Month: June 2023
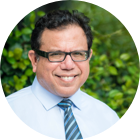
MMS • RSS
Posted on mongodb google news. Visit mongodb google news
Capital One Financial began coverage on shares of MongoDB (NASDAQ:MDB – Get Rating) in a research report released on Monday, The Fly reports. The firm issued an equal weight rating and a $396.00 price objective on the stock.
Several other brokerages have also recently weighed in on MDB. Piper Sandler upped their price target on MongoDB from $270.00 to $400.00 in a research report on Friday, June 2nd. Guggenheim downgraded MongoDB from a neutral rating to a sell rating and upped their target price for the company from $205.00 to $210.00 in a report on Thursday, May 25th. They noted that the move was a valuation call. Barclays increased their price objective on MongoDB from $280.00 to $374.00 in a report on Friday, June 2nd. Royal Bank of Canada increased their price objective on MongoDB from $400.00 to $445.00 in a research report on Friday. Finally, JMP Securities raised their price objective on MongoDB from $245.00 to $370.00 in a research note on Friday, June 2nd. One research analyst has rated the stock with a sell rating, three have assigned a hold rating and twenty-one have issued a buy rating to the company. According to MarketBeat.com, the company presently has a consensus rating of Moderate Buy and a consensus target price of $353.75.
MongoDB Trading Down 2.6 %
Shares of MDB stock opened at $379.98 on Monday. The stock has a market cap of $26.61 billion, a price-to-earnings ratio of -81.37 and a beta of 1.04. The stock has a 50-day moving average of $298.74 and a 200-day moving average of $240.54. The company has a current ratio of 4.19, a quick ratio of 4.19 and a debt-to-equity ratio of 1.44. MongoDB has a 1-year low of $135.15 and a 1-year high of $398.89.
MongoDB (NASDAQ:MDB – Get Rating) last announced its quarterly earnings results on Thursday, June 1st. The company reported $0.56 earnings per share (EPS) for the quarter, topping the consensus estimate of $0.18 by $0.38. MongoDB had a negative return on equity of 43.25% and a negative net margin of 23.58%. The business had revenue of $368.28 million for the quarter, compared to analyst estimates of $347.77 million. During the same quarter in the prior year, the company earned ($1.15) earnings per share. The company’s quarterly revenue was up 29.0% on a year-over-year basis. On average, equities research analysts forecast that MongoDB will post -2.85 earnings per share for the current year.
Insider Buying and Selling at MongoDB
In other MongoDB news, CAO Thomas Bull sold 605 shares of the company’s stock in a transaction on Monday, April 3rd. The stock was sold at an average price of $228.34, for a total value of $138,145.70. Following the completion of the sale, the chief accounting officer now directly owns 17,706 shares in the company, valued at approximately $4,042,988.04. The sale was disclosed in a document filed with the Securities & Exchange Commission, which is available through this link. In other MongoDB news, CAO Thomas Bull sold 605 shares of the stock in a transaction dated Monday, April 3rd. The stock was sold at an average price of $228.34, for a total value of $138,145.70. Following the completion of the sale, the chief accounting officer now directly owns 17,706 shares in the company, valued at $4,042,988.04. The transaction was disclosed in a document filed with the SEC, which is available at this link. Also, CTO Mark Porter sold 1,900 shares of the stock in a transaction dated Monday, April 3rd. The shares were sold at an average price of $226.17, for a total value of $429,723.00. Following the completion of the transaction, the chief technology officer now directly owns 43,009 shares in the company, valued at $9,727,345.53. The disclosure for this sale can be found here. Insiders have sold 108,856 shares of company stock valued at $27,327,511 in the last quarter. 4.80% of the stock is owned by insiders.
Institutional Trading of MongoDB
Institutional investors have recently bought and sold shares of the business. Bessemer Group Inc. purchased a new position in MongoDB during the fourth quarter worth approximately $29,000. BI Asset Management Fondsmaeglerselskab A S purchased a new stake in MongoDB in the fourth quarter valued at $30,000. Global Retirement Partners LLC raised its position in MongoDB by 346.7% in the first quarter. Global Retirement Partners LLC now owns 134 shares of the company’s stock valued at $30,000 after purchasing an additional 104 shares during the period. Lindbrook Capital LLC raised its holdings in shares of MongoDB by 350.0% in the 4th quarter. Lindbrook Capital LLC now owns 171 shares of the company’s stock valued at $34,000 after buying an additional 133 shares during the period. Finally, Y.D. More Investments Ltd purchased a new stake in shares of MongoDB in the 4th quarter valued at $36,000. 89.22% of the stock is owned by institutional investors and hedge funds.
About MongoDB
MongoDB, Inc provides general purpose database platform worldwide. The company offers MongoDB Atlas, a hosted multi-cloud database-as-a-service solution; MongoDB Enterprise Advanced, a commercial database server for enterprise customers to run in the cloud, on-premise, or in a hybrid environment; and Community Server, a free-to-download version of its database, which includes the functionality that developers need to get started with MongoDB.
Featured Articles
Receive News & Ratings for MongoDB Daily – Enter your email address below to receive a concise daily summary of the latest news and analysts’ ratings for MongoDB and related companies with MarketBeat.com’s FREE daily email newsletter.
Article originally posted on mongodb google news. Visit mongodb google news
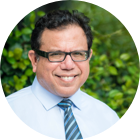
MMS • RSS
Posted on mongodb google news. Visit mongodb google news
MongoDB (NASDAQ:MDB – Get Rating) had its price target lifted by Barclays from $374.00 to $421.00 in a research report released on Monday, The Fly reports.
A number of other equities analysts also recently weighed in on the company. KeyCorp boosted their price target on MongoDB from $229.00 to $264.00 and gave the stock an overweight rating in a research report on Thursday, April 20th. Morgan Stanley increased their price target on MongoDB from $270.00 to $440.00 in a research note on Friday. William Blair restated an outperform rating on shares of MongoDB in a research report on Friday, June 2nd. Credit Suisse Group dropped their price objective on shares of MongoDB from $305.00 to $250.00 and set an outperform rating for the company in a research note on Friday, March 10th. Finally, Royal Bank of Canada increased their target price on shares of MongoDB from $400.00 to $445.00 in a research report on Friday. One research analyst has rated the stock with a sell rating, three have issued a hold rating and twenty-one have issued a buy rating to the company. Based on data from MarketBeat.com, the company currently has a consensus rating of Moderate Buy and an average price target of $353.75.
MongoDB Price Performance
MDB stock opened at $379.98 on Monday. The company has a current ratio of 4.19, a quick ratio of 4.19 and a debt-to-equity ratio of 1.44. The stock has a market cap of $26.61 billion, a PE ratio of -81.37 and a beta of 1.04. The stock has a 50-day moving average price of $298.74 and a two-hundred day moving average price of $240.54. MongoDB has a 1 year low of $135.15 and a 1 year high of $398.89.
MongoDB (NASDAQ:MDB – Get Rating) last issued its earnings results on Thursday, June 1st. The company reported $0.56 earnings per share for the quarter, topping analysts’ consensus estimates of $0.18 by $0.38. The business had revenue of $368.28 million for the quarter, compared to the consensus estimate of $347.77 million. MongoDB had a negative net margin of 23.58% and a negative return on equity of 43.25%. The firm’s revenue for the quarter was up 29.0% on a year-over-year basis. During the same period in the previous year, the company earned ($1.15) earnings per share. Equities analysts forecast that MongoDB will post -2.85 EPS for the current fiscal year.
Insiders Place Their Bets
In other MongoDB news, Director Hope F. Cochran sold 2,174 shares of the business’s stock in a transaction on Thursday, June 15th. The shares were sold at an average price of $373.19, for a total transaction of $811,315.06. Following the sale, the director now owns 8,200 shares of the company’s stock, valued at $3,060,158. The transaction was disclosed in a legal filing with the SEC, which is accessible through this link. In related news, CEO Dev Ittycheria sold 49,249 shares of the business’s stock in a transaction on Monday, April 3rd. The shares were sold at an average price of $227.55, for a total value of $11,206,609.95. Following the sale, the chief executive officer now owns 222,311 shares of the company’s stock, valued at $50,586,868.05. The sale was disclosed in a legal filing with the SEC, which is accessible through the SEC website. Also, Director Hope F. Cochran sold 2,174 shares of the business’s stock in a transaction on Thursday, June 15th. The stock was sold at an average price of $373.19, for a total transaction of $811,315.06. Following the completion of the sale, the director now directly owns 8,200 shares in the company, valued at approximately $3,060,158. The disclosure for this sale can be found here. Over the last quarter, insiders have sold 108,856 shares of company stock valued at $27,327,511. 4.80% of the stock is currently owned by insiders.
Institutional Trading of MongoDB
Institutional investors have recently modified their holdings of the business. Price T Rowe Associates Inc. MD lifted its holdings in MongoDB by 13.4% in the first quarter. Price T Rowe Associates Inc. MD now owns 7,593,996 shares of the company’s stock valued at $1,770,313,000 after buying an additional 897,911 shares during the period. Vanguard Group Inc. lifted its holdings in MongoDB by 1.0% in the third quarter. Vanguard Group Inc. now owns 6,127,231 shares of the company’s stock valued at $1,216,623,000 after buying an additional 62,303 shares during the period. Franklin Resources Inc. lifted its holdings in MongoDB by 6.4% in the fourth quarter. Franklin Resources Inc. now owns 1,962,574 shares of the company’s stock valued at $386,313,000 after buying an additional 118,055 shares during the period. State Street Corp lifted its holdings in MongoDB by 1.8% in the first quarter. State Street Corp now owns 1,386,773 shares of the company’s stock valued at $323,280,000 after buying an additional 24,595 shares during the period. Finally, 1832 Asset Management L.P. lifted its holdings in MongoDB by 3,283,771.0% in the fourth quarter. 1832 Asset Management L.P. now owns 1,018,000 shares of the company’s stock valued at $200,383,000 after buying an additional 1,017,969 shares during the period. Institutional investors own 89.22% of the company’s stock.
About MongoDB
MongoDB, Inc provides general purpose database platform worldwide. The company offers MongoDB Atlas, a hosted multi-cloud database-as-a-service solution; MongoDB Enterprise Advanced, a commercial database server for enterprise customers to run in the cloud, on-premise, or in a hybrid environment; and Community Server, a free-to-download version of its database, which includes the functionality that developers need to get started with MongoDB.
Featured Articles
Receive News & Ratings for MongoDB Daily – Enter your email address below to receive a concise daily summary of the latest news and analysts’ ratings for MongoDB and related companies with MarketBeat.com’s FREE daily email newsletter.
Article originally posted on mongodb google news. Visit mongodb google news
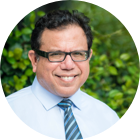
MMS • RSS
Posted on mongodb google news. Visit mongodb google news
Stanley Laman Group Ltd. cut its stake in MongoDB, Inc. (NASDAQ:MDB – Get Rating) by 16.5% during the 1st quarter, according to its most recent Form 13F filing with the Securities and Exchange Commission (SEC). The institutional investor owned 28,076 shares of the company’s stock after selling 5,566 shares during the period. MongoDB accounts for 1.2% of Stanley Laman Group Ltd.’s portfolio, making the stock its 14th largest position. Stanley Laman Group Ltd.’s holdings in MongoDB were worth $6,545,000 as of its most recent filing with the Securities and Exchange Commission (SEC).
Several other institutional investors have also recently added to or reduced their stakes in the stock. Cherry Creek Investment Advisors Inc. raised its holdings in shares of MongoDB by 1.5% during the fourth quarter. Cherry Creek Investment Advisors Inc. now owns 3,283 shares of the company’s stock worth $646,000 after purchasing an additional 50 shares during the period. Allworth Financial LP raised its holdings in shares of MongoDB by 12.9% during the fourth quarter. Allworth Financial LP now owns 508 shares of the company’s stock worth $100,000 after purchasing an additional 58 shares during the period. Cetera Advisor Networks LLC raised its holdings in shares of MongoDB by 7.4% during the second quarter. Cetera Advisor Networks LLC now owns 860 shares of the company’s stock worth $223,000 after purchasing an additional 59 shares during the period. First Republic Investment Management Inc. raised its holdings in shares of MongoDB by 1.0% during the fourth quarter. First Republic Investment Management Inc. now owns 6,406 shares of the company’s stock worth $1,261,000 after purchasing an additional 61 shares during the period. Finally, Janney Montgomery Scott LLC raised its holdings in shares of MongoDB by 4.5% during the fourth quarter. Janney Montgomery Scott LLC now owns 1,512 shares of the company’s stock worth $298,000 after purchasing an additional 65 shares during the period. 89.22% of the stock is owned by institutional investors and hedge funds.
Analyst Upgrades and Downgrades
A number of analysts recently weighed in on the stock. Robert W. Baird increased their price objective on shares of MongoDB from $390.00 to $430.00 in a report on Friday. The Goldman Sachs Group increased their price objective on shares of MongoDB from $420.00 to $440.00 in a report on Friday. Credit Suisse Group dropped their price objective on shares of MongoDB from $305.00 to $250.00 and set an “outperform” rating on the stock in a report on Friday, March 10th. JMP Securities increased their target price on shares of MongoDB from $245.00 to $370.00 in a research report on Friday, June 2nd. Finally, Stifel Nicolaus increased their target price on shares of MongoDB from $375.00 to $420.00 in a research report on Friday. One research analyst has rated the stock with a sell rating, three have given a hold rating and twenty-one have assigned a buy rating to the stock. Based on data from MarketBeat.com, MongoDB currently has a consensus rating of “Moderate Buy” and an average target price of $353.75.
MongoDB Stock Performance
Shares of MDB opened at $379.98 on Tuesday. MongoDB, Inc. has a one year low of $135.15 and a one year high of $398.89. The company has a 50-day moving average of $298.74 and a two-hundred day moving average of $240.54. The stock has a market cap of $26.61 billion, a price-to-earnings ratio of -81.37 and a beta of 1.04. The company has a debt-to-equity ratio of 1.44, a current ratio of 4.19 and a quick ratio of 4.19.
MongoDB (NASDAQ:MDB – Get Rating) last posted its quarterly earnings results on Thursday, June 1st. The company reported $0.56 earnings per share (EPS) for the quarter, topping analysts’ consensus estimates of $0.18 by $0.38. The business had revenue of $368.28 million during the quarter, compared to analyst estimates of $347.77 million. MongoDB had a negative net margin of 23.58% and a negative return on equity of 43.25%. The firm’s revenue was up 29.0% compared to the same quarter last year. During the same period in the prior year, the firm earned ($1.15) earnings per share. On average, equities research analysts expect that MongoDB, Inc. will post -2.85 earnings per share for the current year.
Insider Transactions at MongoDB
In other news, CAO Thomas Bull sold 605 shares of the stock in a transaction that occurred on Monday, April 3rd. The shares were sold at an average price of $228.34, for a total value of $138,145.70. Following the completion of the sale, the chief accounting officer now directly owns 17,706 shares of the company’s stock, valued at $4,042,988.04. The transaction was disclosed in a legal filing with the Securities & Exchange Commission, which is available through the SEC website. In related news, CAO Thomas Bull sold 605 shares of the firm’s stock in a transaction that occurred on Monday, April 3rd. The shares were sold at an average price of $228.34, for a total transaction of $138,145.70. Following the transaction, the chief accounting officer now owns 17,706 shares of the company’s stock, valued at $4,042,988.04. The sale was disclosed in a legal filing with the SEC, which is available through this hyperlink. Also, CFO Michael Lawrence Gordon sold 5,157 shares of the firm’s stock in a transaction that occurred on Monday, April 3rd. The shares were sold at an average price of $228.36, for a total transaction of $1,177,652.52. Following the transaction, the chief financial officer now directly owns 103,706 shares in the company, valued at approximately $23,682,302.16. The disclosure for this sale can be found here. Insiders sold 108,856 shares of company stock valued at $27,327,511 over the last three months. 4.80% of the stock is owned by corporate insiders.
About MongoDB
MongoDB, Inc provides general purpose database platform worldwide. The company offers MongoDB Atlas, a hosted multi-cloud database-as-a-service solution; MongoDB Enterprise Advanced, a commercial database server for enterprise customers to run in the cloud, on-premise, or in a hybrid environment; and Community Server, a free-to-download version of its database, which includes the functionality that developers need to get started with MongoDB.
Featured Stories
This instant news alert was generated by narrative science technology and financial data from MarketBeat in order to provide readers with the fastest and most accurate reporting. This story was reviewed by MarketBeat’s editorial team prior to publication. Please send any questions or comments about this story to contact@marketbeat.com.
Before you consider MongoDB, you’ll want to hear this.
MarketBeat keeps track of Wall Street’s top-rated and best performing research analysts and the stocks they recommend to their clients on a daily basis. MarketBeat has identified the five stocks that top analysts are quietly whispering to their clients to buy now before the broader market catches on… and MongoDB wasn’t on the list.
While MongoDB currently has a “Moderate Buy” rating among analysts, top-rated analysts believe these five stocks are better buys.
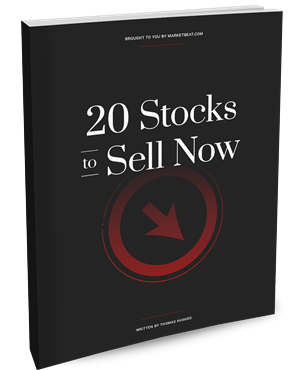
MarketBeat has just released its list of 20 stocks that Wall Street analysts hate. These companies may appear to have good fundamentals, but top analysts smell something seriously rotten. Are any of these companies lurking around your portfolio? Find out by clicking the link below.
Article originally posted on mongodb google news. Visit mongodb google news
MongoDB Launches AI Innovators Program to Help Organizations Innovate with Generative AI
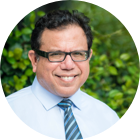
MMS • RSS
Posted on mongodb google news. Visit mongodb google news
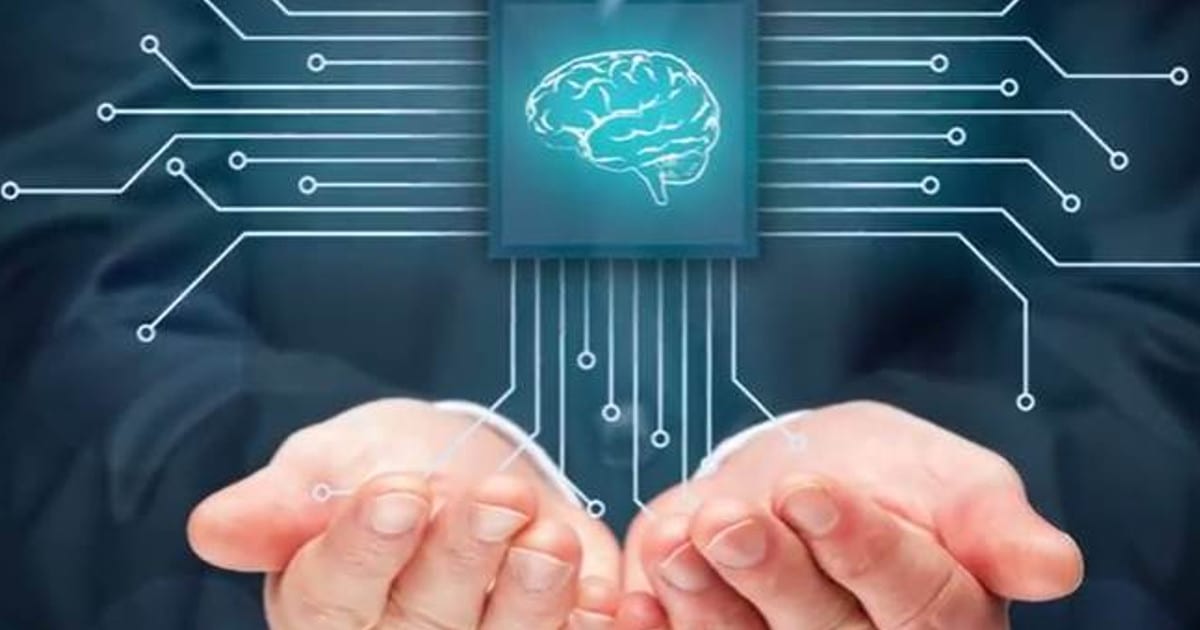
MongoDB today at its developer conference MongoDB.local NYC announced the MongoDB AI Innovators Program—which provides organizations building AI technology access to credits for MongoDB Atlas, partnership opportunities in the MongoDB Partner Ecosystem, and go-to-market activities with MongoDB to accelerate innovation and time to market. The MongoDB AI Innovators Program consists of the AI Startups track for early-stage ventures and the AI Amplify track for more established companies—both of which provide opportunities to join a community of founders, developers, and MongoDB experts to bring AI-powered solutions to market more quickly. To get started with the MongoDB AI Innovators Program, visit mongodb.com/startups/ai-innovators.
Generative AI is changing technology stacks from developer tooling to back-office functions to application end-user experiences. With today’s breakneck pace of innovation, organizations from early-stage startups to established enterprises are exploring ways they can take advantage of generative AI to reimagine business operations, invent entirely new ways of doing business, and disrupt industries. However, organizations face the challenges of incorporating generative AI into their existing applications by bolting on additional technology or having to build new classes of applications from the ground up—both of which are expensive, time consuming, and require specialized knowledge. Because of these challenges, many organizations are unable to establish the best path forward with the right technology stack for their use cases and risk being outpaced by competitors. These organizations want a clearer path to innovation with generative AI and the ability to work with established technology leaders by using the best tooling for the job, working with partners that are subject matter experts, and identifying ways to get their AI-powered applications to market quickly.
With the MongoDB AI Innovators program, organizations of all sizes have the opportunity to work with MongoDB and its global network of partners for free access to technology, go-to-market initiatives, and collaboration with subject matter experts:
Expanded MongoDB Atlas credits: The AI Startup track of the MongoDB AI Innovators Program provides eligible organizations up to $25,000 in credits for MongoDB Atlas—the leading multi-cloud developer data platform that accelerates and simplifies building with data—in addition to credits available via the MongoDB for Startups program. With the capabilities needed to work with large-language model (LLM) embeddings using MongoDB Atlas Vector Search and seamless access to LLM tools and providers (e.g., AWS, Anthropic, Google Cloud, Hugging Face, LangChain, LlamaIndex, Microsoft Azure, MindsDB, and OpenAI), organizations can build AI-powered applications with MongoDB Atlas to reimagine end-user experiences on a single platform with the performance, security, and scale modern applications require. Since 2019, MongoDB has provided over $25 million in credits to more than 8,000 startups to help companies like Vanta, Unqork, and Concured rapidly prototype and iterate applications and bring them to production with MongoDB Atlas. With expanded access to MongoDB Atlas credits, startups have the opportunity to remove technological barriers and go from idea to AI-powered solution more quickly and at less cost. Expanded access to MongoDB Atlas credits is also available to startups that enter the program through MongoDB Partners—including AWS, Google Cloud, and Microsoft Azure—and eligible startups can apply to work with MongoDB Ventures to help secure early-stage funding.
Amplify visibility into products and accelerate time to market: While the AI Startup Track provides early-stage startups with exclusive benefits and additional MongoDB Atlas credits, the AI Amplify track is open to a wide range of business sizes and models. Through this program, AI submissions are fast-tracked by MongoDB experts and evaluated for strategic partnerships and joint go-to-market motions. Companies can take advantage of the AI Amplify track to get greater visibility into projects and exposure to new markets. MongoDB technical experts are also available for solutions architecture and to help identify compelling use cases to use in co-marketing opportunities.
Access to MongoDB Partners and solutions: The MongoDB Partner Ecosystem includes more than 1,000 organizations ranging from Databricks to BigID to Accenture, and MongoDB is the only independent software vendor (ISV) featured in all three major cloud providers’ startup programs. MongoDB customers like Forbes, Toyota, and Powerledger have taken advantage of industry solutions and technology integrations with MongoDB Partners to accelerate their pace of innovation and bring new applications to market more quickly. Organizations that participate in the MongoDB AI Innovators Program will have prioritized access to opportunities with MongoDB Partners, and eligible organizations can be fast-tracked to join the MongoDB Partner Ecosystem to build seamless, interoperable integrations and joint solutions under the Technology path; build modern, resilient, and secure solutions faster in the Powered by MongoDB path; or create innovative solutions with proven expertise and certified skills in the Services path—all with a go-to-market focus and together bringing AI solutions to new and existing users.
“We’re at an inflection point with generative AI, with startups and established companies exploring how they can use new technology to change the way they do business and disrupt industries,” said Peder Ulander, Chief Marketing and Strategy Officer at MongoDB. “We have a long history of partnering with startups and enterprises to help them build new products and services using MongoDB Atlas and are thrilled to offer additional resources and the opportunity for developers and founders to join a community of innovators that are working to build the future with AI.”
Altimeter is a leading technology-focused investment firm built by founders for founders. “Enterprises are seeing a disruptive shift with the rise of AI, particularly generative AI,” said Pauline Yang, Partner at Altimeter. “Every company needs to re-imagine its products and the roles AI will play in both internal and external applications and workflows. Developers play a critical role in ensuring the success of democratizing and deploying this technology.”
Redpoint partners with visionary entrepreneurs to create new markets and redefine existing ones. “Recent advancements in AI have illuminated a transformational shift in our industry that is on par with—and maybe even more impactful than—the advent of mobile,” said Erica Brescia, Managing Director at Redpoint. “New AI-powered platforms for software development promise an explosion of new apps and will eventually redefine what it means to be a developer. In the short term, they are already enabling developers to be several times more productive, and as they continue to become more powerful, the skills a developer needs to be successful will change completely. The only question is how long this will take. One thing seems for sure: it will be years and not decades.”
Article originally posted on mongodb google news. Visit mongodb google news
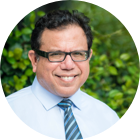
MMS • RSS
Posted on nosqlgooglealerts. Visit nosqlgooglealerts
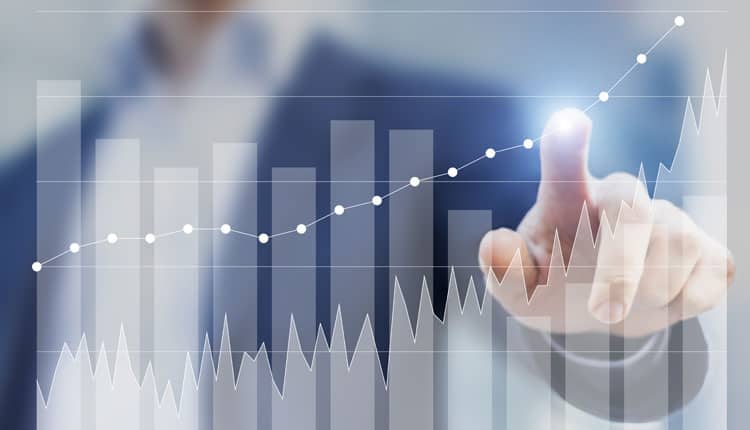
MongoDB announced the general availability of MongoDB Relational Migrator, a new tool that simplifies application migration and transformation—from legacy relational to modern document-based data models—providing organizations a streamlined way to improve operational efficiency and get more out of their data. Data is the foundation of every application with a large portion of it still residing in legacy relational databases where it can’t easily support emerging applications that leverage new technologies using a fully managed, multi-cloud developer data platform with best-in-class security, resilience, and performance. Already in use by tens of thousands of customers and millions of developers around the world, MongoDB Atlas’s flexible document model and scale-out capabilities are helping customers build modern applications that leverage the latest technologies, empowering them to reimagine business operations and end-user experiences. Now, with MongoDB Relational Migrator, more organizations across all industries can quickly, easily, cost-effectively, and with little-to-no risk migrate from legacy databases and embrace the future.
Organizations today have a clear imperative—modernize legacy applications to prepare their businesses for the future. New technologies like generative AI and large language models (LLMs) are another wave in a series of innovations over the past few decades that are opening up new possibilities for what’s possible with software and data for business operations and end-user experiences. Organizations of all sizes want to be able to make use of new technologies to transform their businesses. However, many companies remain locked-in to legacy relational databases in the backend of their applications, limiting their ability to adapt and modernize. These legacy databases are rigid, unadaptable, and difficult to use for supporting modern applications because of the complexity involved in mapping relationships between data when application requirements inevitably change. Additionally, because legacy databases were designed for an era before the advent of cloud computing, it is difficult to scale these databases without incurring significant costs. As a result, incorporating new technologies, quickly adapting to dynamic market changes, or continuously inventing new experiences for end-users are out of reach. For these reasons, customers are increasingly looking to migrate to a more flexible and scalable document-based data model that is easier to use and adapt. However, there is often considerable time, cost, and risk associated with these migrations because they require highly specialized tooling and knowledge to assess existing applications and prepare data for migration. Even then, the migration process can result in data loss, application downtime, and a migrated application that does not function as intended. Together, these challenges often prevent even the most well-funded and technologically savvy organizations from being able to cost-effectively migrate and modernize their applications so they can be ready for the future.
With MongoDB Relational Migrator, customers can migrate and modernize legacy applications without the time, cost, and risk typically associated with these projects—making it significantly faster and easier to optimize business operations and inspire developer innovation. MongoDB Relational Migrator analyzes legacy databases, automatically generates new data schema and code, and then executes a seamless migration to MongoDB Atlas with no downtime required. Customers can quickly get started by simply connecting MongoDB Relational Migrator to their existing application database (e.g., Oracle, Microsoft SQL Server, MySQL, and PostgreSQL) for assessment. After analyzing the application data, MongoDB Relational Migrator suggests a new data schema, transforms and migrates data to MongoDB Atlas with the ability to run continuous sync jobs for zero-downtime migrations, and generates optimized code for working with data in the new, modernized application. Customers can then run the modernized application in a testing environment to ensure it is operating as intended before deploying it to production. Using MongoDB Relational Migrator, organizations of all shapes and sizes can eliminate the barriers and heavy lifting associated with migrating and modernizing applications to ensure they are better equipped to build the next generation of highly engaging, mission-critical applications.
“Customers often tell us it’s crucial that they modernize their legacy applications so they can quickly build new end-user experiences that take advantage of game-changing technologies and ship new features at high velocity. But they also say that it’s too risky, expensive, and time consuming, or that they just don’t know how to get started,” said Sahir Azam, Chief Product Officer at MongoDB. “With MongoDB Relational Migrator, customers can now realize the full potential of software, data, and new technologies like generative AI by migrating and modernizing their legacy applications with a seamless, zero-downtime migration experience and without the heavy lifting. It’s now easier than ever to modernize applications and create innovative end-user experiences at the speed and scale that modern applications require with MongoDB Atlas.”
Customers that want a tailored modernization experience can work with MongoDB Professional Services and MongoDB Ecosystem Partners (e.g., Accenture, Capgemini, Globant, and Tech Mahindra) to unlock what’s possible with the next generation of software and data.
Accenture is a global professional services company with leading capabilities in digital, cloud, and security. “Together, Accenture and MongoDB provide unparalleled expertise to help customers modernize their environments and adopt a cloud-first approach throughout their organizations. Our partnership helps enterprises unlock value from data by modernizing and building new applications faster,” said Stephen Meyer, Associate Director, Cloud First Software Engineering, NoSQL Lead at Accenture. “Along with Accenture’s own capabilities and solutions, the release of MongoDB Relational Migrator will enable customers to accelerate their modernization strategies.”
Capgemini is a global leader in partnering with companies to transform and manage their business by harnessing the power of technology. “Capgemini’s collaboration with MongoDB has been a stepping stone to enhance strong migration offerings and modernizing legacy systems. This has enabled customers to reap the benefits of new technology and helped them build the next generation of applications,” said Prasad Bakshi, Global Head of the Database Migration Practice at Capgemini. “Coupled with Capgemini’s proprietary Data Convert & Compare (DCC) accelerator, MongoDB Relational Migrator will enable us to provide unique database migration as-a-service capabilities to our customers. We’re excited to be able to accelerate the modernization journey for organizations of all shapes and sizes.”
Globant is a digitally native company focused on reinventing businesses through innovative technology solutions. “By leveraging MongoDB, our customers have seen immense benefits including accelerated development, transformation, cost savings and legacy modernization,” said Nicolás Ávila, Chief Technology Officer for North America at Globant. “We are seeing more and more customers leverage MongoDB’s Relational Migrator to migrate from traditional, relational databases to MongoDB Atlas with no downtime, making it a seamless and efficient solution. We look forward to using MongoDB tools to build more unique, modern digital experiences for our customers that help them reinvent their industries and outpace their competition.”
Nationwide is the world’s largest building society as well as one of the largest savings providers and a top-three provider of mortgages in the UK. “Recently, I had the chance to employ MongoDB’s Relational Migrator and I was genuinely amazed by its outstanding performance,” said Peter Madeley, Senior Software Engineer at Nationwide Building Society. “The user interface of the tool is intuitively designed and the entity relationship diagrams proved to be invaluable in offering a detailed visual representation of my data structures. This migrator not only streamlines the transition from relational data to a document model, but it also ensures data integrity and offers a high degree of adaptability.”
Founded in 2016, Powerledger develops software solutions for the tracking, tracing, and trading of renewable energy. “We needed to demonstrate our platform’s ability to ingest a much higher volume of data and cater to the one billion users we aim to serve in the future, which required a level of scalability and flexibility that our previous relational database couldn’t offer,” said Dr. Vivek Bhandari, CTO at Powerledger. “Migrating an entire database is a pretty bold and risky endeavor. Our main priorities—and challenges—were to do a complete data platform migration, as well as add in scalability and flexibility without disrupting the platform or hindering data security. Amazingly, using MongoDB Relational Migrator, we didn’t experience any disruption or downtime.”
Tech Mahindra is a leading provider of digital transformation, consulting, and business re-engineering services and solutions. “The partnership with MongoDB helps unlock the full potential of data, data transformation, migration, and data consistency,” said Kunal Purohit, Chief Digital Services Officer at Tech Mahindra. “Tech Mahindra and MongoDB, together, will navigate the vast sea of information, harness its power, and chart a course towards industry-wide transformation journeys. Our enterprise customers can hugely benefit from this tool by leveraging its readily available migration interfaces, which in turn will help them quickly onboard the required data interfaces onto the target platform.”
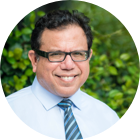
MMS • RSS
Posted on mongodb google news. Visit mongodb google news
MongoDB announced the general availability of MongoDB Relational Migrator, a new tool that simplifies application migration and transformation—from legacy relational to modern document-based data models—providing organizations a streamlined way to improve operational efficiency and get more out of their data. Data is the foundation of every application with a large portion of it still residing in legacy relational databases where it can’t easily support emerging applications that leverage new technologies using a fully managed, multi-cloud developer data platform with best-in-class security, resilience, and performance. Already in use by tens of thousands of customers and millions of developers around the world, MongoDB Atlas’s flexible document model and scale-out capabilities are helping customers build modern applications that leverage the latest technologies, empowering them to reimagine business operations and end-user experiences. Now, with MongoDB Relational Migrator, more organizations across all industries can quickly, easily, cost-effectively, and with little-to-no risk migrate from legacy databases and embrace the future.
Organizations today have a clear imperative—modernize legacy applications to prepare their businesses for the future. New technologies like generative AI and large language models (LLMs) are another wave in a series of innovations over the past few decades that are opening up new possibilities for what’s possible with software and data for business operations and end-user experiences. Organizations of all sizes want to be able to make use of new technologies to transform their businesses. However, many companies remain locked-in to legacy relational databases in the backend of their applications, limiting their ability to adapt and modernize. These legacy databases are rigid, unadaptable, and difficult to use for supporting modern applications because of the complexity involved in mapping relationships between data when application requirements inevitably change. Additionally, because legacy databases were designed for an era before the advent of cloud computing, it is difficult to scale these databases without incurring significant costs. As a result, incorporating new technologies, quickly adapting to dynamic market changes, or continuously inventing new experiences for end-users are out of reach. For these reasons, customers are increasingly looking to migrate to a more flexible and scalable document-based data model that is easier to use and adapt. However, there is often considerable time, cost, and risk associated with these migrations because they require highly specialized tooling and knowledge to assess existing applications and prepare data for migration. Even then, the migration process can result in data loss, application downtime, and a migrated application that does not function as intended. Together, these challenges often prevent even the most well-funded and technologically savvy organizations from being able to cost-effectively migrate and modernize their applications so they can be ready for the future.
With MongoDB Relational Migrator, customers can migrate and modernize legacy applications without the time, cost, and risk typically associated with these projects—making it significantly faster and easier to optimize business operations and inspire developer innovation. MongoDB Relational Migrator analyzes legacy databases, automatically generates new data schema and code, and then executes a seamless migration to MongoDB Atlas with no downtime required. Customers can quickly get started by simply connecting MongoDB Relational Migrator to their existing application database (e.g., Oracle, Microsoft SQL Server, MySQL, and PostgreSQL) for assessment. After analyzing the application data, MongoDB Relational Migrator suggests a new data schema, transforms and migrates data to MongoDB Atlas with the ability to run continuous sync jobs for zero-downtime migrations, and generates optimized code for working with data in the new, modernized application. Customers can then run the modernized application in a testing environment to ensure it is operating as intended before deploying it to production. Using MongoDB Relational Migrator, organizations of all shapes and sizes can eliminate the barriers and heavy lifting associated with migrating and modernizing applications to ensure they are better equipped to build the next generation of highly engaging, mission-critical applications.
“Customers often tell us it’s crucial that they modernize their legacy applications so they can quickly build new end-user experiences that take advantage of game-changing technologies and ship new features at high velocity. But they also say that it’s too risky, expensive, and time consuming, or that they just don’t know how to get started,” said Sahir Azam, Chief Product Officer at MongoDB. “With MongoDB Relational Migrator, customers can now realize the full potential of software, data, and new technologies like generative AI by migrating and modernizing their legacy applications with a seamless, zero-downtime migration experience and without the heavy lifting. It’s now easier than ever to modernize applications and create innovative end-user experiences at the speed and scale that modern applications require with MongoDB Atlas.”
Customers that want a tailored modernization experience can work with MongoDB Professional Services and MongoDB Ecosystem Partners (e.g., Accenture, Capgemini, Globant, and Tech Mahindra) to unlock what’s possible with the next generation of software and data.
Accenture is a global professional services company with leading capabilities in digital, cloud, and security. “Together, Accenture and MongoDB provide unparalleled expertise to help customers modernize their environments and adopt a cloud-first approach throughout their organizations. Our partnership helps enterprises unlock value from data by modernizing and building new applications faster,” said Stephen Meyer, Associate Director, Cloud First Software Engineering, NoSQL Lead at Accenture. “Along with Accenture’s own capabilities and solutions, the release of MongoDB Relational Migrator will enable customers to accelerate their modernization strategies.”
Capgemini is a global leader in partnering with companies to transform and manage their business by harnessing the power of technology. “Capgemini’s collaboration with MongoDB has been a stepping stone to enhance strong migration offerings and modernizing legacy systems. This has enabled customers to reap the benefits of new technology and helped them build the next generation of applications,” said Prasad Bakshi, Global Head of the Database Migration Practice at Capgemini. “Coupled with Capgemini’s proprietary Data Convert & Compare (DCC) accelerator, MongoDB Relational Migrator will enable us to provide unique database migration as-a-service capabilities to our customers. We’re excited to be able to accelerate the modernization journey for organizations of all shapes and sizes.”
Globant is a digitally native company focused on reinventing businesses through innovative technology solutions. “By leveraging MongoDB, our customers have seen immense benefits including accelerated development, transformation, cost savings and legacy modernization,” said Nicolás Ávila, Chief Technology Officer for North America at Globant. “We are seeing more and more customers leverage MongoDB’s Relational Migrator to migrate from traditional, relational databases to MongoDB Atlas with no downtime, making it a seamless and efficient solution. We look forward to using MongoDB tools to build more unique, modern digital experiences for our customers that help them reinvent their industries and outpace their competition.”
Nationwide is the world’s largest building society as well as one of the largest savings providers and a top-three provider of mortgages in the UK. “Recently, I had the chance to employ MongoDB’s Relational Migrator and I was genuinely amazed by its outstanding performance,” said Peter Madeley, Senior Software Engineer at Nationwide Building Society. “The user interface of the tool is intuitively designed and the entity relationship diagrams proved to be invaluable in offering a detailed visual representation of my data structures. This migrator not only streamlines the transition from relational data to a document model, but it also ensures data integrity and offers a high degree of adaptability.”
Founded in 2016, Powerledger develops software solutions for the tracking, tracing, and trading of renewable energy. “We needed to demonstrate our platform’s ability to ingest a much higher volume of data and cater to the one billion users we aim to serve in the future, which required a level of scalability and flexibility that our previous relational database couldn’t offer,” said Dr. Vivek Bhandari, CTO at Powerledger. “Migrating an entire database is a pretty bold and risky endeavor. Our main priorities—and challenges—were to do a complete data platform migration, as well as add in scalability and flexibility without disrupting the platform or hindering data security. Amazingly, using MongoDB Relational Migrator, we didn’t experience any disruption or downtime.”
Tech Mahindra is a leading provider of digital transformation, consulting, and business re-engineering services and solutions. “The partnership with MongoDB helps unlock the full potential of data, data transformation, migration, and data consistency,” said Kunal Purohit, Chief Digital Services Officer at Tech Mahindra. “Tech Mahindra and MongoDB, together, will navigate the vast sea of information, harness its power, and chart a course towards industry-wide transformation journeys. Our enterprise customers can hugely benefit from this tool by leveraging its readily available migration interfaces, which in turn will help them quickly onboard the required data interfaces onto the target platform.”
Article originally posted on mongodb google news. Visit mongodb google news
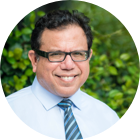
MMS • Javier Fernandez-Ivern
Article originally posted on InfoQ. Visit InfoQ
Stress Free Change Validation at Netflix
Summary
Javier Fernandez-Ivern discusses why a high confidence change process for code bases is needed, how zero-noise diffs help close the confidence gap, and recommended practices for building a diff system
Bio
Javier Fernandez-Ivern is a member of the Playback Experience team at Netflix, where he is responsible for ensuring that customers always enjoy their favorite shows with the best video, audio, text, and other features available. After trying out management at Capital One, he returned to his software engineering roots and joined Netflix.
About the conference
QCon Plus is a virtual conference for senior software engineers and architects that covers the trends, best practices, and solutions leveraged by the world’s most innovative software organizations.
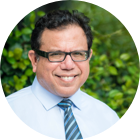
MMS • RSS
Posted on mongodb google news. Visit mongodb google news
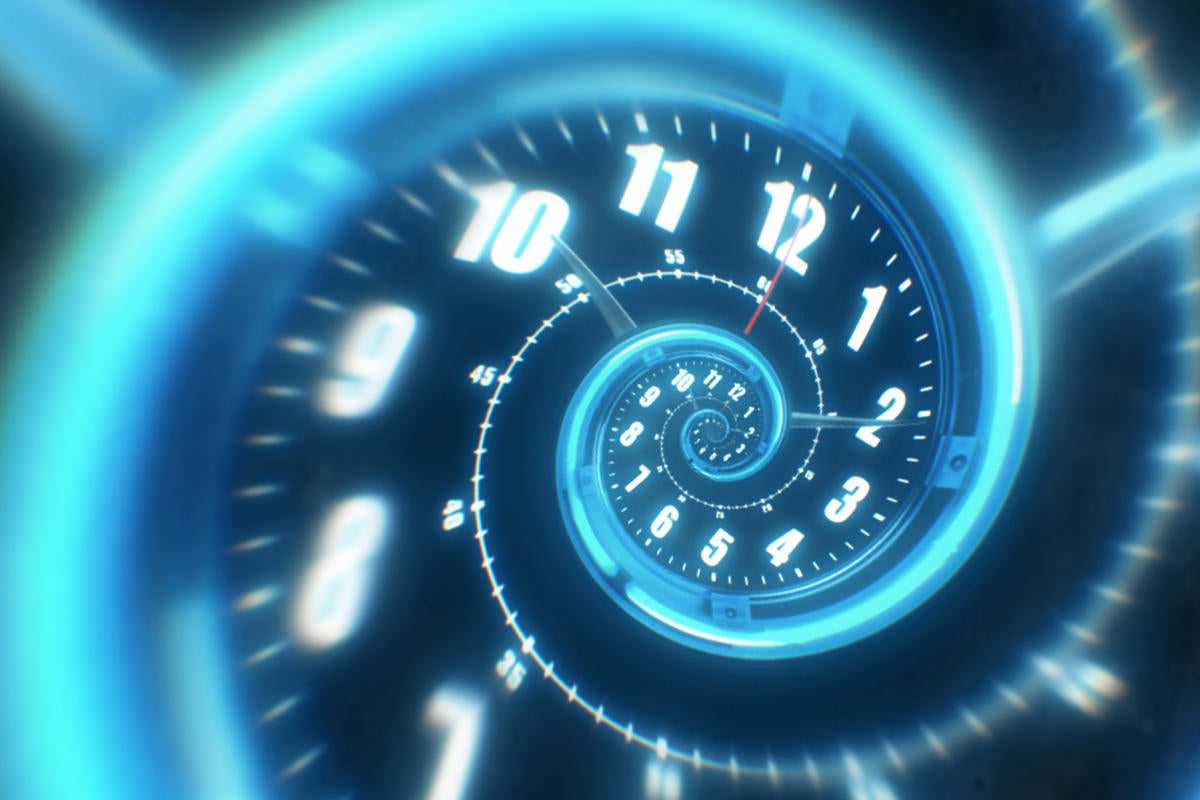
Much of the data accumulated in today’s world is in JSON (JavaScript Object Notation) format. However, many of the databases designed with a JSON-first mindset have not been able to provide the sort of in-app analytics available in classic SQL systems, leaving a huge gap in the amount of the world’s data that is able to be analyzed in real time. In an era when even millisecond lags are too slow, this is a gap in the market that needs to be addressed.
SingleStore Kai API for MongoDB is intended to solve this problem, and to do so in a way that is simple and straightforward. Let’s take a closer look at the key features of SingleStore Kai.
100x faster analytics on JSON data
With SingleStore Kai, you can perform complex analytics on JSON data for MongoDB applications faster and more efficiently. On some benchmarks, SingleStoreDB was able to drive 100x faster analytical performance for most queries. How is this speed boost achieved? The SingleStore MongoDB API proxy translates MongoDB queries into SQL statements that are executed by SingleStoreDB to power real-time analytics for your applications.
Vector functionality for JSON
The new era of generative AI requires real-time analytics on all data, including JSON collections. SingleStoreDB supports vectors and fast vector similarity search using the $dotProduct
and $euclideanDistance
functions. With SingleStore Kai, developers can harness the vector and AI capabilities on JSON collections within MongoDB, enabling use cases like semantic search, image recognition, and similarity matching.
No code changes or data transformations
Developers can continue to use existing MongoDB queries. They don’t have to normalize or flatten data, or do extensive schema migrations to power fast analytics for their applications. SingleStore Kai requires no code changes, data transformations, schema migrations, or changes to existing queries.
Same MongoDB tools and drivers
SingleStore Kai supports the MongoDB wire protocol and allows MongoDB clients to communicate with a SingleStoreDB cluster. Developers can take advantage of fast analytics on SingleStoreDB without having to learn a new set of tools or APIs. And they can continue to use the same MongoDB tools and drivers their customers are most familiar with.
Best of both worlds (NoSQL and SQL)
SingleStoreDB was already MySQL wire protocol compatible. With the addition of SingleStore Kai for MongoDB, the database gives developers essentially the best of both worlds—the schema flexibility and simplicity of a JSON document store and the speed, efficiency, and complex analytical capabilities of a relational SQL database.
Easy data replication
As part of this MongoDB API offering, SingleStoreDB includes a fast and efficient replication service (in private preview) that copies MongoDB collections into SingleStoreDB. This service is natively integrated into SingleStoreDB and leverages one of SingleStore’s most widely used features, SingleStore Pipelines, to drive speedy replication and real-time change data capture, enabling customers to get started quickly and easily.
Real-time data and real-time analytics play a crucial role in modern business. With SingleStore Kai, regardless of whether you traditionally work in SQL or NoSQL, you now have the ability to do real-time analytics on the majority of data in our fast-moving world.
Jason Thorsness is a principal software engineer at SingleStore.
—
New Tech Forum provides a venue to explore and discuss emerging enterprise technology in unprecedented depth and breadth. The selection is subjective, based on our pick of the technologies we believe to be important and of greatest interest to InfoWorld readers. InfoWorld does not accept marketing collateral for publication and reserves the right to edit all contributed content. Send all inquiries to newtechforum@infoworld.com.
Next read this:
Article originally posted on mongodb google news. Visit mongodb google news
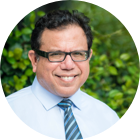
MMS • Steef-Jan Wiggers
Article originally posted on InfoQ. Visit InfoQ
Microsoft recently introduced .NET Framework Custom Code for Logic Apps Standard in public preview, allowing developers to call compiled .NET Framework code from a built-in action in their workflow.
Azure Logic Apps are Microsoft’s automated workflow offering, and its Logic App Standard tier allows developers to run workflows anywhere. Since its general availability in 2021, it has received several updates, such as support of .NET Framework assemblies in XSLT maps in Logic Apps, observability enhancements, and new data mapper. With the introduction of .NET Framework Custom Code, the company aims to push further the standard tier to help customers migrate from BizTalk Server to Azure. In addition, it brings extensibility capability for developers to solve more complex integration problems.
The .NET Framework Custom Code capability for Logic Apps Standard provides developers with a local VS Code experience, allowing them to step through their workflows and code in the same debugging session. Currently, the support is for .NET Framework 4.7.2 assemblies.
The latest Logic Apps extension for VS Code introduces a new logic app workspace template to enhance the development process, enabling streamlined custom code writing, debugging, and deployment within workflows. The template includes a workspace file and two sample projects—one for custom code authoring and the other for workflow authoring. Developers cannot mix custom code and workflows in the same project.
In a question on Twitter about the distinction that the workflow standard service plan allows for side-by-side hosting of function apps and how it differs from using a function or HTTP actions, Kent Weare, a Principal Program Manager of Logic Apps at Microsoft, answered:
Under the hood, we are creating a function, but it runs on the same Logic Apps infra (and service plan), you don’t have an additional security surface, and you can locally debug code and workflow in the same VS Code session. We also support dynamic schema for the token picker.
The company advises developers to use .NET Framework custom code extensibility to complement their low code integration solutions in cases like custom parsing, data validation, and simple data transformations – not for compute-heavy scenarios like streaming, long-running code, and complex batching or debatching.
When asked by InfoQ about what is driving this investment from Microsoft, here is what Weare had to say:
We chose to support .NET Framework 4.7.2 based upon customer feedback where organizations have invested in .NET Framework to extend their BizTalk Server solutions. By supporting .NET Framework 4.7.2, we allow customers to lift and shift their existing custom solutions from on-premises architectures to the cloud. The model we have used to introduce .NET Framework can be extended to support additional languages. This is something we will look to do after .NET Framework support reaches general availability (GA).
Lastly, the documentation pages and walkthrough video show more details and guidance of the .NET Framework Custom Code in Logic Apps Standard.
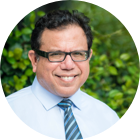
MMS • Deepak Vohra
Article originally posted on InfoQ. Visit InfoQ
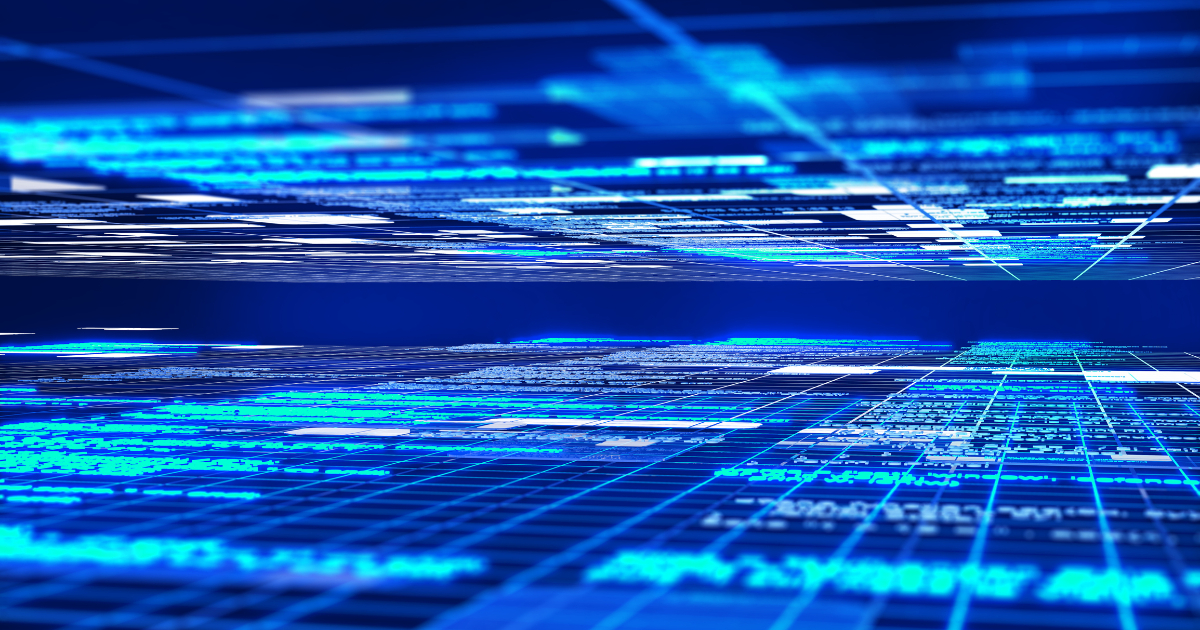
Key Takeaways
- Pattern matching for the
switch
control-flow statement is a new feature introduced in Java 17 and refined in subsequent versions. - A pattern can be used in case labels as
case p
. The selector expression is evaluated, and the resulting value is tested against the case labels that may include patterns. The execution path of the first matching case label applies to the switch statement/expression. - Pattern matching adds support for a selector expression of any reference type in addition to the existing legacy types.
- Guarded patterns can be used with the new
when
clause in a case label pattern. - Pattern matching can be used with the traditional switch statements and with the traditional fall-through semantics of a switch statement.
A switch
statement is a control-flow statement that was originally designed to be a short-form alternative to the if-else if-else control-flow statement for certain use cases that involved multiple possible execution paths based on what a given expression evaluates to.
A switch statement consists of a selector expression and a switch block consisting of case labels; the selector expression is evaluated, and the execution path is switched based on which case label matches the result of the evaluation.
Originally switch could only be used as a statement with the traditional case ...:
label syntax with fall-through semantics. Java 14 added support for the new case ...->
label syntax with no fall-through semantics.
Java 14 also added support for switch expressions. A switch expression evaluates to a single value. A yield
statement was introduced to yield a value explicitly.
Support for switch expressions, which is discussed in detail in another article, means that switch can be used in instances that expect an expression such as an assignment statement.
Problem
However, even with the enhancements in Java 14, the switch still has some limitations:
- The selector expression of switch supports only specific types, namely integral primitive data types
byte
,short
,char
, andint
; the corresponding boxed formsByte
,Short
,Character
, andInteger
; theString
class; and the enumerated types. - The result of the switch selector expression can be tested only for exact equality against constants. Matching a case label with a constant test only against one value.
- The
null
value is not handled like any other value. - Error handling is not uniform.
- The use of enums is not well-scoped.
Solution
A convenient solution has been proposed and implemented to counter these limitations: pattern matching for switch statements and expressions. This solution addresses all the issues mentioned above.
Pattern matching for the switch was introduced in JDK 17, refined in JDK 18, 19, and 20, and is to be finalized in JDK 21.
Pattern matching overcomes the limitations of the traditional switch in several ways:
- The type of the selector expression can be any reference type in addition to an integral primitive type (excluding
long
). - Case labels can include patterns in addition to constants. A pattern case label can apply to many values, unlike a constant case label that applies to only one value. A new case label,
case p
, is introduced in whichp
is a pattern. - Case labels can include
null
. - An optional
when
clause can follow a case label for conditional or guarded pattern matching. A case label with a when is called a guarded case label. - Enum constant case labels can be qualified. The selector expression doesn’t have to be an enum type when using enum constants when using enum constants.
- The
MatchException
is introduced for a more uniform error handling in pattern matching. - The traditional switch statements and the traditional fall-through semantics also support pattern matching.
A benefit of pattern matching is to facilitate data oriented programming, such as improving the performance of complex data-oriented queries.
What is pattern matching?
Pattern matching is a powerful feature that extends the functionality of control-flow structures in programming. This feature allows a selector expression to be tested against several patterns in addition to the test against traditionally supported constants. The semantics of the switch stays unchanged; a switch selector expression value is tested against case labels that may include patterns, and if the selector expression value matches a case label pattern, that case label applies to the execution path of the switch control-flow. The only enhancement is that the selector expression can be any reference type in addition to primitive integral types (excluding long). The case labels can include patterns in addition to constants. Additionally, supporting null and qualified enum constants in case labels is an added feature.
The grammar of switch labels in a switch block is as follows:
SwitchLabel:
case CaseConstant { , CaseConstant }
case null [, default]
case Pattern
default
Pattern matching can be used with the traditional case …:
label syntax with fall-through semantics, and with the case … ->
label syntax with no fall-through semantics. Nonetheless, it’s essential to note that a switch block cannot mix the two types of case labels.
With these modifications in place, pattern matching has paved the way for more sophisticated control-flow structures, transforming the richer way to approach logic in code.
Setting the environment
The only prerequisite to running the code samples in this article is to install Java 20 or Java 21 (if available). Java 21 makes only one enhancement over Java 20, which is support for qualified enum constants in case labels. The Java version can be found with the following command:
java --version
java version "20.0.1" 2023-04-18
Java(TM) SE Runtime Environment (build 20.0.1+9-29)
Java HotSpot(TM) 64-Bit Server VM (build 20.0.1+9-29, mixed mode, sharing)
Because switch pattern matching is a preview feature in Java 20, javac
and java
commands must be run with the following syntax:
javac --enable-preview --release 20 SampleClass.java
java --enable-preview SampleClass
However, one can directly run this using the source code launcher. In that case, the command line would be:
java --source 20 --enable-preview Main.java
The jshell option is also available but requires enabling the preview feature as well:
jshell --enable-preview
A simple example of pattern matching
We start with a simple example of pattern matching in which the selector expression type of a switch expression is reference type; Collection;
and the case labels include patterns of the form case p
.
import java.util.Collection;
import java.util.LinkedList;
import java.util.Stack;
import java.util.Vector;
public class SampleClass {
static Object get(Collection c) {
return switch (c) {
case Stack s -> s.pop();
case LinkedList l -> l.getFirst();
case Vector v -> v.lastElement();
default -> c;
};
}
public static void main(String[] argv) {
var stack = new Stack();
stack.push("firstStackItemAdded");
stack.push("secondStackItemAdded");
stack.push("thirdStackItemAdded");
var linkedList = new LinkedList();
linkedList.add("firstLinkedListElementAdded");
linkedList.add("secondLinkedListElementAdded");
linkedList.add("thirdLinkedListElementAdded");
var vector = new Vector();
vector.add("firstVectorElementAdded");
vector.add("secondVectorElementAdded");
vector.add("thirdVectorElementAdded");
System.out.println(get(stack));
System.out.println(get(linkedList));
System.out.println(get(vector));
}
}
Compile and run the Java application, with the output:
thirdStackItemAdded
firstLinkedListElementAdded
thirdVectorElementAdded
Pattern matching supports all reference types
In the example given earlier, the Collection
class type is used as the selector expression type. However, any reference type can serve as the selector expression type. Therefore, the case label patterns can be of any reference type compatible with the selector expression value. For example, the following modified SampleClass uses an Object type selector expression and includes case label patterns for a record pattern and an array reference type pattern, in addition to the case label patterns for previously used Stack
, LinkedList
, and Vector
reference types.
import java.util.LinkedList;
import java.util.Stack;
import java.util.Vector;
record CollectionType(Stack s, Vector v, LinkedList l) {
}
public class SampleClass {
static Object get(Object c) {
return switch (c) {
case CollectionType r -> r.toString();
case String[] arr -> arr.length;
case Stack s -> s.pop();
case LinkedList l -> l.getFirst();
case Vector v -> v.lastElement();
default -> c;
};
}
public static void main(String[] argv) {
var stack = new Stack();
stack.push("firstStackItemAdded");
stack.push("secondStackItemAdded");
stack.push("thirdStackItemAdded");
var linkedList = new LinkedList();
linkedList.add("firstLinkedListElementAdded");
linkedList.add("secondLinkedListElementAdded");
linkedList.add("thirdLinkedListElementAdded");
var vector = new Vector();
vector.add("firstVectorElementAdded");
vector.add("secondVectorElementAdded");
vector.add("thirdVectorElementAdded");
var r = new CollectionType(stack, vector, linkedList);
System.out.println(get(r));
String[] stringArray = {"a", "b", "c"};
System.out.println(get(stringArray));
System.out.println(get(stack));
System.out.println(get(linkedList));
System.out.println(get(vector));
}
}
This time the output is as follows:
CollectionType[s=[firstStackItemAdded, secondStackItemAdded, thirdStackItemAdded
], v=[firstVectorElementAdded, secondVectorElementAdded, thirdVectorElementAdded
], l=[firstLinkedListElementAdded, secondLinkedListElementAdded, thirdLinkedList
ElementAdded]]
3
thirdStackItemAdded
firstLinkedListElementAdded
thirdVectorElementAdded
The null case label
Traditionally, a switch throws a NullPointerException
at runtime if the selector expression evaluates to null
. A null selector expression is not a compile-time issue. The following simple application with a match-all case label default
demonstrates how a null selector expression throws a NullPointerException
at runtime.
import java.util.Collection;
public class SampleClass {
static Object get(Collection c) {
return switch (c) {
default -> c;
};
}
public static void main(String[] argv) {
get(null);
}
}
It is possible to test a null value explicitly outside the switch block and invoke a switch only if non-null, but that involves adding if-else code. Java has added support for the case null
in the new pattern matching feature. The switch statement in the following application uses the case null
to test the selector expression against null.
import java.util.Collection;
public class SampleClass {
static void get(Collection c) {
switch (c) {
case null -> System.out.println("Did you call the get with a null?");
default -> System.out.println("default");
}
}
public static void main(String[] argv) {
get(null);
}
}
At runtime, the application outputs:
Did you call the get with a null?
The case null can be combined with the default
case as follows:
import java.util.Collection;
public class SampleClass {
static void get(Collection c) {
switch (c) {
case null, default -> System.out.println("Did you call the get with a null?");
}
}
public static void main(String[] argv) {
get(null);
}
}
However, the case null cannot be combined with any other case label. For example, the following class combines the case null with a case label with a pattern Stack s
:
import java.util.Collection;
import java.util.Stack;
public class SampleClass {
static void get(Collection c) {
switch (c) {
case null, Stack s -> System.out.println("Did you call the get with a null?");
default -> System.out.println("default");
}
}
public static void main(String[] args) {
get(null);
}
}
The class generates a compile-time error:
SampleClass.java:11: error: invalid case label combination
case null, Stack s -> System.out.println("Did you call the get with a null?");
Guarded patterns with the when clause
Sometimes, developers may use a conditional case label pattern that is matched based on the outcome of a boolean expression. This is where the when
clause comes in handy. This clause evaluates a boolean expression, forming what is known as a ‘guarded pattern.’ For example, the when
clause in the first case label in the following code snippet determines if a Stack
is empty.
import java.util.Stack;
import java.util.Collection;
public class SampleClass {
static Object get(Collection c) {
return switch (c) {
case Stack s when s.empty() -> s.push("first");
case Stack s2 -> s2.push("second");
default -> c;
};
}
}
The corresponding code, located to the right of the ‘->
‘, only executes if the Stack is indeed empty.
The ordering of the case labels with patterns is significant
When using case labels with patterns, developers must ensure an order that doesn’t create any issues related to type or subtype hierarchy. That is because, unlike constant case labels, patterns in case labels allow a selector expression to be compatible with multiple case labels containing patterns. The switch pattern matching feature matches the first case label, where the pattern matches the value of the selector expression.
If the type of a case label pattern is a subtype of the type of another case label pattern that appears before it, a compile-time error will occur because the latter case label will be identified as unreachable code.
To demonstrate this scenario, developers can compile and run the following sample class in which a case label pattern of type Object
dominates a subsequent code label pattern of type Stack
.
import java.util.Stack;
public class SampleClass {
static Object get(Object c) {
return switch (c) {
case Object o -> c;
case Stack s -> s.pop();
};
}
}
When compiling the class, an error message is produced:
SampleClass.java:12: error: this case label is dominated by a preceding case lab
el
case Stack s -> s.pop();
^
The compile-time error can be fixed simply by reversing the order of the two case labels as follows:
public class SampleClass {
static Object get(Object c) {
return switch (c) {
case Stack s -> s.pop();
case Object o -> c;
};
}
}
Similarly, if a case label includes a pattern that is of the same reference type as a preceding case label with an unconditional/unguarded pattern (guarded patterns discussed in an earlier section), it will result in a compile-type error for the same reason, as in the class:
import java.util.Stack;
import java.util.Collection;
public class SampleClass {
static Object get(Collection c) {
return switch (c) {
case Stack s -> s.push("first");
case Stack s2 -> s2.push("second");
};
}
}
Upon compilation, the following error message is generated:
SampleClass.java:13: error: this case label is dominated by a preceding case lab
el
case Stack s2 -> s2.push("second");
^
To avoid such errors, developers should maintain a straightforward and readable ordering of case labels. The constant labels should be listed first, followed by the case null
label, the guarded pattern labels, and the non-guarded type pattern labels. The default
case label can be combined with the case null
label, or placed separately as the last case label. The following class demonstrates the correct ordering:
import java.util.Collection;
import java.util.Stack;
import java.util.Vector;
public class SampleClass {
static Object get(Collection c) {
return switch (c) {
case null -> c; //case label null
case Stack s when s.empty() -> s.push("first"); // case label with guarded pattern
case Vector v when v.size() > 2 -> v.lastElement(); // case label with guarded pattern
case Stack s -> s.push("first"); // case label with unguarded pattern
case Vector v -> v.firstElement(); // case label with unguarded pattern
default -> c;
};
}
}
Pattern matching can be used with the traditional switch statement and with fall-through semantics
The pattern-matching feature is independent of whether it is a switch statement or a switch expression. The pattern matching is also independent of whether the fall-through semantics with case …:
labels, or the no-fall-through semantics with the case …->
labels is used. In the following example, pattern matching is used with a switch statement and not a switch expression. The case labels use the fall-through semantics with the case …:
labels. A when
clause in the first case label uses a guarded pattern.
import java.util.Stack;
import java.util.Collection;
public class SampleClass {
static void get(Collection c) {
switch (c) {
case Stack s when s.empty(): s.push("first"); break;
case Stack s : s.push("second"); break;
default : break;
}
}
}
Scope of pattern variables
A pattern variable is a variable that appears in a case label pattern. The scope of a pattern variable is limited to the block, expression, or throw statement that appears to the right of the ->
arrow. To demonstrate, consider the following code snippet in which a pattern variable from a preceding case label is used in the default case label.
import java.util.Stack;
public class SampleClass {
static Object get(Object c) {
return switch (c) {
case Stack s -> s.push("first");
default -> s.push("first");
};
}
}
A compile-time error results:
import java.util.Collection;
SampleClass.java:13: error: cannot find symbol
default -> s.push("first");
^
symbol: variable s
location: class SampleClass
The scope of a pattern variable that appears in the pattern of a guarded case label includes the when clause, as demonstrated in the example:
import java.util.Stack;
import java.util.Collection;
public class SampleClass {
static Object get(Collection c) {
return switch (c) {
case Stack s when s.empty() -> s.push("first");
case Stack s -> s.push("second");
default -> c;
};
}
}
Given the limited scope of a pattern variable, the same pattern variable name can be used across multiple case labels. This is illustrated in the preceding example, where the pattern variable s
is used in two different case labels.
When dealing with a case label with fall-through semantics, the scope of a pattern variable extends to the group of statements located to the right of the ‘:
‘. That is why it was possible to use the same pattern variable name for the two case labels in the previous section by using pattern matching with the traditional switch statement. However, fall through case label that declares a pattern variable is a compile-time error. This can be demonstrated in the following variation of the earlier class:
import java.util.Stack;
import java.util.Vector;
import java.util.Collection;
public class SampleClass {
static void get(Collection c) {
switch (c) {
case Stack s : s.push("second");
case Vector v : v.lastElement();
default : System.out.println("default");
}
}
}
Without a break;
statement in the first statement group, the switch could fall-through the second statement group without initializing the pattern variable v
in the second statement group. The preceding class would generate a compile-time error:
SampleClass.java:12: error: illegal fall-through to a pattern
case Vector v : v.lastElement();
^
Simply adding a break;
statement in the first statement group as follows fixes the error:
import java.util.Stack;
import java.util.Vector;
import java.util.Collection;
public class SampleClass {
static void get(Collection c) {
switch (c) {
case Stack s : s.push("second"); break;
case Vector v : v.lastElement();
default : System.out.println("default");
}
}
}
Only one pattern per case label
Combining multiple patterns within a single case label, whether it is a case label of the type case …:
, or the type case …->
is not allowed, and it is a compile-time error. It may not be obvious, but combining patterns in a single case label incurs fall-through a pattern, as demonstrated by the following class.
import java.util.Stack;
import java.util.Vector;
import java.util.Collection;
public class SampleClass {
static Object get(Collection c) {
return switch (c) {
case Stack s, Vector v -> c;
default -> c;
};
}
}
A compile-time error is generated:
SampleClass.java:11: error: illegal fall-through from a pattern
case Stack s, Vector v -> c;
^
Only one match-all case label in a switch block
It is a compile-time error to have more than one match-all case labels in a switch block, whether it is a switch statement or a switch expression. The match-all case labels are :
- A case label with a pattern that unconditionally matches the selector expression
- The default case label
To demonstrate, consider the following class:
import java.util.Collection;
public class SampleClass {
static Object get(Collection c) {
return switch (c) {
case Collection coll -> c;
default -> c;
};
}
}
Compile the class, only to get an error message:
SampleClass.java:13: error: switch has both an unconditional pattern and a default label
default -> c;
^
The exhaustiveness of type coverage
Exhaustiveness implies that a switch block must handle all possible values of the selector expression. The exhaustiveness requirement is implemented only if one or more of the following apply:
- a) Pattern switch expressions/statements are used,
- b) The
case null
is used, - c) The selector expression is not one of the legacy types (
char
,byte
,short
,int
,Character
,Byte
,Short
,Integer
,String
, or an enum type).
To implement exhaustiveness, it may suffice to add case labels for each of the subtypes of the selector expression type if the subtypes are few. However, this approach could be tedious if subtypes are numerous; for example, adding a case label for each reference type for a selector expression of type Object
, or even each of the subtypes for a selector expression of type Collection
, is just not feasible.
To demonstrate the exhaustiveness requirement, consider the following class:
import java.util.Collection;
import java.util.Stack;
import java.util.LinkedList;
import java.util.Vector;
public class SampleClass {
static Object get(Collection c) {
return switch (c) {
case Stack s -> s.push("first");
case null -> throw new NullPointerException("null");
case LinkedList l -> l.getFirst();
case Vector v -> v.lastElement();
};
}
}
The class generates a compile-time error message:
SampleClass.java:10: error: the switch expression does not cover all possible in
put values
return switch (c) {
^
The issue can be fixed simply by adding a default case as follows:
import java.util.Collection;
import java.util.Stack;
import java.util.LinkedList;
import java.util.Vector;
public class SampleClass {
static Object get(Collection c) {
return switch (c) {
case Stack s -> s.push("first");
case null -> throw new NullPointerException("null");
case LinkedList l -> l.getFirst();
case Vector v -> v.lastElement();
default -> c;
};
}
}
A match-all case label with a pattern that unconditionally matches the selector expression, such as the one in the following class, would be exhaustive, but it wouldn’t handle or process any subtypes distinctly.
import java.util.Collection;
public class SampleClass {
static Object get(Collection c) {
return switch (c) {
case Collection coll -> c;
};
}
}
The default
case label could be needed for exhaustiveness but could sometimes be avoided if the possible values of a selector expression are very few. As an example, if the selector expression is of type java.util.Vector
, only one case label pattern for the single subclass java.util.Stack
is required to avoid the default
case. Similarly, if the selector expression is a sealed class type, only the classes declared in the permits clause of the sealed class type need to be handled by the switch block.
Generics record patterns in switch case label
Java 20 adds support for an inference of type arguments for generic record patterns in switch statements/expressions. As an example, consider the generic record:
record Triangle(S firstCoordinate, T secondCoordinate,V thirdCoordinate){};
In the following switch block, the inferred record pattern is
Triangle(var f, var s, var t):
static void getPt(Triangle tr){
switch (tr) {
case Triangle(var f, var s, var t) -> …;
case default -> …;
}
}
Error handling with MatchException
Java 19 introduces a new subclass of the java.lang.Runtime
class for a more uniform exception handling during pattern matching. The new class called java.lang.MatchException
is a preview API. The MatchException is not designed specifically for pattern matching in a switch but rather for any pattern-matching language construct. MatchException may be thrown at runtime when an exhaustive pattern matching does not match any of the provided patterns. To demonstrate this, consider the following application that includes a record pattern in a case label for a record that declares an accessor method with division by 0.
record DivisionByZero(int i) {
public int i() {
return i / 0;
}
}
public class SampleClass {
static DivisionByZero get(DivisionByZero r) {
return switch(r) {
case DivisionByZero(var i) -> r;
};
}
public static void main(String[] argv) {
get(new DivisionByZero(42));
}
}
The sample application compiles without an error but at runtime throws a MatchException
:
Exception in thread "main" java.lang.MatchException: java.lang.ArithmeticException: / by zero
at SampleClass.get(SampleClass.java:7)
at SampleClass.main(SampleClass.java:14)
Caused by: java.lang.ArithmeticException: / by zero
at DivisionByZero.i(SampleClass.java:1)
at SampleClass.get(SampleClass.java:1)
... 1 more
Conclusion
This article introduces the new pattern-matching support for the switch control-flow construct. The main improvements are that switch’s selector expression can be any reference type, and the switch’s case labels can include patterns, including conditional pattern matching. And, if you rather not update your complete codebase, pattern matching is supported with traditional switch statements and with traditional fall-through semantics.