Month: September 2023
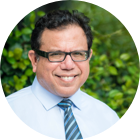
MMS • Sergio De Simone
Article originally posted on InfoQ. Visit InfoQ
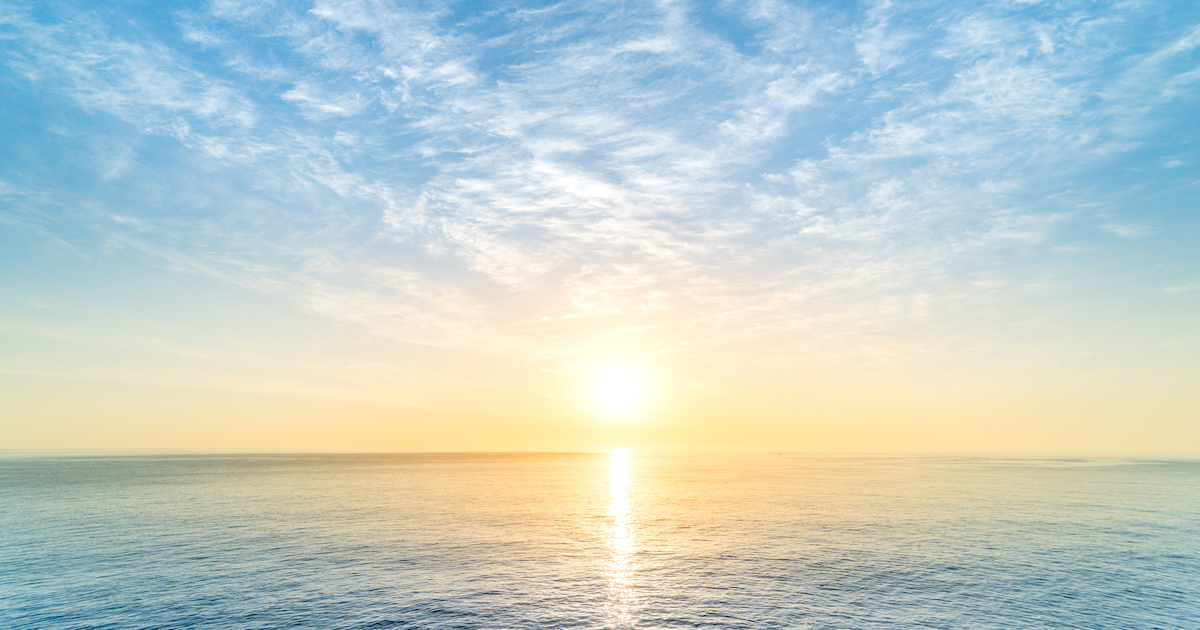
After launching Dependabot’s auto-dismiss policies a few months ago to reduce the number of false positive alerts, GitHub is now adding custom rules support for developers to define the criteria to auto-dismiss and reopen alerts.
While solutions like Dependabot promise to help improve security by automatically identifying vulnerabilities in a project’s dependencies, all comes at a price. With Dependabot this is related to the number of false positive the system may generate, i.e., alerts that do not correspond to a real threat but still require a developer’s attention for manual assessment and triage. False positives include vulnerabilities that are unlikely to be exploited or glitches in the system associated to long-running builds or tests.
In fact, this has led to the creation of the notion of “alert fatigue”, whereas, according to GitHub, at least one in ten alerts are false positives. Paradoxically, alert fatigue could distract developers from addressing real vulnerabilities. To mitigate this situation, GitHub introduced a number of general policies aimed to reduce the volume of false positives by dismissing low impact issues for development-scoped dependencies (a.k.a, devDependencies
in the npm world).
Encouraged by developers’ adoption of the new feature, GitHub is now making a further step in the direction of reducing alert fatigue by enabling the definition of custom rules to control how Dependabot auto-dismisses alerts. This includes two new options, which will either dismiss or snooze an alert until a patch is available or indefinitely.
When defining a rule, developers specify a set of criteria that determine when the rule applies. This include the package name, the vulnerability severity, the ecosystem, the manifest, the scope, and the security advisories. When a rule matches, the alert will be dismissed, either indefinitely or until a patch is available.
In the initial release, rules can be defined on a repository-by-repository base, with organization-level rules coming soon, say GitHub. In future, auto-dismiss rules will expand metadata that can be used as well as available remediation flows, additionally say the organization.
Custom auto-triage rules are available for free on all public repositories, and at a premium for private repositories.
Dependabot, introduced in 2019, is able to scan a project’s dependencies for any vulnerabilities and automatically open PRs for each of them, allowing maintainers to fix security vulnerabilities by simply merging those PRs.
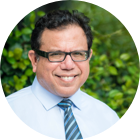
MMS • RSS
Posted on nosqlgooglealerts. Visit nosqlgooglealerts
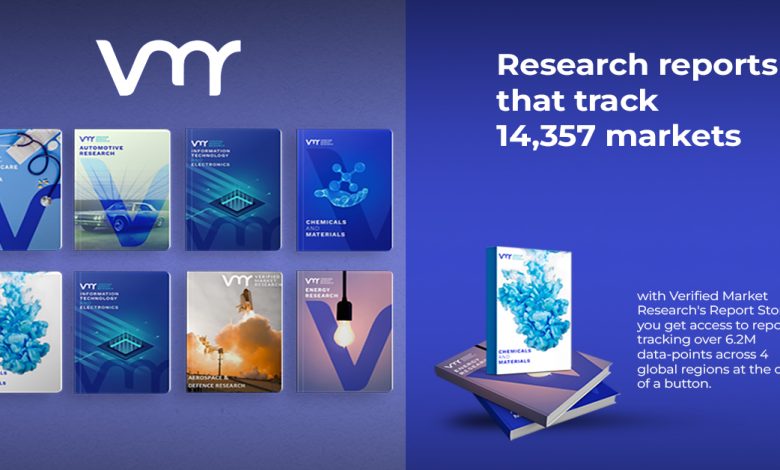
New Jersey, United States – The report on the Global NoSQL Software Market provides a comprehensive and accurate overview of the industry, considering various factors like competition, regional growth, segmentation, and market size in terms of value and volume. It serves as an exceptional research study, offering the latest insights into critical aspects of the Global NoSQL Software market. The report includes market forecasts concerning size, production, revenue, consumption, CAGR, gross margin, price, and other key factors. To ensure reliability, industry-best primary and secondary research methodologies and tools have been employed in its preparation. It encompasses several research studies, such as manufacturing cost analysis, absolute dollar opportunity, pricing analysis, company profiling, production and consumption analysis, and market dynamics.
A crucial aspect for every key player is understanding the competitive landscape. The report sheds light on the competitive scenario of the Global NoSQL Software market, providing insights into competition at both domestic and global levels. Market experts have outlined key aspects of each leading player in the Global NoSQL Software market, including areas of operation, production, and product portfolio. Additionally, the companies in the report are analyzed based on key factors such as company size, market share, market growth, revenue, production volume, and profits.
NoSQL Software Market is growing at a moderate pace with substantial growth rates over the last few years and is estimated that the market will grow significantly in the forecasted period i.e. 2021 to 2028.
Get Full PDF Sample Copy of Report: (Including Full TOC, List of Tables & Figures, Chart) @ https://www.verifiedmarketresearch.com/download-sample/?rid=153255
Leading 10 Companies in the Global NoSQL Software Market Research Report:
Amazon, Couchbase, MongoDB Inc., Microsoft, Marklogic, OrientDB, ArangoDB, Redis, CouchDB, DataStax.
Global NoSQL Software Market Segmentation:
NoSQL Software Market, By Type
• Document Databases
• Key-vale Databases
• Wide-column Store
• Graph Databases
• Others
NoSQL Market, By Application
• Social Networking
• Web Applications
• E-Commerce
• Data Analytics
• Data Storage
• Others
The report comes out as an accurate and highly detailed resource for gaining significant insights into the growth of different product and application segments of the Global NoSQL Software market. Each segment covered in the report is exhaustively researched about on the basis of market share, growth potential, drivers, and other crucial factors. The segmental analysis provided in the report will help market players to know when and where to invest in the Global NoSQL Software market. Moreover, it will help them to identify key growth pockets of the Global NoSQL Software market.
The geographical analysis of the Global NoSQL Software market provided in the report is just the right tool that competitors can use to discover untapped sales and business expansion opportunities in different regions and countries. Each regional and country-wise Global NoSQL Software market considered for research and analysis has been thoroughly studied based on market share, future growth potential, CAGR, market size, and other important parameters. Every regional market has a different trend or not all regional markets are impacted by the same trend. Taking this into consideration, the analysts authoring the report have provided an exhaustive analysis of specific trends of each regional Global NoSQL Software market.
Inquire for a Discount on this Premium Report @ https://www.verifiedmarketresearch.com/ask-for-discount/?rid=153255
What to Expect in Our Report?
(1) A complete section of the Global NoSQL Software market report is dedicated for market dynamics, which include influence factors, market drivers, challenges, opportunities, and trends.
(2) Another broad section of the research study is reserved for regional analysis of the Global NoSQL Software market where important regions and countries are assessed for their growth potential, consumption, market share, and other vital factors indicating their market growth.
(3) Players can use the competitive analysis provided in the report to build new strategies or fine-tune their existing ones to rise above market challenges and increase their share of the Global NoSQL Software market.
(4) The report also discusses competitive situation and trends and sheds light on company expansions and merger and acquisition taking place in the Global NoSQL Software market. Moreover, it brings to light the market concentration rate and market shares of top three and five players.
(5) Readers are provided with findings and conclusion of the research study provided in the Global NoSQL Software Market report.
Key Questions Answered in the Report:
(1) What are the growth opportunities for the new entrants in the Global NoSQL Software industry?
(2) Who are the leading players functioning in the Global NoSQL Software marketplace?
(3) What are the key strategies participants are likely to adopt to increase their share in the Global NoSQL Software industry?
(4) What is the competitive situation in the Global NoSQL Software market?
(5) What are the emerging trends that may influence the Global NoSQL Software market growth?
(6) Which product type segment will exhibit high CAGR in future?
(7) Which application segment will grab a handsome share in the Global NoSQL Software industry?
(8) Which region is lucrative for the manufacturers?
For More Information or Query or Customization Before Buying, Visit @ https://www.verifiedmarketresearch.com/product/nosql-software-market/
About Us: Verified Market Research®
Verified Market Research® is a leading Global Research and Consulting firm that has been providing advanced analytical research solutions, custom consulting and in-depth data analysis for 10+ years to individuals and companies alike that are looking for accurate, reliable and up to date research data and technical consulting. We offer insights into strategic and growth analyses, Data necessary to achieve corporate goals and help make critical revenue decisions.
Our research studies help our clients make superior data-driven decisions, understand market forecast, capitalize on future opportunities and optimize efficiency by working as their partner to deliver accurate and valuable information. The industries we cover span over a large spectrum including Technology, Chemicals, Manufacturing, Energy, Food and Beverages, Automotive, Robotics, Packaging, Construction, Mining & Gas. Etc.
We, at Verified Market Research, assist in understanding holistic market indicating factors and most current and future market trends. Our analysts, with their high expertise in data gathering and governance, utilize industry techniques to collate and examine data at all stages. They are trained to combine modern data collection techniques, superior research methodology, subject expertise and years of collective experience to produce informative and accurate research.
Having serviced over 5000+ clients, we have provided reliable market research services to more than 100 Global Fortune 500 companies such as Amazon, Dell, IBM, Shell, Exxon Mobil, General Electric, Siemens, Microsoft, Sony and Hitachi. We have co-consulted with some of the world’s leading consulting firms like McKinsey & Company, Boston Consulting Group, Bain and Company for custom research and consulting projects for businesses worldwide.
Contact us:
Mr. Edwyne Fernandes
Verified Market Research®
US: +1 (650)-781-4080
US Toll-Free: +1 (800)-782-1768
Email: sales@verifiedmarketresearch.com
Website:- https://www.verifiedmarketresearch.com/
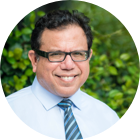
MMS • Mark Saroufim
Article originally posted on InfoQ. Visit InfoQ
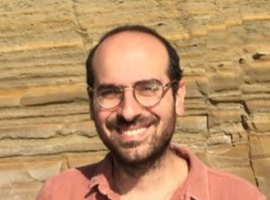
Transcript
Saroufim: I’m Mark Saroufim. I’m here to give you a talk titled, “An Open Source Infrastructure for PyTorch.” I’m an applied AI Engineer here at Meta. Some people think I spend the majority of my time on Twitter, so you can follow me there for my updates. For the most part, I have the luxury of having the majority of my work be out in the open, so you can go check me out on GitHub, for the latest and greatest. Let’s unpack this title a bit more. Why is it that open source is important? What does it really mean to have an infrastructure that’s open source? We all know what PyTorch is. PyTorch is a language to author machine learning models. What does it really mean to have an infrastructure around it? Let’s just dive deeper. The PyTorch organization maintains a bunch of projects that are more related to how do we actually use PyTorch in production. There’s a couple that I personally work on, like PyTorch Serve used for model inferencing, where I’m one of the maintainers. I also contribute to a bunch of more projects like PyTorch Core, Serve, TorchX for infrastructure management. Torchdata for dataset management. An example is just more of an educational repro. My charter is, how do we make it so PyTorch is a joy to deploy in production? This photo on the right is me right at work, right there on the GitHub interface.
Outline
One way of viewing the agenda for this talk is going to be this. We’re going to have lots of digressions. I’m sure you’ve seen lots of pictures by lots of people describing how to do machine learning, and these are all the tools you should use. This is not going to be one of those talks. In this talk really the reason why I’m categorizing things into subproblems, there’s more to talk about them. Ideally, I’m really here to show you what’s interesting about a lot of those problems. It’s really just to get you curious about those subproblems. Just because all lines are generally helpful, the way to think about it is that you’re going to have some data, and that data is not going to be in a tensor format. It may be some images. It may be some text. It may be some audio snippets. How do we actually load, decode, preprocess that data? The tools we’re going to talk about within this context, are what we call the PyTorch domain libraries. There’s going to be things like torchvision, torchtext, torchaudio. There’s going to be our data loading libraries, so things like torchdata. Then when we’re defining a model architecture, hopefully, you’ve had an experience already defining some model with PyTorch. It’s actually really easy. If you don’t want to do that, there’s also pretrained models that you can get from companies like Weights & Biases.
Even then, something that’s becoming more important as you may have heard from the news like, models are getting bigger. What does it really mean to define a large model? We’re going to be discussing tools like DDP and FSDP. That’s distributed data parallel and fully sharded data parallel. Then we’re going to discuss, how do you actually deploy those models. You want to launch a training job, but then if it’s a large job with multiple nodes in it, some of those nodes could fail? What does that really mean? How do you check for the job status when the job is occurring over multiple machines? Of course, after deploying those models, you may realize, this model that I’m trying to train is not cost effective at all, and it is going to take months or years to train. What are the various tools that people use to make models faster, ranging from quantization, or some more inference specific solutions like TensorRT, IPEX, and ONNX. Finally, once we’ve done all of that, and we’ve defined the exact models and weights that we want to serve in production, what does serving a model in production really mean? Here I’m going to talk to you more about TorchServe.
PyTorch Foundation – Democratic Governance
Some piece of news that we’re really excited about and sets the context for why open source is important is the PyTorch Foundation. The goal of the PyTorch Foundation is to foster an open source ecosystem for PyTorch, where the governance is distributed outside of Meta. By governance, this really means both the technical governance, which will be a meritocratic process where if you’ve shown and demonstrated outstanding technical ability and vision, and what PyTorch should look like, you can get nominated as a maintainer, even if you don’t work at Meta. The other aspect is the business governance. This includes things like the website, and all the various assets like the trademark, PyTorch. So far in the governing board, we’re really fortunate to have some of the largest and best companies in machine learning. Companies like AMD, Google, Amazon, Meta, of course, Microsoft, and NVIDIA. This is all going to be managed by the Linux Foundation. Personally, I’m very excited about this. This basically means that the project is growing up. It’s becoming too important to basically only belong to Meta.
Why PyTorch?
What’s up with PyTorch? Why is it that people like PyTorch? My two cents is that PyTorch is easy to use, learn, and debug. Generally, researchers really love it, because you can find lots of pretrained models that you can compose. There’s not too many abstractions, and it’s mostly Python, which means that you can dive into the internals and debug stuff. As your log statements, you can use PDB. That’s one of the benefits of what people call an eager mode framework, as opposed to a graph mode framework. Which also means you can freely intersperse Python and PyTorch code. You can have for loops. You can have if conditions. You can have print statements. For the most part, if you want a model that works, you’re mostly constrained by like, am I really building the right thing, and not really constrained by your tooling? As a researcher, this is great, which means you can wrestle with the foundations of the universe.
This is a dashboard, for example, from Papers With Code, which is a website that tracks the usage of framework per new papers. We can see that PyTorch is an extremely popular option among researchers. Again, this is really because of those three things that I highlighted. It’s easy to use, easy to learn, and it’s easy to debug. When you’re thinking about production, the design space becomes larger. While you also want now the code that was easy to learn, debug, you want it to be easy to maintain. You want it to be easy to accelerate. You want this thing to run fast, because if it’s running fast, it means you can run even more servers, you can make your models even better. It also needs to plug in into an existing infrastructure. Fundamentally, if you look at the research to production journey, it’s very different because the road to production isn’t like a point. It’s almost like a journey, and it’s long, and it’s perilous.
The reason that’s the case is because, one, there’s lots of options. There’s all sorts of tools you can use, like managed services, open source infrastructure versus something homegrown. If it’s homegrown, that’s good. That means you build something that solves a new problem. The problem is, if you’re successful, now this is something you need to maintain forever, in addition to the work you’ve already done. It’s not like you can just learn deep learning, now you have to learn operating systems, CUDA, networking, distributed programming, computer architecture, but also, your own domain. The tools are complex. They’re complex also because of a human imposed complexity. A lot of tools may not necessarily be available just if you put your credit card online. You need to talk to salespeople. You may need to prioritize asks via certain PMs. This is fundamentally why this problem is so complex, and why you can’t essentially have a single engineer to cover the entire research to production stack.
Why Open Source?
I gave this option of, let’s say you’re using something maybe that’s like a managed service, and you have a new feature request. It’s really hard to prioritize it, and you’re just waiting. What do you do? It feels like maybe you’re not some big-shot VP, you’re just some random person, but you’re pretty sure something is a problem so what do you do. Often, I think historically, this has been like, too bad, there’s not much you can do. One of the things I love about open source and why I think the internet is so amazing is because the users of a product are often more aware of its limitations than its maintainers. If you have something that’s a complaint, that’s a feature request, and it’s meritocratic. Because it’s like GitHub, you may not necessarily have your corporate credentials assigned to it. As long as you make a good argument, that means you can control the roadmap of a project, even if it’s backed by a large company. This also means that you control your own destiny, as in, you can add your own features, and you add your own abstractions on top in a fork. Or if they’re useful enough for the broader community, you can make a pull request and then you yourself control how people use this product. I think most importantly, whenever you’re dependent on a single project for your infrastructure, it means you’re also dependent on the company that produces this infrastructure. Companies come and go over time. One way of ensuring the long-term survival of a project is effectively open sourcing it, because then that project becomes in service of its community, and not just in service of its creators.
Good News: Getting Better at Research to Prod
There are some good news here. We are becoming better at converting people from research to production. You can go check out our PyTorch Medium, https://medium.com/pytorch/tagged/case-study. There’s a bunch of case studies there. Ones that I personally liked are things like AI for Agriculture. This was a case study done with Blue River Technology. Others are like using ground penetrating radar. Just radar through material, and look at potential flaws in its manufacturing, without breaking it. This is one thing that I personally never liked about roads, is that they’re not particularly debuggable, you need to break everything before you can look into it. Imagine you needed to do this for software. Another case study was on studying the properties of glass. You can imagine a molecule of glass. I’m not a chemist. Then, it’s a graph, and you want to analyze its properties. Again, this is telling of how expressive, in general, deep learning is. That, as long as you can express your problem as a tensor, from input tensors, to labels, which could also be output tensors, you can pretty much solve a large chunk of problems, whether it’s text, images, graph. In this case, the first are images, and the last ones are graphs. Odds are, you could probably try out using deep learning and maybe get pleasantly surprised.
Data Loading – PyTorch/Data
Let’s dive deep into the tooling. The first problem I want to cover is data loading. What is data loading? The simplest way to view the problem is that you have some data, maybe it’s in some cloud storage, or whatever, and you want to convert this to a tensor. Historically, this has meant that you need to first bring in that remote dataset locally, and now you need to convert it. Then you can do something like for element in batch, like model.fit, or like model.optimizer, model.step, whatever your favorite API is. One trend we’re noticing here is, large models in of themselves are not particularly useful. It’s really just that large models are also great at working with larger datasets. That’s the key. Where are those larger datasets located? They certainly won’t be on basically your local disk locally, instead they’ll be in remote object store. These are things like AWS, like Azure, or GCP, maybe it’s the Hugging Face Hub, maybe it’s NVIDIA AIStore, webdataset. Maybe it’s a TFRecord. Maybe it’s on Gdrive or Dropbox, if you’re doing some small experiments, or maybe just a local compressed file. Fundamentally, we should be able to work with all of those datasets, without requiring you to change your code. That’s exactly one of the things that torchdata was really built to address.
The other problem is, once you have this dataset, you may want to read it, decode it and do some preprocessing on it very quickly. The problem with this is generally this has meant that you do the conversions on CPU, and then you send over the data to GPU. As GPUs get faster, memory bandwidth becomes the bottleneck. Instead, there’s multiple technologies like NVIDIA’s DALI, or ffcv, that are working more on having direct GPU decoding, and accelerate preprocessing so you can directly send images to the GPU. If you do this, you’re basically going to have much higher GPU utilization, which, especially with newer generations of GPUs, is becoming the primary bottleneck for any model training.
Back to torchdata. What was the problem this library was really looking to solve? Historically, you may already know that we have DataLoaders in PyTorch. It was entirely possible for you to write something like for elem, and dataset, and start loading batches one at a time into your training pipeline. The problem was there was a complete lack of modularity and composition in this, so people were writing their own datasets. It wasn’t really obvious how to reuse different parts of this. We redesigned this from the ground up to be much more modular and composable. The main idea is that you want to define a DAG, like a directed acyclic graph, and execute it. Executing that graph essentially lets you do something like for element in graph. I have an example here. Let’s say you’re reading some data from S3, and then you’re reading some data from AIStore, you want to concatenate these two maybe text datasets, then you want to parse them. Then you want to filter out, for example, all the parts that may not necessarily have like, let’s say, English sentences. Then you want to load these in one at a time. This is something I just made up on the spot. There’s a lot more potential you could have in what those nodes are. Maybe the nodes are related to archiving, so maybe they could compress or decompress ZIP data, Gzip, Tar, whatever. They could be more combinatorial, as in, how do I take a dataset and combine it, or split it, or rotate through it cyclically? It could be grouping, like what we just discussed about below. Maybe it’s mapping, as in, you want to map a function over every element of a dataset, which is very useful for anything like preprocessing. Selecting, which is essentially like a filter. Or parsing, which is specific, really, like parsing for text, or decoding for images would be the same.
What’s really interesting about this is that, once you have a DAG like this, you can execute it. We already provide an execution for it. You can create your own. As in, you could have your own engine, and we call this a reading service. You could potentially maybe add support for like multi-threaded, or maybe you have some really custom infrastructure. We’ve effectively split responsibilities between how the preprocessing DAG is defined versus how it’s executed. This even lets us swap things in and out to make things easier. A core requirement for us even early on was that we wanted to make sure that this graph is serializable. As in, you can take this graph, and you can write it to disk. Then once you write it to disk, maybe you can modify it, so maybe someone can create a DAG, and then you come in and you can add more optimized versions of a node. Of course, you can create your own nodes. It’s actually one of the best ramp-up tasks on the library. For example, I personally created a Hugging Face Hub reader, and you can come in and create your own, or you can have somebody within your company and share them freely.
Fundamentally, I think, torchdata is focused on the scenario of streaming datasets. As in, the constraint is that the data does not fit on RAM, and it does not fit on the on-device memory for GPUs, because that’s not growing exponentially. To clarify this distinction in the documentation, we make the distinction between map-style datasets, which is a dataset that you can index into. For example, you can see dataset index. The benefit of this is that these datasets are really easy to shuffle. It’s what we’re here to do. They’re familiar. The con of them is that you need to load the entire dataset in memory, because you’re never really sure which index you’re really going to ask for, unless you’re willing to just pay for the screeds or cache misses. On the other hand, the future, and if you go through streaming only scenarios, you effectively can only look at the next element of a dataset. This is what the Iter really means. The benefit of this is clear. It’s that you only ever need to load the next few batches in memory. I’m really only saying the next few batches instead of the next batch, because you may actually want to do something like prefetching here to have it work efficiently.
Then the problem really becomes shuffling. How can you shuffle data if you can’t see it all? Thankfully, this is a problem that we’ve solved for you in torchdata. I want to make you curious about the library, https://github.com/pytorch/data. Make sure to go to the library, check out how we’ve solved this problem. Just to help you appreciate why this is maybe not an entirely obvious problem is, you may think, maybe I can shuffle elements as I see them. That doesn’t quite work, because then you’re going to be biased towards the elements that are ordered first in the dataset. Maybe you keep a buffer. Then, how big should this buffer be? You keep a buffer and then you fill this buffer, and then you shuffle the elements within that buffer. When the limit of the buffer is the size of the whole dataset, then you get map-style datasets, but then you get the cons of map-style datasets. We don’t want that. How small can we make it? Then, of course, there’s a wrench that gets thrown into all of this which is, ok, what if you’re running a distributed DataLoader, so now the dataset is put over multiple shards. Then you have a random seed that may decide how you’re reading datasets in one at a time. Then, do you share the random seed or not? Do you share the random seed generator? These are all hard problems. You can go check out the answer on the torchdata repo.
Distributed Training – PyTorch/PyTorch w/FSDP
The next thing I want to talk about is distributed training. Distributed training is hard. Let me set the definitions before I start talking about FSDP, or DDP, or anything like that. One form of parallelism is what we call data parallelism. Data parallelism is, you can fit a model on an individual GPU, but the dataset does not. What you do is you split the dataset over multiple devices, and you have the same model on all of those devices. Then you sync, and then you do a gradient step over that individual shard. The problem with this approach is that, since the shards were not seeing the same examples, they’re going to have different gradients. To resolve this problem, you need to synchronize the gradients occasionally, either synchronously or asynchronously, to get them to work. When it comes to large models, effectively, what we mean is model parallelism. Model parallelism is, let’s assume the data can fit on a single machine. The problem is, we can only fit an individual layer at a time. The problem should be somewhat clear. As in, let’s say model part 2 is really slow, but model part 1 is really fast. Then the bottleneck is going to be in model part 2. What does it mean? Should you just wait? No. Ideally, you can just pipeline examples. This is what we call pipeline parallelism. Of course, closely related to model parallelism is CPU offloading, which means, like let’s say you don’t have a GPU per layer, a lot of us don’t, what do you do? Maybe, then what you could do is you could offload extra weights on your CPU, and only load them in exactly when you need them. This way, you can take advantage of the high RAM which could be potentially much larger than the on-device GPU memory, but still also benefit from GPU’s acceleration, when you need it.
To combine all of these ideas, we have FullyShardedDataParallel, which basically lets you automatically just wrap a model. You can just FullyShardedDataParallel wrap model. Your model is just a typical nn module. There’s no magic here. This is exactly the same model you would write if it’s single layer. You can also enable CPU offloading to make sure that you avoid umphs. This is great, because now you can offload params, you can maybe potentially offload gradients. You can store the weights in various kinds of precision. Maybe you want to store them in 32-bit, maybe 16-bit, and take advantage of both data and model parallelism and pipeline parallelism and CPU offloading. This way, you don’t need to think about it.
Deploy Models – PyTorch/TorchX
Let’s say now you’ve defined the model, but you want to actually deploy it on some infrastructure. Where do you run Python large model.py? You may actually run it on Kubernetes on a slurm cluster. Whatever it is that you’re running, TorchX is an easy-to-use option for these things. Specifically, if you have your app.py script, deploying that model on various clusters, whether it’s a ray cluster, whether it’s a slurm cluster, whether it’s aws_batch, whether it’s Kubernetes, it’s really just a question of changing the scheduler parameter. That’s it. Once you do that, you can pretty much deploy wherever you want. Once you deploy it, there is a couple of verbs that are going to be [inaudible 00:24:18]. Maybe you want to set up this infrastructure, so you would run something like ray up or eksctl. Then you want to submit your job against that infrastructure. This is like running something like torchrun. Then, getting the status of that job, that’s a storage check status, so this is where you can know like, did the job fail? Did it succeed? Or maybe you want to get the logs for this job. All of these are just verbs. This makes it really easy for you to figure out, maybe you haven’t settled on an infrastructure yet, so you can try things out. You can try out Kubernetes. You can try out ray. You can try out slurm, and just see what makes the most sense to you. Once you’ve done this, we have built-in components to make it really easy for you to do distributed training or even elastic training. Which is, assume like someone trips on a cable while a job is running, instead of getting a [inaudible 00:25:08] deadlock error, you can just keep going. This is a lot of the benefits that TorchX brings.
Model Optimization
The other aspect is like, great, now you know how to train a model, and you know how to scale it, you know how to load data, and you know how to manage your model. Let’s say your model is just fundamentally too big, but this is the size that you know that you want, how do you make things run faster? Here, I’m going to take my time in explaining these things, because this is really an important idea. A lot of these pictures are borrowed from one of my colleagues, Horace, in his article called Brrr. It’s a really good article, so I’d recommend you check it out. I’m going to summarize it for you here. GPUs are now like memory bandwidth bound. What this means is, essentially, imagine you’re sending work to a factory. Then this factory sends you work, as in, tensors, and you want to run modeled off word. Then this factory is going to send you some output tensors back. Fundamentally, now the factories are becoming so fast that the bottleneck is becoming sending data quickly enough. The problem is actually closer to the right-hand side, which is, our factories keep getting bigger, and so we’re now not able to send enough data on the bus.
One way to solve this problem is what’s called fusions. Imagine, you have a model that looks something like this. It’s running a torch.randn, and then it’s running a cosine, and then another cosine. The way this typically works in eager mode PyTorch, is that you have the model, so you’re doing some operation, and then you write back a to disk. Then you read a from disk. Then you make an operation, and then you write back b to disk. Then same thing would be, you read it from disk, then you write it back. This is going to be extremely slow. We don’t ever really need b. Instead of doing this, we can fuse all of these operations and then do a single read/write for all of these operations into a single fused_op. Instead of thrashing back and forth like this, you can do fusions. The reason I bring this up is because fusions are critically important to understand whether you’re talking about like ONNX, like TensorRT, AITemplate. The majority of those operations take advantage of faster fusions.
There’s two kinds of important fusions I want you to be aware of. One important fusion is vertical fusion. This is what I showed you earlier here, which is, you have a sequential pipeline where you have lots of reads and writes. You can fuse those into a single read and a single write. This is vertical fusions. Another kind of fusion is called horizontal fusion. I know these are confusing terms. Horizontal fusion is something you already do with batch inference. The way batch inference works is you have a single model, but you’re running it on two different examples at once. Now imagine that you have a model that’s taking advantage of a single layer at multiple points, so imagine something with residual connections. You could essentially schedule those operations concurrently with horizontal fusions. Don’t worry about that example that I mention later, just think of batching. That is a form of horizontal fusion. Optimization providers typically benefit from those two operations.
The way you use those runtimes is usually fairly simple. They all look relatively the same, as in, it’s some sort of runtime_load operation that takes in the serialized weights on disk with some runtime_config. Then you make an inference on those things. There’s vertical fusion, which is fusing pointwise ops. Pointwise ops are things like cosine, but the more important one that you may care about is something like ReLU. Horizontal fusion is about parallel general matrix multiplications, or batching. Then memory fusions are if you have fusions that are doing either concatenations or splits. Even though, instead of, for example, allocating more memory to disk, you can just read them or view them. If you are interested in how to find these fusion opportunities automatically, make sure to check out projects like AITemplate, or if you want to see what it looks like to spend a lot of time and writing them by hand, there’s a lot of vendors that will write these fusions by hand in things like CUTLASS. They’ll be in C++, they’re harder to use. If you want something more Pythonic, make sure to check out AITemplate, https://github.com/facebookincubator/AITemplate.
Model Serving – PyTorch/Serve
The last thing I want to talk about is TorchServe. TorchServe is a solution for model inferencing. What is the model inferencing problem? Fundamentally, the model inferencing problem is you have some model weights and now you’re not in the real world, you’re not operating over tensors, you’re operating over binary images, text, and audio. You may want to decode and preprocess this data and then run model.forward. Even then, after you run model.forward, you get a tensor back. Like, who can read a tensor? You also need a post-processing pipeline that can either turn this tensor into a label, maybe it’s an image, if it’s something generative, or text, or audio if it’s something closer to the Stable Diffusion stuff. We have a whole bunch of case studies of people using TorchServe. You can check them out in our news page, https://github.com/pytorch/serve#-news.
I want to briefly talk about, how does a model inferencing framework work at a high level? At a high level, you’re going to be making curl requests, really. Those curl requests will say something like, I want you to make an inferencing here. Then, as the TorchServe instance gets more inference requests that it needs to handle, it’ll batch them, and then, basically treat them all at once. This is what’s called optional batching. It’s one of the important optimizations in TorchServe. Inferencing is one aspect of an inferencing framework. The other is the Management API, which lets you roll back to older versions of a model, do canary rollouts, so you can progressively do 10% rollouts with technologies like KServe, or autoscale a model if the scale gets really larger. Fundamentally, you have a TorchServe instance. This TorchServe instance can deal with multiple kinds of models concurrently. Those models are all in a worker pool. This worker pool will take in requests from a queue, one at a time, for inference. Or take in management requests from this queue as well to change their state. Their state will be written in snapshots, so you can roll back to older versions of a model. Metrics and logs so you can actually debug what was going on. Regardless of these internals, TorchServe is the default way to serve models on SageMaker. We also have partnerships with Docker, Google Vertex, KServe. You can also deploy it locally. The core engine right now is written in Java, but we’re moving towards having the core engine written in C++ for future releases to take advantage of multi-threaded inference. You can follow our progress here, and TorchServe specifically in the PyTorch C++ backend, where you can see the latest and greatest there for the C++ frontend.
Torch MultiPy
When you say parallelism in deep learning, there’s a lot of ideas here. Batching and vectorization are actually both forms of concurrency because you can take multiple examples and batch them all together at once. Or you can take one large example, and split it up into many subproblems. There’s actually another. Historically, in Python, multi-threading is not great because of the GIL. This is also fundamentally a constraint that PyTorch has. One way around it is that you can run multiple Python sub-interpreters at once. Then have those sub-interpreters share the same memory map PyTorch storage tensors under the hood. That way, you effectively get multi-threading in C++. This used to be called torch::deploy, now we call it like torch MultiPy. For now, it’s C++ only, but if you’d like Python bindings, let me know, because this is something I’ve been interested in working on myself. We want to make this easier to use. Effectively, you’ll have a whole bunch of tricks to make inference faster, whether it’s using fusions, whether it’s tuning your model inferencing framework, whether it’s using batching, whether it’s using vectorization. Now, multiple sub-interpreters will be the new kid on the block.
Questions and Answers
Luu: Maybe you can share a bit about the recent news about PyTorch.
Saroufim: There was a super exciting announcement that happened at the PyTorch Conference. We launched PyTorch 2.0. I know the term 2.0 scares people, they’re like, no, you’re going to break backwards compatibility. This is fully backwards compatible, actually. The reason we’re calling it 2.0 is because it’s just like a big change to how we use PyTorch. We still want you to write your eager mode code, so you just write your model.py, and it’s easy to use. Then you can also compile that code. Historically, there has been a tradeoff here where some frameworks are hard to program, but performant, and others are easy to program but they’re slow. Effectively with 2.0, we’ve removed this distinction. Basically, we want it to be easy to program and easy to compile on first. In particular, there’s one compiler that we’ve introduced called inductor that we’re very excited about. What that does is basically, it lets you write CUDA kernels in Python, and also have them be really performant. Inductor is essentially a code generator from Python to inductor. There’s a lot more. There’s a training compiler, which is very rare. You could introduce multiple backend compilers, whether it’s something like XLA, or TVM, or whether it’s TensorRT, or ONNX. I expect to see a lot more innovation in the compiler space now. It’s going to be a lot more possible for people to become compiler hackers, because it’s all for fun.
Luu: Are there any new features that users were not able to do before that now they can do?
Saroufim: The main one is going to be torch.compile, which is like, you basically take your model.py, and then you can compile it into a bunch of intermediate representations. Then take that intermediate representation and pass it into various compiler vendors with just a single flag. You can say torch.compile backend equals inductor equals TensorRT equals ONNX, and that’s it. It’s just you set a string, and then you can try out different compilers. Feature-wise, it’s not that you can do more things, it’s just that you can do the same thing more quickly. Now you can either run a bigger model, or run it for longer and do more iterations.
How do you think fastai library compressed to PyTorch? Is fastai supposed to be higher level and easier to use, and it works on top of PyTorch, and easier for beginners?
The main difference is that PyTorch in of itself doesn’t provide a training loop. What I mean by that is like, it has different facilities that make it easy for you to define your architecture. We try to stay relatively low level with how you’re supposed to use it. That’s why there’s a couple of other libraries that are a bit higher level, like fastai or PyTorch Lightning that will make it a lot more user friendly. The two projects somewhat have different design goals. From our perspective, we just want to make it easy for people to hack on even the low-level bits, whereas higher-level libraries may be more concerned with getting people to solving a business problem more quickly. If you’re a beginner, definitely go for the higher-level libraries. As you get more comfortable and you want more control, then I’d recommend you start diving into the lower-level stuff.
Is the PyTorch versus TensorFlow debate still relevant today? Which would you recommend for a new POC project? Do you see some unification or interop between the two in the future?
Maybe the bait is like Pepsi versus Coke. It’s really whatever you prefer. I can’t comment too much on the tradeoff. One thing that PyTorch did really well was because we focused so much on debuggability, and that you can add print statements to your code, I think it ended up becoming a very researcher friendly framework. Then the flip side of that is, it’s easy to hack, but because it’s in Python, it’s slow. There’s the Python overhead. You can’t do optimizations that cover the whole model, because it’s just executing a line of Python, one line at a time. That’s really why we feel like the Torch compiler will be such a powerful feature. In terms of interop, it’s not like the libraries will interop directly. I think that may be a bit tricky. However, I’ve definitely seen people convert a PyTorch model back to TensorFlow and vice versa using intermediate representations like ONNX, depending on what your infrastructure leverages more. Yes, they do interop, but not directly.
Are there plans to support more GPU types in PyTorch? I know NVIDIA was the initial one, and now there’s support for Apple Silicon. What about AMD GPUs?
AMD GPUs are actually already supported, granted, in torch.compile, because this uses our new compiler called inductor. Inductor generates Python kernels, which is only as far as I know today using NVIDIA. The support in NVIDIA tends to be broader just because the library has been around for longer. It’s one of those things where the performance speaks for itself. I would expect over time to have more hardware vendors and also the performance for those hardware vendors to get better. You should benchmark and see what works for you.
I think one of the interesting things is, historically, it’s been difficult to support a new backend for PyTorch, if you’re a new hardware vendor. Because you need to support all the PyTorch operations, and there’s about 2000 of them. One thing we did, and this was inspired by jax.lax and a bunch of other compilers, where we decompose all of those operations to a smaller subset. I think last I did was like around 250. That way, if the larger subset is a composition of the smaller ones, you only as a hardware vendor only need to implement the smaller ones to get full coverage for PyTorch, as opposed to getting things like unsupported op errors, which are a frustrating user experience for people. Again, I feel like a lot of the infrastructure that was built for 2.0 will make it a lot easier for people to support new backends and new hardware architectures more easily.
Luu: Extensibility, it sounds like, or flexibility.
Saroufim: Yes, and hackability. No one knows how to read C++ anymore. I certainly don’t.
See more presentations with transcripts
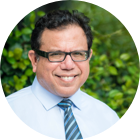
MMS • RSS
Posted on nosqlgooglealerts. Visit nosqlgooglealerts
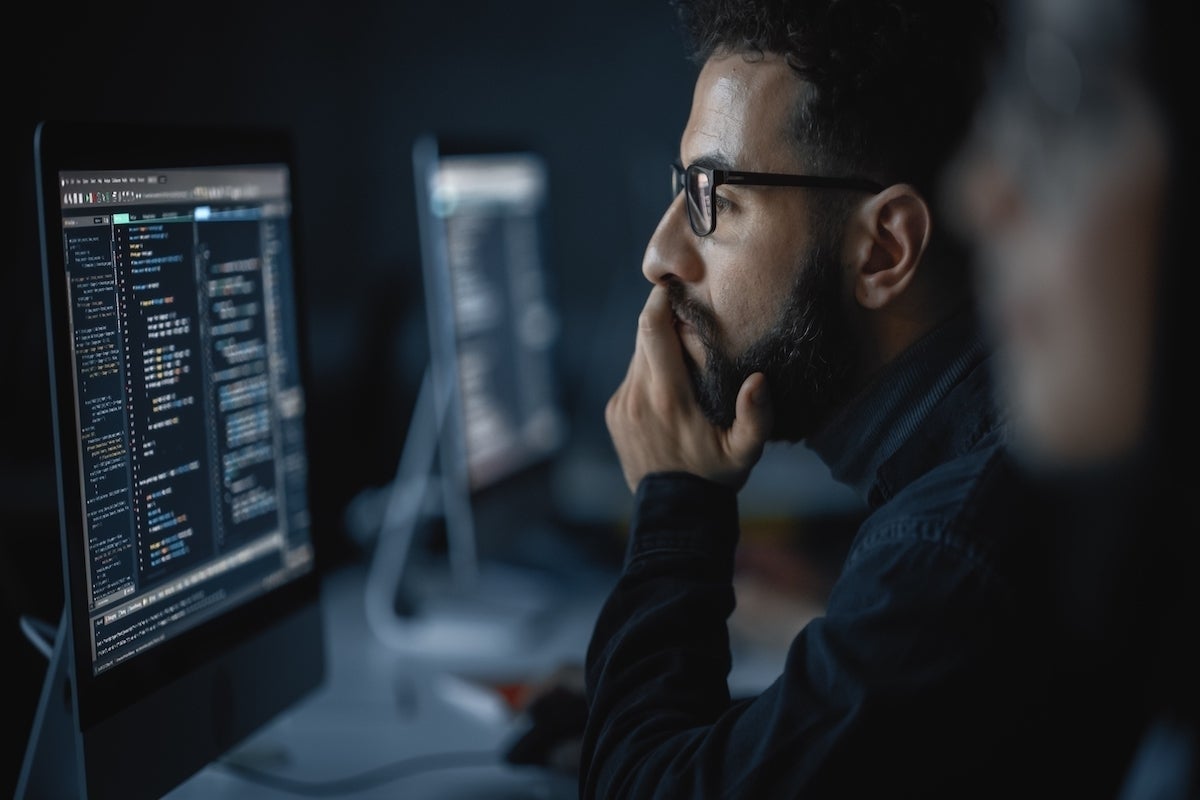
DataStax on Tuesday said that it was releasing a new JSON API in order to help JavaScript developers leverage its serverless, NoSQL Astra DB as a vector database for their large language model (LLMs), AI assistant, and real-time generative AI projects.
Vector search, or vectorization, especially in the wake of generative AI proliferation, is seen as a key capability by database vendors as it can reduce the time required to train AI models by cutting down the need to structure data — a practice prevalent with current search technologies. In contrast, vector searches can read the required or necessary property attribute of a data point that is being queried.
The addition of the new JSON API will eliminate the need for developers trained in JavaScript to have a deep understanding of Cassandra Query Language (CQL) in order to work with Astra DB as the database is based on Apache Cassandra, the company said.
This means that these developers can continue to write code in the language that they are familiar with, thereby reducing the time required to develop AI-based applications which are in demand presently, it added.
Further, the new API, which can be accessed via DataStax’s open source API gateway, dubbed Stargate, will also provide compatibility with Mongoose — one of the most popular open source object data modeling library for MongoDB.
In October last year, DataStax launched the second version of its open-source data API gateway, dubbed Stargate V2, just months after making its managed Astra Streaming service generally available.
In June this year, the company partnered with Google to bring vector search to Astra DB.
Next read this:
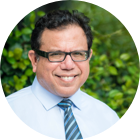
MMS • Anthony Alford
Article originally posted on InfoQ. Visit InfoQ
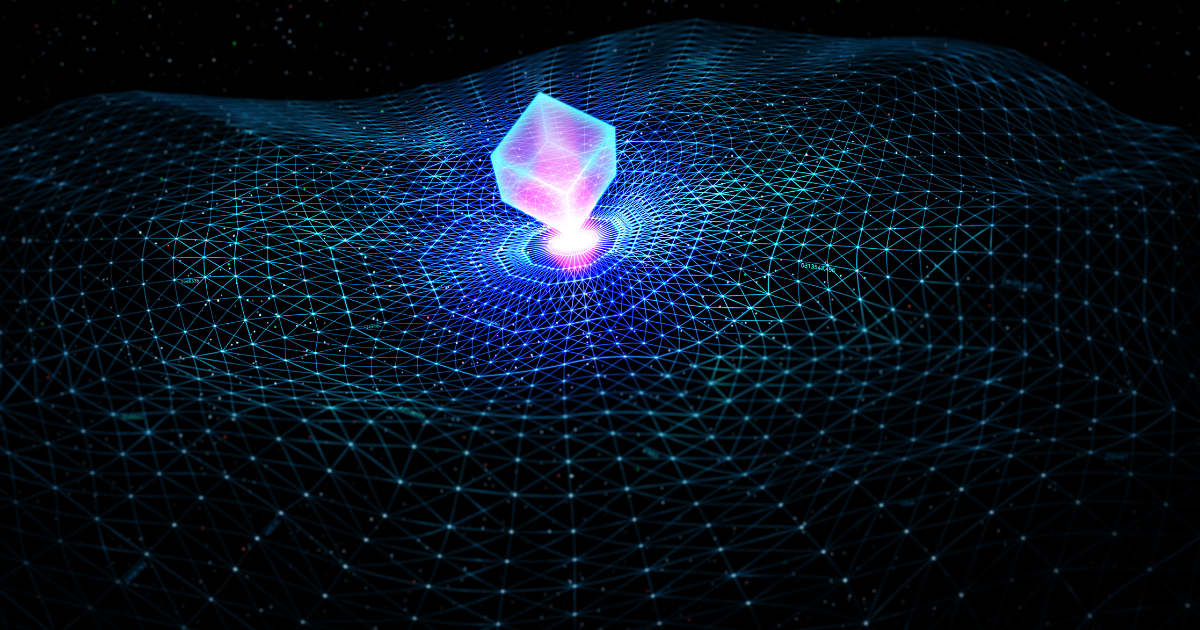
Meta recently open-sourced Massively Multilingual & Multimodal Machine Translation (SeamlessM4T), a multilingual translation AI that can translate both speech audio and text data across nearly 100 languages. SeamlessM4T is trained on 1 million hours of audio data and outperforms the current state-of-the-art speech-to-text translation model.
SeamlessM4T is a multimodal model that can handle both text and audio data as input and output, allowing it to perform automated speech recognition (ASR), text-to-text translation (T2TT), speech-to-text translation (S2TT), text-to-speech translation (T2ST), and speech-to-speech translation (S2ST). The model is released under the non-commercial CC BY-NC 4.0 license. Meta is also releasing their training dataset, SeamlessAlign, which contains 270,000 hours of audio data with corresponding text transcription, as well as their code for mining the data from the internet. According to Meta,
We believe the work we’re announcing today is a significant step forward….Our single model provides on-demand translations that enable people who speak different languages to communicate more effectively. We significantly improve performance for the low and mid-resource languages we support. These are languages that have smaller digital linguistic footprints….This is only the latest step in our ongoing effort to build AI-powered technology that helps connect people across languages. In the future, we want to explore how this foundational model can enable new communication capabilities—ultimately bringing us closer to a world where everyone can be understood.
Meta’s motivation for their research is to build a universal translation system like the Babelfish from The Hitchhiker’s Guide to the Galaxy sci-fi stories. InfoQ has covered several of their previous efforts, including their T2TT model No Language Left Behind (NLLB) which can translate text between 200 languages, and their Massively Multilingual Speech (MMS) model which supports ASR and text-to-speech synthesis (TTS) in over 1,100 languages. InfoQ also covered other work in the area, such as OpenAI’s Whisper which can transcribe and translate speech audio from 97 different languages, Google’s Universal Speech Model (USM) that supports ASR in over 100 languages, and Google’s AudioPaLM, which was the previous state-of-the-art model for S2ST.
SeamlessM4T is based on the UnitY neural network architecture, which consists of a pipeline of three components. First is an encoder that can handle both speech audio and text data input and recognizes the input’s meaning; the audio sub-component is based on w2v-BERT and the text on NLLB. Next is a decoder, also based on NLLB, which converts that meaning into a text output in a target language. Finally, there is a text-to-acoustic unit decoder to convert the target text into speech.
Meta compared their model’s performance to both cascaded approaches, which consist of a pipeline of discrete ASR, T2TT, and TTS models, and to single-model systems. The systems were evaluated on the FLEURS and CVSS benchmarks. On FLEURS, SeamlessM4T “sets a new standard for translations into multiple target languages,” outperforming AudioPaLM by 20%. SeamlessM4T also outperformed cascaded models; on CVSS it was “stronger by 58%.”
Several users discussed SeamlessM4T on Hacker News. One user shared tips on how to get the model to run locally, and pointed out that it had a context limit of 4096 tokens. Another user asked:
Will there be a whispercpp equivalent? Half the reason I love whisper is how dead simple it is to get running. I will take somewhat lower accuracy for easier operation.
The SeamlessM4T code and models are available on GitHub. There is an interactive translation demo available on Huggingface.
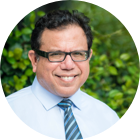
MMS • Edin Kapic
Article originally posted on InfoQ. Visit InfoQ
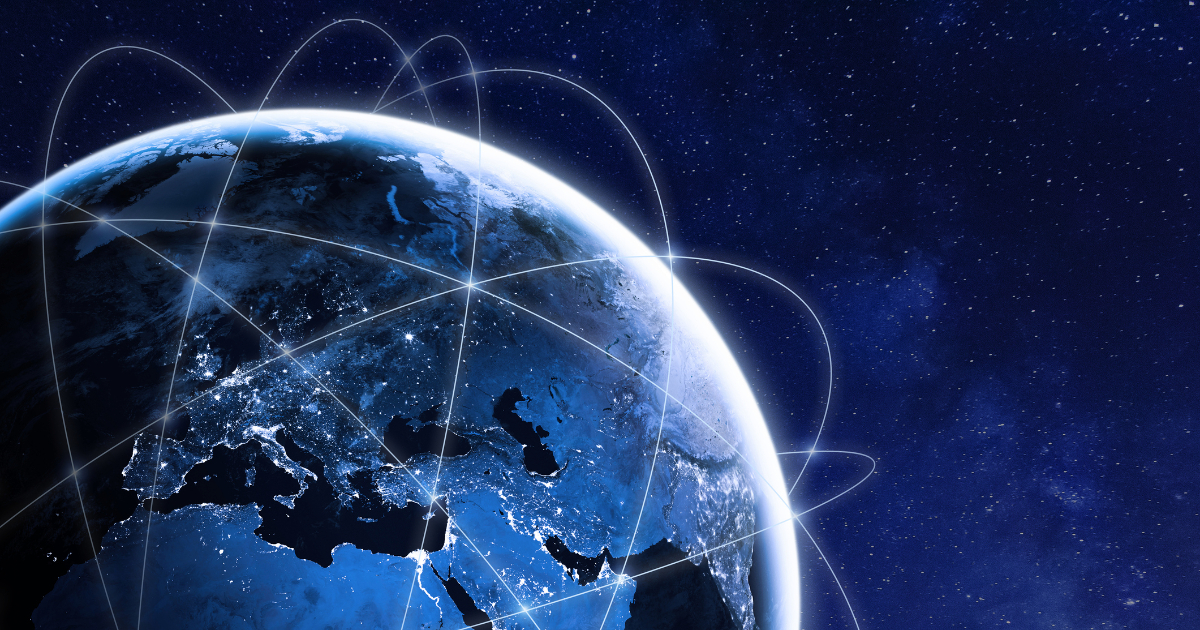
Microsoft recently released version 1.18 of SharePoint Framework with several updates, focused on Viva Connections’ components feature updates but also bringing updates for some long-standing dependencies.
On September 12th, 2023, Microsoft released the new SharePoint Framework (SPFx) 1.18 version, five months after the last minor version 1.17. It is worth noticing that the previous version already saw four patch updates: 1.17.1 to 1.17.4, covering specific errors in library packaging. Patch updates are rarely released for SharePoint Framework, but since version 1.17 Microsoft announced that they were going to release smaller patches more frequently.
Version 1.18 of SPFx is mainly focused on feature updates for Microsoft Viva Connections components. The Viva Connections’ components in SPFx are delivered in the form of Adaptive Card Extensions (ACEs), UI components that leverage Microsoft’s own adaptive cards’ visual language. While adaptive cards are broader in scope and present in several Microsoft products such as Outlook, Teams or SharePoint, adaptive card extensions are a component of Viva Connections.
When support for ACEs was introduced in SharePoint Framework 1.13, the adaptive card extension visualisation in the UI, called card view in SPFx, was restricted. Developers could choose between three fixed templates: BaseBasicCardView
, BaseImageCardView
and BasePrimaryTextCardView
.
In version 1.18, the view for a custom ACE extends a new base class called BaseComponentsCardView
. This class requires developers to override the cardViewParameters getter property to provide the specific components for the card view, namely the card image, the card bar, the header, the body and the footer. To simplify the code and to help with the codebase migration, the old base classes are now available as helpers called BasicCardView
, ImageCardView
and PrimaryTextCardView
. There is a tutorial in the SPFx documentation about migrating the custom ACEs from earlier SPFx versions to version 1.18 and a new UI guidance on how to design useful adaptive cards.
Earlier ACE components allowed only the interaction of clicking a button on the card. In version 1.18, the ACE components can contain a text input control, which is not yet fully supported on the mobile Viva Connections app. The text input control raises an onChange
event which can be intercepted and acted upon.
There is a specific search card template for those ACEs which leverage search capabilities. The search card has a search box, which acts as a text input control mentioned above. The updated documentation contains a tutorial on how to build a people search solution with ACEs.
Another improvement in the interactivity of custom ACEs is the support for Adaptive Cards Execute action. This action allows the developers to send a collection of all the card properties and input values to a specified API endpoint.
Besides all the feature updates for Viva Connections adaptive card extensions, version 1.18 updates some of the base dependency libraries for SPFx developers. Until now, SPFx didn’t support Node 18, leaving developers in need of managing multiple Node versions, as one user on X (formerly Twitter) mentions. SPFx version 1.18 supports Node 18, TypeScript 4.7 and Microsoft’s Fluent UI React library v8. The full release notes of the changes in version 1.18 are available on the GitHub release page.
SharePoint Framework (or SPFx) is the set of tools and libraries to build extensibility options in Microsoft 365. With SharePoint Framework developers can create solutions for Microsoft SharePoint, Microsoft Teams and Microsoft Viva products. The SPFx uses industry-standard tooling such as Yeoman, TypeScript, Lint, WebPack and other client-side libraries. The issues list is hosted on GitHub and currently has 578 open issues.
Cloudflare One Data Protection Suite for Data Security Across Web, Private, and SaaS Applications
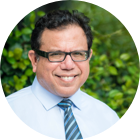
MMS • Steef-Jan Wiggers
Article originally posted on InfoQ. Visit InfoQ
Cloudflare recently announced its One Data Protection Suite, a unified set of advanced security solutions designed to protect data across every environment – web, private, and SaaS applications. The company states the suite is powered by Cloudflare’s Security Service Edge (SSE), allowing customers to streamline compliance in the cloud, mitigate data exposure and loss of source code, and secure developer and AI environments from a single platform.
The Data Protection Suite is a converged set of capabilities across Cloudflare products, such as Data Loss Prevention (DLP), Cloud access security brokers (CASB), Zero Trust network access (ZTNA), secure web gateway (SWG), remote browser isolation (RBI), and cloud email security services onto a single platform. The company packaged it and made it part of Cloudflare One.
Overview of Cloudflare One Data Protection (Source: Cloudflare One Data Protection)
With Cloudflare One Data Protection Suite, the company aims to provide organizations with simple, extendable, comprehensive data controls for all aspects of their internal and external applications. Built natively on Cloudflare’s global network, this suite allows organizations to:
- Rapidly incorporate new capabilities and adopt new security standards and protocols to ensure data protection controls effectively cater to modern use cases, such as safeguarding generative AI code.
- Leverage one management interface, providing administrators with multiple, flexible options to send traffic to Cloudflare for enforcement — including API-based scans, clientless deployments of ZTNA and RBI, a single device client, direct or virtual interconnects, and SD-WAN partnerships.
- Use data protection controls through one single-pass inspection across Cloudflare’s network locations.
- Leverage Data Loss Prevention exact data match with the flexibility to detect organization-specific data defined in custom datasets.
- Discover sensitive data at rest and in line. Integrations will cover most cloud collaboration, productivity, and enterprise code repository tools.
- Control access to data and apps based on behavioral user risk scores, incorporating signals from across Cloudflare One, such as user activities, posture, and settings.
Comparable products or services to Cloudflare One Data Protection are, for instance, Microsoft Defender (D365 and Cloud) or Google Cloud Security, which are more specific to their cloud platforms.
In a Cloudflare press release, Tanner Randolph, chief information security officer at Applied Systems, a SaaS technology provider for insurance brokers, said:
Today, Cloudflare One helps prevent our users from sharing sensitive data and code with tools like ChatGPT and Bard, enabling us to take advantage of AI safely. Over the past few years, Cloudflare has been a critical partner in our digital transformation efforts and has helped us consolidate security controls across our users, applications, and networks. Going forward, we are excited about Cloudflare’s continued innovations to protect data and, in particular, their vision and roadmap for services like DLP and CASB.
When asked by InfoQ what is driving the investment from Cloudflare, here is what James Chang, from Cloudflare One, had to say:
Data continues to explode across more environments than security teams can keep track of. This steady trend, accelerated by recent shifts towards hybrid work, DevOps, and generative AI tools, has made it harder than ever to safeguard sensitive data. Organizations need a cybersecurity strategy and partner that adapts to these escalating risks and makes it easier to protect data without slowing down business. Cloudflare One’s data protection suite fills this exact gap by unifying visibility and controls for data everywhere across web, SaaS, and private applications.
In addition, he said:
It is built on and delivered across our own programmable network, enabling us to build new capabilities quickly and address the evolving risks presented by modern coding and increased usage of AI.
Lastly, more details are available in the documentation pages and technical demo.
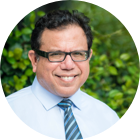
MMS • RSS
Posted on mongodb google news. Visit mongodb google news
MongoDB, Inc. (NASDAQ:MDB – Get Free Report) CFO Michael Lawrence Gordon sold 5,000 shares of the company’s stock in a transaction on Thursday, September 14th. The shares were sold at an average price of $370.82, for a total value of $1,854,100.00. Following the completion of the transaction, the chief financial officer now directly owns 101,509 shares in the company, valued at $37,641,567.38. The transaction was disclosed in a filing with the SEC, which is available through the SEC website.
Michael Lawrence Gordon also recently made the following trade(s):
- On Monday, July 3rd, Michael Lawrence Gordon sold 2,197 shares of MongoDB stock. The stock was sold at an average price of $406.79, for a total transaction of $893,717.63.
MongoDB Stock Down 1.5 %
NASDAQ MDB opened at $356.54 on Tuesday. The business has a fifty day moving average price of $385.06 and a 200 day moving average price of $317.99. MongoDB, Inc. has a one year low of $135.15 and a one year high of $439.00. The company has a debt-to-equity ratio of 1.29, a quick ratio of 4.48 and a current ratio of 4.48. The company has a market cap of $25.44 billion, a P/E ratio of -103.05 and a beta of 1.11.
Wall Street Analyst Weigh In
Several brokerages have recently issued reports on MDB. KeyCorp boosted their price objective on MongoDB from $372.00 to $462.00 and gave the company an “overweight” rating in a report on Friday, July 21st. 22nd Century Group reissued a “maintains” rating on shares of MongoDB in a report on Monday, June 26th. Argus boosted their price objective on MongoDB from $435.00 to $484.00 and gave the company a “buy” rating in a report on Tuesday, September 5th. JMP Securities increased their price target on shares of MongoDB from $425.00 to $440.00 and gave the stock a “market outperform” rating in a report on Friday, September 1st. Finally, Tigress Financial increased their price target on shares of MongoDB from $365.00 to $490.00 in a report on Wednesday, June 28th. One research analyst has rated the stock with a sell rating, three have given a hold rating and twenty-one have given a buy rating to the company’s stock. According to MarketBeat.com, MongoDB has a consensus rating of “Moderate Buy” and an average target price of $418.08.
Get Our Latest Stock Analysis on MongoDB
Institutional Inflows and Outflows
Several hedge funds have recently added to or reduced their stakes in MDB. GPS Wealth Strategies Group LLC purchased a new position in MongoDB in the 2nd quarter worth approximately $26,000. KB Financial Partners LLC purchased a new position in MongoDB in the 2nd quarter worth approximately $27,000. Capital Advisors Ltd. LLC lifted its stake in MongoDB by 131.0% in the 2nd quarter. Capital Advisors Ltd. LLC now owns 67 shares of the company’s stock worth $28,000 after purchasing an additional 38 shares in the last quarter. Parkside Financial Bank & Trust lifted its stake in MongoDB by 176.5% in the 2nd quarter. Parkside Financial Bank & Trust now owns 94 shares of the company’s stock worth $39,000 after purchasing an additional 60 shares in the last quarter. Finally, Coppell Advisory Solutions LLC purchased a new position in MongoDB in the 2nd quarter worth approximately $43,000. 88.89% of the stock is owned by institutional investors and hedge funds.
About MongoDB
MongoDB, Inc provides general purpose database platform worldwide. The company offers MongoDB Atlas, a hosted multi-cloud database-as-a-service solution; MongoDB Enterprise Advanced, a commercial database server for enterprise customers to run in the cloud, on-premise, or in a hybrid environment; and Community Server, a free-to-download version of its database, which includes the functionality that developers need to get started with MongoDB.
See Also
Receive News & Ratings for MongoDB Daily – Enter your email address below to receive a concise daily summary of the latest news and analysts’ ratings for MongoDB and related companies with MarketBeat.com’s FREE daily email newsletter.
Article originally posted on mongodb google news. Visit mongodb google news
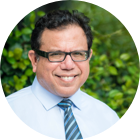
MMS • Matt Campbell
Article originally posted on InfoQ. Visit InfoQ
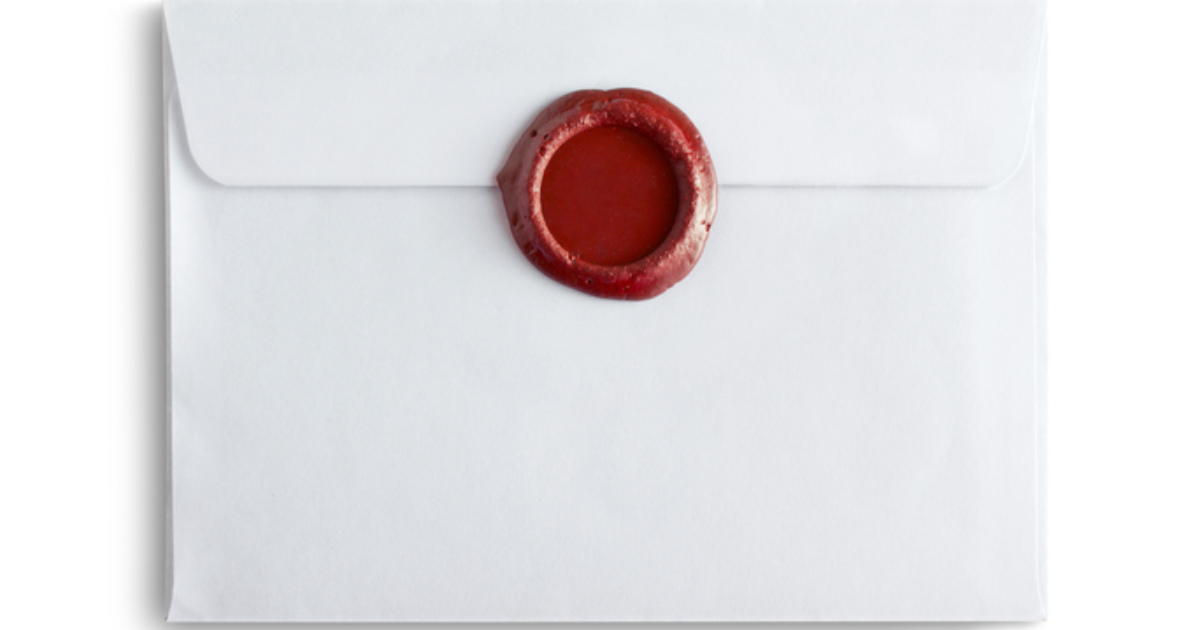
HashiCorp has moved the HashiCorp Vault Secrets Operator for Kubernetes into general availability. This Kubernetes Operator combines Vault’s secret management tooling with the Kubernetes Secrets cache. The operator also handles secret rotation and has controllers for the various secret-specific custom resources.
The Vault Secrets Operators watches for changes to its set of Custom Resource Definitions (CRDs). Each CRD allows for the operator to synchronize a Vault Secret to a Kubernetes Secret. This operator writes the source Vault secret directly to the destination Kubernetes secret. This ensures that all changes made to the source are properly replications. uses Kubernetes custom resources to manage secrets used by services. Applications are able to consume the secrets in a Kubernetes-native manner from the destination.
The operator supports all of the supported Secret customer resources (CRs) including VaultConnection
, VaultAuth
, VaultDynamicSecret
, and VaultPKISecret
. This example shows leveraging the VaultConnection
CR:
apiVersion: secrets.hashicorp.com/v1beta1
kind: VaultConnection
metadata:
namespace: vso-example
name: example
spec:
# address to the Vault server.
address: http://vault.vault.svc.cluster.local:8200
In this example, the VaultDynamicSecret
CR is used with the database secret engine. The following example creates a request to http://127.0.0.1:8200/v1/db/creds/my-postgresql-role
to create a new credential:
---
apiVersion: secrets.hashicorp.com/v1beta1
kind: VaultDynamicSecret
metadata:
namespace: vso-example
name: example
spec:
vaultAuthRef: example
mount: db
path: creds/my-postgresql-role
destination:
create: true
name: dynamic1
A number of new features were included in the GA release including the addition of a number of new authentication methods. Previously, only the Kubernetes authentication method was available. With the GA release, there is now support for JSON Web Token (JWT), AppRole, and AWS as authentication methods.
The GA release also includes validations for common Kubernetes cloud services such as AKS, GKE, EKS, and OpenShift. Another new enhancement has the Vault Secrets Operator attempt to revoke any cached Vault client tokens when the deployment is deleted.
All Vault secrets engines are supported as is TLS/mTLS communication with Vault. Secret rotation is supported for Deployment
, ReplicaSet
, and StatefulSet
Kubernetes resource types. Rotating a static secret can be done using:
vault kv put kvv2/webapp/config username="static-user2" password="static-password2"
Deployment of the operator is supported via a Helm chart and through Kustomize. Once deployed, the operator does require a number of permissions. These include permissions on the Secret
, ServiceAccount
, and Deployment
objects. Access can be restricted as needed to objects within specified namespaces.
Secrets stored directly in Kubernetes, using the Secrets API, are stored unencrypted in the API server’s underlying data store. The Kubernetes documentation recommends setting up encryption at rest, enabling RBAC rules, limiting access to the secrets to only the necessary containers, or using an external Secret store provider. The Secrets Store CSI Driver, in addition to supporting Vault, also supports AWS, Azure, and GCP providers.
The beta release was first introduced in the HashiCorp Vault 1.13 release with the GA release aligning with the Vault 1.14 release. More details for the release can be found on the HashiCorp blog or in the GitHub repository.
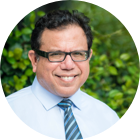
MMS • RSS
Posted on mongodb google news. Visit mongodb google news
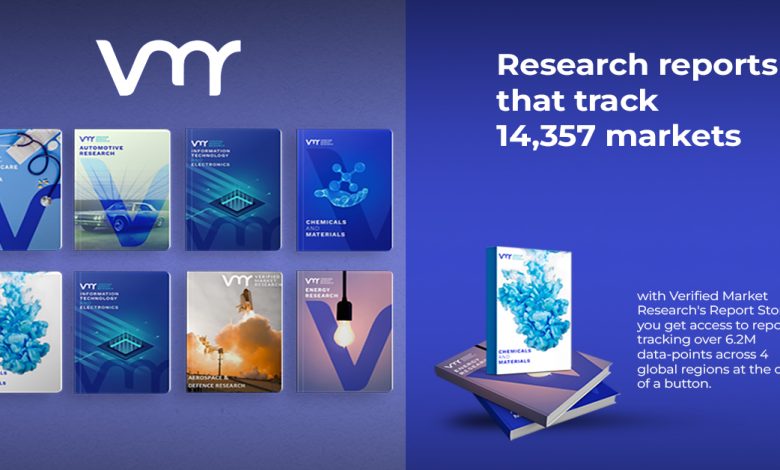
New Jersey, United States – The Global Database Software Market Report is a comprehensive and informative analysis of the industry, designed to provide valuable insights to organizations and stakeholders for making well-informed decisions. The report examines important market trends, growth drivers, challenges, and opportunities. It commences with a detailed examination of the market, defining its scope and segmentation. The study explores the market’s distinctive features, including factors that contribute to its growth, current obstacles, and potential prospects, thereby enabling businesses to anticipate market developments and gain a competitive advantage by comprehending present and upcoming trends.
The report thoroughly analyzes various geographic regions, namely North America, Europe, the Asia-Pacific region, South America, the Middle East, and Africa, in its regional section. It assesses market trends, key players, and growth prospects in each region. Furthermore, the research takes into account economic conditions, governmental policies, and consumer preferences that influence market growth in these regions. The regional analysis offers a comprehensive outlook on the Global Database Software market, empowering companies to tailor their strategies according to specific regional characteristics.
Database Software Market size was valued at USD 142.91 Billion in 2020 and is projected to reach USD 182.95 Billion by 2028, growing at a CAGR of 3.15% from 2021 to 2028.
Get Full PDF Sample Copy of Report: (Including Full TOC, List of Tables & Figures, Chart) @ https://www.verifiedmarketresearch.com/download-sample/?rid=85913
Leading 10 Companies in the Global Database Software Market Research Report:
Teradata, MongoDB, Mark Logic, Couch base, SQLite, Datastax, InterSystems, MariaDB, Science Soft, AI Software.
The growth of the Global Database Software market is significantly shaped by key vendors. The report highlights their market share, product portfolio, strategic objectives, and financial performance, underscoring their importance. Notably, important suppliers are acknowledged for fostering innovation, investing in research and development, and forming strategic partnerships with other businesses to enhance their market position. The competitive landscape is thoroughly evaluated, shedding light on major vendors’ tactics to gain a competitive edge. Businesses aiming to enter or strengthen their position in the global Global Database Software market must fully comprehend the roles played by these significant providers.
The Global Database Software Market Report is recommended for several reasons. Firstly, it offers a detailed examination of the market, considering critical factors such as market size, growth drivers, challenges, and opportunities. This research provides insightful information that aids organizations in formulating effective action plans and making informed decisions. Additionally, the study presents a comprehensive competitive landscape, allowing customers to benchmark their performance against major competitors and identify potential alliances. The report’s geographical analysis helps businesses grasp market dynamics in different regions, enabling them to adapt their strategies accordingly. For companies seeking to understand and thrive in the Global Database Software industry, this report proves to be an invaluable resource.
Global Database Software Market Segmentation:
Database Software Market, By Type of Product
• Database Maintenance Management
• Database Operation Management
Database Software Market, By End User
• BFSI
• IT & Telecom
• Media & Entertainment
• Healthcare
Inquire for a Discount on this Premium Report @ https://www.verifiedmarketresearch.com/ask-for-discount/?rid=85913
What to Expect in Our Report?
(1) A complete section of the Global Database Software market report is dedicated for market dynamics, which include influence factors, market drivers, challenges, opportunities, and trends.
(2) Another broad section of the research study is reserved for regional analysis of the Global Database Software market where important regions and countries are assessed for their growth potential, consumption, market share, and other vital factors indicating their market growth.
(3) Players can use the competitive analysis provided in the report to build new strategies or fine-tune their existing ones to rise above market challenges and increase their share of the Global Database Software market.
(4) The report also discusses competitive situation and trends and sheds light on company expansions and merger and acquisition taking place in the Global Database Software market. Moreover, it brings to light the market concentration rate and market shares of top three and five players.
(5) Readers are provided with findings and conclusion of the research study provided in the Global Database Software Market report.
Key Questions Answered in the Report:
(1) What are the growth opportunities for the new entrants in the Global Database Software industry?
(2) Who are the leading players functioning in the Global Database Software marketplace?
(3) What are the key strategies participants are likely to adopt to increase their share in the Global Database Software industry?
(4) What is the competitive situation in the Global Database Software market?
(5) What are the emerging trends that may influence the Global Database Software market growth?
(6) Which product type segment will exhibit high CAGR in future?
(7) Which application segment will grab a handsome share in the Global Database Software industry?
(8) Which region is lucrative for the manufacturers?
For More Information or Query or Customization Before Buying, Visit @ https://www.verifiedmarketresearch.com/product/database-software-market/
About Us: Verified Market Research®
Verified Market Research® is a leading Global Research and Consulting firm that has been providing advanced analytical research solutions, custom consulting and in-depth data analysis for 10+ years to individuals and companies alike that are looking for accurate, reliable and up to date research data and technical consulting. We offer insights into strategic and growth analyses, Data necessary to achieve corporate goals and help make critical revenue decisions.
Our research studies help our clients make superior data-driven decisions, understand market forecast, capitalize on future opportunities and optimize efficiency by working as their partner to deliver accurate and valuable information. The industries we cover span over a large spectrum including Technology, Chemicals, Manufacturing, Energy, Food and Beverages, Automotive, Robotics, Packaging, Construction, Mining & Gas. Etc.
We, at Verified Market Research, assist in understanding holistic market indicating factors and most current and future market trends. Our analysts, with their high expertise in data gathering and governance, utilize industry techniques to collate and examine data at all stages. They are trained to combine modern data collection techniques, superior research methodology, subject expertise and years of collective experience to produce informative and accurate research.
Having serviced over 5000+ clients, we have provided reliable market research services to more than 100 Global Fortune 500 companies such as Amazon, Dell, IBM, Shell, Exxon Mobil, General Electric, Siemens, Microsoft, Sony and Hitachi. We have co-consulted with some of the world’s leading consulting firms like McKinsey & Company, Boston Consulting Group, Bain and Company for custom research and consulting projects for businesses worldwide.
Contact us:
Mr. Edwyne Fernandes
Verified Market Research®
US: +1 (650)-781-4080
US Toll-Free: +1 (800)-782-1768
Email: sales@verifiedmarketresearch.com
Website:- https://www.verifiedmarketresearch.com/
Article originally posted on mongodb google news. Visit mongodb google news