Month: July 2023
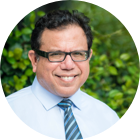
MMS • RSS
Posted on mongodb google news. Visit mongodb google news
DekaBank Deutsche Girozentrale grew its holdings in MongoDB, Inc. (NASDAQ:MDB – Free Report) by 23.4% in the 1st quarter, according to the company in its most recent Form 13F filing with the SEC. The institutional investor owned 13,654 shares of the company’s stock after acquiring an additional 2,591 shares during the quarter. DekaBank Deutsche Girozentrale’s holdings in MongoDB were worth $2,964,000 at the end of the most recent reporting period.
Other institutional investors have also added to or reduced their stakes in the company. Skandinaviska Enskilda Banken AB publ lifted its holdings in shares of MongoDB by 2.5% during the fourth quarter. Skandinaviska Enskilda Banken AB publ now owns 11,467 shares of the company’s stock worth $2,256,000 after purchasing an additional 277 shares during the period. Clarius Group LLC lifted its holdings in MongoDB by 7.7% in the first quarter. Clarius Group LLC now owns 1,362 shares of the company’s stock valued at $318,000 after acquiring an additional 97 shares during the period. Lindbrook Capital LLC lifted its holdings in MongoDB by 350.0% in the fourth quarter. Lindbrook Capital LLC now owns 171 shares of the company’s stock valued at $34,000 after acquiring an additional 133 shares during the period. Principal Financial Group Inc. lifted its holdings in MongoDB by 12.3% in the fourth quarter. Principal Financial Group Inc. now owns 8,452 shares of the company’s stock valued at $1,664,000 after acquiring an additional 924 shares during the period. Finally, Los Angeles Capital Management LLC lifted its holdings in MongoDB by 257.8% in the fourth quarter. Los Angeles Capital Management LLC now owns 14,085 shares of the company’s stock valued at $2,772,000 after acquiring an additional 10,148 shares during the period. Institutional investors and hedge funds own 89.22% of the company’s stock.
MongoDB Stock Performance
Shares of MongoDB stock opened at $398.68 on Friday. MongoDB, Inc. has a 52 week low of $135.15 and a 52 week high of $418.70. The business’s fifty day simple moving average is $346.49 and its 200-day simple moving average is $259.44. The firm has a market cap of $28.14 billion, a PE ratio of -85.37 and a beta of 1.13. The company has a quick ratio of 4.19, a current ratio of 4.19 and a debt-to-equity ratio of 1.44.
MongoDB (NASDAQ:MDB – Get Free Report) last announced its quarterly earnings results on Thursday, June 1st. The company reported $0.56 EPS for the quarter, beating the consensus estimate of $0.18 by $0.38. MongoDB had a negative return on equity of 43.25% and a negative net margin of 23.58%. The business had revenue of $368.28 million for the quarter, compared to analyst estimates of $347.77 million. During the same quarter in the prior year, the company posted ($1.15) EPS. The company’s quarterly revenue was up 29.0% on a year-over-year basis. Equities analysts forecast that MongoDB, Inc. will post -2.8 earnings per share for the current year.
Analyst Ratings Changes
A number of research analysts have issued reports on the company. Needham & Company LLC boosted their price target on MongoDB from $250.00 to $430.00 in a report on Friday, June 2nd. Guggenheim cut MongoDB from a “neutral” rating to a “sell” rating and boosted their price objective for the company from $205.00 to $210.00 in a research report on Thursday, May 25th. They noted that the move was a valuation call. Oppenheimer boosted their price objective on MongoDB from $270.00 to $430.00 in a research report on Friday, June 2nd. Barclays boosted their price objective on MongoDB from $374.00 to $421.00 in a research report on Monday, June 26th. Finally, VNET Group restated a “maintains” rating on shares of MongoDB in a research report on Monday, June 26th. One research analyst has rated the stock with a sell rating, three have given a hold rating and twenty have issued a buy rating to the company. Based on data from MarketBeat.com, MongoDB has an average rating of “Moderate Buy” and a consensus price target of $366.59.
Insider Activity
In related news, Director Dwight A. Merriman sold 2,000 shares of the stock in a transaction dated Wednesday, April 26th. The stock was sold at an average price of $240.00, for a total transaction of $480,000.00. Following the transaction, the director now owns 1,225,954 shares of the company’s stock, valued at approximately $294,228,960. The transaction was disclosed in a filing with the SEC, which is available through this hyperlink. In other MongoDB news, CTO Mark Porter sold 2,673 shares of the stock in a transaction dated Tuesday, May 9th. The stock was sold at an average price of $250.00, for a total value of $668,250.00. Following the sale, the chief technology officer now owns 40,336 shares of the company’s stock, valued at approximately $10,084,000. The transaction was disclosed in a filing with the Securities & Exchange Commission, which is available through the SEC website. Also, Director Dwight A. Merriman sold 2,000 shares of the stock in a transaction dated Wednesday, April 26th. The shares were sold at an average price of $240.00, for a total value of $480,000.00. Following the completion of the sale, the director now directly owns 1,225,954 shares in the company, valued at approximately $294,228,960. The disclosure for this sale can be found here. Insiders have sold a total of 117,427 shares of company stock valued at $41,364,961 in the last quarter. Insiders own 4.80% of the company’s stock.
MongoDB Company Profile
MongoDB, Inc provides general purpose database platform worldwide. The company offers MongoDB Atlas, a hosted multi-cloud database-as-a-service solution; MongoDB Enterprise Advanced, a commercial database server for enterprise customers to run in the cloud, on-premise, or in a hybrid environment; and Community Server, a free-to-download version of its database, which includes the functionality that developers need to get started with MongoDB.
Featured Articles
Receive News & Ratings for MongoDB Daily – Enter your email address below to receive a concise daily summary of the latest news and analysts’ ratings for MongoDB and related companies with MarketBeat.com’s FREE daily email newsletter.
Article originally posted on mongodb google news. Visit mongodb google news
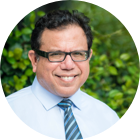
MMS • Renato Losio
Article originally posted on InfoQ. Visit InfoQ
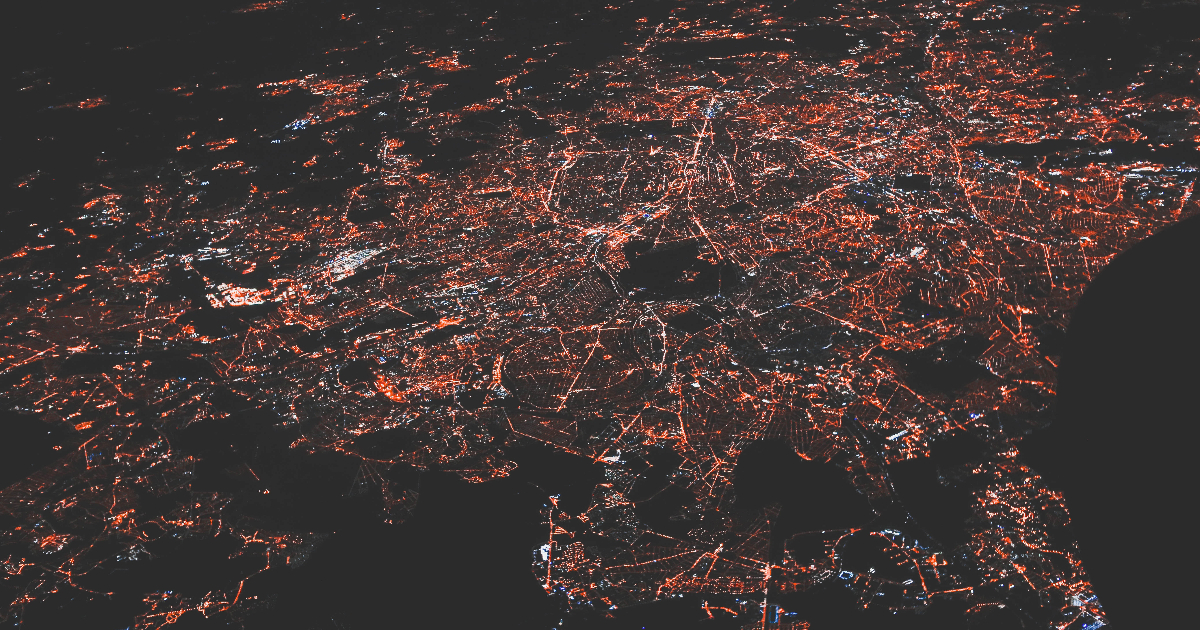
AWS has recently announced the general availability of the C7gn and the Hpc7g instances, both using the new Graviton3E processors. The C7gn instances are designed for network-intensive workloads while the Hpc7g instances are tailored for high-performance computing ones.
According to the cloud provider, the Graviton3E processors deliver higher memory bandwidth and compute performance than Graviton2 and up to 35% higher vector-instruction performance compared to Graviton3 processors. Leveraging ARM Neoverse technology, all of the new instances rely on the latest DDR5 memory and 5th generation AWS Nitro Cards, providing improved latency and 60% higher packet-per-second (PPS) performance.
Announced in preview last November, the C7gn instances are supposed to deliver the highest network bandwidth and be the best price-performance option for network-intensive workloads on AWS. Jeff Barr, chief evangelist at AWS, writes:
The instances are designed for your most demanding network-intensive workloads (firewalls, virtual routers, load balancers, and so forth), data analytics, and tightly-coupled cluster computing jobs. They are powered by AWS Graviton3E processors and support up to 200 Gbps of network bandwidth.
In the Last Week in AWS newsletter, Corey Quinn argues that very few technical details were shared:
This blog post is a good example of how AWS talks a lot about Graviton without saying very much about it. There’s no technical depth into what differentiates Graviton3 from Graviton 3E.
Starting with only 1 vCPU and 2GiB, the C7gn instances are currently available in 8 different instance sizes in only 4 regions: Ohio, Northern Virginia, Oregon, and Ireland.
On the same day, AWS announced the general availability of the Hpc7g, a new instance class for HPC workloads. The cloud provider published two separate articles on the advantages of the Hpc7g instances, the first one on improved performance, benchmarking popular HPC applications like Siemens Simcenter STAR-CCM+ and Ansys Fluent, and the second on the new sizing approach.
The Hpc7g is the first HPC instance to offer multiple sizes, specifically designed to enhance support for commercial software licensed on a per-core basis. Each instance size varies only in terms of the number of physical cores, while the cost remains consistent across all sizes. The instances feature 128 GiB of memory, 200 Gbps of network performance, and 20 Gbps of EBS performance. Karthik Raman, principal application engineer at AWS, explains:
These different sizes provide an easy way for customers to use the Amazon EC2 Optimize CPU options feature on the Hpc7g instances. This enables customers to choose from a range of instance sizes to target maximum performance per instance or maximum performance per core for their HPC workloads.
The Hpc7g instances are currently available only in Northern Virginia.
Some technical details about the different architectures of Graviton2, Graviton3, and Graviton3E are available in the Graviton Technical Guide on GitHub.
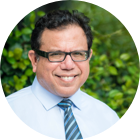
MMS • Mei-Chin Tsai Vinod Sridharan
Article originally posted on InfoQ. Visit InfoQ
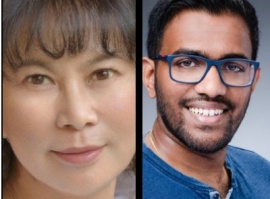
Transcript
Tsai: My name is Mei-Chin. I’m a Partner Director at Microsoft.
Sridharan: I’m Vinod. I’m a Principal Architect at Azure Cosmos DB.
Tsai: Today’s topic is Azure Cosmos DB: Low Latency and High Availability at Planet Scale. First of all, we will start with introduction and overview. Then Vinod will take us deep dive into the storage engine. I will be covering API gateway. We will conclude with learning and take away.
History of Azure Cosmos DB
First of all, let’s start with a little bit about Azure Cosmos DB’s history. Dharma Shukla is a Microsoft technical fellow. In 2010, he’d observed many internal Microsoft applications trying to build highly scalable and globally distributed systems. He started a project, he hoped to build a service that is cloud native, multi-tenant, and shared nothing from the ground up. In 2010, while Dharma Shukla is having a vacation in Florence, his proof of concept started to work. What is Azure Cosmos DB’s codename? Project Florence.
The product name was named as DocumentDB as we started with document API, by 2014 there are plenty sets of critical Azure services and Microsoft developers using Cosmos DB. With continuous evolution, DocumentDB no longer describe the capability of the product. Upon public offer in 2017, the product was renamed as Azure Cosmos DB to reflect the capability more than just document API. Also, to better describe aspiration for a scalability beyond a planet. In the initial offering, we only have document API such as document SQL and Mongo API. By 2018, both Gremlin API and Cassandra API were introduced.
What Does Azure Cosmos DB Offer?
Managing a single tenant fleet is hard, it is usually not cost efficient. To do sharding of any database is also a complex task. What does Azure Cosmos DB offer? These sets of features and characteristics are built into Azure Cosmos DB to support a journey from the box product to the cloud native. On the left-hand side, it is our core feature sets. Azure Cosmos DB is Microsoft’s schema free, NoSQL offering. Document SQL API is Azure Cosmos DB’s native API. Our query engine supports rich query semantics, such as sub-query, aggregation, join, and more, that make it unique and also complex. We also support multi-APIs. There is a huge base of existing Mongo, Cassandra developers and solutions. Providing these OSes APIs, smooths out the migration to Azure Cosmos DB. We will see multi-API choice impacts throughout our system, including storage engine, query, gateway, and more. We have tunable consistency, and conflict resolution. For scalability and performance features, we have geo distribution, active-active, and elasticity. On enterprise front, we are a Ring Zero Azure services. That means wherever there’s a new Azure region build-up happening, Azure Cosmos DB will be there. Encryption, network isolation, role-based access, are all needed to support enterprise software.
Azure Cosmos DB Usage
How is Azure Cosmos DB doing? Azure Cosmos DB is one of the fastest growing Azure services. We have 100% year-over-year growth in transaction and storage. How big can a customer become? I’m just citing one of the single instance customers, one of this instance customers actually supports over 100 million requests per second over petabytes of storage. This customer is globally distributed in 41 regions. Who uses Azure Cosmos DB? We power many first-party partners from Teams Chat, Xbox games, LinkedIn, Azure Active Directory, and more. We also power many critical external workloads, such as Adobe Creative Cloud, Walmart, GEICO, ASOS. All of our APIs have strong customers. We also observed many of our customers use several APIs, not just one, simply because depends on your data modeling, dataset, and your query. Customers tend to pick the right set of APIs to better support that scenario.
Azure Cosmos DB High Level Logical Flow
This diagram illustrates a high-level Azure Cosmos DB architecture. Customers creates a database account. The data is stored in the storage unit. Customer sends various requests from simple CRUD and query operations. What differentiates Azure Cosmos DB the most is actually our customer. On the left-hand side, you can see many of our customer accounts, but they can speak different languages. As you request a write to the write API gateway, request will be translated and planned. Sub-request will be routed to relevant backend storage. Result will be processed in the gateway if needed before sending it back. Azure Cosmos DB is designed with high performing multi-tenancy, that means a VM can contain many storage units, an API gateway process can support multiple customers’ requests. In this talk, you will see multi-API and high performing multi-tenancy plays a big part in our system.
Storage Engine
Sridharan: As we dive deep into the storage engine, I wanted to talk about a couple of themes you’ll see. In Cosmos DB, we believe highly in redundancy. If there’s one of something you should assume that it fails. If there’s more than one, assume that you have to prepare to have them swap out at a moment’s notice, and we will need redundancy at every layer.
Resource Hierarchy
I want to quickly cover some of the simple concepts in the Cosmos DB service. Cosmos DB has a tiered resource hierarchy. At a top level you have the database account, which is how customers interact with the database. Within an account, you can have several databases. A database is just a logical grouping of containers. Containers are like SQL tables. They hold user data and documents that are stored in physical partitions that are then horizontally scaled. Entities such as containers are provisioned with request units, which determine the throughput that you can derive from them. It’s like the currency that you use to interact with Cosmos DB. Each operation in the container ends up consuming these request units, and is restricted to be within the provision value for the container within any one second. Every container also comes with a logical partition key. The logical partition key is basically just a routing key that is used to route user data to a given physical partition where the data resides. Each logical partition key lives in one physical partition, but each physical partition stores many such logical partition keys. That’s pretty much how we ended up getting the horizontal scale we talked about. For instance, consider a container that has the logical partition key, city. Documents with the partition key value Seattle or London may end up in partition one, while Istanbul ends up in the physical partition three. We have several types of partitioning schemes in Cosmos DB. They can be a single field path, or hierarchical, or composite, where you could have multiple keys contributing to the partitioning strategy.
Each partitioning scheme is optimized for its own use scenarios. For instance, when you think about CRUD operations, a single partition key may be good enough. When you consider complex systems where you have data skew, for instance, where you have data skew but you still want to optimize for query usage patterns, hierarchical partition keys can still be useful. For instance, with Azure Active Directory where you have login tenants that are vastly different such as Microsoft or Adobe, but you still want horizontal scalability because a single tenant may be larger than what a physical partition can handle. You want data locality for these queries. You can have hierarchical partition keys where you first partition by the tenant, but also downstream by an organization or team, allowing you to benefit from the horizontal scalability but also data locality for fairly complex queries such as ORDER BYs, or CROSS JOINs across the different partitions.
The Cosmos DB Backend
The Cosmos DB backend is a multi-tenant shared nothing distributed service. Each region is divided into multiple logical fault-tolerant groups of servers that we call clusters. Every cluster contains multiple fault domain boundaries, and servers within the cluster are spread across these fault domains to ensure that the cluster as a whole can survive any failures in a subset of these fault domains. Within each cluster, customers’ physical partitions are spread across the different servers across all the different fault domains. A single partition for a given user comprises of four replicas that each hold copies of the entire data for that partition. We have four copies of your data for every partition. Different partitions even within a container are spread across various machines in a cluster. Across partitions, we divide them across multiple clusters, allowing us to get elastic and horizontal scale-up. Since we’re multi-tenant, every server hosts data from multiple accounts. By doing this, we ensure that we minimize the cost and get better density and packing of customer data per server. To do this, we need strong resource governance.
The Backend Replica
Let’s take a look at some of these replicas and partitions in detail. Each replica of a partition has a document store, which is written to a B-tree, an inverted index, which is an optimized B+ tree holding index terms used for queries, and a replication queue which logs writes as they happen, and is used to build and catch up slow replicas. Simple reads and writes are just served from the document store. We can always just look it up by ID and basically replace or read the value. However, queries that involve more than simple reads such as ORDER BYs, or GROUP BYs, or aggregates, or even sub-queries on any fields are processed in conjunction with the B-tree and the inverted index. Given that you can index pads, or wildcards, or just say, index everything, we end up building these indexes at runtime as you insert these documents. There is a brilliant paper some of our colleagues wrote on schema agnostic indexing and how we make it work that is linked here, https://www.vldb.org/pvldb/vol8/p1668-shukla.pdf.
Write a Single Document
Looking at a specific single write, if a user issues the write request to a given partition, the write is first directed to the primary replica of that partition. The primary replica first prepares a consistent snapshot of the message and dispatches that request to all of the secondaries concurrently. Each of the secondaries then commits that prepared message and updates its local state, which involves updating the B-tree, updating the inverted index, and the replication queue. The primary then waits for a quorum of secondaries to respond confirming that they have acknowledged and committed the write. This means that they’ve written it fully to disk. Once a quorum of secondaries act back, the primary then commits the write locally. It updates the replication queue, its local B-tree, and the inverted index before acknowledging the write to the client. If there’s any replicas that didn’t respond to the quorum write, then the replication queue is used to catch them up, in this case, the middle replica that didn’t act back.
Multi-Region Writes
That works great if you’re in a single region. Once again, if you have a single region, you only have one of something, and we like redundancy at Cosmos DB. We do offer having multiple regions with multi-homing. In the scenario where you have multiple regions, a client in region A will first write to the primary replica in region A. The primary then follows the same sequence as before and commits to its own secondaries. One of the secondaries is then elected to be a cross-region replication primary and replicates the write to region B’s primary replica. This then follows the same channel as before, and region B’s primary commits it to its own secondaries, and writes are transparently propagated across various regions. Conversely, if a user in region B writes to the primary in region B, then the inverse flow happens. First, the write is first committed to its primary and secondaries in region B. One of the secondaries there is elected as the cross-region replication primary, and writes to region A, which then follows the same sequence as before, ensuring that writes are made available transparently across all the different regions.
As both people are writing to both regions, one of the things that can happen is conflicts. Cosmos DB has several tunable conflict resolution policies to help resolve these conflicts. The user can configure, for instance, last writer wins on the timestamp of the document, or they can provide a custom field that defines how to resolve conflicts as some path within the document. The users can also configure a custom stored procedure that allows them to customize exactly how conflict resolution happens. Or they can have a manual feed and say I want to resolve these offline. Additionally, there’s also API specific policies such as Cassandra, which applies last writer wins on a timestamp but at a property level. All of these are configurable at an account or a collection basis.
Reads and Tunable Consistency
When it comes to reads, Cosmos DB has a set of five well-defined tunable consistencies that have been derived based off of industry research and user studies. These consistency levels are overridable on individual reads and allow for trading off between consistency, latency, availability, and throughput. On one side, you have strong, which gives you a globally consistent snapshot of your data. Next to that we have a regionally consistent consistency called bounded staleness, which about half of our requests today use. In this mode, users can also configure an upper bound on the staleness, ensuring that reads don’t lag behind writes by more than N seconds, or K writes across various regions. The next consistency we have is session, which provides read your own write guarantees within a client session. This gives users the best compromise between consistency and throughput. Finally, we have consistent prefix and eventual which gives you the best latency and throughput but the least guarantees on consistency. Of course, within a single region, the write behavior does not change with the consistency model. Writes are always durably committed to a quorum of replicas.
Let’s look at how some of these are achieved in detail. When a client issues a read that is eventual or session, these reads are randomly routed to any secondary replica. Across different reads, because of this random selection, reads are typically distributed across all the secondaries. In the case of a bounded staleness, or a strong read, the reads are pushed to two random secondaries. We also ensure that quorum is met across the two different secondaries. Given different request types, be it writes that go to the primary or reads that go to the secondaries, we basically load balance our workloads across all the replicas in our partition, making sure that we get better use of the replicas and provisioned throughput.
Ensuring High Availability
Coming to availability, across various components, we continually strive to ensure that we have high availability in all of our backend partitions. One of the things we do is load balancing. We constantly monitor and rebalance our replicas and partitions both within and across our clusters to ensure that any one server isn’t overloaded as customer workload patterns change over time. This load balancing is done in various metrics such as CPU or disk usage, or so on. Additionally, things always happen, like our machines go down, there’s upgrades, the power may go out on part of our data center, and we need to react to this. If a secondary replica goes down, because of any of these things, due to maintenance or upgrades or so on, new secondaries are automatically selected from the remaining servers and rebuilt from the current primary using the existing B-tree and replication queue. If a primary goes down, a new primary is automatically elected from the remaining replicas using Paxos. This new secondary is guaranteed to be chosen so that the latest acknowledged write is available. By doing this, we ensure that we have high availability within a partition, as long as there’s enough machines around even as the system evolves and changes.
Finally, we have strong admission control and resource governance. Requests are admission controlled on entry before any processing occurs based on their throughput requirements to ensure that any one customer doesn’t overload the machines, and there’s fair usage based on throughput guarantees. With simpler CRUD requests, this is relatively easy to do. Basically, when you have a write, I know the size of your write, and I can figure out the throughput requirements. For reads, similarly, once I look up the document, I know how much throughput you’re using. However, when you get to a complex query that has like a JOIN, or a GROUP, or you’re deep in the middle of an index or an ORDER BY, resource governance gets harder. Here we have checkpoints in the query VM runtime, where we report incremental progress of throughput, and we yield execution if you exhaust your budget. By doing this, we ensure that customers get the ability to make forward progress as they work through a query, but also respect resource governance and the bounds of a given replica or partition. If, however, the requests do exceed the budget allocated, say you have a large query and there’s not enough budget remaining within that replica to handle that query, they do get throttled on admission, with a delay in the future that allows the partition to operate under safe limits.
Elasticity – Provisioned Throughput
Beyond availability, customer’s data needs change over time. Traffic can be variable with follow the sun, or batch workloads, or spiky workloads, or analytical, and so on. Their stored data can also change over time. Cosmos DB handles this by offering various strategies to help with the elasticity of a service when data needs change. Customers can leverage various strategies like auto-scale, throughput sharing, serverless, or elastic scale out for their containers. The model on the left shows a simple provisioned throughput container. The container has a fixed guaranteed throughput of 30,000 RUs that is spread evenly across all the partitions. The container will always guarantee that 30,000 request units worth of work is available at any one second, and this is divided evenly across all of the different physical partitions. This is useful for scenarios where the workload is predictable, or constant, or well known a priori. On the right, we have an auto-scale container. Customers provide a range of throughput for the container, and each partition gets an equivalent proportion of it. In this case, the workloads can scale elastically and instantaneously between the minimum and maximum throughput specified, but the customer is only billed for the maximum actual throughput consumed, which is great for spiky or bursty workloads. To do this, we rely heavily on our load balancing mechanisms we discussed before, because we need to constantly monitor these servers and ensure that any replicas and partitions that get too hot get moved out if any one server gets oversubscribed.
Elastic Scaling
An additional case is if you need to grow your throughput or storage needs beyond what one physical server can handle. Say a user wants to scale their throughput from 30,000 to 60,000 request units, this may require a scale out operation. If it does, under the cover, the first thing we do is allocate new partitions that can handle the desired throughput. We then detect the optimal split point based on consumption or storage on the existing partitions, and then migrate the data from the old to the new based on the split point with no downtime to the user. New writes arriving to the partition are also copied over so the user doesn’t see any impact from this operation. Finally, when the data is caught up, there is an atomic swap between the old and new partitions, and the newly scaled partitions now take the user traffic. Note that these are all independent operations. Each partition once it’s caught up will automatically transition atomically to the new partitions. Similarly, let’s say that we detect that your storage is growing beyond what a single partition can handle, and it may be better to split. We do partition this the same way as a throughput split, where we create two child partitions, detect the split point, and migrate the data to the new child partitions, which can elastically grow horizontally. We did recently add the ability to merge partitions back as you reduce the throughput needs. While we didn’t need to do this earlier, we found out that this became important especially for scenarios around query where data locality is super important and fanning out to fewer partitions yielded better latencies and performance for the end customer. In this case, we once again follow the same flow where we provision a new partition, copy the data inbound, and then do the swap.
Multi-API Support
Finally, Cosmos DB is a service that supports multiple APIs, from SQL, Mongo, Gremlin, Cassandra, and Tables. For all of these APIs, we provide the same availability, reliability, and elasticity guarantees, while ensuring that API semantics are maintained. Consequently, we leverage a common shared infrastructure in the storage engine across all the APIs. The replication stack, the B-tree and the storage layer, the index and elasticity are all unified. This allows us to optimize these scenarios across all the APIs and ensure that features in this space benefit all APIs equally. We can see this because our active-active solution is available in Cassandra and SQL, and even Mongo, where we have an active-active Mongo API. However, each API can have its own requirements in terms of functionality. In these cases, we have extensibility points in the storage engine to support them. For instance, with patch semantics, or index term and query runtime semantics, where type coercions and type comparisons and such can vary vastly across APIs, or even conflict resolution behavior where resolving conflicts can have API specific behavior like we saw in Cassandra. By carefully orchestrating where and when these extensibility points happen, we can ensure that we give users the flexibility of the API surface while still guaranteeing availability, consistency, and elasticity.
API Gateway Problem Space
Tsai: That’s a very cool database engine. I always joke about it, Vinod got an easy problem. He got to charge our customers and API gateway does not. You had seen this diagram earlier, we are zooming a little bit into that middle box. As you can see, our API gateways have formed a fleet of microservices. Each microservice is specialized in one API. For each API, there are many microservices. Why is our API gateway problem space unique? Our API gateway is designed for multi-API. Today, we support Documents SQL, Mongo API, Cassandra API, and Gremlin API. Each API has its own semantics and protocols. Our gateway needs to be able to understand various protocol and also implement the correct semantics. Just picture key-value store, you probably can picture a simple query and request go over the wire. Think about graph. Graphs usually tend to deal with traverse, and super node as a very computationally heavy request. Our gateway is going up for multi-tenancy. Know that we do not charge our customer for these gateway services, everything here is COGs for us. Unlike the backend storage and CPU, we delegate to the customer as an RU. This gateway needs to be performing and fair, reducing the impact of noisy neighbor, and also contain the blast radius. This gateway needs to be highly available because that’s one of the Azure Cosmos DB [inaudible 00:28:24].
API Gateway Design Choices
This is our API gateway design choices. First, our gateway federation is separate from our backend federation. That gives us the flexibility in scaling independently from the backend for growing or shrinking. Second, to make multi-API implementation effective, we abstract our platform host from API specific interop. This enables us to quickly stand up another protocol or scenario if we desire to, and also allow us to optimize a platform layer once, and can benefit all scenarios. Platform layer including how we talk to the backend, knowing the partitions, and also talking about memory management. We need to be efficient, use the nodes to reduce COGs. Again, these are free services. However, we also need to balance between maximize use of a node and yet maintain high availability at peak traffic. A lot of heuristic and tuning has been put in. We implemented resource governance to reduce blast radius and to contain noisy neighbor. Our platform is deeply integrated with underlying hardware, such as NUMA node boundary conservation and core affinitization.
How We Leverage a VM
This diagram illustrates how we leverage a VM. Our processes are all small and fixed sized, as you can tell from this diagram. With a process per API, we can have better working set and localized locality, such as caching. We have multiple processes per API on a VM. Understanding the hardware that you’re deploying on, this is very important. We never span our process across NUMA node. NUMA stands for Non-Uniform Memory Access. When a process is across NUMA node, memory access latency can increase if you have cache misses. By not crossing the NUMA node makes our performance a lot more predictable. We also affinitize each gateway process to a small set of unique CPU cores. This will allow us be free from OSes from switching us between process, by sharing the cores, and also, between the API gateway process they won’t compete. Second, understand the language and framework that it depends on. Our API gateway is implemented in .NET Core. We manage the process for performance. We put in consideration for potential latency such as GC configuration, throughput heuristic. We also have been leveraging low allocation API and buffer pooling technique to reduce the GC overhead. We are very fortunate that we work closely with .NET team. We are a .NET early adopter. We provide performance and quality feedback to .NET team.
Load Balancing at all Levels
Load balancing is a difficult problem, you want to do it well. Vinod mentioned about load balancing in our backend. The same thing is happening in the frontend. With our API gateway design, load balancing happens in all different levels. Within a VM, there are connection rerouting to balance between process of the same API. Between VMs, account load balancing can happen by monitoring system if a VM is getting too hot in CPU or memory. Account load balancing can happen within a cluster or between clusters. These are passive load balancing, because you load balance when a VM may be out of a comfort zone. What we are working on is active load balancing, where we load balance upon request arrival. We are building a layer 7 customized load balancer. This is a work in progress. It will leverage VM health not just VM’s liveness. It will have capability to leverage historical insight in ML for future prediction. All this load balancing is done in a non-observable way to a customer, and maintain the high availability.
Tuning the System for Performance
Sridharan: Performance is another critical component to manage as the code base evolves. It’s one of the first things to go if you’re not paying close attention to it. Cosmos DB applies multiple approaches to ensure that we retain and improve performance over time. Firstly, for every component, we have a rigorous validation pipeline that monitors the current throughput and latency continuously. We make sure that we never regress this performance, and every change that goes into the product has to meet or exceed this performance bar. I learned this the hard way when I first joined the team. Even a 1% regression in latency or throughput is considered unacceptable. That sometimes means that as changes happen, developers must reclaim performance in other components so that the overall performance bar is met. Within the storage engine, we have a number of things we do to ensure that we have the performance guarantees needed for the service. The first is our inverted index, which uses a lock-free B+ tree optimized for indexing, which was written in collaboration with Microsoft Research. This gives us significant benefits over a regular B-tree when doing multiple batched updates across different pages, which is pretty common in indexes. We also optimize our content serialization format in our storage with a binary format that is length prefixed, so that projections as you’re looking at a deeply nested document is highly efficient for queries, and we can read and write properties super efficiently. We also ensure that we use local disks with SSDs instead of remote disks to avoid the network latency when committing changes. Instead, we use the fact that we have four such replicas to achieve high availability and durability. We also have custom allocators to optimize for various request patterns, for instance, a linear allocator for a query, or we have a custom async scheduler with coroutines that ensures that we can optimize for concurrency and resource governance all through the stack.
Within the API gateway, the optimizations tend to be about managing stateless scale out scenarios that focus on proxying data. We usually use low allocation .NET APIs such as span and memory, and minimize the transforms parsing or any deserialization needed all to reduce variance in latency and garbage collections. Additionally, to ensure predictability in memory access patterns, we have a hard NUMA affinity within our processes. We also use fixed size processes across all of our APIs, so that we can optimize for one process size when it comes to core performance characteristics across the entire fleet that we have. Within Azure, our VMs in our cluster are placed within a single proximity placement group, which allows us to provide guarantees around internode latencies, especially when we’re doing things like load balancing. Finally, we partner closely with the .NET team to ensure that we continue to benefit from the latest high-performance APIs that we can leverage in our software stack.
Takeaways from Building Azure Cosmos DB
Tsai: These are our takeaways from building Azure Cosmos DB. Reliability and performance are features, you must design it in on day one, if not day zero. The architecture had to respect that and everything that you put in had to respect that. Reliability and performance is a team culture. As Vinod mentioned, it could be test by 1000 paper cuts, 1% of a performance regression 20 of those will be 20% of regression. It is very important that continuous monitoring and continuously holding that bar, you must allocate the time to continuously improve your system, even when you think that you are done with the features. Third, leverage customer insight. This is also super important. With all the data in your hand, how do you decide what feature to build, or that a customer can benefit, or what optimizations you should optimize, to prioritize so that most customers can benefit from it. One other good example that we have is actually the recent optimization of query engine. Even though we’ve implemented Mongo API, we know Mongo supports nested arrays, we have so many of our customers who don’t even have nested arrays. Our query engine implements optimization that a single code pass is actually much more performing, and a lot of customers benefit from it. Last, stay ahead of the business growth and trend. Nobody builds services on day one, and power billions of requests, and petabytes of data. You continuously monitor to see if you’re hitting a bottleneck, you need to work on removing that bottleneck. Sometimes you might need to reimplement if your initial design does not support that scalability. Training is also really important to know, what is upcoming, and how do you prioritize those?
Questions and Answers
Anand: Could you expand on how you verify that you don’t have performance regressions?
Sridharan: There’s a number of things that we look at. The primary metrics we’re looking for are throughput, latency, and CPU. We want to make sure that for our critical workloads, at the very least, throughput, latency, and CPU are constant and do not degrade over time. We basically take a single node, we load it up to 90% CPU, for, say, a single document read, a single document write, a fairly well-known query, and so on, and make sure that the throughput that we derive from a node, the latency that we see at 90% CPU, the memory that we see are all meeting the benchmark that we want. Then we basically add specifically well-known workloads over time as we see scenarios that derive from production. We also have observability in our production system where we monitor specific queries, signatures, and so on, and make sure that we don’t regress that as we do deployments and such.
Tsai: I think, in summary, essentially is some layers of guardrails. There’s a first-line guardrail, more like a benchmark unit testing, and then the workload, like simulated workload, and then real workload. Then you will hope that you catch it earlier, because those are the places that you can catch it with more of a precise measurement. Then when you catch low, and further out, then it’s just more like, I’m seeing something that is not what I expected.
Anand: I don’t know if this person was asking in just a standalone question. I don’t think it’s related to the replication thing. Just query performance, I think. What I understood is, you run your own benchmarks based on your query log, a representative query log, is that correct?
Sridharan: Are you talking about for the performance regression stuff?
Anand: Yes.
Sridharan: For variable workloads, yes, there’s a degree of variability, which is why our performance runs similar to what you do with like, YCSB or any of the major benchmarks, so-to-speak. We have a well-known set of queries that we execute to measure the performance to ensure that we meet the bar for latency, CPU, and throughput. A 1% regression is literally just any regression in either latency or throughput core CPU for any of these well-known workloads.
Anand: You have a graph workload, you could have an analytic workload, you can have an OLTP workload, you can have a document retrieval workload, you have a document database, you can have a key-value workload. It’s a little complicated.
Tsai: Now you know how painful it is when people say why don’t you adopt a new release of the operating system, new hardware, all the things that matter that will go in. I think the question there about, like 1%, 1% is actually a very interesting question. Because sometimes your performance environment, 1%, the 1% could be a noise, and we don’t know. We observe on per change, and we also observe the trend. Sometimes we can catch a larger regression immediately. That test by 1000 paper cuts, you hope to catch it after 20 paper cuts, not 1000. That’s how we operate.
Sridharan: We do use known benchmarks like YCSB, but we also have our own benchmarks that we have built on top of that, especially like he said, like when you’re dealing with a graph workload, figuring out the sets of relationships we want to test is also a crucial part of it. For instance, friends of friends is a common query that most people want to ask for graphs, or third hop, or whatever. We tend to build our performance benchmarks around that.
Anand: YCSB is mostly for NoSQL, correct?
Sridharan: Yes. A lot of our APIs are NoSQL, like between Mongo, or Cassandra, or documents API. We’ve extended some of that for our graph database as well.
Anand: What about traditional RDBMS workloads? Do you guys support those? Do you have a storage engine that is like a RDBMS type of engine, like InnoDB, or something like this?
Sridharan: We just announced the Azure Cosmos DB for Postgres. At least, this particular engine that we’re talking about in this talk is geared towards NoSQL, primarily.
Anand: Stay ahead of business growth and trend, what are current research areas being explored related to current business trends in enterprise?
Tsai: I think there’s two observations in a big organization that we observe. One is actually more of industrial training. There’s SQL, there’s NoSQL. Actually, it’s two spectrums of things. We start observing the new trend, more like a distributed SQL, trying to bring NoSQL closer to SQL space, or in light of the SQL capability. The second trend that we observe is actually, we call it SaaS, Software as a Service. Actually, a lot of developers would like to write very little code, and so chaining things together as experience and actually enabling developer’s fast productivity is super key.
Sridharan: I think there’s one aspect, like you said, the distributed SQL and trying to merge what we think of as traditional relational with NoSQL is a direction we’ve been headed towards for the last at least decade. We’re pushing further on, almost getting all three of the consistency, availability, and partitioning, with caveats, and everyone picks their own. That is one area for sure. I think the other part is, how do we get lower and lower latency? We keep talking about people building complex systems with caching on top of databases, because the database takes too long to run your query, and you need to have Redis on top of your database. Or if you have like Text Search, you build some other caching layer or another key-value store you shove the results in? How do we integrate these to provide more of a low latency experience for people who really need the high performance and low latency, but at the same time, give you the flexibility of the query? I think is another area that is currently being explored.
Anand: There’s some knowledge or talk about HTAP, Hybrid Transactional and Analytic Processing. Is that where you’d like to go, with these other things, graph and NoSQL combined in that? There were papers like Tenzing, Google published a while ago, which was this hierarchical storage engines with one API. Those were mostly for analytic processing. It was a paper maybe 10-plus years ago.
Sridharan: HTAP is definitely something we see us looking at, if you look at, for instance, things like the Synapse link where you can ingest with OLTP and then run analytical workloads on top. I was just trying to formulate the answer on how I see the staying ahead parts, and how I can talk about it. At the very least, I know that that is a direction we are exploring as well.
Tsai: The analytic query or information, some are not needed in real time, some are actually needed in real time, and differentiate those and provide different solutions for those, certainly could be a strategy tackling that particular space. We just need to figure out what is in scope with the customer as product as itself. What’s in scope as a solution, end-to-end, because a lot of times people do not just use a product itself. When our customers architect for their problem space, you see many components are chained together. It is about, how do you leverage those, and solve the problem with the best outcome that you will hope for?
See more presentations with transcripts
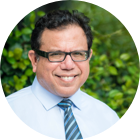
MMS • Natalie DellaMaria Hector Veiga Ortiz
Article originally posted on InfoQ. Visit InfoQ
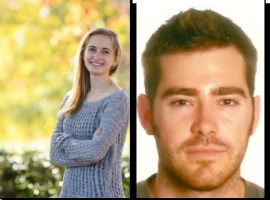
Transcript
DellaMaria: Recent extreme weather events such as Hurricane Ian, a category 4 hurricane, and the heat waves of California that broke hundreds of records, result in devastating power outages that lead to economic losses and harm to human well-being. In the case of Hurricane Ian, we have damaged the physical infrastructure that inhibits the capability of the grid to generate and transmit electricity. In the case of the California heat wave, we have excess demand from residents, businesses, and critical operations that require additional electricity to run cooling systems, air conditioning, and other operations to keep people safe and operations running smoothly. This causes stress on the grid. Since the grid can’t store energy, it means this electricity has to be provided in real time to meet these loads. What this looks like for grid operators is this graph right here. This graph could represent a day of the heat wave for the California grid operator. The blue line represents the grid capacity and the orange line represents forecasted demand. We start to get into issues when the graph looks like this. This has a forecasted demand surpassing the capacity of the grid. This is when grid operators start to initiate rolling blackouts as was the case with the latest California heat wave. Some of you that live in California might even remember receiving daily statewide alerts, urging you to reduce your energy consumption because we were on a brink of these blackouts. The reasons that grid operators do this is because they need to distribute and try to balance the available load and minimize impact to consumers, but, unfortunately, leads to these rotating blackouts. We can see with these recent weather events, the importance of having independent energy, and energy security. We’re going to talk about how Tesla utilizes distributed energy resources to ensure our customers individually and communitively have energy security.
Overview
I’m Natalie DellaMaria.
Ortiz: I am Hector Veiga. What we’re going to go through is that we’re going to explain the benefits of having residential batteries, and how we can extend our utilities using software. Then we’re going to explain how the Tesla Energy platform can increase energy security for a single home. Then, how we can use the same platform to orchestrate fleets of batteries to increase energy security for entire communities. Finally, we will show how to have an integrated user experience where we can give customers control during these critical times.
Why Batteries?
Why Batteries? Traditional power generation has come from burning fossil fuels. That is a source of energy that is reliable and consistent. Renewable sources such as solar or wind are more inconsistent and variable. You may get a lot of sunlight and wind and generate energy when it’s not really needed, or you don’t have enough, when it’s needed the most. That’s where it varies, and software really come in. When we couple batteries and renewable energy, we can store the excess energy in those batteries to be used later and reduce the need to burn fossil fuels. A good example is the Kapolei Energy Storage facility in Hawaii. This energy installation is capable of storing the amount of energy that can power 700,000 homes for an hour. This is also impacting the island by not having the need to have peaking power plants. Peaking power plants or peaker plants, commonly known, are power plants that only run when there is a high need of energy demand, normally burning fossil fuels and due to this irregular use of these plants, the energy generated from them is actually very expensive. Residential batteries are also great. They help our customers to control their solar energy generation. They provide backup in case of power outages. Where they really shine is when you couple them with software.
Why Software?
DellaMaria: Then, why software? We could run software on the edge on devices that utilize information local to the device such as solar production, or historical home load. We also run software on the cloud to relay other information to enable this autonomous decision making on the device. We can also utilize software to create integrated user experiences across products and things such as the mobile app. We can also utilize cloud software to aggregate fleets of individual devices, to expand the impact from an individual battery impact to community impact in what’s known as a virtual power plant. We can think of simple examples of how do we use software to create good customer experiences with their batteries. This is an example of a customer updating their backup reserve, and this is the value that the battery will store in case of a grid outage. There’s times when we want more complex features. For most of our customers, they want to keep a low backup reserve most of the time. This enables them to have capacity to store their own solar. However, when there’s a high likelihood of a grid outage, they’d like to be able to adjust their backup reserve to full, set their battery charge to full and they have a full battery in case the grid goes out. Storms are the leading cause of grid outages, and no one likes to manually watch the weather channel waiting to update their backup reserve. Thankfully, Tesla will do it for you. When you enable StormWatch on your device, it will preemptively charge your battery to full ahead of inclement weather. This way you can leave your backup reserve value low and you don’t have to worry about not having enough power in your battery to charge you when the grid goes out.
The Tesla Energy Platform
Ortiz: First, let’s speak about our platform and how it powers programs like StormWatch. Tesla devices such as batteries are connected to the cloud using secure WebSockets. The connection is bidirectional. From the edge to the cloud, devices can report their alerts and their measurements to inform about their current state. The set of services in the platform that receive, handle, store, and provide these data points are under telemetry domain. Then, we need to give context to this data. That’s where asset services come in. Asset services basically keep the relationships between devices, customers, and installations. Having accurate asset data is critical to power programs such as StormWatch or the virtual power plant. Finally, from the cloud to the edge, we have another set of services that can command and control those devices. These actions that we can send to devices can be things similar to firmware updates, configuration changes, or device mode transitions, which are like charge the battery or discharge the battery. Messages exchanged between the cloud and the edge are formatted using Google protocol buffers. Google protocol buffers or protobuf are a language agnostic format. They’re strongly typed, performant. They allow for schema evolution as long as you follow a few simple restrictions. Since they are contract based, what we have is a shared repository where we keep those protobuf files, and both edge and cloud developers can propose additions and changes.
The three domains in the platform are actually formed by thousands of services, but the bulk of them are written using Akka and Scala. Akka is a toolkit based on the actor model written in Scala. Scala is a functional programming language that runs on the Java Virtual Machine. We use Akka in many aspects of our development and operations. For example, when we need to scale up our applications, we rely on the Akka cluster and Akka cluster sharding modules. It also helps with our stateful applications, which we have a few, through the Akka persistence module where it helps you save the state and restart it when needed. We heavily utilize also their streaming tools, Akka Streams and Alpakka, because they provide built-in backpressure, backoff retry capabilities, and external connectors to reliably interact with other technologies. The Akka ecosystem has proven to be performant and reliable enough to meet our needs.
Let’s talk about a scenario that we need to deal with where we use some of these technologies. Normally, our devices are connected to the internet when they report their data. Sometimes there’s some internet connectivity outage due to an internet service provider problem or a Wi-Fi spotty connection. In those cases, the devices keep collecting measurements, and they basically buffer them locally. When the connection is restored, then they send all the data to our servers, generating more load than expected. Then, to handle this scenario, we use a combination of tools. We use Apache Kafka to store this data temporarily in a distributed topic. Then we rely on the Alpakka Kafka connector to consume this data. If we’re using the Akka Streams built-in backpressure, the consuming services do not consume all this data immediately, but they do their best to consume as fast as possible. However, that results in incrementing lag on our topic. To actually speed up this process, we actually use Kubernetes Horizontal Pod Autoscalers or HPAs. Where an HPA monitors a metric, in this case lag, and if it approaches a predefined threshold, it creates more pods to help decrease the lag as soon as possible. Once we have consumed all the lag, the HPAs get rid of the unnecessary pods and we go back to normal operation.
The services in the platform are exposed through a set of standard APIs. We have two flavors. We do expose services using REST, the common REST architecture using HTTP/1.1, but we have heavily invested in gRPC, which in our experience offers some advantages over the traditional REST architecture. The main one is that service and messages are contract based, are defined by protobuf. That makes sure that whatever messages your request and your response are is what you’re expecting. Also, thanks to this contract-based solution, there are tools to generate code for both client and server that helps us move faster, and don’t repeat ourselves by wasting time implementing that code. Also, since we run gRPC over HTTP/2, HTTP/2 has a better use of connections because it implements streaming and multiplexing. It is through these APIs that the platform allows other systems to provide higher order functions, such as metric dashboards. It powers UIs like the Tesla mobile app, or the programs that run on top of the platform, like StormWatch or the virtual power plant.
DellaMaria: Sometimes our platform services need to interact with third parties, as is the case with StormWatch. We rely on third parties to provide us with weather alerts that let us know which areas will be affected by inclement weather. These areas are designated either by a list of regions or by polygons that can consist of hundreds of latitude and longitudinal points. We’ve abstracted away the processing of these incoming alerts to an internal service we call Weather API. Having this internal layer decouples the rest of our platform services from our external data providers. This means our data providers can be updated, changed, added, or moved, and we have the flexibility to do all that without affecting our clients in our platform services. They maintain consistent data integration through our Weather API. Another key functionality of the Weather API is transforming these various forms of geographic references into a standard format. We use the WKT or Well-Known Text format, which is just a string representation of a geospatial object. What this might look like is this, for the hurricane warning that we saw earlier. This is an example of a WKT polygon. From the context of our StormWatch service, we are constantly pulling Weather API for these active alerts.
StormWatch Architecture
I’m going to talk a little bit about the architecture of our StormWatch service. It’s running on multiple pods in Kubernetes that form a single service. We model individual customer batteries as actors. Actors are objects that contain some state and will communicate with asynchronous message parsing. These battery installation actors are distributed across the pods in our clustered application. We utilize the Akka framework here to support our actor system. It abstracts away the need for us to create and handle our own locks in order to prevent concurrency and race conditions when reading and writing to the state of these distributed actors. It also supports location transparency. This is the concept that the sender of a message to one of these individual entities doesn’t need to know the physical location of that actor or what pod it is running on. This is really helpful.
The way we pull for weather alerts is with actually a singleton actor since there’s only one running on all of the pods, and only single pod in the cluster. This is because we really only need to get the alerts once and then can then distribute them amongst the affected actors. It’s really important that our actors can handle alert, duplications, updates, creations, and cancellations. This requires individual battery actors to maintain some state. Again, this is very important because there’s downstream impacts in the real world such as notifications, and then battery control plans. Going back to our Hurricane Ian story, we’ve detected an alert that’s been picked up in our Weather API. It’s constructed a standardized format for any of our services to consume. Our StormWatch service then picks it up in its next pulling cycle. The next step is how to determine which batteries are actually going to be affected by this weather alert. To do that we utilize optimized geosearch queries in our asset service. Our asset service is backed by a Postgres database and uses PostGIS which is a geospatial database extender.
Here we have this incoming alert, and we’re going to focus on the hurricane warning. This red polygon right here. This is the WKT polygon that we saw earlier. It’s pretty complex. Our initial approach was to use this direct WKT polygon, now that’s pretty complex, and do a direct intersection query in our asset database. However, these queries were taking a very long time. They were taking resources away from our concurrent queries, so this query was getting canceled, due to the replication routine happening in the database. Instead of utilizing this complex polygon, we instead decided to use an approximate polygon. This is the 2D bounding box surrounding the complex polygon that we saw earlier. This was much quicker. PostGIS works really well with rectangular polygons and uses index only scans. It means it doesn’t have to go to the buffer cache or to disk to retrieve the index, it’s right there in memory. This turned the installation batteries much quicker.
However, we now faced an issue where we might have some false positives. We might have some sites that fall within our approximate query that don’t fall in with our original direct complex polygon, sites like this one. We implemented a process, client side, that would iterate through these installation candidates that came back from our approximate query. We then filter out the ones that don’t fall into the complex query. You might be wondering, why is it faster to doing this two-step process? We’re getting the same result. These same batteries are being returned in this two-step process that would have been returned had we done all this in our database with the original complex direct query. That’s because this final process to iterate through each installation battery and determine if it intersects with a complex query, takes a lot of CPU. When that CPU is being used in our database node, it’s taking CPU away from concurrent queries that were coming from the rest of our platform services. We moved that to a stateless process. We can then scale this stateless process horizontally or per pod. That’s much easier than having to continuously upsize our database, which is why we now have this multi-step process.
Now that we’ve resolved the installations that are actually affected by this incoming alert, we can then message them and let them know, so then they can make independent control decisions based off each installation’s system capabilities, and customer preferences. Now that we fanned out, each of these actors is processing in parallel using streaming, as that means that one slow site is not going to affect another one’s site dispatch. We use this system to ensure that our customers can have backup support when they need it the most. This is an example of the mobile app during an active storm. We can see the battery is charging from the grid, and StormWatch is active, notifying our users why their battery is behaving this way.
Takeaways (StormWatch Architecture)
Some key takeaways here is, if you can abstract interfaces with external data providers to an internal service, then this decouples the rest of your platform services from external providers and prevents data provider lock-in, or being limited to your external data provider’s interface. Geosearches and other queries can be expensive. If you can switch to a multi-step approach and move out CPU intensive tasks to a stateless service, it’s much more scalable. In-order message processing in a clustered environment or distributed environments, can take extra considerations. We utilize the actor system to enable us to decide when we want to have parallel processing, as is the case with each of our individual battery actors processing per site capabilities, and when we want to handle things only once per cluster, and in a serialized format, as we do with our weather pulling.
The Hierarchies Approach
Ortiz: StormWatch has proven to increase individual energy security for thousands of customers, but we’re going to strive for more. We know there is local value in having a residential battery, but how can we take that local value and provide energy security for entire communities? Power outages caused by grid stress, like it happened during the California heat wave are a bit different than the ones that are happening due to damage to physical infrastructure, like it happened during Hurricane Ian. They are basically a deficit in the amount of available energy. If we can provide an interface for customers that have the battery systems that can store energy and utilities who need the support, then we can basically let customers opt in and support the grid. Tesla is in a unique position to provide this value. The platform that controls the batteries is already in place, and we can send control plans to them. Tesla is the actual critical link between the utilities that need the support and the customers who can provide this value. When we see a graph like this, we are all in trouble. There might be blackouts and those can be problematic. The first thing that we need to do to help the grid is identify who can help the grid. We do that by allowing eligible customers to join emergency grid support programs. We do that through the mobile app, which is an app that they already own. That thing is, in our opinion, novel. We know that utilities are normally not moving as fast as technology companies do. In these cases, it is critical that we move together fast to be able to support the grid. Also, for customers that are participating in these events, they are getting some compensation for it.
Our software builds upon the same foundational Tesla Energy platform that we were using for the StormWatch feature. One critical component for our virtual power plants programs is to basically identify and group different installations based on their utility instead of their geographic region. We have an available tool in our platform to do that, and it’s called hierarchies. A hierarchy is a logical way to group installation based on a common characteristic or feature. You can think about it as a way to tag a group of installations. These installations or these groups are identified by a single identifier, and it is through this identifier in our platform where you can resolve which installations belong to that particular group. The hierarchies tool also allow you to aggregate groups into a larger group, creating a multi-layer tree-like schema. Hierarchies also bring fine-grained security into picture because asset services can provide or deny access based on the group identifier. Asset services rely on Postgres to store all the asset data, but in this particular case, they use the ltree extension instead of the PostGIS extension. The ltree extension is an extension for Postgres that allows you to manage and query efficiently a tree-like structure. Let’s see an example.
We can create a really simple table that we will call a hierarchy, with only one column, that will be called path of the type, ltree. Then we can insert some sample values. Here in the sample values, we can see that the ltree extension uses the (.) as the connector between nodes in the tree. How can we actually get children of a given node in this tree? The ltree extension provides a new operator, the .
In the context of our VPP programs, our enrollment flow also relies on the hierarchies table, where each group represents a state in the enrollment flow, from eligible, to pending, to enrolled. All these transitions are actually recorded by making a request to asset services. Also, if a customer decides to no longer participate in these programs, then the enrollment flow will make the appropriate request to asset services to remove their installations from those groups. You might be wondering why we decided to run this enrollment flow with hierarchies instead of a simpler tagging solution. Hierarchies was an already available solution in our platform and the California VPP program had to be developed in a very short period of time, so we were able to reuse a solution to this particular case. Also, the enrollment flow now can be reused for future programs. Having these well-defined segregated groups also helps us reach out to customers depending on their needs. For example, this is a notification a customer will receive if they are in the eligible group and they have not yet enrolled, or a notification that a customer that has enrolled will receive if there’s a scheduled event in the near future.
Thanks to this multi-layer hierarchy approach, we can identify what are all the installations under a whole program by creating a group higher in the hierarchy that contains all the enrollment groups under it. Now that we have identified who can help the grid, then we can use the same digital twin architecture that we were using for StormWatch to track event changes and customer participation changes. In this case, instead of getting events from weather providers, we get events from our relationship with the grid operators. Once we get an event, we basically resolve which installations can help support the grid and dispatch control plans to them. In this case, the control plans has two phases. The first phase is to charge the battery as much as you can during off-peak hours. The second phase is to discharge during the event until the duration of the event, or until the battery hits its backup percent limit. When we combine our hierarchies tool with our telemetry pipelines, we can get aggregated energy data for any given group. In the context of our VPPs, this can show what is the total contribution that a particular group is discharging into the grid. In this particular view that our customer that has enrolled and is actually participating on one of these events, the customer will be able to see their contribution, which is like 3.1 kilowatts, but what is the total contribution, highlighting that they are part of a larger community impact.
During the California heat wave event, there were multiple dispatching events. We were able to peak 33 megawatts, which is the same power of a small peaker plant. This was thanks to over 4500 customers who enrolled in this Tesla emergency grid support programs that allow Tesla and utilities to preemptively charge their batteries and discharge them during times of high demand. These customers were compensated $2 per kilowatt hour discharged to the grid. Tesla VPP programs created the largest distributed battery in the world to help keep California’s energy clean and reliable. Providing an engaging user experience where customers have the agency to feel that they are part of something larger than the installation.
Takeaways (The Hierarchies Approach)
Some takeaways here. Hierarchies as a multi-level tagging and security tool that allows you to identify quickly entities through the ltree extension. Reusability. We’re big fans of reusing components, and make them generic so we can move and deliver faster. Short-notice trigger events requires you to do as much preprocessing as possible to ensure a successful outcome, in our case, understanding who can participate in these programs. Dynamic programs like virtual power plant actually need reactive processes and applications that need to provide value at any given moment and handle any adverse scenarios such as devices becoming offline, or customers enrolling or unenrolling at any given moment.
Recap – Tesla VPPs and StormWatch Features
DellaMaria: The Tesla Virtual Power Plants and StormWatch features are two critical user facing applications that from a powerful perspective are complete opposites, but from a software perspective are actually quite similar. Let’s recap how both these features work. With both features, we need to detect discrete events. We need to resolve which homes are affected. We need to run arbitration and decision making to determine if these batteries are capable and should participate in the event. We need to notify our customers. We need to actually communicate to the battery their respective control plans. We can already see the duality in these two features, basically two sides of the same coin, and behind powering both these feature experiences is the same application. This enables our customers to both enroll in our virtual power plant programs, at the same time, they can enable the StormWatch feature to ensure they have the independent energy security. Having this reuse of this same architecture allows us to save reimplementation of common logic and common integrations. It also provides a clean space for when we need to do event arbitration. When we do have conflicting StormWatch events with our virtual power plant events, we can utilize the fact that these customer battery actors already need to store some state to support each independent feature. They need to be able to handle future alert updates, cancellations, duplications. All that’s required to support conflicting events is to add minimal logic into these existing actor entities to then respect customer priorities. All I have to do is add a simple experience to the mobile app to give our customers control and agency over exactly how they want their battery to be used in such scenarios.
It’s a very dynamic and a very bursty system. When we have events going on, it’s very active. There’s a lot of messaging thing happening between the mobile app and external events. However, it’s relatively calm at all other times. This is a system that you can’t shed load. When it’s very active and we have a lot of load, is when it’s very critical. This is when our customers need us the most. Ways we handle this is utilizing reactive streaming concepts throughout the system, and with how we interact with our downstream services. This includes backoff retries, backpressure, and throttling. We also utilize parallelization between the actors. Once we fan out to each individual battery actor entity, those processes and streaming is happening in parallel. This way, one offline device is not going to slow down or stop another customer’s experience. Additionally, we made sure that this application was horizontally scalable. These actors are persistent and cluster sharded. That means they will store their state in a persistence datastore that we use, backed by the Akka framework. Then when we spin up new pods to handle and distribute load, these actor entities could then move over to that new pod and use those new resources. This allows us to scale to meet our growing user base.
Another key thing to notice is that these battery installation actors also support the mobile app experience. These digital twins that really represent the physical batteries of an owner, they can then see the state of them right there in the mobile app. As they make changes, they can opt in and out of individual events, programs as a whole, and update that priority, and that will get sent down to their battery and then reflected back in the mobile app, showing them the state of their system in real time. Then this, again, enables them to have unique control over how they want their battery to be used at any time, enabling them for their own use cases, like this customer was able to opt out of an individual storm event because he already had full capacity based off his excess solar at his own home.
Takeaways
Some takeaways here is, design upfront for horizontal scalability. We took the time initially to implement persistent cluster sharded actors. This gives us the freedom to know that we can just upscale our application horizontally when we need to. Reactive streaming concepts are very critical when you have dynamic and bursty systems that can’t shed load, so that backoff retries, throttling, backpressure are very critical. Give control to your customers through great mobile app experiences or other integrated user experiences. This allows you to have simpler logic in your own backend systems. Don’t be afraid to use toolkits to help in building these distributed systems. We are supported by Akka, but you can also implement similar things in Erlang/OTP, and other functional programming toolkits.
Why Cloud?
Ortiz: With the increasing capabilities of the edge devices where those keep having more memory and more CPU these days, some of you might wonder why we’re running this decision making and arbitration in the cloud instead of in the devices themselves. There are actually pros and cons for both approaches. Since what we’re interested in is in actually moving faster, we can iterate faster on the cloud than at the edge. That helps us create new features quickly and mature these existing programs quicker. In addition, we have the luxury to have vertical integration when we manage both the cloud software and the edge software. We can selectively send down pieces of our logic to our edge, where we believe those could make a great impact and help expand our programs and features.
Looking Forward
What is next? Traditionally, our customers have thought about batteries or residential batteries, as a way to only increase individual energy security. With this new virtual power plant programs, that is a paradigm still unknown to many, this expands these possibilities. As these things are becoming more popular, the interest is actually growing. Is this the way to think about batteries in the future? We don’t believe so. We think it is the way to think about batteries right now. We believe that right now are in an educational period. Utilities and customers are learning about these programs. As these programs are becoming more widespread, more people want to become part of them. Thanks to the California heat wave event, we were able to showcase that this is a real use case for batteries and there’s a high potential to grow and scale. Also, from the software perspective, a battery either small or large is the same to manage. This very fact actually opens the possibility to expand current programs and create new ones.
Conclusion
It is thanks to the combination of smart connected devices such as batteries, coupled with reliable cloud software such as the Tesla Energy platform, that we continue pushing the limits to increase energy security, both individually and collectively, to increase the quality of life, to accelerating the world transition to sustainable energy.
DellaMaria: If you’re interested in learning more about the Tesla Virtual Power Plants, or the Tesla Energy platform, we highly recommend our colleagues Colin Breck and Percy Link’s talk that they gave at QCon London in 2020. Then, if you’re interested in helping solving hard real-world problems, helping increase energy security and supporting the grid, while accelerating the transition to a sustainable world, please come join us at Tesla.
See more presentations with transcripts
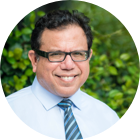
MMS • RSS
Posted on mongodb google news. Visit mongodb google news
In a recent disclosure with the Securities & Exchange Commission (SEC), it has been revealed that the State of New Jersey Common Pension Fund D has decreased its holdings in shares of MongoDB, Inc. by 1.9% during the first quarter of this year. The fund now owns 41,239 shares of the company’s stock after selling 809 shares in the said period. This amounts to approximately 0.06% ownership of MongoDB, with a valuation of $9,614,000 at the time of the SEC filing on July 14, 2023.
MongoDB, Inc. is a prominent provider of a general purpose database platform that caters to users worldwide. Its range of offerings includes MongoDB Atlas, which serves as a hosted multi-cloud database-as-a-service solution. Additionally, they provide MongoDB Enterprise Advanced, an exclusive database server that targets enterprise customers looking to utilize cloud services or opt for on-premise or hybrid environments. Furthermore, MongoDB offers Community Server, an accessible version of their database software that satisfies developers’ requirements and can be easily downloaded free-of-cost.
As trading commenced on Friday following this announcement, shares of MongoDB were priced at $407.21 per share. Examining its financial position more closely, we observe that the company maintains a debt-to-equity ratio of 1.44 – indicative of its financial leverage – alongside a current ratio and quick ratio standing at 4.19 each. These figures suggest a healthy liquidity position for the organization.
Over the past fifty-two weeks, there has been significant fluctuation in MongoDB’s stock performance. The lowest recorded price was $135.15 while the highest was reported as $418.70 – showcasing substantial volatility within this period alone.
In terms of market trends and investor sentiment towards MongoDB, Inc., it is evident that confidence has been steadily building over time. This is evidenced by the fact that despite the prevailing uncertainties and challenges faced by businesses, the company’s 50-day moving average price has reached $343.34. Moreover, the 200-day moving average price stands at an impressive $258.04, showcasing a positive and consistent upward momentum in the valuation of MongoDB stock.
As the State of New Jersey Common Pension Fund D divests from MongoDB, it is crucial to analyze the reasons behind such a decision. Given that pension funds typically make long-term investments and prioritize stability and growth potential, we can make certain assumptions about their motive for reducing their holdings in MongoDB. However, without further information or confirmation from the fund itself, it remains speculative to draw any definitive conclusions about their strategy.
In summary, MongoDB, Inc. has faced a decrease in its shares held by the State of New Jersey Common Pension Fund D during the first quarter of this year. Nevertheless, with its diverse range of database solutions and a growing market sentiment towards the company, MongoDB continues to stride forward. With an impressive fifty-two week high and optimistic moving average prices, it remains an entity worth monitoring for investors seeking opportunities in this sector.
Hedge Funds and Insider Trading Impact MongoDB’s Stock Position
MongoDB, Inc. (NASDAQ:MDB) has seen changes in the positions of several hedge funds, as reported on July 14, 2023. Bessemer Group Inc. acquired a new position in MongoDB during the fourth quarter, valued at $29,000. Similarly, BI Asset Management Fondsmaeglerselskab A S also acquired a new position in the same period with an estimated value of $30,000. Lindbrook Capital LLC saw significant growth in its holdings of MongoDB by 350% during the fourth quarter, now owning 171 shares worth $34,000 after acquiring an additional 133 shares. Y.D. More Investments Ltd purchased a new position in MongoDB with a valuation of $36,000 during the fourth quarter as well. Lastly, CI Investments Inc. increased its holdings in MongoDB by 126.8%, now owning 186 shares worth $37,000 after acquiring an additional 104 shares.
Institutional investors and hedge funds currently possess approximately 89.22% of the company’s stock.
The opinions of research analysts further shed light on MDB shares’ situation recently. Piper Sandler raised their target price for MongoDB from $270 to $400 on June 2nd. JMP Securities also increased their price objective from $245 to $370 in a research report published on the same day. Needham & Company LLC raised their price objective from $250 to $430 for MDB stock on June 2nd as well. Royal Bank of Canada also adjusted their price objective for MDB stock from $400 to $445 on June 23rd. Finally, Sanford C.Bernstein shifted their price objective for MDB stock from $257 to $424 on June 5th.
Analysts have given mixed ratings for the company’s stock; one analyst rated it as sell while three others have given a hold rating and twenty have provided buy ratings so far.
According to Bloomberg, the consensus rating for MongoDB is currently marked as “Moderate Buy” with an average target price of $366.59.
MongoDB, Inc. operates as a general purpose database platform globally. The company offers several products, including MongoDB Atlas, which is a hosted multi-cloud database-as-a-service solution. They also provide MongoDB Enterprise Advanced, a commercial database server designed for enterprise customers to run in the cloud, on-premise or in a hybrid environment. Additionally, they offer Community Server, which is a free-to-download version of their database containing all the essential features required by developers when starting to use MongoDB.
The financial performance of MongoDB was last posted on June 1st. The company reported earnings per share (EPS) of $0.56 for the quarter, surpassing the consensus estimate of $0.18 by $0.38. Their revenue for the same quarter was $368.28 million compared to analyst estimates of $347.77 million. MongoDB had a negative return on equity (ROE) of 43.25% and a negative net margin of 23.58%. However, their revenue increased by 29% compared to the corresponding quarter last year.
In recent news related to insider trading, CTO Mark Porter sold 2,734 shares of MDB stock on July 3rd at an average price of $412.33 per share, resulting in a total transaction value of $1,127,310.22. After this transaction concluded, Porter now directly owns 35,056 shares valued at approximately $14,454,640.48.
Another sale involving an insider occurred when Director Dwight A.Merriman sold 2,000 shares on April 26th at an average price of $240 per share with a total value of $480,000.
Following this transaction’s completion,
Director Merriman currently possesses 1,225,
954 shares valued at approximately $294,228,960.
Insiders have sold a total of 117,427 shares over the past ninety days with a collective value of $41,364,961. As of now, insiders hold 4.80% of MongoDB stock.
Overall, MongoDB has seen some significant activity in terms of positions held by hedge funds and institutional investors. With mixed opinions from research analysts and insider trading activities in play, it is crucial for investors to closely monitor the company’s performance and evaluate whether it aligns with their investment goals and risk tolerance.
In conclusion, while MongoDB presents a compelling case as a general purpose database platform, investors should approach the stock with caution due to its volatile market behavior and potential risks associated with the company’s financial performance.
Article originally posted on mongodb google news. Visit mongodb google news
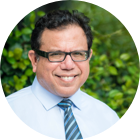
MMS • RSS
Posted on mongodb google news. Visit mongodb google news
The recommendations of Wall Street analysts are often relied on by investors when deciding whether to buy, sell, or hold a stock. Media reports about these brokerage-firm-employed (or sell-side) analysts changing their ratings often affect a stock’s price. Do they really matter, though?
Let’s take a look at what these Wall Street heavyweights have to say about MongoDB (MDB) before we discuss the reliability of brokerage recommendations and how to use them to your advantage.
MongoDB currently has an average brokerage recommendation (ABR) of 1.50, on a scale of 1 to 5 (Strong Buy to Strong Sell), calculated based on the actual recommendations (Buy, Hold, Sell, etc.) made by 22 brokerage firms. An ABR of 1.50 approximates between Strong Buy and Buy.
Of the 22 recommendations that derive the current ABR, 16 are Strong Buy and three are Buy. Strong Buy and Buy respectively account for 72.7% and 13.6% of all recommendations.
Brokerage Recommendation Trends for MDB
Check price target & stock forecast for MongoDB here>>>
While the ABR calls for buying MongoDB, it may not be wise to make an investment decision solely based on this information. Several studies have shown limited to no success of brokerage recommendations in guiding investors to pick stocks with the best price increase potential.
Do you wonder why? As a result of the vested interest of brokerage firms in a stock they cover, their analysts tend to rate it with a strong positive bias. According to our research, brokerage firms assign five “Strong Buy” recommendations for every “Strong Sell” recommendation.
This means that the interests of these institutions are not always aligned with those of retail investors, giving little insight into the direction of a stock’s future price movement. It would therefore be best to use this information to validate your own analysis or a tool that has proven to be highly effective at predicting stock price movements.
Zacks Rank, our proprietary stock rating tool with an impressive externally audited track record, categorizes stocks into five groups, ranging from Zacks Rank #1 (Strong Buy) to Zacks Rank #5 (Strong Sell), and is an effective indicator of a stock’s price performance in the near future. Therefore, using the ABR to validate the Zacks Rank could be an efficient way of making a profitable investment decision.
ABR Should Not Be Confused With Zacks Rank
In spite of the fact that Zacks Rank and ABR both appear on a scale from 1 to 5, they are two completely different measures.
Broker recommendations are the sole basis for calculating the ABR, which is typically displayed in decimals (such as 1.28). The Zacks Rank, on the other hand, is a quantitative model designed to harness the power of earnings estimate revisions. It is displayed in whole numbers — 1 to 5.
It has been and continues to be the case that analysts employed by brokerage firms are overly optimistic with their recommendations. Because of their employers’ vested interests, these analysts issue more favorable ratings than their research would support, misguiding investors far more often than helping them.
On the other hand, earnings estimate revisions are at the core of the Zacks Rank. And empirical research shows a strong correlation between trends in earnings estimate revisions and near-term stock price movements.
In addition, the different Zacks Rank grades are applied proportionately to all stocks for which brokerage analysts provide current-year earnings estimates. In other words, this tool always maintains a balance among its five ranks.
There is also a key difference between the ABR and Zacks Rank when it comes to freshness. When you look at the ABR, it may not be up-to-date. Nonetheless, since brokerage analysts constantly revise their earnings estimates to reflect changing business trends, and their actions get reflected in the Zacks Rank quickly enough, it is always timely in predicting future stock prices.
Is MDB a Good Investment?
Looking at the earnings estimate revisions for MongoDB, the Zacks Consensus Estimate for the current year has increased 1.6% over the past month to $1.51.
Analysts’ growing optimism over the company’s earnings prospects, as indicated by strong agreement among them in revising EPS estimates higher, could be a legitimate reason for the stock to soar in the near term.
The size of the recent change in the consensus estimate, along with three other factors related to earnings estimates, has resulted in a Zacks Rank #2 (Buy) for MongoDB. You can see the complete list of today’s Zacks Rank #1 (Strong Buy) stocks here >>>>
Therefore, the Buy-equivalent ABR for MongoDB may serve as a useful guide for investors.
Want the latest recommendations from Zacks Investment Research? Today, you can download 7 Best Stocks for the Next 30 Days. Click to get this free report
MongoDB, Inc. (MDB) : Free Stock Analysis Report
To read this article on Zacks.com click here.
Article originally posted on mongodb google news. Visit mongodb google news
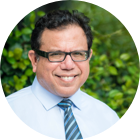
MMS • Almir Vuk
Article originally posted on InfoQ. Visit InfoQ
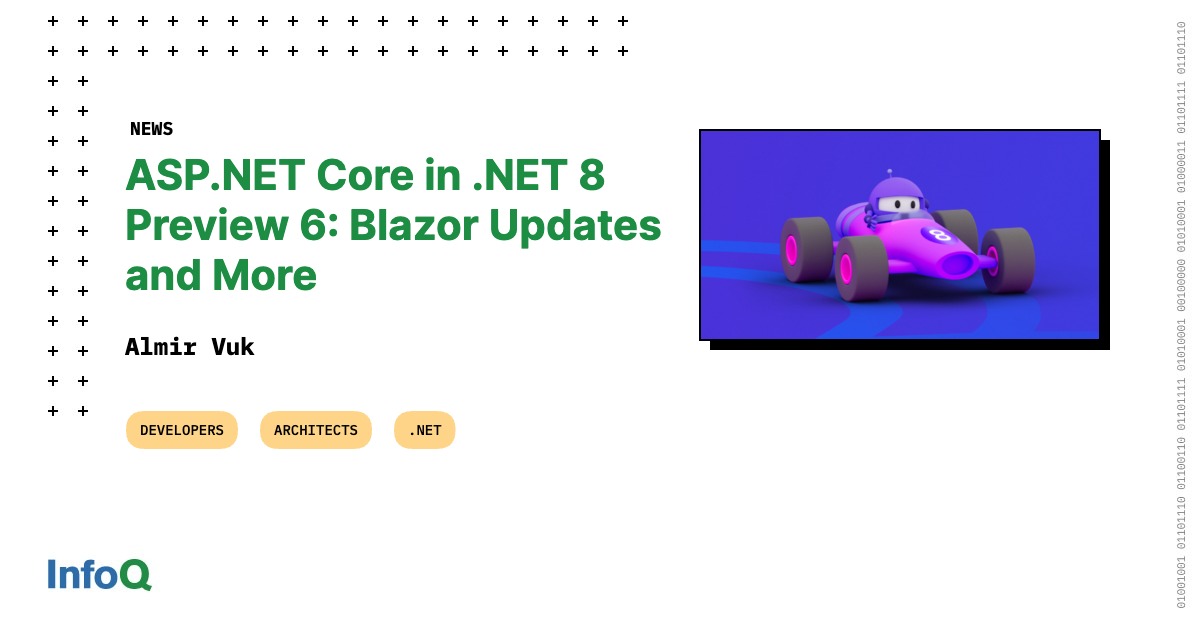
The latest release of .NET 8 Preview 6 brings significant additions and changes to ASP.NET Core. The most notable enhancements for this release of ASP.NET Core, are related to the Blazor alongside the updates regarding the debugging experience, testing metrics, API authoring, servers, middleware and many more.
Regarding the Blazor, several new features have been introduced to enhance its server-side rendering mode. One notable addition is the ability to model bind and validate HTTP form post values. This can be achieved by applying the SupplyParameterFromForm
attribute to a component property, enabling easier data binding from form requests.
Moreover, Blazor now offers enhanced page navigation and form-handling capabilities. By intercepting requests and applying the response to the existing DOM, the framework preserves as much of the page as possible, resulting in a smoother user experience to a single-page app (SPA) while still utilizing server-side rendering.
Blazor’s streaming rendering has been enhanced to preserve existing DOM elements during updates, leading to faster and smoother interactions. Developers now have the ability to specify the render mode for a component instance using the @rendermode directive attribute, granting more precise control over rendering behaviour. Enabling call site @rendermode usage requires setting the Razor Language Version to 8.0 in the project file, though this step will be automated in future framework releases, eliminating the need for manual adjustments.
Blazor WebAssembly now supports interactive rendering of components, although this option is not yet exposed in the Blazor Web App template. Interested developers can enable this functionality manually to take advantage of the interactive rendering capabilities offered by Blazor WebAssembly. A demo sample is provided to illustrate the setup of WebAssembly-based interactivity for a Counter component rendered within the Index page. This sample serves as a practical example of implementing interactivity using WebAssembly in Blazor applications.
There were a couple of improvements to how Blazor sections interact with other Blazor features. These enhancements include changes to how cascading values are handled, with values now flowing into section content from their point of definition rather than where they are rendered in a section outlet. Additionally, unhandled exceptions are now handled by error boundaries around the section content, rather than the section outlet. Furthermore, the decision on whether section content should use streaming rendering is now determined by the component where the section content is defined, rather than the component defining the section outlet.
Other significant Blazor-related changes include the introduction of cascading query string values to Blazor components, the incorporation of a Blazor Web App template option for enabling server interactivity, and template consolidation.
From Preview 6 developers can now benefit from improved testing of metrics in their applications. The introduction of ASP.NET Core metrics in a previous .NET 8 preview has made it easier to test metrics, thanks to the IMeterFactory API. This API integrates metrics with dependency injection, simplifying the process of isolating and collecting metrics for testing purposes. Whether conducting unit tests or running multiple tests simultaneously, developers can rely on IMeterFactory to gather accurate data specifically tailored to their testing needs.
ASP.NET Core reinforces its commitment to metrics support with the introduction of new, improved, and renamed counters. These enhancements aim to enhance observability in reporting dashboards and enable custom alerts for ASP.NET Core applications. The new counters include metrics for routing success and failure, exception handling, unhandled requests, and rate limiting. Improved counters now provide additional details on connection duration and transport types
Other notable changes included in this release are related to complex form binding support in minimal APIs, HTTP.sys kernel response buffering, Redis-based output caching and improved debugging experience for the Web Application type. The team recommends the installation of the latest Visual Studio 2022 Preview and there is also a full list of breaking changes in ASP.NET Core for .NET 8 so developers can check that out along the way.
Lastly, the comment section on the original release blog post has been full of reactions, regarding the changes and improvements for Blazor and the blog post has generated significant activity, with users engaging in numerous questions and discussions with the development team. To get a comprehensive understanding of the various perspectives, it is highly recommended for users explore the comment section and engage in the ongoing discussion.
NextGen Healthcare to pay $31 mln to settle false claims act allegations -Justice Dept.
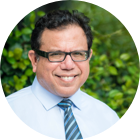
MMS • RSS
Posted on mongodb google news. Visit mongodb google news

July 14 (Reuters) –
Electronic health records vendor NextGen Healthcare Inc has agreed to pay $31 million to resolve allegations that the company violated the False Claims Act, the U.S. Justice Department said on Friday.
NextGen made the alleged violation by misrepresenting the capabilities of certain versions of its electronic health records software and providing unlawful remuneration to its users as an inducement to recommend the company’s software, according to a statement from the Justice Department. (Reporting by Ismail Shakil in Ottawa; Editing by Caitlin Webber)
Article originally posted on mongodb google news. Visit mongodb google news
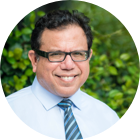
MMS • RSS
Posted on mongodb google news. Visit mongodb google news
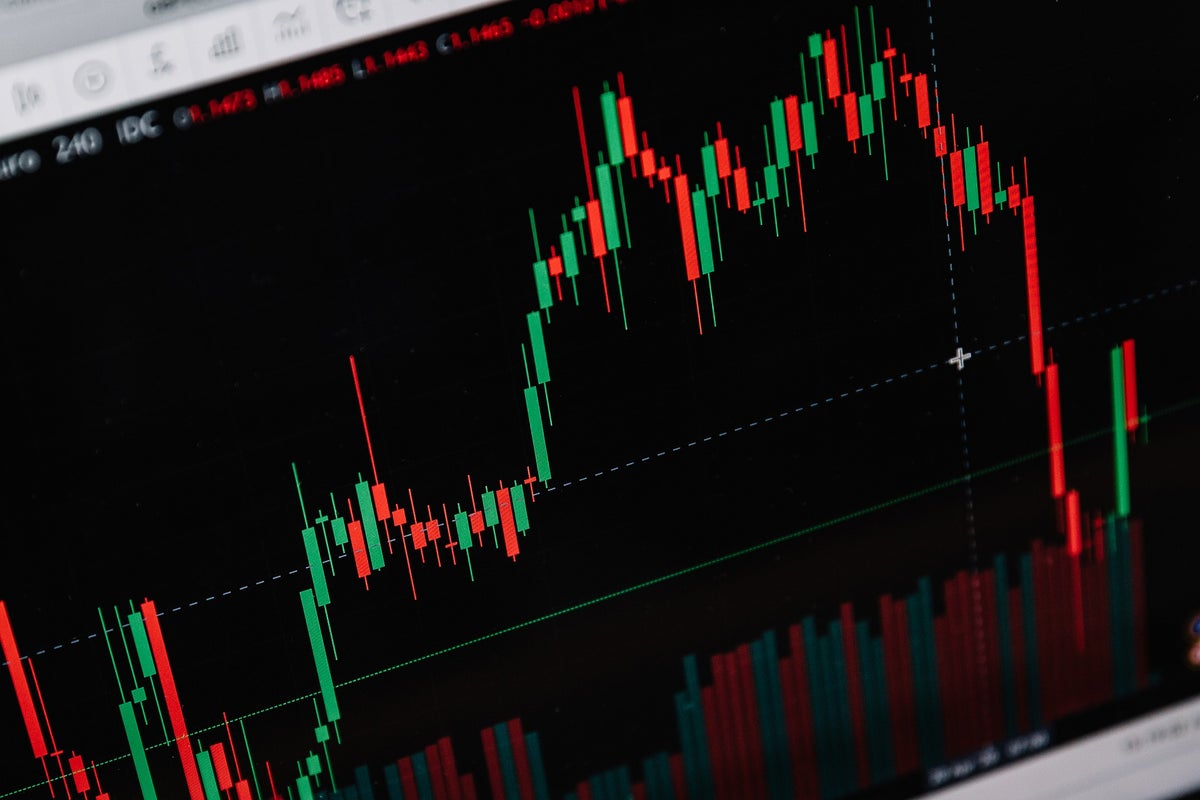
A whale with a lot of money to spend has taken a noticeably bullish stance on MongoDB.
Looking at options history for MongoDB MDB we detected 10 strange trades.
If we consider the specifics of each trade, it is accurate to state that 60% of the investors opened trades with bullish expectations and 40% with bearish.
From the overall spotted trades, 3 are puts, for a total amount of $101,090 and 7, calls, for a total amount of $376,165.
What’s The Price Target?
Taking into account the Volume and Open Interest on these contracts, it appears that whales have been targeting a price range from $390.0 to $560.0 for MongoDB over the last 3 months.
Volume & Open Interest Development
In terms of liquidity and interest, the mean open interest for MongoDB options trades today is 176.38 with a total volume of 279.00.
In the following chart, we are able to follow the development of volume and open interest of call and put options for MongoDB’s big money trades within a strike price range of $390.0 to $560.0 over the last 30 days.
MongoDB Option Volume And Open Interest Over Last 30 Days
Biggest Options Spotted:
Symbol | PUT/CALL | Trade Type | Sentiment | Exp. Date | Strike Price | Total Trade Price | Open Interest | Volume |
---|---|---|---|---|---|---|---|---|
MDB | CALL | SWEEP | BULLISH | 07/21/23 | $400.00 | $90.0K | 635 | 18 |
MDB | CALL | TRADE | BULLISH | 02/16/24 | $390.00 | $65.6K | 20 | 9 |
MDB | CALL | SWEEP | BULLISH | 07/14/23 | $405.00 | $61.5K | 299 | 206 |
MDB | CALL | SWEEP | NEUTRAL | 07/14/23 | $405.00 | $54.9K | 299 | 27 |
MDB | PUT | SWEEP | BULLISH | 08/18/23 | $560.00 | $44.1K | 0 | 3 |
Where Is MongoDB Standing Right Now?
- With a volume of 237,396, the price of MDB is up 0.24% at $408.18.
- RSI indicators hint that the underlying stock may be approaching overbought.
- Next earnings are expected to be released in 47 days.
What The Experts Say On MongoDB:
- Barclays has decided to maintain their Overweight rating on MongoDB, which currently sits at a price target of $421.
- Truist Securities has decided to maintain their Buy rating on MongoDB, which currently sits at a price target of $420.
- Capital One downgraded its action to Equal-Weight with a price target of $396
- Piper Sandler downgraded its action to Overweight with a price target of $400
- Morgan Stanley has decided to maintain their Overweight rating on MongoDB, which currently sits at a price target of $440.
Options are a riskier asset compared to just trading the stock, but they have higher profit potential. Serious options traders manage this risk by educating themselves daily, scaling in and out of trades, following more than one indicator, and following the markets closely.
If you want to stay updated on the latest options trades for MongoDB, Benzinga Pro gives you real-time options trades alerts.
Article originally posted on mongodb google news. Visit mongodb google news
Upcoming Opportunities in Database Middleware Market: Future Trend and Analysis of Key …
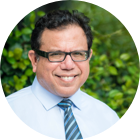
MMS • RSS
Posted on mongodb google news. Visit mongodb google news
A complete study of the global “Database Middleware Market” is carried out by the analysts in this report, taking into consideration key factors like drivers, challenges, recent trends, opportunities, advancements, and competitive landscape. This report offers a clear understanding of the present as well as future scenarios of the global Database Middleware industry. Research techniques like PESTLE and Porter’s Five Forces analysis have been deployed by the researchers. They have also provided accurate data on Database Middleware production, capacity, price, cost, margin, and revenue to help the players gain a clear understanding of the overall existing and future market situation.
The Database Middleware research study includes great insights into critical market dynamics, including drivers, restraints, trends, and opportunities. It also includes various types of market analysis such as competitive analysis, manufacturing cost analysis, manufacturing process analysis, price analysis, and analysis of market influence factors. It is a complete study of the global Database Middleware market that can be used as a set of effective guidelines for ensuring strong growth in the coming years. It caters to all types of interested parties, viz. stakeholders, market participants, investors, market researchers, and other individuals associated with the Database Middleware business.
Updated Version PDF Report & Online Dashboard will help you understand:
➤ 2023 Latest updated research report with Overview, Definition, TOC, updated Top market players
➤ 115+ Pages Research Report
➤ Provide Chapter-wise guidance on the Request
➤ Updated 2023 Regional Analysis with Graphical Representation of Size, Share & Trends
➤ Updated Research Report Includes a List of tables & figures
➤ Report Includes updated 2023 Top Market Players with their latest Business Strategy, Sales Volume, and Revenue Analysis
➤ COVID-19 Pandemic Impact on Businesses
➤ Facts and Factors updated research methodology
Purchase the Latest Report Version Now with an Incredible Discount! (Up to 70%) : https://www.stratagemmarketinsights.com/promobuy/171379
Leading players of the global Database Middleware market are analyzed taking into account their market share, recent developments, new product launches, partnerships, mergers or acquisitions, and markets served. We also provide an exhaustive analysis of their product portfolios to explore the products and applications they concentrate on when operating in the global Database Middleware market. Furthermore, the report offers two separate market forecasts – one for the production side and another for the consumption side of the global Database Middleware market. It also provides useful recommendations for new as well as established players in the global Database Middleware market.
Scope of Database Middleware Market:
Emerging trends, The report on the Database Middleware market gives the complete picture of demands and opportunities for the future that are beneficial for individuals and stakeholders in the market. This report determines the market value and the growth rate based on the key market dynamics as well as the growth-improving factors. The entire study is based on the latest industry news, market trends, and growth probability. It also consists of a deep analysis of the market and competing scenario along with a SWOT analysis of the well-known competitors.
Database Middleware Market Segments:
According to the report, the Database Middleware Market is segmented in the following ways which fulfill the market data needs of multiple stakeholders across the industry value chain –
The Leading Players involved in the global Database Middleware market are:
- Cloudra
- AWS
- SphereEx
- EMQX
- Confluent
- MongoDB
- Rapid7
- Mycat
- Connecting Software
- Amoeba
- Alibaba Cloud
- Huawei Cloud
- CtWing
- ZTE
- Qihoo 360
- Beijing Com and Lan Tech
- Apiseven
Segmentation by Type:
- Database Synchronization Middleware
- Across Databases Migrating Middleware
- Database Sub-table Sub-database Middleware
- Data Incremental Order and Consumption Middleware
Segmentation by Applications:
- Public Cloud Service
- Private Cloud Service
- Hybrid Cloud Service
Our market analysts are experts in deeply segmenting the global Database Middleware market and thoroughly evaluating the growth potential of each and every segment studied in the report. Right at the beginning of the research study, the segments are compared on the basis of consumption and growth rate for a review period of nine years. The segmentation study included in the report offers a brilliant analysis of the global Database Middleware market, taking into consideration the market potential of the different segments studied. It assists market participants to focus on high-growth areas of the global Database Middleware market and plan powerful business tactics to secure a position of strength in the industry.
The regional analysis provided in the Database Middleware research study is an outstanding attempt made by the researchers to help players identify high-growth regions and modify their strategies according to the specific market scenarios therein. Each region is deeply analyzed with a large focus on CAGR, market growth, market share, market situations, and growth forecast.
Important Facts about This Market Report:
This research report reveals this business overview, product overview, market share, demand and supply ratio, supply chain analysis, and import/export details.
The Industry report captivates different approaches and procedures endorsed by the market key players to make crucial business decisions.
This research presents some parameters such as production value, marketing strategy analysis, Distributors/Traders, and effect factors are also mentioned.
The historical and current data is provided in the report based on which the future projections are made and the industry analysis is performed.
The import and export details along with the consumption value and production capability of every region are mentioned in the report.
Porter’s five forces analysis, value chain analysis, and SWOT analysis are some additional important parameters used for the analysis of market growth.
The report provides the clients with facts and figures about the market on the basis of the evaluation of the industry through primary and secondary research methodologies.
What to Expect in Our Report?
☛ A complete section of the Database Middleware market report is dedicated to market dynamics, which include influence factors, market drivers, challenges, opportunities, and trends.
☛ Another broad section of the research study is reserved for regional analysis of the global Database Middleware market where important regions and countries are assessed for their growth potential, consumption, market share, and other vital factors indicating their market growth.
☛ Players can use the competitive analysis provided in the report to build new strategies or fine-tune their existing ones to rise above market challenges and increase their share of the global Database Middleware market.
☛ The report also discusses competitive situations and trends and sheds light on company expansions and mergers and acquisitions taking place in the global Database Middleware market. Moreover, it brings to light the market concentration rate and market shares of the top three and five players.
☛ Readers are provided with the findings and conclusions of the research study provided in the Database Middleware Market report.
Key Questions Answered in the Report:
What are the growth opportunities for the new entrants in the global Database Middleware industry?
Who are the leading players functioning in the global Database Middleware marketplace?
What are the key strategies participants are likely to adopt to increase their share in the global Database Middleware industry?
What is the competitive situation in the global Database Middleware market?
What are the emerging trends that may influence the Database Middleware market growth?
Which product type segment will exhibit high CAGR in the future?
Which application segment will grab a handsome share in the global Database Middleware industry?
Which region is lucrative for the manufacturers?
Grab the Hottest Deal: Purchase the Latest Report Version Now with an Incredible Discount! (Up to 70%): https://www.stratagemmarketinsights.com/promobuy/171379
Stay ahead of the curve and drive your business forward with confidence. The Future of Industries report is your indispensable resource for navigating the ever-evolving business landscape, fueling growth, and outperforming your competition. Don’t miss this opportunity to unlock the strategic insights that will shape your company’s future success.
About SMI:
Stratagem Market Insights is a global market intelligence and consulting organization focused on assisting our plethora of clients to achieve transformational growth by helping them make critical business decisions. We are headquartered in India, have an office at global financial capital in the U.S., and sales consultants in the United Kingdom and Japan. Our client base includes players from across various business verticals in over 32 countries worldwide. We are uniquely positioned to help businesses around the globe deliver practical and lasting results through various recommendations about operational improvements, technologies, emerging market trends, and new working methods.
Contact Us:
Mr. Shah
Stratagem Market Insights
U.S.A: +1-415-871-0703
UK: +44-203-289-4040
JAPAN: +81-50-5539-1737
Email: [email protected]
Website: https://www.stratagemmarketinsights.com/
Article originally posted on mongodb google news. Visit mongodb google news