Month: September 2023
MongoDB announces new database capabilities to improve developer experience – SD Times
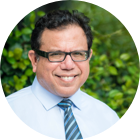
MMS • RSS
Posted on mongodb google news. Visit mongodb google news
At an event MongoDB held in London today, the company announced a number of product announcements, including updates to Atlas, a new edge platform, and more.
According to MongoDB, developers’ time is an organization’s “most precious commodity.” Therefore, the company would like to improve the experience around the most common tasks a developer does every day. It narrowed this down to two areas that could be improved and is releasing new capabilities around those.
The first area is making the Atlas database easier to work with. It is now possible for developers to use the Atlas CLI to manage their development environments locally using the same experience they have in the cloud. Atlas CLI has also been updated to include Atlas Search and Atlas Vector Search, which enables developers to create and manage search indexes within development workflows.
“By giving developers the power of Atlas at their fingertips no matter their preferred development environment, MongoDB continues to expand the scope and capabilities of its developer data platform while placing a premium on developer experience,” MongoDB wrote in a blog post.
The second area is making it easier to write MongoDB queries. Developers can now use plain English to ask questions, and the MongoDB GUI, Compass, will automatically generate a query for the request. The company also has a private preview for SQL query conversion in Relational Migrator, which enables queries and procedures to be converted to the MongoDB query language.
The company also is making MongoDB accessible from more places. It announced Atlas for the Edge, which brings data processing and storage closer to where the data lives. Atlas Edge Servers can be deployed anywhere, enabling developers to create customer experiences that need low latency, heavy computation close to where data is generated, or run applications in locations that have intermittent internet.
“With MongoDB Atlas for the Edge, organizations can now use a single, unified interface to deliver a consistent and frictionless development experience from the edge to the cloud — and everything in between. Together, the capabilities included with MongoDB Atlas for the Edge allow organizations to significantly reduce the complexity of building edge applications and architectures,” MongoDB wrote in a blog post.
MongoDB has also announced its own publishing company, MongoDB Press, which will make it easier to publish knowledge about MongoDB. Currently two books have been published: one is on aggregations and the other on MongoDB 7.0.
The company has also published a new solutions library that features use cases from across the industry to give developers inspiration on how they can use MongoDB. And finally, it has also added new content to MongoDB University.
Article originally posted on mongodb google news. Visit mongodb google news
Data on Kubernetes Community Announces Schedule for DoK Day at KubeCon North America 2023
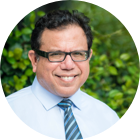
MMS • RSS
Posted on mongodb google news. Visit mongodb google news
Schedule includes case studies and techniques for running data on Kubernetes
OREGON CITY, Ore., September 26, 2023–(BUSINESS WIRE)–The Data on Kubernetes Community (DoKC), the community for end users running data workloads on Kubernetes, today announced the schedule for the third annual Data on Kubernetes Day North America (DoK Day), an industry event to share best practices and use cases, forge critical relationships, and learn about advancements in the use of Kubernetes for data. DoK Day will be co-located at KubeCon + CloudNativeCon North America 2023 taking place in Chicago, Illinois on November 6.
Running data workloads on Kubernetes has a transformative impact on organizations including increased productivity, revenue growth, market share, and margin. As organizations rely more on Kubernetes for infrastructure management, practitioners who are building and operating data-centric applications on Kubernetes are increasingly reliant on a vendor-neutral environment like DoKC that encourages the sharing of knowledge, best practices and use cases for how Kubernetes can be leveraged as a standard to revolutionize data.
DoK Day attendees include Kubernetes developers, data application developers, product managers, technical management and community leads who are looking to expand their knowledge of Kubernetes for infrastructure management in a vendor-neutral setting. The schedule covers a breadth of key topics impacting K8s, including use cases and best practices, DoK Day 2, emerging and advanced technologies, and DoK security.
Enterprises including Altinity, Google, Intuit, and VMware will share their experiences with running stateful data on Kubernetes in production environments, while speakers from Bytedance, MongoDB, Portworx, among others, share advanced engineering techniques, best practices, and industry trends. The full schedule is available here.
Schedule highlights include:
-
Transforming Data Processing with Kubernetes: Journey towards a Self-Serve Data Mesh – Rakesh Subramanian Suresh, Intuit
-
Should you run your database on Kubernetes – George Hantzaras, MongoDB
-
Stateful Workloads in Kubernetes: A Deep Dive – Kaslin Fields and Michelle Au, Google
-
Distributed Vector Databases – What, Why, and How – Steve Pousty, VMware
-
Stop worrying and keep querying, using Automated Multi-region Disaster Recovery – Sergey Pronin, Percona and Shivani Gupta, Elotl
-
Is it safe? Security Hardening for Databases using Kubernetes Operators – Robert Hodges, Altinity
-
Zero-touch fault-tolerance for Cloud-native Geo-distributed Databases – Selvi Kadirvel, Elotl
-
More Than Kubernetes: The Data on Kubernetes Ecosystem – Melissa Logan, Data on Kubernetes Community
Sponsorship
DoK Day would not be possible without support from sponsors including Gold Sponsors EDB and Percona.
Registration
DoK Day is a KubeCon + CloudNativeCon North America CNCF-hosted co-located event. In-person event attendees may register for an All-Access In-Person KubeCon + CloudNativeCon pass which will include entry to DoK Day and other co-located events. All-Access Registration rate starts at US$1979. More registration details, including prices and pass types can be found here.
The DoK Community was founded in June of 2020 to bring practitioners together to share their experiences in running Data on Kubernetes. Since then, the DoK Community has grown rapidly with more than 200 scheduled meetups and more than 10,000 members across its channels.
About Data on Kubernetes Community (DoKC)
Kubernetes was initially designed to run stateless workloads. Today it is increasingly being used to run databases and other stateful workloads. The Data on Kubernetes Community was founded in June 2020 to bring practitioners together to solve the challenges of working with data on Kubernetes. An openly governed community, DoKC exists to assist in the emergence and development of techniques for the use of Kubernetes for data. https://dok.community/.
View source version on businesswire.com: https://www.businesswire.com/news/home/20230926878178/en/
Contacts
Article originally posted on mongodb google news. Visit mongodb google news
MongoDB revs up its vector search feature to power faster and more accurate generative AI apps
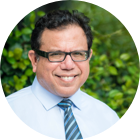
MMS • RSS
Posted on mongodb google news. Visit mongodb google news
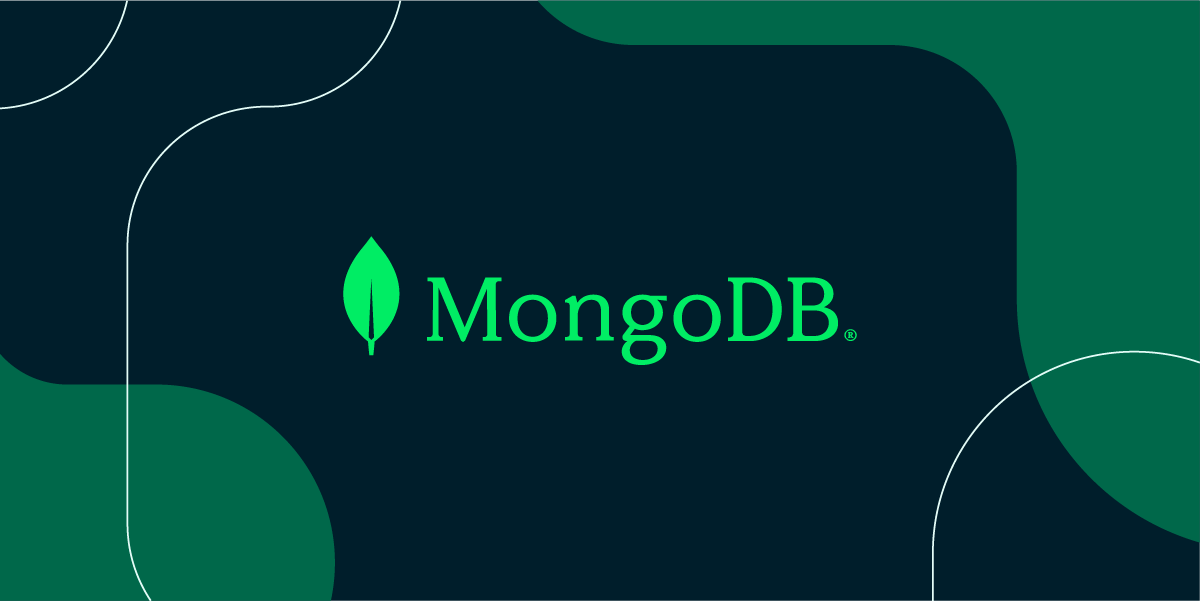
Cloud database provider MongoDB Inc. today announced a slew of updates to its platform that are designed to help software developers build applications that can harness the power of generative artificial intelligence.
Among other improvements, it announced new Vector Search functionality that improves the way it filters and aggregates unstructured data, making it easier for generative AI applications to access. Besides enabling generative AI, MongoDB is also using it to power more intelligence experiences for developers that aim to improve their productivity when using the MongoDB Atlas cloud platform.
The company also announced a new version of its flagship database, MongoDB Atlas for the Edge. It said that will make it easier to deploy applications that can process data closer to where it’s created.
The new capabilities in MongoDB Atlas, announced at the company’s MongoDB.local London developer conference, should add further momentum to a generative AI push that began earlier this year. Back in June, MongoDB announced a new Vector Search feature, and today it’s building on that foundation.
MongoDB is the creator of the document-oriented MongoDB database, which is used for a wide range of data-intensive applications and prized for its ability to store information in multiple different formats. MongoDB Atlas is the cloud-hosted version of that database.
The company believes the choice of database is critical for enterprises that want to build generative AI applications. It argues that AI developers need to build on a database that’s unified, fully managed, flexible and scalable.
MongoDB says it provides all of these things, and more besides, the most important of which is its enhanced Vector Search platform. Vector search is vital for AI as it provides a way to create numerical representations – vectors – of unstructured data such as images, written notes and audio. It makes this data accessible to generative AI models so they can be trained on it.
MongoDB presents Atlas as a unified solution to process data for generative AI apps, marrying structured data with vectors in a single platform. It launched Vector Search in preview in June, and today it announced significant performance improvements that reduce the time it takes to create data indexes by 85%. In addition, MongoDB Atlas Vector Search now integrates with fully managed data streams from Confluent Cloud, making it easier to access real time data for AI.
One of the new capabilities today enables developers to create a dedicated data aggregation stage with Vector Search using MongoDB’s Query API syntax and Aggregation Pipeline too. This makes it easier to filter results and improve the accuracy of information retrieval, thereby reducing AI “hallucinations,” or the tendency of AI models to generate inaccurate responses.
The indexing acceleration is a key improvement too, because generating vectors is the first step in the preparation of AI training data. Once the vectors are created, developers must build an index for that data to be queried for information retrieval. It’s also necessary to update the index every time data is changed or new data is added.
As for the real-time data streams using Confluent Cloud, it can be helpful in building more engaging and responsive generative AI applications that can learn and respond in real time, MongoDB said. With today’s update, developers can integrate Confluent Cloud data streams within MongoDB Atlas Vector Search to provide their apps with “ground-truth data,” or the most accurate and up-to-date information, from a variety of sources. This will make generative AI apps more responsive to changing conditions, the company said.
Constellation Research Inc. analyst Doug Henschen said the vector search capabilities are focused on the needs of developers and help MongoDB set itself apart from other platforms and grow its adoption. He said the most impressive thing about MongoDB’s vector search updates is that it’s not just announcing new capabilities, but also illustrating how it aids in innovation.
“MongoDB is sharing the real-world customer examples of Dataworkz, Drivly, ExTrac, Inovaare Corp., NWO.ai, One AI and VISO Trust along with plenty of details on how it’s helping them to innovate,” Henschen said. “This makes the announcement come across as much more real, and will inspire other customers to begin experimenting.”
New generative AI experiences for developers
While MongoDB is making it easier for developers to build more sophisticated generative AI apps, it has also built its own generative AI tools for them to use. The new developer experiences announced today make use of generative AI to improve productivity, reducing the time and effort they spend on mundane tasks so they can focus on the more taxing aspects of their work.
The company announced a new experience for MongoDB Compass, which is now able to generate queries and aggregations from natural language to build data-driven applications faster and more easily. Meanwhile, MongoDB Atlas Charts users can now create rich data visualizations for business intelligence using natural language prompts.
MongoDB Documentation gains a new generative AI chatbot that provides rapid answers to technical questions, while MongoDB Relational Migrator can now convert Structured Query Language to MongoDB Query API Syntax to help automate database migrations.
Henschen said MongoDB’s new generative AI-powered features are mostly in line with what other database platforms are doing, using the technology to improve developer productivity. “All but the chat feature are currently only in preview, but it’s still a good set of capabilities that shows MongoDB is not conservative when it comes to infusing its products and services with the latest generative AI tools,” he added.
MongoDB Atlas lands at the edge
With MongoDB Atlas at the Edge, the company is bringing its advanced database functionality to edge environments, making it simpler for organizations to deploy their apps closer to where data is generated.
It will enable companies to deploy distributed applications that can reach end users faster, providing real time experiences, the company said. MongoDB Atlas for the Edge makes it possible for data to be stored and synchronized in real-time across data sources and locations, and will provide advantages in use cases such as connected cars, smart factories and supply chain optimization, the company said.
“MongoDB has long had its mobile database and synchronization capabilities, but with Atlas at the Edge it’s clear that it’s now driving more consistency and continuity, helping to simplify edge-to-cloud use cases with two-way interactivity,” Henschen said.
MongoDB Chief Product Officer Mark Porter stopped by theCUBE, SiliconANGLE Media’s mobile livestreaming studio, during the MongoDB .Local NYC 2023 event in June to discuss the company’s ambitions to play a key role in the future of AI:
Image: MongoDB
Your vote of support is important to us and it helps us keep the content FREE.
One-click below supports our mission to provide free, deep and relevant content.
Join our community on YouTube
Join the community that includes more than 15,000 #CubeAlumni experts, including Amazon.com CEO Andy Jassy, Dell Technologies founder and CEO Michael Dell, Intel CEO Pat Gelsinger and many more luminaries and experts.
THANK YOU
Article originally posted on mongodb google news. Visit mongodb google news
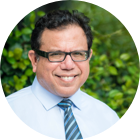
MMS • RSS
Posted on mongodb google news. Visit mongodb google news
Couchbase BASE recently announced that Centrica PLC has selected Couchbase Capella Database-as-a-Service (DBaaS) to provide customer and job history information to its 7,000 field engineers, allowing them to effectively serve its customers in the U.K. and Ireland.
Centrica serves more than 10 million customers, offering energy and services under various brands like British Gas, Bord Gáis Energy and Hive. Given the critical nature of its services, customers expect swift and accurate customer support, especially for urgent problems like broken boilers or disrupted energy supply.
Centrica is set to use Couchbase Capella on Amazon AMZN Web Services for its customer 360 application. This application stores and provides access to customer information, including product data and billing history.
This information is crucial for field engineers who use a mobile app to address customer issues. By using Capella, Centrica ensures efficient, dependable and secure access to this data. This enables Centrica’s applications to quickly pinpoint and share essential information that engineers need to resolve.
Couchbase, Inc. Price and Consensus
Couchbase, Inc. price-consensus-chart | Couchbase, Inc. Quote
The Adoption of Generative Artificial Intelligence (AI) to Fend Off Competitors
Couchbase recently added Generative AI to its DBaaS, Couchbase Capella. This is expected to help developers write SQL++ and application-level code in a more time efficient way. It will also enhance developer experience and increase efficiency.
Shares of this Zacks Rank #3 (Hold) company have gained 31.7% year to date compared with the Zacks Computer and Technology sector’s rise of 32.5% due to increasing advancements in AI by competitors like MongoDB MDB, Oracle ORCL Database and Amazon Redshift,.
You can see the complete list of today’s Zacks #1 Rank (Strong Buy) stocks here.
MongoDB Atlas is a cloud-hosted database service with the primary aim to simplify the deployment, management and scaling of MongoDB databases, reducing the need for extensive administrative work. MongoDB Atlas recently integrated advanced AI and analytics capabilities directly into the development process, aligning with developers’ preferred workflows, frameworks and programming languages.
Oracle has announced its intention to introduce semantic search features utilizing AI vectors in Oracle Database 23c. These enhancements empower Oracle Database to store the semantic information from documents, images and other unstructured data as vectors and use them to efficiently perform similarity queries.
Amazon Redshift machine learning (Amazon Redshift ML) is a robust cloud-based service designed to simplify the utilization of machine learning technology for analysts and data scientists, regardless of their skill levels. With Amazon Redshift ML, the users can provide the data they wish to use for model training. These models can subsequently be applied to generate predictions for new input data without incurring extra expenses.
Centrica has been giving developer productivity increasingly more importance due to high demand for AI applications. This is expected to boost the company’s service revenues in the upcoming quarters.
The Zacks Consensus Estimate for 2023 subscription revenues is pegged at $164.24 million, indicating year-over-year growth of 14.9%. The Zacks Consensus Estimate is pegged at a loss of 78 cents per share, indicating a year-over-year rise of 13.33%.
Want the latest recommendations from Zacks Investment Research? Today, you can download 7 Best Stocks for the Next 30 Days. Click to get this free report
Amazon.com, Inc. (AMZN) : Free Stock Analysis Report
Oracle Corporation (ORCL) : Free Stock Analysis Report
MongoDB, Inc. (MDB) : Free Stock Analysis Report
Couchbase, Inc. (BASE) : Free Stock Analysis Report
To read this article on Zacks.com click here.
Article originally posted on mongodb google news. Visit mongodb google news
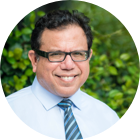
MMS • Christina Yakomin
Article originally posted on InfoQ. Visit InfoQ
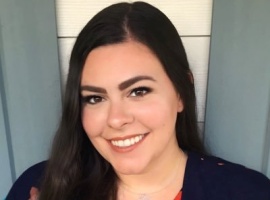
Transcript
Yakomin: Welcome to, did the chaos test pass? My name is Christina Yakomin. In this presentation, I’ll be talking to you about a new stage of maturity with chaos experimentation that my team has reached at Vanguard. Where now, for the first time, we have an appetite to determine the success or failure of a certain subset of the chaos experiments that we’re running. I’ll also talk a little bit about what it takes to be a part of that subset, and why we aren’t determining a pass or a failure for every single experiment that we run.
I am a senior architect at Vanguard in my current role. I’ve been specializing in site reliability engineering since about 2018, starting out with a role as a site reliability engineer supporting one of our most prominent cloud platforms for our public cloud application that we were deploying. After that, I went on to our, at the time, very new chaos engineering team, where I helped design and develop a homegrown tool for chaos engineering that I’ve spoken about a lot in the past. After that, I helped guide Vanguard through the adoption of a formal SRE practice through our SRE coaching team. This really helped us to enable our IT organization to adopt a DevOps model for product support. Through the enrichment and education that we’ve provided for the SRE roles that we’ve deployed, we’ve been able to make it easier for product teams to balance the workload of delivering features for their business, and also delivering on the non-functional requirements, including those related to resilience, through activities like performance engineering, and chaos engineering.
I’ve been speaking at a variety of conferences over the past couple of years, including QCon London, this year, where I spoke about our process for hazard analysis, and how we decide which chaos experiments we’re going to run. In my current role, I’m an architect supporting our engineering enablement and advancement department, which encompasses the SRE practice, and chaos engineering, and performance engineering that I spoke previously about. Also, our continuous integration and continuous delivery pipeline, our developer experience function, as well as several other key functions, all focused around making sure that engineers at Vanguard are having a positive experience and are able to efficiently deliver both features and non-functional requirements for the products that they support.
About Vanguard
Now, a little bit about Vanguard, and the importance of technology at a company like Vanguard. We’re a finance company first. We are one of the largest global providers of mutual fund products. We have trillions of dollars in assets under management. We’re talking really big numbers, really large numbers of clients. All of the work that we do, all of the interactions that we have with those clients of Vanguard is virtual. Unlike other asset management companies, Vanguard does not have brick and mortar locations where you can go in person and talk to an advisor or talk to someone about setting up your account. The interactions we have with our clients either happen via our call center, or ideally, for both us and our clients, through the web without needing to interact with an individual. That means it’s really important to us to not only make our web experience positive for clients to use, but to keep it available, because the vast majority of the time when clients are trying to interact with their portfolios at Vanguard, they’re doing so through the web. If we don’t keep our website available, they won’t be able to make key transactions on their portfolios, which can really be a timely activity, especially with the market volatility that we’ve seen over the past several years. Technology is at the forefront of our focus as a company, so much so that IT is the largest division at our company.
Chaos Engineering and Experimentation
The role that chaos engineering and experimentation plays. I want to spend one slide setting some context for everyone. Fundamentally, chaos engineering refers to the practice of injecting a fault into a running system, and then observing the resulting system behavior. There are a few different types of testing you can do. I like to categorize chaos engineering a few different ways. The first category is exploratory testing. This refers to going into your system and injecting a fault when you’re not quite sure how the system will respond. You do this for the purpose of learning about your system, when you know how you’ve designed it but this particular stimulus might be more of a mystery to you. You typically do experiments like this under careful observation in a controlled environment, that would be isolated from potential client impact. On the flip side, you might be testing with really well-formed hypotheses about your expectations of system behavior. These types of tests, maybe you did a hazard analysis activity upfront, you developed an, if we inject this fault, then the system will respond in these ways sort of hypothesis. By injecting the fault during the test, you’re either validating or disproving the hypothesis that you came in with. Just because you have a hypothesis doesn’t mean that that’s the actual behavior that you’re going to end up seeing from your system. You’re usually coming in with some level of confidence that you know how the system is going to behave.
Another way to categorize chaos experiments is testing the sociotechnical system versus testing just the technology. What this means is, when you’re testing the technology, you’re injecting the fault with the intention of observing how the system independently will handle it through automation. Whether you’ve built in some automated resilience mechanism through failover, autoscaling, or some other type of automated recovery through a fallback mechanism or something else, you want to make sure that without any human intervention, the technology is able to handle the fault that you are injecting, without any adverse effect or with minimal adverse impact to the client experience. Testing the sociotechnical system involves bringing the human element into the test. When we test the sociotechnical system, we’re talking about injecting a fault that in some way we expect the humans that support our systems will need to get involved in. Maybe you inject a certain fault, and the plan for recovering from that is failover, but it’s failover that isn’t automated. You run this test so that your engineers can practice the incident response procedure that they’d have for this situation and ensure that you have the appropriate access to the toggles, you know where to go to find them, and that the failover happens the way that you expect it to. A combination of human and system behavior being observed.
At this point at Vanguard, we’ve done tests that fall into all of those categories, and somewhere in between. When I say we’re reaching a new level of maturity with chaos experimentation, what I mean is that our highly motivated engineers are finding that when they sit down for their game days, whether that’s quarterly, monthly, even more frequently than that, oftentimes the list of chaos experiments that they’ve tried in the past and that they want to run again, and the new chaos experiments they want to run is growing pretty quickly. At a rate that they’re having trouble keeping up with, and they’re having trouble fitting it all in. They’re noticing that a lot of the time, they’re just repeating tests they’ve run before expecting the same results they’ve seen before. The only reason they’re repeating these tests is because they’ve continued to iterate on their system. They understand that the underlying system that they are testing has been changing, so the test, just like a suite of unit tests, needs to be repeated in order to ensure that the resilience we expected and observed in the past is still present. If this is something we’ve done before, and we’ve seen the results, so we know what to expect. In other aspects of software engineering, what we talk about there is an opportunity for automation. We want to reduce the cognitive load that the development teams are encountering with chaos experimentation by taking those tests that are repetitive, and automating them, so that we can confirm that as teams have been iterating on both the code bases and the system configurations, they haven’t introduced any adverse effect on the resilience that we’ve previously observed in our fault injection tests.
How Do We Know if a Chaos Test Has Passed?
That brings us to, how do we actually know particularly from a programmatic standpoint, if a chaos test has passed? First, you have to determine, is the app even healthy to begin with, before you do anything? Especially if, like Vanguard, you’re running your tests in a non-production environment, where the expectation for stability isn’t quite the same as your production environment. Then after you’ve determined you have a healthy application, you have to make sure you can programmatically assert what you actually expect will happen. Just in general, this needs to be the kind of test you come into with a hypothesis. You need to have an expectation of what will happen. It doesn’t always mean the expectation is the app will stay healthy. We’ll talk a little bit more about that. You do need an expectation. Then we need to, again, programmatically determine, does the actual observed behavior in response to the fault align with the expectation that we have, with the hypothesis that we came in with? Observed behavior in this case, like I said, doesn’t always mean a totally stable system. We might be ok with observing a failover with no client impact, but something happening behind the scenes. We might want to make sure that it’s ok if we have a dip in our availability for a minute or two. Once we start to talk about five or more minutes of downtime, we’re not ok with that. We can start to make some assertions around the speed of recovery, given a certain fault. Lots of different complex expectations we may have that we’re now trying to programmatically assert.
Chaos Test Assertions at Vanguard
At Vanguard, we did have some tailwinds working in our favor to help us accomplish this. I want to acknowledge some of those upfront before we continue. At Vanguard, the vast majority of chaos experiments that we are executing are being orchestrated through this homegrown tool that I’ve talked about in the past called, the Climate of Chaos. You can search for my past talks, Cloudy with a Chance of Chaos, is the title I used for a few different talks on that topic, to get more information about why we built that tool, and how we built that tool, and what it does. Then later in this presentation I’ll show some architecture specific to the chaos test assertions piece. Another tailwind we had is, in most applications at Vanguard we’re using CloudWatch alarms. There was some standardization there for the notification of an application becoming unhealthy. Several of our core cloud platform teams had centralized on CloudWatch alarms. The types of teams that were leveraging chaos experimentation, and were ready to automate, were also the types of teams that were leveraging that standardized platform. Or at least, were comfortable enough using CloudWatch alarms to determine application health statuses. We figured if we can take the product that we built, and since we’ve built it, we have the ability to customize it. We integrate that with the existing CloudWatch alarms or the ability to create new CloudWatch alarms. We can create assertions that we can apply in the context of our experiments. That’s exactly what our chaos engineering team has done.
Chaos Test Assertions, Dynamo Table
Here, this is the same information displayed twice on the screen, once in the form of a JSON object, and then two at the bottom, which is what I’ll run through, an excerpt similar to what our Dynamo Table looks like. You can follow along with whichever one is more palatable to your eye. This is the core of the chaos test assertions, is this Dynamo Table that we built in AWS. We have a unique name that we give every assertion so that it can be uniquely identified. Then we tie it to some other metadata. We associate each assertion with an application identifier, three-letter code, whatever you want to call your unique identifier for an application. This is the same key you can use in our CMDB to pull up metadata about the application, including the application owner, the department, the cost center, the business purpose. Next, we tie it to a CloudWatch alarm. Every assertion is a one-to-one relationship with a CloudWatch alarm, so we get the AlarmName, and the region of AWS where that alarm is stored.
Next up, we have a Cooldown period. When we’re talking about chaos test assertions, we want to talk about the app’s state, both before the experiment, during the experiment, and after the experiment. In order to get that after, we need to configure, how long do we wait before we consider this after? That’s what the Cooldown does. This is in terms of seconds here. You wait 60 seconds, 45 seconds. Some teams will wait 5 minutes or more before they actually go ahead and check the after state. It’s all depending on what your recovery time objective is going to be. Next, we have a description. This is optional. If you have maybe multiple assertions, more certain experiments that you want to keep track of, you can add the description there, so you know what the assertion is doing beyond just the uniquely identifying name that you gave it. Then we have a bunch of Boolean values. Some of these I’ll talk about now, some I’ll save for later. It’s basically that, if we see the alarm go into alarm state before, during, and after the experiment, at any of those points, if it’s in an alarm state, do we consider the experiment a failure? Most teams are definitely going to say, we consider it a failure if the alarm is in an alarm state after the experiment. Some teams will want to say it’s a failure before we even get started. You don’t want to run an experiment on an unhealthy app, so we can abort. Others it’s, we want stability throughout, so during the experiment, even if the experiment is ongoing, we shouldn’t see the alarm state. It depends on the team. ShouldPoll and DidAlarm, we’re going to save those for the next slide.
Chaos Test Assertion Checker
There’s two key workflows that I’m going to walk us through. This is where we’re going to start to get into the technical implementation details of this, and all the icons that you’ll see here are going to be AWS specific. There are ways that you could implement this in other clouds or even in an on-premise environment, just using different underlying technologies. Since a lot of folks are going to be familiar with the technologies at AWS, I figured I would include the AWS services that we are leveraging. The Chaos Test Assertion Checker is something you can think of as the background process. The main purpose of the Chaos Test Assertion Checker is to help us figure out if we go into an alarm state during an experiment, not before, not after, only in that during period. The way that we do this is with a CloudWatch Events Rule that fires up every single minute on a Cron schedule. That rule’s entire purpose is to trigger our lambda function to get going. The first thing that the lambda function does is reach out to a Dynamo Table, the one that we talked about on the previous slide, with the Chaos Test Assertions. It’s going to look for that ShouldPoll column that we skipped over. If ShouldPoll is set to true, what that means is that we are currently in the process of running an experiment for that particular assertion. We want to poll the status of that alarm associated with the assertion.
If we don’t find any, that’s a pretty normal case. Usually, the majority of the time, we may not see that an experiment is ongoing with an assertion, especially overnight outside the context of the business day. If we don’t see any item in the table, with ShouldPoll set to true, then we complete our execution here without proceeding. If we do find any with ShouldPolls, that’s true, let’s say we found a couple of them. Then the lambda function is going to make a request to the CloudWatch API and retrieve the current status of all the alarms that had ShouldPoll set to true. We’ll send the name and regions of those two alarms. We’ll interact with the CloudWatch API, and get back the current status of those alarms. We only want to do this for those alarms where ShouldPoll is set to true, because working in a large enterprise, there are times when you run up against your API interaction limits for AWS. While once per minute isn’t going to end up with us getting throttled by the CloudWatch API, other teams are also using the CloudWatch API in the same AWS accounts. We want to be as mindful as possible about our consumption of those APIs. Anything that we can do to limit both lambda execution time for cost purposes and API consumption on the AWS side, we’re going to try to do.
We’re going to get those alarm statuses back from the CloudWatch API. If any of the alarms were in the alarm state, at that point, we make another request to the Dynamo Table. This is where that final table column comes in, the DidAlarm. We will set that to true if we found an alarm that’s in an alarm state. Because what that means is, during the context of the experiment, as indicated by ShouldPoll is currently true, the CloudWatch alarm was alarming, and so we want to reflect in the table that it DidAlarm. We got to store this in the table, because by the time we get to the end of the experiment, the alarm might no longer be in an alarm state. We have to capture this during status. There’s nothing going on in our actual execution of the experiment that allows us to constantly watch the CloudWatch alarm status. A lot of information thrown at you there about this background process. Its primary purpose is to update the DidAlarm column in the table. Now as we move forward, I’ll talk to you about the actual experiment execution workflow and how we set ShouldPoll to true and back to false, and how we consider the results of this background process.
Chaos Test Assertions Flow
This is that Chaos Test Assertions Flow. It’s really the broader chaos experiment workflow for an experiment with an assertion associated with it. There’s 10 steps here. I’m going to go through them one by one. I know that there’s a lot on this slide to take in, but I’ll highlight the relevant portions of this diagram as we go through, and then try to give you time at the very end to digest everything that we have talked about. The first step in the process is simply, a chaos experiment was initiated. You’re going to kick off an experiment any number of ways. You may use CloudWatch Events Rules to schedule your Chaos experiment to run weekly, daily, hourly, any Cron schedule will do. A lot of teams for something like this do have their experiments running on a schedule on a regular basis, so there is no interaction from the team required whatsoever. You may also have a programmatic execution via an API, whether you’re kicking that off from a script that you have scheduled to run some other way, or you are hitting a button and executing your script. We give our engineers an API for this tool so that they have some customizability in the way that they choose to invoke their experimentation. Or, if that’s not your speed, if you’re not necessarily even an engineer, maybe you’re a project manager who wants to run an experiment, that’s ok, too. You don’t need to understand how to stand up AWS infrastructure, or how to write code. We’ve got a UI as well, where you might be able to come in and insert just a few key details about the experiment you want to run, and kick off the execution manually that way. Regardless, one way or another, an experiment is initiated. There’s an assertion associated with the experiment for the purposes of this particular explanation.
Step two I include because it does exist, but it’s not particularly relevant to the assertions. I’ll go through it quickly. Note that for the purposes of keeping this slide as simple as possible, some dependencies have been excluded. This is one of those steps where dependencies are not shown. We do a variety of pre-experiment actions and checks here. We check the global kill-switch state because we do have one of those, in the event that we maybe want to halt all experimentation in an environment. Maybe you’ve got a really important performance test going on that’s very large scale, or maybe you have a production issue that you’re trying to fix forward, and you want to ensure stability in the non-production environment for testing, we do have that kill-switch that we, the chaos engineering team can flip at any time. At this point, we also write a record of test history, so that we make sure we have a record of the fact that the experiment was initiated. We’ll update that later if we get to later steps with the result of the experimentation.
Step three is the first time that we’re really doing anything related to this experiment having an assertion associated to it. This lambda function is going to first retrieve all the information we need about the assertion that’s attached to this experiment from the Dynamo Table. We’re going to get the AlarmName for CloudWatch. Should we abort if we’re in alarm before the experiment? Should we fail if we’re already in alarm without proceeding? We’re going to get all that information out of the table, and then retrieve the current status of the alarm from the CloudWatch alarms service through the CloudWatch API. Armed with that information, we now reach decision state. If the CloudWatch alarm is in an alarm state, and the assertion configuration indicates that we should not proceed because the experiment is already a failure if we’re in alarm before the experiment, we don’t have to do anything else. We can follow that dotted line on the screen and skip to the experiment result lambda at the very end, and record the experiment as failed. Because I want to explain the rest of the steps to you, we’re going to assume that we reached out to CloudWatch alarms and the alarm is in an ok state, so we’re able to proceed to the next lambda in the Step Functions workflow.
Next up, this lambda is where we’re going to start the during phase of the experiment. We’re going to update the item in the Chaos Assertions Dynamo Table, setting that ShouldPoll attribute to true. Now that means the Chaos Test Assertions Checker separately in the background, every single minute, when that CloudWatch Events Rule wakes up, it’s going to be polling the status in CloudWatch alarms to see if we enter an alarm state. At this point, we’re setting that ShouldPoll to true. The one other thing that we do is we update that DidAlarm attribute and make sure it’s set to false, because we’re just now entering the during state anew, and we don’t want to accidentally get the result from a previous experiment associated with this assertion. We want to make sure that that DidAlarm is set to false, so we’ve got a clean slate to start the experiment. Step six, as indicated by our little storm icon here, a play off of our Climate of Chaos tools theme, it’s time for fault injection. It’s also represented by this nonstandard icon because this can look like a lot of different things. It could be an individual lambda function. In most cases, it’s actually a nested Step Functions workflow with a whole bunch of other activities inside a combination of lambdas, wait states, decision states, and maybe even reaching out to other resources not pictured. Through a series of actions and wait states, we inject some fault into the system and experience a period of time known as our, during state, which can be several minutes or even longer. Which is why we have that background process just constantly, every minute, checking the status of the alarm outside of the context of the Step Functions workflow.
Once we finish the fault injection portion, we use that lambda that we just interacted with in step five, to go back to the Chaos Assertions Dynamo Table, this time setting ShouldPoll back to false, bringing us to the end of the during state of the experiment. Back in the Dynamo Table, we had an attribute called Cooldown. In between step seven and step eight, not pictured on the screen, we’re going to have a wait state. That’s going to say, ok, we’ve completed the during, how long do we wait before we check the after status? Is it 45 seconds, 60 seconds? Is it 2 minutes, 4 minutes, 10 minutes? However long you want to wait. Then, in a lambda function, we’re going to retrieve the current status of the alarm via yet another CloudWatch API call. This is where we find out, we’re finally after the experiment, and after our configured recovery time objective. We’re going to determine what the current state of the alarm is. At this point in time, we also want to check the Chaos Assertions Dynamo Table. What we’re looking for there is the DidAlarm attribute. Because the Chaos Test Assertions Checker, that background process has been looking all along through the during state to see if it should update that attribute. We want to check at any point during the experiment, did that flip to true that we DidAlarm during the experiment?
In step nine, we have all the information we need to make the determination of whether or not the experiment overall was a success. We know if we were in alarm state before because of step three. We know if we were in alarm state during the experiment, because of the reach out to the Dynamo Table in step eight, which tells us the results that the Chaos Test Assertions Checker observed. We also know the alarm state after again because of step eight. All the way back in the beginning in step three, we have the information from the Dynamo Table, about when we should consider the experiment a failure if we were in alarm state before, during, or after. Coalescing all of that information, we finally assign a status of success or failure to the experiment. All that’s left to do after that is record the results, and end the state machine execution. This is that 10-step workflow, all the way from beginning to end without any of those highlights. You probably don’t need the numbers because it is fairly linear on this slide. Due to the decision states, the nested Step Functions, you’re welcome for not including an actual screenshot of the state machine on the screen because it does get pretty complex when you look at it in the AWS console. AWS Step Functions is one of my favorite services in AWS for its ability to orchestrate complex workflows like this one, with a nice visualization for you that follows things around. That’s a really nice trace for you where the failure occurred if you encounter an error throughout the process.
Conclusion
I do want to give one quick reminder, that you don’t actually always want this. This referring to the assertions. Not every chaos test needs one. Personally, I don’t think there’s any world in which 100% of chaos experimentation can or should be automated like this, because I think that you really shouldn’t be automating exploratory testing. Of course, you can’t be automating the testing that requires the human intervention, the testing of the sociotechnical system. If you could, then the humans wouldn’t be getting involved, and that’s a different type of experiment. The goal with something like this, is to make sure that we’re optimizing and maximizing the time spent by engineers on chaos experimentation. If we’re able to take those repeatable tests that we’ve done before, and we’ve confirmed our expectations in the past, then we free up the engineers when they do sit down for their game days to perform new and interesting exploratory tests or participate in those fire drill activities where they’re trying out incident response, preparing for on-call, ensuring that our incident response playbooks are up to date, and that their access is where it needs to be. On top of all that, if they’re spending less time manually executing chaos experiments and observing the result, they’ve got more time to actually build things, whether that’s increased system resilience to enable you to run some more tests, or maybe, once in a while, we actually build some new features.
See more presentations with transcripts
MongoDB Unveils Generative AI Features for Atlas Vector Search – Analytics India Magazine
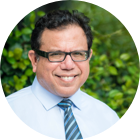
MMS • RSS
Posted on mongodb google news. Visit mongodb google news
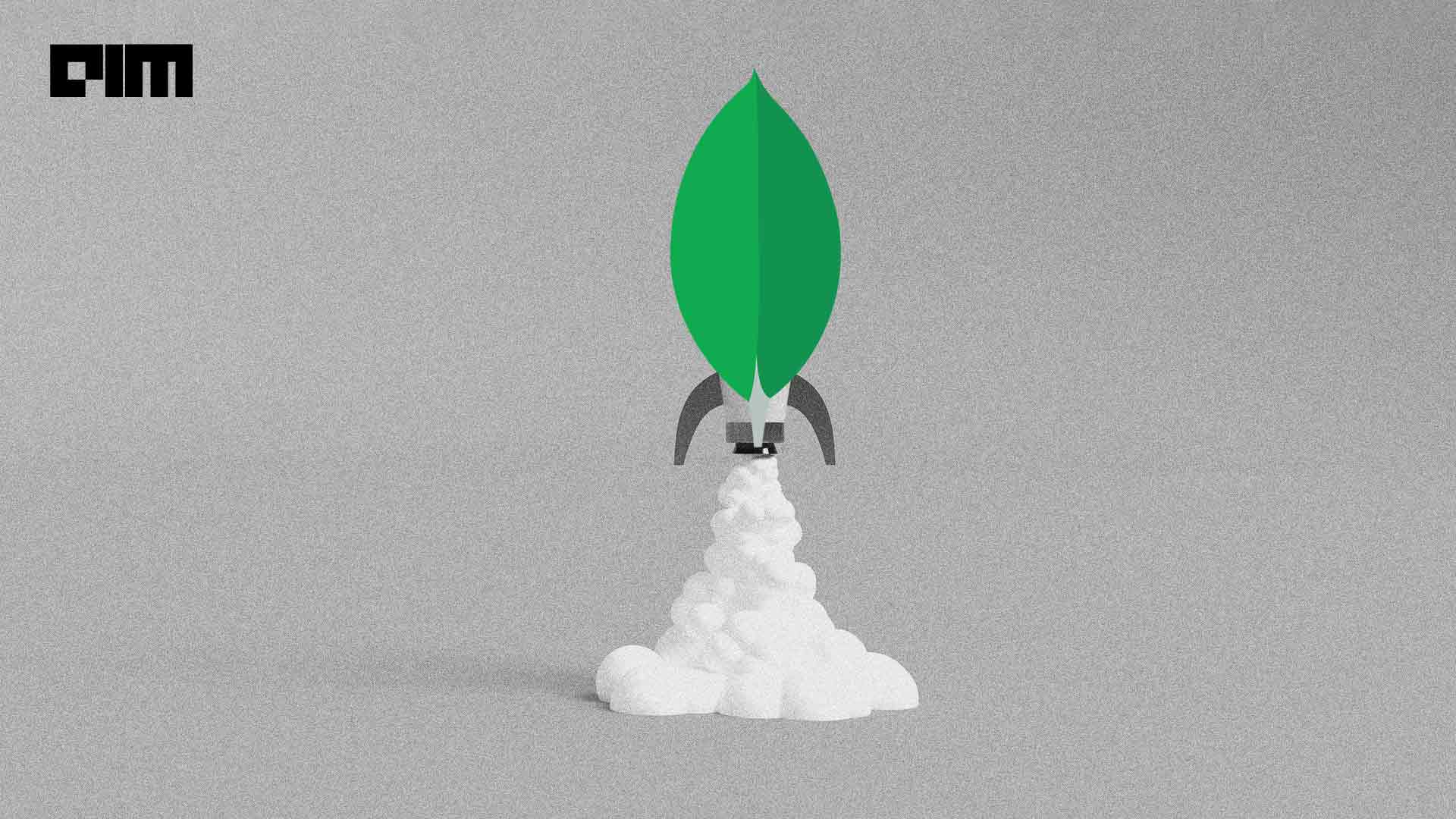
Listen to this story |
Data forms the crux of generative AI applications. And, MongoDB’s vector search capabilities are driving generative AI by transforming diverse data types like text, images, videos, and audio files into numerical vectors, simplifying AI processing and enabling efficient relevance-based searches. The company has unveiled a series of features in MongoDB Atlas Vector Search that offer several benefits for generative AI application development.
Boost Information Accuracy for LLMs: Generative AI applications aim to provide precise and engaging experiences, but they can sometimes hallucinate information due to a lack of context. By expanding MongoDB Atlas’s query capabilities, developers can create a dedicated data aggregation stage with MongoDB Atlas Vector Search. This helps filter results from proprietary data, significantly improving information accuracy and reducing inaccuracies in AI applications.
Speed Up Data Indexing for Generative AI Applications: Generating vectors is crucial for preparing data for use with LLMs. After creating vectors, an efficient index must be built for data retrieval. MongoDB Atlas Vector Search’s unified document data model simplifies the indexing process for operational data, metadata, and vector data, facilitating faster development of AI-powered applications.
Use Real-Time Data Streams: Developers can leverage Confluent Cloud’s managed data streaming platform to power real-time applications. Through the Connect with Confluent partnership, Confluent Cloud data streams can be integrated into MongoDB Atlas Vector Search. This integration offers generative AI applications access to real-time, accurate data from various sources across a business. By using a fully managed connector for MongoDB Atlas, developers can make their applications more responsive and provide users with more precise results that reflect current conditions.
Read more: MongoDB Ups the Ante with Vector Search for Generative AI
Customer Success Stories
Several organizations are utilizing MongoDB Atlas Vector Search to enhance their services. Data innovators company Dataworkz is merging data, transformations, and AI to create high-quality, LLM-ready data for AI applications, while Auto API platform Drivly is employing AI embeddings and Atlas Vector Search to empower AI car-buying assistants. Risk analytics firm ExTrac is using it to augment LLMs and analyze various data modalities, including text, images, and videos, for real-time threat identification. Inovaare Corporation leverages MongoDB to improve healthcare compliance operations, thanks to the capabilities of Atlas Vector Search in reporting and data-driven insights. NWO.ai enhances its consumer intelligence platform by integrating Atlas Vector Search to search and analyze embeddings for real-time insights. Finally, One AI uses it to enable semantic search and information retrieval, enhancing customer experiences. Cyber security company VISO Trust is also employing it to provide comprehensive vendor security information, streamlining decision-making for risk assessments.
Read more: Is MongoDB Vector Search the Panacea for all LLM Problems?
Article originally posted on mongodb google news. Visit mongodb google news
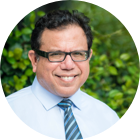
MMS • RSS
Posted on mongodb google news. Visit mongodb google news
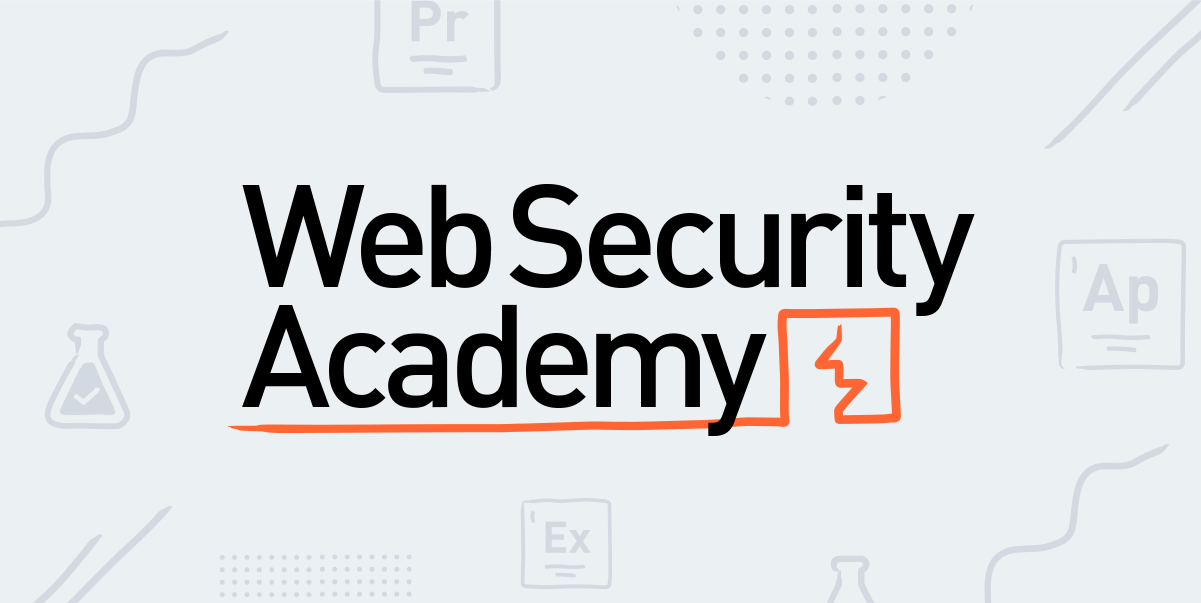
NoSQL databases store and retrieve data in a format other than traditional SQL relational tables. They are designed to handle large volumes of unstructured or semi-structured data. As such they typically have fewer relational constraints and consistency checks than SQL, and claim significant benefits in terms of scalability, flexibility, and performance.
Like SQL databases, users interact with data in NoSQL databases using queries that are passed by the application to the database. However, different NoSQL databases use a wide range of query languages instead of a universal standard like SQL (Structured Query Language). This may be a custom query language or a common language like XML or JSON.
NoSQL database models
There is a wide variety of NoSQL databases. In order to detect vulnerabilities in a NoSQL database, it helps to understand the model framework and language.
Some common types of NoSQL databases include:
- Document stores – These store data in flexible, semi-structured documents. They typically use formats such as
JSON, BSON, and XML, and are queried in an API or query language. Examples include MongoDB and Couchbase. - Key-value stores – These store data in a key-value format. Each data field is associated with a unique key string.
Values are retrieved based on the unique key. Examples include Redis and Amazon DynamoDB. - Wide-column stores – These organize related data into flexible column families rather than traditional rows. Examples
include Apache Cassandra and Apache HBase. - Graph databases – These use nodes to store data entities, and edges to store relationships between entities. Examples
include Neo4j and Amazon Neptune.
Article originally posted on mongodb google news. Visit mongodb google news
New MongoDB Atlas Vector Search Capabilities Help Developers Build and Scale AI Applications
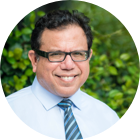
MMS • RSS
Posted on mongodb google news. Visit mongodb google news
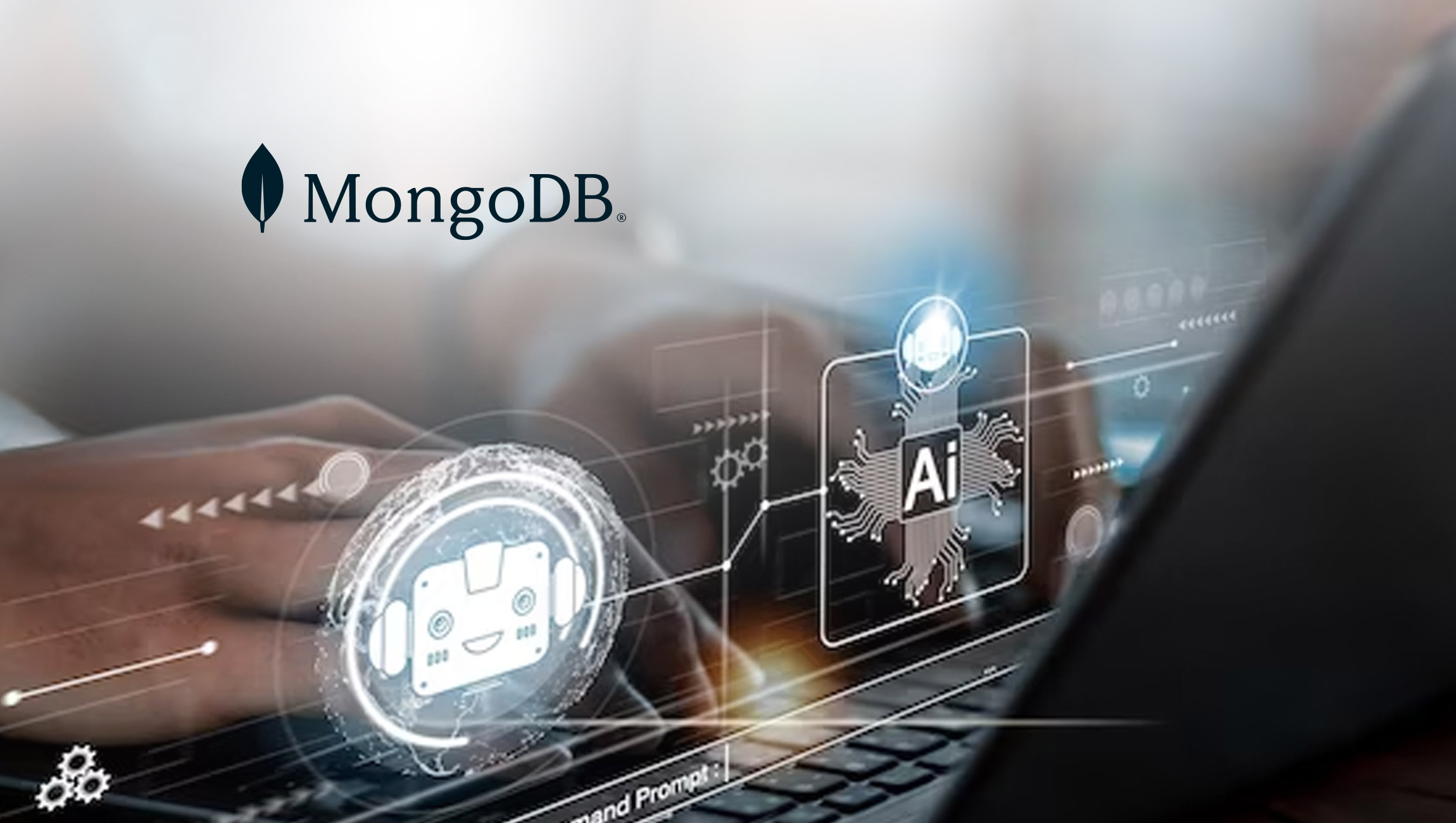
<!–
Article originally posted on mongodb google news. Visit mongodb google news
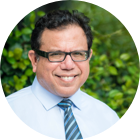
MMS • RSS
Posted on nosqlgooglealerts. Visit nosqlgooglealerts
PRESS RELEASE
Published September 26, 2023
No. of Pages: [115] | 2023, “NoSQL Market” Magnificent CAGR of 31.08% | End User (Retail, Gaming, IT, Others), Types (Key-Value Store, Document Databases, Column Based Stores, Graph Database), with United States, Canada and Mexico Region in what way to Growth and Advance Beneficial Insights from this Business Tactics, Customer Acquisition and Collaborations. A High-class Data Report Graph, which provides qualitative and quantitative perspectives on SWOT and PESTLE Analysis, Statistics On Industries, and New Business Environments. Global report Referring on governance, risk, and compliance, vertical classification, business revolution and progressions. The global NoSQL market size was valued at USD 7520.13 million in 2022 and is expected to expand at a CAGR of 31.08% during the forecast period, reaching USD 38144.35 million by 2028. | Ask for a Sample Report
Competitive Analysis: – Benefits your analysis after businesses competing for your main customers. NoSQL Market Share by Company Information, Description and Business Overview, Revenue and Gross Margin, Product Portfolio, Developments/Updates, Historical Data and more…
Who are the Important Global Manufacturers of NoSQL Market (USD Mn & KT)?
- Microsoft Corporation
- Neo Technology Inc.
- MarkLogic Corporation
- Aerospike Inc.
- DataStax Inc.
- Google LLC
- Amazon Web Services Inc.
- PostgreSQL
- Couchbase Inc.
- Objectivity Inc.
- MongoDB Inc.
Get a Sample PDF of report – https://www.industryresearch.biz/enquiry/request-sample/22376195
NoSQL Market Overview 2023-2031
The global NoSQL market size was valued at USD 7520.13 million in 2022 and is expected to expand at a CAGR of 31.08% during the forecast period, reaching USD 38144.35 million by 2028.
The report combines extensive quantitative analysis and exhaustive qualitative analysis, ranges from a macro overview of the total market size, industry chain, and market dynamics to micro details of segment markets by type, application and region, and, as a result, provides a holistic view of, as well as a deep insight into the NoSQL market covering all its essential aspects.
For the competitive landscape, the report also introduces players in the industry from the perspective of the market share, concentration ratio, etc., and describes the leading companies in detail, with which the readers can get a better idea of their competitors and acquire an in-depth understanding of the competitive situation. Further, mergers & acquisitions, emerging market trends, the impact of COVID-19, and regional conflicts will all be considered.
In a nutshell, this report is a must-read for industry players, investors, researchers, consultants, business strategists, and all those who have any kind of stake or are planning to foray into the market in any manner.
Why Invest in this Report?
- Leveraging Data to Drive Business Decisions and Identify Opportunities.
- Formulating Growth Strategies for Multiple Markets.
- Conducting Comprehensive Market Analysis of Competitors.
- Gaining Deeper Insights into Competitors’ Financial Performance.
- Comparing and Benchmarking Performance Against Key Competitors.
- Creating Regional and Country-Specific Strategies for Business Development.
- And Many More…!!
Get a Sample Copy of the NoSQL Report 2023
Why is NoSQL Market 2023 Important?
– Overall, NoSQL market in 2023 is essential for businesses to understand the market landscape, identify Growth Opportunities (Strategies, Services, Customer Base), Mitigate Risks (Economic Factors, Impact Business, Minimize Risks), Insight into Market Trend (Latest Trends, Developments, Consumer Preferences, Emerging Technologies, Dynamics, Top Competitive) make informed decisions, and achieve sustainable growth in a competitive business environment. Strategic Decision-Making (Data-Driven Insights, Pricing Strategies, Customer Satisfaction, Competitive Advantage), Validate Business Plans (long-term success of the business).
Market Segmentation:
The Global NoSQL market is poised for significant growth between 2023 and 2031, with a positive outlook for 2023 and beyond. As key players in the industry adopt effective strategies, the market is expected to expand further, presenting numerous opportunities for advancement.
What are the different “Types of NoSQL Market”?
Product Type Analysis: Production, Revenue, Price, Market Share, and Growth Rate for Each Category
- Key-Value Store
- Document Databases
- Column Based Stores
- Graph Database
What are the different “Application of NoSQL market”?
End Users/Application Analysis: Status, Outlook, Consumption (Sales), Market Share, and Growth Rate for Major Applications/End Users
- Retail
- Gaming
- IT
- Others
Inquire more and share questions if any before the purchase on this report at – https://www.industryresearch.biz/enquiry/pre-order-enquiry/22376195
Which regions are leading the NoSQL Market?
- North America (United States, Canada and Mexico)
- Europe (Germany, UK, France, Italy, Russia and Turkey etc.)
- Asia-Pacific (China, Japan, Korea, India, Australia, Indonesia, Thailand, Philippines, Malaysia and Vietnam)
- South America (Brazil, Argentina, Columbia etc.)
- Middle East and Africa (Saudi Arabia, UAE, Egypt, Nigeria and South Africa)
This NoSQL Market Research/Analysis Report Contains Answers to Your Following Questions:
- How is NoSQL market research conducted?
- What are the key steps involved in conducting NoSQL market research?
- What are the sources of data used in NoSQL market research?
- How do you analyze NoSQL market research data?
- What are the benefits of NoSQL market research for businesses?
- How can NoSQL market research help in identifying target customers?
- What role does NoSQL market research play in product development?
- How can NoSQL market research assist in understanding competitor analysis?
- What are the limitations of NoSQL market?
- How does market research contribute to making informed business decisions?
- What is the difference between primary and secondary market?
- How can NoSQL market research help in assessing customer satisfaction?
- What are the latest trends and technologies in NoSQL market?
- What are the ethical considerations in conducting NoSQL market research?
- How can NoSQL market help in pricing strategies?
- What is the future outlook for NoSQL market research?
TO KNOW HOW COVID-19 PANDEMIC AND RUSSIA UKRAINE WAR WILL IMPACT THIS MARKET – REQUEST A SAMPLE
Detailed TOC of Global NoSQL Market Research Report, 2023-2031
1 NoSQL Market Overview
1.1 Product Overview
1.2 Market Segmentation
1.2.1 Market by Types
1.2.2 Market by Applications
1.2.3 Market by Regions
1.3 Global NoSQL Market Size (2018-2031)
1.3.1 Global NoSQL Revenue (USD) and Growth Rate (2018-2031)
1.3.2 Global NoSQL Sales Volume and Growth Rate (2018-2031)
1.4 Research Method and Logic
1.4.1 Research Method
1.4.2 Research Data Source
2 Global NoSQL Market Historic Revenue (USD) and Sales Volume Segment by Type
2.1 Global NoSQL Historic Revenue (USD) by Type (2018-2023)
2.2 Global NoSQL Historic Sales Volume by Type (2018-2023)
3 Global NoSQL Historic Revenue (USD) and Sales Volume by Application (2018-2023)
3.1 Global NoSQL Historic Revenue (USD) by Application (2018-2023)
3.2 Global NoSQL Historic Sales Volume by Application (2018-2023)
4 Market Dynamic and Trends
4.1 Industry Development Trends under Global Inflation
4.2 Impact of Russia and Ukraine War
4.3 Driving Factors for NoSQL Market
4.4 Factors Challenging the Market
4.5 Opportunities
4.6 Risk Analysis
4.7 Industry News and Policies by Regions
4.7.1 NoSQL Industry News
4.7.2 NoSQL Industry Policies
5 Global NoSQL Market Revenue (USD) and Sales Volume by Major Regions
5.1 Global NoSQL Sales Volume by Region (2018-2023)
5.2 Global NoSQL Market Revenue (USD) by Region (2018-2023)
6 Global NoSQL Import Volume and Export Volume by Major Regions
6.1 Global NoSQL Import Volume by Region (2018-2023)
6.2 Global NoSQL Export Volume by Region (2018-2023)
7 North America NoSQL Market Current Status (2018-2023)
7.1 Overall Market Size Analysis (2018-2023)
7.1.1 North America NoSQL Revenue (USD) and Growth Rate (2018-2023)
7.1.2 North America NoSQL Sales Volume and Growth Rate (2018-2023)
7.2 North America NoSQL Market Trends Analysis Under Global Inflation
7.3 North America NoSQL Sales Volume and Revenue (USD) by Country (2018-2023)
7.4 United States
7.5 Canada
Get a Sample PDF of report @ https://www.industryresearch.biz/enquiry/request-sample/22376195
8 Asia Pacific NoSQL Market Current Status (2018-2023)
8.1 Overall Market Size Analysis (2018-2023)
8.1.1 Asia Pacific NoSQL Revenue (USD) and Growth Rate (2018-2023)
8.1.2 Asia Pacific NoSQL Sales Volume and Growth Rate (2018-2023)
8.2 Asia Pacific NoSQL Market Trends Analysis Under Global Inflation
8.3 Asia Pacific NoSQL Sales Volume and Revenue (USD) by Country (2018-2023)
8.4 China
8.5 Japan
8.6 India
8.7 South Korea
8.8 Southeast Asia
8.9 Australia
9 Europe NoSQL Market Current Status (2018-2023)
10 Latin America NoSQL Market Current Status (2018-2023)
11 Middle East and Africa NoSQL Market Current Status (2018-2023)
And more…………
Key Reasons to Purchase:-
– To gain insightful analyses of the market and have comprehensive understanding of the global NoSQL Market and its commercial landscape.
– Assess the production processes, major issues, and solutions to mitigate the development risk.
– To understand the most affecting driving and restraining forces in the NoSQL Market and its impact in the global market.
– Learn about the NoSQL Market strategies that are being adopted by leading respective organizations.
– To understand the future outlook and prospects for the NoSQL Market.
– Besides the standard structure reports, we also provide custom research according to specific requirements
Purchase this report (Price 3250 USD for a single-user license) – https://www.industryresearch.biz/purchase/22376195
About Us:
Market is changing rapidly with the ongoing expansion of the industry. Advancement in technology has provided today’s businesses with multifaceted advantages resulting in daily economic shifts. Thus, it is very important for a company to comprehend the patterns of the market movements in order to strategize better. An efficient strategy offers the companies a head start in planning and an edge over the competitors. Industry Research is a credible source for gaining the market reports that will provide you with the lead your business needs.
Contact Us:
Industry Research Biz
Phone: US +1 424 253 0807
UK +44 203 239 8187
Email: [email protected]
Web: https://www.industryresearch.biz
For More Trending Reports:
2023 Sesame Paste Market Latest SWOT Analysis with Booming Demand in Near Future by 2030
PRWireCenter
New MongoDB Atlas Vector Search Capabilities Help Developers Build and Scale AI Applications
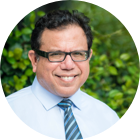
MMS • RSS
Posted on mongodb google news. Visit mongodb google news
MongoDB Atlas Vector Search now includes extended capabilities for querying contextual data and performance improvements to accelerate building generative AI applications
New integration with Confluent Cloud and MongoDB Atlas Vector Search allows developers to access real-time data streams from a variety of sources to fuel generative AI applications
Dataworkz, Drivly, ExTrac, Inovaare Corporation, NWO.ai, One AI, and VISO Trust among organizations building with MongoDB Atlas Vector Search
LONDON, Sept. 26, 2023 /PRNewswire/ — MongoDB, Inc. (NASDAQ: MDB) today at MongoDB.local London announced new capabilities, performance improvements, and a data-streaming integration for MongoDB Atlas Vector Search that make it even faster and easier for developers to build generative AI applications. Organizations of all sizes have rushed to adopt MongoDB Atlas Vector Search as part of a unified solution to process data for generative AI applications since being announced in preview in June of this year. MongoDB Atlas Vector Search has made it even easier for developers to aggregate and filter data, improving semantic information retrieval and reducing hallucinations in AI-powered applications. With new performance improvements for MongoDB Atlas Vector Search, the time it takes to build indexes is now significantly reduced by up to 85 percent to help accelerate application development. Additionally, MongoDB Atlas Vector Search is now integrated with fully managed data streams from Confluent Cloud to make it easier to use real-time data from a variety of sources to power AI applications. To learn more about MongoDB Atlas Vector Search, visit mongodb.com/products/platform/atlas-vector-search.
“It has been really exciting to see the overwhelmingly positive response to the preview version of MongoDB Atlas Vector Search as our customers eagerly move to incorporate generative AI technologies into their applications and transform their businesses—without the complexity and increased operational burden of ‘bolting on’ yet another software product to their technology stack. Customers are telling us that having the capabilities of a vector database directly integrated with their operational data store is a game changer for their developers,” said Sahir Azam, Chief Product Officer at MongoDB. “This customer response has inspired us to iterate quickly with new features and improvements to MongoDB Atlas Vector Search, helping to make building application experiences powered by generative AI even more frictionless and cost effective.”
Many organizations today are on a mission to invent new classes of applications that take advantage of generative AI to meet end-user expectations. However, the large language models (LLMs) that power these applications require up-to-date, proprietary data in the form of vectors—numerical representations of text, images, audio, video, and other types of data. Working with vector data is new for many organizations, and single-purpose vector databases have emerged as a short-term solution for storing and processing data for LLMs. However, adding a single-purpose database to their technology stack requires developers to spend valuable time and effort learning the intricacies of developing with and maintaining each point solution. For example, developers must synchronize data across data stores to ensure applications can respond in real time to end-user requests, which is difficult to implement and can significantly increase complexity, cost, and potential security risks. Many single-purpose databases also lack the flexibility to run as a managed service on any major cloud provider for high performance and resilience, severely limiting long-term infrastructure options. Because of these challenges, organizations from early-stage startups to established enterprises want the ability to store vectors alongside all of their data in a flexible, unified, multi-cloud developer data platform to quickly deploy applications and improve operational efficiency.
MongoDB Atlas Vector Search addresses these challenges by providing the capabilities needed to build generative AI applications on any major cloud provider for high availability and resilience with significantly less time and effort. MongoDB Atlas Vector Search provides the functionality of a vector database integrated as part of a unified developer data platform, allowing teams to store and process vector embeddings alongside virtually any type of data to more quickly and easily build generative AI applications. Dataworkz, Drivly, ExTrac, Inovaare Corporation, NWO.ai, One AI, VISO Trust, and many other organizations are already using MongoDB Atlas Vector Search in preview to build AI-powered applications for reducing public safety risk, improving healthcare compliance, surfacing intelligence from vast amounts of content in multiple languages, streamlining customer service, and improving corporate risk assessment. The updated capabilities for MongoDB Atlas Vector Search further accelerate generative AI application development:
- Increase the accuracy of information retrieval for generative AI applications: Whether personalized movie recommendations, quick responses from chatbots for customer service, or tailored options for food delivery, application end-users today expect accurate, up-to-date, and highly engaging experiences that save them time and effort. Generative AI is helping developers deliver these capabilities, but the LLMs powering applications can hallucinate (i.e., generate inaccurate information that is not useful) because they lack the necessary context to provide relevant information. By extending MongoDB Atlas’s unified query interface, developers can now create a dedicated data aggregation stage with MongoDB Atlas Vector Search to filter results from proprietary data and significantly improve the accuracy of information retrieval to help reduce LLM hallucinations in applications.
- Accelerate data indexing for generative AI applications: Generating vectors is the first step in preparing data for use with LLMs. Once vectors are created, an index must be built for the data to be efficiently queried for information retrieval—and when data changes or new data is available, the index must then be updated. The unified and flexible document data model powering MongoDB Atlas Vector Search allows operational data, metadata, and vector data to be seamlessly indexed in a fully managed environment to reduce complexity. With new performance improvements, the time it takes to build an index with MongoDB Atlas Vector Search is now reduced by up to 85 percent to help accelerate developing AI-powered applications.
- Use real-time data streams from a variety of sources for AI-powered applications: Businesses use Confluent Cloud’s fully managed, cloud-native data streaming platform to power highly engaging, responsive, real-time applications. As part of the Connect with Confluent partner program, developers can now use Confluent Cloud data streams within MongoDB Atlas Vector Search as an additional option to provide generative AI applications ground-truth data (i.e. accurate information that reflects current conditions) in real time from a variety of sources across their entire business. Configured with a fully managed connector for MongoDB Atlas, developers can make applications more responsive to changing conditions and provide end user results with greater accuracy.
Organizations Already Innovating with MongoDB Atlas Vector Search in Preview
Dataworkz enables enterprises to harness the power of LLMs on their own proprietary data by combining data, transformations, and AI into a single experience to produce high-quality, LLM-ready data. “Our goal is to accelerate the creation of AI applications with a product offering that unifies data, processing, and machine learning for business analysts and data engineers,” said Sachin Smotra, CEO and co-founder of Dataworkz. “Leveraging the power of MongoDB Atlas Vector Search has allowed us to enable semantic search and contextual information retrieval, vastly improving our customers’ experiences and providing more accurate results. We look forward to continuing using Atlas Vector Search to make retrieval-augmented generation with proprietary data easier for highly relevant results and driving business impact for our customers.”
Drivly provides commerce infrastructure for the automotive industry to programmatically buy and sell vehicles through simple APIs. “We are using AI embeddings and Atlas Vector Search to go beyond full-text search with semantic meaning, giving context and memory to generative AI car-buying assistants,” said Nathan Clevenger, Founder and CTO at Drivly. “We are very excited that MongoDB has added vector search capabilities to Atlas, which greatly simplifies our engineering efforts.”
ExTrac draws on thousands of data sources identified by domain experts, using AI-powered analytics to locate, track, and forecast both digital and physical risks to public safety in real-time. “Our domain experts find and curate relevant streams of data, and then we use AI to anonymize and make sense of it at scale. We take a base model and fine-tune it with our own labeled data to create domain-specific models capable of identifying and classifying threats in real-time,” said Matt King, CEO of ExTrac. “Atlas Vector Search is proving to be incredibly powerful across a range of tasks where we use the results of the search to augment our LLMs and reduce hallucinations. We can store vector embeddings right alongside the source data in a single system, enabling our developers to build new features way faster than if they had to bolt-on a standalone vector database—many of which limit the amount of data that can be returned if it has meta-data attached to it. Because the flexibility of MongoDB’s document data model allows us to land, index, and analyze data of any shape and structure—no matter how complex—we are now moving beyond text to vectorize images and videos from our archives dating back over a decade. Being able to query and analyze data in any modality will help us to better model trends, track evolving narratives, and predict risk for our customers.”
Inovaare Corporation is a leading provider of AI-powered compliance automation solutions for healthcare payers. “At Inovaare Corporation, we believe that healthcare compliance is not just about meeting regulations but transforming how healthcare payers excel in the entire compliance lifecycle. We needed a partner with the technological prowess and one who shares our vision to pioneer the future of healthcare compliance,” said Mohar Mishra, CTO and Co-Founder at Inovaare Corporation. “MongoDB’s robust data platform, known for its scalability and agility, perfectly aligns with Inovaare’s commitment to providing healthcare payers with a unified, secure, and AI-powered compliance operations platform. MongoDB’s innovative Atlas Vector Search powers the reporting capabilities of our products. It allows us to deliver context-aware compliance guidance and real-time data-driven insights.”
NWO.ai is a premier AI-driven Consumer Intelligence platform helping Fortune 500 brands bring new products to market. “In today’s rapidly evolving digital age, the power of accurate and timely information is paramount,” said Pulkit Jaiswal, Cofounder of NWO.ai. “At NWO.ai, our flagship offering, Worldwide Optimal Policy Response (WOPR), is at the forefront of intelligent diplomacy. WOPR harnesses the capabilities of AI to navigate the vast oceans of global narratives, offering real-time insights and tailored communication strategies. This not only empowers decision-makers but also provides a vital counterbalance against AI-engineered disinformation. We’re thrilled to integrate Atlas Vector Search into WOPR, enhancing our ability to instantly search and analyze embeddings for our dual-use case. It’s an exciting synergy, and we believe it’s a testament to the future of diplomacy in the digital age.”
One AI is a platform that offers AI Agents, Language Analytics, and APIs, enabling seamless integration of accurate, production-ready language capabilities into products and services. “Our hero product – OneAgent – facilitates trusted conversations through AI agents that operate strictly upon company-sourced content, secured with built-in fact-checking,” said Amit Ben, CEO and Founder of One AI. “With MongoDB Atlas, we’re able to take source customer documents, generate vector embeddings from them that we then index and store in MongoDB Atlas Vector Search. Then, when a customer has a question about their business and asks one of our AI agents, Atlas Vector Search will provide the chatbot with the most relevant data and supply customers with the most accurate answers. By enabling semantic search and information retrieval, we’re providing our customers with an improved and more efficient experience.”
VISO Trust puts reliable, comprehensive, actionable vendor security information directly in the hands of decision-makers who need to make informed risk assessments. “At VISO Trust, we leverage innovative technologies to continue our growth and expansion in AI and security. Atlas Vector Search, combined with the efficiency of AWS and Terraform integrations, has transformed our platform,” said Russell Sherman, Cofounder and CTO at VISO Trust. “With Atlas Vector Search, we now possess a battle-tested vector and metadata database, refined over a decade, effectively addressing our dense retrieval requirements. There’s no need to deploy a new database, as our vectors and artifact metadata can be seamlessly stored alongside each other.”
About MongoDB Atlas
MongoDB Atlas is the leading multi-cloud developer data platform that accelerates and simplifies building applications with data. MongoDB Atlas provides an integrated set of data and application services in a unified environment that enables development teams to quickly build with the performance and scale modern applications require. Tens of thousands of customers and millions of developers worldwide rely on MongoDB Atlas every day to power their business-critical applications. To get started with MongoDB Atlas, visit mongodb.com/atlas.
About MongoDB
Headquartered in New York, MongoDB’s mission is to empower innovators to create, transform, and disrupt industries by unleashing the power of software and data. Built by developers, for developers, our developer data platform is a database with an integrated set of related services that allow development teams to address the growing requirements for today’s wide variety of modern applications, all in a unified and consistent user experience. MongoDB has tens of thousands of customers in over 100 countries. The MongoDB database platform has been downloaded hundreds of millions of times since 2007, and there have been millions of builders trained through MongoDB University courses. To learn more, visit mongodb.com.
Forward-looking Statements
This press release includes certain “forward-looking statements” within the meaning of Section 27A of the Securities Act of 1933, as amended, or the Securities Act, and Section 21E of the Securities Exchange Act of 1934, as amended, including statements concerning MongoDB’s technology and offerings. These forward-looking statements include, but are not limited to, plans, objectives, expectations and intentions and other statements contained in this press release that are not historical facts and statements identified by words such as “anticipate,” “believe,” “continue,” “could,” “estimate,” “expect,” “intend,” “may,” “plan,” “project,” “will,” “would” or the negative or plural of these words or similar expressions or variations. These forward-looking statements reflect our current views about our plans, intentions, expectations, strategies and prospects, which are based on the information currently available to us and on assumptions we have made. Although we believe that our plans, intentions, expectations, strategies and prospects as reflected in or suggested by those forward-looking statements are reasonable, we can give no assurance that the plans, intentions, expectations or strategies will be attained or achieved. Furthermore, actual results may differ materially from those described in the forward-looking statements and are subject to a variety of assumptions, uncertainties, risks and factors that are beyond our control including, without limitation: the impact the COVID-19 pandemic may have on our business and on our customers and our potential customers; the effects of the ongoing military conflict between Russia and Ukraine on our business and future operating results; economic downturns and/or the effects of rising interest rates, inflation and volatility in the global economy and financial markets on our business and future operating results; our potential failure to meet publicly announced guidance or other expectations about our business and future operating results; our limited operating history; our history of losses; failure of our platform to satisfy customer demands; the effects of increased competition; our investments in new products and our ability to introduce new features, services or enhancements; our ability to effectively expand our sales and marketing organization; our ability to continue to build and maintain credibility with the developer community; our ability to add new customers or increase sales to our existing customers; our ability to maintain, protect, enforce and enhance our intellectual property; the growth and expansion of the market for database products and our ability to penetrate that market; our ability to integrate acquired businesses and technologies successfully or achieve the expected benefits of such acquisitions; our ability to maintain the security of our software and adequately address privacy concerns; our ability to manage our growth effectively and successfully recruit and retain additional highly-qualified personnel; and the price volatility of our common stock. These and other risks and uncertainties are more fully described in our filings with the Securities and Exchange Commission (“SEC“), including under the caption “Risk Factors” in our Quarterly Report on Form 10-Q for the quarter ended April 30, 2023, filed with the SEC on June 2, 2023 and other filings and reports that we may file from time to time with the SEC. Except as required by law, we undertake no duty or obligation to update any forward-looking statements contained in this release as a result of new information, future events, changes in expectations or otherwise.
MongoDB Public Relations
[email protected]
View original content to download multimedia:https://www.prnewswire.com/news-releases/new-mongodb-atlas-vector-search-capabilities-help-developers-build-and-scale-ai-applications-301938447.html
SOURCE MongoDB, Inc.
Article originally posted on mongodb google news. Visit mongodb google news