence (AI) is poised to transform the landscape of software development, empowering developers to write code with unprecedented speed, quality, and completeness. Mark Porter, the Chief Technologist of MongoDB, a leading document database maker, asserts that AI, particularly generative AI like OpenAI’s ChatGPT, will provide a significant advantage to developers, revolutionizing their productivity and capabilities.
Empowering developers with enhanced code creation and testing
Generative AI is not about replacing developers; instead, it serves as a powerful tool to augment their skills. Mark Porter specifically mentioned that generative AI assists developers in multiple aspects of their work, such as code creation, test case generation, bug identification, and faster documentation lookup. This technology enables developers to produce code of superior quality, speed, and completeness—a long-standing aspiration in software development.
Porter believes AI will enable developers to write code at the desired level of quality, speed, and completeness that has long been aspired for in the industry. He anticipates developers can leverage generative AI and other robust models that have evolved over the past 15 to 20 years, resulting in transformative changes to how code is written.
AI integration in the developer ecosystem
AI represents an acceleration of the developer ecosystem, according to Porter. He believes that the adoption of AI will lead to an increased number of applications being developed, debunking the notion that it will render developers obsolete. The traditional perception of the time-consuming nature of software development and the challenge of getting it right will undergo significant changes because of generative AI’s immense potential.
Porter affirms,
Generative AI will change software development in significant ways, enabling developers to write applications at their desired speed, with exceptional quality. It revolutionizes the process, empowering developers to craft apps precisely as they envision them.
Mark Porter, MongoDB CTO
MongoDB’s AI capabilities and the impact on software development
During MongoDB.local, the company’s developer conference held recently in New York, a major focus was on showcasing the new AI capabilities of the MongoDB database. MongoDB serves as the foundation for numerous companies building AI solutions, reinforcing its position in the AI landscape.
Porter emphasizes the introduction of vector values as a native data type in MongoDB. By incorporating vectors, developers can store and retrieve context vectors produced by large language models. These vectors facilitate relevance searches and enable precise answers to queries, enhancing the user experience.
Porter explains the process: “I’m going to get a vector of that question, and then I’m going to put that vector into my database, and I’m then going to ask for vectors near it.” The database retrieves relevant articles based on the query, allowing developers to prompt their large language models with specific articles for answering questions.
Also, Porter highlights the capability of LLMs to summarize lengthy articles, showcasing the division of labor between AI and the database. The combination of AI and database technology streamlines operations and eliminates the need for separate systems and data copying.
Reshaping the future of software development
The role of AI in software development is set to revolutionize the industry, as affirmed by Mark Porter, MongoDB’s CTO. Far from replacing developers, AI will empower them with the tools and capabilities to write code faster, with greater quality and completeness. Generative AI, alongside other mature models, will transform the developer ecosystem and boost application development. MongoDB’s AI capabilities, including the integration of vector values, provide a glimpse into the future of software development. As AI continues to advance, developers will remain crucial decision-makers who listen to their customers and leaders, leveraging AI as a powerful tool to drive innovation and shape the future of software development.
Article originally posted on mongodb google news. Visit mongodb google news
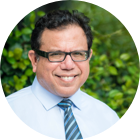
MMS • Almir Vuk
Article originally posted on InfoQ. Visit InfoQ
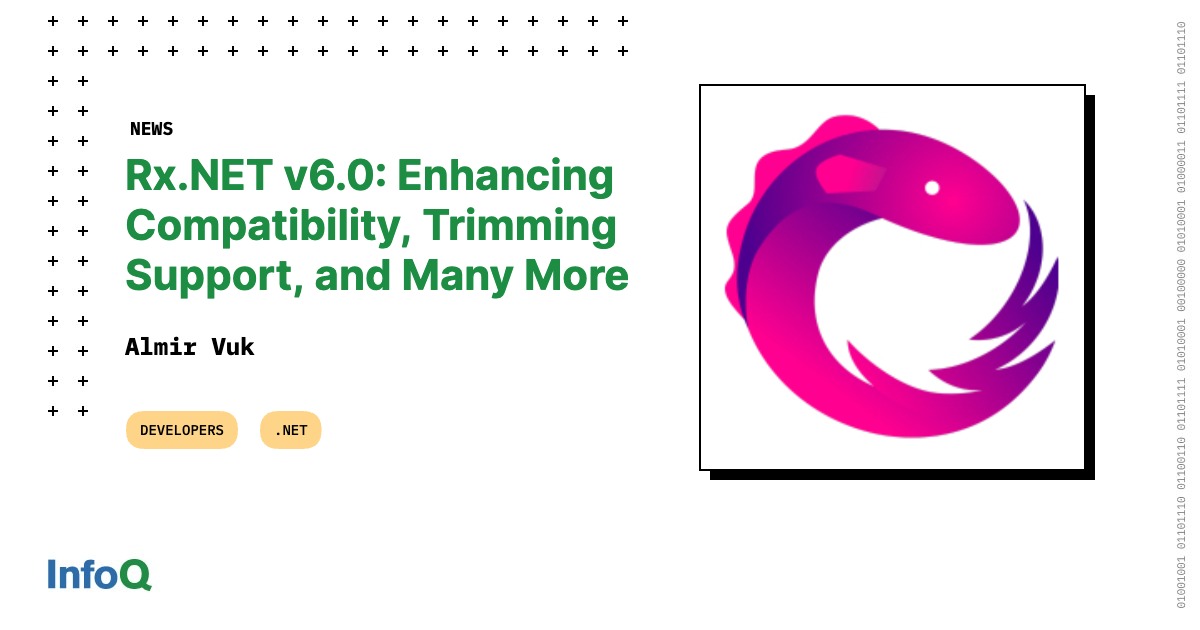
At the end of last month, the team behind Reactive Extensions for .NET announced the release of Rx.NET major version 6.0. The latest version of the library brings several improvements and aligns itself with the current .NET ecosystem. The original announcement blog post reveals that this update doesn’t introduce significant new functionality, it focuses on enhancing compatibility, supporting the latest versions of .NET, and addressing common pain points for developers.
The first notable change is that Rx.NET 6.0 now extends its support to the current versions of .NET, including .NET 6.0 and .NET 7.0. Previously, although Rx 5.0 was compatible with these versions, it lacked explicit targeting for them, which caused confusion among potential users. In response, the Rx team removed support for out-of-date frameworks and added the net6.0 target, aligning with Microsoft’s supported platforms.
Based on the report, it is important to take note that even though the System.Reactive NuGet package doesn’t include a net7.0 target, it fully supports .NET 7.0. The note that the absence of a specific target doesn’t imply a lack of support, as stated in the announcement blog post:
Any package that targets net6.0 will run on .NET 7.0. In fact it’s perfectly valid for a NuGet package to specify targets corresponding to out-of-support versions of .NET, because current .NET runtimes support packages that target older versions. For example, a package targeting netcore3.1 will run on .NET 7.0 even though .NET Core 3.1 went out of support some time ago.
To improve trimming capabilities, Rx.NET 6.0 introduces trimmability annotations in the net6.0 target. Trimming allows for reducing the size of the System.Reactive.dll component with results in smaller application sizes and improved startup performance. By leveraging these annotations, developers observed significant reductions in the size of the library, making trimming a valuable feature for certain deployment scenarios.
In terms of new features, Rx 6.0 incorporates enhancements to handle unhandled exceptions from wrapped Tasks. Applications can now opt to swallow these failures silently, which can be useful in specific scenarios where exceptions occur after the application code has unsubscribed. While swallowing exceptions is generally discouraged, the Rx library handles this situation to maintain compatibility and support edge cases where it can’t be avoided.
Additionally, the public availability of the SingleAssignmentDisposableValue class is another notable change. Previously this class was marked as internal, but this type is now accessible, bringing it in line with other disposable types in the library. Its inclusion provides increased flexibility to developers who require this functionality.
As reported, the Rx.NET team has invested significant effort in aligning the codebase with the current .NET tooling. This ensures that the library builds seamlessly on modern versions of Visual Studio and the .NET SDK. As part of these updates, the unit tests have been transitioned from XUnit to MSTest to address limitations with running tests on UWP. MSTest provides better support for UWP, a platform that Rx has been catering to for a long time.
Looking ahead, the Rx team has outlined their roadmap for version 7.0, primarily focusing on resolving code bloat issues in UI frameworks. The plan involves extracting UI-framework-specific functionality into separate packages to avoid unnecessary dependencies and reduce application size. By decoupling Windows Forms, WPF, and UWP code from the main System.Reactive component, Rx.NET will enable more efficient integration with various UI frameworks and reduce the overall footprint of applications.
Lastly, Rx.NET 6.0 represents a step forward in ensuring compatibility, improving trimming capabilities, and laying the groundwork for future enhancements. The team is actively addressing open issues and working on comprehensive documentation to aid developers in understanding and utilizing Rx effectively. With a commitment to supporting the latest .NET ecosystem and addressing user feedback. The future roadmap and project progress can be followed on the official GitHub repository.
Presentation: Ubiquitous Caching: A Journey of Building Efficient Distributed and In-Process Caches at Twitter
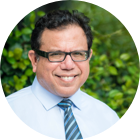
MMS • Juncheng Yang
Article originally posted on InfoQ. Visit InfoQ
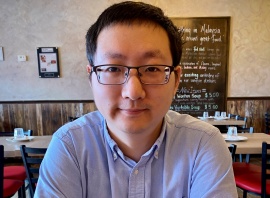
Transcript
Yang: My name is Juncheng Yang. I’m a fifth year PhD student at Carnegie Mellon University. I started working on caching since 2015. I’m going to talk about ubiquitous caching, a journey of building efficient distributed and in-process caches at Twitter. Today’s talk is going to have three parts. First, I’m going to talk about three trends in hardware, workload, and cache usage, and how they motivate the design of Segcache, a new cache store design that is more efficient and more performant. Second, I’m going to discuss the design philosophies and tradeoffs that we make in Segcache. Third, I’m going to describe how you reduce microservice tax by using small in-process caches, to build up our Segcache.
Caching Is Everywhere
Let’s start with the microservice architecture. Here I’m showing a typical architecture. You have an application. The application sends requests to an API gateway. The API gateway forwards requests to different microservices. These microservices are usually stateless. Serving some of the requests require fetching data from the backend. However, always reading from the storage backend can be slow, and it can be less scalable. A typical practice is to add a distributed cache in between the service and to the backend, so that reading data can be directly from the cache, much faster and scalable always. At first glance, it seems there’s only one cache in this diagram. That’s not true. To the right of the distributed cache, we have storage service, whether the storage is running the Linux or BSD they will have page cache, which reduce a significant amount of [inaudible 00:02:21]. To the left of the distributed cache, they’ve placed application services. These services even though they are stateless, they may also have cache, in-process caches, that stores a subset of most frequently used data, so that using this data to run across the network stack. Further to that, in API gateway, we’re increasingly seeing usage of cache to cache dynamic response, that’s just got QL response. Your API gateway may also have a cache embedded. Going out of your core data center, the edge data center, which is closer to the user, and the edge data center such as the CDN edge data center, serve static objects for you, such as image or videos, that’s out of big cache. We will look at this diagram. Almost each box or each part of that diagram have a cache, and the cache is almost everywhere.
What’s New in Caching?
The study and use of cache has a long history, dating back to ’60s and ’70s. Unlike other areas, such as AI and machine learning, or even databases, there hasn’t been huge news in caching. What’s new in caching these days? Maybe there’s some new eviction algorithms, or maybe there’s some new architecture, or maybe there’s some new features, or maybe there’s some new hardware we can deploy a cache on. If you look closely, it’s actually a mixture of all of that. All this excitement, all these advancement and improvements boil down to three things: performance, efficiency, and new use cases. First, performance. As a cache user, cache designer, and a cache operator, we all want better performance. There are works to reduce the latency of cache is much tail latency, and increase the throughput and improve the scalability of the cache design. Besides a common case of performance, tail performance and the predictable performance, especially predictable tail performance is very important if we run cache at large scale. There are some works to improve the logging, the monitoring, and the observability towards more caching, so that when your cache goes wrong, you will know it. It will be easier for you to diagnose and find out what goes wrong.
The second is efficiency. There are quite some works like product stuff to actually improve the efficiency or the cost effectiveness of caching. For example, Meta open sourced CacheLib last year. A CacheLib enables you to cache both small objects and large objects on flash. At Twitter, we open sourced Segcache last year too. Segcache is a better storage design that allows you to cache more data in your cache. Besides this, there are also some staff who work on workload modeling. The workload modeling allows cache operators to do better capacity planning and resource provisioning. Besides performance and efficiency, we are increasingly seeing new cache use cases, mostly in caching dynamic data. For example, we have some works on products to do cache API responses, while some others trying to cache dynamic data structures.
At Twitter, we created Pelikan. Pelikan is an open source caching framework that will replace Memcached and Redis. Pelikan adopts a modular design with clear boundary between each module of components. Pelikan was written in C a few years ago. In the last two years, they have migrated Pelikan from C to Rust. By we here, I really mean we. I personally only contributed a very limited amount of code to the code base. The project is led by Yao and Brian, who are the core developers of Pelikan that’s contributed to majority of the code base and has led the transition from C to Rust.
Hardware Trend
I’m going to focus on the new datastore on storage component, which we introduced two years ago, which is called Segcache. The design of Segcache was motivated by three trends: hardware, workload, and cache usage. First, let’s talk about hardware trend. We observed that the memory capacity scaling had slowed down, but the computing has not. This figure comes from Jim Handy, the memory guy expert being semiconductor memories. It shows the DRAM price per gigabyte in the past 30 years. We observe that for the first 20 years in this figure, we see that the DRAM price dropped significantly, which is almost exponential. Notice log scale on y axis. In the past decade, from around 2011, the DRAM price per gigabyte remains almost constant, of course with some fluctuations. In contrast, the computing power has been constantly increasing. This figure shows the number of CPU cores in a high-end storage CPU from 2007 to 2014. We see that from 2007 to 2014 the number of cores increased by 8 times, which doubles almost every 2 years. From 2014 up to 2022, AMD released CPUs with over 100 cores, which if you consider hyper-threads as 1 core, which is more than 200, another 8 times increase since 2014.
Also, there has been some debate on whether Moore’s Law is dead or not. Whether it’s dead or not, will not stop that computing power continues to increase. Especially consider the use and wide deployment of accelerators. We believe that the computing power will continue to increase for the foreseeable future. The memory’s capacity stopped scaling, and the computing power continued to increase, makes the memory capacity become more important. Making better use of your limited memory capacity or limited cache size becomes increasingly important, especially in an economy environment where cutting costs have become the new law. According to Wanclouds, close to 40% of the companies do not want to spend more money on cloud computing on the infrastructure cost. While more than 40% of the companies want to cut the cost either dramatically, or marginally on cloud computing, and only less than 20% of companies are willing to spend more money on cloud computing. Then comes the first takeaway, making a good use of limited cache space becomes increasingly important. That’s the first trend, the hardware trend.
Workload Trend
The second trend is workload trend. We are in a digital era. Many of our social activities have moved online, especially after the pandemic. As a result of this, we’re seeing exploding data creation rate. For example, according to IDC, the volume of data created and replicated worldwide increased exponentially. For example, compared to 9 years ago, 2013, in 2022, the volume of data is almost 10 times higher. If we further compared to 2025, just in 3 years, the amount of data created worldwide will almost double again. What does this mean for us as a cache researcher and storage engineers? It means that when you think about the capacity needed for this data, and we consider how to serve this data, maybe separate the cold data from the hot data, and what else. If we look closer at this hot data and how they’re accessed over time, we observe that popularity decay has become the new trend.
In this slide, I’m comparing the relative popularity of two workloads, one collected in 2007, and one collected in 2021. The y axis shows the relative popularity measuring popularity of getting a request in a 5-minute window, while x shows time. The figure is created by averaging medians of objects. We can see that the relative popularity of objects created in 2007 does not change much after the data being created. While for our workload from 2021, objects show significant popularity decay over its lifetime. For example, compared to 5 minutes old, the data around 1 day old have 10 times less request popularity, or 10 times less popularity. Of course, we see spikes of some time points like 1 day, 2 day, 3 day, and the 4 days, the congestion is caused by a recommendation system rewiring the data. What’s the takeaway for this popularity decay? It means that objects become less malleable over time, and LRU may not be the best eviction algorithm anymore, especially if we consider the scalability problem it introduced and its property of being sized [inaudible 00:14:44]. This makes LRU much less attractive compared to other more fancier algorithms.
The second workload trend is object size. Technically speaking, it’s not really a trend but it was a property of key-value cache, or communication workload. We observe that object size are often small. For example, the five largest cache clusters at Twitter have mean object size between 50 bytes and 200 bytes. Across all cache clusters, which is more than 100, the 25 percentile mean object size is 100 bytes, and the median is 300 bytes. What problem does small object brings? The problem is metadata overhead. For example, Memcached uses 56 bytes metadata per object. If you have an object like workload with object size around 100 bytes, so what set of your cache size will be span metadata. If you examine your cache usage, you will see a universe of metadata, and we can call this Metaverse. Besides the object size being small, object size are also non-uniform. This is in contrast to the page cache and CPU cache where the granularity of data being cached, it’s usually big size. In key-value cache, object size is non-uniform. Every one of us knows about it.
What’s the problem of being non-uniform? The problem is fragmentation. If you have ever run large scale cache clusters, especially Linux clusters, we had run into memory usage I wasn’t expecting, or even out-of-memory errors. This is most likely true because of fragmentation. Fragmentation itself is an important problem. Because we always run cache in full capacity, so we never run in 50% capacity, and you shouldn’t run at 50% capacity, because it’s cache. We run the cache at 100% capacity, creating a new object plus evicting an object. Putting this in terms of allocation, or memory allocation, a cache constantly allocates and deallocates objects. The design of memory allocator have a big impact on the efficiency and performance of the cache. In fact, we are seeing some staff working to improve the performance of memory allocator. The third takeaway from the trend is that memory allocation and memory management is critical for cache design.
Usage Trend
The last trend is usage trend. We’re seeing increasing use of time-to-live in professional code. Time-to-live stands for TTL. This is set when the object is written into the cache, and when an object expires it cannot be used. Why do we use TTL? There are a couple of reasons. First, because a distributed cache has different ways to have stale data, or inconsistent data, which is bad. TTL is introduced as an approach to guarantee that stale data do not stay for a long time. Second, TTL is used for periodic refresh. For example, some machine learning prediction service may cache the compute itself in the cache for a few seconds or a few minutes. It does not need to recompute certain data, and save some computation. Third, TTL can be used for implicit deletion. For example, where you implement rate limiters, you can use TTL for time window. Or if your company enforces GDPR regulation, you may have to use TTL to guarantee that the data of this user will not stay for more than a certain amount of time in your cache. Last, we see some engineers use TTLs to signal objects’ lifetime, especially when the objects have a short lifetime. In production, we observe some short TTLs are widely used, and this figure shows the distribution of the smallest TTL given the cache cluster service. We observe that for more than 30% of the cache cluster service at Twitter, the smallest TTL is less than 5 minutes, or for more than 70% of the cache clusters, the smallest TTL is less than 6 hours. It’s pretty small compared to the data retention time in your backend key-value store.
What does this imply? We measure the working set size of the same workload when we consider TTL, and when we don’t consider TTL. We see that if we don’t consider TTL, the working set size constantly grow. While if we consider TTL, and if the cache can remove expired objects in time, then the working set size is bounded, and we do not need a huge cache size for this workload. Of course, this is assuming the expired objects can be removed entirely, but it’s just not the reality in most cases. When we compare expiration and eviction, expiration removes objects that cannot be used in the future, and eviction removes objects that may potentially be used in the future. In the past, I saw some confusion and misconceptions around expiration and eviction. Some people think that expiration is a way for eviction, or eviction is one type of expiration. I don’t think this is true. Expiration and eviction are two different concepts. Expiration are talking about user, while eviction is an internal operation to the cache that’s used to make room to run new objects. Don’t mix expiration and eviction. The first takeaway of the trend is that removing expired objects is actually more important than eviction, because expired objects are using it.
Let’s look at the existing approach, a trend that’s being used in production systems to remove expired objects. We see that existing approaches are good, but they’re not sufficient, or efficient. For example, modern approach uses the Memcached scanning, which scan the cache periodically to remove expired objects. This is sufficient because it can remove all the expired objects if you scan parsing out. However, it’s not efficient because it consumes a lot of CPU cycles, and memory penalty. Similarly, the approach used in Redis are sampling, which is both not efficient and not sufficient, because it cannot remove all the expired objects without sampling from the cluster. Random memory access is also very expensive in terms of computation. How do we find and remove expired objects?
Segcache – Segment-Structured Cache
In the second part, I want to talk about Segcache. Segcache was motivated by the trends I just talked about, and by the problems I just mentioned. Segcache stands for segment-structured cache. It is a highly efficient and high-performance cache storage design that has the following features. First, it supports efficient TTL expiration, meaning that once an object expires, it will be removed from the cache within a few seconds. Second, it has a tiny object metadata, which is only 5 bytes. This is 90% reduction compared to Memcached. Third, Segcache uses an efficient segment-based memory allocator which has almost no memory fragmentation. Third, Segcache uses a popularity-based eviction, which allows Segcache to perform fewer bookkeeping operations while remaining efficient. As a result of this, Segcache use less memory, but it gives a lower miss ratio. It uses fewer cores, but it provides you a higher throughput. Segcache can be deployed both as a distributed cache and as an embedded cache. We open sourced Segcache in the following URLs, https:/open-source.segcache.com, https:/paper.segcache.com. The first URL links to the open source code. The second URL links to a paper which, in detail, describes the design of Segcache.
Segcache Design
I do want to briefly describe the high-level architecture of Segcache. Segcache has three components. The first one is called object store. Object store is where objects are stored. We divide object store into segments, where each segment is a small log, storing objects of similar TTL. This is somewhat similar to the slab storage in Memcached. Slab storage stores objects of similar size, whereas the segment stores objects of similar TTLs, but can be of any size. The second component of Segcache is called TTL buckets. We break all possible TTL [inaudible 00:26:09] into ranges and assign each range to one TTL bucket. The first TTL bucket links out to a segment chain. When we write objects into segments, it is append only. The segment chain is from altering an append only way. All objects in the segment chain are sorted by creation time. Because objects in the same segment chain have similar TTLs, they’re also sorted by expiration time. This allows Segcache to quickly discover and remove expired objects. The third component of Segcache is the hash table. Hash table is common in many cache designs. In contrast to the object chaining hash table in Memcached, Segcache use a packet chaining hash table, where objects are first going to hash buckets, then we perform chaining. When we write an object into Segcache, it first finds the overhead TLL buckets, so we find the last segment in this segment chain, then we append to that segment. When it’s reading an object, we first look up in the hash table, it finds a pointer to the segment and we read the data from the segment. That’s how read and write works in Segcache.
Key Insight
The design of Segcache is driven by one key insight, that key-value cache is not the same as key-value store plus eviction. Why? A couple of reasons. First, key-value cache and key-value store have different design requirements. When we design a key-value cache, the top priority is performance and efficiency. You want your cache to be as fast as possible, and you want your cache to store as many objects as possible. While if you design a key-value store, you want durability, you want consistency. You don’t want data loss and you don’t want data corruption. You may [inaudible 00:28:31] key-value store. This different design requirement makes key-value cache and key-value store to have different designs and different focus. In addition to design requirements, key-value cache and key-value store also have these different workloads. As we show in the microservice architecture, key-value cache usually sits in front of the key-value store, meaning that all the traffic taken by the key-value store will be filtered first by the key-value cache. The key-value cache usually sees more skewed workloads. Key-value cache workloads usually use shorter TTLs. Because of this difference, key-value cache and key-value store are completely different things. Next time when someone asks you what’s the difference between Memcached and RocksDB, not only the storage media is different, it’s not only the indexing structure is different, the core difference is one being a key-value cache, the other one being a key-value store.
Segcache Design Philosophies
I want to quote Thomas Sowell, a famous economist. Once he said that, there are no solutions, there are only tradeoffs. This also applies to computer system design. In Segcache design, we try to make the right tradeoff for caching. We have three design philosophies. First, we want to maximize metadata sharing via approximation. You notice that many metadata in cache designs does not need to be fully precise, in other words, they can be approximate. For example, timestamps, TTLs, they do not need to be fully accurate. Our design choice is to share metadata between objects in the same segment, and in the same hash bucket. The tradeoff here is the metadata precision versus space saving. We believe that space saving is more important for cache. As a result, let’s see what it looks like. This a Memcached object store. We have a huge object metadata that is the data. Here is Segcache object store. We still have the object metadata, but it’s much smaller, and we lift most of the metadata to the segment level. Such metadata includes all timestamps and your fence counters and some of the pointers. Besides sharing in the service segment, we also have sharing between objects in the same hash bucket. This type of sharing allows Segcache to have tiny object metadata.
The second design philosophy of Segcache is be proactive, do not be lazy. Segcache wants to remove expired objects, timely and efficient. It uses approximate TTL bucketing and indexing to discover expired objects and remove them. The tradeoff here is that because the TTL is approximate, Segcache may remove or may expire some objects slightly earlier than what the user specifies. This is an example for cache, because the alternative of removing objects slightly earlier is evicting an object. The object that’s being evicted may still have future use, but the object that’s being expired slightly earlier, it’s highly likely it won’t have future use before it expires. Expiring objects slightly earlier is often a better choice than evicting the object. That’s why we made this design decision.
The third design philosophy in Segcache is macro management. Segcache manages segments instead of objects. The insight here is that many objects in a cache are short-lived and do not need to be promoted. If you use LRU, you will have object LRU chain and a free chunk chain such as in Memcached. Each operation needs some bookkeeping operations, and also needs logging. This significantly limits the scalability of Memcached. By managing segments, Segcache performs expiration and eviction on the segment level, this will reduce a lot of the unnecessary bookkeeping operations, and also reduce the locking frequency by thousands to ten thousand times because each segment has a thousand to ten thousand objects. The tradeoff here is that it may slightly increase the miss ratio in some rare workloads where they do not exhibit popularity decay. The benefit we get here is we obtain a higher throughput and a better scalability. Meanwhile, we reduce miss ratios in the common cases where workload do exhibit popularity decay. That’s the tradeoff we made here. Those are the three design philosophies.
Evaluation Setup
Let’s look at some results. We evaluate Segcache together with Twemcache, Memcached, and Hyperbolic. Hyperbolic is a cache design from Princeton. We were using 5-week long production traces when we performed all evaluations of Twitter production fleet. This evaluation only evaluates the storage, so it does not involve the RPC stack. In the first evaluation, we want to understand how much memory is needed to achieve production miss ratios. We use Twemcache as the baseline, which is 100%. This result is the lower the better. Compared to Twemcache, both Memcached and Hyperbolic reduce the footprint to some extent. However, if we look at Segcache, it further reduces the memory footprint that’s needed to achieve production miss ratio compared to both Memcached and Hyperbolic. Specifically, compared to Twemcache, it can reduce the memory footprint by 40% to 90%. The largest cache cluster at Twitter, which is the [inaudible 00:35:49] cache cluster, it can reduce memory footprint by 60%. Compared to state of art, which is the best of all these systems, Segcache can reduce memory footprint by 22% to 60%. That’s a huge saving, especially for the monitoring workload, which have many different TTLs that’s used and different types of objects with different lifetime. This is where Segcache shines the most.
Next, we evaluate the single-threaded throughput performance of different systems. First, I’m showing Twemcache, which has pretty high throughput. Next, I show Memcached and Hyperbolic. Compared to Twemcache, these two are slightly slower or sometimes significantly slower. This is because both Memcached and Hyperbolic need to perform a lot of bookkeeping and expensive operations doing each eviction or doing each request, even Get request need to perform some operations, while Twemcache perform almost no operation upon each category. This is why Twemcache is faster. However, compared to Segcache, Segcache and Twemcache have similar models in terms of throughput. When we compare Segcache to other systems, such as Memcached and Hyperbolic, we observed that Segcache is significantly faster with up to 40% higher throughput compared to Memcached. That’s single-threaded throughput.
Let’s look at multi-threaded throughput. We compare Segcache with Memcached at different number of threads. We observe that Memcached stopped scaling at around 8 threads, while Segcache continued to scale up to 24 threads. At 24 threads, Segcache provides 8 times higher throughput compared to Memcached. Notice that this result is slightly different from the result we can see online [inaudible 00:38:12], this is because production traffic shows more locality. It causes more contention compared to synthetic workloads such as YCSB, or different workload. That’s Segcache and how Segcache performs compared to other systems.
JSegcache – Microservice Tax
In the last part, I’m going to talk about JSegcache, which is Segcache for JVM. Earlier, I showed this slide of microservice architecture and I said that cache is everywhere. We just talk about the big orange box, the distributed cache. In this part, I want to talk about the in-process cache, which sits inside each service. Why do we use in-process cache? There are a couple of reasons. The biggest reason why we use in-process cache is we want to reduce microservice tax. Microservice architecture does not come for free. You need to pay tax for it. Here the tax means, first, long data access latency. Because we’ve separated out the state from the stateless service, each time when we need to access the data, we need to use RPC cost. Compared to DRAM access, RPC cost is thousands of times slower. Second, using a microservice architecture requires a lot of RPC calls, and these RPC calls consume a lot of CPU cycles. According to a report from Meta, up to 82% of their CPU cycles are spent on microservice tax on data orchestration. Here data orchestration includes I/O processing, encryption and decryption, serialization and deserialization, compression and decompression. It’s a lot of CPU cycles being consumed by the microservice tax. By caching a small subset of data in-process, we can reduce the amount of RPC calls we need to make. Therefore, you can significantly reduce the CPU cycles. Last, because usually we shard our stateful service, we shard this storage servers into different sizes. When the size is small, hotspot, load imbalance have happened very frequently. It can cause long tail latency or even timeout and cascading failures. By adding a small in-process cache, it can reduce the microservice tax because it can reduce the data access latency, it can reduce the CPU cycles used on RPC communication, and it also can provide provable load balancing on the backend. Previously, researchers have already shown that by caching NlogN objects in your frontend, can guarantee that there’s going to be no hotspot in your backend. Here, N is the number of backend servers. N is not the number of objects. N is the number of backend servers. If you’re interested in this, here are two papers, you can take a look.
Caching in JVM
Caching in-process can reduce microservice tax. However, Twitter is a JVM shop and JVM caching is expensive because of two reasons. First, caching in JVM incurs huge garbage collection overhead. Many garbage collectors or garbage collection episodes are generational GC, meaning that they assume that objects either have a short or long lifetime. However, caching in JVM breaks this assumption, because objects in a cache have median lifetime to do eviction, expiration, and refresh. Therefore, caching in JVM consumes a lot of CPU cycles and leads to long pause time. Second, caching in JVM is expensive because it has huge metadata. For example, [inaudible 00:42:59] int uses 4 bytes, while an integer object uses 16 bytes in JVM. An empty string uses 40 bytes in JVM. That’s huge. If you’re familiar with JVM caching, you’re probably familiar with Guava and Caffeine. Caffeine is a popular cache library for JVM or Java. A caffeine cache entry uses 88 bytes, or 152 bytes for each cache entry. Many cache workloads have mean object size from tens of bytes to hundreds of bytes. Such huge metadata will cause you to spend a lot of space on storing the metadata. That’s why it’s expensive.
Off-Heap Caching for JVM
We’ve designed and built JSegcache, which is off-heap caching for JVM services. JSegcache is a generalized wrapper around Segcache. It allows caching in JVM based microservices, with shorter GC pause with smaller metadata. It also allows us to have larger caches because the cache is no longer on a JVM heap, and because Twitter uses 32-bit JVM, which limits the heap size to 72 gigabytes. By moving the cache off heap, now the cache size is no longer bounded by the heap size. It looks like it’s an amazing idea, but why haven’t people used it in the past? There are a couple of reasons. First is native code is less portable. Java code is very portable, and you run it once and you run it everywhere. This is not a big problem for data center applications, because in data centers, the architecture is mostly homogeneous, especially at Twitter.
Second is serialization overhead. Because JVM memory layout is different from native memory layout. To cache a Java object, you need to perform serialization, and you find a common language that can be spoken by both the JVM and native language. In this case, it’s the binary. Caching off-heap requires serialization, and this adds CPU overhead. However, we see that this still boils down to a tradeoff that we need to make, a tradeoff between GC and serialization overhead. With off-heap caching, we need to pay serialization overhead for each request, while with on-heap caching, we need to pay the GC overhead, which is less predictable, which can cause long tail latency, and which can also consume more CPU cycles. Which one is better? It depends on your use case and usage scenario. In our case, we find that paying the serialization overhead is actually better than paying the GC overhead. That’s why we need to bring Segcache to JVM based microservice using JNI.
Evaluation: JSegcache Microbenchmark
Now let’s look at some results. First, we evaluate JSegcache and we compare with Guava and Caffeine, two popular JVM cache libraries. We replay a production workflow. The first figure shows the throughput at different concurrency. We observe that compared to Guava and caffeine, JSegcache has higher throughput compared to Guava, but lower throughput compared to Caffeine. This is because JSegcache needs to pay the serialization overhead. Second, we show the number of cores being used in the benchmark. Because Guava and Caffeine needs to pay the GC overhead which consumes a lot of CPU cycles, they consume significantly more CPU cycles compared to JSegcache, especially when the concurrency is low, or the load is low, such as at 1 or 2. Let’s see the DRAM requirement to run this microbenchmark. Compared to Guava and Caffeine, which requires GC to collect garbage, JSegcache does not require GC to collect garbage and manages object lifetime within Segcache. It has significantly lower DRAM requirements.
Staging Experiment 1: Double Cache Size
Moving from microbenchmark to production. I’m going to show you two staging experiments. The first shows the case where we have low DRAM, we want to use more hardware resources if we want to get better performance. This figure shows by doubling the cache size, JSegcache can further reduce miss ratio by 15%. It enables faster data access. JSegcache does not change GC frequency, it reduces the GC pause time by around 50%. You start off cheaper GC, the CPU utilization actually goes down, even though we pay serialization overhead for each request.
Staging Experiment 2: Shrink Heap Size by 30%
While the first staging experiment shows the scenario where we want to spend more hardware resources. The second staging experiment shows the scenario where we want to cut cost in order to shrink the DRAM usage. We shrink heap size by 30%. We observe that JSegcache achieves a similar miss ratio by using a smaller heap and it has a lower GC pause. In conclusion, JSegcache enables better performance and lower cost compared to off-heap cache.
Takeaways
I talked about the three trends that motivates the design of Segcache: the hardware trend, the workload trend, and the cache usage trend. I’m going to talk about the three design philosophies and tradeoffs in Segcache: maximize sharing, be proactive, and macro management. Then I talked about how we reduce microservice tax by adding a small in-process cache in our JVM based microservices. There are two other important messages I want you to walk away with. First, expiration and eviction are different. Some people mix this together, and think expiration is a way of eviction, or eviction is a way of expiration. This is not true. Expiration is triggered by a user but eviction is an internal operation through the cache. We shouldn’t mix them together. These are two different things. Second, key-value cache is not a key-value store plus eviction. Key-value cache and key-value store have completely different design requirements, even though they share the same interface. Next time, if someone says Memcached is a key-value store in DRAM, it’s correct then because Memcached never offers you an option to disable eviction, it’s never meant to be a key-value store. Rather, it’s a key-value cache. Last, Segcache is open source and JSegcache will be open sourced.
See more presentations with transcripts
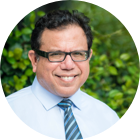
MMS • Ben Linders
Article originally posted on InfoQ. Visit InfoQ
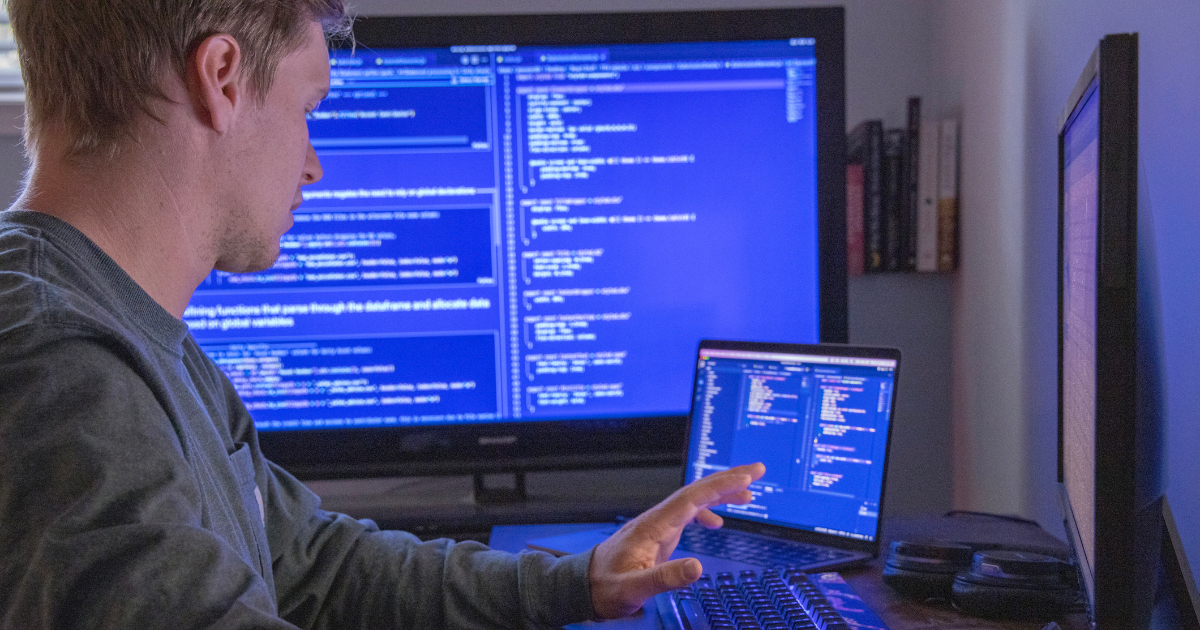
Remote mob programming helped a team in a high-stakes environment to be resilient, work under pressure, and deliver successfully. Setting expectations on the first call and being serious about the reasons for doing mob programming ensured that the team kept doing it.
Giovanni Asproni gave a talk about remote mob programming at XP 2023.
Asproni mentioned that they had some strong constraints in terms of quality, security, and accessibility and, at the same time, very high visibility—especially for failure, which would end up in the news. They also needed resilience, therefore they couldn’t afford to depend on any specific individuals. So they figured out that mob programming was the way to go, despite being in a high-stakes environment, Asproni said.
As the designated tech lead, Asproni had set his expectations on the first call with the rest of the team:
We needed high quality, full automation, four-eyes principle, collective ownership, collective accountability, and teamwork—job grades were irrelevant (mine included), what counted was a contribution towards reaching the goal
They had very little time, about half of their best estimate, Asproni mentioned, and the release date was announced to the public.
Being serious about the reasons for doing mob programming and about the expectations they set for their work was important to assure that the team kept doing mob programming, despite being under pressure, Asproni mentioned.
The urgency of a situation can take a big toll on well-being. Mob programming worked out well for them, Asproni said, it enabled them to work effectively, without cutting any corners, and keeping quality high, for long hours under pressure.
InfoQ interviewed Giovanni Asproni about his experiences with mob programming in a high-stakes environment.
InfoQ: How did you prepare and set things up for remote mob programming?
Giovanni Asproni: I made sure the team had the authority and the autonomy they needed to make decisions without me for a couple of reasons: I had to attend many meetings each day and I didn’t want to become a bottleneck; and they had better knowledge than me in many respects.
The main challenge was for me to make sure I walked the talk–by giving space to the team and trusting them to do the right thing, and avoiding any inadvertent mistakes that would have invalidated the expectations I set with them. I attended the mobbing sessions as often as I could, but not as often as I wished, as I was generally busy with calls with all other stakeholders.
InfoQ: What made the team continue to do mob programming, despite being under pressure?
Asproni: Most people say all the right things, then, when the pressure mounts, panic and do something else—cut corners, forget about automation, “parallelize” work by giving different tasks to different people, etc.
In our case we were convinced we were doing the right thing, so we kept doing it. The fact that we were distributed helped, since nobody outside the team could see how we were working, so had no reasons to panic.
InfoQ: What’s your advice to teams that are considering doing remote mob programming?
Asproni: Go for it. It will be intense, especially at the beginning, so take breaks. Don’t worry if your team does it differently from others, each team has to find its way. The speed, quality, and job satisfaction you will achieve will surprise you.