Month: September 2023
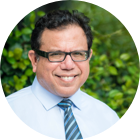
MMS • RSS
Posted on mongodb google news. Visit mongodb google news
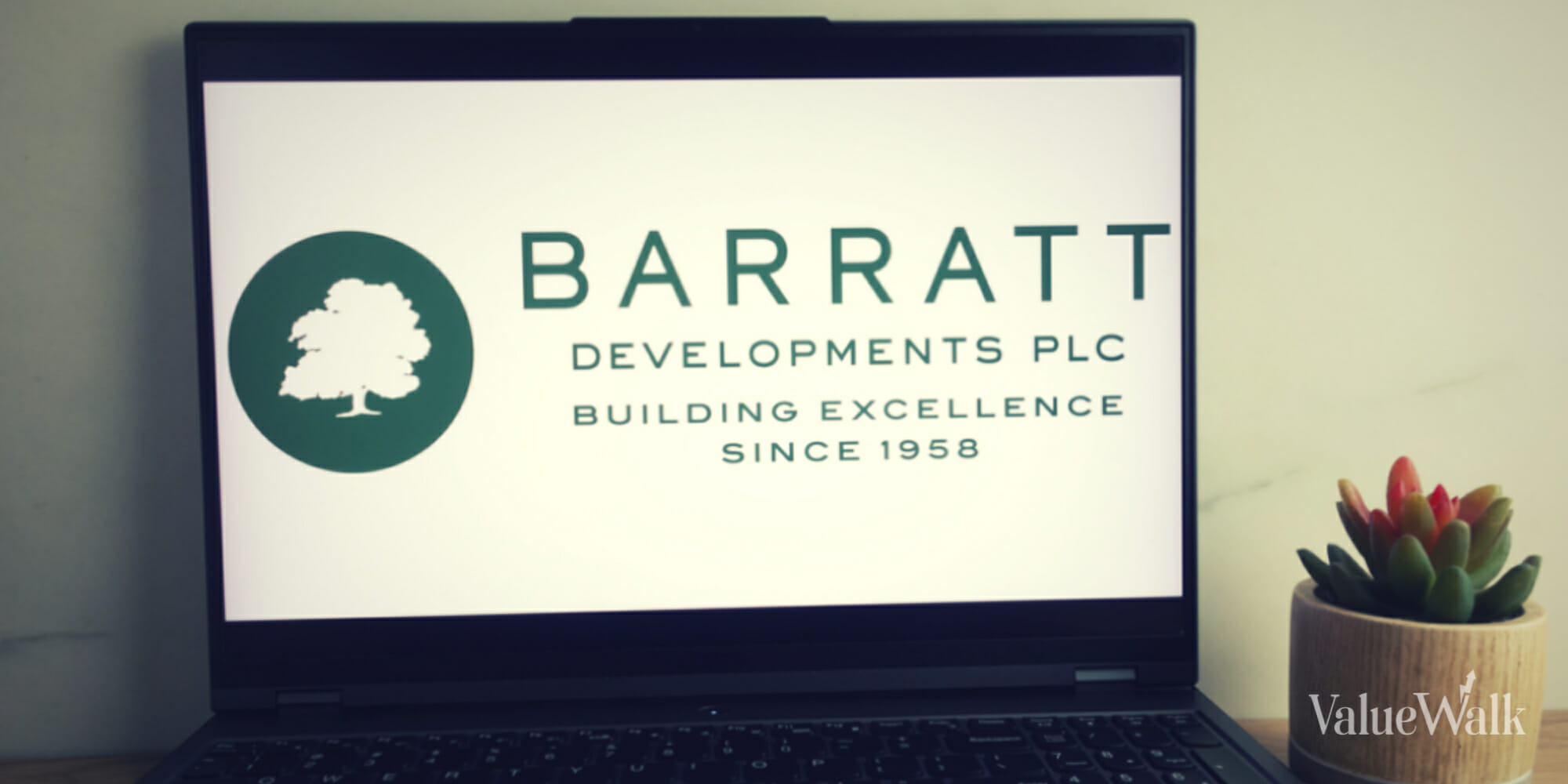
- Full-year revenues were up 1.0% to £5.3bn
- Underlying operating profit fell 18.2% to £862.9m
- Total home completions declined 3.9% to 17,206
Barratt Developments Earnings
“Barratt Developments PLC (LON:BDEV), the country’s largest housebuilder and sector bellwether, issued a largely unsurprising set of full-year results. Rate rises throughout the year have pushed up borrowing costs for buyers, making mortgage affordability much more difficult. Add to the mix the closure of the Help to Buy scheme and the fallout from the fiscal event back in September 2022 and you’ve got a potent cocktail, which saw Barratt’s net private reservation rates fall by around a third last year. All of this translated to a steep decline in underlying operating profit.
But it’s not all doom and gloom. Build cost inflation looks set to ease to mid single-digits this year. And a sharp reduction in land spend last year more than offset the share buyback programme, helping to keep Barratt’s net cash position broadly flat at a mighty £1.1bn. That provides plenty of flexibility to smooth out any future bumps in the road.
With interest rates set to remain higher for longer, consumer confidence and spending will continue to come under pressure this year, and it could be a while before momentum really picks back up again. Barratt’s valuation’s already trading well below the long-term average, so the market slowdown looks well priced in.”
For access to stock reports and articles please visit the Hargreaves Lansdown share research homepage or sign up to our updates here.
Article by Aarin Chiekrie, equity analyst at Hargreaves Lansdown
Article originally posted on mongodb google news. Visit mongodb google news
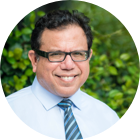
MMS • RSS
Posted on mongodb google news. Visit mongodb google news
On September 5, 2023, it was reported that Principal Financial Group Inc. had reduced its stake in MongoDB, Inc. by 16.4% during the first quarter of the year. According to the filing with the Securities and Exchange Commission, the institutional investor now owns 7,068 shares of MongoDB’s stock after selling 1,384 shares.
The value of Principal Financial Group Inc.’s holdings in MongoDB amounted to $1,648,000 as of their most recent filing with the Securities and Exchange Commission. This move by the financial group suggests a shift in their investment strategy or outlook on MongoDB’s future performance.
MongoDB, Inc. is a global provider of a general-purpose database platform. The company offers several products and services including MongoDB Atlas, a hosted multi-cloud database-as-a-service solution; MongoDB Enterprise Advanced, a commercial database server designed for enterprise customers to run either in the cloud, on-premise or in hybrid environments; and Community Server, which is a free-to-download version of its database that includes functionality for developers to get started with MongoDB.
As per data from NASDAQ on September 5th, MDB opened at $392.88 on Tuesday. The stock has shown a 50-day moving average price of $390.24 and a 200-day moving average price of $306.34. In terms of its yearly performance, MDB’s lowest point over the past twelve months was recorded at $135.15 while its highest point stood at $439.00.
With a market capitalization of $27.73 billion and a P/E ratio of -113.55, MongoDB holds significant presence in the industry it operates in despite reporting negative earnings per share (EPS). Its beta stands at 1.11 indicating moderate volatility compared to the market average.
MongoDB also maintains healthy liquidity ratios with both quick ratio and current ratio standing at 4.19 as reported in the most recent financial statements. These ratios signify the company’s ability to cover short-term obligations and suggest a stable financial position.
While Principal Financial Group Inc.’s decision to reduce its stake in MongoDB may have caught attention, it is important to note that investment decisions are complex and subject to various factors and considerations. Investors and analysts alike will be interested in watching how this adjustment by Principal Financial Group Inc. influences MongoDB’s future trajectory and if there will be further developments in their relationship moving forward.
Institutional Investors Show Confidence in MongoDB’s Future, Directors Sell Off Shares
September 5, 2023 – In recent news, there have been notable changes in the positions of hedge funds and institutional investors regarding the stock of MongoDB, Inc. These changes reflect a degree of confidence or skepticism held by these entities towards the company’s future prospects.
Cherry Creek Investment Advisors Inc. increased its stake in MongoDB by a modest 1.5% during the fourth quarter, acquiring an additional 50 shares. With this purchase, Cherry Creek now owns a total of 3,283 shares valued at $646,000. Similarly, CWM LLC also raised its position in MongoDB by 2.4% during the first quarter by purchasing an additional 52 shares. CWM LLC now holds 2,235 shares worth $521,000.
Cetera Advisor Networks LLC showed even more confidence in the company’s potential as it raised its position by 7.4% during the second quarter. The firm purchased an additional 59 shares, bringing its total holdings to 860 shares valued at $223,000. First Republic Investment Management Inc., on the other hand, took a more conservative approach but still increased its stake in MongoDB by 1%, equivalent to purchasing an additional 61 shares worth $1,261,000.
Janney Montgomery Scott LLC made a similar move and raised its holdings in MongoDB by 4.5% during the fourth quarter. This increase amounted to an acquisition of an extra 65 shares valued at approximately $298,000. Collectively, these institutional investors now own approximately 88.89% of the company’s stock.
In other significant news relating to MongoDB’s stock activity and ownership structure:
Director Hope F.Cochran sold off a considerable number of shares recently; specifically, on June 15thAmounting to a total value of $811315USD having sold-off roughly2174 sharesHope F.Cochran retained ownership over further8200 remaining shares
The company’s stock activity also saw Director Dwight A. Merriman undertake a similar move by disposing of 1000 shares on July 18th. The sale was completed at an average price of $420 per share, resulting in a total transaction value of $420,000. After the sale, Dwight A. Merriman currently owns 1,213,159 shares in the company valued at approximately $509,526,780.
These transactions were disclosed in filings with the Securities & Exchange Commission (SEC), aiming to provide transparency and accountability to investors. Interested individuals may access these filings via the official SEC website.
As a whole for this quarter alone-related insider dealings-includes multiple sales totaling 76,551 stocksUltimately representing near $31 million sold(stock value), those within work or directly associated accounted for around4.80% of the corporation’s complete stockheld.
MongoDB Inc., based in the United States, operates as a provider of general-purpose database platforms to clients worldwide. The company offers various products such as MongoDB Atlas and MongoDB Enterprise Advanced to cater to different business needs.
MongoDB Atlas is a hosted multi-cloud database-as-a-service solution that enables users to access databases from various cloud platforms seamlessly. Meanwhile, MongoDB Enterprise Advanced caters specifically to enterprise customers who require commercial database servers that can operate in multiple environments – cloud-based, on-premise, or hybrid solutions.
In addition to its paid offerings, MongoDB also provides Community Server – a free-to-download version of its database platform designed specifically with developers in mind. This version includes essential functionality required for developers exploring their initial steps into implementing MongoDB technology.
Recently, several analysts have commented on the stock’s performance and provided recommendations for potential investors. KeyCorp analysts boosted their target price from $372 to $462 while issuing an “overweight” rating for the stock back on July 21st. Truist Financial followed suit by raising their target price from $420 to $430 and also giving the stock a “buy” rating.
Capital One Financial began coverage on MongoDB stock on June 26th, providing an “equal weight” rating alongside a target price of $396. Meanwhile, Stifel Nicolaus analysts increased their target price from $420 to $450, giving the stock a “buy” rating. Lastly, Barclays analysts increased their target price from $421 to $450 and also provided an “overweight” rating for the stock on Friday.
According to Bloomberg data, one research analyst has assigned a sell rating for MongoDB’s stock, three have given it a hold rating, and twenty have recommended buying shares in the company.
Based on these various assessments and recommendations by experts and financial institutions alike, MongoDB Inc. appears to be experiencing favorable attention in terms of its investment potential. While individual investors should conduct further research into the company, its offerings, and overall market conditions that may affect the stock’s performance; this collection of insights serves as an important starting point for anyone considering investment opportunities within this industry.
For updated analysis regarding MongoDB Inc.’s stock performance or more comprehensive information derived through detailed research findings it is suggested readers keep in mind regular updates published by reputable financial publications or consult with certified finance professionals prior to
Article originally posted on mongodb google news. Visit mongodb google news
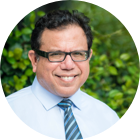
MMS • RSS
Posted on nosqlgooglealerts. Visit nosqlgooglealerts
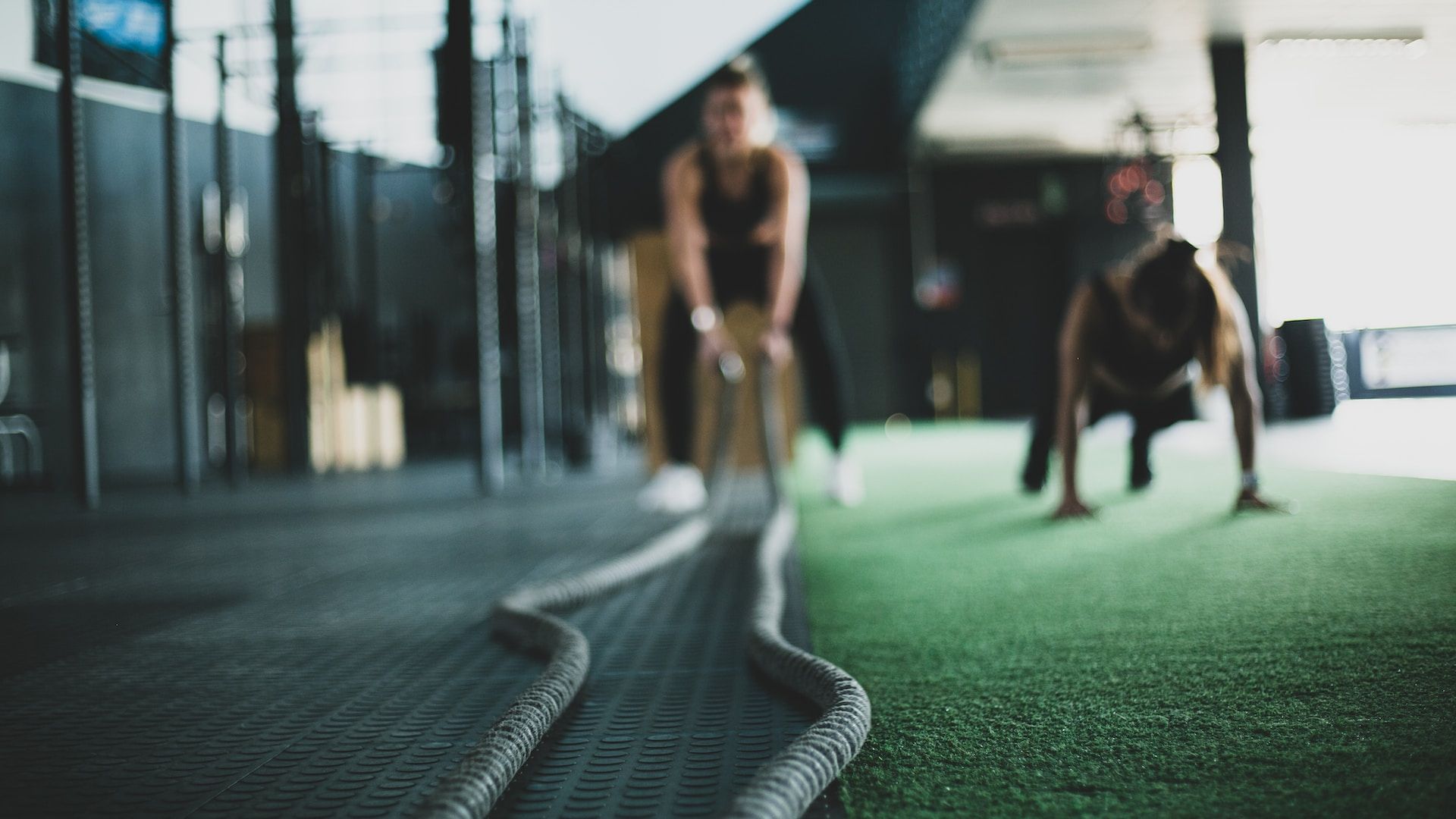
Whenever you spin up a server, it inevitably leads to the consumption of fossil fuels for its power source—and you will get a bill for it, regardless of whether it’s discounted by a hyperscaler or not, writes
IT bloat results in financial expenses and a surge in electricity usage—and given how few ‘Green’ data centres there are on the power grid, it poses a threat to the environment. As any diligent CIO will always seek to rationalise and optimise their infrastructure, what measures can be taken here?
Fortunately, there is hope for rebalancing in the form of a bit of rethinking by developers and adopting more efficient software architectures in the cloud. By slimming the need for so many servers—and so trimming electricity consumption—we genuinely can make a difference to our power consumption profile. Given that enterprise IT alone accounts for 1.5% of the planet’s total energy consumption (even discounting deliberately wasteful fringe IT activity like Bitcoin mining), such savings might soon be very noticeable for both your company’s bottom line and the climate’s long-term health.
Thankfully, there is an opportunity to restore balance through a return to some good computer science basics and an examination of the basic data structures used to attack the problem at hand. An excellent illustration of this is how Adobe eventually discovered a highly-tuned way to deliver a popular online service.
Fewer database nodes, but the size of the dataset had more than doubled
Adobe offers a platform called Behance, launched in 2005, which allows individuals to showcase and discover creative work in a social media-like environment. Behance is a way for millions of artists to quickly upload and share their projects, with over 24 million members as of October 2020.
Behance became part of Adobe in 2012, and work started on replatforming. This commenced with a shift to NoSQL document-oriented database MongoDB for introducing the activity feed.
Initially, the configuration for Behance consisted of 125 nodes in a dataset of 20 terabytes. The Adobe IT team tasked to look after Behance recognised the need for optimisation and conducted a second port. They decided to stick with the NoSQL approach, but this time opted for Cassandra, a wide-column store NoSQL database management system.
Over time, several improvement projects were conducted to enhance the delivery of the platform. Three years later, the service was successfully running on fewer database nodes, specifically 48. However, the size of the dataset needed to power the platform had more than doubled to 50 terabytes.
The graph alternative
Users liked a lot of the features, but performance was slow. A third migration was initiated, leading to the adoption of a significantly different database approach, that of graph database technology. And this step proved to be highly successful, serving as the current home for the system. It also exemplifies the benefits of some hard thinking about what the optimum foundation for a computationally parsimonious SaaS app might be.
As a result, Behance using graph technology runs perfectly seamlessly for its millions of users using just three database nodes and maintaining a dataset size of 30 to 50 gigabytes—a thousandth of the previous storage requirements. In fact, the system’s efficiency goes beyond that, as each node has eight cores, two terabyte SSDs, a mere 118 gigabytes of RAM per instance, a 50-gigabyte page cache allocation, and a 15-gigabyte heap. This all adds up to a much smaller data centre footprint than the two previous iterations.
It’s a fascinating odyssey, I’m sure you’ll agree – filled with choices that presented their own set of advantages and challenges at each stage. With Mongo, for example, despite being a highly flexible option, database reads were slow because connections had to be computed at high cost along the way. However, with the transition to Cassandra, the slow read problem was solved by introducing something called ‘fanouts’, a popular workaround commonly employed in social feed systems.
Indeed, as the number of users and data size continued to grow, this approach started to become an issue as it put a large overhead on the system’s web infrastructure. After all, a popular Behance poster with 10,000 followers would require 10,000 individual database writes to publish a new project on each follower’s Behance feed.
That functionality is actually central to the user experience: Adobe calls it ‘Activity Feed’, something that enables users to follow their favourite creatives and curate galleries based on preferences. When a user follows a creative, they receive alerts and an updated feed whenever that creative logs an activity within the app.
The move to the Cassandra version did prove to be an improvement compared to the Mongo iteration. However, keeping the Cassandra version going required much attention and management from the Adobe ops team.
But upon deeper consideration of the application’s nature, it’s clear that Behance functions as a social network where people follow certain individuals. By adopting a graph database, that inherently focuses on relationships instead of other data structures, the fanout model required by Cassandra became redundant overnight. This was a big contributor to the huge reduction of the dataset size. Losing the fanout model allowed Adobe to deliver the activity feed users wanted to see in under one second, compared to the potential four-second delay previously experienced.
The best way to solve your programming problem
I am not saying that every problem can be rewritten and optimised with graphs.
On the other hand, it’s also true that not every IT problem necessitates the utilization of extensive resources like 1,000 servers and Apache Spark.
So let’s get back to basics and think about the most efficient way to solve a problem resource-wise, not just with cloud brute force. Doing so will potentially lead to cost savings and reduced environmental impact.
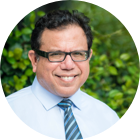
MMS • Sherin Thomas Roland Meertens Daniel Dominguez Anthony Alfor
Article originally posted on InfoQ. Visit InfoQ
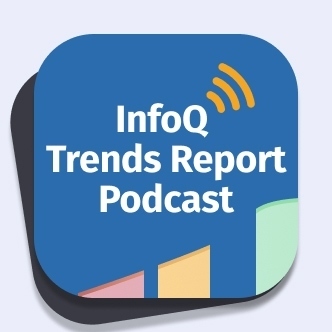
Subscribe on:
Introduction
Srini Penchikala: Hey, folks. Before we get into today’s podcast, I wanted to share that InfoQ’s International Software Development Conference, QCon, will be back in San Francisco from October 2nd through 6th. QCon will share real-world technical talks from innovative senior software development practitioners on applying emerging patterns and practices to address current challenges. Learn more about the conference at qconsf.com. We hope to see you there.
Hello everyone. Welcome to this podcast. Greetings from the InfoQ, the AIML and the data engineering team and their special guest. We are recording our podcast for the 2023 trends report. This podcast is part of our annual report to share with our listeners what’s happening in the AIML and data engineering space. My name is Srini Penchikala. I serve the team as the lead editor for the AIML and data engineering community at InfoQ. I’ll be facilitating our conversation today. We have an excellent panel for today’s podcast with subject matter experts and practitioners in the AI/ML and data engineering areas.
Let’s first start with their introductions. I will go around our virtual room and ask the panelists to introduce themselves. We will start with our special guest, Sherin Thomas. Hi, Sherin. Thank you very much for joining us and taking part in this podcast. Would you like to introduce yourself and tell our listeners what you’ve been working on?
Sherin Thomas: Hey folks, thank you so much for inviting me. I’m so excited to be here. I’m Sherin. I’m a staff engineer at a FinTech company called Chime. I’m based in San Francisco. Before this, I spent a little bit of time at Netflix. Before that, Lyft, Twitter, Google, and for the last six years or so I’ve been building data platforms, data infrastructure, and I have a keen interest in streaming. Been active in the Flink community and very recently have also been thinking a lot about data discoverability, governance, operations, and the role data plays in new advancements in AI. In my free time, I have been advising nonprofits working in the climate change area, basically helping them architect their software stack and so on. So yeah, again, very excited. Thank you. Thank you so much for inviting me.
Srini Penchikala: Thank you. Next up, Roland.
Roland Meertens: Hey, yes, my name is Roland Meertens. I am working at a company called Bumble, which is making dating apps. So I am literally a date and data scientist and I’m mostly working with computer vision. So that’s my background.
Srini Penchikala: Thank you, Roland. Daniel?
Daniel Dominguez: Hi everyone. Glad to be here for another year. I am Daniel. I’m an engineer. I have experience in software product development. I have been working with companies from Silicon Valley startups to Fortune 500. I am AWS community builder on machine learning as well. And my current company, we’re developing artificial intelligence and machine learning products for different industries.
Srini Penchikala: Thank you. And Anthony?
Anthony Alford: Hi, I’m Anthony Alford. I’m a director of development at Genesys where we make cloud-based customer experience and contact center software. In terms of AI, I’ve done several projects there, customer experience related, and back in the 20th century I actually studied robotics in graduate school and did intelligent robot software control. So I’m really excited to talk about some of the advancements there today.
Generative AI [03:22]
Srini Penchikala: Thanks Anthony. Thank you everybody. Welcome to this podcast. I am looking forward to speaking with you about what’s happening in the AIML engineering, and maybe I should say what’s not happening. So where we currently are and more importantly, what’s going to be coming up that our listeners should be aware of and should be keeping an eye on. So before we go to the main podcast discussion, a quick housekeeping information for our listeners. There are two major components to these trends reports. The first part is this podcast, which is an opportunity for you to listen to the panel of expert practitioners on how the new and innovative technologies are disrupting the industry and how you can probably leverage them in your own applications.
The second part of the trends report is a written article which will be available on InfoQ website. It’ll contain the trends graph, which is one of my favorite content on the website. This trend graph will highlight the different phases of technology adoption and it also provides more details on individual technologies that have been added or updated since last year’s podcast. So I recommend everyone to check out the article, as well as of course this podcast, when the article is published. Back to the podcast discussion. There are so many interesting topics to talk about, but let’s start with the big elephant in the AIML technology space. That is the generative AI or also known as GenAI, a large language model, the LLMs, which leads GenAI is based out of, and of course the ChatGPT. Who hasn’t heard about ChatGPT? So generative AI has been getting a lot of attention since GPT-3 was announced a couple of years ago, and especially since GPT-4 and ChatGPT came out earlier this year.
So ChatGPT was used by 170 plus million people in the first two months of its release. So it has taken over pretty much every other technology and solution in terms of faster adoption. So all the big players in this space have announced their plans for GenAI. We know that ChatGPT is from OpenAI. Other than that, Google also announced Bard data AI solution and Meta AI released the LLaMA 1 and LLaMA 2. So there’s a lot of activity happening in this space. So in this podcast we want to highlight the value and benefits these technologies bring to the users, not just all the hype that’s out there.
So Anthony and Roland, I know you both have been working on or focusing on these topics. Anthony, would you like to kick off the discussion on GenAI and how it’s taking the industry by the storm? Also, for our listeners who are new to this, can you start by defining what is generative AI, what is an LLM and why they’re different from the traditional machine learning and deep learning techniques?
Anthony Alford: I actually was trying to think what is a good definition of generative AI? I don’t think I have one. I don’t know. Roland, do you have a good definition?
Roland Meertens: No, I was actually surprised because I was at a conference about AI a couple of weeks ago and they were running a parallel session. They were running a separate conference on generative AI. And I was surprised because I love the field. I’ve been giving talks about generative AI for years now, and I just didn’t know it was such a big topic that it would warrant its own separate conference. So I think that’s what people normally nowadays use as generative AI, is just all AI-based on auto completing a certain prompt. So you start with a certain input and you just see what AI makes up with this. And this is super powerful in terms of you can do zero-shot learning, you can take your data and turn it into actionable written out language. There’s so many options you have here. Yeah, so I think that’s what I would take as a definition.
Anthony Alford: What’s interesting, we kind of think of this as new, but looking back, it didn’t start that recently. Generative adversarial network models, or GANs, for image generation have been around for almost 10 years and it seems like the language models just all of a sudden caught fire. I think probably in 2019 when GPT-2 came out and OpenAI said, “We’re not going to release this to the general public, because it’s too dangerous.” Now, they changed their tune on that, but 2020 was GPT-3 and 2021 was DALL-E, a different kind of image generator, but I think just the second half of 2020 things just took off. And so we’re talking about large language models a lot, but the image ones were hotter in the summer of 2022, the Stable Diffusion. And so we’ve got both now. We’ve got the generative AI for images, we’ve got generative AI for text. People are putting them together and having ChatGPT create the prompts for the image generation to tell a story and illustrate it. So we’re starting to see humans cut out of the loop there altogether. So what are your thoughts on what’s next?
Roland Meertens: Yeah, from my side, what I noticed is this massive improvements in the generation of the text itself. So ChatGPT really took a step up with GPT-3.5 and GPT-4 is, for me, in a whole different ballpark. You can very clearly see amazing improvements for the generation itself. Again, it’s just larger networks, more data, higher quality data, and I was kind of amazed that everybody now started adopting it. And because we talked about this technology last year, a year ago when it was not such a big thing, and already on a very good level and very usable. And I think what we can see is that with ChatGPT, the usability experience makes that now everybody is using it, including my father. He’s a big adopter of ChatGPT and he is not a technical person. He emailed me because his URL I bookmarked for him broke.
But so everybody can now use it. And what I find amazing about the state of the art in image generation is that for me, the clear winner at the moment is Midjourney. They have absolutely the best generated images in my opinion, but the way you work with it is that you have to type your prompts in Discord to a bot. The company, I think, only has seven employees at the moment. So there’s no usability and still it’s so good that people are happily paying their $35 a month it costs to have a subscription and are still happily working with it. So I think that in terms of trends, once we go over this hurdle of it being difficult to use, once that goes open to the general public as well, that will be an amazing revolution.
Daniel Dominguez: I think all this term that has been happening with ChatGPT, it’s amazing because as Roland said, I mean everyone is using it. For example, right now we’re doing a project internally in the company for one client and we did all of this generative AI for his own solution and I believe we use the GPT technology, we use TensorFlow, we use the DaVinci model, and at the end there the client said, “But what is the difference between this and ChatGPT?” And we said, “ChatGPT has the same technology, it’s a product, but we’re using the same technology for your own product.”
But the thing is that when you mention artificial intelligence or generative AI, ChatGPT… I mean, it’s the same that when you say you don’t surf the web, you Google it. So right now, we ChatGPT is like you need something on artificial intelligence, you use ChatGPT and that’s the magic of OpenAI, being the first one on the market doing this. And as we’re going to see there are a lot of companies and there are a lot of competence right now regarding the generative AI, but right now with ChatGPT is like the synonymous of generative AI and the thing that everybody knows how to use and anything that everybody’s using right now.
Sherin Thomas: And to me what’s interesting is how a new cottage industry of little companies built on top of generative AI are cropping up. Everything from homework assignments to code generation, all kinds of things. So for me, I’m really interested in all these creative things, creative ways people use this. So I’ll be staying tuned for that.
Roland Meertens: What I find amazing is that there are now a couple of companies, I see this happening on LinkedIn, I see this happening with my friends who are in big companies. These companies are now creating their own vision, their own roadmap for how they are going to use generative AI. And I’m so eagerly waiting to see more companies apply it because we already mentioned this last year, but the playing field is wide open for everyone who wants to use these APIs. People just need to find a way to add value to people’s lives, which goes beyond just the simple cookie cutter approach. Everyone is now getting with ChatGPT, and I think people will come up with applications we cannot even dream of existing right now and it’s so easy to integrate this into your product. So I’m very excited about this. I think there’s just more room for creativity at the moment than there are technological hurdles. So yeah, I’m really hoping to see more companies experiment.
Anthony Alford: In terms of trends, what’s interesting is when GPT-3 came out, the value proposition was it does not need to be fine-tuned, it works great with just in context learning. But now we’re starting to see, with these large language models in particular, people are fine-tuning them, especially the smaller and open source ones like LLaMA, people are using those, they’re fine-tuning those. We’re starting to see things like with Google’s PaLM model, they did their own fine-tuning, they created a version for medicine, they created a version for cybersecurity. So they’re fine-tuning for specific domains. It’s getting easier to do because the models are so large, you need pretty hefty hardware to do that as well as you need a bit of a dataset. But we’re starting to see those models shrink a bit and now people have the ability to fine-tune them themselves. I don’t know if anyone has thoughts on that.
Srini Penchikala: Yeah. I just want to chime in. Anthony, I was also looking at who actually are using the companies that we know of, of ChatGPT. The list is pretty long. So it starts with Expedia, the travel services company, to Morgan Stanley to Stripe to Microsoft Slack and so on. So the adoption is increasing, but like you said, we have to see how these adoption evolves over the time. So any other trends you guys are seeing that are interesting to our business?
Prompt engineering [13:32]
Anthony Alford: I don’t know if we got into prompt engineering. Roland mentioned prompts a little bit.
Srini Penchikala: Yeah, we can go to that one. So do you have any comments on that, Anthony? On prompt engineering?
Anthony Alford: One of the most interesting developments to me was the idea of the so-called chain of thought prompting. So if researchers were using these language models, they found that if you tell it, “Explain your thoughts step-by-step,” the results came out a lot nicer. So that’s been something that was built into some of the models like PaLM where you can get a very big variation in the quality of your results based on your prompt. And it’s similar for the image generation as well. Depending on what you tell the model, you can get quite a bit of a different result.
Srini Penchikala: And also prompt engineering, the way I’m understanding it, is going to be a discipline in its own, its own way. So I’m wondering how our prompt engineering responsibility role, or whatever, our tasks will be integrated into the traditional software development process. So would we have a dedicated prompt engineer in each project to help the team with how to do things. So does anybody have any thoughts on that?
Roland Meertens: Well, maybe to go the other direction or maybe to go against what you think might be happening. I think that the miracle is that everyone is using this at the moment. So I think there will be as many prompt engineers in your team later as there are Google engineers who are helping you Google things. That would be ridiculous, right? Everybody has somehow learned how to do this. There’s no class about this in high school. I don’t think there will be a need for prompt engineering class. Everybody will know this at some point, except of course your grandmother, who by then will do the opposite thing. Instead of typing in Google, how can I bake the best cookies? And she learns the best cookie recipe, she will now go to ChatGPT and just type “best cookies” and ChatGPT has no clue what to do with it.
Srini Penchikala: I think it’ll become part of our lingo, I guess. Okay, we talked about ChatGPT mainly today, but we also mentioned LLaMA from Meta and Bard from Google and also there is another product called Claude. I haven’t done much research on this. Does anybody know how it’s different from others?
Daniel Dominguez: And also Amazon is now with Amazon Bedrock, is the bet on generative AI. So that’s also another one, which should take a look to this year, what is going to happen with that.
Srini Penchikala: Yeah, thanks Daniel. So what do you guys think about the next step for LLMs? It’s going so fast, we don’t even know where we’ll be in six months or one year.
Anthony Alford: Well, what I mentioned, the smaller models and people shrinking them and doing the LORA… I can’t remember what the A is for, but distilling and shrinking the models and especially, for example, OpenAI language models are quite famously not available for you to download. Whereas Meta has been releasing theirs, Meta has released LLaMA. Now people give them a hard time about the license. It’s a non-commercial license, but still they give you the weights and people are taking those and fine-tuning them. So we have a proliferation of these takeoff names from LLaMA like Vicuna and Alpaca and so forth. So people are fine-tuning these models. They’re smaller than ChatGPT, they’re not as good, but they’re pretty good. And so that lets companies who have concerns about using a closed API and sending data out to who knows what, that lets them alleviate that concern.
I think that’s a pretty interesting trend. I expect it will continue. Another one and then I’ll let you all chime in, is the sequence length. That’s how much history you can put into the chat. And as we know, the output of the language model is really one word, it’s one token. You give it a history, it outputs one token, the next token, then you got to put it all the way back in. They’re auto aggressive. Eventually you run out of that context, it’s called. GPT-4 has a version that supports up to 32,000 tokens, which is quite a bit. And there’s more research into supporting maybe up to a million tokens.
At that point, you could basically put Wikipedia almost as the… Maybe not, but you could put a book as the context and that’s the key for this so-called in-context learning. You could give it a whole book and have it summarize, answer questions. You could give it your company’s knowledge base and you could ask questions on it. So I think these two trends are going to help bring these models and their abilities into the enterprise, into premise maybe, whatever you want to call it, basically out of the walled garden.
Srini Penchikala: I can definitely see that changing the adoption in a positive way. So if companies can use some of the solutions within their own environments, can be on-premise or can be in the cloud, but with more flexibility, that will change the name of the game as well.
Sherin Thomas: So speaking about summarizing, this is a trend that I’m seeing quite a bit. A lot of law firms are using this to summarize legal documents and then I’ve been working with a group called Collaborative Earth where scientists are looking at papers and they’re like 30,000 papers and they need to understand, some pull tags out of this. So that’s another area where I see a lot of application of this and people are onboarding this trend of summarizing papers and documents.
Srini Penchikala: Thanks, Anthony. Thanks, Sherin. The other one I know we can talk about is a speech synthesis. So how can we use these solutions for analyzing the speech data? So, Anthony, do you have some thoughts on this?
Anthony Alford: What’s interesting is that both Google and Meta seem to be working quite hard on this. They’ve both released several different models just this year. Of course OpenAI released Whisper at the end of last year and they actually did basically open source that and Whisper is quite good for speech synthesis. Meta and Google, they’re doing multilingual things in particular. So Google is doing speech-to-speech translation. So it does the speech recognition of one language and does the output of it in speech synthesis in another language. In my industry in particular, people are excited about that because you could have an agent on the phone with a customer, maybe they don’t speak the same language, but with this in the middle it’s like the Hitchhiker’s guide, right? It’s the thing in the ear that can do automatic translation for you. That’s pretty exciting.
The other one recently that came out with Meta, released one called Voicebox and it basically does… In images, we’d call it in-painting, but basically it can take a speech audio and kind of replace bits of it. So it could take a podcast like this and edit out a barking dog. It could change what I say from saying, “I love AI,” to, “I don’t like AI,” or something like that. So they’re in the situation OpenAI was in where they’re not sure they want to release this because they’re not sure how it could be abused. So if you guys hear me say something that you don’t think I would say, well, blame AI.
Srini Penchikala: Oh, that’s going to add that new dimension to the deep fake, right?
Anthony Alford: Exactly. It’s literally what it is. Yeah.
Srini Penchikala: Definitely. I know it kind of brings a lot of ethical and responsible AI consequences. Anthony, we’ll go to the topic later in the podcast, but it’s a good one to keep in mind. Anybody else have any comments on the speech side of the AI?
Daniel Dominguez: Yes, I remember that I wrote an article on that on InfoQ with the update of the Google AI regarding the universal speech model, which has the 1000 language initiative. It’s going to be huge, all of these things happening and obviously for all the prompts that are also involved with speech, for example the Google products or Alexa, all that stuff, there’s going to be a whole new way of prompts with all artificial intelligence or all these models once they’re implemented on their own hardware and on their product. So it’s going to be amazing to see what is going to happen. Now asking Alexa or now asking Google to give better insights of their answers based on the prompts, on the voice that were giving on those prompts. So that’s something that eventually is going to have a lot of improvement on the prompt side for these companies.
Srini Penchikala: So with all this innovation happening with text-to-data, speech and images, so with large language models, billions of parameters. So once we start seeing a lot of this adoption and these applications, the enterprise applications are deployed in production, whether they’re on-premise or in the cloud, one big thing that teams will need to own and maintain will be the operation side. So there’s this new LLMOps term coming up. So what does operations mean for LLM-based applications? Sherin, I know you have some thoughts on this, so please go ahead and share with us what you think what’s happening here.
Sherin Thomas: The MLOps kind of brings rigor to the whole process of building and launching pipelines. So in that sense, those same operational requirements apply to LLMs as well. But I see that there are some nuances or some requirements for LLMs that make that a little bit more challenging or we need to think a little bit differently about operationalizing LLM. So I guess one is maybe around collecting human feedback for reinforcement learning, maybe prompt engineering as we discussed. That is going to be a big piece that will be coming up.
And then also performance metrics for LLMs are kind of different and this is a very constantly emerging area right now, so we don’t even know how this is going to pan out in the future. So that might completely differ. Also, the whole LLM development life cycle consists of the data ingestion, data prep, and prompt engineering. There may be some complex tasks of chaining LLM calls that make also external calls, like a knowledge base, to answer-question-answer. So this whole life cycle requires some rigor. And from that sense, I feel like LLMOps might just end up being its own little thing with MLOps being just like a subset of it.
Srini Penchikala: Yeah, definitely. I think that will become more critical when we have a significant number of apps using these LLM languages. So does anybody else have any other thoughts on that? What LLMOps should look like?
Daniel Dominguez: I think definitely that’s something that is going to be increasing in the industry and in the companies because for example, right now with the clients that we’re working is like, but it’s artificial intelligence, so that’s done. That’s an artificial intelligence problem or whatever. But even though you have to consider that behind that artificial intelligence, you have a team, you have to tune out the data, you have to work continually in the prompt engineering, you have to continually see what is happening on the server on those architecture and that stuff. So it’s not thinking that the artificial intelligence is there and is doing everything. No, behind that artificial intelligence there will be a team between all this stuff to make sure that generative AI should work correctly and that generative AI will work correctly. There should be a team behind making sure that all these things happening out of the space, are working correctly.
Vector search databases [24:13]
Srini Penchikala: Right. Thanks, Daniel. Thanks, Sherin. Yeah, we can switch our focus here a little bit. The other area that’s getting a lot of attention is the vector database technology, the embedded stores. So I have seen a lot of use cases on this. One of them, interestingly, is using the sentence embedding approach to create an observability solution for generative AI applications. So Roland, do you have any thoughts on this? I think you mentioned this in the past vector databases.
Roland Meertens: Yes, maybe let’s first start with the question, why do you need a vector search database? As we already mentioned at the moment, these large language models have a limited history of, I heard Anthony say, 33,000 tokens. I mean that’s about half a podcast, maybe a whole podcast. But what if you want to know something about Wikipedia, or I heard Sherin say, but if you have a lot of legal documents, one thing which I think will happen more and more with companies is that they can make a summary of a certain document that will be stored as a certain feature vector. So like you and I, we just write down this document is about X and Y. But of course large language models can just create a feature vector and that will maybe leave you with thousands, millions, hundreds of millions depending on how many documents you have of feature vectors.
And if you want to find similar vectors, or maybe you can query your large language model with, “Hey, I am searching for this document which probably contains this.” You can find a similar feature vector inside these vector databases. So where with normal databases, you’re just going through all the documents and finding what is the most relevant documents, but once you have too many documents, which are all summarized features, you want to have a vector search database where you can find the nearest neighbors of the thing you’re searching for.
And what I find interesting about this or what intrigued me as a trend over the last year is that I saw a tiny increase in adoption from developer’s perspective, which is good because these things are amazing, but I saw a massive increase in funding for these technologies. So in this case it looks like investors have rightfully realized that vector databases are going to be a big part of the future and somehow developers are kind of lagging behind. It’s maybe a difficult topic to get into. So I really think that next year we are going to see more adoption. We are going to see that more people will realize that they have to work with a vector search database such as Pinecone or Milvus. So I think that these technologies will keep growing.
Srini Penchikala: Roland, I also heard about Chroma, I think, is the open source solution in that space.
Roland Meertens: Yeah, I mean maybe we can have a dedicated podcast and interview someone who is working on these technologies. I think the bottom line is that depending on what is in your feature vectors, some things make more sense than others. So depending on what kind of a hyperdimensional space you’re searching in, do you have lots of similar data? Do you have data over the place? You want to use one version or another one.
Srini Penchikala: Make sense. Yeah, definitely something to keep in mind. And a feature stores have definitely become a big part of the machine learning solutions. This one now probably will have the same importance. Anybody else have any thoughts on that? Vector databases, it’s still an emerging area.
Sherin Thomas: I think, again, the applications of similarity search are also going up. So a couple of years ago I was working on a site project with NASA FDL where they wanted to ingest… Basically they applied self supervised learning on all the images of the earth collected from NASA satellites. And when scientists are searching for weather phenomena like hurricanes and things like that, they want to search other images of hurricanes that happened over time and that’s a problem that they’re still trying to solve. And that was two, three years ago, we tried using Pinecone AI and now I’ve seen that these technologies have really developed, rapid improvement, in the last two years and at that time it wasn’t there yet. So yeah, this is an amazing space as well.
Robotics and drone technologies [28:11]
Srini Penchikala: Let’s switch to the next topic, which is also very interesting. So the robotics and the drone technologies. Roland, I know you have recently published a podcast from the ICRA conference. A good delivery, a good podcast, a lot of great information. So would you like to lead the discussion on this and where we are with robotics and drone technologies and what’s happening in this space?
Roland Meertens: Yeah, absolutely. From my side, I was super excited to go to this ICRA conference and see what’s happening here, so we have a separate podcast on this. And one thing which I think we see as a trend overall in the entire tech industry, is that we see less investments also with robotics, which always makes me sad because I think that robotics is a very promising field, but it always needs a lot of money. We do see that Boston Dynamics has started an AI Institute, so that seems very promising and we do see cheaper and cheaper remote control robots, besides Boston Dynamics who are still leading their leg robot race, but a couple of years ago it was unthinkable that you could buy a legged balancing robots who could walk over some unstable terrain. And nowadays, I think the cheapest ones you can probably get for only $1,500.
So it’s getting more and more viable to buy a robot as a platform and then integrate with that API to put your own hardware on top of it. So yeah, hopefully we can soon see computers go to places where they have not gone before. And yeah, the robot operating system is still seen as leading software with more and more adoption to ROS 2. I also have seen one company, VIAM, which has also started to build a bit of a middleware where you can easily add some plugins and configure some plugins. So that’s exciting. Overall, it’s an interesting field with lots of developments, which is always kind of slowly, invisibly moving in the background. Yeah, super exciting.
Anthony Alford: What’s interesting to me is how Google in particular has been publishing research where they’re taking language models and using them to control robots. They’re basically using it as the user interface. So instead of having a planner, you tell the language model, “Go get me a Coke.” And it uses that chain of thought prompting to do step-by-step, down to basically the robot primitive of, “Drive here, pick up here, come back.” I think that’s a pretty interesting development, as well, especially considering how hard that was for us back in the nineties. Google’s also trying to integrate sensor data into this. I’m not sure why Google is doing robotics research, but they are doing a lot of it and it’s very interesting. I don’t know if they had any posters at ICRA or anything like that, Roland.
Roland Meertens: No, but I’m also very interested in this topic. You indeed see that, for example, for planners in the past you had to say on a map, “Here is a fridge,” and you had to say on a map, “Here’s the couch.” So if you have to bring someone a beer, you have to program the commands, walk to the fridge, walk to the couch. But now with these large language models and also with large computer vision models, why not recognize where the fridge is and remember that on some kind of semantic map. And if someone describes it in a different way, why not try to figure out what they could mean? And I think that’s very exciting because it’s definitely traditionally a very hard world to work in. So I’m very excited to see where we can go next in that domain.
Srini Penchikala: Yes, definitely. I think the one area that can definitely use this, unless it’s already using some of this is manufacturing. So there is the virtual manufacturing platforms, there is the digital twins idea. So definitely I think we can bring the physical manufacturing plan closer to the virtual side and try to do a lot of these things that we can not afford to do in the physical space because of the cost or the safety and try it out with the virtual and semi-virtual, with the drones and the robots.
Daniel Dominguez: But I think with robot technology it is going to happen the same that happened with other industries that, I mean everybody knows that there is a lot of research and cool stuff happening, but there’s not a real product that people can see that robot is there. So probably in the next year. For example Tesla, which is doing with the Optimus robot, a humanoid, that’s going to happen. That we’re going to start seeing robots more approachable as products and not only as research. So I think with all this advance and the things that are happening, we are years ahead of seeing physical robots on the streets.
Ethical AI [32:29]
Srini Penchikala: Makes sense. Let’s jump to that, another big item here. So with all this power of technologies comes the responsibility of being ethical and being fair. So let’s get into the whole ethical dimension of these AI/ML technologies. So I know we hear about the bias, the AI hallucinations, the LLM attacks. There’s so many different ways these things can go bad. So Daniel, you mentioned about the regulations, how the governments are trying to keep a check on this. So what do you think is happening there and what else should happen?
Daniel Dominguez: I think obviously as we talked last time, the technology is really cool, but once we know what is happening with all this cool stuff, we need to consider the other aspect. And that’s where AI ethics is very important. So for example, you need to consider all the biases and discriminations. So how the AI algorithms can perpetrate biases and discriminations on decision making, we need to take into consideration privacy and security. So what is the potential risk to personal data and privacy in the need of the office security systems? We need to consider ethical decisions. So how can we ensure that AI systems make ethical decisions and accurate information? We need to consider all this unemployment and economic impact that is going to happen, which AI is the potential displacement of jobs and economics regarding the AI adoption. And we need to consider sustainability because obviously addressing all this environmental impact in the long-term with AI technology is going to have an implications.
I think right now the governments in different parts of the world are thinking of their own solutions. I don’t know from my personal perspective, if that’s something that is going to work because I mean the AI is something that is going to affect the entire humanity, and it’s not only the governments and their citizens in different locations. So probably the things that the United States is thinking regarding the artificial intelligence regulations are different based on the United Kingdom, are different based in Europe, different in Latin America, different in Asia, different in Japan. But probably at the end it’s going to be something that the entire United Nations is going to take care of what is going to happen with humanity because this is going to affect the entire humanity and not only the citizens of the different countries. So I think we’re just starting to see governments taking care and thinking about this responsibility and the regulation, but at the end it’s something that needs to be done by the entire humanity.
Srini Penchikala: Roland, I know you had a podcast on this topic with Mehrnoosh, right? So do you want to mention that? What were the takeaways from that?
Roland Meertens: Yes, indeed. So from my perspective, I can really recommend the InfoQ podcast episode with Mehrnoosh Sameki, who I interviewed at InfoQ here in London. And personally from my perspective, what I find so interesting is that everybody agrees that safety is an important topic in generative AI. But on the other hand, people are also complaining about ChatGPT becoming dumber and dumber where people say, “Hey, a couple of months ago it answered this query about medications for me,” or, “It answered this query about acting as my psychologist and now it refuses to do this. It refuses to do my homework. I don’t know what.”
And I think this is very interesting that I’m also very torn between, whoa, this technology is moving fast, we need to put a hold on it. But then also I’m also very done with the mandatory starting with, “Hey, as an AI language model, I cannot do this for you.” I know you can, I just want some information, I don’t want to listen to you. So I think there’s an interesting thing which we will definitely see as a trend this year that people will start discussing this more and more.
Anthony Alford: I don’t know, that’s almost as annoying as having to accept or reject cookies on every site. You mentioned ChatGPTs output. These companies that are serving these language models are of course extremely concerned. The models often say things that are just not true. Nobody knows what to do about that. They also can say things that are very hurtful or possibly even criminal. They’re trying to put safeguards in there, but they’re also really not sure how to guarantee that. I think this is a problem that nobody’s really sure how to solve and it looks like it’s going to get worse.
I just had an InfoQ news piece where a research team figured out how they could automatically generate these attacks. They could basically automatically come up with prompts for so-called jailbreaks, not just ChatGPT, but basically any language model. So in a way, obviously when you do research like this, it’s like white hat hacking. You’re trying to show that there’s a problem so that hopefully people will work on it. We’ll see… Like Roland said, it’s already kind of a problem and I think it may just get worse.
Roland Meertens: Maybe from my perspective, the two things I want to make sure that this is takeaway from the podcast, is I think my two tips are that it’s important as we improve the lives of all the people and all your users. So don’t just say, oh, but it works for a couple of specific users or it works for me. I think it’s always important to make sure that it really works for… Consider all the possible edge cases you can have on your platform and also consider everything which ChatGPT can do for you. So consider both the false positives but also the false negatives. So this needs to be more ingrained in the minds of people because if you start using ChatGPT for the first 10 minutes, you are of course amazed by all the things it can do. And only if you start digging a bit, you find some false positive cases, you find some false negative cases.
If you are creating a new application which you roll out to the entire world, there will be a lot of false positives and false negatives. So in that sense, of course, it’s important to remind users that this was generated by a large language model and maybe it cannot do all the things for you because at some point you’re getting into the more dangerous iffy waters of what you want a large language model to show for you.
Srini Penchikala: Yes, it makes sense. So discrimination even for one demography or one user is not acceptable in some use cases. The other similar topic here is the explainable AI. Sherin, would you like to talk about this a little bit? So what is the explainable AI for somebody who’s new to this and what’s happening in this space?
Sherin Thomas: So explainable AI in a gist is basically a way to explain how a model came to a result or a conclusion. So it could be like what were the data points it used or how did it make this decision, et cetera. And I think this is going to take center stage and become really important as we are talking about ethics of AI, and as Daniel mentioned, how a lot of governments are making regulations, discussing new laws. And when Roland talked about how models sometimes are getting dumber and we want to know why it is doing what it’s doing. And the way I see this might play out, a few years ago we saw a big disruption in data governance and data privacy automation as a result of GDPR and CCP and those laws coming into the picture. And I see that push happening on the AI explainability side as well.
And moreover, we’ve already seen some AI failures because of bad data. Famously there was a… I don’t know if you’ve heard about this. A few years ago, Amazon, I think they were using a model to make decisions to interview people and it disproportionately selected more men because it was trained on last 10 years of data, which was disproportionately men’s resumes that were coming to Amazon. So things like that. So yeah. So I feel like in this new world of AI explainability, data discovery, lineage, labeling operation, and good model development practices are all going to become super important.
Data Engineering [40:10]
Srini Penchikala: So we can do a quick transition here and talk about some data engineering topics as well, similar to how AIML space has been going through a significant number of developments and innovations. A lot of emerging trends and patterns are happening in the data engineering space as well. So let’s look at some of these important topics that our leaders should be looking at in their data management projects. Sherin, I know you have done a lot of work on this and you are probably the expert on these topics in this group. Would you like to talk about what do you see happening in the data side, whether it’s data mesh or data observability or even data discovery and data contracts?
Sherin Thomas: A few trends that I’m noticing. One, is there is a lot of emphasis on speed and low latency. So earlier most data organizations were batch first and streaming maybe like 10% of use cases would be like streaming. But now that piece of the pie is increasing. There are a lot of unified batch and streaming platforms coming out. Car part architecture is gaining adoption. Then data mesh has been a buzzword. As data is increasing and organizations are getting more complex, now it’s no longer sufficient for just a central data team to manage everybody’s use cases and data mesh came out of that need. Another buzzword that I’m hearing is data contracts. So there is a lot of emphasis on measuring data quality, having data observability, and this is just going to become more and more important with this whole new world of AI that we are entering.
Srini Penchikala: What do you think about data observability? It is definitely becoming a main pattern in the data side. I recently hosted a webinar on data observability and I learned that it is not what it was a few years ago.
Sherin Thomas: So earlier when we used to talk about observability, it was mostly around measuring observability at the systems and infrastructure level. But as we are adding more and more abstractions, so now we talk about data products as an abstraction, and then on top of data products we have a machine learning pipeline. That is another abstraction. So now it’s no longer sufficient to have observability just at the systems and infrastructure level. We need observability at those different abstraction layers as well. As I mentioned earlier, data contracts is a theme that I’m hearing a lot where with data teams getting more and more distributed and with a lot of actors being involved in a whole full life cycle of data ingestion and processing and serving and all of that, it makes sense to have contracts across those boundaries to make sure that it’s almost like unit tests. Make sure that systems and data products are behaving as expected.
I also notice a lot of companies coming up in this space, so Monte Carlo, Anomalo, and one person that I follow, Chad Sanderson, he has a lot of great opinions about the subject. So I encourage you to follow him on LinkedIn or I think he has a blog as well. And I see with AI the whole need for data observability is just going to increase. And we talked about AI explainability earlier. So now we want to know what kind of data we are getting, what is the distribution, all sorts of things. And we have heard so many stories of AI failing because of data. So the whole Zillow debacle, and I already spoke about the Amazon recruitment model thing. So now the data observability is also around what type of data or what distribution of data is coming in. It’s not just system info.
Srini Penchikala: Definitely. I see the data disciplines that you discussed under the AI side we talked about are basically two sides of the same coin.
Sherin Thomas: Yes.
Predictions for the next year of AI [43:50]
Srini Penchikala: You need to have all of this in place to have an end-to-end data pipelines to manage the data and the machine learning models. I think we can wrap up with that discussion. I think we have talked about all the significant innovations happening in our space, so we can go into the wrap up. So I have two questions so you can answer both the questions or pick one question to wrap up. And then we will go to the concluding remarks. So the two questions are, what is your one prediction in AI or data engineering space that may happen in one year? So when we do this podcast next year, we will actually come back and see if that prediction has come true or not. We will start with Sherin.
Sherin Thomas: I think, I’m noticing that a lot of companies are feeling that data teams are becoming a bottleneck. So I see data mesh adoption going up in the coming year. So that, and the second part is around explainability. I think that is also an emerging topic and I think there might be a lot more adoption in that area.
Srini Penchikala: Okay. Anthony?
Anthony Alford: Predictions are hard. Can I make it a negative prediction? I predict we will not have artificial general intelligence. I know people think maybe the LLMs are a step on that. Certainly not next year. I’d be surprised if it happens in my lifetime to be honest. But I’m over the hill, so maybe we’ll see. You never know.
Srini Penchikala: AGI, right?
Anthony Alford: Yeah, I know that was not a very brave prediction, but I’m going to predict, no.
Srini Penchikala: It’s good to know what’s not going to happen. Daniel?
Daniel Dominguez: I think AI is here to stay. I think this year we saw with ChatGPT and all these new things happening and focusing on the product base also where people can start using it and massifying all this technology, AI is here to stay. Probably, I would say by next year there’s going to be more new cool products, more new cool stuff to use. I know, for example, Elon Musk is going to start working on artificial intelligence in many of his own companies. So there are going to be more and more approaches to the artificial intelligence for normal people and not only for the research and for the things that we were used to do, which was to read research on papers and all that stuff. But now the artificial intelligence is going to be on more and more products that people are going to start using it more and more.
Srini Penchikala: Roland.
Roland Meertens: So from my side, the one thing I am personally very excited about, is the field of autonomous agents. So right now you are taking the API of OpenAI or whatever and you have to feed it prompts and then you have to connect it to these other APIs. What I’m really excited about are these autonomous agents where you simply say, “Come up with an interesting product to sell,” and then the autonomous agent will by itself start looking at what’s a good product to make. And then it’ll autonomously email some marketing companies saying, “Hey, can you help me market my new product?” And it will automatically email some factories saying, “Hey, can I get this?”
And I think it’ll be super powerful if you could have maybe a couple of basic things in a year connected to this. So maybe I could say I want to go to a romantic restaurant in this city where I’m traveling to, and then it’ll automatically start finding a couple of romantic restaurants, read up on what’s the most romantic restaurant is, and then also on my name, on my behalf, using my Gmail email the restaurant owner asking, “Hey, can I have a table?” And then the date. I think that would be amazing, these autonomous agents.
Anthony Alford: If I could outsource buying Valentine’s gifts and so forth, sign me up.
Srini Penchikala: Well Anthony, I think Roland is in the dating app development business.
Anthony Alford: Oh, right.
Srini Penchikala: These are good features to add.
Anthony Alford: Roland, he didn’t do a prediction, he gave us his product roadmap.
Srini Penchikala: He’s talking about his product roadmap. Okay. Yeah, I think my prediction is I think the LLMs are going to be a little bit more, I don’t want to call mainstream, but a little bit more in the reach of the community. We heard about LangChain, which is the open source LLM framework technology. I mean, solutions like these will be more available next year, and LLMs will not be just a closed source type of solution. So okay, let’s go to the last question and then we can conclude after that. So I know ChatGPT is more powerful because of a lot of the plugins that are available there. We can start with Roland on this. So I want to ask you guys what ChatGPT plugin would you like to see added next?
Roland Meertens: I think just how right now I am not remembering things anymore, but I remember how to Google for the things I want to remember. I think the next step would be a ChatGPT plugin for my life, such as maybe starting with WhatsApp and Gmail, such as it’ll remember things for me. So it would be like a Remembrall in Harry Potter, where suddenly some ball will become red and you think, “Ah, I forgot something.” And if you’re lucky, if you upgrade to the premium version, it’ll also tell you what you forgot.
Srini Penchikala: Cool. So the basic model and the premium model. Exactly. That’ll help me out. I forget a lot of things. Okay. How about you, Anthony?
Anthony Alford: I’m kind of liking the restaurant plugin. I think, Roland, you need to get on that for me.
Srini Penchikala: Yeah, there you go. Okay. Daniel?
Daniel Dominguez: I would like to see something with a voice. For example, ask ChatGPT something and instead of typing or other stuff, just like you do with Google or Alexa, say something and the answer. And if the answer is good… For example, say, “Answer an email that I have, I need the answer,” and I just send that email without me touching the keyword. Something like that would be very nice.
Srini Penchikala: Yeah, that’ll help. Sherin, what do you think?
Sherin Thomas: I’ll give all my money to whatever plugin can make decisions for me. Just make my decision, run my life for me, and I’ll be happy. Yeah.
Srini Penchikala: I think for me, along the same lines. I would like to have a plugin that will tell me what I don’t know I don’t know. So, unknown unknowns. Okay, we can wrap it up guys. Thanks for joining in this excellent podcast and sharing your insights and predictions, what’s happening in the AI/ML space and data engineer space. To our listeners, we hope you all enjoyed this podcast. Please visit infoq.com website and download the trends report that will be available along with this podcast in the next few weeks. And I hope you join us again for another podcast episode from InfoQ. So before we wrap up, any remarks you have, we’ll start with Daniel.
Daniel Dominguez: No, I think it’s going to be very important to see what is going to happen. And as I mentioned, I think AI is here to stay. So let’s see how, if it’s going to be good for humanity or bad for humanity, it’s all going to depend on the way that everything develops. But I think this is a very new way to explore things that were before unexplored and things are getting more approachable to all of us in terms of this technology. So we’ll see what is going to happen in terms of the use at the end of humanity is going to give to this technology.
Srini Penchikala: Okay. Anthony.
Anthony Alford: Definitely interesting times. Stay tuned to infoq.com to keep up with the trends and new developments.
Srini Penchikala: There you go. Roland.
Roland Meertens: As a large language model, I’m unable to create any closing remarks.
Srini Penchikala: Okay. It’ll be available in the premium version. How about you, Sherin?
Sherin Thomas: Let me just quickly ask ChatGPT to generate a closing for me. Yeah, it was so nice chatting with you all and yeah, I hope the listeners enjoy our chit-chat.
Srini Penchikala: Thank you. Thanks everybody. So we’ll see you all next time and we’ll see how many predictions have come true and what all happened in the last one year when we talk again. So thank you. Have a great one. Until next time. Bye.
.
From this page you also have access to our recorded show notes. They all have clickable links that will take you directly to that part of the audio.
Google Cloud Unveils AlloyDB AI: Transforming PostgreSQL with Advanced Vector Embeddings and AI
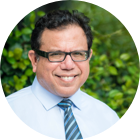
MMS • Steef-Jan Wiggers
Article originally posted on InfoQ. Visit InfoQ
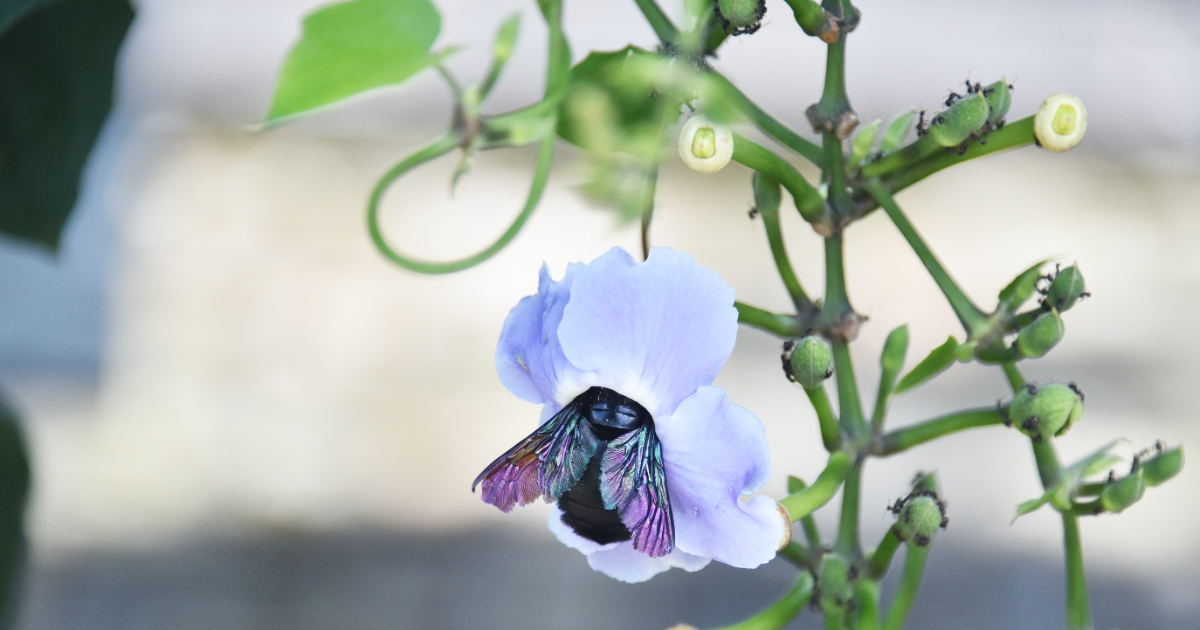
During the recent Google Cloud Next, Google announced AlloyDB AI in preview as an integral part of AlloyDB for PostgreSQL, allowing developers to build generative (gen) Artificial Intelligence (AI) applications leveraging large language models (LLMs) with their real-time operational data through built-in, end-to-end support for vector embeddings.
Earlier, the company launched support for the pgvector on Cloud SQL for PostgreSQL and AlloyDB for PostgreSQL, bringing vector search operations to the managed databases, allowing developers to store vector embeddings generated by Large Language Models (LLMs) and perform similarity searches. AlloyDB AI is built on the basic vector support available with standard PostgreSQL, providing developers with the ability, according to the company, “to create and query embeddings to find relevant data with just a few lines of SQL — no specialized data stack required, and no moving data around.”
In addition, AlloyDB AI brings a few other new capabilities into AlloyDB that can help developers incorporate their real-time data into gen AI applications:
- Enhanced vector support, faster than standard PostgreSQL queries, through tight integrations with the AlloyDB query processing engine. Furthermore, the company also introduced quantization techniques based on its ScaNN technology to support four times more vector dimensions and a three-times space reduction when enabled.
- Access to local models in AlloyDB and remote models hosted in Vertex AI, including custom and pre-trained models. Developers can train and fine-tune models with the data stored in AlloyDB and then deploy them as endpoints on Vertex AI.
- Integrations with the AI ecosystem, including Vertex AI Extensions (coming later this year) and LangChain, which will offer the ability to call remote models in Vertex AI for low-latency, high-throughput augmented transactions using SQL for use-cases such as fraud detection.
Andi Gutmans, GM & VP of Engineering, Google Cloud Databases, wrote in a Google blog post:
AlloyDB AI allows users to easily transform their data into vector embeddings with a simple SQL function for in-database embeddings generation, and runs vector queries up to 10 times faster than standard PostgreSQL. Integrations with the open-source AI ecosystem and Google Cloud’s Vertex AI platform provide an end-to-end solution for building gen AI applications.
A respondent on a Reddit thread asked, based on the Andi statement, if Google tries to Embrace, extend, and extinguish (EEE) PostgreSQL with AlloyDB AI, with another answering:
I think what you’re trying to say is that just because someone – especially [large company] – tries to improve/integrate popular open projects doesn’t mean it’s always EEE.
Which I doubt EEE is purposeful the majority of the time initially, even if has the potential to become that later. In the case of Google, I think this would be a case of “how do we add value to our product to sell” followed by “this feature costs us too much resources to maintain, let’s cut it and focus on [new feature]”
In addition, other database- and public cloud providers already support vector embeddings, including MongoDB, DataStax’s Cassandra database service Astra, open-source PostgreSQL (via Pgvector), and Azure Cognitive Search. The latter recently has a new capability for indexing, storing, and retrieving vector embeddings from a search index in preview.
Lastly, AlloyDB AI is available in AlloyDB on Google Cloud and AlloyDB Omni at no additional cost. The pricing details of AlloyDB are available on the pricing page.
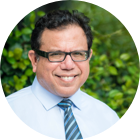
MMS • RSS
Posted on mongodb google news. Visit mongodb google news
MongoDB (NASDAQ:MDB – Free Report) had its price objective boosted by Argus from $435.00 to $484.00 in a report published on Tuesday, MarketBeat Ratings reports. The brokerage currently has a buy rating on the stock.
Several other equities analysts have also weighed in on MDB. Needham & Company LLC raised their price objective on shares of MongoDB from $430.00 to $445.00 and gave the company a buy rating in a research report on Friday, September 1st. Barclays raised their target price on MongoDB from $421.00 to $450.00 and gave the company an overweight rating in a report on Friday, September 1st. VNET Group restated a maintains rating on shares of MongoDB in a report on Monday, June 26th. Tigress Financial lifted their price target on shares of MongoDB from $365.00 to $490.00 in a research report on Wednesday, June 28th. Finally, Stifel Nicolaus lifted their price target on shares of MongoDB from $420.00 to $450.00 and gave the company a buy rating in a research report on Friday, September 1st. One analyst has rated the stock with a sell rating, three have issued a hold rating and twenty-one have given a buy rating to the stock. Based on data from MarketBeat, the company has an average rating of Moderate Buy and a consensus price target of $418.08.
Check Out Our Latest Stock Analysis on MDB
MongoDB Price Performance
Shares of NASDAQ:MDB opened at $394.13 on Tuesday. The company has a current ratio of 4.19, a quick ratio of 4.19 and a debt-to-equity ratio of 1.44. MongoDB has a 1 year low of $135.15 and a 1 year high of $439.00. The business has a fifty day simple moving average of $390.36 and a 200 day simple moving average of $307.62. The firm has a market cap of $27.82 billion, a price-to-earnings ratio of -113.91 and a beta of 1.11.
Insider Buying and Selling
In other news, Director Dwight A. Merriman sold 1,000 shares of the business’s stock in a transaction on Tuesday, July 18th. The shares were sold at an average price of $420.00, for a total value of $420,000.00. Following the sale, the director now owns 1,213,159 shares in the company, valued at approximately $509,526,780. The sale was disclosed in a filing with the Securities & Exchange Commission, which can be accessed through the SEC website. In other news, Director Dwight A. Merriman sold 1,000 shares of the stock in a transaction on Tuesday, July 18th. The stock was sold at an average price of $420.00, for a total transaction of $420,000.00. Following the sale, the director now owns 1,213,159 shares in the company, valued at $509,526,780. The sale was disclosed in a document filed with the Securities & Exchange Commission, which is available through the SEC website. Also, Director Hope F. Cochran sold 2,174 shares of the stock in a transaction on Thursday, June 15th. The stock was sold at an average price of $373.19, for a total value of $811,315.06. Following the sale, the director now owns 8,200 shares in the company, valued at approximately $3,060,158. The disclosure for this sale can be found here. Insiders sold 76,551 shares of company stock worth $31,143,942 in the last 90 days. 4.80% of the stock is owned by company insiders.
Hedge Funds Weigh In On MongoDB
A number of hedge funds and other institutional investors have recently added to or reduced their stakes in the stock. Raymond James & Associates lifted its holdings in shares of MongoDB by 32.0% during the 1st quarter. Raymond James & Associates now owns 4,922 shares of the company’s stock valued at $2,183,000 after purchasing an additional 1,192 shares in the last quarter. PNC Financial Services Group Inc. raised its holdings in shares of MongoDB by 19.1% in the 1st quarter. PNC Financial Services Group Inc. now owns 1,282 shares of the company’s stock valued at $569,000 after purchasing an additional 206 shares during the period. MetLife Investment Management LLC acquired a new position in MongoDB during the first quarter worth approximately $1,823,000. Panagora Asset Management Inc. raised its stake in MongoDB by 9.8% in the first quarter. Panagora Asset Management Inc. now owns 1,977 shares of the company’s stock valued at $877,000 after buying an additional 176 shares during the period. Finally, Vontobel Holding Ltd. raised its position in shares of MongoDB by 100.3% in the first quarter. Vontobel Holding Ltd. now owns 2,873 shares of the company’s stock valued at $1,236,000 after purchasing an additional 1,439 shares during the period. Institutional investors own 88.89% of the company’s stock.
About MongoDB
MongoDB, Inc provides general purpose database platform worldwide. The company offers MongoDB Atlas, a hosted multi-cloud database-as-a-service solution; MongoDB Enterprise Advanced, a commercial database server for enterprise customers to run in the cloud, on-premise, or in a hybrid environment; and Community Server, a free-to-download version of its database, which includes the functionality that developers need to get started with MongoDB.
Recommended Stories
Receive News & Ratings for MongoDB Daily – Enter your email address below to receive a concise daily summary of the latest news and analysts’ ratings for MongoDB and related companies with MarketBeat.com’s FREE daily email newsletter.
Article originally posted on mongodb google news. Visit mongodb google news
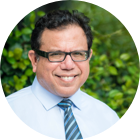
MMS • RSS
Posted on nosqlgooglealerts. Visit nosqlgooglealerts
PRESS RELEASE
Published September 6, 2023
The NoSQL market was valued at $2,410.5 million in 2022, and it is expected to reach $22,087 million by 2030, growing at a compound annual growth rate (CAGR) of 31.4% between 2023 and 2030.
NoSQL databases are designed to store and retrieve both structured and unstructured data using methods that differ from the tabular relationships found in relational databases (RDBMS). They encompass a diverse set of database technologies created to manage the growing influx of data produced and stored due to factors like the Internet of Things (IoT) and the internet. Due to the diverse origins of data and the need for distributed data storage with substantial storage capacities, NoSQL solutions are often preferred.
Download Free Sample Report- https://www.marketdigits.com/request/sample/744
The NoSQL market is poised for growth due to various factors, including increasing demand in sectors like e-commerce, web applications, and social game development. Research in this field encompasses the examination of NoSQL software, encompassing revenue generated from commercial licenses and upgrade fees for open-source NoSQL solutions. Nevertheless, a notable obstacle hindering widespread adoption of NoSQL technology remains the complexity of organizing and testing intricate queries compared to traditional relational database methods. Despite this challenge, it is expected that NoSQL databases will gain rapid acceptance in the coming years as awareness grows, particularly in managing the expanding commercial data volumes, notably within the social networks, retail, and e-commerce sectors.
Key Drivers in Nosql Market
- Cloud Dominance: The continued growth of cloud computing has had a significant impact on the enterprise application market. More businesses are migrating to cloud-based solutions for their enterprise applications due to scalability, flexibility, and cost-efficiency.
- AI and Machine Learning Integration: Enterprise applications are increasingly incorporating artificial intelligence (AI) and machine learning (ML) capabilities. These technologies are being used for data analysis, automation, and improving decision-making processes.
- Enhanced Security: With the rise in cyber threats, security has become a top priority in enterprise applications. Vendors are focusing on providing robust security features, including multi-factor authentication, encryption, and real-time threat detection.
- Integration of IoT: The Internet of Things (IoT) is being integrated into enterprise applications to enable businesses to collect and analyze data from connected devices. This helps in monitoring equipment, optimizing operations, and enhancing customer experiences.
- Mobile-First Approach: As mobile devices become increasingly essential for business operations, enterprise applications are being designed with a mobile-first approach. This ensures that employees can access critical data and perform tasks on their mobile devices securely.
- Low-Code/No-Code Platforms: The emergence of low-code and no-code development platforms is simplifying the process of creating and customizing enterprise applications. This trend allows businesses to build and modify applications with minimal coding skills.
Get a discount on report- https://www.marketdigits.com/request/discount/744
Major Classifications are as follows:
By Type
- Key-Value Store
- Document Database
- Column Based Store
- Graph Database
By Application
- DATA STORAGE
- MOBILE APPS
- WEB APPS
- DATA ANALYTICS
- OTHERS
By Industry Vertical
- Retail
- Gaming
- IT
- Others
By Region
- North America (U.S., Canada)
- Europe (Germany, UK, France, Rest of Europe)
- Asia-Pacific (Japan, China, India, Rest of Asia-Pacific)
- LAMEA (Latin America, Middle East, Africa)
Key Players
COUCHBASE, AEROSPIKE, GOOGLE LLC, OBJECTIVITY, INC., MARKLOGIC CORPORATION, NEO4J, INC., MICROSOFT CORPORATION, MONGODB INC., DATASTAX, AMAZON WEB SERVICES, INC.
Request for enquiry before buying- https://www.marketdigits.com/request/enquiry-before-buying/744
Key Questions Addressed by the Report
• What are the growth opportunities in the Nosql Market?
• What are the major raw materials used for manufacturing Nosql Market?
• What are the key factors affecting market dynamics?
• What are some of the significant challenges and restraints that the industry faces?
• Which are the key players operating in the market, and what initiatives have they undertaken over the past few years
Nosql Market Frequently Asked Questions (FAQs):
- What are the NoSQL databases available in the market?
- What makes NoSQL faster?
- What are the types of NoSQL databases?
- What are the advantages of using NoSQL databases?
About MarketDigits:
MarketDigits is one of the leading business research and consulting companies that helps clients to tap new and emerging opportunities and revenue areas, thereby assisting them in operational and strategic decision-making. We at MarketDigits believe that market is a small place and an interface between the supplier and the consumer, thus our focus remains mainly on business research that includes the entire value chain and not only the markets.
We offer services that are most relevant and beneficial to the users, which help businesses to sustain in this competitive market. Our detailed and in-depth analysis of the markets catering to strategic, tactical, and operational data analysis & reporting needs of various industries utilize advanced technology so that our clients get better insights into the markets and identify lucrative opportunities and areas of incremental revenues.
Contact US:
1248 CarMia Way Richmond,
VA 23235, United States.
Phone: +1 510-730-3200
Email: [email protected]
Website: https://www.marketdigits.com
Newsmantraa
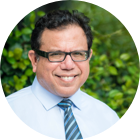
MMS • RSS
Posted on mongodb google news. Visit mongodb google news
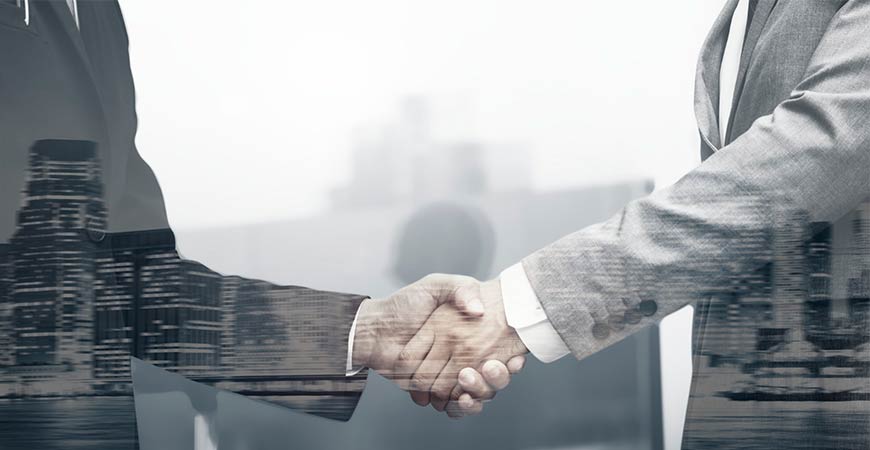
The fresh collaboration unlocks velocity, extensibility and protection for corporate assets.
Singapore-based artificial intelligence (AI) and cloud technology company CloudMile has formed a strategic partnership with source-available database MongoDB. This alliance will allow CloudMile’s corporate clients to utilize MongoDB Atlas, a cloud-native developer data platform, on Google Cloud. This collaboration offers developers the tools for flexible and scalable enterprise application creation.
CloudMile’s clients stand to benefit from MongoDB Atlas by enhancing product development, fortifying application security and extracting valuable data insights. The merger of MongoDB’s data platform with Google Cloud offers unparalleled speed, scalability and security.
Addressing modern data challenges
Modern businesses grapple with data-related issues such as isolated data storage and sluggish data processing. The CloudMile and MongoDB alliance aims to counter these challenges. By combining forces, they’re presenting a unified developer data platform that offers valuable insights into various business aspects, from customer interactions and product metrics to operational efficiency.
A notable element of this union is the seamless integration of MongoDB Atlas with Google Cloud’s BigQuery. Such an alignment could potentially enhance operational data, improving customer interactions. MongoDB Atlas, known for its prompt response times in high-demand applications, collaborates effortlessly with BigQuery. This synergy facilitates data aggregation, in-depth analytics and advanced machine learning applications.
Boosting efficiency in mobile gaming development
Highlighting the significance of this partnership, the Asian mobile gaming industry, which is witnessing a 3-5% compound annual growth rate (CAGR), stands to benefit. A testimony to this is a leading Taiwanese gaming firm that capitalized on the CloudMile-MongoDB partnership. By doing so, they streamlined operational costs and enhanced their product development phase.
Furthermore, MongoDB Atlas features, from efficient data synchronization utilizing ACID Transaction to robust security measures, promise a dependable gaming landscape. It streamlines game developers’ tasks with a unified platform and ensures utmost security during data storage and transmission. Coupled with Google Cloud’s inherent security, it aims to deliver an uninterrupted gaming experience.
Industry voices echoing optimism
Several key industry figures have expressed their optimism regarding this collaboration. Patrick Wee of Google Cloud highlighted CloudMile’s prowess in Malaysia, emphasizing the tripartite commitment of CloudMile, MongoDB and Google Cloud to optimize data management. Lester Leong of CloudMile Malaysia spotlighted MongoDB’s scalability, suggesting the partnership promises growth and untapped opportunities. Simon Eid from MongoDB described the collaboration as a blend of AI, cloud tech and their data platform, underscoring a mutual goal to spur regional growth.
In essence, the synergy between CloudMile and MongoDB is not just a business collaboration; it signifies a step forward in evolving cloud technology solutions, with an overarching aim to bolster business growth and customer trust.
Also read:
Header image courtesy of Freepik
Article originally posted on mongodb google news. Visit mongodb google news
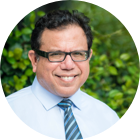
MMS • RSS
Posted on mongodb google news. Visit mongodb google news
On September 5, 2023, Canaccord Genuity analyst David Hynes expressed his positive outlook on MongoDB (NASDAQ:MDB) by maintaining a Buy rating and raising the price target from $410 to $450. This indicates a bullish sentiment towards the company’s stock.
It is worth noting that the average outperform rating and price target range for MongoDB, as reported by analysts polled by Capital IQ, is between $250 and $500. This suggests a wide range of expectations among market experts regarding the future performance of the stock.
Previously, on June 6, 2023, Canaccord Genuity analyst David Hynes had already given MongoDB a Buy rating and had increased the price target, although the specific amount was not disclosed. This implies a consistent positive sentiment towards the company over time.
According to MarketBeat, the consensus price target for MongoDB among analysts is $407.39, with a projected upside of 3.7% from its current price of $392.88. This indicates that analysts, on average, expect the stock to experience a modest increase in value.
Overall, the outlook for MongoDB appears to be positive, with analysts expressing confidence in its growth potential. However, it is important to consider that these projections are subject to change as market conditions evolve.
MDB Stock Performance: Mixed Results on September 5, 2023
On September 5, 2023, MongoDB Inc. (MDB) experienced a mixed performance in the stock market. MDB’s previous close was $393.32, and the stock opened at $389.55 on September 5th. Throughout the day, the stock’s price fluctuated between a low of $389.55 and a high of $398.40. The trading volume for the day was 53,042 shares, which is significantly lower than the average volume of 1,767,765 shares over the past three months.
MDB is considered a mid-sized company in the technology services sector with a market capitalization of $28.0 billion. The company’s earnings growth last year was -5.89%, indicating a decline in profitability. However, this year’s earnings growth has seen a significant improvement, with an impressive growth rate of +92.12%. Looking ahead, analysts forecast a more modest earnings growth rate of +8.00% over the next five years.
MDB’s revenue growth last year was +46.95%. This indicates that the company has been successful in increasing its top line. However, it’s important to note that the company’s profitability has been impacted negatively, as evidenced by the negative net profit margin of -26.90%.
MDB’s price-to-sales ratio stands at 11.45, while the price-to-book ratio is 37.12. These ratios indicate that the stock may be trading at a premium compared to its industry peers. It’s worth noting that the price-to-earnings (P/E) ratio is not available (NM), suggesting that the company may not have positive earnings at the moment.
On September 5th, MDB’s stock price experienced a decline of -0.93, representing a -0.29% change from the previous day’s close. This performance is in line with the overall downward trend observed in the technology services industry on that day. Other notable companies in the industry, such as ANSS (ANSYS Inc) and HUBS (HubSpot Inc), also experienced negative price changes of -0.29% and -1.15%, respectively. Take-Two Interactive (TTWO) had a minor decline of -0.19%.
MDB’s next reporting date is scheduled for December 6, 2023. Analysts are forecasting earnings per share (EPS) of $0.27 for the current quarter. The company’s annual revenue for the previous year was $1.3 billion, while the annual profit was -$345.4 million.
In conclusion, MDB’s stock performance on September 5, 2023, was relatively mixed. While the stock experienced a slight decline, the company’s earnings growth this year has been impressive. However, profitability remains a concern, as indicated by the negative net profit margin. Investors should closely monitor the company’s future earnings reports and keep an eye on industry trends to make informed investment decisions.
MongoDB Inc (MDB) Stock Forecast: Analysts Predict 13.78% Growth with a Median Target Price of $450.00
On September 5, 2023, MongoDB Inc (MDB) had a median target price of $450.00, according to 23 analysts offering 12-month price forecasts. The high estimate was $500.00, while the low estimate was $250.00. This median estimate represented a 13.78% increase from the last price of $395.51.
The consensus among 28 polled investment analysts was to buy stock in MongoDB Inc. This rating had remained unchanged since September, indicating a consistent positive sentiment towards the company’s stock.
In terms of financial performance, MongoDB Inc reported earnings per share of $0.27 for the current quarter. Additionally, the company recorded sales of $389.8 million. The reporting date for these figures was December 6.
Based on the analyst forecasts and the consensus buy rating, it seems that investors have high expectations for MongoDB Inc’s stock performance. The median target price of $450.00 suggests that analysts believe the stock has room to grow by approximately 13.78%.
Investors should conduct thorough research and analysis before making any investment decisions.
Article originally posted on mongodb google news. Visit mongodb google news
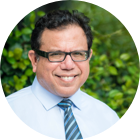
MMS • RSS
Posted on mongodb google news. Visit mongodb google news
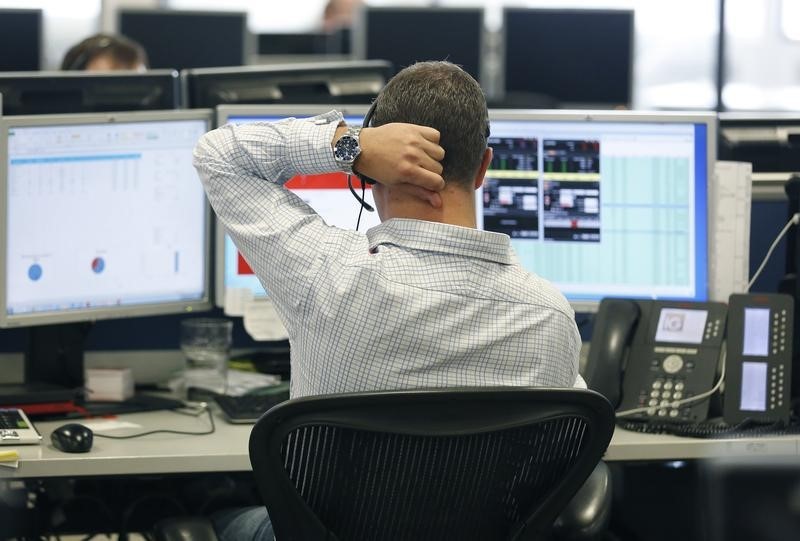
Database as a service company Couchbase (NASDAQ: BASE)
will be reporting results tomorrow after the bell. Here’s what investors should know.
Last quarter, Couchbase reported revenues of $41 million, up 17.6% year on year, beating analyst revenue expectations by 3.08%. However, it was a weaker quarter for the company, with revenue and operating loss guidance for the next quarter coming in below analysts’ expectations.
Is Couchbase buy or sell heading into the earnings? Find out by reading the original article on StockStory.
This quarter analysts are expecting Couchbase’s revenue to grow 4.83% year on year to $41.7 million, slowing down from the 34% year-over-year increase in revenue the company had recorded in the same quarter last year. Adjusted loss is expected to come in at -$0.22 per share.
Majority of analysts covering the company have reconfirmed their estimates over the last thirty days, suggesting they are expecting the business to stay the course heading into the earnings. The company has a history of exceeding Wall St’s expectations, beating revenue estimates every single time over the past two years on average by 6.04%.
Looking at Couchbase’s peers in the data storage segment, some of them have already reported Q2 earnings results, giving us a hint of what we can expect. MongoDB (NASDAQ:MDB) delivered top-line growth of 39.6% year on year, beating analyst estimates by 8.42%, and Commvault Systems reported revenues up 0.09% year on year, exceeding estimates by 0.49%. MongoDB traded up 8.5% on the results, and Commvault Systems was down 2.5%.
Read the full analysis of MongoDB’s and Commvault Systems’s results on StockStory.
Investors in the data storage segment have had steady hands going into the earnings, with the stocks down on average 0.78% over the last month. Couchbase is up 7.88% during the same time, and is heading into the earnings with analysts’ average price target of $21.1, compared to share price of $17.25.
One way to find opportunities in the market is to watch for generational shifts in the economy.
Almost every company is slowly finding itself becoming a technology company and facing cybersecurity risks and as a result, the demand for cloud-native cybersecurity is skyrocketing. and with revenue growth of 70% year on year and best-in-class SaaS metrics it should definitely be on your radar.
The author has no position in any of the stocks mentioned.
Article originally posted on mongodb google news. Visit mongodb google news