Month: September 2023
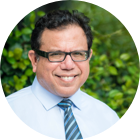
MMS • RSS
Posted on mongodb google news. Visit mongodb google news
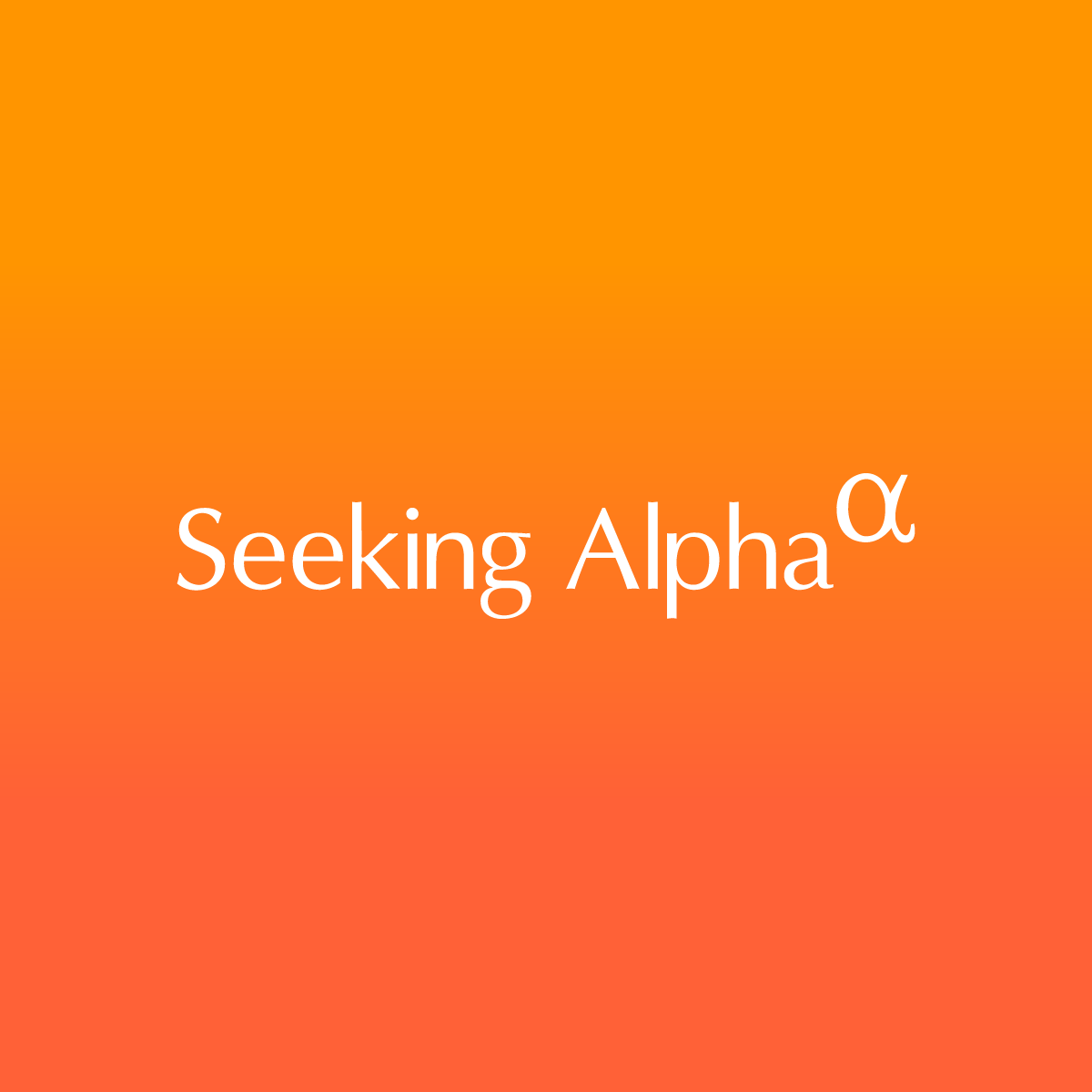
To ensure this doesn’t happen in the future, please enable Javascript and cookies in your browser.
If you have an ad-blocker enabled you may be blocked from proceeding. Please disable your ad-blocker and refresh.
Article originally posted on mongodb google news. Visit mongodb google news
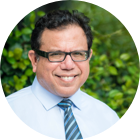
MMS • RSS
Posted on mongodb google news. Visit mongodb google news
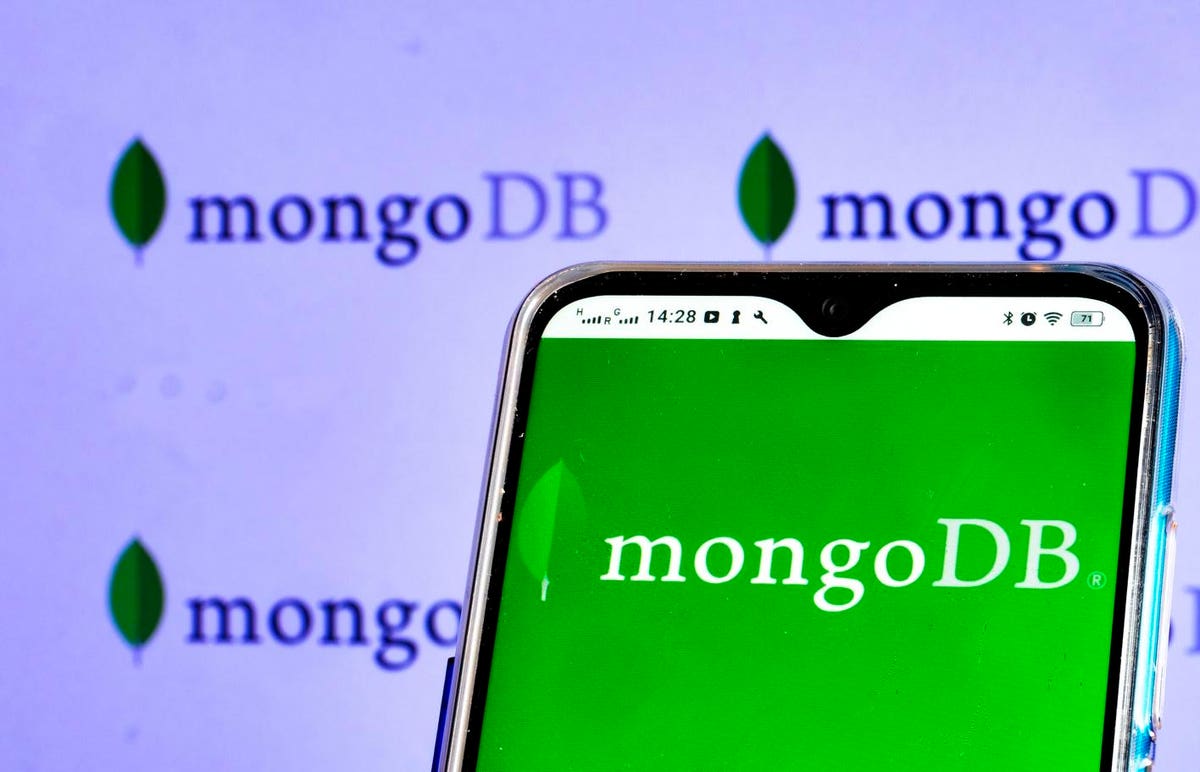
Shares of MongoDB (MDB) have been awarded an AI premium.
Bullishness about the company’s long-term AI opportunity has provided an added tailwind for the stock, which hit a 52-week high of $439 in July. Recently trading around $394, the shares have doubled YTD.
There’s a trend emerging in which more customers are selecting the company’s Atlas cloud database as a key platform to both build and run new AI applications, according to MongoDB CEO Dev Ittycheria.
He thinks the shift to AI will favor modern platforms that offer a rich set of capabilities. Ittycheria also believes many existing applications will be re-platformed to be AI-enabled, arguing that this is a compelling reason for customers to migrate to MongoDB from legacy technologies.
At the MongoDB developer conference held this summer, the company announced several new products along with advanced features for its Atlas cloud platform that make it faster and easier for customers to build modern applications for any workload or use case. Included among the new features and products were Atlas Vector Search (for highly relevant information retrieval) and Atlas Stream Processing (for high-velocity streams of complex data).
Atlas Vector Search simplifies bringing generative AI and semantic search into various applications to deliver more engaging end-user experiences, while Atlas Stream Processing transforms the building of event-driven applications that respond in real-time by unifying how developer teams work with data in motion and data at rest. With Atlas Stream Processing, customers have a single interface to easily extract insights from high-volume streaming data.
One of MongoDB’s major goals is to bring in new workloads from existing customers. The company recently announced the general availability of Relational Migrator, a tool that simplifies application migration and transformation from legacy relational databases to the company’s document-based model. The Migrator tool enables customers to quickly, easily and cost-effectively move off of older database technology, improving operational efficiency.
At the developer conference, Sahir Azam, chief product officer at MongoDB, said legacy relational databases remain the main competition. But he noted that they were optimized for a time when hardware was very expensive. Today, scalable hardware is quite cheap, but hiring developers and making them productive is the challenge. The document model makes it “very natural” for developers to build, iterate and improve applications over time, Azam said.
He explained that rows and tables in relational databases are not how developers think. Instead, developers see things such as managing a sale or managing products in a catalog as objects. Thus, the rise of object-oriented programming over the past 20 years. Azam said MongoDB gives developers a natural way to map object-oriented programming directly into the data model. With MongoDB, developers have a flexible database that is easy to scale effectively.
As more organizations see that MongoDB offers many of the mission-critical features offered by traditional relational databases, demand should continue to grow for the company’s document-based database. Demand will come in the form of new workloads as well as the shifting of workloads over from relational offerings. The Migrator tool helps users create a document-oriented schema within MongoDB, transfer data out and even rewrite associated code.
In fiscal Q2 (ended July), MongoDB’s total revenue rose 40% to $423.8 million, well above the consensus estimate of $393.3 million. It’s impressive that top-line growth reaccelerated from 29% in the previous quarter. Atlas cloud revenue (representing 63% of total revenue) advanced 38%.
MongoDB in FQ2 added 1,900 new customers, including a number of AI accounts (with smaller workloads that will expand over time). The company now has more than 45,000 total customers, up 22% year over year.
For FQ3 (Oct.), MongoDB’s total revenue outlook of $400 million to $404 million came in above the consensus of $389.1 million.
Piper Sandler called out the FQ2 revenue acceleration in raising its MongoDB price target to $425 from $400, noting that the upside was aided by large, multi-year licensing deals and renewal expansions. Needham raised its target to $445 from $430. The firm views MongoDB as a key beneficiary of generative AI, especially as organizations continue to iterate and discover new use cases.
RBC Capital believes MongoDB has the ability to become a major developer data platform by consolidating more workloads. The firm thinks MongoDB is well-positioned to be a long-term beneficiary of the generative AI platform shift.
Goldman Sachs is becoming increasingly constructive on MongoDB’s ability to sustainably land new customers and add workloads that can ultimately translate into $6 billion in revenue longer-term. MongoDB is enriching its platform with new functionality to meet the requirements of the “next big paradigm shift” of generative AI, according to the firm. Goldman sees MongoDB as a big beneficiary of accelerated app development.
Article originally posted on mongodb google news. Visit mongodb google news
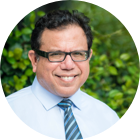
MMS • RSS
Posted on nosqlgooglealerts. Visit nosqlgooglealerts
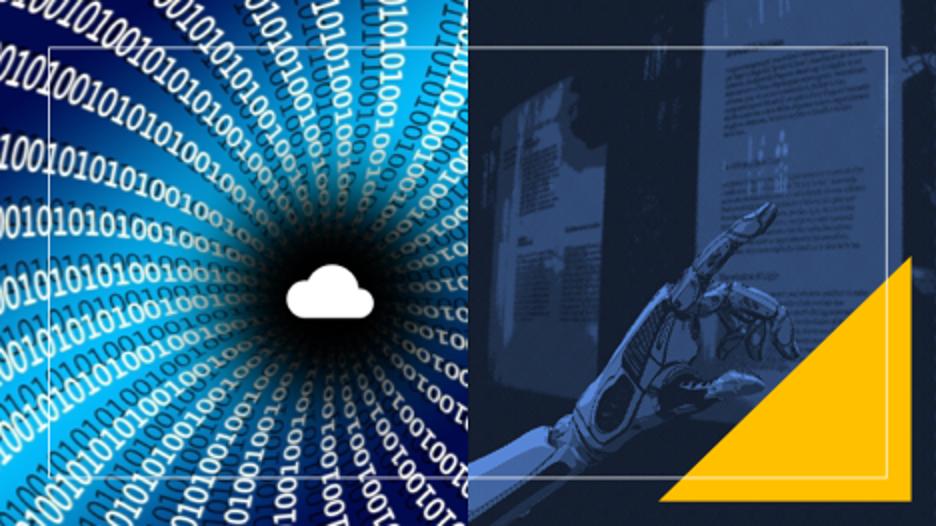
Cloud database provider Couchbase has launched two strategic initiatives aimed at improving developer productivity while fostering greater collaboration in the fast-growing field of AI-driven application development.
AI-assisted coding with Capella iQ
The first prong of this dual initiative is Capella iQ, a feature set that enriches Couchbase’s Capella database-as-a-service (DBaaS) platform. What sets Capella iQ apart is its incorporation of generative AI technologies that exploit natural language processing to automatically create sample code, data sets and even unit tests. By doing this, the tool is streamlining the development process substantially, thereby enabling developers to focus more on high-level tasks rather than the nitty-gritty of code writing. Not just that, Capella iQ brings additional utilities to the table by aiding in SQL++ writing and generating essential syntax indexes, among other programming tasks. Scott Anderson, senior vice president of product management and business operations at Couchbase, underscores that these advancements aim to “fuel innovation at a faster pace for customers.”
The goal of Couchbase’s initiatives is to maximize the value of data in Capella by integrating generative AI and positioning it alongside developer tools such as Vercel (a front-end platform aiding developers to build with speed and reliability) and IntelliJ (an integrated development environment [IDE] for JVM languages aimed to enhance productivity). The recent updates to Capella focus on improving operational efficiency, cloud database security and the developer experience as a whole. Key features include integration with popular developer platforms, enhanced scaling capabilities and reinforced security measures such as PCI DSS 4.0 and Cloud Security Alliance STAR Level 2 compliance. This complements the SOC 2 Type II and HIPAA compliance that Couchbase Capella has already achieved.
Enabling partners with AI
Couchbase’s second initiative is its AI Accelerate Partner Program. This new program is part of the company’s broader PartnerEngage effort and is explicitly designed to engage a diverse range of organizational types and partners. The overarching objective is to catalyze the development and deployment of AI-centric applications while fortifying the broader ecosystem around Couchbase’s suite of products.
The AI Accelerate Partner Program offers numerous benefits for companies aiming to develop AI-driven applications using Capella. This initiative provides partners with essential resources to support their journey, including early access to beta releases of Couchbase AI and insights into the company’s product roadmap. They can also attend AI-focused workshops led by Couchbase experts and benefit from a streamlined integration process for Capella, often complemented by extended trial periods. Couchbase’s PartnerEngage portal offers many educational resources, including self-paced online training. Furthermore, members are given opportunities for joint marketing endeavors, potentially including being featured on Couchbase’s website.
The benefits of the program are evident for enterprises contemplating the adoption of Couchbase’s tools and services. Generative AI in particular promises to reduce development cycles dramatically. This could translate into considerable cost savings and operational efficiencies. However, it’s essential to proceed cautiously; integrating AI capabilities into existing systems demands a meticulously thought-out strategy.
Database competitive landscape
Let’s look at Couchbase’s competitors and consider the key differences among them, because each database brings distinct advantages to the table.
Database-as-a-service competitive landscape
Robert Kramer
In the realm of databases, Couchbase stands out with its comprehensive features tailored for organizations emphasizing scalability, managing significant data volumes and requiring a flexible schema. MongoDB is particularly favored by businesses needing a dynamic schema combined with potent real-time data management. Redis positions itself as the go-to for businesses with high-speed transactional needs. For those aiming at user-friendliness paired with scalability, Amazon DynamoDB, an AWS offering, brings reliability to the table. Microsoft Azure Cosmos DB appeals to businesses eyeing a globally accessible database thanks to its universal accessibility. For entities deeply embedded in the Oracle ecosystem, the Oracle NoSQL Database offers seamless integration, making it a predictable pick. Cassandra is a recognized choice when there’s a need to manage vast amounts of data in a distributed environment; it claims unmatched fault tolerance in distributed settings. Meanwhile, Neo4j, a graph database, is increasingly used by businesses aiming to efficiently store, analyze and query complex interrelated data. Each database, from Couchbase to Neo4j, offers distinct features. Given these nuances, the final choice hinges on specific business prerequisites, underscoring the importance of thorough research and comparison.
Let’s summarize
Couchbase’s recent initiatives signal a drive towards creating a modernized, AI-powered development ecosystem. With emerging technologies, it’s prudent for potential adopters to evaluate any tool based on its speed, cost-effectiveness and functionality. The key takeaway in this case is that while generative AI is making rapid strides, it also requires careful integration into existing systems and processes because of its nascent state.
That said, Couchbase is delivering real results. The company recently released its financial results for the second quarter of fiscal 2024, reporting revenue of $43 million, of which $41 million stemmed from subscriptions. Another highlight is the company’s 24% growth in annual run rate (ARR), which is now pegged at $180.7 million. In a fiercely competitive sector, Couchbase is sustaining its growth as it continues to implement new features and capabilities.
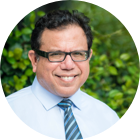
MMS • RSS
Posted on nosqlgooglealerts. Visit nosqlgooglealerts
Couchbase has announced that its Capella managed service NoSQL database will from now on include “generative AI capabilities”
Couchbase is a distributed NoSQL cloud database that combines the properties of a distributed document database (JSON) with features of a traditional DBMS including distributed SQL, transactions, and ACID guarantees.
Capella was launched at the end of 2021 with support for AWS, GCP and Azure. The Couchbase team says the new features will enhance developer productivity and accelerate time to market for modern applications. The new feature, called Capella iQ, can be used by developers to write SQL++ and application-level code more quickly through the use of AI to create recommended sample code.
Capella iQ is an AI cloud service that developers can interact with using natural language conversations. It can suggest common prompts, and it knows Couchbase-specific context such as database, collection, and index definitions. iQ is powered by ChatGPT, and will soon let the developer pick the LLM.
The Couchbase team says that the use of JSON by Capella iQ
provides an ideal format for storing both data and metadata designed to feed prompts, that JSON can also store conversation context from session to session, and JSON arrays can hold vector embedding data.
Alongside the introduction of Capella iQ, Couchbase has added several other improvements, starting with the ability to use other IDEs including Vercel and the IntelliJ family of IDEs.
Performance scaling is another improvement, so that Capella can now dynamically scale disk storage and input/output operations per second (IOPS). Security has also been improved, and Capella and Couchbase have now achieved independent validation for PCI DSS 4.0 and CSA STAR Level 2 compliance. Capella has also added the ability to download backup files to S3 or other locations.
Capella iQ is available as a private preview now.
More Information
Related Articles
Couchbase 7 Adds Relational Support Model
Insights From Couchbase Connect
To be informed about new articles on I Programmer, sign up for our weekly newsletter, subscribe to the RSS feed and follow us on Twitter, Facebook or Linkedin.
Summer SALE Kindle 9.99 Paperback $10 off!!
Comments
or email your comment to: comments@i-programmer.info
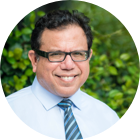
MMS • RSS
Posted on nosqlgooglealerts. Visit nosqlgooglealerts
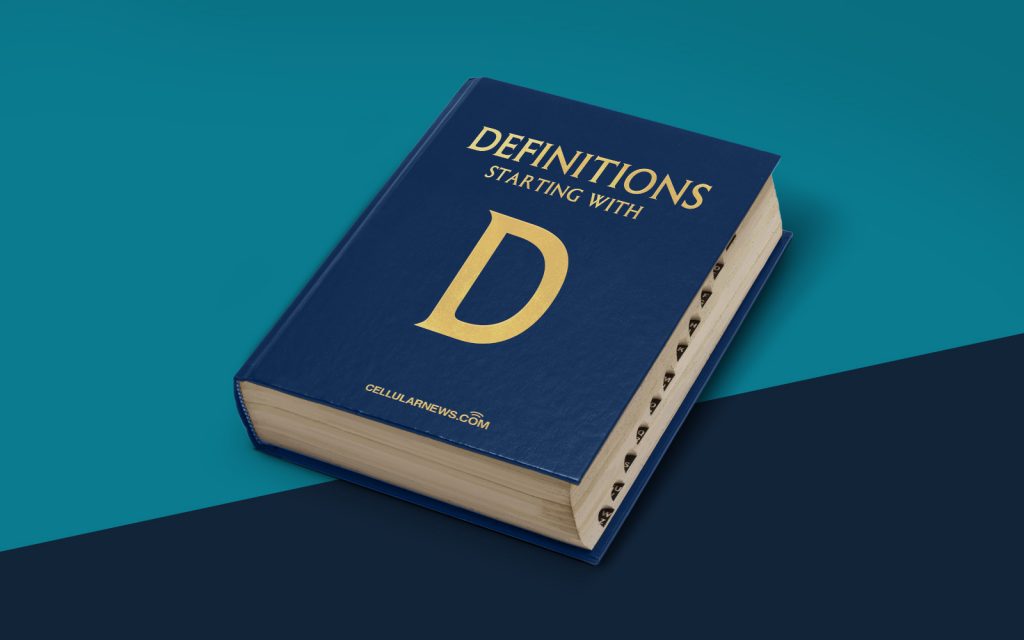
–>
<!–
–>
What is a Database Model? – A Definitive Guide
Have you ever wondered how data is organized in a computer system? Or how companies handle massive amounts of information efficiently? Look no further! In this blog post, we’ll dive into the world of database models and demystify their importance in managing and organizing data.
Key Takeaways:
- A database model is a conceptual representation that defines the structure, relationships, and constraints of a database.
- It acts as a blueprint to guide the design and implementation of a database.
Let’s start by answering a fundamental question – what is a database model? In simple terms, a database model is a way of structuring and organizing data in a computer system. Just like a well-designed filing cabinet with labeled folders and categorized documents, a database model serves as a framework for storing, managing, and retrieving information efficiently.
Database models provide a structured approach to represent the relationships between various data elements within a database. They define how the data is stored, organized, and accessed by users or applications. By using a database model, businesses can streamline their data management processes, improve data integrity, and enhance decision-making capabilities.
Now, let’s explore two popular types of database models:
1. Relational Database Model:
The relational database model is the most widely used and recognized model in the industry. It organizes data into tables, where each table represents a specific entity or concept. These tables are then linked together through relationships, such as primary keys and foreign keys, which ensure data consistency and integrity.
With a relational database model, businesses can perform complex queries, retrieve data based on specific criteria, and analyze information efficiently. Popular database management systems that follow the relational model include MySQL, Oracle, and Microsoft SQL Server.
2. NoSQL Database Model:
–>
As the name suggests, the NoSQL database model departs from the traditional tabular structure of the relational model. It offers a more flexible and scalable approach to store and retrieve data, particularly in modern web applications with large volumes of data and high transaction rates.
NoSQL databases use a variety of data models, such as key-value stores, document stores, columnar databases, and graph databases, allowing developers to choose the most suitable model for their specific use case. Some popular NoSQL databases include MongoDB, Cassandra, and Redis.
In conclusion, a database model is a fundamental building block for managing data in computer systems. It provides a framework for organizing and structuring data, enabling businesses to efficiently store, retrieve, and analyze information. Whether you opt for a relational database model or a NoSQL model, it’s crucial to choose the right model that aligns with your business requirements and scalability needs. So, the next time you hear about database models, you’ll know that they are the secret sauce behind the organized and structured world of data management.
Key Takeaways:
- A database model is a conceptual representation that defines the structure, relationships, and constraints of a database.
- It acts as a blueprint to guide the design and implementation of a database.
–>
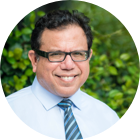
MMS • Lukas Krecan
Article originally posted on InfoQ. Visit InfoQ
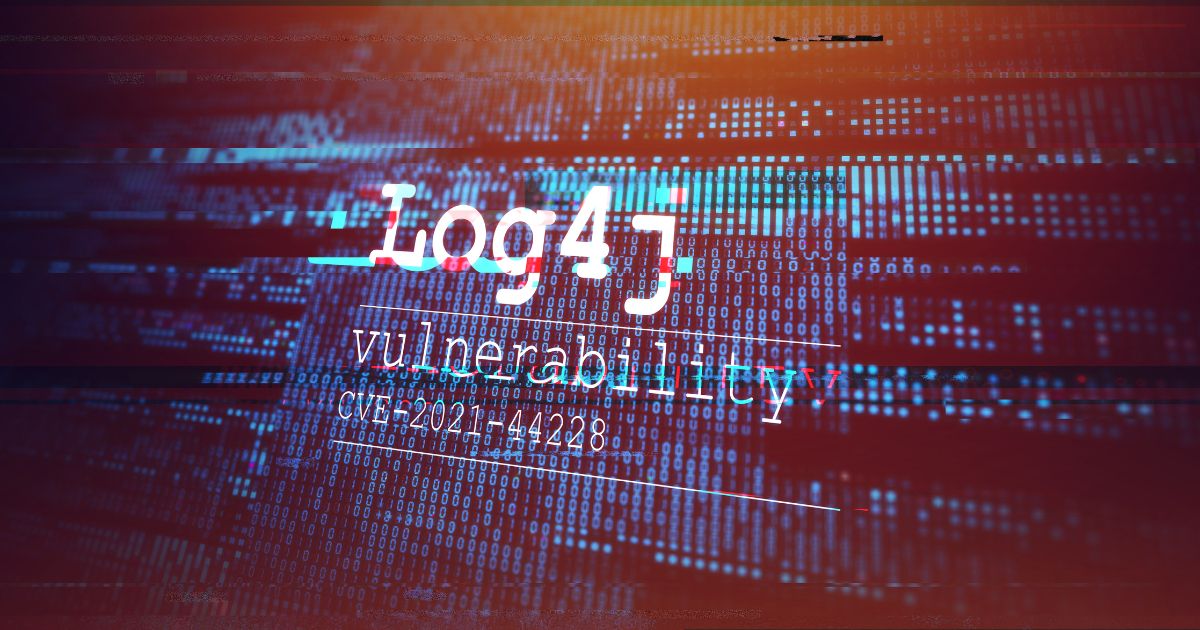
Key Takeaways
- Including a dependency vulnerability check (Software Composition Analysis or SCA) as part of a continuous integration or continuous delivery pipeline is important to maintain an effective security posture.
- The same vulnerability can be critical in one application and harmless in another. Humans should be “kept in the loop” here, and only the developers maintaining the application make an effective decision.
- It is essential to prevent vulnerability alert fatigue. We should not get used to the fact that the dependency check is failing. If we do, critical vulnerability may pass unnoticed.
- It is crucial to quickly upgrade vulnerable dependencies or suppress false positives even if we are maintaining dozens of services.
- Developers should invest in tools that help with discovery, detection, analysis and resolution of vulnerabilities. Examples include OWASP dependency check, GitHub Dependabot, Checkmarx, Snyk and Dependency Shield.
Modern Java applications are built on top of countless open-source libraries. The libraries encapsulate common, repetitive code and allow application programmers to focus on delivering customer value. But the libraries come with a price – security vulnerabilities. A security issue in a popular library enables malicious actors to attack a wide range of targets cheaply.
Therefore, it’s crucial to have dependency vulnerability checks (a.k.a. Software Composition Analysis or SCA) as part of the CI pipeline. Unfortunately, the security world is not black and white; one vulnerability can be totally harmless in one application and a critical issue in another, so the scans always need human oversight to determine whether a report is a false positive.
This article will explore examples of vulnerabilities commonly found in standard Spring Boot projects over the last few years. This article is written from the perspective of software engineers. The focus will shift to the challenges faced when utilizing widely available tools such as the OWASP dependency check.
As software engineers are dedicated to delivering product value, they view security as one of their many responsibilities. Despite its importance, security can sometimes get in the way and be neglected because of the complexity of other tasks.
Vulnerability resolution lifecycle
A typical vulnerability lifecycle looks like this:
Discovery
A security researcher usually discovers the vulnerability. It gets reported to the impacted OSS project and, through a chain of various non-profit organizations, ends up in the NIST National Vulnerability Database (NVD). For instance, the Spring4Shell vulnerability was logged in this manner.
Detection
When a vulnerability is reported, it is necessary to detect that the application contains the vulnerable dependency. Fortunately, a plethora of tools are available that can assist with the detection.
One of the popular solutions is the OWASP dependency check – it can be used as a Gradle or Maven plugin. When executed, it compares all your application dependencies with the NIST NVD database and Sonatype OSS index. It allows you to suppress warnings and generate reports and is easy to integrate into the CI pipeline. The main downside is that it sometimes produces false positives as the NIST NVD database does not provide the data in an ideal format. Moreover, the first run takes ages as it downloads the whole vulnerability database.
Various free and commercial tools are available, such as GitHub Dependabot, Checkmarx, and Snyk. Generally, these tools function similarly, scanning all dependencies and comparing them against a database of known vulnerabilities. Commercial providers often invest in maintaining a more accurate database. As a result, commercial tools may provide fewer false positives or even negatives.
Analysis
After a vulnerability is detected, a developer must analyze the impact. As you will see in the examples below, this is often the most challenging part. The individual performing the analysis must understand the vulnerability report, the application code, and the deployment environment to see if the vulnerability can be exploited. Typically, this falls to the application programmers as they are the only ones who have all the necessary context.
Resolution
The vulnerability has to be resolved.
- Ideally, this is achieved by upgrading the vulnerable dependency to a fixed version.
- If no fix is released yet, the application programmer may apply a workaround, such as changing a configuration, filtering an input, etc.
- More often than not, the vulnerability report is a false positive. Usually, the vulnerability can’t be exploited in a given environment. In such cases, the report has to be suppressed to prevent becoming accustomed to failing vulnerability reports.
Once the analysis is done, the resolution is usually straightforward but can be time-consuming, especially if there are dozens of services to patch. It’s important to simplify the resolution process as much as possible. Since this is often tedious manual work, automating it to the greatest extent possible is advisable. Tools like Dependabot or Renovate can help in this regard to some extent.
Vulnerability examples
Let’s examine some vulnerability examples and the issues that can be encountered when resolving them.
Spring4Shell (CVE-2022-22965, score 9.8)
Let’s start with a serious vulnerability – Spring Framework RCE via Data Binding on JDK 9+, a.k.a. Spring4Shell, which allows an attacker to remotely execute code just by calling HTTP endpoints.
Detection
It was easy to detect this vulnerability. Spring is quite a prominent framework; the vulnerability was present in most of its versions, and it was discussed all over the internet. Naturally, all the detection tools were able to detect it.
Analysis
In the early announcement of the vulnerability, it was stated that only applications using Spring WebMvc/Webflux deployed as WAR to a servlet container are affected. In theory, deployment with an embedded servlet container should be safe. Unfortunately, the announcement lacked the vulnerability details, making it difficult to confirm whether this was indeed the case. However, this vulnerability was highly serious, so it should have been mitigated promptly.
Resolution
The fix was released in a matter of hours, so the best way was to wait for the fix and upgrade. Tools like Dependabot or Renovate can help to do that in all your services.
If there was a desire to resolve the vulnerability sooner, a workaround was available. But it meant applying an obscure configuration without a clear understanding of what it did. The decision to manually apply it across all services or wait for the fix could have been a challenging one to make.
HttpInvoker RCE (CVE-2016-1000027, score 9.8)
Let’s continue to focus on Spring for a moment. This vulnerability has the same criticality as Spring4Shell 9.8. But one might notice the date is 2016 and wonder why it hasn’t been fixed yet or why it lacks a fancy name. The reason lies in its location within the HttpInvoker component, used for the RPC communication style. It was popular in the 2000s but is seldom used nowadays. To make it even more confusing, the vulnerability was published in 2020, four years after it was initially reported due to some administrative reasons.
Detection
This issue was reported by OWASP dependency check and other tools. As it did not affect many, it did not make the headlines.
Analysis
Reading the NIST CVE detail doesn’t reveal much:
Pivotal Spring Framework through 5.3.16 suffers from a potential remote code execution (RCE) issue if used for Java deserialization of untrusted data. Depending on how the library is implemented within a product, this issue may or [may] not occur, and authentication may be required.
This sounds pretty serious, prompting immediate attention and a search through the link to find more details. However, the concern turns out to be a false alarm, as it only applies if HttpInvokerServiceExporter
is used.
Resolution
No fixed version of a library was released, as Pivotal did not consider it a bug. It was a feature of an obsolete code that was supposed to be used only for internal communication. The whole functionality was dropped altogether in Spring 6, a few years later.
The only action that to take is to suppress the warning. Using the free OWASP dependency check, this process can be quite time-consuming if it has to be done manually for each service.
There are several ways to simplify the flow. One is to expose and use a shared suppression file in all your projects by specifying its URL. Lastly, you can employ a simple service like Dependency Shield to streamline the whole suppression flow. The important point is that a process is needed to simplify the suppression, as most of the reports received are likely false positives.
SnakeYAML RCE (CVE-2022-1471, score 9.8)
Another critical vulnerability has emerged, this time in the SnakeYAML parsing library. Once again, it involves remote code execution, with a score of 9.8. However, it was only applicable if the SnakeYAML Constructor
class had been used to parse a YAML provided by an attacker.
Detection
It was detected by vulnerability scanning tools. SnakeYAML is used by Spring to parse YAML configuration files, so it’s quite widespread.
Analysis
Is the application parsing YAML that could be provided by an attacker, for example, on a REST API? Is the unsafe Constructor
class being used? If so, the system is vulnerable. The system is safe if it is simply used to parse Spring configuration files. An individual who understands the code and its usage must make the decision. The situation could either be critical, requiring immediate attention and correction, or it could be safe and therefore ignored.
Resolution
The issue was quickly fixed. What made it tricky was that SnakeYAML was not a direct dependency; it’s introduced transitively by Spring, which made it harder to upgrade. If you want to upgrade SnakeYAML, you may do it in several ways.
- If using the Spring Boot dependency management plugin with Spring Boot BOM,
- a. the
snakeyaml.version
variable can be overridden. - b. the dependency management declaration can be overridden.
- a. the
- If not using dependency management, SnakeYAML must be added as a direct dependency to the project, and the version must be overridden.
When combined with complex multi-project builds, it’s almost impossible for tools to upgrade the version automatically. Both Dependabot and Renovate are not able to do that. Even a commercial tool like Snyk is failing with “could not apply the upgrade, dependency is managed externally.”
And, of course, once the version is overridden, it is essential to remember to remove the override once the new version is updated in Spring. In our case, it’s better to temporarily suppress the warning until the new version is used in Spring.
Misidentified Avro vulnerability
Vulnerability CVE-2021-43045 is a bug in .NET versions of the Avro library, so it’s unlikely to affect a Java project. How, then, is it reported? Unfortunately, the NIST report contains cpe:2.3:a:apache:avro:*:*:*:*:*:*:*:*
identifier. No wonder the tools mistakenly identify org.apache.avro/avro@1.9.0
as vulnerable, even though it’s from a completely different ecosystem.
Resolution: Suppress
Summary
Let’s look back at the different stages of the vulnerability resolution and how to streamline it as much as possible so the reports do not block the engineers for too long.
Detection
The most important part of detection is to avoid getting used to failing dependency checks. Ideally, the build should fail if there is a vulnerable dependency detected. To be able to enable that, the resolution needs to be as painless and as fast as possible. No one wants to encounter a broken pipeline due to a false positive.
Since the OWASP dependency check primarily uses the NIST NVD database, it sometimes struggles with false positives. However, as has been observed, false positives are inevitable, as the analysis is only occasionally straightforward.
Analysis
This is the hard part and actually, the one when tooling can’t help us much. Consider the SnakeYAML remote code execution vulnerability as an example. For it to be exploitable, the library would have to be used unsafely, such as parsing data provided by an attacker. Regrettably, no tool is likely to reliably detect whether an application and all its libraries contain vulnerable code. So, this part will always need some human intervention.
Resolution
Upgrading the library to a fixed version is relatively straightforward for direct dependencies. Tools like Dependabot and Renovate can help in the process. However, the tools fail if the vulnerable dependency is introduced transitively or through dependency management. Manually overriding the dependency may be an acceptable solution for a single project. In cases where multiple services are being maintained, we should introduce centrally managed dependency management to streamline the process.
Most reports are false positives, so it’s crucial to have an easy way to suppress the warning. When using OWASP dependency check, either try a shared suppression file or a tool like Dependency Shield that helps with this task.
It often makes sense to suppress the report temporarily. Either to unblock the pipeline until somebody has time to analyze the report properly or until the transitive dependency is updated in the project that introduced it.
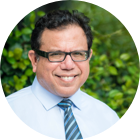
MMS • Daniel Dominguez
Article originally posted on InfoQ. Visit InfoQ
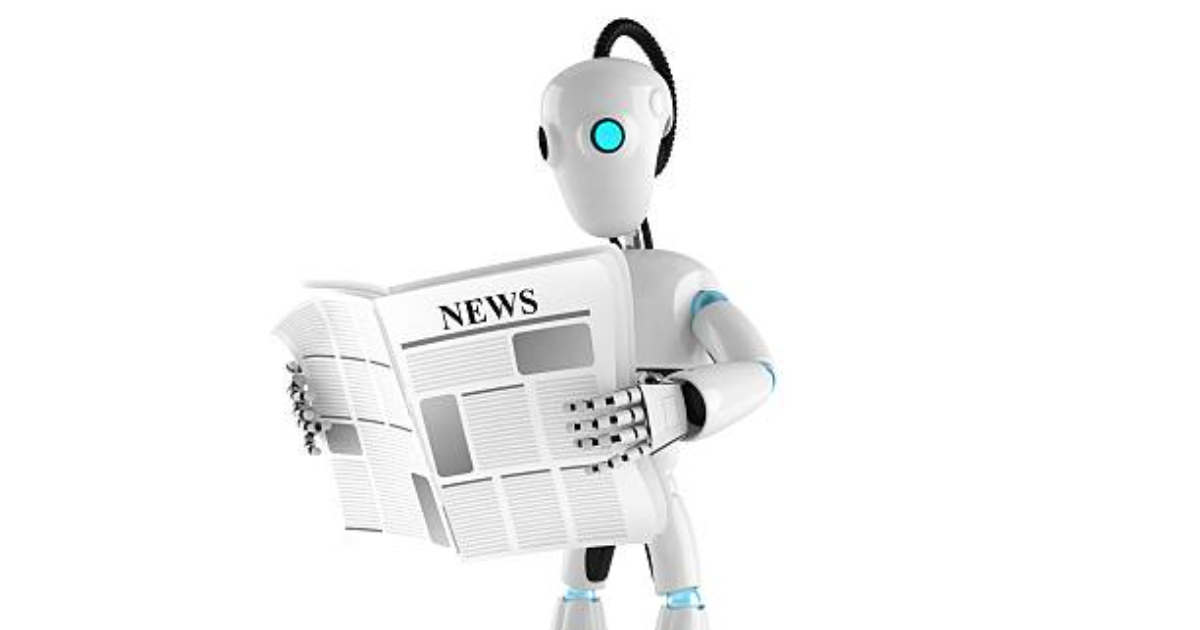
The most recent update, which covers developments through September 4, 2023, highlights significant pronouncements and accomplishments in the fields of artificial intelligence, machine learning, and data science. Developments from Stability AI, Google, OpenAI, and Meta were among this week’s significant stories.
Stability AI Launches Stable Chat
Stable Chat is a novel AI-powered chat platform designed to prioritize stability and consistency in conversation with users. Stable Chat, developed by Stability AI, aims to reduce the potential for misinformation and misunderstandings in AI-driven conversations by focusing on delivering reliable responses instead of generating creative or unpredictable ones.
This approach could find applications in critical domains like healthcare and customer support, where maintaining clarity and correctness in communication is paramount. The platform’s unique focus on stability makes it an intriguing addition to the evolving landscape of AI chatbots and conversational agents.
Vertex AI Search and Conversation is Now Generally Available
Google Cloud’s Vertex AI Search and Conversation service has officially become generally available. This development empowers organizations to enhance their applications with AI-driven search and conversational capabilities, facilitating more intuitive and efficient interactions with users. With features like semantic search and natural language understanding, Vertex AI Search and Conversation enables businesses to build intelligent search engines and conversational agents that can provide relevant information and engage users in natural conversations.
This launch represents a significant step forward in leveraging AI and machine learning technologies to improve customer experiences and drive innovation in various industries.
OpenAI Introduces ChatGPT Enterprise
OpenAI has launched ChatGPT Enterprise, a subscription-based service aimed at businesses to leverage its powerful language model for various applications. This offering provides enhanced language capabilities tailored for professional use, including improved security features and access controls. With ChatGPT Enterprise, organizations can harness the potential of natural language understanding and generation to enhance customer support, automate tasks, and develop customized AI solutions while maintaining data privacy and compliance.
OpenAI’s move signifies its commitment to catering to enterprise needs and expanding the adoption of AI-powered language models in business contexts.
OpenAI has made GPT-3.5 Turbo Available to Developers
OpenAI has introduced the GPT-3.5 Turbo, an advanced iteration of its language model. This new version allows fine-tuning, enabling users to customize and adapt the model for specific tasks.
OpenAI also unveils updates to the API pricing structure, making it more cost-effective for developers to experiment and deploy GPT-3.5 Turbo-powered applications.
Meta Open-Sources Code Llama
Meta has unveiled Code Llama, a novel AI tool designed to assist developers in writing code more efficiently. Code Llama employs large language models and deep learning techniques to understand and generate code, aiming to simplify the coding process and boost developer productivity.
This tool is a significant addition to the rapidly evolving landscape of AI-powered development tools and further exemplifies Meta’s commitment to advancing AI technologies.
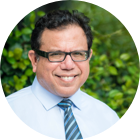
MMS • Mark Fussell Yaron Schneider
Article originally posted on InfoQ. Visit InfoQ
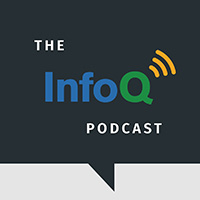
Subscribe on:
Thomas Betts: Hey folks. Before we get to today’s podcast, I wanted to let you know that InfoQ’s International Software Development Conference, QCon is coming back to San Francisco from October 2nd to the 6th. At QCon, you’ll hear from innovative senior software development practitioners, talking about real world technical solutions that apply emerging patterns and practices to address current challenges. Learn more at QConsf.com. We hope to see you there.
Hello and welcome to the InfoQ Podcast. I’m Thomas Betts and today I’m joined by Mark Fussell and Yaron Schneider. Mark is CEO and Yaron is CTO of Diagrid, a developer focused startup. They’re both co-creators of the Distributed Applications Runtime Project, Dapr. For the last couple of years, Dapr has been on the InfoQ Architecture and Designs Trends Report. This year was part of the discussion around design for portability and cloud bound applications. Today we’ll be talking all about Dapr, from its creation in 2019, through its adoption by the CNCF and where it’s currently headed. We’ll cover the use case of Dapr and help you determine if it might be a good fit for your projects. Mark and Yaron, welcome to the InfoQ Podcast.
Yaron Schneider: Thank you so much for having us.
Mark Fussell: Wonderful to be here.
What is Dapr? [01:06]
Thomas Betts: So let’s start at the beginning. What is Dapr and what problem is it trying to solve?
Mark Fussell: Dapr is a developer productivity suite of APIs, so effectively enables developers to build distributed applications in half the time they would do normally. And effectively what you have with Dapr is APIs that codify the best practices for building distributed applications.
What this means in practice, is that we’ve seen developers struggle to build on top of platforms, even ranging from single VMs, but particularly around Kubernetes. To run and build applications that codify, like event-driven architectures, or messaging between services, or orchestration of applications, and particularly around microservices architecture. So instead we took all the learnings from that, understood the problem space, and when you get down to the complexities of these applications, there’s many things you have to think about. So we took all that, codified it into a set of APIs that you can access from any framework of your choice, whether it’s the language of your choice or framework of your choice over HTTP or GRPC, to be able to use these APIs to productively create distributed applications.
Thomas Betts: And then how does Dapr compare to other ways? You mentioned you’re codifying, the ways you’re implementing, say event-driven architecture or microservices architecture. Is this just in comparison to building all of that from scratch? Is that the only other option?
Yaron Schneider: Yes, mostly we see developers write all of these functionality themselves, and so they end up with these huge chunks of boilerplate code they then need to maintain sometimes for years to come. And all of this code becomes even more complex if they run in a multi-cloud environment. So they have multiple code bases for different environments they’re running in and Dapr provides them with these abstractions that allow them to, in a multi-cloud world, run very easily on top of different clouds.
But it’s also a lot more than that. For example, if you need a workflow engine or encryption between your services or pub/sub, you might take three different technologies to do that and then you need to learn different SDKs and how to secure and make them reliable. But with Dapr, it’s all a one-stop shop where you can take one API and incrementally adopt others, or you can just use it all together, but the developer experience stays the same. And so it’s very, very consistent in terms of security, reliability and the productivity gains the developer get when using it.
Sample use case: Storing secrets [03:25]
Mark Fussell: I would dive into a little bit on the problem space again, and that is when you actually build these applications, let’s just take a simple example of just getting hold of a secret that you need to talk to a database. We see this time and time again, that I had to get a hold of a secret that I need to manage and get a hold of it to talk to a database or some other resource and things like this. And there’s many different places where you store those secrets. And so what happens is that Dapr has this concept of an API that you can call as a developer. It’s a very straightforward API of HTTP, but behind the APIs we have this concept of components that allow you to map the infrastructure into that API. So you can map HashiCorp Vault, or one of the cloud providers like Azure Key Vault, or GCP Secret Store into that API.
So now you’re able to keep your code consistent, call the API in exactly the same way, but retrieve secrets from numeral different types of infrastructure in order to say, talk to your database and things. So that abstraction layer, but the plugability of having this backend infrastructure makes your code very portable. You can develop things locally, take it to the cloud, have multi-cloud deployment, change your choice of technology, and yet your code doesn’t change and remains consistent. And so what happens is it allows you to have a decision or an understanding across your team about how you access secrets in a consistent way, whether you’re a Python developer or a Java developer, it doesn’t matter.
Yaron Schneider: Yes. I think you touched on a really great point here, Mark. I think Dapr really simplifies the common design patterns that developers today are looking for in systems. So for example, a developer needs to grab hold of a secret, use that to connect to a database or a pub/sub, then write some state to a database and once that states been written. They will want to communicate some business event that occurred and that’s a very, very hard problem and there’s lots of boilerplate infrastructure code they need to write and maintain.
But with Dapr, you actually do that with literally three lines of code and one or two YAMLs. And so this is a really, really big aspect of Dapr, just simplified developers’ lives and as Mark correctly mentioned, not hijack their infrastructure. So Dapr can actually connect to their existing infrastructure and meet operators and developers where they are, instead of having to have them change their core infrastructure model and just use something that does only that specific part.
Dapr is not just for greenfield development
Thomas Betts: I want to say that point you said, it doesn’t hijack your infrastructure and meets people where they are. That sounds like this isn’t just for Greenfield applications, so I can add some Dapr in, because I need to do a new connection to a key vault. I need to have my secret store and instead of saying, “Well, let’s just write a connection to the key vault, I’ll call Dapr to find that we’re using key vault behind the scenes and then my .NET code works and my Java code also has a very similar interface to that.” Is that simplifying it pretty well?
Yaron Schneider: Yeah, spot on.
Mark Fussell: Yeah. And in fact, the whole adoption of Dapr is very much a brownfield approach. You can use one API, two APIs, it’s not expected in any way that you throw away your code and start to use Dapr as the sole framework, it’s very incrementally adopted. In fact, that’s the most common use case we see, that people first just use an API to retrieve a secret. And then they decide they’re taking their monolith and splitting it into microservices and now doing service communication between them or event driven between them. And now they adopt the service invocation API, that allows them to securely call between two services, or they need to talk to a completely different external system and just send a message to it that has got nothing to do with Dapr, and they can do that over the pub/sub APIs. And so incremental adoption and integration with other systems is a key tenant of Dapr.
Dapr can help ease the transition from a monolith to microservices [06:57]
Thomas Betts: So that monolith to microservices adoption, there’s a lot of people who struggle with that and trying to find the right size service. Is it a monolith? Is it a 100 microservices? Is it something in between? You’re basically saying, Dapr can fit in whatever your architecture is and if you’re making a transition, can it help smooth that transition from one to the other?
Yaron Schneider: Exactly, yes. We see people using Dapr from complete monoliths. They have a huge code base, it’s run on one huge machine. They use Dapr there because Dapr gives them other security and reliability benefits. But when people really get to the nitty-gritty details of breaking down the monolith architecture into separate services, that’s where Dapr really, really shines, because it gives you these microservices best practices really from out of the box.
So for example, in the microservices architecture, you want to have each service manage its own state, and that’s really hard to code to, but Dapr does that out of the box. So it’ll actually do data segregation between services and make sure that they’re all secure, that they’re all encrypted based on the identity of the service calling it. So when you transition to microservices, Dapr basically takes all of the infrastructure boilerplate that you’d need to write and lets you focus on what you as a developer really want to focus on most, which is your business logic.
Architectural trade-offs [08:08]
Thomas Betts: And what are the architectural trade-offs? So if I’m looking at bringing Dapr in, how do I say this is a good place where we can add Dapr and what am I trading off if I were to go on, I guess traditional code path of not using Dapr?
Yaron Schneider: I would say what you’re trading off are specific features from the underlying infrastructure that you’re using. So because Dapr gives you a consistent API, you need to adhere to that API and not every feature from, let’s say Kafka, or Redis, or whatever system you’re using is going to be there, but most of the time if you’re missing a feature, you become a part of our big community. You go to GitHub, you’re raising an issue, you ask for the feature and most chances are it’ll get added. But if you’re really using esoteric specific features from some implementation that you really rely upon for your hot path, Dapr might not be the best fit there.
Mark Fussell: Dapr also runs in a sidecar model as well, which runs next to your process and that’s actually quite important, because it actually segregates out your code from Dapr’s runtime code. It allows you to determine and you have very, very, very low latency and Dapr is highly performant in terms of end-to-end calls, but because you have that separation of Dapr runtime code from your code, I think it also helps with understanding debugging and where responsibility lies.
Benefits for developers [09:20]
Thomas Betts: Well, and that gets to my next question. So we talked a little bit about architecture. How does this change for developers? And you’re saying it simplifies what they have to debug, because the Dapr runtime is not something you’re dealing with. It’s almost like the infrastructure is not something you have to deal with, that you just focus on your code and your code has less infrastructure built into it then.
Mark Fussell: One thing Dapr provides, it does, is not only just these APIs, but it provides cross-cutting concerns. So it provides end-to-end observability of all your calls, it provides end-to-end resiliency policy in your calls, and it also provides zero-trust security in many cases and I think these are also key things that developers have to build. So for example, let’s take observability. Dapr, by taking hold of the observability and having calls through the pub/sub API and service invocation uses W3 tracing combined with open telemetry. So you can see calls that go through pub/sub, service invocation and you see this complete picture. And so when you take Dapr and run it in the production service, you’re now able to push all that telemetry data into any observability tool of your choice and now see a full picture of how your application is running. I think the same with resiliency as well, is that you have these resiliency policies that help make your calls between services more reliable, retries, timeouts, circuit breakers and make sure that you can run resiliency at scale.
Yaron Schneider: I think one more thing that Dapr does really well, is extend all of those features that Mark just mentioned, from service to service calls. Which is something you might get with something like service mesh, for example, like Istio or Linkerd and Dapr really extends that to your entire infrastructure stack. So Dapr can not only secure and make reliable calls between your different services, it can also do that between your services and their database, or configuration store, or secret store, or workflow engine. And so Dapr really takes the concept of zero trust security and layers it holistically across all of your infrastructure stacks.
Benefits for platform engineering teams [11:12]
Thomas Betts: I want to go back one little bit. You said that you lose the flexibility of having all of the features brought up. Is an analogy to this, Terraform is a way to write your infrastructures code, but it’s cloud-agnostic. Like you wrote, I need a new service, I need a new pub/sub, but it can work on Azure, it can work on AWS. And so you may not get those individual features that Amazon or Microsoft provides you, but you get the commonality and Dapr sounds like the same thing. You get all the best common features and you said it’s the best practices. So what are you losing and what are you gaining by making that trade-off?
Yaron Schneider: So you’re losing the specific features that we talked about earlier. If you need very specific esoteric implementations, you’re probably not going to find it immediately. But then again, if you go to a community and you ask for it, there’s a good chance that someone will pick that up and add it in the future. But what you’re gaining are consistent APIs in a very consistent way architecturally to do security and resiliency, and also observability between your services. And if you’re in a multi-cloud world, then it greatly simplifies your entire development endeavor going forward.
Mark Fussell: We’ve seen Dapr being adopted by a lot of internal developer platform teams and the reason why they’re doing it, and in fact it’s written into a CNCF paper, if you look at the CNCF paper. But the reason why they’re doing it is because they can provide these consistent APIs from a platform team to the application teams consuming it, along with the underlying infrastructure that they have as well. So they can define infrastructure that you have, but then well, how do they surface up that infrastructure? Dapr can provide that sort of consistent set of APIs in platform teams, to multiple application teams who are consuming platforms as well. We’ve seen this happen particularly well in pub/sub, for example, with large organizations who want to bring existing legacy applications and new ones together under a single umbrella of APIs.
Yaron Schneider: It’s also worth mentioning the Dapr isn’t strictly a lowest common denominator, but in many cases it actually adds features that you don’t find if you were just going straight into the default implementation. So one example of that is Kafka in topic authorization. With Kafka open source, you don’t get to decide which applications can subscribe or publish to very specific topics, but if you pair up Dapr and Kafka, you suddenly get that. And these type of features that Dapr just adds, that weren’t there before it, are many. So you can pair up Dapr with different technologies and it’ll just add on top of what you would’ve been getting.
Dapr is the golden path for writing cloud native distributed applications [13:34]
Thomas Betts: You mentioned the platform teams use this, and that’s why I was wondering who is responsible for managing Dapr and using Dapr? And it sounds like it’s not the individual developers writing their business application, it falls onto that platform team. So it’s the platform team, instead of writing everything by hand, they’re now using Dapr to make their lives easier, which then makes the developer’s lives easier as well.
Mark Fussell: Well, I would start with developers still. It’s still a developer centric set of APIs. It’s just that anything that runs in platform teams, there’s a control plane services that Dapr installs. For example, in Kubernetes where it does things like deploy certificates or issue certificates to all of the running services that you have, in order to do secure communication. So there are operational aspects of it that you have to have when running in a production environment, but it’s still a developer-centric thing. So it crosses those boundaries as Yaron talked about earlier.
Thomas Betts: So when I’m writing my code, I need to know the Dapr interface, or is there a opinion that my platform team can interject and say, “My company decided we’re going to put a layer of abstraction on top of Dapr and this is how we, as a company talk about these things?” Is that something you build in or does everyone talks to Dapr API in their code?
Yaron Schneider: Yeah, usually it’s the former, everyone talks to Dapr API. That’s what we normally see, but we’ve also come across companies who wrap Dapr inside of their own abstractions. So we see both really.
Thomas Betts: I guess it’s all a matter of, again, the trade-offs of what your team and how big your services are and how complicated your platform is, whether you think it’s beneficial to add that layer of abstraction, or to provide that to your customers that are the developers.
Yaron Schneider: Yeah, that’s a fair assessment.
Mark Fussell: But Dapr is designed as a set of APIs that you can use directly. And going back to the essence of it, again, we just saw time and time again, common best practices that you have that are written about extensively everywhere in the internet and the like. Here’s how you should design systems that do event processing, here’s how you communicate between services, lots and lots of guidance, but very little practical implementation of those. And if they were, they very isolated and it was very small pieces of libraries you had to stitch together yourself and there wasn’t a cohesive single platform or runtime that you can bring together. And that’s what Dapr does, it take all that experience that’s written about, put it into a set of APIs that work well together and help develop and solve all the problems they’ve experienced, so that they can get to building their application. And we’ve seen that people who use Dapr, 20 or 30% more productive getting their application done in time in terms of the productivity they get from using it, as opposed to having to stitch it together themselves.
Thomas Betts: I think if you see companies that have a really good platform engineering team that says, “Here’s the platform we build on.” They understand the developer experience and how you can have that 10x effect of I can create one thing and then we have a codified way that we as a company build this. And you’re saying you don’t even need to define it yourself, we have Dapr, which defines it for us.
Yaron Schneider: Exactly. I think a major piece of any platform engineering team is to provide golden paths for the developers and Dapr is the golden path for writing cloud native distributed applications.
New features are continually added to Dapr [16:36]
Thomas Betts: So you’ve mentioned a few things, and I’m sure there’s a whole list we can point to on the website, but things like it can abstract away Kubernetes and individual services, microservices architecture. You mentioned event-driven architecture, secret stores and is there all of those little pockets of domain knowledge. Is this something that Dapr is continually adding to and we’re adding new features that say, “Oh, we don’t support this yet, but we’re working on it?
Yaron Schneider: Yes. I think we’ve added around three new APIs in the last year alone, and there’s more up and coming. For example, recently we’ve added workflows and the cryptography API that we didn’t have earlier. And on a roadmap, we have proposals for things like document APIs, SQL APIs and others. So the Dapr committee is always thinking ahead about what are the next developer challenges that the project can help solve?
Mark Fussell: Yeah. And one of the APIs I mentioned until now is a configuration API. You see a lot of systems that need feature flagging type approach, where they deploy things and they want to turn on/off features at runtime while the application is running. That provides a configuration API that does exactly that feature flagging in a dynamic application. So those are sort of things that have been added, that up until then you’d have to go and find a library, build it yourself, things like that. So Dapr very much is about discovering those APIs, finding the problem space that they solve, integrating them with a huge range of different infrastructure options as they exist, but helping you be productive, whether it’s communication, or feature flagging, or secrets management. That’s what added, then as you alluded to it, I think an amazing one that’s coming down the pipeline is workflow.
The Dapr origin story [18:10]
Thomas Betts: Let’s go back to the beginning. So Dapr, as far as I know, started at Microsoft. Is that correct?
Mark Fussell: Yes.
Thomas Betts: And did both of you come from Microsoft before you went to Diagrid? So give me a little bit of the backstory, what’s the origin story of how did this come about? Why did Microsoft decide to build this? And I guess I really want to know, why is it not just Azure specific? Why is it not just .NET specific?
Yaron Schneider: So Mark and I both worked at a team called the Azure incubations team, which reported to the Azure CTO, Mark Russinovich. And in that team we were looking at how we can improve developers’ lives running cloud native applications, because we saw Kubernetes really as the way to inject these defacto APIs for hosting apps, but application developers largely went ignored.
So we went on this research path, Mark and I both came at it from different directions, and then we got together and we basically brought together a prototype that demonstrated how developers can build applications easier on top of platforms like Kubernetes. And we really decided that in order for that to really change the industry, it needs to be open source, vendor neutral, and driven by the larger community. So we’ve decided consciously not to make it a Microsoft Azure specific thing, because the vendor neutrality of the project would mean that you’re inclusive to all developers coming from all clouds, and this is really what’s needed to make something like this really become an industry changing piece of technology.
Dapr runs anywhere [19:33]
Thomas Betts: And so it plays well on any cloud. Can you also run it internally on your own infrastructure if you want it to?
Yaron Schneider: Yes, definitely.
Mark Fussell: Oh yeah, totally. I mean, one of the original Dapr goals was you can run it as a process, you can run it as a container, so it’s not bound by that. You can integrate it with any underlying host infra platform. So Dapr will run happily today on a set of VMs, it runs on your local machine. Of course, the main deployment environment we see is Kubernetes in terms of production, but you could integrate it with any other distributed systems platform of your choice. Of course, Kubernetes has become to dominate in the world nowadays and the likes of Mesosphere, that don’t really exists anymore. But I mean, there’s no reason why Dapr couldn’t run on any of those platforms, it’s not just bound to Kubernetes and so that’s its flexibility. In the end, it’s a set of APIs that can run anywhere, but of course, Kubernetes in production, local machine for development. But that’s the idea and that was one of its original goals.
Yaron Schneider: And it’s really easy to run Dapr, it just runs as a single binary that executes and starts up in less than 10 milliseconds on every VM, including your own personal developer machine, whether it’s Windows, Linux, or Mac.
Mark Fussell: And it’s super lightweight as well. So you get all of these huge benefits in a very lightweight process that runs next to your machine.
Dapr is written in Go [20:47]
Thomas Betts: And how did you get it to be that efficient? I believe it’s written in Go, is that correct?
Yaron Schneider: Yes. Go’s allows us for really good network primitives and high concurrency, while retaining a small binary size and low memory usage. So Go fits perfectly within that usage model for things like Dapr.
Mark Fussell: So going back to your origin story, Yaron and I sat down and went like, no one does anything for application developers. So we thought about, how is it you have a world of best practices, APIs running on any platform, independent to vendor, that allows you to singularly adopt APIs and that you’re not having to say, “Throw away all your code and rewrite this whole brand new framework.” And that was exactly his original goal, and what’s happened is it’s achieved all those goals and more.
I mean, we’re just surprised by the enormous success of Dapr, how it’s grown, the community, the contributors, the organizations and it’s accelerated since it got donated to CNCF. So it’s gone far beyond our expectations that we ever thought it will do.
Dapr is currently a CNCF incubation project [21:47]
Thomas Betts: And so it started at Microsoft and you said it was open source from the beginning and not cloud specific, not language specific, anyone can use it. And like you said, it runs as a sidecar, so it’s not in your process, it’s just sitting next to it. Once it left the Microsoft sphere, what happened to it? I know it’s a CNCF project. Can you tell me more details about that and how it’s currently being supported?
Yaron Schneider: Yeah. So Dapr actually had a steering committee running the project before we joined CNCF, and those same four vendors that started out with driving the project direction are still there today. Those companies are Microsoft, Alibaba Cloud and they are running Dapr at scale inside of their own internal infrastructure, Intel and today, Diagrid. So these are the four vendors running the project and as I said, it was out there before we joined CNCF, but of course this plays really well with the CNCF concept of vendor neutrality. And since we’ve joined, we’ve seen lots of contributors coming from any other companies, helping contribute to the project to make it better for both their use cases and for our use cases of other developers, who are just telling us what features they need on GitHub publicly.
Mark Fussell: In terms of the size of the project, Dapr is the 10th largest project in terms of numbers of contributors for its project inside CNCF, as measured in the CNCF Velocity Reports that come out. And so that is something that’s an indication of its success as well.
Yaron Schneider: Yes, today we have over 2,850 contributors to the project.
Thomas Betts: Wow, that is definitely more than I expected.
Dapr should become a graduated CNCF project soon [23:12]
Thomas Betts: So it’s in an incubator status right now, where does it go next? I’m not fully up to speed on how the whole CNCF adoption process works.
Yaron Schneider: Yes, we are looking to graduate the project of course, because that’s the CNCF expectation of any project that goes into it after two years, and so it’s going to be two years this September. So we don’t have the official decision to graduate just yet, but I think you can surmise from what I’m saying about what’s going to come next.
Mark Fussell: Yes. Graduation with inside CNCF is an important direction for the project, it shows and validates its maturity. Dapr can achieve that, because it shows a huge amount of end user adoption, as CNCF calls it, it has a huge number of contributors. The project continues to grow, has a great collaboration across many companies. Those are all the indicators they look for success, because graduated projects are there for the long-term, for not only because CNCF want them to succeed, but they want to continue to show that they’ve got mass adoption and mass contribution. And everything in Dapr points to success of doing that in the next year as well, or probably less.
Thomas Betts: As the main point of having that graduated CNCF status to give a stamp of approval, that people who may have been waiting, that they weren’t the innovators and early adopters, they’re like, “We need to have a little bit more validation before we pull it in.” That’s saying, “Hey, this is good to go and saying you can trust to be there for a while.”
Yaron Schneider: Yeah, definitely. It’s going to help a lot of the late adopters and enterprises out there.
Mark Fussell: We also work quite hard with CNCF, publishing case studies as well. So if you go to CNCF and you look up Dapr case studies, we published one recently there from a company called DeFacto, which is the largest clothing retail inside Turkey and they deployed a huge cluster on top of Red Hat OpenShift. It was running Dapr, it was 200 plus services that they were running on top of Dapr. And it’s just a great case study of how they built something that dealt with the scale of their orders and in their retail world of things. And we’ve got more coming along in terms of financial, IoT, and a whole range of different types of industries that Dapr gets used in.
Thomas Betts: And that just speaks to, it’s not meant to solve one specific business problem, it’s meant to solve a platform-level problem. And every industry has these challenges, especially when you get to a certain scale these make sense to use.
Mark Fussell: Yeah, that’s exactly right. It’s industry agnostic, anyone who’s building these backend distributed systems, whether you’re in healthcare, medical, manufacturing, IoT, retail, they’re all building teams. Many of those are on top of Kubernetes today, as Kubernetes grows, Dapr grows with it, but that’s the goal. Make developers productive, stop you having to stitch together a thousand different technologies yourself. Just allow me to use a consistent set of APIs that integrate with my infrastructure and let me go while and get my project done.
Getting started with Dapr and running locally [25:51]
Thomas Betts: So some of our listeners are like me and want to just get their hands dirty and start using this. What’s a good hello world, how do I get started?
Yaron Schneider: We have amazing tutorials and quick starts. So I suggest you go to docs.Dapr.io and in there there is a getting started section, which will guide you exactly on how to get started. It’ll also point you to our tutorials and samples page, you can just fire those up, you can get started with Dapr in less than five minutes really.
Thomas Betts: And you said I can run all this on my local machine, I don’t have to have it deployed to a cloud yet, and I can just experiment around and see what it does.
Yaron Schneider: That’s correct.
Mark Fussell: Yeah. In fact, that was one of the key goals of Dapr, was install the CLI locally, install a developer environment that you can run, build everything locally, test it locally. You can have a great experience and as we mentioned before, the beauty then is that you literally switch out component infrastructure from local state stores. For example, running an old machine, to the state store that you use in the cloud and none of your code changes. So the local developer experience with the CLI is very straightforward. In fact, that’s one of the key benefits we hear from many, many developers, great local development and I can move to the cloud and none of the friction between them all, and it’s been one of its key success points.
Thomas Betts: And that’s something I’d be interested to know more about is, so am I running everything locally or is it all just mocked out? You said there’s things like a local state store instead of whatever store I’m using and Dapr locally knows, is it just YAML configuration to tell it what to do in those situations?
Yaron Schneider: It’s a YAML configuration, and when you use the Dapr CLI, we install Redis on your local machine, just because Redis is also lightweight and it can run anywhere. So we use that as the default dev tool. But then if you want to change it to something like AWS DynamoDB, if you’re later deploying to a cloud environment, all you need to do is really change that Redis YAML into an AWS DynamoDB YAML, but your code actually stays the same.
Mark Fussell: And the same with service invocation, for example, you can build and deploy multiple services on your machine. You’re talking between them like your order processing, say to your other services, receiving orders and you just communicate between them all. And then you take that code and you deploy it on, say your Kubernetes cluster and nothing changes. Other than that you just have to annotate in a YAML deployment in your Kubernetes YAML deployment, make sure this piece of code runs, has a Dapr sidecar instantiated next to it all, and that’s all you have to do. So the ability to move code, particularly to a Kubernetes environment is seamless.
Thomas Betts: And so that’s something I just put into my CI/CD pipeline that says, swap this out and use these configurations, and here’s our production environment versus my local development environment.
Yaron Schneider: Yes. Dapr is very configuration driven in that sense.
Connecting with the Dapr community [28:24]
Thomas Betts: Well, I think that covers everything I was hoping to get to. If you want to follow this and know what’s coming for Dapr, and you mentioned the docs, where else should they go to stay up to date?
Mark Fussell: Oh, yes. We should talk about the fact that we have a Dapr Discord community. So there’s a Discord server, that’s the place that you really should go to for all your questions. We have a community there of 6,500 Discord members on Dapr Discord, so it’s kind of growing and has a huge community, that’s the place you can answer your questions. Of course, come to the repo as well. The repo is a place where we outline the project goals, but also what’s in a project milestone, so you can see what’s being developed inside that. And between those two, as well as many calls, actually every two weeks we have a Dapr community call as well. In fact, we have one this Wednesday, but every two weeks on a Wednesday we have a Dapr community call and you can join up tonight and ask questions and see demos.
Yaron Schneider: We also have a growing YouTube channel with almost 4,000 members, one of the biggest in CNCF actually.
Thomas Betts: That just speaks to this very much being an open source project. Sounds like you’re trying to get feedback from everybody and get contributions and just keep the whole process very open.
Mark Fussell: We love our community calls, because what happens is we are forever trying to reach out to end users and say, “Come and demo something that you’ve built, a project you worked on.” Just to keep that excitement going, so people see what other people are doing with it. And it’s very inspirational actually, because you see some amazing projects that people build with Dapr, ways that it gets used that you wouldn’t have imagined.
Thomas Betts: Well, I think that’s about it for today. I want to thank again, Mark Fussell and Yaron Schneider for joining me today on the InfoQ Podcast.
Yaron Schneider: Thank you so much.
Mark Fussell: Thank you for having us.
Thomas Betts: I hope you’ll tune in again very soon. Have a good day.
Resources
.
From this page you also have access to our recorded show notes. They all have clickable links that will take you directly to that part of the audio.
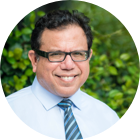
MMS • Daniel Dominguez
Article originally posted on InfoQ. Visit InfoQ
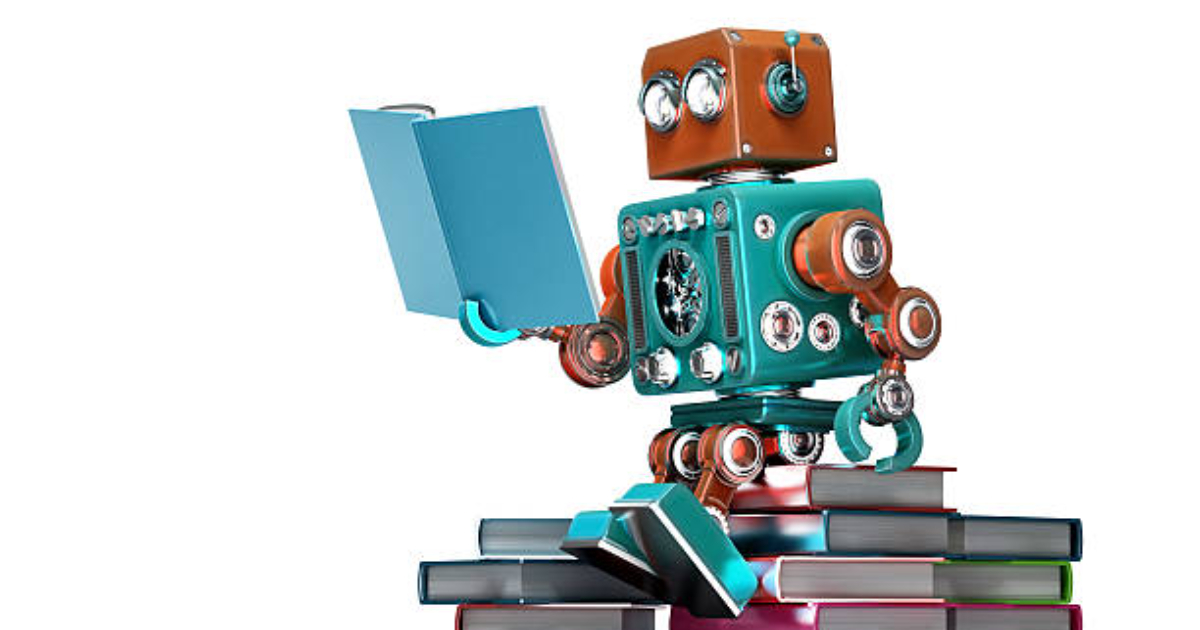
The most recent compilation of advanced research, inventive applications, and notable unveilings in the realm of Large Language Models (LLMs) during the week starting September 4th, 2023.
PointLLM: Empowering Large Language Models to Understand Point Clouds
This paper introduces PointLLM, a novel approach aimed at enhancing Large Language Models’ (LLMs) understanding of 3D data, particularly point clouds. PointLLM processes colored object point clouds with human instructions, demonstrating its ability to grasp point cloud concepts and generate contextually relevant responses. Evaluation benchmarks, including Generative 3D Object Classification and 3D Object Captioning, show that PointLLM outperforms existing 2D baselines, with human evaluators finding it superior in over 50% of object captioning samples.
Codes, datasets, and benchmarks are available at https://github.com/OpenRobotLab/PointLLM
WALL-E: Embodied Robotic WAiter Load Lifting with Large Language Model
This paper explores the integration of Large Language Models (LLMs) with visual grounding and robotic grasping systems to enhance human-robot interaction, exemplified by the WALL-E (Embodied Robotic Waiter load lifting with Large Language model) system. WALL-E utilizes ChatGPT’s LLM to generate target instructions through interactive dialogue, which are then processed by a visual grounding system to estimate object pose and size, enabling the robot to grasp objects accordingly. Experimental results in various real-world scenarios demonstrate the feasibility and effectiveness of this integrated framework.
More information can be found on the project website https://star-uu-wang.github.io/WALL-E/
AskIt: Unified Programming Interface for Programming with Large Language Models
In this paper, authors discuss AskIt, a domain-specific language (DSL) designed to simplify the integration of Large Language Models (LLMs) in software development. AskIt offers type-guided output control, template-based function definitions, and a unified interface that bridges the gap between LLM-based code generation and application integration. It leverages Programming by Example (PBE) for few-shot learning at the programming language level, achieving significant prompt length reduction and improved speed in benchmark experiments. AskIt aims to streamline the efficient and versatile utilization of LLMs’ emergent abilities in software development, with implementations available in TypeScript and Python.
The implementations of AskIt in TypeScript and Python are available at https://github.com/katsumiok/ts-askit and https://github.com/katsumiok/pyaskit, respectively
Jais and Jais-chat: Arabic-Centric Foundation and Instruction-Tuned Open Generative Large Language Models
This paper introduces Jais and Jais-chat, an Arabic-focused large language model (LLMs) with 13 billion parameters. These models outperform existing Arabic and multilingual models in Arabic knowledge and reasoning capabilities and remain competitive in English despite being trained on less English data. They provide a detailed account of their training, tuning, safety measures, and evaluations, and both the foundation Jais model and instruction-tuned Jais-chat variant are released to foster research in Arabic LLMs.
Accessible at https://huggingface.co/inception-mbzuai/jais-13b-chat
Java News Roundup: JDK 22 Release Schedule, Project Babylon, Helidon 4.0-RC2, MicroProfile 6.1-RC1
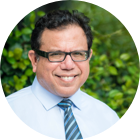
MMS • Michael Redlich
Article originally posted on InfoQ. Visit InfoQ
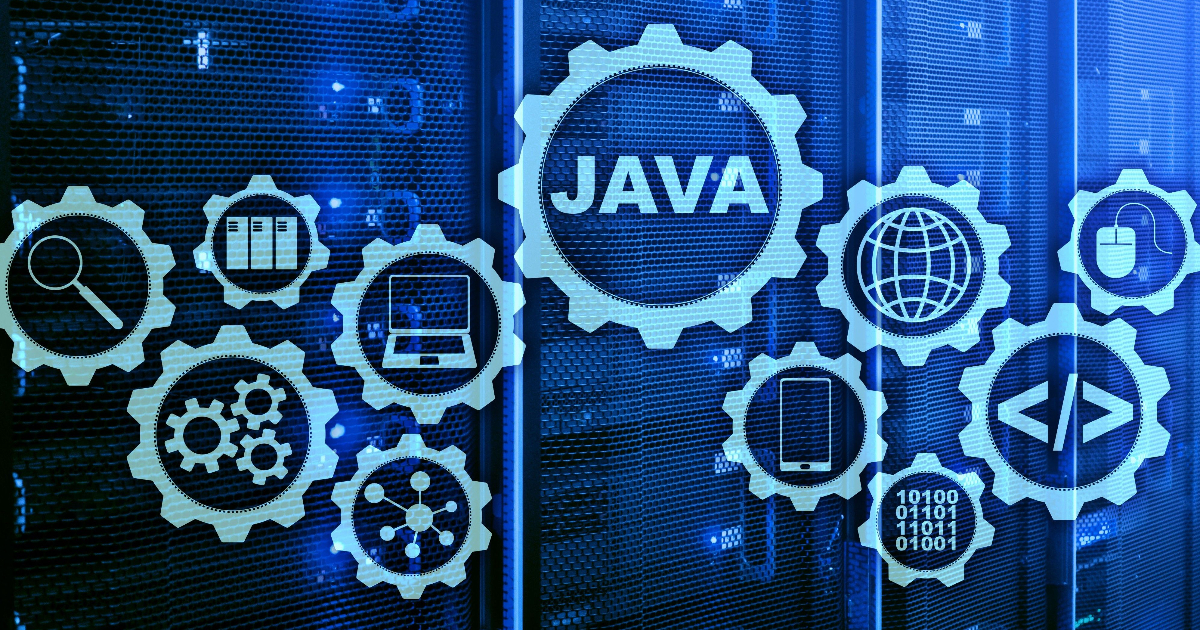
This week’s Java roundup for September 4th, 2023 features news from OpenJDK, JDK 22, JDK 21, GraalVM Native Build Tools 0.9.26, Quarkus 3.3.2, MicroProfile 6.1-RC1, MicroProfile Config 3.1-RC2, Helidon 4.0.0-M2, Open Liberty 23.0.0.9-beta, Hibernate Search 7.0.0.Beta1, Infinispan 14.0.17.Final, Eclipse Mojarra 4.0.4, JDKMon 17.0.71, JHipster 8.0.0-beta.3 and 7.9.4 and JavaZone 2023 Conference.
OpenJDK
Paul Sandoz, Java architect at Oracle, has initiated a proposal to start a new Java project named Babylon. The primary goal will be to “extend the reach of Java to foreign programming models such as SQL, differentiable programming, machine learning models, and GPUs.” Babylon can achieve Java’s reach to foreign programming models with code reflection, an enhancement to reflective programming in Java, to enable standard access, analysis, and transformation of Java code in a suitable form. Support for a foreign programming model can then be more easily implemented as a Java library.
Sandoz has offered to lead this new project with an initial reviewer list to include Maurizio Cimadamore, software architect at Oracle and Project Panama lead, Gary Frost, software architect at Oracle, and Sandhya Viswanathan, principal software engineer at Intel. Developers can learn more by watching this YouTube video from the recent JVM Language Summit.
Sandoz also introduced JEP Draft 8315945, Vector API (Seventh Incubator) this past week. This JEP, under the auspices of Project Panama, incorporates enhancements in response to feedback from the previous six rounds of incubation: JEP 448, Vector API (Sixth Incubator), to be delivered in the upcoming GA release of JDK 21; JEP 438, Vector API (Fifth Incubator), delivered in JDK 20; JEP 426, Vector API (Fourth Incubator), delivered in JDK 19; JEP 417, Vector API (Third Incubator), delivered in JDK 18; JEP 414, Vector API (Second Incubator), delivered in JDK 17; and JEP 338, Vector API (Incubator), delivered as an incubator module in JDK 16. The most significant change from JEP 448 includes an enhancement to the JVM Compiler Interface (JVMCI) to support Vector API values.
JDK 21
Build 35 remains the current build in the JDK 21 early-access builds. Further details on this build may be found in the release notes.
JDK 22
Build 14 of the JDK 22 early-access builds was made available this past week featuring updates from Build 13 that include fixes to various issues. More details on this build may be found in the release notes.
Mark Reinhold, chief architect, Java Platform Group at Oracle, formally proposed the release schedule for JDK 22 as follows:
- Rampdown Phase One (fork from main line): December 7, 2023
- Rampdown Phase Two: January 18, 2024
- Initial Release Candidate: February 8, 2024
- Final Release Candidate: February 22, 2024
- General Availability: March 19, 2024
Comments on this proposal from JDK committers and reviewers are open for discussion until September 15, 2023 at 23:00 UTC. If there are no objections at that time, then as per the JEP 2.0 process proposal, this will be the schedule for JDK 22.
For JDK 22 and JDK 21, developers are encouraged to report bugs via the Java Bug Database.
GraalVM
On the road to version 1.0, Oracle Labs has released version 0.9.26 of Native Build Tools, a GraalVM project consisting of plugins for interoperability with GraalVM Native Image. This latest release provides notable changes such as: use of the AttributeProvider API to fix compatibility with Gradle 8.3; explicitly declare the dependencies for the Plexus-Xml and Plexus-Utils libraries to fix compatibility with Maven 3.9.x; and prepare Native Build Tools for the upcoming release of GraalVM for JDK 21. Further details on this release may be found in the changelog.
Spring Framework
In terms of releases, things have been quiet over at Spring these past two weeks, but that hasn’t stopped Josh Long, Spring developer advocate at VMware. Along with his weekly “This Week in Spring” blog posts, Long has published: a personal recap of SpringOne 2023; his latest blog post on how Spring Boot 3.2, GraalVM native images, Java 21 and virtual threads with Project Loom all work together; and “Bootiful” podcasts with Rob Winch, Spring Security lead at VMware, Daniel Garnier-Moiroux, senior member of technical staff, Spring Engineering at VMware, and Chris Richardson, founder and CEO at Eventuate and president at Chris Richardson Consulting.
Quarkus
The release of Quarkus 3.3.2 ships with dependency upgrades and notable changes such as: improvements to the OIDC Auth0 in the Dev UI; a removal of the imagePushSecret()
method from the BuildConfig
class that has been deemed invalid when using the internal registry; and a fix for a Quarkus build using the quarkus.container-image.builder=jib
property that does not consider the auth.json
file from Podman. More details on this release may be found in the changelog.
MicroProfile
The MicroProfile Working Group has provided the first release candidate of MicroProfile 6.1 featuring updates to the MicroProfile Config, MicroProfile Metrics and MicroProfile Telemetry specifications. Therefore, the final feature set of MicroProfile 6.1, scheduled for a GA release in early October, will include:
- Jakarta EE 10 Core Profile
- MicroProfile Config 3.1
- MicroProfile Fault Tolerance 4.0
- MicroProfile Metrics 5.1
- MicroProfile Health 4.0
- MicroProfile Telemetry 1.1
- MicroProfile OpenAPI 3.1
- MicroProfile JWT Authentication 2.1
- MicroProfile Rest Client 3.0
It is important to note that four of the seven specifications contained within the Jakarta EE 10 Core Profile, namely: Jakarta Contexts and Dependency Injection (CDI) 4.0; Jakarta RESTful Web Services 3.1; Jakarta JSON Processing 2.1; and Jakarta JSON Binding 3.0, are the evolved JSR specifications from Java EE 7 and Java EE 8 from the early days of MicroProfile.
The second release candidate of MicroProfile Config 3.1 delivers notable changes such as: an update to the TCK to align with breaking changes in CDI 4.0 that include an empty beans.xml
file and change in bean discovery mode from all
to annotated
; and the MissingValueOnObserverMethodInjectionTest
class, that asserts a DeploymentException
, fails a different reason due to the the ConfigObserver
bean being defined as @ApplicationScoped
(proxyable) and final
(not proxyable). Further details on this release may be found in the list of issues.
Helidon
The second release candidate of Helidon 4.0.0 delivers: a baseline of JDK 21; full integration of the Helidon Níma web server; significantly refactored Helidon SE APIs to optimize imperative/blocking use cases; and numerous enhancements to the Web Server and Web Client components to achieve feature parity with Helidon 3.0. More details on this release may be found in the changelog.
Open Liberty
IBM has released version 23.0.0.9-beta of Open Liberty to provide continuous improvement: for Liberty Spring Boot Support 3.0 with capability to “thin” an application when they are created in containers; and the early preview of the Jakarta Data specification.
Hibernate
The first beta release of Hibernate Search 7.0.0 delivers a number of dependency upgrades, namely: JDK 11 as a baseline, a migration to Jakarta EE, Hibernate ORM 6.3.0.Final, Lucene 9.7.0, Elasticsearch 8.9.0 and OpenSearch 2.9.0.
Infinispan
Version 14.0.17.Final of Infinispan has been released featuring notable fixes such as: the cache created by ServerEventLogger
class blocks the cache join with the potential for a deadlock; the DefaultExecutorFactory
class creating unnecessary multiple instances of a Java ThreadGroup
; and add missing cross-site metrics for the implementation of the RpcManager
interface. Further details on this release may be found in the list of issues.
Eclipse Mojarra
The release of Eclipse Mojarra 4.0.4 delivers notable changes such as: a fix for Mojarra failing to initialize when the Bean Deployment Archive in Weld is empty; a more robust implementation of the RetargetedAjaxBehavior
class; and return a static empty data model from the UIData
class if its current value is null
. More details on this release may be found in the release notes.
JDKMon
Version 17.0.71 of JDKMon, a tool that monitors and updates installed JDKs, has been made available this past week. Created by Gerrit Grunwald, principal engineer at Azul, this new version provides additional information about the remaining days to the GA release of JDK 21 and the next OpenJDK update.
JHipster
The third beta release of JHipster release 8.0.0 with enhancements such as: support for JDK 20 and JDK 21; a cleanup of the JHipster Domain Language (JDL) to move file manipulation, configuration and validation to the JDL generator; and a fix for the H2 console not loading due to an incorrect path setting. Further details on this release may be found in the release notes.
Similarly, the release of JHipster 7.9.4 features bug fixes and support for Node.js 18. More details on this release may be found in the release notes.
JavaZone Conference
The JavaZone conference was held at the Oslo Spektrum in Oslo, Norway this past week featuring speakers from the Java community who presented talks and workshops on topics such as: garbage collection, quantum computing, Haskell, Kubernetes, application monitoring, micro frontends, JavaScript and Quarkus.