Month: August 2023
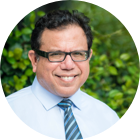
MMS • RSS
Posted on mongodb google news. Visit mongodb google news
Strong expansion in the Big Data and Analytics Market is being driven by a number of causes. Innovational items with improved features and functionalities have been made possible by technological advancements. Moreover, the demand for Big Data and Analytics goods and services is being driven by an expanding worldwide population and rising disposable incomes. Additionally, growing urbanization and elevated environmental awareness have accelerated the Market’s adoption of sustainable and eco-friendly solutions. Market penetration has been further enhanced by strategic alliances and collaborations, creating new growth potential. The Market is positioned for long-term growth and a bright future as it develops, propelled by these driving forces.
GET | Sample Of The Report: https://www.marketresearchintellect.com/download-sample/?rid=349161
The study uncovers key advancements in both organic and inorganic growth strategies within the worldwide Big Data and Analytics Market. Many enterprises are prioritizing new product launches, approvals, and other strategies for business expansion. The study also delivers profiles of noteworthy companies in the Big Data and Analytics Market, which includes SWOT analyses and their market strategies. The research puts emphasis on leading industry participants, providing details about their business profiles, the products and services they provide, recent financial figures, and significant developments. The section on Company Usability Profiles is as follows:
- Microsoft Corporation
- MongoDB
- Predikto
- Informatica
- CS
- Blue Yonder
- Azure
- Software AG
- Sensewaves
- TempoIQ
- SA OT
- IBM Cor Cyber Grou Splunk
The Big Data and Analytics Market Statistical Research Report also includes extensive forecasts based on current Market trends and descriptive approaches. Quality, application, development, customer request, reliability, and other characteristics are constantly updated in the Big Data and Analytics Market segments. The most critical adjustments in the item model, production technique, and refining phase are facilitated by little changes to an item.An Analysis of Big Data and Analytics Market segmentation.
This Report Focuses On The Following Types Of Market :
- Data Intergration
- Data Storage
According To this Report, The Most Commonly Used Application Of the Market Are:
The paper exhibits a thorough awareness of competitor positioning, global, local, and regional trends, financial projections, and supply chain offerings in addition to information on segment classification. A thorough overview of the industry, including details on the supply chain and applications, is provided by Big Data and Analytics industry research. A study has been conducted to analyse the Big Data and Analytics Market’s current state and its potential for future growth.
Big Data and Analytics Market Report Provides The Following Benefits To Stakeholders:
- Detailed qualitative information on Market s with promising growth can be found in the analysis, as well as insights on niche Market s.
- This report provides information on Market share, demand and supply ratios, supply chain analysis, and import/export details.
- There is a detailed analysis of current and emerging Market trends and opportunities in the report.
- An in-depth analysis provides an understanding of the factors that will drive or inhibit the Market ‘s growth.
- It is conducted a thorough analysis of the industry by monitoring the top competitors and the key positioning of the key products within the Market context.
- The Big Data and Analytics Market Report offers a detailed qualitative and quantitative analysis of future estimates and current trends and assists in determining the Market potential for the present.
What questions should ask an expert? And Ask For Discount: https://www.marketresearchintellect.com/ask-for-discount/?rid=349161
What is the Purpose Of The Report?
It provides an in-depth analysis of the overall growth prospects of the global and regional Market s. Moreover, it provides an overview of the competitive landscape of the global Market . Furthermore, the report provides a dashboard overview of leading companies, including their successful Market ing strategies, Market contributions, and recent developments in both historic and current contexts.
What is the impact of Big Data and Analytics Market forces on business?
An in-depth analysis of the Big Data and Analytics Market is provided in the report, identifying various aspects including drivers, restraints, opportunities, and threats. This information allows stakeholders to make informed decisions prior to investing.
Our key underpinning is the 4-Quadrant which offers detailed visualization of four elements:
- Customer Experience Maps
- Insights and Tools based on data-driven research
- Actionable Results to meet all the business priorities
- Strategic Frameworks to boost the General Purpose Transistors growth journey
GET FULL INFORMATION ABOUT Click Here: Big Data and Analytics Market Size And Forecast
Overview Of The Regional Outlook of this Big Data and Analytics Market
The report offers information about the regions in the Market, which is further divided into sub-regions and countries. In addition to the Market share of each country and sub-region, information regarding lucrative opportunities is included in this chapter of the report. Share and Market growth rate of each region, country, and sub-region are mentioned in this chapter of the report during the estimated time period.
Geographic Segment Covered in the Report:
The Big Data and Analytics report provides information about the market area, which is further subdivided into sub-regions and countries/regions. In addition to the market share in each country and sub-region, this chapter of this report also contains information on profit opportunities. This chapter of the report mentions the market share and growth rate of each region, country and sub-region during the estimated period.
About Us: Market Research Intellect
Market Research Intellect is a leading Global Research and Consulting firm servicing over 5000+ global clients. We provide advanced analytical research solutions while offering information-enriched research studies.
We also offer insights into strategic and growth analyses and data necessary to achieve corporate goals and critical revenue decisions.
Our 250 Analysts and SMEs offer a high level of expertise in data collection and governance using industrial techniques to collect and analyze data on more than 25,000 high-impact and niche markets. Our analysts are trained to combine modern data collection techniques, superior research methodology, expertise, and years of collective experience to produce informative and accurate research.
Our research spans a multitude of industries including Energy, Technology, Manufacturing and Construction, Chemicals and Materials, Food and Beverages, etc. Having serviced many Fortune 2000 organizations, we bring a rich and reliable experience that covers all kinds of research needs.
Contact the US:
Mr. Edwyne Fernandes
Market Research Intellect
US: +1 (650)-781-4080
US Toll-Free: +1 (800)-782-1768
Article originally posted on mongodb google news. Visit mongodb google news
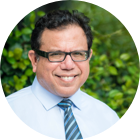
MMS • Aditya Kulkarni
Article originally posted on InfoQ. Visit InfoQ
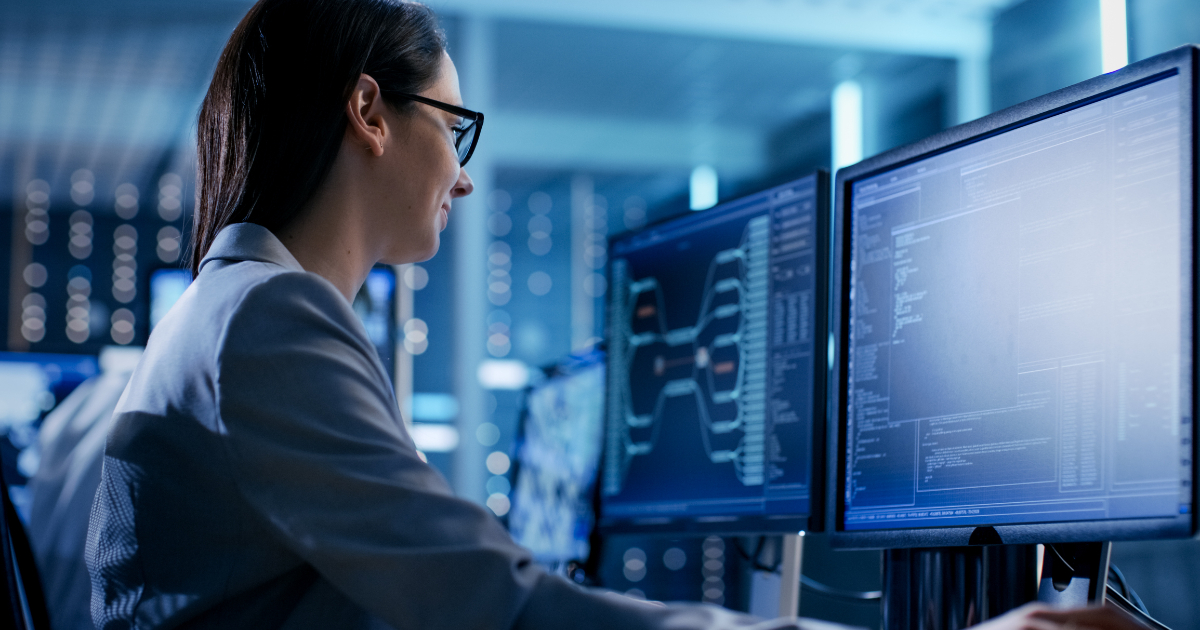
DoorDash recently leveraged Open Policy Agent to enhance the efficiency of their developers. The infrastructure team at DoorDash observed several advantages from this, including quicker reviews of changes to infrastructure policies, more comprehensive tagging of resources, and a notable decrease in the number of incidents resulting from policy violations.
At QCon New York 2023, Lin Du, Senior Software Engineer at DoorDash, provided a detailed overview of a self-serve infrastructure that utilizes policy automation.
A few years back, DoorDash encountered an incident that caused their order volume to drop. While the infrastructure team managed to resolve the incident in an hour, the root cause was the accidental removal of essential AWS resources. This unintended removal occurred within a Terraform code that also included around 90 other resources, seemingly innocuous in nature. This realization prompted the use of policy automation as a safeguard against such critical oversights in the future.
At DoorDash, the team has used Atlantis, an open-source orchestrator for Terraform plans. This orchestrator manages the Terraform plan lifecycle. When users create infrastructure pull requests on GitHub, a webhook event is triggered to an Atlantis worker. This worker retrieves Open Policy Agent (OPA) policies from a designated S3 bucket.
DoorDash crafts the policy rules using Rego queries to identify deviations from the expected system state. The conftest tool, employed by DoorDash, leverages these OPA policies to validate data against policy assertions.
Atlantis then runs conftest against the Terraform plan, aligning it with OPA-defined policies. The results, alongside Terraform plan details, are added as comments on the GitHub pull requests.
DoorDash further streamlines the process with Pull Approve, a GitHub integration handling code review, assignment, and policy. With the required approvals in place, Atlantis executes changes to AWS resources as per the Terraform plan.
Du further illustrated the policies that can be written using this automation. He categorized the policies into four types – Reliability, Velocity, Efficiency, and Security.
For reliability, consider a scenario where it is important to safeguard critical resources from deletion. Du illustrated this by presenting an example where a policy was set up to identify these crucial resources. Subsequently, a verification step was introduced, necessitating an administrative review before any modifications to these resources could take place. To optimize the velocity of review Du showcased an example where the policy checked the Terraform module in a given PR from the already-approved list of modules. If the team is using a module that is not listed, the policy encourages using an already-approved terraform module.
As an outcome of the policy automation, the DoorDash infrastructure team saved time spent reviewing the pull requests, thereby working towards product improvements as a whole. The team could also prevent incidents caused by policy violations, as they could identify policy issues in pull requests early. Finally, the team increased their resources tagging coverage and standardization from 20% to 97.9%, leading to cost and team member optimization.
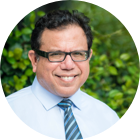
MMS • Sergio De Simone
Article originally posted on InfoQ. Visit InfoQ
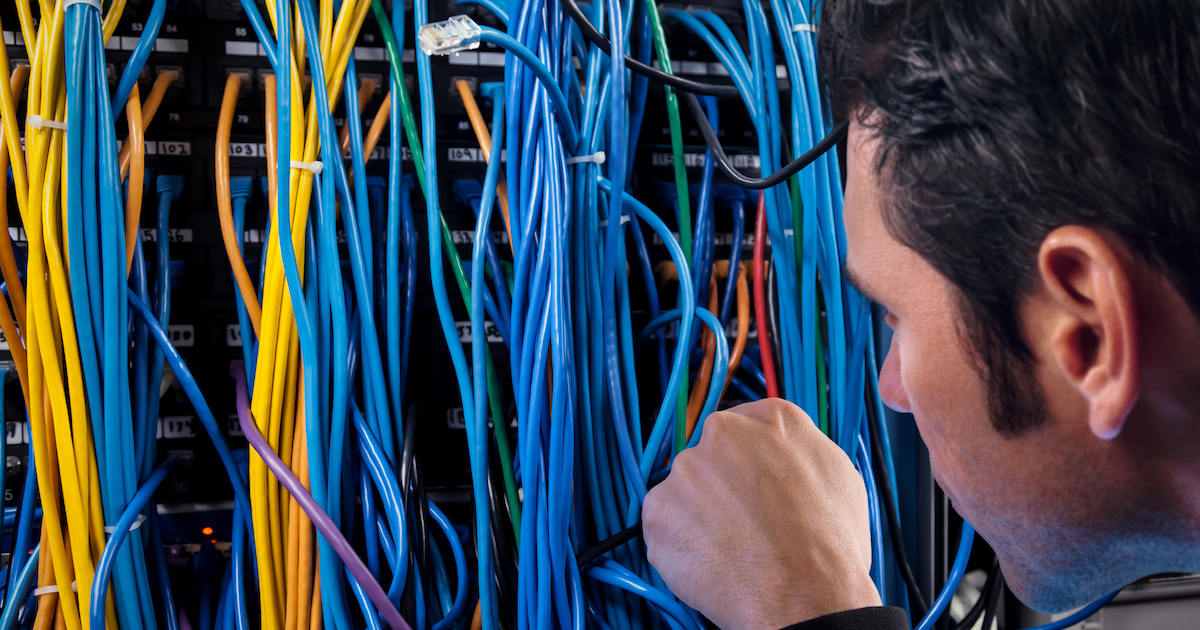
To make it easier for developers to expose their local services to the Internet while developing them, ngrok is now providing them the possibility to create and use one static domain for free, instead of relying on time-limited, random domains.
Ngrok domains are often used, e.g., to make an under-development API running on a developer’s machine accessible to testers or other developers so they can use it from a different machine not belonging to the same intranet/extranet.
Ngrok has long offered a free tier to its users, albeit with some limitations. In particular, the tunnel link associated to a free account expires after eight hours, which requires the ngrok service to be restarted to set up a new tunnel, ensuing in the creation of a new random domain to use to access the exposed service.
Static domains are unique domains that are yours and don’t change. With your free static domain, you can focus on developing your application, and no longer need to worry about broken links caused by agent restarts. There’s no need to constantly keep updating webhook providers or mobile apps with new URLs or send new links to your team.
Free static domains should not be confused with custom domains, also known as “branded” domains, which remain a premium feature. A static domain is a subdomain of one of number of ngrok-owned domains, such as ngrok.io
, ngrok-free.app
and others, and have thus the form panda-new-kit.ngrok-free.app
, whereas a non-static domain includes a randomly generated ID, such as 85ee564738gc.ngrok.io
. Setting up a custom domain is a slightly more complex process, requiring some configuration on both ngrok’s and your DNS provider’s side.
The first step to set up a static domain with your free ngrok account is heading to your ngrok dashboard to create a new Cloud Edge domain. This will provide a URL you can use from the ngrok CLI to create the tunnel:
ngrok http -–domain=panda-new-kit.ngrok-free.app 80
or, alternatively, via any of the ngrok Agent SDKs, which are available for a variety of languages including go, rust, and others.
As a last note, a static ngrok domain also enables using ngrok’s Kubernetes Ingress Controller, which can be seen as a Kubernetes-native API wrapped around ngrok’s platform to provide public and secure ingress traffic to k8s services.
Skandinaviska Enskilda Banken AB publ Increases Holdings in MongoDB, Inc. while … – Best Stocks
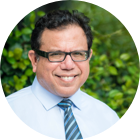
MMS • RSS
Posted on mongodb google news. Visit mongodb google news
On August 25, 2023, it was reported that Skandinaviska Enskilda Banken AB publ has increased its holdings in MongoDB, Inc. by 4.6% during the first quarter of the year. The institutional investor now owns 11,997 shares of the company’s stock after purchasing an additional 530 shares during this period. According to a recent filing with the Securities & Exchange Commission (SEC), Skandinaviska Enskilda Banken AB publ’s holdings in MongoDB were valued at $2,795,000.
In other news related to MongoDB, Cedric Pech, the Chief Revenue Officer (CRO), sold 360 shares of the company’s stock on July 3rd. The transaction took place at an average price of $406.79 per share, resulting in a total value of $146,444.40. Following this sale, Cedric Pech now directly owns 37,156 shares in the company, which are valued at approximately $15,114,689.24. This transaction was disclosed in a document filed with the SEC and is available through a provided hyperlink.
Additionally, another key executive named Thomas Bull, the Chief Accounting Officer (CAO), sold 516 shares of MongoDB stock on July 3rd at an average price of $406.78 per share. The total transaction value for these shares amounted to $209,898.48. As a result of this sale, Thomas Bull currently holds 17,190 shares in the company which are valued at approximately $6,9925,,548,.20 according to disclosures made by MongoDB.
It is worth noting that insiders have collectively sold a total of 102,220 shares of company stock over the past three months with an estimated value of $38,7635,,571.. These sales by insiders represent approximately 4.80% ownership stake in MongoDB.
In conclusion, Skandinaviska Enskilda Banken AB publ has increased its holdings in MongoDB, Inc. during the first quarter of 2023. Meanwhile, key executives such as Cedric Pech and Thomas Bull have sold shares of the company’s stock. These transactions were disclosed in documents filed with the SEC, indicating a significant level of insider selling activity.
Large Institutional Investors and Hedge Funds Increasing Stake in MongoDB Inc., Analysts Bullish on Company’s Potential
August 25, 2023 – Large investors have been making significant moves in relation to their stakes in MongoDB, Inc. (NASDAQ: MDB). Raymond James & Associates, for instance, increased its holdings in the company by 32.0% during the first quarter of this year. Consequently, they now possess 4,922 shares of the company’s stock valued at $2,183,000 after buying an additional 1,192 shares during the last quarter.
PNC Financial Services Group Inc., on the other hand, lifted its position in MongoDB by 19.1% during the same period. They now own 1,282 shares of the company’s stock valued at $569,000 after acquiring an additional 206 shares in the last quarter.
MetLife Investment Management LLC took a different approach and acquired a new stake in MongoDB during the first quarter valued at $1,823,000. Meanwhile, Panagora Asset Management Inc. increased its position in the company by 9.8% during that same period and currently owns 1,977 shares valued at $877,000.
Lastly,Vontobel Holding Ltd raised its stake in MongoDB by a staggering 100.3% in the first quarter alone. They now possess 2,873 shares of the company’s stock valued at $1,236,000 after purchasing an additional 1,439 shares during that period.
These are just some notable examples of large institutional investors and hedge funds actively increasing their involvement with MongoDB Inc., as shown through regulatory filings according to Bloomberg data. In fact,enough so that it can be confidently stated that around a staggering89.22% of all outstanding MDB stock is owned by institutional investors and hedge funds alike.
With regards to analyst commentary on MDB,Numerous brokerages have provided insights on MongoDB’s performance recently.Stifel Nicolaus,believing great things are ahead for MDB,lifted the price target on shares of MongoDB from $375.00 to a whopping $420.00 back in June. Oppenheimer also maintained their bullish outlook on the company’s prospects by increasing their price objective from$270.00 to $430.00 that same month.Moreover, VNET Group has recently reaffirmed its “maintains” rating for MongoDB while Tigress Financial has raised its own price objective on the stock from $365.00 to an impressive $490.00.The overall consensus according to Bloomberg is that MDB is a company with great potential; it currently has a majority “Moderate Buy” rating and an average price target of $378.09, as determined by analysts.
On Friday, MDB shares opened at $360.75, reflecting a relatively stable start for the trading day ahead. The business boasts a quick ratio and current ratio both standing at 4.19 respectively.Additionally, the company possesses a debt-to-equity ratio of 1.44.MDB has displayed upward momentum in recent market performance within the past year alone ranging from its low point of$135.15 to its high mark of $439.00.
Regarding MongoDB’s financial performance, they last revealed quarterly earnings data on Thursday, June 1st.While analysts’ consensus estimates stood at an EPS of $0.18 for that quarter,MongoDB managed to surpass market predictions,revealing an actual EPS of $0.56.Utilizing this information,it can be established that revenues during that aforementioned quarter amounted to roughly$368.28 million.This figure surpassed earlier projections which had placed expected revenues around$347.77 million.MongoDB’s revenue was up 29% when compared to figures reported during the equivalent period during previous years interestingly enough.When considering net margin, MongoDB fell short slightly reporting negative results at23.58%.Similarly,negative returns on equity were observed standing out negatively at43.25%. However,in spite of these concerning results,asset management firms and hedge funds possibly appreciate the company’s revenue growth during this quarter.
Looking ahead, analysts predict that MongoDB will post a negative EPS of -2.8 for the current fiscal year in aggregate. Although such figures are rather bleak, it is not surprising given the current economic climate and market conditions. Investors and analysts alike remain cautiously optimistic about MongoDB’s overall prospects for growth in the coming years.
Article originally posted on mongodb google news. Visit mongodb google news
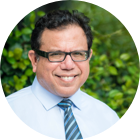
MMS • RSS
Posted on mongodb google news. Visit mongodb google news
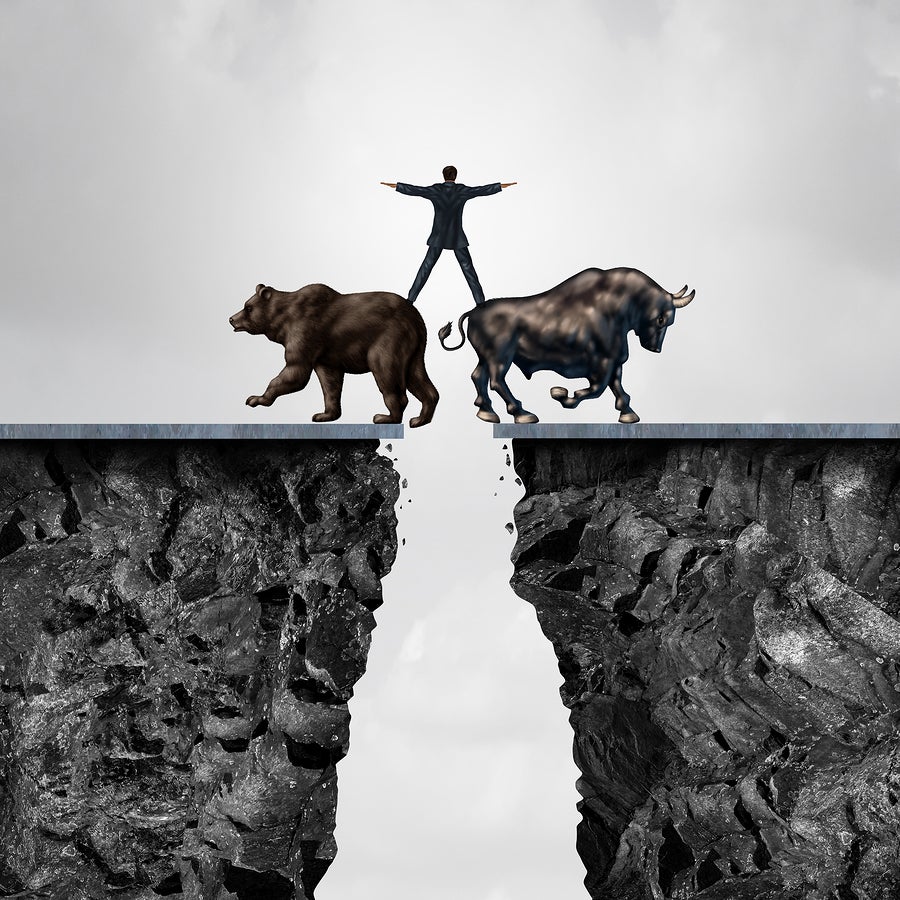
MongoDB (MDB – Free Report) closed the most recent trading day at $363.46, moving +0.75% from the previous trading session. The stock outpaced the S&P 500’s daily gain of 0.67%. Meanwhile, the Dow gained 0.73%, and the Nasdaq, a tech-heavy index, added 0.94%.
Coming into today, shares of the database platform had lost 10.61% in the past month. In that same time, the Computer and Technology sector lost 3.27%, while the S&P 500 lost 3.8%.
MongoDB will be looking to display strength as it nears its next earnings release, which is expected to be August 31, 2023. On that day, MongoDB is projected to report earnings of $0.45 per share, which would represent year-over-year growth of 295.65%. Meanwhile, our latest consensus estimate is calling for revenue of $389.93 million, up 28.41% from the prior-year quarter.
For the full year, our Zacks Consensus Estimates are projecting earnings of $1.51 per share and revenue of $1.54 billion, which would represent changes of +86.42% and +19.78%, respectively, from the prior year.
Investors should also note any recent changes to analyst estimates for MongoDB. Recent revisions tend to reflect the latest near-term business trends. With this in mind, we can consider positive estimate revisions a sign of optimism about the company’s business outlook.
Based on our research, we believe these estimate revisions are directly related to near-team stock moves. Investors can capitalize on this by using the Zacks Rank. This model considers these estimate changes and provides a simple, actionable rating system.
The Zacks Rank system, which ranges from #1 (Strong Buy) to #5 (Strong Sell), has an impressive outside-audited track record of outperformance, with #1 stocks generating an average annual return of +25% since 1988. Within the past 30 days, our consensus EPS projection remained stagnant. MongoDB currently has a Zacks Rank of #3 (Hold).
Digging into valuation, MongoDB currently has a Forward P/E ratio of 239.1. Its industry sports an average Forward P/E of 36.52, so we one might conclude that MongoDB is trading at a premium comparatively.
The Internet – Software industry is part of the Computer and Technology sector. This group has a Zacks Industry Rank of 85, putting it in the top 34% of all 250+ industries.
The Zacks Industry Rank gauges the strength of our industry groups by measuring the average Zacks Rank of the individual stocks within the groups. Our research shows that the top 50% rated industries outperform the bottom half by a factor of 2 to 1.
You can find more information on all of these metrics, and much more, on Zacks.com.
Article originally posted on mongodb google news. Visit mongodb google news
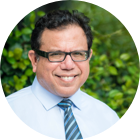
MMS • RSS
Posted on nosqlgooglealerts. Visit nosqlgooglealerts
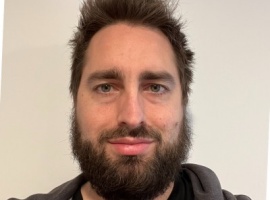
Transcript
GV: My name is Felix. I am a Committer on the Venice Database Project, which was open sourced last year. I’ve been doing data infrastructure since 2011, the majority of which I spent at LinkedIn. You can find some of my thoughts on my blog at philosopherping.com. I’d like to cite one of the nice quotes from Winston Churchill. The nice thing about having the modern data stack, data mesh, real-time analytics, and feature stores is you always know exactly which database to choose for the job. Of course, Winston didn’t say that. As far as I know, no one ever said that. In fact, I hear people saying the opposite. Year after year, they say, the database landscape is getting more confusing. There are just too many options to pick from. Here’s a bunch of data systems, most of which you’re probably familiar with, or at least heard about. What I want to do in this talk is give you a new lens to look through this landscape, and categorize these systems, because within this bunch of systems hiding in plain sight, are several derived data systems. I think it’s useful if we understand the differences between primary data and derived data, and then which systems are optimized for each. Then we can make better choices about our architectures.
Outline
First, I want to lay the groundwork for this discussion by talking about, what is primary data. Then I want to use that to contrast it with derived data, and what is that? Finally, the third section is about four derived data use cases that we have at LinkedIn, including real-time analytics, graph, ML feature storage, and search. For each of those, I’m going to cover the specialized system that we use to serve these use cases at scale at LinkedIn.
What Is Primary Data?
What is primary data? Some related terms, you could say primary data is source of truth data. You could say a primary data system is a system of records. Here’s an attempt at defining primary data. Primary data is that which is created or mutated as a direct result of a user action. Here, I’m putting the emphasis on direct. Hang on to that, it will make sense when we contrast with derived data. What are we looking for in a primary data system? Typically, we’ll want that system to provide durability. That means when you write the data into the primary data system, you expect the data to be persistent. You don’t want it to be only in memory, and then you have a power outage and you lose that, or something like that. You would want it to be on durable medium, like spindles or flash. You might also want the system to guard against machine failures, in which case, you would benefit from the system having replication. Besides machine failures, you might also care about human failures. I deployed a version of my application that corrupts the data, let’s roll back to yesterday’s backup. Backups may be useful to guard against that, or other scenarios, of course.
Finally, perhaps the most important is that, usually, primary data systems benefit from strong consistency. I say usually, and I’m putting some caveats on these things, because none of these are actually hard requirements. You could choose to put your primary data anywhere you’d like. It turns out that in many cases, these are useful characteristics. For strong consistency in particular, the thing is, for user experience reasons, we want to have that. Imagine that the user is editing their account details in your system. If the user submits their edit to the account detail information, and then they reload the page, or maybe they even load it on a different device that they have, they would expect to see the edit that was just acknowledged to be there. You achieve that by having strong consistency.
Some examples of primary data systems include the following. We’ve got the traditional relational databases of the transactional flavor, like OLTP databases, like MySQL, Postgres, and others. You could also store primary data in a NoSQL offering, like Cassandra or Mongo. Of course, nowadays, the cloud is all the rage, so Cosmos DB, DynamoDB, and other similar offerings could certainly host primary data as well. One last thing about primary data is, how does the data escape that system that it’s in? Because sometimes we want to get access to that data elsewhere. That’s critical to our talk here. One way is to ETL a whole snapshot of the database to another location, like your Hadoop grid, or maybe some cloud storage like S3 or Azure Blob Store. Another way is to listen in on the changes as they happen. We call that Change Data Capture. At LinkedIn, we’re pretty big on Change Data Capture, and we built Databus more than a decade ago, then replaced it with our next-gen Change Data Capture system called Brooklin. This one we open sourced a few years ago. It supports data capture out of MySQL, Oracle, and others. There are other options as well, like Debezium is a pretty popular Change Data Capture system as well nowadays.
What Is Derived Data?
Once the primary data escapes the system it’s in, somehow, then it might become derived data. What does it mean? Here are some related terms. Derived data may be processed, or computed, reformatted, massaged, inferred from other data. You can pick and choose any of these terms. Sometimes, many of these things are going to happen at the same time. Then the output of this transformation basically is the derived data, and it is derived from some other data. Therefore, because this data is processed, it is indirect. Earlier, I told you to hang on to the notion that primary data is a result of a direct action of the user, derived data may also be indirectly tied to a user action. That’s an important distinction. It’s not direct. There’s been some processing hub in between. Maybe it’s been loaded into a Hadoop grid or some object storage in order to get processed in batch over there, or maybe we’ve been doing some stream processing on the Change Data Capture. Sometimes we’re doing both of these at the same time, like a Lambda architecture.
There are many different types of derivation of the data. I’m going to go over many examples of how data can be derived for specialized use cases throughout the rest of the talk. I think it’s useful to subcategorize derivation into two flavors, physical and logical. What I mean by that is physical derivation doesn’t change the content of the data, but it changes how it’s organized. For example, you may have the table in your database, which is organized in row-oriented format, which makes it ideal for looking up a single row or a small amount of rows, and retrieving all of that quickly. Then, if you want to do some analytics on this data, that will still work, the row-oriented table will still work. It could be more optimal to reformat the same data into column-oriented format. That would be an example of massaging the data into a different structure. The data is the same, but it’s optimized for another use case.
A logical derivation is where the data itself changes. The input is different from the output. It could be a little different or very different. An example of a logical derivation, where the data is just a little different is maybe you’re putting the data in lowercase. Or maybe you’re stripping out the plural suffix to the word when you find it. These are like tokenization techniques that you may find in a search use case, for example. The data looks pretty similar, but it’s a little bit transformed. That makes it more useful for this type of use case. It could also have a logical derivation that makes it completely unrecognizable. For example, in machine learning, if you have a training job that takes as input some text that’s been generated by users, and then maybe also some user actions about whether they liked a certain thing, or whether they shared it, and so on, all of these input signals. Then, the output of the ML training job is just a bunch of floating-point values. Those may be the weights of a neural net, or maybe some scores about a prediction of the likelihood that the user will click on a given article, if it was presented to them. It had some relation to the input, but it’s completely unrecognizable from our perspective. That would be an example of a logical derivation that changes the data a lot.
We covered in the last section, what are the qualities that a primary data system may benefit from. Let’s do the same for derived data. Given that derived data is processed, it’s great if the derived data system has first class support for ingesting these processed outputs, so ingesting batch data, ingesting stream data. There wouldn’t be much of a point in having the derived data system if it didn’t have some specialized capability. If the derived data system does exactly the same stuff that your primary data system does, then you might as well just have your primary data system. Either these are capabilities that are not found at all in the primary data system, or maybe they’re there but not as efficiently implemented in the primary data system. That brings me to the last point that oftentimes a derived data system is going to offer some superior performance in some niche application.
The thing which we typically do not find in a derived data system is strong consistency. That’s in opposition to the primary data systems. If you think about it, again, from the user standpoint, the user experience, typically, it does not matter for a derived data system to have strong consistency. Because the data in it is not appearing there as a direct result of a user action. It’s happening after some background process or asynchronous process has done something with the data. By the time the data comes out of the stream processor that generated it, it doesn’t matter too much at that point if the last mile, like the interaction between the stream processor and the derived data system, whether that is strongly consistent or not. It doesn’t really matter. Because the whole thing that happened before that was already asynchronous anyway. Therefore, from the user’s perspective, the whole thing is asynchronous, regardless of whether the last mile is strongly consistent or not. Therefore, if you don’t have strong consistency, then you don’t have read after write semantics either. It’s important when using those systems to be cognizant of that and to make sure we don’t misuse the systems by assuming they provide something that they do not.
Here are a few examples of open source derived data systems. There are search indices like Solr and Elasticsearch. Real-time analytics systems like Pinot, Druid, ClickHouse, and some ML feature storage systems like Venice. I want to linger for a little moment on these two search indices, Solr and Elasticsearch. I want to linger here to highlight some of the subtlety about consistency, because these systems, Solr and Elasticsearch, both offer a REST endpoint for writing documents into the index. You have a REST endpoint, you post to it, and then it gets acknowledged as a 200 OK, something like that. You could easily assume that because the REST endpoint acknowledged your request, that’s it, your data is there. It’s persistent and readable. If you do a query against the search index, you will find the document that you just put in there. That would be only halfway true. When the POST comes back successfully, your data is durable, but it is not yet readable. That’s an important distinction. Why is it not durable? Because these search indices have a periodic process that will go in and do what they call a refresh or a commit. Depending on the system, the terminology is different, but it’s the same idea. Then, after this refresh of the index happens, then the data that was persisted after the last refresh, then that data becomes readable also.
You could force the system to give you strong consistency. You could say, I’ll post my document, and then I’ll force a refresh. That would make it look like the search index has strong consistency. It would truly have strong consistency at this point. What I want to get at is that this is not really a production grade way to use this system. If you were doing this trick, where you post your document and then force a refresh, you could theoretically use Solr or Elasticsearch as your system of record, this could be your source of truth. When you look at the best practices for these systems, you see that this is not quite desirable. When you look at the best practices, they tell you, actually, don’t even bother sending one document at a time, because that’s awfully inefficient for this system. Instead, accumulate some batch of documents. Then once you reach some sweet spot, like maybe 5000 documents, or it depends on the size of each document, of course, then at that point only, send me the batch. That is the more efficient way of using this system. If you send me too much documents to index, the system may kick it back. It may say, I’m overloaded, you got to back off, and try again later.
You see that these best practices don’t align very well with this being a system of record. In fact, the production grade way to run this system is to put a buffer in front. Imagine that you have Kafka as your buffer, you write documents, one at a time into Kafka. Then you have some connecting application like Kafka Connect, that will accumulate those documents into batches of the right size, and then post those batches to the search index at the rate the search index prefers. If the search index kicks it back, then the connecting application will take care of the backoff, retries, and all of the logic to make this reliable and scalable and efficient. The interesting thing here is, although Solr and Elasticsearch, which are quite old, maybe more than a decade old, although those systems were built with a REST endpoint that makes it look like you’re going to get strong consistency out of this thing, in practice, it’s not really standalone. You ought to use something else as part of the mix. If you look at more recent derived data systems, like Venice and Pinot, you see that those systems were built from the ground up to use a buffer. Essentially, their write API is a buffer. You give a buffer to those systems, or they have a buffer built in even. You see the evolution of these derived data systems, like we cut to the chase. In the newer systems, instead of dumping the complexity on the user to stand up all this infrastructure around it, the system is just built in to work in the production grade way.
Derived Data Use Cases at LinkedIn – Real-Time Analytics
Speaking of production grade, I want to now make this even more concrete by talking about four categories of derived data use cases that we have at LinkedIn, because each of those has between hundreds and thousands of unique use cases. Let’s get started with real-time analytics. For that we use Pinot, which ingests a bunch of data from Hadoop and Kafka. At LinkedIn, we’re old school, so we still use Hadoop. We probably have one of the biggest Hadoop deployments on the planet at this point. In your mind’s eye, whenever you see this slide, you can substitute Hadoop for S3 or Azure Blob Store, whatever favorite thing you have for storing batch data. Then, similarly, instead of Kafka, you could substitute like Kinesis, or Pulsar, or whatever, as the streaming input. An example of use case served by Pinot is, who viewed my profile. Here you can see the count of unique viewers per week in the past 90 days, and you have various toggles at the top to filter the data. Pinot is great for that. You can ingest events data, like profile view events, for example, and index it inside Pinot. That works great because Pinot is perfect for immutable data, meaning that not that the whole dataset is immutable, but rather, each record in the dataset is immutable. You can append a new record, but you cannot change old records. It’s also great for time-series data. If you have a metric that comes out once per minute, and then maybe there’s a bunch of different metrics that have different dimensions attached to them, and each of them comes out once a minute, this would be also a great fit. Once you have that data inside Pinot, you can do expressive SQL queries on top of that, like filtering, aggregation, GROUP BY, ORDER BY. This is like what I just showed in the example use case.
In terms of types of derivation that are supported by Pinot, one of them is to transcode the data from a bunch of formats. The data coming in from the stream into Pinot could be in Avro format. That’s what we use at LinkedIn, or it could be in protobuf, or Thrift format, or others. The data that comes from the batch source could be in ORC, Parquet. Pinot will transcode all of that into its native representation of each of the data types. Then, it’s going to take those bits of data and encode them using column-oriented techniques. For example, dictionary encoding is, if you have a column of your table that has a somewhat low cardinality, then instead of encoding each of the values itself, you can instead assign an ID for each of the values and encode the IDs, which if the IDs are smaller than the value, then you have a space saving. Then, you can also combine that with run-length encoding, which means that instead of recording every single value of the column, you encode, essentially, how many times each of the values have it. For example, I had a value 10, 100 times, and then I had value 12, 150 times, and so on. This makes the data even smaller, especially if the column is sorted, but even if it’s not sorted, you can still leverage run-length encoding. Depending on the shape of the data, it could yield some pretty big space savings.
Why does that matter? In the case of Pinot, for many of the use cases, we want to have the whole dataset in RAM in order to support very fast queries. How big the data is, directly affects cost, because RAM is costly. Furthermore, the less data there is to scan the faster our scans of the data can go. Minimizing space improves both storage cost and query performance. Besides these physical derivation techniques, Pinot also supports a variety of logical derivations. You can, for example, project some of the columns of the data and throw away the rest. You can also filter out some of the rows of the data. If you have some complex types in the data, as you could find in Avro or protobuf, like some struct or nested record type of situation in the data, then you can flatten that out, which makes it easier to query in SQL. Pinot is open source. If you want to find information about it, there’s a bunch of great information on the web. The official documentation is really good, you can find it at docs.pinot.apache.org. There’s also a bunch of videos, interviews, podcasts, and so on about Pinot. I’m sure you’ll find information if you’re looking for it.
Use Case – Graph
Our next system is our graph database, which is called LIquid. It also ingests data from Hadoop and Kafka, in our case. I want to quickly clarify, though, graph databases are not inherently derived data systems. There are graph databases, which are intended to be primary data systems. For example, Neo4j from my understanding is designed to be a primary data system, it provides strong consistency and so on. In our case, what we wanted to do with this graph data is to join data coming out of a bunch of other databases. We have a bunch of source of truth databases for each of our datasets, and we want to join all of those into the economic graph, and then run queries on that economic graph. For us, it made sense to build LIquid as a derived data system. One of the usages is finding out the degrees of separation between the logged in member and any other member of the site. Next to each name, you see, first, second, third. This is the degree of separation between you and another member. You see that sprinkled across the whole site, like next to each name, but it also affects things you don’t see. There are visibility rules, for example, that are based on the degree of separation. This affects a bunch of things throughout the whole site.
Another use case served by LIquid is mutual connections. If you look at someone’s profile, you can see the mutual connections, which are essentially my first-degree connections who also happen to be first-degree connections of the other person. That is achieved by a graph traversal query in LIquid. Another query you can do with LIquid is your connections that work at a company. This means, essentially, give me all of my first-degree connections who work at a given company. There’s a bunch more. These graph traversal queries can be used to express even more complex things. For example, I could say, give me all of my second-degree connections that have gone to one of the same schools that I went to, and where the time that we went there overlaps. Essentially, we have a chance of having been classmates. You could do these things with joins in SQL. In some cases, that works great. If the query is a bit complex, if it has self joins, or recursive joins, you can do these things in SQL, but sometimes it starts getting pretty hairy. A graph-oriented language is another tool in the toolkit, and sometimes it makes it much easier to express some of these queries. In some cases, also makes it much more performant as well.
What kinds of derivation are supported in LIquid? We transcode the data from Avro and other formats, as is the case with Pinot, because LIquid has its own representation of the data. We convert the data to triples. That means subject, predicate, object. An example of a triple is member ID connected to member ID. Another example might be member ID works at company ID, or member ID studied at school ID. There can be a variety of things that are represented. Essentially, this is the whole data model. You can represent everything via triples. It’s a very simple, but also very powerful abstraction for the data. This allows us to join together a bunch of disparate datasets that exist throughout our ecosystem. In terms of logical derivation, as data gets ingested into LIquid, you can filter out if there are some records you don’t want in there. You can also do other kinds of massaging to the data to make it more amenable to being queried in the graph.
Use Case – ML Feature Storage
For ML features, we store them primarily in Venice. Again, Venice ingests data from Hadoop and Kafka. Venice was open sourced last year. It is a project I work on. One of the use cases that we serve with Venice is people you may know. Actually, people you may know is served by both LIquid and Venice. The flow of data in this use case goes roughly like this. First of all, we query LIquid to find out what are all the second-degree connections that the logged in user might know. That’s a huge set, way too many to present. There is some early filtering happening right there inside LIquid based on some heuristics that are part of the economic graph. What comes out of that is a set of 5000 candidates. These are people you potentially might know. Five thousand is still a lot, so we want to dwindle that down further. At that point, we turn around and query Venice. For each of the 5000 members, we’re going to query a bunch of ML features that relate to them, and we’re also going to push down some ML feature computation inside Venice. There are embeddings, which are arrays of floats representing the member. We do things like dot product or cosine similarity, these types of vector math inside Venice, and return just a result of that computation. These features that are either fetched directly or computed all come out of Venice, and then are fed into a machine learning model that will do some inference. For each of the members that are part of the candidate set, we will infer a score, which is how likely is it that you might know this person. Then we’ll rank that and return the top K. That’s why you see very few recommendations but hopefully highly relevant ones.
The feed is another example where we’re going to take the posts that have been created by your network. That’s going to be a huge set, again, so we cannot present all of that. We want to do some relevance scoring, for which we retrieve a bunch of ML features out of Venice, and then pass that into an ML inference model, and score each of the posts, rank that, and return the top K most relevant ones. Both of these, people you may know and the feed, are what we call recommender use cases. Venice is great for loading lots of data quickly and frequently. The output of ML training jobs is very high volume. The ML engineers want to refresh that data frequently, maybe once a day or several times a day, the whole dataset. Or, alternatively, they may want to stream in the changes as soon as they happen. Once the data is in Venice, the queries that are supported on the data are fairly simple comparatively to the other systems we looked at. There is NoSQL, or graph traversal, or anything like that, it’s essentially primary key lookups. There is a little more than that, like you can project some fields out of the document, or you can push down some vector math, like I said earlier, to be executed inside the servers, colocated with the data for higher performance. Essentially, these are the types of capabilities that are useful for ML inference workloads.
In terms of the types of derivation supported in Venice, it’s basically all physical. We reorganize the data in certain ways, but we don’t really change the logical content of the data. I mentioned earlier that ML training is a type of logical derivation, but it’s not happening directly inside Venice. It’s happening in some processing framework upstream of Venice, before it lands in Venice. In terms of what happens inside Venice itself, we transcode data from other formats. If the data is already in Avro, then we keep it in Avro, inside Venice. Then we sometimes compress the data if it’s amenable to that, like if there’s a lot of repetition in the data, we have found that using Zstandard compression is extremely effective. What we do there is, because a lot of our data comes from batch pushes, we have all the data there in a Hadoop grid, which is a great place to inspect the data and train a dictionary on it. Then we train a dictionary that is optimized for that specific dataset and push that alongside the data. That allows us to leverage the repetitiveness that exists across records in the dataset. In the most extreme cases, we’ve seen datasets get compressed 40x, a huge compression ratio. In most cases, it’s not that good. Still, it gives us a lot of savings on this system, which is primarily storage bound, lots of data stored in it, and lots of data getting written to it.
Then we put the data in our storage engine, which is RocksDB. We use both of RocksDB’s formats, block based, which is optimized for when the dataset is larger than RAM and you have an SSD for the rest, for the cold data. Then we have the plain table format, which is optimized for the datasets that are all in RAM. Depending on the use case, we choose one or the other. Then, besides that, we join datasets from various sources, like similar idea as in LIquid. We also have a type of derivation that we call collection merging. What that means is, in your Venice table schema, you can define that some column is actually a collection type, like a map or a set. Then, you can add elements into that, one at a time, and Venice will do the merging for you. You can also remove elements in the same way without needing to know what the rest of the collection is. If you want to find out more about Venice, we’ve got our documentation up at venicedb.org. We’ve got all our community resources there, like our Slack instance, our blog posts, and stuff like that, if you want to learn more.
Use Case – Search
The last system I want to cover is for our search use cases. That system is called Galene. Again, it ingests data from batch and streams. An example is the search box you see at the top. If you type Ashish, in there, you’ll see a bunch of people named Ashish, and a few companies also named Ashish, in there. If you typed the right keyword, you could also find schools or pages and so on. This is what we call a federated search. It queries many search verticals, and then coalesces all of that into a single view. Galene is great for retrieving documents that match some predicate that you’re interested in. Then scoring the relevance of each of those documents, which is achieved via arbitrary code and ML models that run directly inside the searchers. Then, ranking those documents by their relevance score, and returning the top K most relevant ones. This is essentially what Galene does. The way that it achieves that is it has two kinds of data structures, which are essentially the way that it physically derives the data coming into it. Actually, there are two flavors of forward index, one for the data that is present in the documents, like maybe the documents are tagged with the author of the document, the date it was modified. These are characteristics that may be useful as part of the search or as part of the ranking. These are stored in a forward index, which looks like the left-hand table here. For doc ID, here is some associated value, like the content or maybe some metadata about the document, like the author, or timestamp, and other things. There’s another flavor of forward index, which is called Da Vinci, which is used for ML features. Da Vinci is actually a subcomponent of Venice, the previous system I talked about. Venice is actually a component inside Galene, and the Da Vinci part is open source. You can reuse that in your own applications if you’d like.
The inverted index is implemented as Lucene, which is the same technology underpinning Solr and Elasticsearch, at least from my understanding. In addition to Lucene, we’ve implemented our own proprietary special sauce on top of that to make it more performant. The inverted index looks roughly like the table on the right-hand side. For each of the tokens that are present in the corpus, for each of these tokens, we store a list of the document IDs that are known to contain these tokens. What do I mean by token? Tokenization is a type of logical derivation that helps make the data easier to search. The simplest form of tokenization could be viewed as a physical derivation. It’s just like split the text by spaces, and each of the words are going to be a token. That can work, but it’s a little simplistic and it doesn’t work super great, because that those tokens are not clean enough. In order to make the tokens more useful for searching, we’re going to do additional things, like we’re going to strip out punctuation, we’ll put them all in lowercase. We might substitute words for some root word, like let’s say, if the language has a notion of gender, of course, all the languages have plural and singular, these are all variants of the same root word. We may index on the root word instead. Then, also, similarly, transform at query time, whatever the user provides into the root word, and then it matches inside the inverted index. You could also do synonyms. All of these allow the tokens to make it more likely that you get a hit when you search.
Here’s a high-level architecture of what the Galene system looks like. This is a simplified architecture. Essentially, you got a request coming from the application hitting a broker node, that will then essentially split the request across a bunch of shards, which are searchers. Each of these searchers has the three data structures I talked about, the inverted index for doing the document retrieval, then the forward index that contains other metadata about the documents that we may want to use for scoring, and ML features that we also use for scoring that are stored in Da Vinci. The reason we have Da Vinci in there is it supports a higher rate of refresh of the data, which our ML engineers prefer. We’ve got all of this inside the searcher, and then the searcher returns the top K most relevant documents that it found, to the broker. The broker coalesces all of those results, does another round of top K, and then returns that to the application.
Conclusion – To Derive or Not to Derive?
First of all, small scale is easier. If your data is of a small scale, maybe you can do everything I just talked about in a single system. You could do everything in Postgres, for example. Why bother having more moving parts, if one will do the job? Even if you’re at a large scale, you could still take advantage of this principle, by trying out an experiment at smaller scale. Let’s say you don’t yet have a dedicated solution for search, and you want to add a search functionality to your business, but you feel like you don’t have the business justification for it. Maybe you can build a very quick and dirty prototype of search on top of your relational database, expose that to just 1% or a 10th of a percent of your users, gather some usage data on that. Maybe that solution is sufficiently scalable for that fraction of your users, and it gives you some useful input about whether that’s a good product market fit. It can help you justify, now that this is demonstrably useful, let’s build a large-scale version of this with a specialized system.
Another way to say what I just said is, use what works until it doesn’t. Make your stack as simple as possible, but no simpler. That’s important, because sometimes having fewer moving parts is what makes your stack simpler, but sometimes fewer moving parts is actually what makes it more complicated. At some point, you reach a level of workload where doing everything all in one system is a liability. If you have a transactional workload that gets negatively impacted by some analytical workload that happens in the same box, that may not be a good thing. In that case, you may think about splitting it into separate systems. Maybe you still choose the same tech on both sides here, maybe Postgres is your transactional, and Postgres is your analytical, and they’re just connected by some replication in between. Maybe that works great, at least for a while. Then, since you have that separation anyway, you could also start feeding that replication stream into a more specialized solution as well. Keep your options open. Most importantly, avoid sunk cost fallacy. Just because your architecture worked five years ago, doesn’t mean it’s still the best today. Your workload constantly grows and changes, so you have to adapt to that and not be beholden to the past design choice. That’s difficult, but try to stay agile and adapt as much as you can.
See more presentations with transcripts
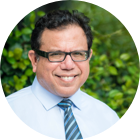
MMS • RSS
Posted on mongodb google news. Visit mongodb google news
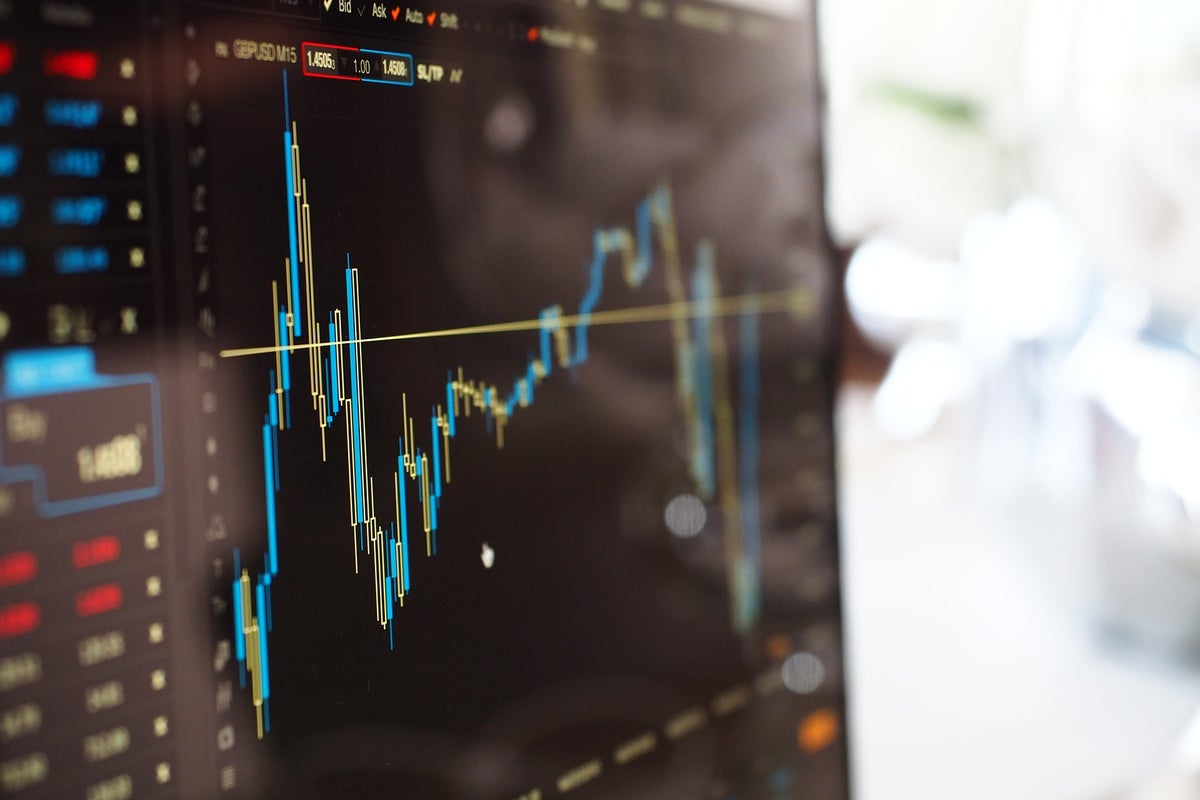
A whale with a lot of money to spend has taken a noticeably bearish stance on MongoDB.
Looking at options history for MongoDB MDB we detected 14 strange trades.
If we consider the specifics of each trade, it is accurate to state that 21% of the investors opened trades with bullish expectations and 78% with bearish.
From the overall spotted trades, 12 are puts, for a total amount of $1,616,969 and 2, calls, for a total amount of $72,877.
What’s The Price Target?
Taking into account the Volume and Open Interest on these contracts, it appears that whales have been targeting a price range from $340.0 to $430.0 for MongoDB over the last 3 months.
Volume & Open Interest Development
Looking at the volume and open interest is a powerful move while trading options. This data can help you track the liquidity and interest for MongoDB’s options for a given strike price. Below, we can observe the evolution of the volume and open interest of calls and puts, respectively, for all of MongoDB’s whale trades within a strike price range from $340.0 to $430.0 in the last 30 days.
MongoDB Option Volume And Open Interest Over Last 30 Days
Biggest Options Spotted:
Symbol | PUT/CALL | Trade Type | Sentiment | Exp. Date | Strike Price | Total Trade Price | Open Interest | Volume |
---|---|---|---|---|---|---|---|---|
MDB | PUT | SWEEP | BEARISH | 09/01/23 | $340.00 | $360.7K | 97 | 897 |
MDB | PUT | SWEEP | BEARISH | 09/01/23 | $340.00 | $292.9K | 97 | 551 |
MDB | PUT | SWEEP | BEARISH | 09/01/23 | $340.00 | $216.7K | 97 | 159 |
MDB | PUT | SWEEP | BEARISH | 09/01/23 | $340.00 | $138.2K | 97 | 401 |
MDB | PUT | TRADE | NEUTRAL | 09/29/23 | $365.00 | $136.3K | 4 | 0 |
Symbol | PUT/CALL | Trade Type | Sentiment | Exp. Date | Strike Price | Total Trade Price | Open Interest | Volume |
---|---|---|---|---|---|---|---|---|
MDB | PUT | SWEEP | BEARISH | 09/01/23 | $340.00 | $360.7K | 97 | 897 |
MDB | PUT | SWEEP | BEARISH | 09/01/23 | $340.00 | $292.9K | 97 | 551 |
MDB | PUT | SWEEP | BEARISH | 09/01/23 | $340.00 | $216.7K | 97 | 159 |
MDB | PUT | SWEEP | BEARISH | 09/01/23 | $340.00 | $138.2K | 97 | 401 |
MDB | PUT | TRADE | NEUTRAL | 09/29/23 | $365.00 | $136.3K | 4 | 0 |
Where Is MongoDB Standing Right Now?
- With a volume of 180,210, the price of MDB is up 0.22% at $361.54.
- RSI indicators hint that the underlying stock may be approaching oversold.
- Next earnings are expected to be released in 6 days.
Options are a riskier asset compared to just trading the stock, but they have higher profit potential. Serious options traders manage this risk by educating themselves daily, scaling in and out of trades, following more than one indicator, and following the markets closely.
If you want to stay updated on the latest options trades for MongoDB, Benzinga Pro gives you real-time options trades alerts.
Article originally posted on mongodb google news. Visit mongodb google news
Senior Database Administrator – MySQL / NoSQL / Tuning / Optimization (m/f/d) in Hamburg
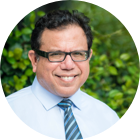
MMS • RSS
Posted on nosqlgooglealerts. Visit nosqlgooglealerts
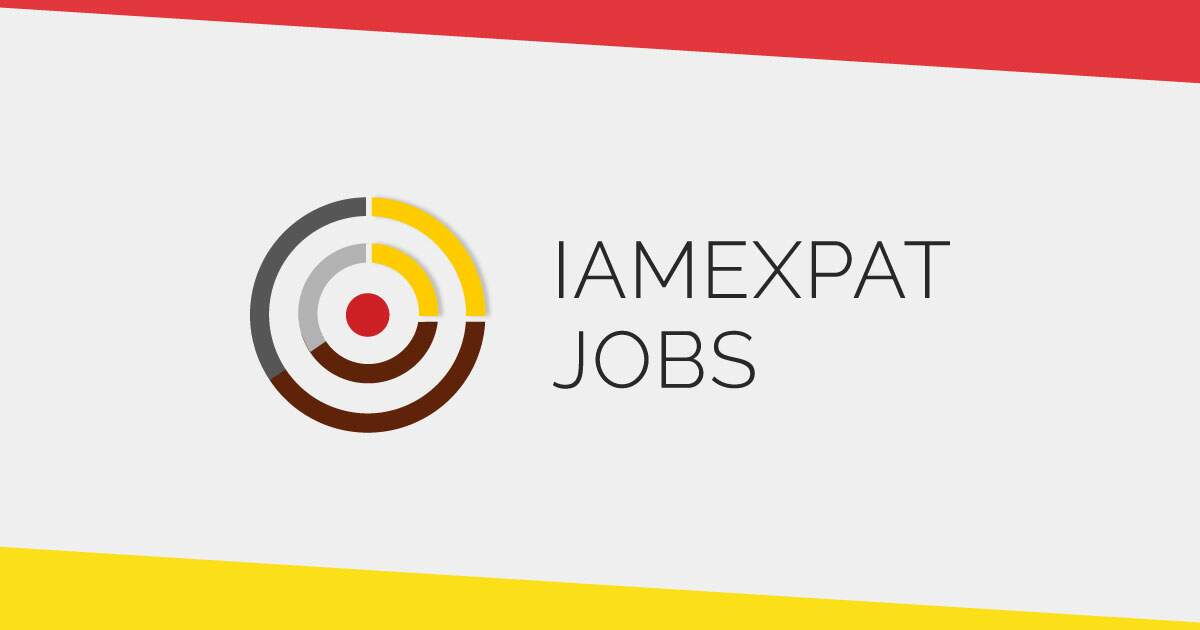
Gravite, a registered brand of AddApptr, a global mobile ad tech company dedicated to optimizing revenue for app publishers, is looking for a Database Administrator (f/m/d) to join our engineering team in the beautiful city of Hamburg!
We are a leading provider of mobile programmatic advertising solutions for major app publishers, offering our services to numerous global companies who rely on our technology and solutions to optimize their revenue models. With billions of ad requests optimized every month, our mission is to generate maximum advertising revenue for our clients.
What can you expect?
You will join a dynamic and communicative team with a truly international and multicultural atmosphere, constantly striving to build a successful business.
In our team, your goal will be to ensure that our databases run smoothly and efficiently, while communicating and supporting a variety of interdisciplinary teams and users.
What should you bring along?
- You have at least four years of proven experience administering relational databases through MySQL or MariaDB in a Linux environment
- Your profile is well-rounded by 3+ years of experience administering column oriented databases on a Linux platform. Ideally in ClickHouse, MySQL or and other comparable tool.
- You bring along experience with set up, installation, maintenance, monitoring, and troubleshooting of databases, with tuning of database parameters to ensure optimal performance as well as experience with optimizing data models and queries
- You are dedicated and enjoy working with huge amounts of data
- You preferably worked with Debian Linux and Clickhouse before
- You offer very good kledge of the English or German language
What do we offer?
- Attractive and performance-based compensation: Continue to benefit from additional benefits such as lucrative employee vouchers, bicycle leasing (JobRad), company cell phone and subsidized transportation ticket.
- Attractive working environment: Whether remote, hybrid or on-site. Our modern work models, flexible working hours and an attractive vacation package allow you to achieve a good work-life balance.
- Room for co-design and further development: With us, everyone can contribute and drive topics forward. We work at eye level, with agile dynamics and flat hierarchies. We offer a wide range of internal and external training opportunities for your personal and professional development.
- We offer the opportunity to work in a team of top experts with the leading players in the industry and to actively shape one of the most technologically exciting tech areas. You will work in the atmosphere of a startup and enjoy all the benefits of an international environment with flat hierarchies and short decision-making paths.
- A unique corporate culture with regular team events and lunches, sponsorship of language courses to drive outstanding ideas and solutions, where strong commitment and employee well-being is fundamental.
Our job offer Senior Database Administrator – MySQL / NoSQL / Tuning / Optimization (m/f/d) sounds interesting? Then we are looking forward to receiving your
application via Campusjäger by Workwise.With our partner Campusjäger, you can apply for
this job in just a few minutes without a cover letter and track the status of your
application live.
Work Hours:
40 hours per week hours per week
About the company:
We are an independently owned and operated global mobile ad tech company dedicated to optimizing revenue for app publishers.
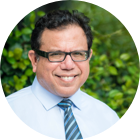
MMS • RSS
Posted on mongodb google news. Visit mongodb google news
US Bancorp DE raised its holdings in MongoDB, Inc. (NASDAQ:MDB – Free Report) by 24.0% during the first quarter, according to the company in its most recent Form 13F filing with the Securities & Exchange Commission. The fund owned 2,740 shares of the company’s stock after acquiring an additional 530 shares during the period. US Bancorp DE’s holdings in MongoDB were worth $639,000 at the end of the most recent quarter.
Other hedge funds and other institutional investors have also recently bought and sold shares of the company. GAM Holding AG acquired a new stake in shares of MongoDB during the first quarter valued at about $5,394,000. Clearstead Advisors LLC acquired a new stake in shares of MongoDB during the first quarter valued at about $36,000. Y Intercept Hong Kong Ltd acquired a new stake in shares of MongoDB during the first quarter valued at about $2,591,000. Banco Bilbao Vizcaya Argentaria S.A. boosted its stake in shares of MongoDB by 24.1% during the first quarter. Banco Bilbao Vizcaya Argentaria S.A. now owns 1,369 shares of the company’s stock valued at $312,000 after purchasing an additional 266 shares in the last quarter. Finally, Brighton Jones LLC acquired a new stake in shares of MongoDB during the first quarter valued at about $1,270,000. Institutional investors own 89.22% of the company’s stock.
Insiders Place Their Bets
In other news, Director Dwight A. Merriman sold 6,000 shares of the firm’s stock in a transaction on Friday, August 4th. The stock was sold at an average price of $415.06, for a total value of $2,490,360.00. Following the completion of the transaction, the director now directly owns 1,207,159 shares of the company’s stock, valued at $501,043,414.54. The transaction was disclosed in a filing with the Securities & Exchange Commission, which is available through this hyperlink. In related news, Director Dwight A. Merriman sold 6,000 shares of MongoDB stock in a transaction on Friday, August 4th. The stock was sold at an average price of $415.06, for a total transaction of $2,490,360.00. Following the completion of the sale, the director now owns 1,207,159 shares of the company’s stock, valued at $501,043,414.54. The transaction was disclosed in a filing with the Securities & Exchange Commission, which is available through this link. Also, Director Dwight A. Merriman sold 1,000 shares of MongoDB stock in a transaction on Tuesday, July 18th. The shares were sold at an average price of $420.00, for a total value of $420,000.00. Following the sale, the director now directly owns 1,213,159 shares of the company’s stock, valued at approximately $509,526,780. The disclosure for this sale can be found here. Insiders sold 102,220 shares of company stock valued at $38,763,571 over the last ninety days. 4.80% of the stock is currently owned by insiders.
Analyst Upgrades and Downgrades
MDB has been the subject of several analyst reports. Guggenheim lowered shares of MongoDB from a “neutral” rating to a “sell” rating and raised their price target for the stock from $205.00 to $210.00 in a report on Thursday, May 25th. They noted that the move was a valuation call. KeyCorp upped their target price on MongoDB from $372.00 to $462.00 and gave the company an “overweight” rating in a research report on Friday, July 21st. Citigroup increased their price target on MongoDB from $363.00 to $430.00 in a report on Friday, June 2nd. Morgan Stanley increased their price target on MongoDB from $270.00 to $440.00 in a report on Friday, June 23rd. Finally, Mizuho increased their price target on MongoDB from $220.00 to $240.00 in a report on Friday, June 23rd. One equities research analyst has rated the stock with a sell rating, three have issued a hold rating and twenty have assigned a buy rating to the stock. Based on data from MarketBeat.com, the company has an average rating of “Moderate Buy” and a consensus target price of $378.09.
Read Our Latest Analysis on MongoDB
MongoDB Stock Performance
NASDAQ:MDB opened at $360.75 on Friday. The company has a debt-to-equity ratio of 1.44, a current ratio of 4.19 and a quick ratio of 4.19. The business has a fifty day simple moving average of $391.10 and a two-hundred day simple moving average of $297.69. MongoDB, Inc. has a 1 year low of $135.15 and a 1 year high of $439.00. The company has a market capitalization of $25.46 billion, a P/E ratio of -77.25 and a beta of 1.13.
MongoDB (NASDAQ:MDB – Get Free Report) last released its earnings results on Thursday, June 1st. The company reported $0.56 earnings per share (EPS) for the quarter, topping analysts’ consensus estimates of $0.18 by $0.38. MongoDB had a negative return on equity of 43.25% and a negative net margin of 23.58%. The business had revenue of $368.28 million during the quarter, compared to the consensus estimate of $347.77 million. During the same period last year, the business posted ($1.15) earnings per share. The business’s revenue for the quarter was up 29.0% on a year-over-year basis. On average, analysts expect that MongoDB, Inc. will post -2.8 earnings per share for the current year.
MongoDB Company Profile
MongoDB, Inc provides general purpose database platform worldwide. The company offers MongoDB Atlas, a hosted multi-cloud database-as-a-service solution; MongoDB Enterprise Advanced, a commercial database server for enterprise customers to run in the cloud, on-premise, or in a hybrid environment; and Community Server, a free-to-download version of its database, which includes the functionality that developers need to get started with MongoDB.
See Also
Want to see what other hedge funds are holding MDB? Visit HoldingsChannel.com to get the latest 13F filings and insider trades for MongoDB, Inc. (NASDAQ:MDB – Free Report).
Receive News & Ratings for MongoDB Daily – Enter your email address below to receive a concise daily summary of the latest news and analysts’ ratings for MongoDB and related companies with MarketBeat.com’s FREE daily email newsletter.
Article originally posted on mongodb google news. Visit mongodb google news
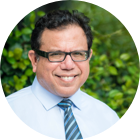
MMS • Pia Nilsson
Article originally posted on InfoQ. Visit InfoQ
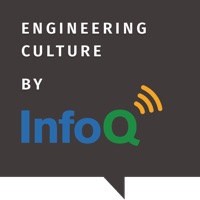
Subscribe on:
Transcript
Shane Hastie: Hey folks. Before we get into today’s podcast, I wanted to share that InfoQ’s international software development conference, QCon will be back in San Francisco from October two to six. QCon will share real world technical talks from innovative senior software development practitioners on applying emerging patterns and practices to address current challenges. Learn more at qconsf.com. We hope to see you there.
Good day, folks. This is Shane Hastie for the InfoQ Engineering Culture podcast. Today I’m sitting down across many, many miles with Pia Nilsson. Pia is the lead platform developer, experience lead at Spotify. Did I get that right Pia?
Pia Nilsson: That’s right.
Shane Hastie: Welcome. Thanks very much for taking the time to talk to us today. The place I like to start with my guests is who’s Pia?
Introductions [00:58]
Pia Nilsson: Thanks for having me. Delighted to be on the podcast. So who am I? I’ve been an engineer for 14 years and then I went into leadership, so about seven years of that. I think my passion is platform engineering or has become over the years platform engineering because I sort of during my engineering years got tired of re-implementing stuff. So the duplication became very evident to me and I wanted to help. So I gravitated towards working in the DevOps space. So with CICDs, I joined Spotify to lead the CICD team, which is a passion of mine as well. So I think that’s who I am.
Shane Hastie: What is developer effectiveness?
Developer effectiveness is about flow [01:42]
Pia Nilsson: Oh, that’s a fantastic question. I want to say flow, because if you go back to yourself, when do you feel effective? I believe it’s when you are informed about the context around you and enabled to keep your flow. So we do measure this at Spotify in our engineering satisfaction survey every quarter, and this is a real metric that we sort of try to help us inform decisions on, are these tools that we’re providing to our engineers actually helping them remain in flow or are they being interrupted?
Shane Hastie: Are the tools helping with flow? To delve into the cause-effect relationship here? How do you know? Is it the tool or is it something else?
Pia Nilsson: Well, exactly. It is not only the tools, because flow is something that is created within sort of a team that you’re in, and the context you’re in, and the tools you’re working with. It’s all of that at play together. So what we of course have discovered is psychological safety of course matters a whole lot for a team to be effective and also just in general interruptions and being empowered matters a lot. So we have a very strong culture of autonomy at Spotify and I believe that is something that I have started to really connect with. People who feel empowered usually are more often in flow than people who aren’t feeling empowered and it’s a mix of so many different things.
Shane Hastie: One of the things I’ve noticed working with organizations, almost to the point of being a bit cynical, psychological safety has become a buzzword. Of course we have a psychologically safe environment, you are empowered, but you made a strong statement there. People that feel empowered versus what’s being said. And I’ve often seen that, of course my people are empowered, when actually they don’t really feel that way. So how do you make it real?
Building products developers want to adopt [03:49]
Pia Nilsson: Yes, that’s a great point. So I totally agree with, it’s a challenge for us as an industry, I believe, to really nail. Well, how do we get there? Everyone believes in psychological safety, but how do we actually get there in an authentic way? I think we sort of stumbled upon it a bit at Spotify. So I have seen one way of getting there in an authentic way. As a platform organization at Spotify, we didn’t have the mandate to say to our 4,000 engineers, these are the tools you’re going to use. These are the standards you’re going to follow. That mandate did not exist actually to a very large extent. You have to have some mandate when it comes to some sort of compliance and security of course, but then there is a lot more than that and there was no mandate. It was pure autonomy basically, which led to a technical sprawl I had never seen before.
So the lack of mandate for a platform organization that care about rolling out best practices led us to building products that people wanted to adopt because that was the only way we could affect change in the engineering population. Well, if you need to build products that people want to adopt, you automatically become customer oriented and you have empathy for your customers. And also we kind of, of course, identified with the customer a whole lot because we were using our products ourselves, we were applying them to ourselves, which also dogfooding is also I think a really good way of getting there in an authentic way.
Shane Hastie: Oh, dogfooding. I so much prefer drinking our own champagne. I don’t want to eat any dog food personally. So thinking of that, drinking our own champagne, using the tools ourselves and building that empathy. When your customers are technologists, that’s a very, very different… Or is it a different mindset to thinking about the consumer customer?
Pia Nilsson: I would really think so, Yes. I think the people we recruit, the highly skilled engineers, the product managers, engineering managers and so forth, they need to co-create our solutions because they come in and join our organization and they kind of know how to be effective already. So I think it’s very important to not think that we sort of have the solution and then we’re going to help people use it, because I think it’s way more of a mutual relationship when you’re building tools for an engineering population, because effectiveness is a little bit elusive, so it needs to be co-created. Maybe that sounds a little too fluffy, but I think this simple need of actually enjoying using a tool, enjoying work matters a whole lot when it comes to actually being in flow.
Shane Hastie: Enjoying work, but we want people to be busy. We want to use their time efficiently. Or do we?
Pia Nilsson: That’s a very common, I feel, misunderstanding. I held that belief myself in the early days of my career. I was very, very busy. And then I see when we have hack weeks at Spotify, I’m not just speaking about the annual hack week, we have often hack weeks on smaller levels as well. There are just so many great things coming out of hack week. It’s like the hive mind all of a sudden produce these great features and ideas. I’ve heard people speak about over a coffee, but nobody had time to take a look at it if it actually is some substance in this idea. So I’m sort of realizing that effectiveness is when you actually let someone who is passionate about what they’re doing actually think freely more often. We can take leaps instead of steps. That’s how I feel. I kind of want to have hack weeks, I don’t know, at least once a quarter. It’s incredibly effective I feel.
Shane Hastie: I like that. Taking leaps instead of steps. One of the things that you’ve been very involved in is Backstage, which Spotify has released into the wilds and makes freely available. Tell us a little bit about that.
Introducing Backstage [06:08]
Pia Nilsson: Yes, Backstage was launched in 2018 at Spotify, built upon an already existing system that was only targeting backend services at the time. So out of that grew Backstage and we created it because there was such a clear need from our engineers that they couldn’t find the things in our infrastructure anymore. This technical sprawl had become just quite overwhelming. We had an onboarding time that we measure over 60 days, which our target date was 20 and that onboarding metric is measured like how many days does it take an engineer to merge their 10th pull request. At the time, it was a widely spread metric to use, so we had borrowed it from some of our peers. So people couldn’t find things and also people were interrupted very often. They were saying, because we guessed that was because other people couldn’t find things and tapping these folks on the shoulder.
We called it, we had rumour driven development because you actually had to speak to someone in order to understand how to integrate with a certain API, which of these APIs actually finding the docs, et cetera, finding the updated docs if there were any, et cetera. So we really relied heavily on personal connections and you can also imagine that also is not very inclusive. So unintentionally that kind of culture with a lack of documentation, lack of clear interfaces, it wasn’t easy to onboard, it wasn’t easy to feel included and empowered in this environment. So out of that grew Backstage sort of. We wanted to democratize knowledge about the infrastructure, so you should be able to find, create, and manage your components, your team’s components without having to lean on anyone really for these very simple sort of obvious tasks that everyone wants to do on the first day at work. So that’s how Backstage was created.
Shane Hastie: And what’s happened since you’ve released it into the wild, into the community?
Pia Nilsson: Yes, it’s been fantastic. We decided to open source at 2020 because we were relying on it so heavily internally and we needed to protect it from another industry standard coming along, which we knew we would then have to migrate to, as Backstage was an internally built developer portal. So we open sourced and donated it to the CNCF to protect ourselves from a migration. And then the interest in Backstage just skyrocketed. It’s fantastic. Today we have, I don’t know, 1,300 customers that are using Backstage in production. I mean, since 2020. So they are now pretty mature Backstage users, many of these companies. And we estimate it’s like 1.4 million developers are using Backstage right now. So the interest is just massive and we’re incredibly happy about this. So we are spending a lot of time supporting the open source product.
Shane Hastie: One of the things we were chatting about earlier is the silver bullet thinking that is still prevalent in the industry that we’ll install something, so we’ll install Backstage, magically a miracle will occur and our developer experience will be magnificent. Of course, that’s not the reality. What are the steps? How do we get from, oh, we want this product to, we’ve actually got improved developer experience?
Improving developer experience is a journey over time, there is no silver bullet [11:29]
Pia Nilsson: Yes, that’s a lovely question. I think the more we as an industry think about what are the concrete challenges in my organization that my engineering population are experiencing, the more successful we would be with any developer tool really. So when it comes to Backstage, even though I’m really convinced this is a helpful tool for any engineering organization that has the same kind of problems Spotify had as in technical sprawl and just visibility of your infrastructure, it’s still very important to look sort of self-awareness for the company adopting. I’ve spoken to so many companies and they do have nuances of differences in what are our challenges. Some companies, their main challenge is how to onboard quicker because for several reasons that may be very technically challenging and you need to jump through a hundred hoops in order to actually onboard. Other are more like Spotify. We had this huge technical sprawl.
I mean, we had the onboarding problem as well, but huge technical sprawl, and we had to focus on how do we drive best practices and standards across our fleet. So I think the first step would be to really decide on which problem to pick, because that’s going to help build enthusiasm and understanding for rolling out any developer portal really. So if you identify that problem of yours, then set up some fantastic success metrics that everyone can sort of see, this would be a great thing. Like we did, we had the onboarding metric. Everyone across all layers in the company agreed that that would actually matter, if we could get that down to 20. And after that, then the steps are becoming more and more clear because you have a little bit more clear target because the developer portal clinic can of course be useful in so many different ways, but it’s still very good to start with one problem.
Shane Hastie: That organizational self-awareness though is often uncomfortable and again, in my experience, frequently hard to get. How do we, I want to say, overcome the egos, overcome the sensitivity even around having these metrics?
The challenges around metrics [13:43]
Pia Nilsson: I think the challenges I’ve seen aren’t around sensitivity around the metrics, but the sensitivities around already existing products within an organization that then would have to comply with this new idea, this new developer portal that the platform organization want to roll out. That’s been the challenge that I’ve seen in many customer places. What are your thoughts on the metrics? What metrics were you thinking of there?
Shane Hastie: Well, the ultimate metrics for any commercial organization need to be things like employee engagement, customer satisfaction, time to market, profitability. So those have got to be at the top. But then how do we cascade down to something that, I love the example that you’re using of employee onboarding time to 10th pull request. Beautiful, because it’s very, very explicit and can be clearly defined. To tease out those useful metrics can be, I’m going to say a challenge particularly, and maybe I’m being unfair, but in organizations with a lot of politics. This one I see being difficult, some of these.
Pia Nilsson: Yes, that’s very true. I think the way I’ve seen it successful, and it took us a few years to get this right too, is to really go to the organization within your company, approach these leaders with empathy sort of, and help them co-create metrics that would matter to them. For example, if you want to onboard the security, whatever developed portal to your Backstage instance. So that would be one way of organizationally sort of gluing these pieces together, but also technically just having them integrate with Backstage. I think the way I’ve seen us do that in a successful manner and also at customer sites is to approach them with respect. So it’s very important that these internal customers of Backstage are the champions. They’re actually domain expertise and we need to be careful. They are leading their own spaces. I think one of the challenges we are trying to fight often at Spotify is centralization versus decentralization.
So if you have this example of security versus sort of the centralized Backstage team, one has to, instead of saying, we’re going to centralize all of it, then you’re going to just have to comply with exactly how we would like you to inform your customers, et cetera. One really needs to go at it from the completely different angle, as in how do security want to speak with their customers? How could the Backstage team empower them to do so? What toil do they have today that we can remove so that they want to onboard Backstage?
And this is just a very concrete example, but I think if you sort of apply this approach, then these success metrics for developer effectiveness, they don’t tend to become that sensitive any longer because I think the sensitivity is coming from lack of control. So if you steal the control, if I would steal the control from security in this example, certainly I would create a very sensitive topic. They would feel measured, big brotherly treated, et cetera, and then you will really never have real adoption. You will always have to be in this struggle of trying to get their features deployed to the Backstage instance, and it will not sort of fly. Again, they need to want to use this.
Shane Hastie: A term I often use when working with organizations and embracing new ways of working in any area is the concept of invitation over imposition and bringing people along on the journey. And I love what you were saying earlier about that co-creation and people feeling engaged, and that’s how people do start to feel empowered. Empowered comes from within, not from without, in my experience.
Pia Nilsson: Yes. I think one has to be pretty passionate about people in order to reach effectiveness and really empathize with, well, what if I was that receiving engineer? Would I have appreciated this? Then the answers become pretty straightforward.
Shane Hastie: So we come back to culture, don’t we? That big term that is in many ways nebulous, but is really at the core of every successful organization. I’m going to make that assertion anyway, is the culture that makes people want to be there and to do good work. Spotify is held up as an organization that does have an amazing engineering culture. What do you think puts you on the spot, make Spotify that good? What’s the secret sauce? Is there a secret sauce?
What makes the Spotify engineering culture great? [18:35]
Pia Nilsson: I think it has to do with the origin story of Spotify R&D organization. That’s at least how I have felt when I joined Spotify 2016. One of the many differences I noticed was how very autonomous people were feeling. That led to a lot of challenges in technical sprawl, blah, blah, blah, all of that. Too much isolated work, but also it was this deep care for sort of your craft and a lot of joy on an everyday basis, which really influenced me. It was a different way of working that I have seen, at least. And while we needed to work on, yes, we need some best practices overall and we need to have a bit fewer differences between the technical stacks on how to, for example, the CI systems, so everyone had their own built Jenkinses or something else of their choice to build their services.
Those were obvious sort of things we need to fix, but that core belief in, well, people are the whole value of this company. I think that has been why it has been fairly easy for Spotify platform organization to invest in bringing product managers along already 2016 when that was very rare actually, to have product folks leading platform tools and development as well as designers, even more rare I would say. So very early on because of this culture that we’re building for ourselves and we respect the people at Spotify, working at Spotify. I think that is sort of the secret sauce as I see it.
Shane Hastie: Thank you very much. That’s a lovely insight. Pia, there’s a lot of ideas that we’ve explored here together, and thank you very much for your time. If people want to continue the conversation, where do they find you?
Pia Nilsson: Well, they can always reach out to me at Spotify, or they can reach out to the Backstage organization if they are interested in listening in. So backstage.spotify.com. And join our community meetings as well. They would find that on the page to learn more and get in touch with a bunch of Spotifyers and our customers, of course. So there are many ways.
Shane Hastie: Wonderful. Again, thank you so much for your time.
Pia Nilsson: Thanks for having me.
Mentioned
.
From this page you also have access to our recorded show notes. They all have clickable links that will take you directly to that part of the audio.