Month: July 2023
Presentation: Microservices Retrospective – What We Learned (and Didn’t Learn) From Netflix
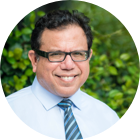
MMS • Adrian Cockcroft
Article originally posted on InfoQ. Visit InfoQ
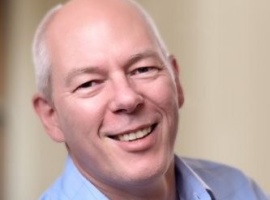
Transcript
Cockcroft: I’m Adrian Cockcroft. I’m going to talk to you about microservices retrospective: what we learned and what we didn’t learn from Netflix. I was at Netflix from 2007 to the end of 2013. We’re going to look a bit at that, and some of the early slide decks that I ran through at the time. It’s a retrospective. I don’t really know that much about retrospectives, but a good friend of mine does. I read some of Aino’s book, and figured that there’s a whole lot of these agile rituals being mentioned in this book, along with retrospectives. It turns out, Netflix was extremely agile, but was not extreme, and was not agile. We did extreme and agile with a lowercase e and a lowercase a, we did not have the rituals of a full extreme, or full agile. I don’t remember anyone being a scrum master of all of those kinds of things. We’re going to talk a fair amount about the Netflix culture. The Netflix culture is nicely documented in this book, “Powerful: Building a Culture of Freedom and Responsibility,” by Patty McCord, who ran the HR processes and talent. Basically, she was the CTO for Netflix, which was the Chief Talent Officer. Amazing woman, you can see some of her talks. I figured that I should adopt some of the terminology anyway. I’ve got some story points. I’m going to talk about some Netflix culture. Pick up some of the slide decks from those days. Go over some of the things that were mentioned, and then comment on them. What we did. What we didn’t do. What seemed to work. What got left out along the way. I’ll talk a bit about why don’t microservices work for some people. Then a little bit at the end, just talking about systems thinking and innovation.
Netflix Culture – Seven Aspects of Netflix Culture
Netflix culture, these seven points. The first point is that the culture really matters. They place a high value on the fact that values are what they value. Then, high performance. This is a high-performance culture. It’s not trying to build a family, they’re trying to build an Olympic winning team, or a league winning team. Pick your favorite sport, how do you build the best team ever for that sport? You go find the best players, and you stack everything in their direction. Those players are the best in the world, so you get out of their way. You do what they tell you to do. There’s a bit of coaching, but fundamentally, they have the freedom, but they’re also responsible for being the best in the world. What that means is, as a manager, you’re giving them context, not control, setting everything up to be successful. Then the teams are highly aligned. We have a single goal to win whatever we’re trying to win. Launch in a market or take over something. The teams are loosely coupled, they were individually working on these things, and they have clear APIs where they touch.
If you’re building the best team in the world, with the best people in the world, you have to pay top of market. This is something Netflix does. It’s always been one of the smaller companies in the Bay Area, and it is very savvy, so it’s pretty much the highest paying engineering company in the Bay Area. Then, promotions and development. Netflix management don’t have to worry too much about promotions. It’s up to you to develop yourself. Part of the adult freedom responsibility thing is figure out how to develop yourself. There was no official mentoring program when we were there. You’re supposed to be at the top of your game already. You already know how to maintain that. You get to run your own exercise regime effectively. By having very wide salary bands and very wide career talent levels, they call it grade levels effectively, you have to have very many promotions. You can move up financially, and you’re just a senior engineer. When I was there, there were senior engineers, managers, directors, and VPs. Those were the only titles you could have. We didn’t have any junior engineers, and there wasn’t any grade scales in between. The managers didn’t have to worry about it. There’s a whole lot of things that are just unusual here. This is part of the system that lets Netflix do things really quickly and effectively.
Netflix Systems Thinking
Netflix is a systems thinking organization. Lots of interesting feedback loops, lots of subtleties in the way they put things together. Optimize for agility. Minimize processes. This ability to evolve extremely rapidly. Unafraid and happy to be the first to do something, pioneers. Netflix was the first company ever to use NGINX. The guy who built NGINX had to found a company so that we could pay him for support. One of the first customers for JFrog’s Artifactory. One of the first customers for AppDynamics. One of the first big enterprise customers for AWS and probably some other companies that I’ve forgotten along the way. By being a pioneer customer, for an interesting startup, you get to really leverage them, they will do all kinds of things for you. You get a great discount, because they love the feedback. It works out. There’s a system here, but you have to be good at doing it.
Then, you have to be comfortable with ambiguity, that’s a very complex system. There’s a lot going on. Things aren’t done one way. There’s a lot of creativity, but you have to have self-discipline and combine the two together. Then, there’s this interesting point that flexibility is more important than efficiency. If you crunch something down and make it super-efficient, it becomes inflexible. You don’t want to waste things. You don’t want to squeeze them to be super-efficient, either, because you’re taking away the flexibility that lets you innovate and try things. If everyone is so crazy busy all the time, because you’re running a super-efficient system and everyone’s super busy, they don’t have time to learn new things. They don’t have time to do their best work basically. Then the final principle, steer pain to where it can be helpful. One of the things that we did, certainly, while I was there, was we put engineers on-call, developers on-call. If you were writing code, and you ship the code that day, you were on-call to fix it if it broke that night. That caused developers to be much more careful about the code they deployed. They learned a lot of good practices.
Netflix in the Cloud
Here’s the first deck. This was from November 2010. It’s the deck that I presented at QCon San Francisco. I did do a little meetup before that, where I tried out some of this content. This was really the first time that we presented Netflix to the world. There’s a whole bunch of things here. Undifferentiated heavy lifting, wanted to stop doing that. We went through what we were doing. These goals would work for just about any transition that you might want to make, to the cloud or to some new architecture. You want to be faster, more scalable, more available, and more productive.
Old Data Center vs. New Cloud Arch
In the old data center, we had a central SQL database, so it was Oracle. What we wanted to do is move to a distributed key-value, NoSQL, denormalized our system so that everyone could have their own backend. We weren’t trying to do queries and all kinds of things that wouldn’t scale. In the old system, we had a sticky in-memory session. A customer would connect, and that thread would have the session cookie route that customer to that thread again for the next part of the request. Cause all kinds of issues. It’s not very scalable. What we did was we stored all of that session information outside the instances in Memcached, so that we could basically have a stateless system. Every time you came in, you could talk to a totally different frontend. It would look in Memcached, find the session information and respond. We just built it to be that way. In the data center, we had pretty chatty protocols. The machines were all sitting next to each other. There’s no reason not to. In the cloud, we actually set up everything so that it was a long way away. We were working in the East Coast data center, U.S.-East, and Netflix’s engineers were on the West Coast, so everything was long latency anyway. That actually helped people build latency tolerant protocols, because as they were testing things, they had 100 millisecond latency to deal with.
We had very tangled service interfaces, and we moved that to be layered. We instrumented code in the data centers, but we instrumented service patterns, so you built a certain new service, and you would just write your code, build the templates. All of the monitoring and stuff would already be there once you got it running, because we had that in the service pattern. We had very fat, complex objects. I’ll talk a bit more about what that looks like. We wanted to move to lightweight serializable objects. Typically, the work of an engineer or developer in the data center was you built a JAR file, and you checked it in, and a bit later somebody in QA would check out all the latest JAR files and try to make a build on the monolith and see if they could get it to work. We do that every two weeks. In the new architecture, you take that JAR file, you wrap a service around it. You give it an API on the front. You wrap it into a machine image and you fire it up. You deploy a whole bunch of them. It’s up to you to do your own QA, to develop it, to get it out there, and see if it works, and deploy it whenever you feel like it. That’s what I mean by components or services.
Tangled Service Interfaces
Looking at these tangled interfaces, the data center, we had SQL queries and business logic, really deep dependencies for sharing these patterns where you call an object to get to something else through it, so trampolining through objects, all kinds of bad stuff. Then, when you call the data provider, we had sideways dependency. If you tried to call, it was a library that you’d use to do something, you’d pull that library into your code, and you’d end up where the whole universe would come in. There’s this sense that you call a dependency, you add one more dependency to your repo, and you’d go over the event horizon of a black hole. Eventually, the entire repo would turn up in your build. We were trying to cut that dependency.
Untangled Service Interfaces
We untangled them. We built an interface. We sometimes use Spring runtime binding, and a lot of the Netflix patterns ended up in Spring Cloud a bit later on. The service interface is the service. This is something that I think people don’t quite get. I’m doing a movie service, and the way that I expose that movie service, is I build a movie service JAR file, and I put it in Artifactory. Somebody else wants to use the movie service and they pull that JAR and it’s got an API, and they call that API. They don’t actually know or mostly don’t care what’s going on behind that. There may be two or three actual microservices behind that library. The only thing you actually need to know is the first time you deploy it, you have to go figure out the security connections, because otherwise you can’t connect to services. You have to go to modify the security groups to add you as a customer of those services. From a code point of view, the implementation is hidden, and you can mess around locally, remotely. You can iterate. You can evolve the underlying calls on the wire independently of the API that you’re giving to the customer that’s trying to use this thing. I think this is something that got lost along the way. I don’t think this is a lesson that wasn’t learned. The wire protocol shouldn’t be the interface between microservices. There’s a good example of this. If you ever use AWS, most of the time, you use an AWS SDK for the language you’re doing. That’s exactly the AWS SDK is AWS. The fact that there’s API calls underneath and stuff on the wire, it’s hidden from you.
If you do that as one big library, a couple pulls in everything. You pull in the AWS SDK, all of a sudden, you’ve got a big pile of stuff. What we actually wanted to do was split that. The first thing was a basic layer, usually built by the people that built the underlying service behind it. We called the service, access library. It’s like a device driver. It does serialization, error handling. It minimizes its sideways dependencies. Logically, it’s like a SCSI driver talking to a disk. You never talk directly to a disk, you use the SCSI driver to talk to a disk or NVMe, whatever the latest SSD interfaces are. You don’t actually talk directly to that very often. Most of the time, you use a file system, and file systems are weird and complicated. There’s lots of different ones, but they sit on top of disk drivers that are used by all of them. What you’ve got is a disk driver, a service access library, and then you have an extended library above that.
Service Interaction Pattern (Sample Swimlane Diagram)
Seeing this is a neat pattern. Here’s a relatively complex way of doing something like that. You read this top to bottom and left to right. An application calls the movie library, say, “I have a movie. I want to know something about this movie, because I’m going to present it. I want to get back the boxshot, the synopsis, or a bunch of information about it that I’m going to present and build into a response to the web page so that I can render that movie.” That ESL looks in a local cache, doesn’t find it. Then it calls the driver for a cache, which goes to a cache service and says, no, we haven’t got it in the cache. Then it calls the actual movie service. This is transparently and within this ESL, it calls the movie driver, which goes across and calls the movie service, which returns something. Then saves that for next time, and actually pokes it into the cache from behind so that next time somebody looks for it in the cache, they’ll find it. There’s a working set of common movies which are currently popular, which the movie site is trying to render right now. There’s just a way to maintain that. Then you put it in the local cache and return the result, and the application is often done. This is one way of building something showing the different layering that can be done. Notice the cache access layer is used by the movie service and by the presentation service there, but they have different things above it. You’ve just got the minimum things you need.
Boundary Interfaces
One of the things this let us do was move forward, when external dependencies didn’t exist. The first time we tried to move to cloud, there was no identity system. We hadn’t figured out how to do identity yet, so we built a fake driver that just had in it the actual developers that were writing code, so about 20 people that were working on this at the time. We built an identity service, which would only return those 20 people. We were the only 20 people that were going to log into the cloud and try and do anything. Then we were able to build all the other layers above it. You can unblock and decouple things by basically stubbing these services and then putting them in later. This let us build this much more interesting interface on top early.
One Object That Does Everything, & An Interface for Each Component
In the data center, we had the movie object and the customer object, and they did everything, and you use one or the other, or both to do anything. They would be horribly complex. They’d been developed, they got super tangled. A lot of the problems we had with our 100-person development team was just about anything you did, touched those objects, and then you’d be trying to resolve all the different people that had touched the movie objects in this biweekly build we were putting together, really problematic. We wanted to do something different. What we did was basically say we’re going to have video and visitor, so your code will not work if you ship your code that talks to movies and customers in the data center. You turn up in the cloud environment, no, sorry, we have totally different object model. The video is just an idea. It just contains a number, it’s just a large integer. The visitor is just an integer. If you want to serialize 100 movies and send it to somebody, it’s a list of 100 integers. It is the type of video, it’s an array of 100 videos. When you get to the other end, you say, ok, I have a video, but I want to present this. I’m going to take that video and just say, as a PresentationVideo, and the system would basically fill in all of that facet, all of the information. You’d need a presentation layer, boxshot, and synopsis, and whatever. Then the MerchableVideo, it’s got a whole bunch of factors, which had to do with the personalization algorithm, needs a whole bunch of information that the presentation doesn’t need. You end up with multiple different views of the video. When you’re passing the system around, you’re just using this integer. It was fully type safe and managed and built out in Java. A lot of work into that. I think that got lost along the way as well. I’m not sure if the Netflix code uses it still. It was a pretty neat idea of making it clean. The response to this talk in 2010 was a mixture of incomprehension and confusion. Most people thought we were crazy. Somebody actually said, you’re going to be back in your data centers when this fails and blows up in your face.
Replacing Data Center Oracle with Global Apache Cassandra on AWS
Less than a year later, I did another talk. This was at the Cassandra Summit, “Replacing Data Center Oracle with Cassandra on AWS.” This is one of my favorite slides, all the things we didn’t do. We didn’t wait. We didn’t get stuck with the wrong config. We didn’t file tickets. We didn’t wait to ask permission. We didn’t run out of space and power. We didn’t do capacity planning. We didn’t have meetings with IT, and all these things that other people were waiting for. We just didn’t do because everything was API driven. Your developer just was doing it self-service. The problem we had was data centers. We couldn’t build them fast enough for what we were doing. We were transitioning from Netflix shipping DVDs model, which had a fairly small footprint, to a streaming model, which was an exponentially growing footprint. This is what it looked like. This is a nice exponential growth of just about one year. The beginning of January 2010, we said we need to be out of the data center before the end of this year. We’re not going to build a new data center, so we’ve got to do it. Because we predicted we would have blown up, Netflix had massive outages at the end of the year if we hadn’t done the move to cloud. This was API call rate, 37x. This is what happens when customers instead of shipping a DVD and poking at the API once or twice a week, they’re streaming continuously. Then the proportion of customers that were streaming was rapidly increasing.
We got high availability with Cassandra by using availability zones, and they’re actually 10 kilometers to 100 kilometers apart. I’ve got three copies of my data that are separated by a good number of miles, and kilometers. It all works well. Then we also used the same model to go around the world. We had this nice model, which is basically saying, instead of being stuck in one data center, we’ve just unblocked ourselves with this NoSQL, eventually consistent Cassandra system that we can be anywhere in the world. This is the Australian map of the world, which puts Hawaii and Australia in the center, because what you really need to know is, how far are you away from Hawaii so you can go there on vacation.
The other thing we were doing was Chaos Monkey. This was partly to make sure systems were resilient. The real thing we were doing was it was a design control, because we were autoscaling everything, you want to be able to scale down. You can always scale up by just adding instances. If you’re really careful when you’re releasing a new service, you can fail. We didn’t want to do that. We wanted to be able to scale down super aggressively. We wanted to also be able to roll out new versions of something and switch traffic to it and turn off the old one, and just have the system just retrial and keep going and not notice. Your customers wouldn’t notice me. Maybe an individual request would be half a second slower because it was retrying something. That’s not going to be a big deal. If you really want to autoscale down, you should be running a Chaos Monkey, because that’s really just proving that you can. The response to this was that Netflix was a unicorn, and it might work for us. I admitted it was working for us, but it wasn’t relevant to anybody else. No one else is going to do anything like this, you just are kind of weird thing.
Cloud Native Applications: What Changed?
About 18 months later, beginning of 2013, I did another talk. This time we’d launched our open source program. This is one of the talks from one of the meetups where we launched the open source program and a whole bunch of other stuff. This is also where I started using the words cloud native and microservices, one of the early uses for that. Cloud native applications, what changed? February 2013. 2006, EC2 launched. What people did with it was they forklifted old code to the cloud, because it was faster than asking somebody in IT for an instance. What we developed is these new anti-fragile patterns, microservices, chaos engines, and composing highly available systems from ephemeral components. Then we used open source platforms, so these techniques spread rapidly. This is a totally new set of development patterns and tools. Enterprises were just baffled by it. The startups and the scaled cloud native companies, the Uber, Lyft, Airbnb, and Netflix, those kinds of companies, we all were doing this. The enterprises weren’t at this point. Capital One was probably one of the first enterprises to really dive in and try and figure this out. Nike was starting to try and figure it out. A few people were, but it was pretty slow in terms of enterprise adoption.
Typically, the people use Cloud, they just stuck their app servers in the cloud and stuck a data center somewhere else. This was the way you were supposed to use cloud because we didn’t trust putting data in the cloud. The way we did it with Cassandra, was to spread everything over three zones. If we lost an availability zone, everything just kept working. We tested this and proved that it just kept working. That was a cloud native architecture. This is a way of running a system, which you never saw in the data center. Master copies of the data are cloud resident, it’s dynamically provisioned. It’s ephemeral. For me, cloud native meant that the application was built to only run in the cloud and optimize for that way. I think the CNCF co-opted the term, so cloud native currently is our Cloud Native Computing Foundation. It means Kubernetes or something nowadays. I think that’s much more operations. That isn’t the way you built your application, it’s more about how you’re operating it.
Looking at availability, one of the things with availability is not, does it stay up, but I need a machine. A few minutes later, I’ve got it in the cloud. In the data center you might spend months waiting for it. The first thing about availability is I got it. Then I can have it running in all different places so it’s available. I can have machines in Brazil or South Africa. I think AWS is about to launch in New Zealand and Israel and wherever, lots of different places around the world. That’s another point of availability. I am available in lots of different places. Those places can be far enough apart that if something breaks in one of them, we can use another one. That was an interesting point. Then, from a development point of view, we had these nice anti-fragile development patterns, and the Hystrix circuit breakers, pretty influential, successful project. This is the whole diagram of how it works. Basically, you call in to the frontend, and it’s sitting there building this nice isolation layer that has backend services and has a bunch of observability. You can see what’s happening. You can monitor it. You can actually see, this backend service has stopped working, and we’ve stopped trying to call it, and you should do something about it. In the meantime, we’ve got a fallback, which just returns some static content, or that part of the response, part of a website, web page, doesn’t appear for a while, because that backend is not behaving itself. Maybe the history service has gone down, so your rental history isn’t appearing for now. You can do everything else. You just can’t see that one part of the system.
We had this nice cartoon we came up with, the strange world of the future, stranded without video, no way to fill their empty hours, victims of the cloud of broken streams. One of the things with Netflix was, yes, it’s not life-critical. If you’re using it as a digital babysitter, people expect their TV sets to just work. They’re supposed to just work. They’re not supposed to be, only work if some random thing on the other side of the world happens to be up or up right now. We wanted to make it feel like it just worked. You should just be watching a TV show and you should not be thinking about the fact that it’s rebuffering, and somewhere on the other side of the world something’s happening. You should just enjoy it.
We talked a bit about configuration management. Data center configuration management databases, woeful. They’re an example of a never consistent database. Anything in a CMDB, you can guarantee that the actual state of the data center is different to what’s currently in the CMDB. That’s about the only statement you can make. Cloud is a model driven architecture. If you query the cloud, you’re actually finding what is really there. It’s dependably complete. You can actually do things properly now that you could not do in a data center. This is a really significant thing. We built something called Edda, which is the stories of the gods. We have a lot of Norse god naming schemes going on at that time. We didn’t just put all of the cloud information that we scraped out of AWS in there, we put all the metadata about our services: when it came up, what it was doing, what version it was, and when it went away, again, instance-by-instance, was in there. Also, stuff about the request flow going back and forth between things that we were collecting with AppDynamics, we put that in there. The monkeys could look at this, but fundamentally, you had the ability to query the entire history of your infrastructure. You could say, something broke, and here’s an IP address and a log file. Which instance was that? I can go and find every instance that’s ever had that IP address. I can go and find out, which one was it? When was it here? All those kinds of things. Or changes to security groups. If you’re doing an audit log for security purposes, you can go see all these kinds of things. This is super powerful. I’m not sure if people really build this into their architectures nowadays. We did all kinds of things sitting on top of this. This is a lesson, I’m not sure if it really got learned, but it’s super powerful.
There’s another thing I did, we spent about an hour running some benchmark configurations. We were trying to figure out, so we’ve got Cassandra running at 48 instances. If we got a limit, what happens? We just kept doubling it up. After about an hour, we gave up and said, we hit a million requests a second, more than a million, that’s fine. That’s plenty. It’s way more than we’re going to need. It looks like it’s linear. Then we shut it down again. This benchmark config only existed for about an hour. This is something that I find really interesting, because this is a frictionless infrastructure experiment, you can go and try stuff out. I keep running into people that their cloud environment is so locked down, that they can’t just go and try something. That’s a sad thing. This benchmark was used by DataStax. It was advertised all over the internet. At the time it just kept popping up when I was browsing around web pages because they were targeting me for it.
Netflix Open Source Program
Netflix open source. This is interesting because open source has really become a common way to build a technology brand, influence the industry. Netflix wasn’t the first company to do this, but we did it very intentionally, and I think quite successfully. It was a project I kicked off, got management support for, drove. We had contests. We had logos. We had meetups. We had some of the best attended meetups. Hundreds of people would turn up at Netflix to hear about the open source program. It worked extremely well for us. I think other people then copied that. Capital One have a very good open source program, lots of other people did. This is an interesting approach. I then moved to Amazon later on, and redid some of the things I’d learned from running this program as well. Open source, why are we doing it? As the platform evolved, it went from bleeding edge innovation to common patterns. Then, what we really wanted to do was go to shared patterns, because if you get out ahead of the market, and then the market does something different, you’ve got to keep moving back to the mainstream. Netflix did end up off the mainstream a little bit, I think, in a few cases. This was a serious attempt to try and keep Netflix aligned with the rest of the industry by sharing what we were doing with the rest of the industry. Instead of running our wagon train across the prairie, and just trying to dodge between different hillocks, you lay down a railway line. This is why California came into being, there was a railway line from Chicago to Los Angeles, and they grew fruit in Los Angeles and shipped it back to Chicago. Lots of people in Chicago, say, it’s warm there, and I can go work on a fruit farm. That was what happened.
These are the goals for the Netflix open source program. Try to establish what we’re doing as best practice and standards. Hire, retain, and engage top engineers. That worked pretty well. Build a technology brand, which has worked stupendously well. It’s why I’m doing this talk today. Benefit from a shared ecosystem, get contributions from outside that helped Netflix. There are some pieces of ZooKeeper that Netflix built, a thing called curator that is now a top-level Apache project. Netflix originally built it. The engineer eventually left Netflix, and has carried on working on it. Netflix just gets to use this. They don’t have to go build it anymore. Doesn’t have to build this code, because everyone else decided it was good, and just maintains it for them.
What we were trying to do is add more use cases and more features and fix a few things. The thing that really didn’t work out here that we learned quite a lot from was it was too hard to deploy. There was a key internal component, which was the machine image. What we were running internally was a really hacked up version of CentOS. It was so hacked up, we didn’t want to share it. We were trying to move to a standardized version of Ubuntu, at the same time I was trying to get everything out. Because we didn’t have the final version, and we didn’t have engineering resources internally to go and just build a quick version that we could export, this whole thing was much too hard to deploy, for much too long. Eventually, this got fixed after about a year, but it was too difficult. One of the things you need to do is if you’re going to build something and open source it, think about the deployment, think about supporting it, and you really need a little bit of engineering resources to get good adoption going. I just love this acronym at the end, meantime, between idea and making stuff happen. You want to have low MTBIAMSH.
Docker Containers, and Microservices Patterns
I left Netflix in January 2014, joined Battery Ventures. One of the reasons I joined them was because Netflix had been using so much startup stuff, I got to know all the VCs, so I got to go hang out with the VCs. What actually happened in 2014 was Docker Containers started to take off as a pattern, which actually implemented a lot of the ideas that Netflix had built. Talking about a time when if you say, how much do people care about microservices? This deck I just showed you was using the word microservices back at a time when nobody else was. Then, it just became more popular over time. At the end of my time at Battery, just before I joined AWS, I did a mega deck with over 300 slides, which is a daylong workshop of everything I knew about microservice all carefully arranged into different chunks. This is excerpts from that deck. As I mentioned earlier, they thought we were crazy in ’09. They said it wouldn’t work in 2010. We were unicorns in 2011. 2012, they said, ok, we would like to do it. We can’t figure out how to get there. In 2013 we’d shared enough OSS code that quite a few people were actually trying to use it and trying to copy us. I think that was pretty influential.
Lessons Learned at Netflix, and the Innovation Loop
What did I learn at Netflix? Speed wins. Take friction out of product development. High trust, low process, no hand-offs between teams, APIs between teams. The culture, undifferentiated heavy lifting. Simple patterns with lots of tooling. Then, like I said, for benchmarking, Self-Service Cloud makes impossible things instant, like a million write per second benchmark that was done in an hour. This is the innovation loop that I was pushing at the time. You observe, orient, decide, act. You see the competitors do something or a customer pain point, you do some analysis. Your innovation moves to big data. You plan a response. You just do it. You share your plans. You don’t ask for permission. You share your plan so that other people can comment on them, so you get feedback on your plans but you don’t ask permission. You decide what you want to do. You build it out as incremental features. You do an automatic deploy, launch an A/B test. Then you measure the customers to see if they liked it, and you keep going around that loop. We go around that loop maybe once a week, or once every few weeks for a new feature or a new idea.
Non-Destructive Production Updates
One of the things here is non-destructive production updates. You can always launch new code, a new version of the code in production safely, and you can test it in production safely. People can’t figure out how we did that, so I’m going to explain it. It’s immutable code. Old code is still in service, you launch a new one alongside it as a new service group. The production traffic is talking to the old service group until you tell it to route some of the traffic to the new one. That means I can launch code, but I don’t actually have to send production customer traffic to there. I can actually do overrides at a very detailed level, so that I can basically create a test cell, or an environment so that the only people in that test cell, get the new experience through the new thing that I just deployed. The only first people in that test cell are developers and QA people. We just got to use Netflix, and we’re getting this new experience, and we’re seeing if it works. When we finally decide it works well enough, then we add a cohort of users to it, make sure that works, and eventually add everything else. That’s why you don’t have to wait for anyone else. You don’t need permission. You’re testing in production, you’re just putting it out. It’s completely safe to do that. This is really critical. At Netflix, we were accused of ruining the cloud, because we weren’t using Chef and Puppet to update our code in place by starting these new instances. We had a little fun with that. Ultimately, this is the pattern that Docker and containers went with. This idea of version-aware routing between services is another idea that seemed to get lost along the way. I know there’s lots of microservices into libraries for calling between services that don’t have this version-aware routing built into it.
Microservices
One of the things we were doing was cutting the cost and the size and the risk of change, and that sped everything up. Like I just said, I can push stuff to production, test in production completely safely. I can go faster. Chaos engineering, I’m being sure that this is going to work. I can go faster because of chaos engineering. This is the definition I had then of microservices, loosely coupled, service-oriented architecture with bounded context. If it isn’t loosely coupled, then you can’t independently deploy. If you don’t have bounded context, then you have to know too much about what’s around you. Part of the productivity here is I only need to understand this service and what it does, and I can successfully contribute code to the entire system. If you’re in a monolith, you need to understand pretty much the whole monolith to safely do anything in that monolith.
If you’re finding that you’re overcoupled, it’s because you didn’t create those bounded contexts. We used inverted Conway’s Law. We set up the architecture we wanted by creating groups that were that shape. We typically try to have a microservice do one thing, one verb, so that you could say, this service does 100-watts hits per second, or 1000-watts hits per second. It wasn’t doing a bunch of different things. That makes it much easier to do capacity planning and testing. It’s like, I’ve got a new version of the thing does more watts hits per second than the old one. That’s it, much easier to test. Mostly one developer producing the services. There’d be another developer buddied with them, code reviewing, and then either of them could support in terms of being on-call. In order to get off-call, you have to have somebody else that understands your code. You’d code review with some other people in your team, so that you could go off-call and they could support whatever you were currently doing. Every microservice was a build. We deployed in a container, which was initially Tomcat in a machine image, or it was Python or whatever in a machine image. Docker came along, and that’s a fine pattern. Docker copied this model. It’s all cattle, not pets. The data layer is replicated ephemeral instances. The Cassandra instances were also ephemeral, but there was enough of them that you never lost any data. There’s always at least three copies of all the data. In fact, when we went multi-region, there were nine copies of the data. If you lost a machine, it would rebuild itself from all the others. A whole bunch of books. I want to point out, “Irresistible APIs.” Kirsten Hunter was one of the API developers at Netflix, and I worked with her and I wrote the foreword for that book. Think about writing APIs that are nice to use. A bunch of other interesting books here.
What’s Missing? (Timeouts and Retries)
What’s missing? A whole bunch of advanced topics here. I’m going to talk about timeouts and retries. This is something else that got lost along the way. First of all, we’re running on TCP/IP, most of the time. TCP is connection oriented. The first time you try to make a call to someone, it sets up a connection, and then it sends the request. If it’s a secure connection, it does extra handshake first. Most of the time, at the higher level, we’re just trying to call another service so this gets lost in translation somewhere. Be really careful when thinking about connection timeout, which is one hop, it’s relatively short, versus request timeout, which is end-to-end through multiple hops. These are usually set up incorrectly. They’re usually global defaults. A lot of the time systems collapse with retry storms, just because the timeouts aren’t set up properly. Most people set timeouts that are far too long, and have far too many retries. I like to cut this back a lot. What this ends up with is services doing work that can never be used, and retry storms.
I’m going to try and illustrate this with some clunky diagrams that I built. Here’s a system that’s working. It makes a call. It gets a response. If anything breaks, the service in the middle, because it’s 2 retries and 2-second timeouts, the edge service is not going to respond for 6 seconds. The thing in the middle is going to be managing 9 outbound requests. I’ve got 9 threads running there instead of one. That’s going to run out of memory. It’s going to run out of threads. That service in the middle, which was perfectly fine, because there’s a downstream service, it’s going to keel over, and the edge service is going to keel over. You end up with this cascading problem that one failed service deep in the system causes the entire system to do work amplification, and to collapse. The only thing you need to do to get this to happen, is have the same timeout everywhere and have more than one retry. You have many retries. Even if you partially fail, and you fail the first time and you respond the second time, you’re still causing extra work in that service in the middle, which is never actually going to be used because it’s retrying, but the edge has already given up and called you again.
How do you fix this? You want a timeout budget that cascades. You want a large timeout at the edge, and you want to telescope. You want all of the timeouts that fit to fit inside that, like the pieces of a telescope that fit in, all the way to however deep it goes. If you have a fairly simple architecture, and you know what the sequence is, you can do it statically, but mostly you need some dynamic budget or deadline you pass with the request. Like, ok, I’m going to give you a 10-second timeout from the edge, that means 10 seconds in the future. Here’s my give-up time. I’m going to give up at this point in the future. Then somewhere deep in the system you get, I’m never going to be able to respond in the time, we’re past the deadline, let’s just chuck everything away. That kind of approach. The other thing is, don’t ask the instance the same thing, retry in a different connection. Here’s a better way of doing it. My edge service is 3 seconds. I’ve got a service in the middle of 1 second. There’s one retry. If I got a failed service, it’ll try it twice, it’s going to respond in 2 seconds. That’s before the edge times out. You’ve got a nice, effectively a fast-fail system, and it’s behaving itself.
Why did I try the same service? Just because that service is probably doing a garbage collector or something, and it’s just stopped talking to everybody for 10 seconds, or a minute, or something. There’s a whole bunch of other ones. What I want to do is call on a different connection that goes to a different service. My first request will fail, but my second one will tend to succeed. We built this in at Netflix. Again, I don’t know why everybody doesn’t do this. This sounds like it’s so obvious, and it’s so resilient. I think this is another lesson that wasn’t learned somewhere along the way. I still see lots of frameworks, lots of environments, having retry storms. We fixed it at Netflix more than a decade ago, and I wrote these slides in 2015 or so. You can’t get with the program. There’s a good book on systems thinking by Jamshid Gharajedaghi. “We see the world as increasingly more complex and chaotic, because we use inadequate concepts to explain it. When you understand something, you no longer see it as chaotic or complex.” Best example of this is just driving a car through a city. If you’ve never seen a car, you’ve never seen a road, you have no idea what’s going on, it’s chaotic. It’s complex. It’ll freak you out completely. Pretty much any functioning adult is able to do this, so it’s not seen as chaotic or complex.
Microservices Is Many Tactics
We tried microservices, and it didn’t work. There’s all kinds of people complaining that maybe we should stop doing microservices because it’s not working for us. Why isn’t it working for you? Maybe you aren’t a unicorn, maybe you’re just a donkey with a carrot tied to its forehead. You’re a wannabe unicorn. Microservices is one of many tactics that reinforce each other, and that forms a system that is fast, resilient, scalable. If it isn’t just microservice, it’s many tactics. Let’s just look at a few of these. You’re too slow. You’re probably using chattier, inefficient protocols or your system boundaries aren’t right. If everything needs to happen in milliseconds, you build one big service that does this. It’s how ad servers work, they have to respond super quick. Yes, a monolith, because you’re responding in milliseconds, and you have to. Or, you’re doing high frequency trading, sure, build that monolithically, and be really careful how you build it. If you’re responding to building a web page for somebody on the other side of the world, you don’t need to put everything in one instance. You’re more interested there in getting the fast development practices and the ability to innovate more quickly.
If you’re not resilient, you probably haven’t fixed retry storms. Or maybe your developers are writing unreliable code, and you should put them on-call. Or you’re not using chaos engineering, you’re not leveraging AZs properly. You’re not scalable, maybe you didn’t denormalize your data model. You’ve got some SQL query things sitting in the backend that keeps keeling over. Maybe you haven’t tuned the code for vertical scaling, and your code just is not running efficiently on the instances at all. That leads into overall efficiency, like cost efficiency, in particular. You should be autoscaling so deeply, that the average utilization across all of your instances is like 40% to 50%, average. What I see is single digit averages in almost all the cloud environments I see. The difference between 10% and 40% is 4x. That means you’re spending four times as much as you should. Forget all of the buying reservations and savings plans and things, just get your utilization to double and your cloud bill will halve. You get there by autoscaling super deeply, turning things off, maybe using serverless so you’re 100% utilized for the serverless functions. There’s lots of things you can do. This is, I think, the biggest cost lever that I don’t see people using nowadays. If you left out most of the system, it’s not surprising. It’s not going to work for you.
Systems Thinking at Netflix and Beyond
Systems thinking at Netflix and beyond. This is a talk I gave at a French conference that was held in the Louvre, at The Carrousel du Louvre. A whole bunch of things talking about from a systems thinking approach, what is Netflix doing? It is driving higher talent density, by a culture optimized for fully formed adults. Netflix had no graduate hires, and no interns. You’re building an Olympic team. You wouldn’t have high school athletes on an Olympic team unless they were absolutely operating at that Olympic level. You got to go through the leagues and get better, if you want to play for the top league and the top team in the top league. That’s the attitude. There was yes, definitely explicitly skimming off the top talent that they could offer for everybody. You spend 5, 10 years at Google or Facebook or something, and then come work at Netflix. It’s not a family. This became a pretty famous quote, “We can’t copy Netflix, because it has all these superstar engineers, those Olympic athletes, we don’t have those people.” The guy that told me this, I looked at his badge, and we just hired somebody from them. I said, “We hide them from you, but we got out of their way.” We got vastly more out of the engineers that we hired than the people that previously employed them. You can say, is it a 10x engineer, or whatever? A lot of the 10x engineer is getting out of the way of somebody that is just being systematically slowed down and made unproductive in their previous place. Whereas you get to Netflix, Ben Christensen said this. He started doing something, we’re just egging him on. It’s like, we just pushed him out, grew wings and just flew. This is the guy that built Hystrix. He actually felt like that, instead of being pushed back, he was being pulled forward. That was probably the most distilled quote I’ve seen of that.
It’s a little complicated here. Purposeful systems representing the system’s view of development. We assume plurality in all three dimensions: function, structure, and process. What he was really saying here, there’s multiple ways to do things. There’s multiple ways to look at functions, multiple structures, multiple processes. If you lock everything down to one function, one structure, one process, you’re not actually representing a useful system that is fit for purpose. There’s another way of looking at this, which is W. Ross Ashby’s Law of Requisite Variety, because if you’re trying to manage something, the thing that’s managing it has to have at least as much complexity as the thing it’s trying to manage. One of the problems here is people tend to simplify, and they do efficiency drives, everything looks too complicated. You get a new CIO in, and they try and simplify everything, and they kill off everything, and they make it so it doesn’t work anymore. Because the complexity of the business was being managed by complexity. The ability to evolve means that things are ambiguous and unstable. It’s chaos. It’s complexity. If you can manage it, then you can go super-fast.
How does that work? This is another quote from the Netflix culture deck, “If you want to build a ship, don’t drum up the people to gather wood, divide the work and give orders. Instead, teach them to yearn from the vast and endless sea.” The point here is that, go, we want to build TV for the world. We’re building the first global TV station. Get inspired by that, go and figure out how to do that, rather than detailed, step-by-step things. Then, this is like the fourth version of Conway’s Law from 1968. This talk had excerpts from all these decks. A bunch of different things, you can go find them. They’re either on SlideShare or on my GitHub site. I’m going to take a PDF of this entire deck, a final version and put that on GitHub as well. You can also find me giving a lot of these talks, videos of this on YouTube, and I try to build some links there.
Conclusion
I started off with the retrospectives book by Aino. One of the antipatterns that she highlights in that book is the prime directive ignorance. You ignore the prime directive, because you didn’t protect unprepared organizations from the dangers of introducing advanced technology, knowledge, and values before they were ready. Maybe at Netflix, we came up with stuff and we shared it with people and a few people took it home and tried to implement it before their organizations were really ready for it. I think we had a bit of that happening, as well as all the cool companies that actually did pick it up and take it to new heights, and we all learn from each other. Just a little worry about the dangers of coming home from a conference and saying, I saw a new thing and let’s go do it.
See more presentations with transcripts
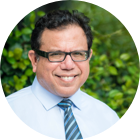
MMS • Robert Krzaczynski
Article originally posted on InfoQ. Visit InfoQ
.NET Lambda Annotations Framework is now generally available. Last year this framework was introduced in the preview version. It simplifies AWS Lambda development for .NET developers using custom attributes and source generators, allowing easy translation into standard Lambda programming during compilation. Moreover, it supports Dependency Injection and CloudFormation integration, improving Lambda function creation and deployment in AWS.
The .NET Lambda Annotations Framework is a new approach for .NET developers to build AWS Lambda functions effortlessly. By leveraging custom C# attributes and source generators, the framework translates annotated Lambda functions into the standard Lambda programming model during compilation, leaving the Lambda runtime unaffected. No additional tools are needed except for the NuGet Annotations Lambda package, making it compatible with various Lambda deployment tools utilizing CloudFormation. This includes AWS Toolkit for Visual Studio, Lambda .NET CLI, or SAM. With this natural programming model, developers can streamline Lambda function creation and deployment in AWS, boosting development productivity significantly.
In order to get started with the .NET Lambda Framework, the following things must be installed and configured: Visual Studio 2022, the latest version of AWS Toolkit for Visual Studio, an IAM user with the appropriate permissions to develop APIs using the Amazon API Gateway pods, AWS Lambda Functions, and Amazon Simple Storage Service. If an IDE other than Visual Studio is used, the same project template can be created from the Amazon.Lambda.Templates NuGet package:
dotnet new install Amazon.Lambda.Templates
dotnet new serverless.Annotations --output LambdaAnnotations
Lambda function using the Lambda Annotations Framework (Source: AWS Developer Tools Blog)
The created project from a template contains a collection of Lambda functions, defined in the Function.cs
file. The LambdaFunction attribute designates the C# method as a Lambda function, synced with the CloudFormation template. Moreover, the HttpApi
attribute incorporates the API Gateway event configuration into the CloudFormation template. This integration ensures smooth deployment and synchronization of Lambda functions with the API Gateway, all orchestrated through the CloudFormation infrastructure.
The project contains a serverless.template file that serves as a CloudFormation template for implementing Lambda functions. When a C# language method is tagged with the LambdaFunction attribute, it dynamically creates a matching declaration in the template.
The .NET community is wondering about the usage of this solution. A Reddit user left a question/insight:
Tempted to try this, but cold starts are a big pain point already with .Net Lambda, and splitting an API into a Lambda per endpoint would only exacerbate this?
More details about .NET Lambda Annotations Framework can be found on the GitHub page or Developer Documentation.
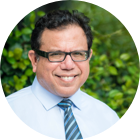
MMS • RSS
Posted on mongodb google news. Visit mongodb google news
MongoDB, Inc. (NASDAQ:MDB – Get Free Report) saw some unusual options trading activity on Wednesday. Traders acquired 23,831 put options on the stock. This represents an increase of approximately 2,157% compared to the typical volume of 1,056 put options.
Insider Activity
In other MongoDB news, Director Dwight A. Merriman sold 2,000 shares of the firm’s stock in a transaction dated Thursday, May 4th. The shares were sold at an average price of $240.00, for a total value of $480,000.00. Following the completion of the transaction, the director now directly owns 1,223,954 shares in the company, valued at $293,748,960. The sale was disclosed in a filing with the SEC, which is accessible through this link. In other MongoDB news, CRO Cedric Pech sold 360 shares of the firm’s stock in a transaction dated Monday, July 3rd. The stock was sold at an average price of $406.79, for a total transaction of $146,444.40. Following the completion of the sale, the executive now directly owns 37,156 shares in the company, valued at approximately $15,114,689.24. The sale was disclosed in a filing with the Securities & Exchange Commission, which is available at this hyperlink. Also, Director Dwight A. Merriman sold 2,000 shares of the company’s stock in a transaction dated Thursday, May 4th. The shares were sold at an average price of $240.00, for a total value of $480,000.00. Following the completion of the transaction, the director now owns 1,223,954 shares in the company, valued at approximately $293,748,960. The disclosure for this sale can be found here. In the last quarter, insiders sold 116,427 shares of company stock worth $41,304,961. Corporate insiders own 4.80% of the company’s stock.
Institutional Investors Weigh In On MongoDB
A number of institutional investors have recently bought and sold shares of the business. Victory Capital Management Inc. boosted its holdings in MongoDB by 18.3% during the second quarter. Victory Capital Management Inc. now owns 39,231 shares of the company’s stock valued at $16,124,000 after purchasing an additional 6,059 shares in the last quarter. Virtu Financial LLC bought a new position in shares of MongoDB during the 2nd quarter worth approximately $1,323,000. B. Riley Wealth Advisors Inc. boosted its stake in MongoDB by 12.9% in the 2nd quarter. B. Riley Wealth Advisors Inc. now owns 1,645 shares of the company’s stock valued at $676,000 after buying an additional 188 shares in the last quarter. GAM Holding AG grew its position in MongoDB by 32.7% in the second quarter. GAM Holding AG now owns 30,696 shares of the company’s stock valued at $12,616,000 after acquiring an additional 7,560 shares during the period. Finally, IFM Investors Pty Ltd raised its stake in MongoDB by 15.2% during the second quarter. IFM Investors Pty Ltd now owns 14,012 shares of the company’s stock worth $5,759,000 after acquiring an additional 1,853 shares in the last quarter. Institutional investors and hedge funds own 89.22% of the company’s stock.
Wall Street Analysts Forecast Growth
A number of brokerages have issued reports on MDB. Mizuho lifted their target price on shares of MongoDB from $220.00 to $240.00 in a research note on Friday, June 23rd. Guggenheim cut MongoDB from a “neutral” rating to a “sell” rating and lifted their price objective for the company from $205.00 to $210.00 in a research report on Thursday, May 25th. They noted that the move was a valuation call. Sanford C. Bernstein upped their target price on MongoDB from $257.00 to $424.00 in a research report on Monday, June 5th. 22nd Century Group reiterated a “maintains” rating on shares of MongoDB in a report on Monday, June 26th. Finally, JMP Securities increased their price objective on MongoDB from $400.00 to $425.00 and gave the stock an “outperform” rating in a report on Monday. One research analyst has rated the stock with a sell rating, three have issued a hold rating and twenty have given a buy rating to the company. According to data from MarketBeat.com, MongoDB presently has a consensus rating of “Moderate Buy” and a consensus target price of $378.09.
MongoDB Price Performance
Shares of MongoDB stock opened at $403.58 on Friday. The company has a debt-to-equity ratio of 1.44, a current ratio of 4.19 and a quick ratio of 4.19. The company has a market cap of $28.48 billion, a PE ratio of -86.42 and a beta of 1.13. The firm has a fifty day moving average price of $372.82 and a two-hundred day moving average price of $273.58. MongoDB has a fifty-two week low of $135.15 and a fifty-two week high of $439.00.
MongoDB (NASDAQ:MDB – Get Free Report) last released its earnings results on Thursday, June 1st. The company reported $0.56 earnings per share for the quarter, beating the consensus estimate of $0.18 by $0.38. The business had revenue of $368.28 million during the quarter, compared to analysts’ expectations of $347.77 million. MongoDB had a negative net margin of 23.58% and a negative return on equity of 43.25%. The firm’s revenue was up 29.0% on a year-over-year basis. During the same quarter last year, the company earned ($1.15) earnings per share. Analysts expect that MongoDB will post -2.8 EPS for the current year.
MongoDB Company Profile
MongoDB, Inc provides general purpose database platform worldwide. The company offers MongoDB Atlas, a hosted multi-cloud database-as-a-service solution; MongoDB Enterprise Advanced, a commercial database server for enterprise customers to run in the cloud, on-premise, or in a hybrid environment; and Community Server, a free-to-download version of its database, which includes the functionality that developers need to get started with MongoDB.
Recommended Stories
Receive News & Ratings for MongoDB Daily – Enter your email address below to receive a concise daily summary of the latest news and analysts’ ratings for MongoDB and related companies with MarketBeat.com’s FREE daily email newsletter.
Article originally posted on mongodb google news. Visit mongodb google news
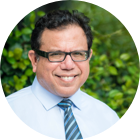
MMS • Karsten Silz
Article originally posted on InfoQ. Visit InfoQ
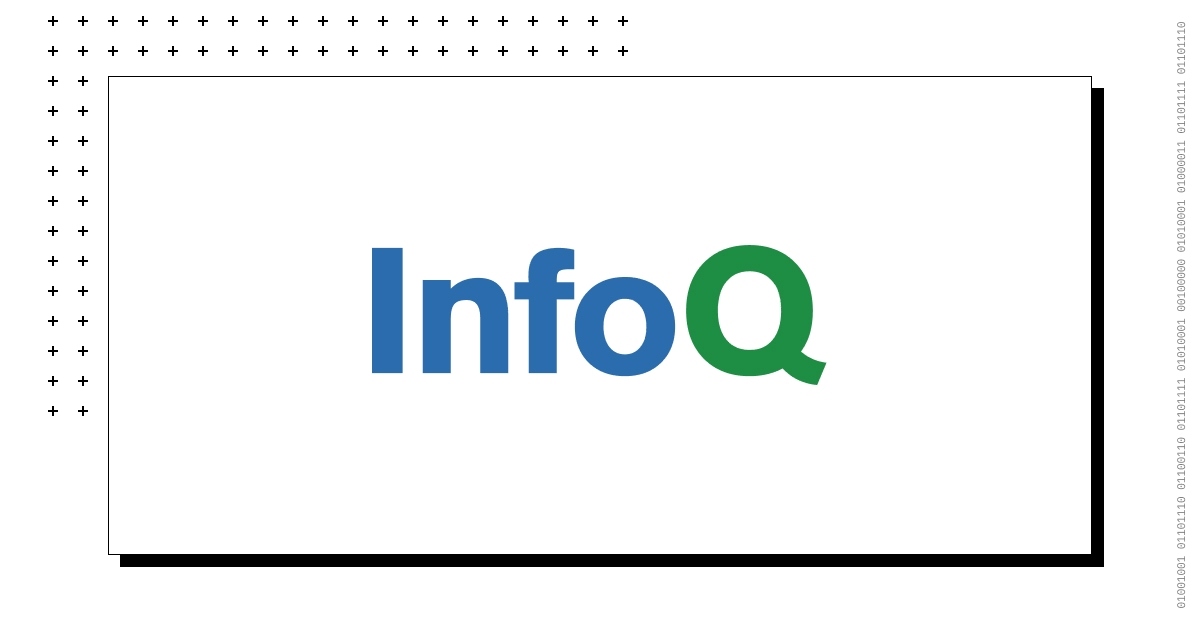
The GraalVM Native Image Ahead-of-Time (AOT) compiler for Java creates native executables that start much faster, use less CPU and memory, are more secure, and have a smaller disk size than traditional Java applications with a Just-in-Time (JIT) compiler. With Oracle GraalVM for Java 17 and 20, Oracle kills the Enterprise Edition and makes its three performance-boosting features free for production use under a new license. That pushes native Java executables closer to the speed of Java applications with a JIT compiler. GraalVM will simultaneously release with new Java versions, supporting the current Java and LTS versions, and the previous LTS version for one additional year.
The first two performance boosters copy Just-in-Time Java features: the G1 garbage collector and compressed object headers and pointers. The third one adapts a Just-in-Time Java feature: Profile-Guided Optimizations (PGO) collect profiling information at runtime. But unlike the JIT compiler, GraalVM Native Image uses this profiling data at build time for application-specific optimizations, not at runtime.
The new GraalVM Free Terms and Conditions (GFTC) license makes these former Enterprise Editions free for production use. But that’s “free as in free beer” – it’s not an open-source license. On the other hand, the GraalVM Community Edition (CE) is still available under the open-source GNU General Public License (GPL). But the CE lacks these three closed-source performance boosters. Further details may be found at the Oracle FAQ page.
Oracle benchmarked Native Image with G1 and PGO against the HotSpot C2 JIT compiler in the Spring PetClinic sample application on Java 20. Native Image started in 0.22 seconds, just 3% of HotSpot’s 7.18 seconds. Memory usage measured with the Resident Set Size (RSS) was 694 MB – 40% of HotSpot’s 1,751 MB. But in peak throughput, Native Image still trailed the JIT compiler with 10,249 requests/s, 80% of the 12,800 requests/s HotSpot achieved. Still, Oracle says, “In many cases, we see AOT even ahead of JIT for peak throughput.”
Combining memory usage and requests/s, Native Image reached 14,780 requests/GB/s, twice HotSpot’s 7,310 requests/GB/s. Oracle pointed out that this improved efficiency can save cloud costs. Running more requests may require more CPU power if processing these requests is CPU-constrained.
Native Image solves the “long-term pain points of Java’s slow startup time, slow time-to-peak performance, and large footprint,” as Java Language Architect Mark Reinhold stated in April 2020. But using GraalVM Native Image comes at the price of some possibly showstopping constraints and a more expensive troubleshooting process. The OpenJDK project Coordinated Restore at Checkpoint (CRaC) only solves startup time and slow time-to-peak performance but comes with fewer constraints.
The GraalVM team recommends using an application framework with Native Image. Helidon, Micronaut, Quarkus, and Spring Boot (since version 3.0 from November 2022) support GraalVM in production. The official GraalVM Community Survey 2022 showed that Spring Boot is the most popular, with 49% usage among respondents, up from 34% last year. “No framework” came in second at 26%. Quarkus placed third at 19%, up slightly from 18% year-over-year. “Other” was fourth at 15%, down from 25% the year before. And Micronaut was fifth at 14% versus 12% in 2021.
The GraalVM project is part of Oracle Labs. Apart from Native Image, it also contains the Graal JIT compiler, written in Java. The Graal JIT compiler now includes the ZGC garbage collector. Oracle announced last year that these two Java compilers would join OpenJDK. Back then, the GraalVM Enterprise Edition was not expected to move there.
The other GraalVM projects did not move: The Truffle framework for running languages like JavaScript or Python on GraalVM; and Java on Truffle, a Java replacement for the entire Hotspot VM. They received new features in this GraalVM release.
Native Image was improved with various slight speed boosts in this release, including machine learning for better branch prediction. The Native Image developer experience has also improved with: reduced build process memory footprint, automatic build environment setup in Windows, more user-friendly error handling, and reports with build details. Native Image can now also create a Software Build of Materials (SBOM).
Native Image enabled remote management over JMX as an experimental feature in this release and added three new JDK Flight Recorder (JFR) events. AWT now works in Native Image on Linux. That leaves macOS as the last platform without support for Java desktop applications.
Native Image Bundles are new and enable reproducible builds. Alongside the native executable, such bundles contain a JAR file of the application and the necessary build information. The GraalVM JDK also now includes Native Image by default and has stable download URLs, easing life for container image authors and CI/CD systems.
Alina Yurenko, Developer Advocate for GraalVM at Oracle Labs, was happy to answer some questions for InfoQ.
InfoQ: This is the first GraalVM release after the GraalVM Java compilers started their move to OpenJDK. How has that move been going so far?
Alina Yurenko: At JavaOne 2022, we announced our plans to contribute the most applicable portions of the GraalVM just-in-time (JIT) compiler and Native Image technology to OpenJDK. A few months ago, we announced Project Galahad, where this work will begin. However, for GraalVM users, the first tangible result of this effort is aligning the GraalVM release schedule with OpenJDK’s schedule.
InfoQ: The initial announcement (see the last question) didn’t suggest any changes for the GraalVM Enterprise Edition. Now many of its EE features, such as PGO and G1, are available for free under a new license. What prompted this change?
Yurenko: The initial announcement and subsequent communications did talk about the alignment with OpenJDK: “The plan is to align all the GraalVM technologies with Java both from a release perspective and from a licensing perspective.” Having GraalVM releases that support the latest Oracle-designated LTS release under a free license is a crucial part of the alignment.
InfoQ: The distribution is now called “Oracle GraalVM”. What do you know about the plans of other organizations to ship GraalVM distributions? We have many OpenJDK distributions, after all.
Yurenko: There are many GraalVM distributions as well. Oracle provides the GraalVM Community Edition under an open-source license. And third parties such as Gluon, Red Hat, and Bellsoft provide their forks of GraalVM CE-based code. We would not be surprised to see further distributions become available over time.
InfoQ: Why and when should Java developers consider using the Graal JIT compiler over the standard Hotspot JIT compiler?
Yurenko: Here are some areas where developers and applications may see benefits:
- Partial escape analysis. Removes unnecessary object allocations by performing scalar replacement in branches where the object does not escape and ensuring that the object exists in the heap in branches where it must escape. This reduces both the memory footprint of the application and the CPU load incurred by GC. The impact of this optimization is even larger for more modern Java code that uses lambdas or otherwise allocates many short-lived objects.
- Auto-vectorization. GraalVM can automatically recognize various loop structures and perform automatic vectorization to provide additional performance benefits where possible.
These advantages and resulting performance benefits have, for example, been observed by researchers working on the Renaissance benchmark suite, the Facebook engineering team, and Ionut Balosin and Florin Blanaru in their “JVM Performance Comparison for JDK 17” article.
Any Java developer using the default JIT compiler should consider giving the Graal compiler a try and measure how much it improves the performance of their workloads. Generally, predicting how much performance users can gain from switching to Graal is challenging, as every workload is different and has different performance profiles and goals.
InfoQ: A Native Image practitioner recommended using Java with a JIT compiler during development last year. He said using Native Image on developer machines and debugging native Java executables should be the exception. What are your recommendations on using GraalVM Native Image during development today?
Yurenko: Using Java with a JIT compiler during development is correct: Use it to debug your core program logic – and debug early. Fast debug/test cycles are one of the strengths of the Java ecosystem. Most developers spend most of their development time here.
However, once your basic logic works and you want to debug and test in more production-like conditions with a broader set of tests, you might want to try Native Image. You probably need to test in the cloud rather than using mocked versions of cloud services then. You aren’t going to notice the difference in Java programs with Native Image except under more production-like conditions anyway.
Where the Native Image build fits into your development process depends on the likelihood of encountering problems with building a native image. Suppose you use a framework like Spring Boot 3 or Micronaut with library dependencies known to work with Native Image. In that case, you probably can do the Native Image build late in your development cycle and only in your CI/CD pipeline since Native Image builds won’t create or reveal more problems. Testing with Native Image then helps you understand how your program works at scale or in cloud conditions. But if you use older libraries that require the native image tracing agent to handle dynamic Java features like reflection, you do more native image builds on developer machines to debug configuration problems with native executables. Then you will use Native Build Tools and native debugging.
InfoQ: How do you define success for the GraalVM project? And how do you measure it?
Yurenko: The GraalVM project was created to provide a universal language runtime that can be embedded in any environment in various modes and that leverages the strengths of the Java ecosystem. Much of the initial research focused on demonstrating that we can run multiple programming languages with comparable efficiency to the single-language runtimes (see our work on R, Ruby and Java). We also demonstrated that we could embed GraalVM into various environments, from mobile devices to databases. The goal of Native Image is to marry the best of traditional Java with the benefits of native code, such as lower startup and footprint, and more predictable behavior.
As we have deployed GraalVM technologies into production in the past five years, the first step was the Hippocratic oath of system software — do no harm (tolerate no regression). Initially, we focused on the headline benchmarks of peak throughput. More recently, we’ve worked on other metrics, like memory footprint for both the runtime and compiler, build times, debuggability, and observability. We’ve also worked on the high level of interoperability that the Java ecosystem expects.
We’ve made tremendous progress over the last decade. Graal features are ready to be used in production. Native image build times have come down a lot and are reasonable for most projects. Startup time for our JIT compiler and peak throughput for native image-compiled binaries are better for many workloads. Plus, Oracle GraalVM (the former EE) is now free for production use.
Most importantly, GraalVM is now getting broad ecosystem support from other framework vendors. All major Java microservices frameworks, such as Spring Boot, Micronaut, Quarkus and Helidon, offer first-class support for GraalVM Native Image and the most popular cloud providers. More than 150 libraries and frameworks have adopted Native Image. 1,300 projects use GraalVM’s GitHub action on GitHub alone, and you can also find a list of 30+ language implementations built on top of GraalVM’s Truffle framework.
So, we certainly can claim excellent success for the project so far. Meanwhile, the Oracle Labs team is using the experience of GraalVM on various cloud development and deployment features. Be sure to follow our social media to keep updated on any announcements.
InfoQ: The GraalVM team gets feedback from many sources and could work in many areas – the Java compilers, the Truffle framework and its languages, the developer experience, compatibility with libraries and frameworks, etc. How does the team analyze feedback and prioritize work, especially now that it’s part of OpenJDK?
Yurenko: Oracle looks at feedback related to GraalVM through the lens of research and product. Our engineering team is directly engaged with the community on social media, involved in our GitHub repos, working on new PRs, resolving issues, and reviewing community contributions. We aim to reduce developer pain points where most developers are affected and add new technologies with the most impact. Of course, as a commercial Oracle product, we also have to meet our customers’ expectations for our regular support terms and conditions.
Embedded JavaScript in the JVM and other environments, like the Oracle Database and NetSuite, is also getting much attention. We continue to track improvements in both Java and JavaScript standards and the increasing use of WASM in JavaScript programs. GraalPy is also one of our priorities, and it was exciting that we were recently able to pass the PyTorch test suite with the JVM. We are getting optimistic that GraalPy will be a reasonable choice for Python developers doing data science in the next year. Embedded use cases are also on our radar: Oracle will invest in embedding GraalVM in more products shortly, and third parties like Enso are doing so as well. Beyond all those sensible product-oriented directions, Oracle Labs will continue to use GraalVM as the basis for research projects that are much further out there, and we expect only to gain momentum there.
The GraalVM home page provides more information on the Java compilers and the other GraalVM projects. GraalVM for Java 21 will release simultaneously with Java 21 on September 19, 2023, ending support for Java 20. The last GraalVM release for Java 17 is planned for October 24, 2023. GraalVM intends to ship simultaneously with the next non-LTS Java version 22 on March 19, 2024.
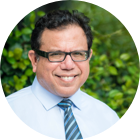
MMS • RSS
Posted on mongodb google news. Visit mongodb google news
MongoDB, Inc. (NASDAQ:MDB – Get Free Report) saw some unusual options trading activity on Wednesday. Traders bought 23,831 put options on the stock. This is an increase of 2,157% compared to the typical daily volume of 1,056 put options.
Insider Buying and Selling at MongoDB
In other MongoDB news, CAO Thomas Bull sold 516 shares of the company’s stock in a transaction dated Monday, July 3rd. The shares were sold at an average price of $406.78, for a total value of $209,898.48. Following the completion of the transaction, the chief accounting officer now owns 17,190 shares in the company, valued at $6,992,548.20. The sale was disclosed in a legal filing with the SEC, which is available at this hyperlink. In related news, Director Dwight A. Merriman sold 2,000 shares of the company’s stock in a transaction dated Thursday, May 4th. The shares were sold at an average price of $240.00, for a total transaction of $480,000.00. Following the completion of the transaction, the director now owns 1,223,954 shares in the company, valued at approximately $293,748,960. The transaction was disclosed in a legal filing with the SEC, which can be accessed through the SEC website. Also, CAO Thomas Bull sold 516 shares of the stock in a transaction dated Monday, July 3rd. The shares were sold at an average price of $406.78, for a total transaction of $209,898.48. Following the completion of the sale, the chief accounting officer now owns 17,190 shares of the company’s stock, valued at approximately $6,992,548.20. The disclosure for this sale can be found here. Over the last three months, insiders have sold 116,427 shares of company stock valued at $41,304,961. 4.80% of the stock is owned by insiders.
Institutional Investors Weigh In On MongoDB
A number of institutional investors have recently bought and sold shares of the stock. Capital Advisors Ltd. LLC grew its stake in MongoDB by 131.0% during the second quarter. Capital Advisors Ltd. LLC now owns 67 shares of the company’s stock worth $28,000 after buying an additional 38 shares during the period. Tealwood Asset Management Inc. purchased a new position in shares of MongoDB in the second quarter valued at $202,000. Simplicity Solutions LLC boosted its stake in shares of MongoDB by 2.2% in the second quarter. Simplicity Solutions LLC now owns 1,169 shares of the company’s stock valued at $480,000 after purchasing an additional 25 shares during the period. Waycross Partners LLC purchased a new position in shares of MongoDB in the second quarter valued at $709,000. Finally, McGlone Suttner Wealth Management Inc. purchased a new position in shares of MongoDB in the second quarter valued at $201,000. 89.22% of the stock is currently owned by hedge funds and other institutional investors.
MongoDB Stock Up 1.3 %
MDB stock traded up $5.42 during trading on Thursday, reaching $410.56. 277,829 shares of the company were exchanged, compared to its average volume of 1,746,527. MongoDB has a 52-week low of $135.15 and a 52-week high of $439.00. The stock’s 50 day moving average price is $365.22 and its 200 day moving average price is $269.99. The company has a quick ratio of 4.19, a current ratio of 4.19 and a debt-to-equity ratio of 1.44.
MongoDB (NASDAQ:MDB – Get Free Report) last posted its quarterly earnings data on Thursday, June 1st. The company reported $0.56 earnings per share for the quarter, beating analysts’ consensus estimates of $0.18 by $0.38. The business had revenue of $368.28 million during the quarter, compared to analyst estimates of $347.77 million. MongoDB had a negative net margin of 23.58% and a negative return on equity of 43.25%. The company’s revenue was up 29.0% on a year-over-year basis. During the same quarter in the previous year, the business posted ($1.15) earnings per share. As a group, equities research analysts forecast that MongoDB will post -2.8 EPS for the current year.
Analysts Set New Price Targets
A number of equities analysts recently weighed in on MDB shares. KeyCorp increased their price objective on shares of MongoDB from $372.00 to $462.00 and gave the stock an “overweight” rating in a research report on Friday, July 21st. VNET Group reiterated a “maintains” rating on shares of MongoDB in a report on Monday, June 26th. Capital One Financial started coverage on shares of MongoDB in a report on Monday, June 26th. They set an “equal weight” rating and a $396.00 target price on the stock. 22nd Century Group reiterated a “maintains” rating on shares of MongoDB in a report on Monday, June 26th. Finally, William Blair reiterated an “outperform” rating on shares of MongoDB in a report on Friday, June 2nd. One research analyst has rated the stock with a sell rating, three have given a hold rating and twenty have issued a buy rating to the stock. Based on data from MarketBeat, the company presently has a consensus rating of “Moderate Buy” and an average target price of $378.09.
About MongoDB
MongoDB, Inc provides general purpose database platform worldwide. The company offers MongoDB Atlas, a hosted multi-cloud database-as-a-service solution; MongoDB Enterprise Advanced, a commercial database server for enterprise customers to run in the cloud, on-premise, or in a hybrid environment; and Community Server, a free-to-download version of its database, which includes the functionality that developers need to get started with MongoDB.
See Also
This instant news alert was generated by narrative science technology and financial data from MarketBeat in order to provide readers with the fastest and most accurate reporting. This story was reviewed by MarketBeat’s editorial team prior to publication. Please send any questions or comments about this story to contact@marketbeat.com.
Before you consider MongoDB, you’ll want to hear this.
MarketBeat keeps track of Wall Street’s top-rated and best performing research analysts and the stocks they recommend to their clients on a daily basis. MarketBeat has identified the five stocks that top analysts are quietly whispering to their clients to buy now before the broader market catches on… and MongoDB wasn’t on the list.
While MongoDB currently has a “Moderate Buy” rating among analysts, top-rated analysts believe these five stocks are better buys.
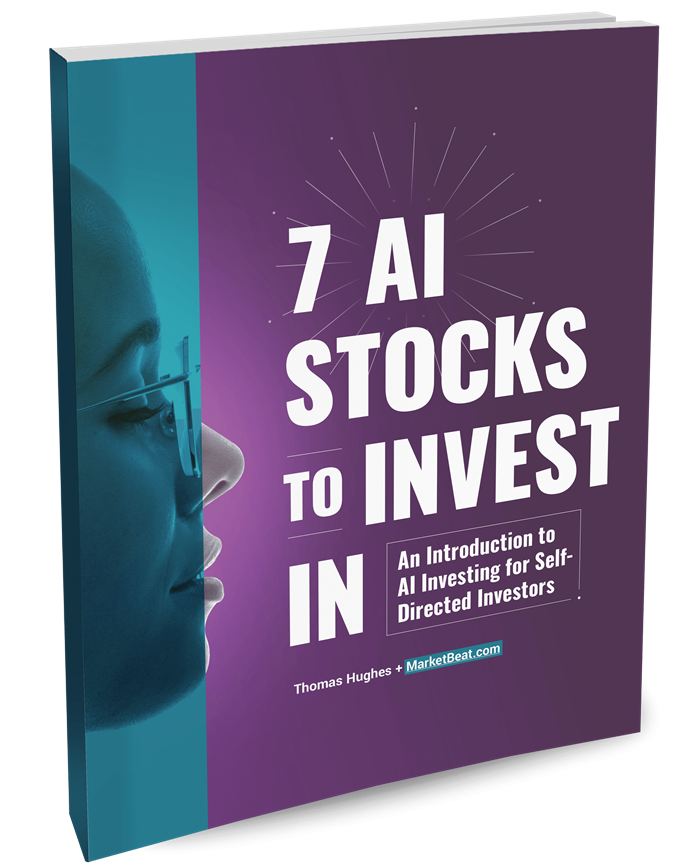
As the AI market heats up, investors who have a vision for artificial intelligence have the potential to see real returns. Learn about the industry as a whole as well as seven companies that are getting work done with the power of AI.
Article originally posted on mongodb google news. Visit mongodb google news
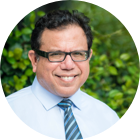
MMS • Sergio De Simone
Article originally posted on InfoQ. Visit InfoQ
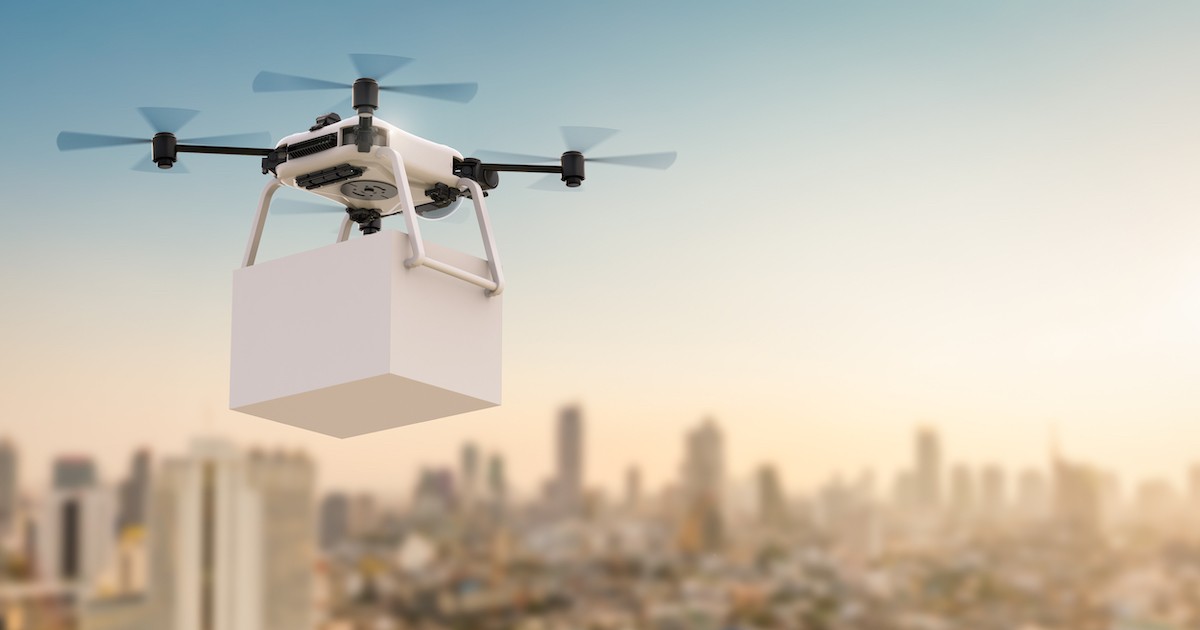
Android Studio Giraffe is now stable, bringing in the new IntelliJ 2022.3, a new IDE look and feel, improved Live Edit, Compose animation previews, and more.
Ten years after its initial release in 2013, Android Studio remains the IDE to go for Android development. Its latest release introduces a number of changes in distinct areas, including IDE enhancements, coding productivity, and build system improvements.
Android Studio Giraffe sports a new opt-in IDE look and feel aimed at reducing visual complexity. It strives to simplify access to the most commonly used features while making more complex functionality easily accessible when needed but less prominent in normal use. In addition, it provides a new theme that makes the IDE visual look more modern:
With the Giraffe release, we’ve started adopting the new UI, with several Android Studio specific changes, such as optimizing the default main toolbar and tool windows configurations for Android and refreshing our iconography in the style.
The new IDE also includes an updated Device Explorer, which enables inspecting files and processes of any connected device, including the possibility of copying or deleting files, killing processes or attaching the debugger to a running process.
On the front of code productivity, Android Studio Giraffe offers the possibility of previewing UI changes in composables without re-deploying the app to the simulator or physical device. This feature can be enabled via Settings / Editor / Live Edit and requires the Android Gradle Plugin (AGP) 8.1 or higher and Jetpack Compose Runtime 1.3.0 or higher.
Related to preview facilities, Compose animation preview has got support for a number of additional Compose APIs, including animate*AsState, CrossFade, rememberInfiniteTransition, and AnimatedContent. Animations can be played, paused, scrubbed, and so on.
A final help to code productivity comes from the new Android SDK Upgrade Assistant.
The new Android SDK upgrade assistant lets you see the steps required to upgrade the targetSdkVersion, or the API level that your app targets, directly in the IDE.
The assistant will show all information related to the upgrade option you select, so you won’t need to browse that information separately and is able to highlight the major breaking changes for each migration step.
Speaking of the build system, you can now use Kotlin DSL in Gradle build scripts and leverage its compile-time checking as well as consolidate all of your project code under one single language.
Additionally, we’ve also added experimental support for TOML-based Gradle Version Catalogs, a feature that lets you manage dependencies in one central location and share dependencies across modules or projects.
As a final note, Android Studio Giraffe can show dependency download info while Gradle is syncing. This will allow you to detect inefficiencies in your repository configuration.
There is much more to Android Studio Giraffe than what can be covered here. If interested in the full detail do not miss the official announcement.
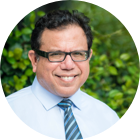
MMS • RSS
Posted on mongodb google news. Visit mongodb google news
July 27, 2023 – MongoDB Inc. (NASDAQ:MDB), a popular American technology company specializing in software products for database management, experienced an unusual surge in options trading on Wednesday. Stock traders acquired an extensive 36,130 call options on the company, representing a staggering increase of 2,077% compared to the usual volume of 1,660 call options.
The boost in trading activity has caught the attention of investors and analysts alike. Hedge funds have been actively buying and selling shares of MongoDB stock in recent times, indicating their confidence in its long-term potential. GPS Wealth Strategies Group LLC recently acquired a new stake in the company during the second quarter, worth approximately $26,000.
Capital Advisors Ltd. LLC also expressed their belief in MongoDB’s prospects by increasing their stake by 131.0% during the same period. This translates to them now owning 67 shares of the company’s stock valued at $28,000 after acquiring an additional 38 shares.
Another notable investor is Global Retirement Partners LLC who raised their stake by a massive 346.7% in the first quarter. They now hold 134 shares worth $30,000 after purchasing an additional 104 shares.
Pacer Advisors Inc., looking to capitalize on MongoDB’s potential growth, increased their stake by a considerable margin as well – a noteworthy uptick of 174.5% during the second quarter alone. Pacer Advisors currently owns 140 shares valued at $58,000 after adding another 89 shares to their holdings.
In addition to these hedge fund investments, Bessemer Group Inc., renowned for its successful venture capital portfolio, purchased a new stake in MongoDB last year valued at approximately $29,000. These investments made by hedge funds and institutional investors collectively amount to approximately 89.22% ownership of the company’s stock.
Further news emerged regarding director Dwight A. Merriman’s recent sale of 1,000 shares of MongoDB stock on Tuesday, July 18th. The transaction took place at an average price of $420.00 per share, resulting in a total transaction value of $420,000.00. Merriman’s remaining stake now stands at 1,213,159 shares in the company, valued approximately at $509,526,780.
In addition to Merriman’s sale, CAO Thomas Bull sold 516 shares on Monday, July 3rd at an average price of $406.78 per share, generating a total value of $209,898.48. With this recent sell-off by insiders, a notable 116,427 shares have been disposed of over the last ninety days alone. Insiders now own only 4.80% of MongoDB’s outstanding stock.
The buying and selling activities by hedge funds and directors have triggered analysis reports from financial institutions keen on tracking MongoDB’s performance. Oppenheimer, one such institution dealing with equity research and investment banking services increased their target price for the company from $270.00 to $430.00 in early June.
VNET Group has also reaffirmed their confidence in MongoDB by maintaining their rating for the company in late June. Meanwhile, The Goldman Sachs Group raised their price objective from $420.00 to $440.00 around the same period.
Barclays also revised their target price upwards from $374.00 to $421.00 during June while Piper Sandler increased their price objective even more significantly; raising it from $270.00 to $400.00.
With these varied endorsements and analyst opinions forming a consensus rating of “Moderate Buy,” as reported by Bloomberg.com; it becomes evident that MongoDB enjoys robust support within the investment community.
Investors and market enthusiasts await eagerly for MongoDB’s next move as it continues to capture attention amidst significant trading activity and positive analyst evaluations
MongoDB’s Resilience Shines Amidst Market Fluctuations: A Financial Performance Analysis
MongoDB Shines Amidst Volatility: An Analysis of Financial Performance
July 27, 2023 – MongoDB (NASDAQ:MDB), a prominent technology company specializing in database management solutions, witnessed a tumultuous year marked by numerous market fluctuations. Despite the prevailing volatility, MongoDB has managed to exhibit impressive financial performance and garner significant investor interest. In this article, we delve into MongoDB’s recent stock data, key financial ratios, and quarterly earnings report to analyze its standing in the corporate landscape.
Stock Performance and Market Capitalization:
As of the opening bell on July 27, 2023, MongoDB’s shares commenced trading at $405.14. The company holds a substantial market capitalization of $28.59 billion. Demonstrating resilience against market dynamics, MongoDB achieved an impressive 52-week low of $135.15 and a staggering high of $439.00.
Financial Ratios:
MongoDB exhibits robust liquidity with both its quick ratio and current ratio standing at an exceptional 4.19. This signifies the company’s ability to cover short-term liabilities comfortably through its available assets.
Furthermore, the debt-to-equity ratio for MongoDB is reported at 1.44, suggesting that the company relies more on equity financing rather than debt funds for its operations.
Earnings Data:
MongoDB last released its quarterly earnings report on June 1st, portraying remarkable results that surpassed analysts’ expectations significantly. The company recorded earnings per share (EPS) of $0.56 for the quarter, surpassing the consensus estimate by an impressive $0.38.
Revenue figures also illustrated promising growth as MongoDB reported $368.28 million for the quarter, surpassing analysts’ projections of $347.77 million by a considerable margin. This represents a substantial increase of 29% compared to the same period last year.
Analysts further noted that MongoDB had experienced a negative return on equity (ROE) of 43.25%, indicating a decline in profitability. However, the company managed to achieve a steady year-over-year revenue growth, curbing the effects of negative net margins, which stood at 23.58%.
Outlook:
MongoDB’s Q2 fiscal performance offers insights into its ability to overcome challenges amid market fluctuations and generate substantial earnings. Prospects appear optimistic for the company as analysts predict MongoDB to post -2.8 earnings per share for the current fiscal year.
Conclusion:
In a period marked by market turbulence and economic uncertainties, MongoDB stands out as a resilient technology firm. Its noteworthy stock performance, robust liquidity ratios, and significant quarterly earnings reflect its ability to adapt and thrive amidst challenging circumstances. With an innovative product offering in the competitive database management sector, MongoDB is well-equipped to leverage emerging market opportunities and secure its position as a key player within the industry.
Article originally posted on mongodb google news. Visit mongodb google news
The Iterative Nature of Language Development in Insurance – Fagen Wasanni Technologies
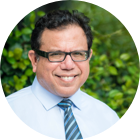
MMS • RSS
Posted on nosqlgooglealerts. Visit nosqlgooglealerts
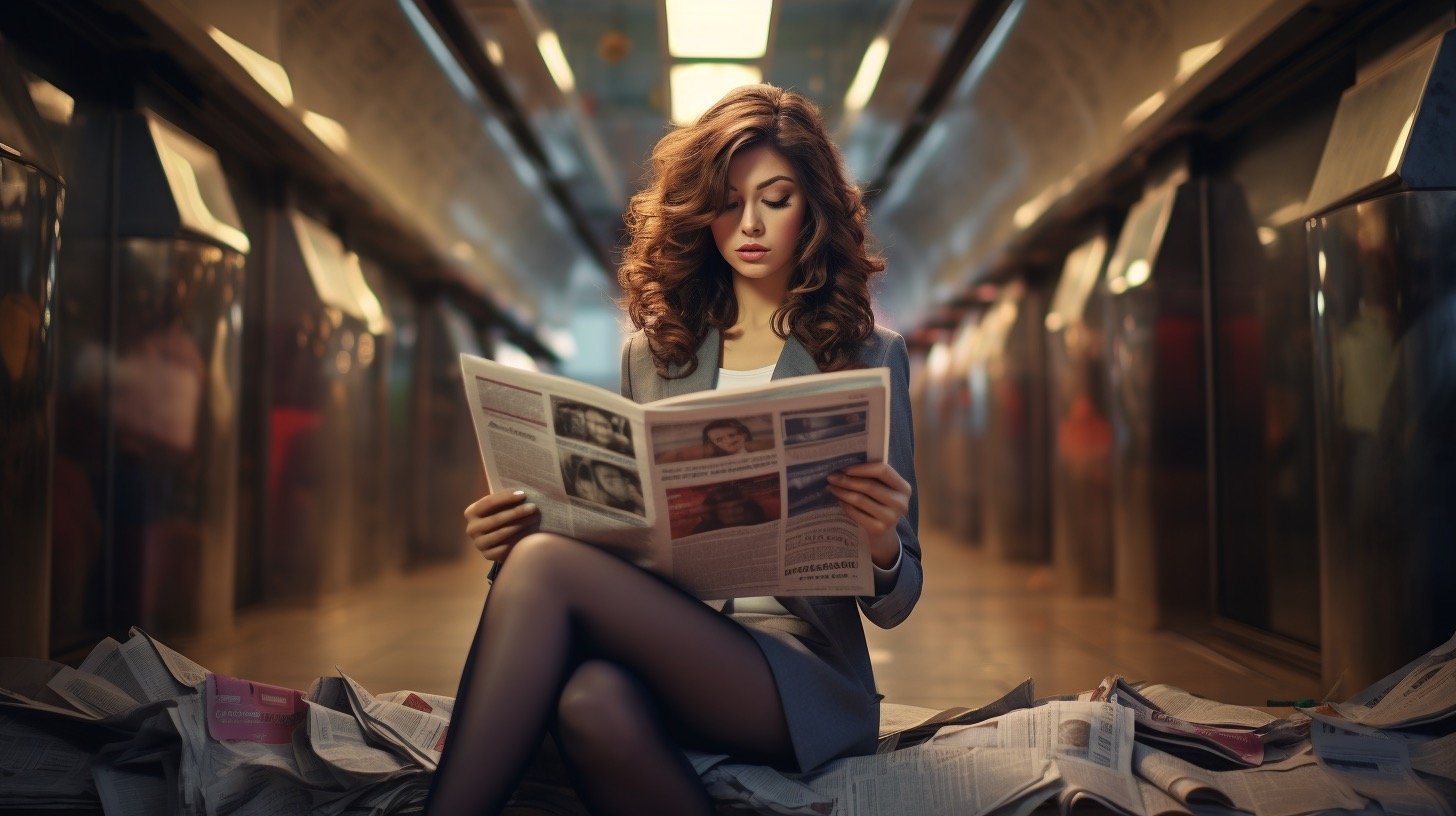
The language used in the insurance industry is undergoing a generalized and expansive transformation over time. This iterative process involves a careful consideration of various factors and risks associated with insurance policies.
Insurance companies deal with a multitude of lines of business, each with its own specific risks and exposures. Additionally, the geographic scope of these operations adds further complexity to the language used in insurance.
While the development of this new language may take longer than some might anticipate, it is a crucial aspect of shaping the modern insurance landscape. Greenberg emphasizes the importance of this iterative approach, acknowledging that the process requires time and thoughtful deliberation.
As the insurance industry continues to evolve, companies are focusing on developing a language that accurately captures the diverse range of risks and variables associated with various lines of business and geographic locations.
The goal is to create a language that effectively communicates, mitigates, and assesses risk across different areas of insurance. This iterative process ensures that the language used within the industry evolves alongside changing business requirements and operating landscapes.
By recognizing the iterative nature of language development, insurance companies are actively working towards a more comprehensive and adaptable system. This evolution is necessary for modern insurance companies to effectively communicate with and serve their clients.
Overall, the ongoing development of a generalized and large language within the insurance industry signifies a commitment to understanding and managing the complex array of risks involved. This iterative approach ensures that insurance companies remain relevant and well-equipped to navigate the ever-changing landscape of the insurance market.
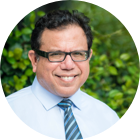
MMS • RSS
Posted on nosqlgooglealerts. Visit nosqlgooglealerts
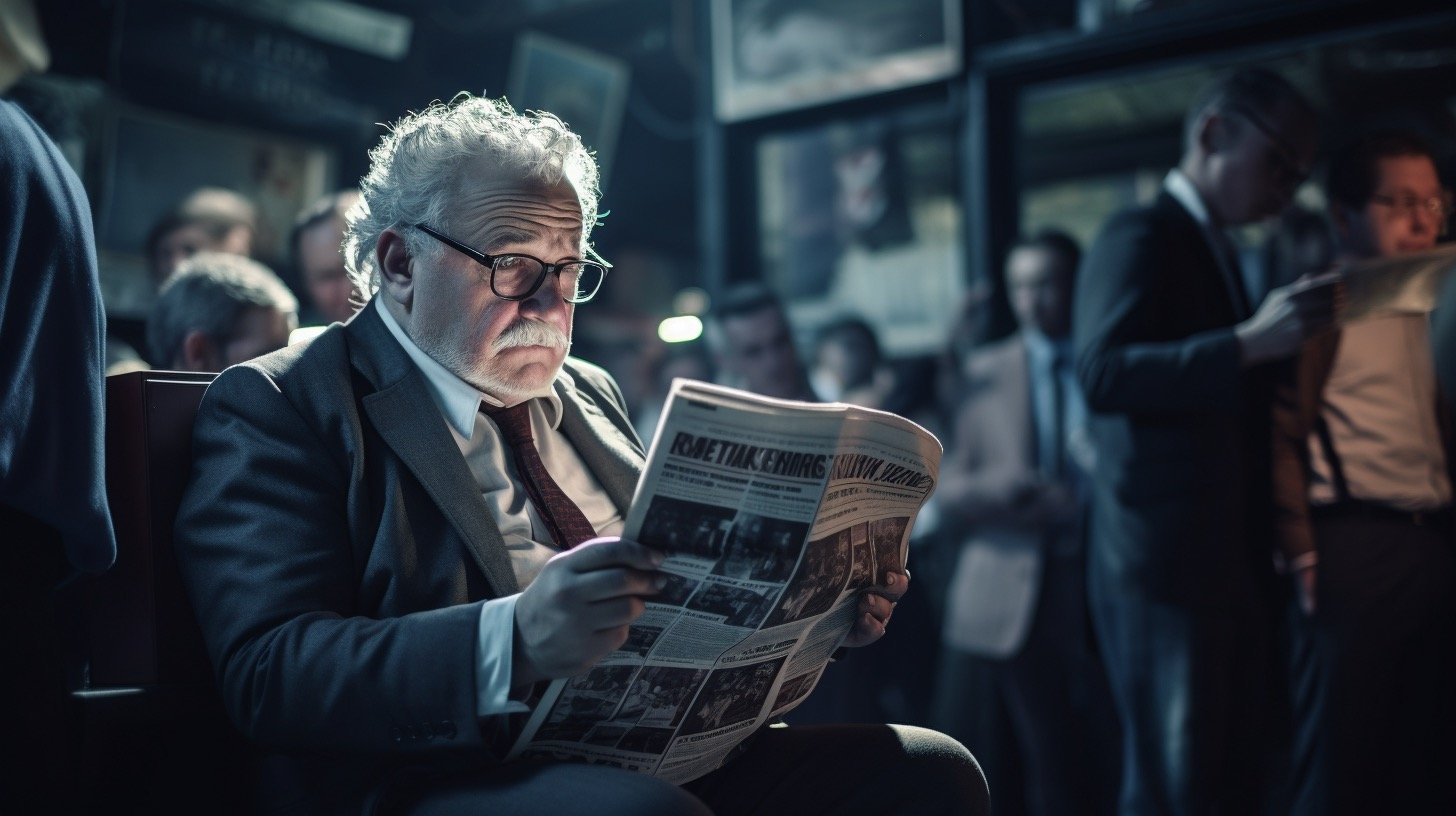
Unveiling the Influence of NoSQL on Worldwide Business Strategies
NoSQL, an innovative database technology, is making a significant impact on global business strategies. This technology, which stands for “not only SQL,” is a flexible, scalable, and high-performance database management system that is transforming the way businesses handle and analyze data.
The rise of NoSQL is a direct response to the limitations of traditional SQL databases. SQL, or Structured Query Language, has been the standard for managing and manipulating data for decades. However, as businesses have grown and evolved, so too have their data needs. The rigid, tabular structure of SQL databases can struggle to handle the volume, variety, and velocity of data that modern businesses generate.
Enter NoSQL. Unlike SQL, NoSQL databases are not bound by a fixed schema. They can handle structured, semi-structured, and unstructured data with equal ease. This flexibility allows businesses to capture and analyze a wider range of data types, from social media posts to sensor data, enhancing their ability to derive insights and make data-driven decisions.
Moreover, NoSQL databases are designed for scalability. As businesses expand globally, their data needs grow exponentially. NoSQL databases can scale out horizontally across servers and locations, providing a cost-effective solution for managing large volumes of data. This scalability is particularly beneficial for businesses operating in the digital economy, where data is a critical asset.
The impact of NoSQL on global business strategies is profound. Businesses are leveraging NoSQL to gain a competitive edge in various ways. For instance, e-commerce companies are using NoSQL databases to personalize customer experiences, while financial institutions are employing them to detect fraudulent activities in real-time.
In the healthcare sector, NoSQL is enabling the analysis of vast amounts of patient data to improve diagnoses and treatments. In the logistics industry, companies are using NoSQL to optimize routes and reduce delivery times. The list goes on, underscoring the transformative potential of NoSQL across industries and regions.
Furthermore, NoSQL is facilitating the adoption of advanced technologies like artificial intelligence (AI) and machine learning (ML). These technologies require large, diverse datasets to function effectively, and NoSQL databases are ideally suited to provide them. By enabling AI and ML, NoSQL is helping businesses to automate processes, enhance customer service, and drive innovation.
However, the adoption of NoSQL is not without challenges. Businesses need to invest in new skills and tools to effectively manage and secure NoSQL databases. Data privacy and security are paramount concerns, especially given the global nature of data flows. Businesses must ensure they comply with data protection regulations in all the jurisdictions they operate in.
In conclusion, NoSQL is reshaping global business strategies by providing a flexible, scalable, and high-performance solution for managing and analyzing data. Its impact is felt across industries and regions, enabling businesses to derive deeper insights, personalize customer experiences, and drive innovation. As businesses continue to navigate the digital economy, the influence of NoSQL on their strategies is set to grow. However, businesses must also address the challenges associated with NoSQL adoption, particularly around data privacy and security, to fully harness its potential.
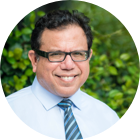
MMS • RSS
Posted on mongodb google news. Visit mongodb google news
FerretDB moves your application workloads from MongoDB to PostgreSQL. Why is that big a deal?
FerretDB, previously MangoDB (yes, Mango, this is not a typo!) is:
a stateless proxy, which converts MongoDB protocol queries to SQL, using PostgreSQL as a database engine.
It is compatible with MongoDB drivers, and should work as a drop-in replacement to MongoDB in many cases.
Thus allowing developers to use the powerful MongoDB interface while storing data in PostgreSQL and letting PostgreSQL understand queries from applications written for MongoDB. This way, MongoDB drivers and even tools can be used with an application which would otherwise rely on MongoDB, while still benefiting from the ease and flexibility of a document database, especially given Postgres’ strong JSON capabilities.
The other reason for using FerretDB is wanting to use a true Open Source MongoDB. But wait, isn’t MongoDB itself open source? Yes, but in 2018 it introduced an extreme copyleft licence, Server Side Public License(SSPL) which, while a source-available software license is unusable for many Open Source and Commercial Projects. In contrast, FerretDB is released under the Apache 2.0 license.
After much time in development, FerretDB is now production-ready, adding notable features such as support for aggregation pipelines, indexes, and authentication. One of those features is the addition of the “createIndexes” command:
This will enable you to specify the fields you want to index, and also the type of index to use (e. g. ascending, descending, or hashed). For instance, suppose you have a users collection containing several fields, including “age”, “name”, and “email”, and you want to create an index on the “age” field. You can now run the following command:
db. users. createIndex({ age: 1 })
and create an ascending index on the “age” field, which will speed up any queries that filter on that field.
Another feature in helping you gather more information about your collections, databases, and server performance. For instance to retrieve statistics about a collection, use the collStats command like this:
db. runCommand({ collStats: ‘users’ })
While FerretDB is based on PostgreSQL and as such it is the backend which will get the newest features, FerretDB is fully plugable and can support other backends like
- Tigris
- SAP HANA
- SQLite
with more backends coming soon.
The engine is offered as a Docker image for production use and development, as well as in RPM and DEB packages.
If you would like to test FerretDB, there’s also an All-in-one Docker image, containing everything you need to evaluate it with PostgreSQL.
More Information
Related Articles
PlanetScale’s MySQL Course For Developers
To be informed about new articles on I Programmer, sign up for our weekly newsletter, subscribe to the RSS feed and follow us on Twitter, Facebook or Linkedin.
Comments
or email your comment to: comments@i-programmer.info
Article originally posted on mongodb google news. Visit mongodb google news