Month: October 2023
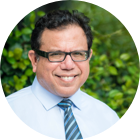
MMS • Davit Buniatyan
Article originally posted on InfoQ. Visit InfoQ
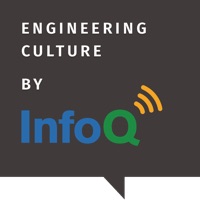
Subscribe on:
Transcript
Shane Hastie: Good day, folks. This is Shane Hastie for the InfoQ Engineering Culture podcast. Today, I’m sitting down with Davit Buniatyan. Did I get that right, Davit?
Davit Buniatyan: Yes, almost.
Introductions [00:20]
Shane Hastie: Welcome. Davit is the CEO and founder of Activeloop. He has a PhD in neuroscience and computer science, which I’m going to ask him to tell us a little bit more about. And we’re going to be talking about large language models, we’re going to be talking about AI developers, and we’ll see where the conversation goes.
Davit, welcome. Thanks for taking the time to talk to us today.
Davit Buniatyan: Shane, thank you very much for having me here. I’m super excited to be here. Unfortunately, I didn’t finish my PhD at Princeton, though I was in the computer science department I’d actually dropped to start the company, what I was very, very excited to work on. But yeah, happy to give you shared experience I went through both during the PhD and post-PhD.
Shane Hastie: Cool. So yeah, tell us the story. Who’s Davit? What’s brought you to where you are today?
Davit Buniatyan: I’m originally from Armenia, did my undergrad in U.K. And then came here to start my PhD at Princeton University, at a neuroscience lab, working on this field called Connectomics, which is a new branch in neuroscience that tries to understand how the brain works. Actually, most of our algorithm that we use today for if you heard about large language models or generative AI, et cetera, and under the hood deep learning are all based on our understanding of how the brain works from 1970s. But the neuroscience evolved so much that actually the understanding that we have in computer science, how the brain works, is way different than the current actually biologically-inspired algorithms should operate.
In our research, the goal is to figure out or reverse engineer how the learning is happening inside the brain while I was a computer scientist at the research lab. So, the problem that we were trying to solve is that we’re trying to reconstruct the connectivity of neurons inside a mouse brain. We’re taking one millimeter volume of a mouse brain, cutting into very thin slices, imaging each slice. Each slice was a hundred thousand behind a thousand pixels, and then we had 20,000 of those slices. So the dataset size was getting into petabyte scale sitting on top of AWS.
And our goal was to use deep learning and computer vision to be able to separate those images or pixels from each other to separate the neurons from each other, which is called the segmentation process, find the connections or the synapses, and then reconstruct the graph so later neuroscientists can do their own research. And during that process, that whole operation of computation on the cloud was costing us a lot of money, up to seven figure. And we had to reduce the cost by 5X by rethinking how the data should be stored, how it should be streamed from the storage to the computing machines, should we use CPUs or GPUs and what kind of models to use. And those inspired us to actually take a leave of absence from the university, and then later drop, apply to Y Combinator, get into it, move to Bay Area, and start working with early companies to help them to be more efficient in terms of their machine learning operations.
Shane Hastie: What’s it mean to be more efficient in machine learning operations?
Efficiency in machine learning operations [03:18]
Davit Buniatyan: Well, I don’t know if you’ve heard, but training the GPTs and all these large language models cost orders of tens of millions of dollars of computing costs, mostly GPU hours time, and they reach to a order of a hundred million. And if you have also seen a lot of companies now raise significant amount of money to be able to actually go and buy the GPUs from Nvidia so that they can have the infrastructure to train these large language models that go into up to hundred billions of parameters of model scale.
And that whole process is actually inefficient. So apparently when we train those models, in average case we use 40 to 60% of the GPU utilization. The rest of the time is actually being underutilized. Currently, to be fair, the bottleneck for GPU training is actually the most of it is because of the networking. So you need to synchronize the model across thousands of GPUs in a data center. However, there are also cases when you train those models, the bottleneck is actually bringing of the data from the storage to the model onto the GPU. And that’s where actually we have been focused on solving this problem.
We had actually a customer about four years ago. That was way before GPT was there. There was transformers, which is attentional, you need the paper, was only one year old. And they asked us like, “Hey, can you guys help us to train a large language model on 18 million text documents?” The current approach that they were taking was taking them two months and we said, “Sure, we can do it in a week.” And we actually took the data, started in very efficient manner on the cloud and then did a bunch of optimizations for doing this distributed training at scale on the cloud. That was four years ago. So whatever technology was there available and we reduced the training time from two months to a week project.
We cut compute costs and storage costs, and also on top of that we run an inference on 18 million text documents at 300 GPUs. So fairly, that number was large at that time, now it’s pretty small because a lot of operations are handled now in orders of thousand GPUs as you see with GPT-4, et cetera. But at that time we did a lot of optimizations, how we can do all this processing time and efficiency. And what we realized is that a lot of time the bottleneck is actually how you move the data over the data center infrastructure.
And while I worked with another company as well who was processing petabyte scale air image data, they had airplanes flying over the fields collecting a lot of air image data to provide insights to the farmers where there’s a disease on the field field or dry down area, and we help them to build the data pipelines. And what we realized is that you have all these awesome databases, data warehouses, data lakes, lake houses specialized for analytical workloads, but you don’t have one for deep learning and AI.
Deep learning frameworks [06:11]
And we looked into how PyTorch and TensorFlow, those are the deep learning frameworks that under the hood are used to train and deploy those large language models and deep learning models in general and understand how can you design the storage behind the scenes. So those models that usually take a tensor in and output a tensor out and usually tensor, just an N-dimensional array, think of it. And while you have all these formats, storages, data lakes, et cetera, table formats, they all operate on top of columns which are just one dimensional arrays and nobody actually stores unstructured data, especially like images, video, audio inside the tables, all of them put it as a blob storage on top of let’s say a cloud.
So what we did is that we said actually you can treat them as another column, but instead of being one dimensional, it can be n-dimensional or in case of let’s say images, you have width, height and also the RGB, the color channel. So then you can have a column of, let’s say if you have million images, million by 512 by 512, by three, and store this in a way on top of the cloud storage like AWS S3, or GCS, or Azure blob storage so that it can efficiently move the data over the network. Think of it as Netflix for datasets. So this really helps to reduce the time that the GPU is wasted while the data being transferred over the network to the GPU as if the data was local to the machine.
So you no longer need any distributed file system or network file system. You can directly stream the data from very cost-efficient storage, which is S3, as if it was like an in-memory database. And to be fair, people try to store images in traditional databases, even ’90s, Oracle tried to have images. But if you talk to any salesperson at Oracle, nobody even they were being best at sales will recommend you to store the images inside their database. And the reason is because storing data in memory is very expensive. And for unstructured data, for blob data, that’s why you prefer to have colder storages like object storage solutions like S3. That’s a bit on a technological deep dive, but we did Open Source it. Initially the name was Hub, but then we renamed it to Deep Lake, which is a data lake for deep learning applications, and started running webinars and building the community. And it started trending across all GitHub repositories, number two, number one in Python languages.
We built a community of thousands, hundreds of data scientist and data engineers and we really started focusing on these use cases that the data scientists today, they operate with files the same way as you operate with your laptop where you have all these different folders, different files messing around and there’s no version control, there’s not any structure; even if you operate on top of unstructured data or complex data, et cetera. And Deep Lake actually helps to take all the great learnings and understanding of how databases and data lakes should operate for data scientists mostly while focusing on deep learning applications.
Shane Hastie: What are some practical use cases? So if I think of our audience, the technologists, the enterprise software development community being asked to either implement some of these large language models, bringing in AI, or at least exploring and evaluating. What are some of the practical use cases that are there?
Practical use cases for developers [09:31]
Davit Buniatyan: So that was like two years ago where deep learning specifically was very, very for very niche audience. People didn’t know anything about GPT-4 and all the discharge GPT style applications, et cetera. So the use cases were fairly very small or niche. For computer vision, you need to do object recognition, object detection, you want to do classification, et cetera. For language modeling, you want to do semantic understanding of the text or doing search capabilities as well. But it was fairly early days.
And what happened last seven, eight months maybe a year already because of the ChatGPT and GBT-4, people started to realize that we need a database for AI. And the reason is the following, is that first of all, the large language model input is fairly limited. And to be able to overcome that problem is you need to extend the context size and for that you need a vector database. So this whole notion of vector databases has been born earlier this year. I mean it was there for last two or three years, but it became widely adopted in any enterprise software company that’s building gen AI solutions sooner or later, going to need vector database in place.
That’s number one. Number two is that because of this generative AI hype, a lot of companies need to change their strategy. Because all these large network models are doing is they’re actually commoditizing software development. A lot of companies, products that they built over the last seven years can be doable using “AI”. What it means is that while your models and the solutions become commoditized, what keeps the company moat is actually the data. So then the amount of the data that you collect becomes the castle that you built to be able to offer differentiated solutions or products to your customers or end use cases.
And that’s where the realization of, oh, now we have to actually protect our data. You have seen Reddit, Twitter, Stack Overflow, all initially being super open about their data use now they convert it into selling their data, or not letting anyone to scrape the data sets themselves. And the main reason is that because economically the data is becoming their key source of differentiation across organizations or across products, et cetera.
I didn’t mention yet totally fairly new use cases, but there are many new use cases has been born because of the generative AI. The first one is the generation of images with the models like Stable Diffusion, companies like Midjourney or as with Photoshop with their Firefly that lets you to generate a lot of content. More specifically for on the image side. You have companies like RunwayML that let you to generate videos from the images. That’s something that hasn’t been possible before.
And from the ChatGPT interface side, what was unique and different is was the first time that any person could interact with a chatbot or a language model that could have a similar level of understanding or discussion that a human would have. And that drastically changed the way perception of anyone interacting with these chatbots or large language models, which enables the use cases such as enterprise search, basically building conversation with the data that an enterprise has, or being able to do code search, or code understanding, or code generation. There are more use cases. We’ll see which ones will stick and bring the value, but I do believe that these two ones that I mentioned have the highest value impact so far in the industry.
Shane Hastie: Those are some clear use cases. What are the skills? If we think about our enterprise software developer today, what do they need to learn?
Skills that developers need to learn [13:12]
Davit Buniatyan: As it happened with web development era, we are also seeing the same, then cloud era, then et cetera. Then now we’re seeing with gen AI era, while AI, let’s say, putting in quotes “deep learning”, was for very niche audience, mostly PhD level folks, being able to train these models. Actually building generative applications became generally available. I had a one user conversation that was using our Deep Lake database and he was like, “Hey guys, you have been building this product for the last two, three years but it was only specialized for enterprises, but now average Joe (pointing to himself) has access to these AI models and access to the vector databases, and we can build solutions now”. We don’t need this advanced level of understanding, et cetera, to work operate on top of those models. So I think it changes the field in a way that any developer, or even non-developer, can interact with these large language models because they’re mostly trained on human level text, and either build solutions or use them for automating their tasks.
So there’s one big problem. First of all, this large language models, they actually hallucinate a lot of data. So when you interact with them, they can come up with the data that didn’t exist before, which is great for creative tasks, which is very bad if you’re doing any specific operation. So you as a developer who wants to build a solution now need to have some control on top of how this both the models will start to operate. So that’s where the so-called prompt engineering comes into the place, where you start to condition these large language models on very specific constraints so that the generation of the data that it outputs is fairly under control. I think prompt generation itself has been used widely because you as either a developer or non-developer, you don’t have to worry too much. You just condition, add the text, or system instructions into this large language models and you have some control.
That’s the first thing that you can do. Second thing, you can start collecting data, which is your training dataset. They can start to fine-tuning those models. I think OpenAI recently released their fine-tuning guide for GPT 3.5 that lets anyone to fine tune the model. You don’t have to go and use PyTorch or TensorFlow and load the data model. Or if you want that you can also go and take Open Source models which are also super widely available and start training your own proprietary models. And you have to be careful there with the licenses. Some of the models are commercially free to use, some of them are very restrictive, but the whole of this Open Source movement is also trying to catch up with private organizations like OpenAI to be able to get similar accuracy what GPT-4 is providing on a closed source manner.
The way we build software changes with these models [15:50]
And then you get into understanding, okay, how the vector databases will work. In fact we realized that there’s a gap between this, think of it as a new way of thinking versus the old way of thinking, where the understanding of how you need now need to start building software is also going to change. And there are now pretty famous frameworks like Langchain and Llama Index, they help you to connect a lot of tools together and connect those tools into large language models so you can solve your problems. And we actually in fact released free certification course in collaboration with Intel and Towards AI, which has a big community of data scientists and users that helps you to go from zero to hero. And the main difference of being this dummy for generative AI course, it’s actually established on building useful use cases that you can take it in your enterprise.
Shane Hastie: So let’s dig a little bit into that course. As a professional developer, I’m probably proficient in Java. In my case I was very proficient in C and C++, I will admit not anymore. But if I wanted to get into this space, I’ve got that programming background. I understand relational databases, I understand front ends, middleware, backend, so forth. What are you going to teach me?
Learn to think about systems differently [17:10]
Davit Buniatyan: Yeah, let’s say the full stack engineering development background. Well first of all, I think in terms of the languages, apparently most of the AI development have been happened on Python, and now there’s also a lot of popularity on JavaScript as well. But the main thing is that I think that you’re going to learn already the insight is that if you want to get from A to B instead of building all these heuristics, you’re going to think about systems differently, about those models that you need to technically teach them how to operate in life. And it’s very similar to what happened with self-driving cars like five, seven years ago, it was so easy to build a prototype self-driving car, put it on a street, took your video camera, how this is driving over the street, but you would never trust this to put on a highway, and sit inside. And the main reason was actually these edge cases.
What it took both companies like Cruise, Tesla, and Waymo over the last few years is to collect a lot of edge case data to be able to fully scope out what does it mean to actually drive on a street in any place. I don’t know if you’ve watched recently on Elon Musk taking a live recording of driving him Tesla in Palo Alto, but there was a very key interesting insight. So this is the first time they built an end-to-end neural network that drives the car. And there’s not any human engineer wrote If… Else Statement how basically the car should drive. And the Tesla was not stopping at the stop sign. Now you have to wait until one to three seconds. So what they find out from the data they collected from Tesla cars is that in average a human stops at a stop sign using Tesla just 0.5 seconds.
How do you solve this problem? Okay, you can’t just drive illegally. So one fair solution was like hey, you can go and add an If statement, say “Hey, if you see a stop sign, you’re going to stop here.” Wait, I don’t know, one or three seconds before you drive. But the way they solve this problem actually was totally different. They said, “Okay, let us go and collect more data where good drivers are stopping long time on stop sign and feed this data an ops sample manner to the model when we do the next training.” So the way you think of building systems and software is also changes. You’re not thinking, “Hey, let me go in myself condition this edge case and solve this problem.” But you think more a systematic approach, “Okay, how can I collect more training data to teach these models to do what I want them to do in a good manner?”
And why self-driving is not yet a solved problem is because nailing down this last mile of edge cases is so tough problem. It’s like humans are incapable of writing all the conditions that a car potentially can drive through. You can throw hundreds of engineers on this problem, but maintain this software and put all these conditions on different scenarios and all their combinations becomes super, super difficult. And that’s where developers now becoming more like teachers how to tune this junior engineers to do useful work. It’s fairly early times you should not expect wonders to happen from these models, but the incremental updates that we are seeing especially over the last year, but it has started actually five years ago, or 10 years ago is we can predict that the change is going to be dramatic next few years, but it will take some time to nail down all these rough edge cases with large language models.
I can give you an example as well here. If you heard about this retrieval augmented generation, which is a fancy word for search use case where let’s say you have million documents and you want to be able to text and ask questions and find these million documents. A more specific example is, let’s say, you have all the reportings, quarterly reportings of Amazon for the last two years, and you want to be able to ask questions like, “Hey, what was the revenue for the last quarter?” Which is simply a straightforward question that can be answered by a ChatGPT, or GPT-4 having all the context inside. And for you to be able to implement this solution, you have to build, use a vector database, get all the data into vector database and then run a query.
However, let’s say you ask this question, give me the quarter reportings of last three quarters, and not any vector search can solve this problem. What you have to do is you have to go to each quarter, get the revenue, take the last three quarters and then sum them together and give this to the GPT-4 so that they can synthesize the final answer to you.
Hallucinations leading to mistrust [21:34]
So this is just a one very basic example where no any search or basic search or large language can answer this question. Apparently if you take all these edge cases, if you get to 70% of accuracy answering questions across the data, that’s already remarkable. And the biggest problem is that when the ChatGPT, or GPT-4, or any large language model foundation models don’t have the right enough context, they start hallucinating the data, and they come up with new information. So for us, will you trust the system to be in a hospital working with a physician, like having all your records data and maybe some other records and give you 70% accurate answers for your diagnosis? Fairly no, right? That means that we still have the time to really make those systems really production grade, enterprise grade deployable into highly sensitive critical situations.
Shane Hastie: From an ethics perspective, I want to jump on that and say, but people are already doing it.
Davit Buniatyan: Let me ask you, doing what?
Shane Hastie: So the hype, what we hear is, and maybe this is the hype cycle playing out, but 70% accuracy, but we trust the results.
Davit Buniatyan: Do you all be wondered how many times we have junior team members, we ask them, “Hey, go and figure out this problem.” And they come up with a solution, they come to me is like, “Hey, we figured out the solution.” But the solution looks fairly wrong and it’s like, “How do you get to this answer?” And then they say, “Oh, this is the reasoning.” It’s like, “No, no, no, this is not way possible.” And they start arguing back why this is possible. And ask at the end, can they bring a proof of GPT-4 said this, and they put a screenshot in front of me. And I’m like, you can’t do that. You can’t put this in the context of building. You know how people started trusting Google and internet and Wikipedia for a lot of questions. Obviously most of them are right, most of them, but there are these edge cases are very specific cases that the domain expert or knowledge can actually outweigh this misinformation that exists in the common knowledge.
So in our case it was not critical application, but yes, you’re right. If you ask, you’ll be wondered how many people now already take the ChatGPT answers as facts. As factual information, you can use it for actions or any items which is knowing how things work behind the scenes and how they have been trained. You’ll never ever do that. Though it’s not saying that they’re not useful. Actually we already passed six months of this hype cycle, and fairly the hype is still going up. So we haven’t came to that point of realization, oh, we have these over expectations across this technology, and fairly we’re going to go down. I feel like we’re going to go down in terms of the expectations at some point and then the most useful use case is going to evolve and become mainstream. But certainly everyone says this as well. This is a totally different wave compared to the blockchains, and more similar to what happened with mobile revolution, with dotcom era, et cetera. So how the internet started in the early days.
Shane Hastie: Where are we going?
Where are we going? [24:38]
Davit Buniatyan: Well, definitely at least to what I believe is not the Terminator-style AI revolution, I think that’s the one side of false future that’s not going to happen. And then on the other hand, you have nothing going to change. We still developers going to continue building the software ourselves like using Java, Python, whatever current language do we use, et cetera. I think there will be certainly changes and those changes going to have huge positive impact with obviously all the negative consequences taken consideration into.
For example, at least on myself, I see that me having a lot of experience writing a lot of software using the large language models really helped me with code development. Saved so much time writing all this nonsense code that I have to write to get there that automates for me. That’s just me using it for a year. I tried, by the way, using this two years ago and the state was fairly not there. I tried this about three years ago with fairly small models and the auto-completion was nowhere near to the usefulness that we have the systems now deployed at scale, so at least we have got this usefulness.
The second thing is I think a lot of interface is going to change. Now you go and give everyone access to generate code and do that in fairly conversational way they’re used to, and you’ll see a lot of non-technical people will become technical or at least leverage these technologies to uplevel their skills. This could be just even learning from the foundational models so that you can take it from educational perspective. I do believe the large language models will have a huge impact in education space, especially this will let you to get personalized education for each student depending on their speed and performance, how they’re moving forward.
For one student you can teach basic math. The other student’s super excited into quantum computing and explain how the quantum theory works. So then you can have personalization there and this will definitely have impact in automation. We have seen companies like UiPath, et cetera, on RPA side that help you to automate certain very manual tasks on the computer. And this is the next level where you can actually write a text and ask to generate a plan and start executing this plan, which is this notion of agents, what that operate on self.
Actually, there was a very interesting story recently that Stanford published this simulation life where you have 16 different agents, all of them connected to a large language model living into a very simulated small space. And you give a task to one of these agents, like organize a birthday party for your next day, and then what you see this agent, which all the agents just use behind the scenes large language models, you see this agent goes and asks nearby agents like, “Hey, can you help me with organizing the birthday party?” And the other agent says, “Yes. So I will do that. Let me start creating these invitation letters and inviting all other 14 agents to the birthday party.” And some of the agents say, “Hey, I’m busy, I can’t come tomorrow,” et cetera. And they organize this birthday, which is very exciting. You have this kind of a simulation style life where you have these agents working.
On the other hand you have projects like AutoGPT and BabyAGI, which had a lot of excitement in the space, but they went down because of the error rate they operate. A very simple prompt can actually have a butterfly effect on their reasoning and how they think. So they’re nowhere close to be fault tolerant. And I had a friend of mine called me and said, “Hey Davit, I’m really thinking to automate …” He’s in the trucking industry. Trying organization of these phone calls with the truck drivers, et cetera, using AutoGPT. And it’s like, “Wait a second, that’s nowhere … You’re calling me one week after the AutoGPT was released, it’s like we are nowhere close. I tried this tool. It’s like we are very, very far from this putting into production usage.” So I think if you ask me how the future looks like, we are going to have a lot of now developers thinking and building generative AI applications which are ready to deploy in real life in production, but the development itself of those applications going to be different than what we used to do before.
Shane Hastie: As a developer, my job is not at risk, but I need to learn something new. That’s been the case in development since I started in 1982, and it was happening a long time before then too.
AI as assistant rather then replacement [29:03]
Davit Buniatyan: We have senior engineers in our team. They’re like, “I’ll never use ChatGPT to advise me on how to write the code. I have been doing this last 30 years,” or 20 years. Who also touches our ego as well is like, “Oh, there could be an AI that writes better software than us, how this is possible?” And I do think that the most efficient look into this, that the current generation of AI at least is going to be more assistance, and your pair programmers and going to continue where the humans lack the, not just the experience, but also … I myself, I can write code six hours per day, but then I get super tired, and I can do so stupid mistakes. I believe every developer does these stupid mistakes. At least it saves the time on searching across Google and Stack Overflow so you can be more efficient.
But basically what I also found is that it can really boost you in very highly precision certain tasks that you know roughly that these things should work like this, but you don’t have an experience doing this. So it boosts senior engineers to be extra senior. It enables the junior engineers to have all this knowledge that they lack or missed before, and then it helps the non-developers to become developers. So you get this boost across all the fronts and you don’t have anyone get replaced here, just get enabled at scale.
Shane Hastie: Thank you so much. Some really, really interesting thoughts and ideas, and thoughts about the future. If people want to continue the conversation, where do they find you?
Davit Buniatyan: Well, they can find me on Twitter, LinkedIn, all the social networks. Fairly, also do a lot of work at Google AI. We have a blog that we publish, a lot of use cases and case studies with folks that we are working on. And I think today the best thing you can do is contribute to the Open Source and learn from the Open Source. And that also will uplevel everyone in terms of what’s happening in the AI space especially.
There’s a big difference to what’s happening now versus what happened 10 or 20 years ago is that AI and Open Source are intertwined together, and this really boosts the innovation. I know this is a longer answer to what you asked for, but it’s one more exciting thing I want to share is that I was talking to one of my friends at a large company, I won’t share which one. But they basically spend maybe last four years on working on these generative AI applications generating images, and they definitely have state-of-the-art models that no any competition had. But they left that aside and said, “Hey, let us just switch to Open Source.” Even though the Open Source models, they lack some performance and accuracy, the change that’s happening, they’re definitely going to outweigh what we are doing internally. So now our common wisdom of building the systems and models together is way more than any private organization can do. So I think we’re living one of the exciting times in the whole humanity life horizon that we had. And yes, super excited to be part of this.
Shane Hastie: Love it. Thank you so much.
Davit Buniatyan: Likewise, Shane. Great chatting with you and thanks for the questions.
Mentioned
.
From this page you also have access to our recorded show notes. They all have clickable links that will take you directly to that part of the audio.
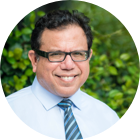
MMS • Olga Sermon
Article originally posted on InfoQ. Visit InfoQ
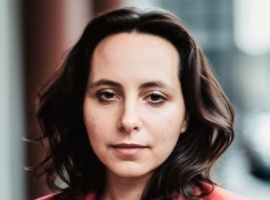
Transcript
Sermon: My name is Olga Sermon. I look after the platform teams at Superawesome, that’s infrastructure and data platforms. I’ll be talking about our journey from DevOps consultancy, to building platform as a product. I’ll explain what it means to us to be a platform product team, how we work with our users, and how we make sure that what we build is actually interesting to them and gets adopted. First, a few words about Superawesome. We are a kidtech company. Our mission is to build a safer internet for the next generation. In practice, this means that we’re building tools for creators and developers to enable them to engage children and young people online, safely and securely, and with respect to their privacy. In 2020, we were acquired by Epic Games, and our products are now built into enormously popular games such as Fortnite, and some of them such as consent management are available for free for all developers from Epic online services.
The Days of the Heroes
In the beginning, our team was really tiny, first two, then three and four people. Our function was to provide DevOps services to other teams. Those were the days where our team was on call every night, and people were actually called most nights, in fact, multiple times every night because the budget was so tight, we used to do really silly things like run databases on Kubernetes. We were very close with our customers. There weren’t that many of them, just three other teams. We used to attend every standup every day. We used to attend most design discussions, and draw them a lot of the time. We were there for every big launch, setting up things like deployments, and observability for all new services. In other words, we used to act as a DevOps consultancy. Some people genuinely enjoyed those times. They had an opportunity to act as heroes but it wasn’t a sustainable way to work, and we lost some very good people in those days. Most importantly, this approach just didn’t scale. As the company grew, it was no longer possible to be so close to all our customers.
Cognitive Load
In 2019, a book came out called, “Team Topologies.” The book has revolutionized the way we think about organization design, and DevOps teams in general. It stated that we can significantly increase the speed of delivery if we optimize for two important parameters, cognitive load, and team autonomy. Let’s look a little bit closer into the concept of cognitive load. There are three types of it. First, is intrinsic cognitive load. That’s the skills necessary to develop the application. If we’re developing over web, intrinsic skills would be finding the library to manage connections or to establish secure connections. Then there is extraneous cognitive load. That’s the mechanics of development. For example, for a web app, it would be skills necessary to deploy it to production. Finally, there is Germaine cognitive load, that’s domain knowledge. For our web app, it would be the purpose of the app. If we’re building something in app tech, that will be app technology.
Types of Teams
Team Topologies introduces four different types of teams. It all starts with stream-aligned teams, also referred to as product teams. Their purpose is to deliver value to the customer. They are what comprises majority of the teams in modern companies. Then there is enabling teams. These are teams or individuals, they have specific knowledge. Their purpose is knowledge sharing. For example, an agile coach in most companies will join different teams and help them set up their agile development process. There is complicated subsystem teams. These deal with exactly that, complicated subsystems, such as authentication system, or sometimes a data warehouse. Their job is to deliver the system as a service to the product teams. Then, finally, there is the platform teams, us. Their job is to create tools to enable stream-aligned teams to be autonomous by reducing extraneous cognitive load. This means that things like deployment and observability stops the product teams feeling like this, and start feeling like this. At least, that’s the dream. That’s what we’re aiming for.
What Is Building Platform as a Product?
SA Platform was born. Our mission was to provide training and tooling to enable Superawesome engineers of all experience levels to provision and run infrastructure independently, quickly, and easily, in a consistent, scalable, and secure manner. In other words, we have set off to build a platform as a product. What does it really mean to us? What is it to build infrastructure platform as a product? There are a few key things about product that we need to bear in mind. First of all, a product is self-service. This means that our users should be able to use the product independently without us holding their hands, telling them what to do, or making any decisions for them. It doesn’t mean that we can’t interact with our users at all. It does mean that the nature of the interaction changes from knowledge sharing, to learning. When we just discover a problem, and we find the best approach for it, we will interact with the users quite closely, often shadowing and doing what we need to learn about their needs. Then we will run a discovery and deliver our small solution to them to see if we understood what they needed correctly, and if it would work for them if they would be able to use it. Once we have established the right solution, we will move away and deliver the solution as a service to enable them to work independently.
A product is something that’s flexible. It’s something that evolves and takes advantage of new technologies and user feedback. We now have a roadmap. It isn’t just running from fire to fire anymore. This roadmap is actually user driven, where we present our ideas to the users and collect their feedback about them continuously. Our product is optional. It means our users can choose to use it or they may choose to go to market vendors. This means that our product needs to bring clear value, and it should be fun and easy to use. To help us with that, we collect continuous feedback about our tools and services, about what it’s really like to be able to use them. Finally, a product is something that’s measurable. It has a clear set of features with clear boundaries, and it has measured outcomes. Team Topologies actually goes as far as defines a perfect set of platform metrics. First of all, we have to measure the product metrics themselves. Do our tools do what they’re meant to, and how well they’re doing it. Then we collect user satisfaction scores, or NPS score. We collect reliability metrics. These are very important. It’s imperative that the platform is stable. The stability of the platform is what empowers our developers to experiment, and create software safely. Finally, most importantly, we collect adoption statistics so we can understand if we have been successful. If what we have delivered actually brought value, or if we need to go back and iterate on that.
How Do We Enable Adoption?
How do we go about it? How do we enable our platform adoption? Just like with any product, if we want something to be adopted, if we want something to be purchased by our users, we want it to be attractive, so we want to make sure it actually brings value to them. We want it to be affordable in terms of cognitive load. In other words, we want to reduce the price of adoption as much as possible. We want it to be accessible. In other words, we want to make sure that it covers enough use cases to be interesting to as large user base as possible. I’m going to cover what we have tried so far, what we’re hoping to try. I’ll explain what worked and what didn’t work as well, for us.
How Can We Make the Platform Attractive?
Our first approach to our platform adoption was really quite simple. We thought that we could just build it and tell the customers to use it. After all, we have been working with them very closely for a very long time, we knew exactly what their needs were, so why wouldn’t that work? This is how we went about building something called the Matrix. We can see a screenshot of that on the slide. The Matrix project was approached in the spirit of the typical developer dream. When the developer goes away, and then builds something absolutely astonishing, and everybody is so impressed, they fall in love with their creation from the moment they lay their eyes on it. The matrix was meant to be a service discovery tool. It actually was a really good idea. It would bring a lot of values to our new joiners learning about our systems. It would bring a lot of value to teams like InfoSec, because it recorded all the technologies that our services were using, and it would have been easy for them to identify if something was compromised by a security vulnerability. It was also very difficult to use because all these details were meant to be filled in manually and unabated on regular cadence. We suggested three to six months. We spent a very long time building that, months actually. Did a lot of research about our services and about the needs of various compliance and legal departments. When we presented it to our users, they didn’t quite fall in love with it. In fact, they hated it so much, they complained about it. None of them bothered to field it.
Our next approach was a bit more user driven. We figured the easiest way to build something that our users want is to just ask them what it is they desire. We issued a user survey. We asked our users to propose a solution to explain what problem it would solve, and to invite them to comment on each other’s solutions. That has produced a lot of results. A lot of people went to the trouble of recording their suggestions for our team, but it didn’t quite work as well as we hoped because most of the suggested solutions were just specific to the needs of particular individuals and their teams. While it would definitely be something that our users desired, only a very small percentage of the users desired each particular solution. We knew we had to try something else, and the next thing we tried was looking at it from a different perspective. We looked at it from the problem perspective. Instead of asking our users to tell us which problems we need to solve, we used our knowledge of the system to propose which problem we wanted to solve to them. We would explain the problem statement, the goals of the solution, and the use cases. We would list some possible solution candidates. Then we would invite our users to comment on that. On one hand, we picked a problem that was relevant to a considerable portion of our user base. On the other hand, we were developing the solutions in collaboration with our users, immediately addressing the value risk before any engineering effort was invested in the solution. Out of all the things we tried, this approach worked the best and we have been using it now for several years.
How Can We Make the Platform Easy to Adopt and Use?
How can we make our platform easy to adopt and use? Of course, we have to reduce the price of adoption. We have to reduce the cognitive load of learning how to use it. There are a number of tools that can help us. First of all, we have to consider the usability risk as early as possible. It’s very difficult to make a feature more usable once it’s already in production. You really have to consider usability right at the beginning of the design stage. You have to build it into your POC, the proof of concept. You want to automate as much as you can. Zero effort adoption is the easiest adoption possible. Finally, there is one other method of adoption, that’s adoption by default. We try to avoid that for things that users can see, because we want them to maintain their autonomy. For things that are happening behind the scenes, it’s just perfect. They don’t need to worry about them at all. They just quietly, magically happen. For example, if we’re talking about an autoscaler, that helps them to find their instances quicker, and cheaper, and in line with their reliability requirements. In order to address the risk of usability, we use journey maps, just starting to use them really. It’s when we write down everything that these are all the user actions, and we consider their interaction with the system from several different perspectives. For every step of the way, we think, who owns it? What the user is doing during those steps. What are their needs and pains? What do they want to achieve in that step? What do they want to avoid? We think about touchpoints, that is the part of the system the users interacts with. We think about their feelings. Based on all that, we think about opportunities, things we can do better during that stage.
Another important tool that really is key to adoption is documentation. If the users can learn how to use it, they will be more likely to adopt it. Of course, documentation needs to be self-service, meaning it should be easily discoverable and our users should be able to understand it on their own without any help from our engineers. We also have to live with something called example application, a quick start service. It’s literally a button that they could press and get the service running in production in about 15 minutes. The service, of course, is very simple. It’s just a Hello World web page app. It does have things like deployment pipelines built in. It does have quality scanning. It has observability. All the nice little bits that a service should really have. One other thing we thought about but we haven’t tried properly yet, is a CLI interface. It’s a nice, easy-to-use tool for engineers. Something that they are already used to. Potentially, it could provide a single interface to all our tools and documentation.
I would like to say a few more words about documentation, because it is so important. We treat it as a first-class deliverable. This means we’re segregating staging and production. Documentation is delivered to our internal space first, where people can review it and make suggestions. Once we are all happy with it, then it can be promoted to a place where the users can find it. We audit it regularly because it does go out of date ridiculously quickly. We try to make it as simple as possible. Most of our user facing documentation doesn’t assume any prior knowledge of the platform. We stick to consistent formats. Every section will have an overview. It will have some how-to guides. It will have references, for example, common error codes, and troubleshooting. Finally, there is a little form at the bottom of every page where we are asking users for feedback.
When everything else fails, the users still have to be able to find a way to use our products. We want them to be able to come to us for support when they need it. We define clear support interfaces with a clear remit of responsibilities. There is a page on our documentation space, which we maintain religiously, which defines which parts of the infrastructure we provide, and where we are relying on the product teams themselves. Finally, there are several support channels for different types of requests, and they have different support workflows, from very urgent where the users can get help within 15 minutes, to medium where most of the questions are resolved under an hour, to longer term requests, things like requests for new features and consultant requests. These might have a timespan of several days, up to weeks depending on the request really.
How Can We Make the Platform Accessible?
The last question I want to talk about is, what we do to make our platform accessible. What we do to make sure that it covers enough use cases to be useful to most of our users? This is actually a very difficult balance to strike. For example, several years ago when people first approached us about serverless, we said no to them, because it was too exotic, and it was not really used in production. However, when people approached us again this year, we agreed to extend the platform to serverless architecture because it became more viable and more teams were interested. What do we do? What do we use to find whether something is worth adding to the platform? There are a number of tools we use to work with our users. First is a user survey. It’s an anonymous survey, where we ask them about our tools, how easy or difficult it is to use them, and if there is anything missing, if there is anything else they would like us to do with the platform. We run interactive sessions with our users, shadowing them. just doing their day-to-day work. Trying to learn what it is they’re dealing with and what problems they’re facing, even if they don’t notice these problems themselves, because they’re so used to doing them. For example, I learned loads recently, when I shadowed somebody creating a new service. We have a user steering group. These are quite interesting, and I would like to go into a bit more detail there.
First thing when creating a steering group is to find a representative sample of our users. We try to make sure that there is both senior and junior engineers. We try to make sure that we have both people who have been with the company for a while, and new joiners. We try to make sure that there are people from different business units, just because people do things slightly differently, different teams. If the user base is very diverse, it’s probably worthwhile running separate user steering groups, just so you can address their needs more effectively. Because every meeting with a user group needs to have clear objectives. We want to tackle a specific use case, or a specific problem, for example, how do you deploy a new service? Of course, these are feedback sessions. It’s actually non-trivial sometimes to listen to their feedback. When people talk about their day-to-day work, they tend to talk about it in absolute terms, providing unconstructive positive and negative feedback. Our job is to dig into that, is to ask, can you please help me understand, what makes it difficult to deploy a new service for you? Or, what do you find most exciting about it? Then we follow up and we make sure to find the success stories and to tell them, because our user groups are also our champions. There is an interesting pattern in running user groups because of it. Generally, we have a few sessions where we try to understand the problem we’re trying to tackle. Then we go away and we find a solution. We engage with the users again, to understand that the solution is working for them, and then eventually deploy it to production. Then there will be a break until we find another tricky problem to solve, and we need to get our user group together again.
Conclusion
Building platforms is a very interesting and a very challenging enterprise. We’ve tried a lot of things over the years. Some of them worked well. Some of them didn’t work as well. That’s what we’re going to do in future as well: keep trying new things, keep finding what works best.
See more presentations with transcripts
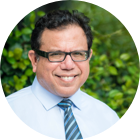
MMS • RSS
Posted on nosqlgooglealerts. Visit nosqlgooglealerts
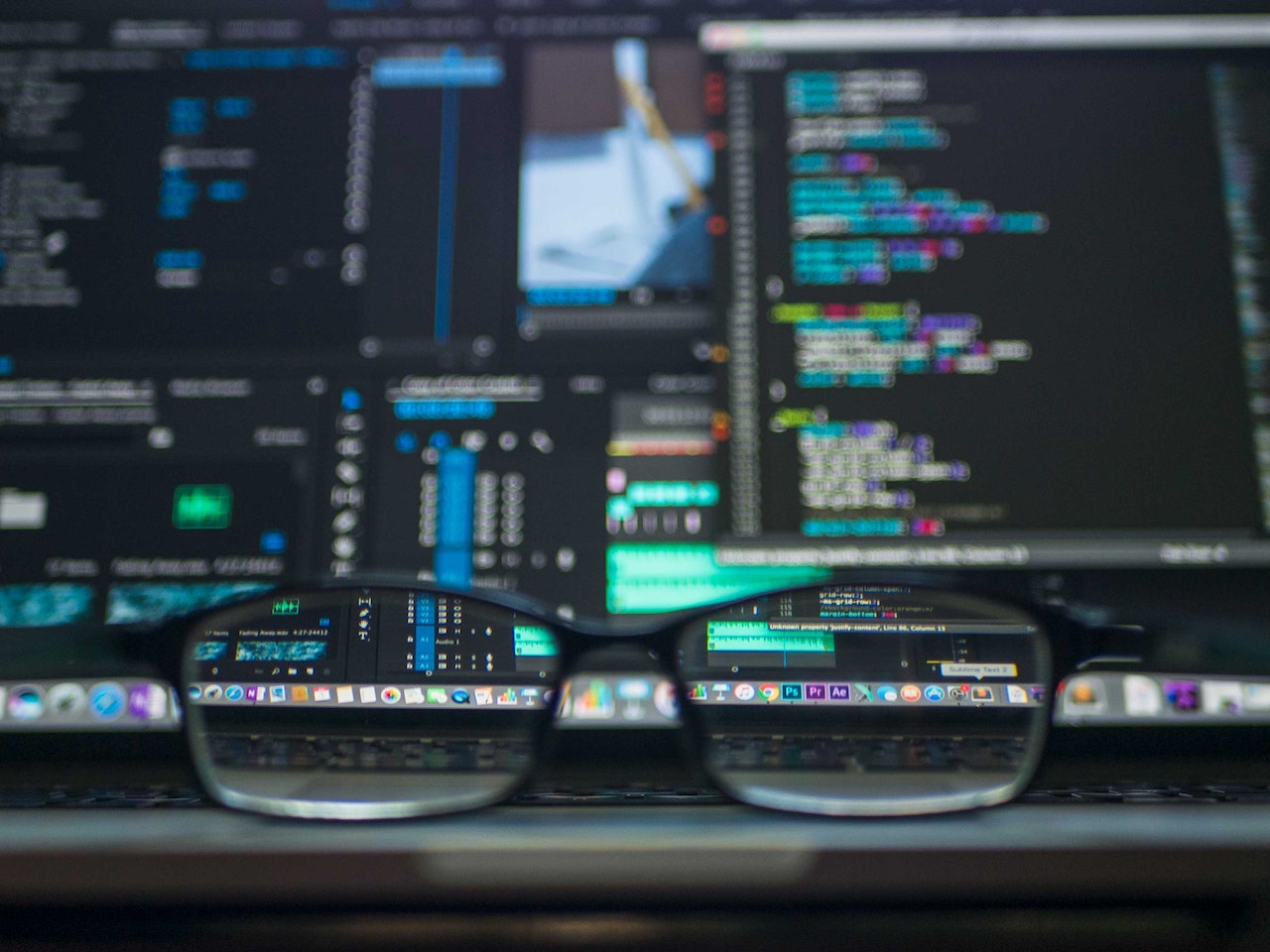
NoSQL databases have carved a niche for themselves in the world of data management, providing an alternative to traditional relational databases. In these diverse and unpredictable data settings, NoSQL databases excel as they provide a scalable base for efficient data management and storage.The data-driven industry, including e-commerce, social media, and big data analytics, depends on NoSQL databases to meet dynamic data needs.
Today we will dive into the core principles of NoSQL databases, discuss the diverse categories within the NoSQL industry and spotlight some of the standout databases that have gained widespread recognition. Whether you’re a seasoned database administrator looking to stay on top of the latest trends or a fresher looking to explore new horizons, this blog is your one-stop resource for all things NoSQL databases.
1. MongoDB
MongoDB offers a high degree of flexibility in its data management approach. It utilizes documents that can encompass sub-documents within complex hierarchies, providing an expressive and adaptable structure for data storage. This NoSQL database is kind of like a digital filing cabinet for your information. Instead of using the classic tables and rows you’d find in a regular database, MongoDB keeps everything in what it calls “documents.” These documents are like little folders where you can put all your data, and they’re stored in what’s called a “collection.” So, instead of having separate tables for different kinds of information, you can just toss them all into collections as documents. The best part is that MongoDB speaks the same language as your web applications, using JSON-like documents.
MongoDB seamlessly maps objects from various programming languages, ensuring an effortless implementation and maintenance process. Moreover, its flexible query model enables users to selectively index specific parts of each document or craft queries based on regular expressions, ranges, or attribute values. This approach accommodates as many properties per object as needed at the application layer. Native aggregation features empower users to extract and transform data within the database, which can then be loaded into new formats or exported to other data sources.
2. Apache Cassandra
Cassandra, an open-source software developed by Apache, is freely available for use and consists of a community focused on Big Data. It seamlessly integrates with other Apache tools like Hadoop, Apache Pig, and Apache Hive. With its peer-to-peer architecture, Cassandra operates as a collective of equal nodes, enhancing fault tolerance and enabling effortless scalability. Its high availability, fault tolerance, and data replication ensure reliable data storage.
Its high performance and column-oriented data storage make it ideal for data-intensive organizations, while its tunable consistency levels allow users to choose the desired data safety and replication options, accommodating various use cases. The NoSQL database offers a cost-effective, community-supported, and flexible solution for managing and storing Big Data. Its strong attributes make it a good choice for organizations that want to manage data efficiently and effectively.
3. Couchbase
Couchbase has several notable advantages. Firstly, it excels in maintaining strong data consistency and durability, ensuring data precision and continuous accessibility. Additionally, it accommodates a diverse array of data types, including JSON and binary data. The database also stands out for its built-in caching support, making it a preferred choice for applications that require rapid data retrieval. Furthermore, it offers advanced functionalities such as multi-dimensional scaling, mobile synchronization, and full-text search. However, it’s important to acknowledge some of its drawbacks. Managing and configuring Couchbase can be more complex when compared to traditional relational databases. It demands higher memory resources than other NoSQL databases and has seen limited adoption compared to well-established NoSQL databases like MongoDB and Cassandra.
4. Amazon DynamoDB
Amazon DynamoDB is a fully managed NoSQL database service offered by Amazon Web Services. It’s designed to provide a convenient and efficient way to store and manage data for a wide range of applications, including websites, mobile apps, online games, and Internet of Things (IoT) devices. DynamoDB is engineered to handle large and growing datasets while maintaining consistent and reliable performance.
One of the key features of DynamoDB is its data model. It supports both the key-value and document data models. In simple terms, this means it can manage data in a format where each piece of information is stored as an attribute-value pair. This flexibility allows it to handle structured data, like traditional databases, as well as unstructured data, such as JSON documents. It can also accommodate various data types, including text (strings), numbers, binary data, and sets. The benefits of using Amazon DynamoDB are numerous. First and foremost, it’s fully managed, meaning that Amazon takes care of the underlying infrastructure, hardware provisioning, software updates, and data backups. This frees up developers from the operational burden and allows them to concentrate on building and improving their applications.
5. Azure Cosmos DB
Azure Cosmos DB is a robust global database service developed by Microsoft, known for its ability to provide fast and flexible data storage and retrieval. What sets it apart is its compatibility with a wide range of data models, allowing it to seamlessly integrate with popular programming languages. This feautre makes it a valuable tool for diverse applications.
One of Azure Cosmos DB’s standout features is its capacity to deliver rapid data access on a global scale, all while ensuring data accuracy and high availability. This makes it particularly well-suited for mission-critical applications. The service’s ability to scale both up and down as needed ensures that it can meet the demands of your application, no matter the size or complexity. Furthermore, Azure Cosmos DB offers built-in support for replication and backups, adding an extra layer of data protection.
Despite its impressive capabilities, Azure Cosmos DB may not be the most budget-friendly choice, especially for high-usage scenarios. The cost of using this service can quickly add up, and it’s essential to manage resources efficiently to avoid unexpected expenses. Additionally, working with Azure Cosmos DB requires a solid understanding of distributed systems, which could seem tough for those new to the technology.
Today we have gone through a selection of the most prominent NoSQL databases, highlighting their key features, strengths, and potential applications. Whether you opt for the flexibility of MongoDB, the high performance of Cassandra, or any other NoSQL database, it’s essential to carefully evaluate your specific project requirements and objectives. By doing so, you can make an informed decision to harness the power of NoSQL technology and unlock new possibilities in data management and application development.
JetBrains Rider 2023.3 EAP 2 Is Out: Debugger Data Flow Analysis, All-In-One Diff Viewer and More
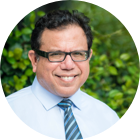
MMS • Robert Krzaczynski
Article originally posted on InfoQ. Visit InfoQ
JetBrains released Rider 2023.3 EAP 2. This Early Access Program for Rider contains such features as Debugger Data Flow Analysis, Quick Search feature and All-In-One Diff Viewer. The JetBrains Rider team also presented the Entity Framework Core user interface plugin and added some new inspections within Dynamic Program Analysis.
EAP 2 contains a change around the debugger. Data Flow Analysis (DFA) anticipates potential issues within the code and notifies of them, all without running the code. DFA has the ability to emphasise code branches that are likely to be executed, visually suppress segments of the application that will not be run, and also provide alerts when the application execution culminates with an exception. In the example below, the debugger’s dataflow analysis helps to immediately see that the method will not be executed beyond the RandomizeBool()
call, as this will raise a NotImplementedException
.
Example of Data Flow Analysis (Source: JetBrains blog)
Another new feature is Quick Search. It allows quick navigation in tool windows and dialogues. It is now available via a shortcut. Once the focus is on a tree or list, the search can be easily invoked from the Options menu of the tool window, by pressing ⌘ F on macOS or Ctrl+F on Windows or Linux, or by simply starting to type in a query. Alternatively, it is possible to assign the shortcuts to the quick search function in Settings / Preferences | Keymap.
In Rider 2023.3 EAP 2, the approach to checking modifications has changed. Instead of checking individual files one at a time, when viewing a set of changes, it is now possible to view all changed files in a single, scrolling frame. This improved difference viewer can be accessed through the Git Log tool window and integrates seamlessly with the GitLab and GitHub platforms.
The Entity Framework Core UI plugin is now integrated into JetBrains Rider. This allows users to access the Entity Framework Core command functions in Rider to perform tasks such as generating migrations, updating databases with selected migrations (including autocomplete migration names), creating a DbContext framework from existing databases or generating SQL scripts from a specified migration range.
ASP.NET Core applications now benefit from new inspections through Dynamic Program Analysis. These inspections are designed to identify instances of extended execution times in various application components, such as MVC controller methods, Razor page handler methods, and View Component methods. They are intended to help pinpoint potential backend performance issues that may affect the responsiveness of an application.
The community reacted positively to the new release, especially to information about the All-In-One Diff Viewer. One user asked below the official release post if there are plans to use it for commit diffs as well. Sasha Ivanova, a marketing content writer in .NET tools at JetBrains, answered that they are considering this, and currently weighing all the pros and cons.
The entire changelog of this release is available on YouTrack.
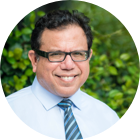
MMS • RSS
Posted on nosqlgooglealerts. Visit nosqlgooglealerts
Role: Tech Lead/Anchor engineer
Location: Southfield, MI – Onsite
This is for our direct client
The tech lead should have over 10+ years of experience in:
Java spring boot and/or C#
SQL and NoSQL experience
5+ in AWS/Azure
Angular/React
The person in the role will participate in all aspects of the agile software development
lifecycle by supporting existing software development efforts, troubleshooting issues as they occur, and
developing new software across multiple projects. This role will also lead the design of software
components and suggest new ideas for growth for the product. You will also work closely with product
owners in reviewing, interpreting, and developing in accordance with project requirements.
Specific duties and responsibilities include:
Play a critical role in supporting the Road Ready platform lifecycle including design, development,
testing and releases.
Collaborate closely with technical and product owners from internal, external, onshore, and
offshore resources.
Produce deliverables with clean, well-documented, and easily maintainable code that adheres to
coding best practices.
Triage production issues and work with the team to provide solutions.
Influence the discovery and decision-making process when changes to standards and technology
are required.
Design backend database schemes.
Stay up to date on emerging technologies.
Education: Bachelor’s degree in Computer Science or other branches of engineering.
10+ years of experience with MongoDB, PostgreSQL, and RabbitMQ
10+ years of Angular or React development experience.
5+ years of proficiency with NGNIX and Apache Tomcat
10+ years of experience with Ubuntu Linux
Excellent knowledge in Relational database design
3+ years of experience with Amazon Web Services and Amazon EC2
Good understanding of RESTful APIs with NodeJS, Swagger, and Postman.
10+ years of Java and/or C# development experience a plus
5+ years of Atlassian JIRA, Confluence, Azure DevOps and GitHub
Proficiency with Agile project management methodologies
Automotive or trucking experience is a plus
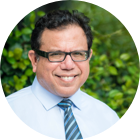
MMS • RSS
Posted on nosqlgooglealerts. Visit nosqlgooglealerts
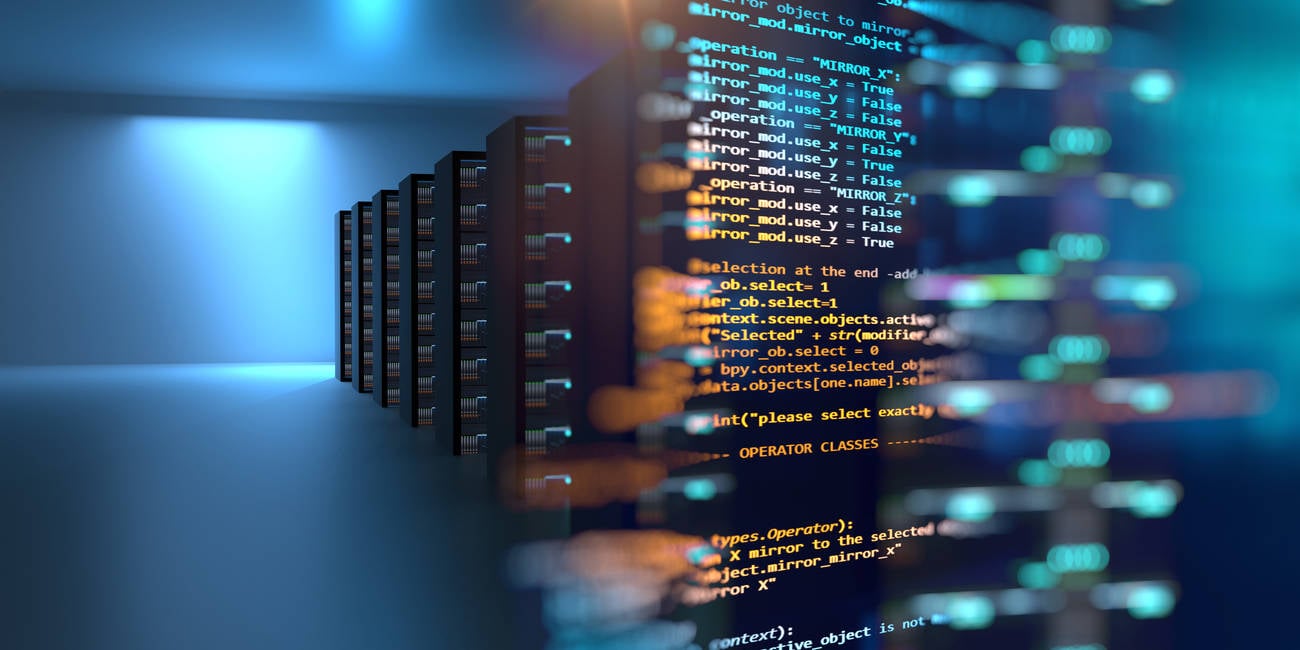
Redis, the go-to in-memory database used as a cache and system broker, is looking to include disk as part of a tiered storage architecture to reduce costs and broaden the system’s appeal.
Speaking to The Register, CEO Rowan Trollope said he hoped the move would help customers lower costs and simplify their architecture. Redis counts Twitter X, Snapchat, and Craigslist among its customers, and it’s popular among developers of modern internet-scale applications owing to its ability to create a cache to prevent the main database from overloading.
Trollope said the sub-millisecond distributed system gives devs the performance they need, but admitted other systems built for internet scale, such as MongoDB, might offer price advantages. To address this, the company has already created a tiered approach to memory by offering flash support behind its in-memory system.
“We have a half-step between disk and memory. For some specific use cases, in gaming for example, a company might use us for leaderboards and other in-game stats, which they need in real time,” he said.
However, after an initial flush of the game launch, a large chunk of users would finish the game and their accounts would go dormant until the release of a new episode or some new content, when they might return. Trollope said using flash allowed users to dynamically tier memory. “We can take the lesser-used data that hasn’t been touched in a while and shuttle it off to flash where it can sit for a while. When the user comes back eventually, it’s very easy for us to seamlessly move it from flash back into memory. And that allows the company to save costs,” he said.
Redis is now planning to extend the concept to disk-based memory to offer support for a three-tiered architecture.
The business started life in 2009 as the brainchild of developer Salvatore Sanfilippo, who stepped back from the project in 2020. In the 2023 Stack Overflow Survey, Redis was named the sixth most popular database among professional developers and the second most popular NoSQL database. Around 23 percent of pro devs use the system. In November last year, Redis acquired RESP.app, a popular developer tool used to ease developer experience on the key-value database.
In 2020, Redis became the most popular database on AWS, according to research from systems monitoring firm Sumo Logic.
Trollope argues the popularity of the database in part owes much to the lack of competition. “We don’t really compete with anyone else,” he said, before admitting that other global in-memory systems such as Aerospike were, in fact, competition.
In August, Aerospike Graph announced support for graph queries at extreme throughput across billions of vertices and trillions of connections. The company said benchmarks show a throughput of more than 100,000 queries per second with sub-5 ms latency. Aerospike customers include Sony Entertainment, PayPal, and Airtel.
“What I was trying to say is, you know, take the most popular databases in the world, and we’re the leading in-memory database and nobody else is like that. Mongo doesn’t do that. And none of cloud providers do that, like [Azure] Cosmos DB, or Oracle or any of the Amazon technologies like DynamoDB: they’re not in-memory databases. We are used alongside all the other top ten databases, but we don’t really compete with them,” Trollope said.
Aerospike is not listed by Stack Overflow among the top 30 databases used by professional developers. Database ranking service DB-Engines puts it at 65, while Redis sits at number six.
One main criticism of Redis had been its lack of support for SQL, the ubiquitous query language. Trollope said that was fixed now. A module RediSQL is available on GitHub.
It is part of the drive to make Redis “more like your classic database,” he said. In the future, support for natural language queries and enhanced vector and feature store capabilities will be added. This initiative aligns with Redis’s ambition to be seen as more than just a fast, albeit expensive, cache. ®
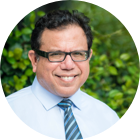
MMS • Ben Linders
Article originally posted on InfoQ. Visit InfoQ
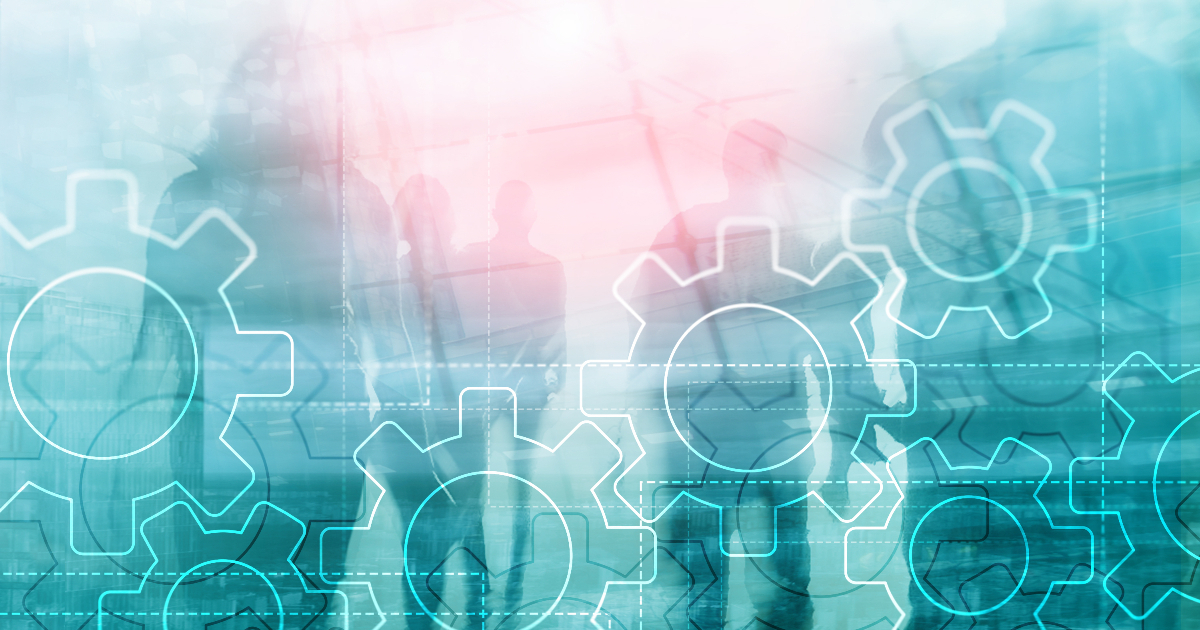
It’s challenging to grow into a new role when you are still holding on to what you have been good at and really love, and what you’ve been doing in your previous role. By attaching to everything you used to do, you are also depriving the people around you of an opportunity to grow and learn to master those skills and take on those responsibilities too.
Audrey Troutt spoke about helping others to grow in order to grow yourself at QCon New York 2023.
As you grow, you increase both the breadth and depth of your responsibility and expertise, Troutt said; first across technology, and then deeper into different parts of the stack, then across different development practices, then more profound skills are developed in those areas. Finally, it extends more broadly to the processes and business context in which your teams operate, etc.
Troutt mentioned that over time, we tend to alternate between increasing the breadth and depth of our responsibilities and expertise. And sometimes to get to the next level, we need to do both, but it is hard to grow in two directions at the same time, Troutt said.
One of the biggest challenges Troutt faced during her career was realising that she had created a bottleneck for her team, and that it was holding her back and holding her teams back too. The solution she applied was to help her team build the skills and expertise needed to carry out what she was previously single-handedly owning (like complex feature dev, team processes, platform release coordination, etc) so that she wasn’t a bottleneck anymore.
As a team leader, she learned to help other engineers grow to become leaders as well, in order for them to be able to plan and implement features independently, to triage production issues, and to be able to think and act without her.
With your bottleneck removed, your team can do more in parallel, Troutt explained:
Growing other engineers and letting go of being the only go-to expert for everything gave me the time to learn about and influence more projects and systems and work on larger technical problems. This gave me time and space to broaden my responsibility and deepen my expertise.
Troutt suggested looking at the role you play today on your team:
What are things that only you can do? What are things that really any engineer can learn to do? Are there responsibilities or skills that you can help the engineers around you learn, that would then free you up to work on other broader areas and in more depth?
Focus on doing what only you can do in your position with the context and expertise that you have, Troutt said. For everything else, be a force multiplier: coach, delegate, support your teammates:
Don’t deprive them of an opportunity to grow! I believe that the teams that approach growth this way are often the most healthy, effective, and resilient, which is great for you, great for your business, and great for our industry as a whole.
InfoQ interviewed Audrey Troutt about growth, both in herself and her people.
InfoQ: What did you learn when becoming a feature leader?
Audrey Troutt: To grow into a feature leader, you need to increase the depth of your technical and product understanding, and you need to increase the breadth of your skills to include communicating technical ideas. It is really important to break through this growth point, because what happens is that as a feature leader you become a force multiplier — you can create clarity and direction for your teammates the same way senior engineers did for you when you were starting out as a trusted contributor. This is the stage when you make the transition from follower to leader.
InfoQ: How did you grow to become a team leader?
Troutt: As a team leader, you have the experience and the context to see the big picture for your team and shape the future of the code. That’s what you should be doing. Figuring out implementation details, writing code, and reacting to errors in production is something all engineers can eventually do—let at least some of that go. And remember, it’s not about delegating work that is “beneath you” – you have to give them opportunities to plan, implement, fail, and learn while supporting them and providing overall technical direction.
InfoQ: What’s your advice for people who want to grow toward the next step in their career?
Troutt: Figure out which dimensions you need to stretch in next in terms of the breadth and depth of your responsibilities and expertise. Depending on what that is, you might need to get more practice, or read and study, or ask for new responsibilities. Then, make sure you aren’t holding yourself back by not letting go of things you are really good at that you could help other engineers learn to be good at too.
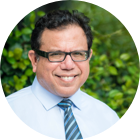
MMS • Nimisha Asthagiri
Article originally posted on InfoQ. Visit InfoQ
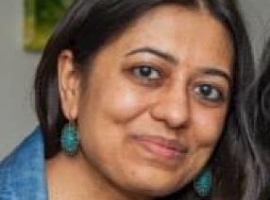
Transcript
Asthagiri: Put yourself in the shoes of a regular internet user. Let’s call her Alicia. As a typical user, Alicia spends about 7 hours per day online. She benefits from the services provided by the 200 million websites active today. As a typical user, Alicia is inundated with managing passwords for each site. She has about 100 passwords, and is locked out of about 10 accounts every month. Even if she manages to create another account, satisfying yet another set of password requirements, there’s no telling whether she’ll have access to her own data when she wants it. Eighty-two percent of sites had at least two outages the past three years, and on average, at least four hours unplanned downtime. Sometimes the downtime was as long as two weeks. Or if the site is knocked down, it’s possible the site is gone. While there are over 1 billion websites today, technically, less than 200 million are actually active. Think about Alicia’s photos that she has now lost forever. Maybe the site is up, but Alicia, she can’t share her own data across applications. She has to reenter and submit her medical history to multiple doctors. Poor Alicia, she may not even know when her own data is breached. Her data is lost in the crowd of 22 billion data records breached within the year. Does it sound familiar? Does it really have to be this way? I say no.
I’m Nimisha Asthagiri. A principal consultant at Thoughtworks. I’m here to tell you that a privacy-first architecture is more than possible by decentralizing data ownership. You see, what if we design it such that Alicia herself is at the center, not the applications and not the technologies, not the providing organizations? As before, Alicia chooses her computers and hardware. What if Alicia makes her own decisions on where her data is stored? For example, she may store data locally on a device or on external storage that she controls. Or yes, she may even backup her data to a cloud-based storage provider, especially for unreliable devices. Now that the data is under Alicia’s control, what if she freely chooses which application service providers to use? In this Alicia centric world, she makes her own decision of which particular features to use from each application in the competitive landscape. In fact, maybe she uses the best in breed app for searching through her photos, and a different app for editing her photos. Plus, since the data is in her hands, she controls access to the data.
What if while the storage providers store the data, they don’t have access to the underlying clear text of the data. What if while the application services access to the data, they don’t control access to the data, Alicia does? She can easily change secrets or change access since the data is in her control. Alicia is so empowered that she can even control how her data is used for machine learning, and other data science applications. She can choose the cohort with whom to train a cohort personalized machine learning model. Finally, what if Alicia chooses her own identity provider? Not an identity provider that an application service chooses for her, not an identity provider that her device chooses for her, but rather, she chooses an identity provider that she herself trusts. One that vouches for who she is and her credentials. One she trusts will protect her identity and her PII, or her personally identifiable information. Then, once we’re in this world, then when Alicia’s identity changes in any way, by name, by gender, by credential, she interacts with only her own identity provider to make those changes. She doesn’t need to update her identity everywhere else, in all applications, on all devices, storage spaces. Have you or someone you know needed to change information of your identity? It’s painful, isn’t it? Our identities are spread throughout the internet. Since applications are decoupled from data backends, then when Alicia wants to switch application providers, she takes her own data with her. That’s right. There’s no need for data portability across applications when the data remains grounded with Alicia.
Groove (Peer-to-Peer Application)
For those of you who are thinking, this is great, but how is this even possible? It’s just not where we are today. I’m here to tell you that we’ve done it. In fact, in the late ’90s, I was part of a team that built a peer-to-peer application called Groove. In retro style here in black and white on the left, you see a faint imagery of a past Groove UI. We had virtual spaces where groups of individuals could come together and have discussions, share files, messages. Mind you, there were no centralized servers in the picture. In fact, if you wanted to authenticate other people, you could share Groove fingerprints out of band. Fingerprint as in a hash of a Groove identity’s public key. Or you could use the TOFU style of authentication, the trust on first use, that is as you build relationships online, you began to alias people in and choose personal nicknames to associate with those online Groove identities. Even the public sector, they found Groove to be incredibly powerful. It was used in the Iraqi war to assess humanitarian needs of people affected by the war. Since no central servers were required, Groove functioned in unreliable network situations. It was also used in negotiations between Sri Lanka and the Tamil Tiger rebels. Neither side trusted the other to run a server. They couldn’t even cross certify each other because of intense political sensitivities, so they used the peer-to-peer aspects of Groove instead.
Let’s diagram Groove’s architecture using the modeling elements from before. Now here we depict users in the center, and each user is a peer to another. Each user runs a Groove application on their own device, and their own storage of choice. They control their own Groove identity. Local access to their data is protected with encryption at rest in their storage. Then, shared data and messages are automatically encrypted over the wire. When a peer communicates with another peer, peer-wise keys are automatically generated using Diffie-Hellman protocols. When group membership changes, shared group keys are automatically generated, distributed, and rekeyed. There’s full end-to-end encryption over the wire. In the end, the data, it’s in clear text only on the devices of the users. We did leverage servers in certain situations, but the servers are only additive and ephemeral, not core to the architecture. We use storage in the cloud called relay servers, but they were only dumb routers. They were storing data temporarily while someone was offline. The data was still end-to-end encrypted so these storage providers, they didn’t have access to the underlying data. Plus, they were configurable, that is, you could specify in your own profile which relay server I should use if I want to send you a message while you’re unreachable. Similarly, we support identity providers, if you chose to leverage an authority to additionally vouch for your identity. Your primary identity, though based on your public key, that still remained in your control. The identity provider was used only as an additional managed credential.
Groove as we had built it, it’s not in use today. As with many product failures, there may not be a single reason but a confluence of conditions. For Groove specifically, it may be the way we positioned it in the market, or because it ran only on Microsoft devices, or the fact that it required an 18 megabyte download at the time when the first cloud-based SaaS companies were just emerging. Also, many in the corporate world, they may have distrusted peer-to-peer since P2P origins were then associated with Napster. Napster is a notorious P2P music sharing platform that was eventually shut down due to legal suffocation. What could be the reason? Perhaps Groove was just ahead of its time, before internet users had the chance to feel the pains that we do today, which brings us back to where we are.
Centralized Silos
Today, we have centralized silos created by software, hardware, and cloud providers. Each provider decides, controls, and serves its own siloed package of storage, applications, identity providers, access control, and machine learning, and data science all in their own silo. This pushes Alicia far out of the center today. She’s almost an afterthought. It’s even worse. If we take a peek inside one of these centralized silos put together by a typical organization today, you’ll see a depiction that looks more like the right-hand side. These essentials, they’re all tightly coupled together. Let’s hear what Rich Hickey has to say, he is the author of Clojure, the programming language. He tells us, this is where complexity comes from, complecting. He says, it’s very simple, just don’t do it. Don’t interleave, don’t entwine, don’t braid. It’s best to just avoid it in the first place. When you build a system that’s complected, it becomes very hard to expand on it and innovate further. Instead, find the individual components. Those can then be composed and placed together to build robust software. Let’s take Rich’s design principles beyond the single programming language like Clojure, and even beyond software application design. Let’s apply it to enterprise architecture, and yes, even industry wide architecture.
First, how do we know which individual pieces to compose? For that, let’s take a look at what Steve Jobs and Pablo Picasso have to offer. In the mid-1940s, Picasso spent 12 hours a day for 4 months, creating series of lithographic prints, including one of a book. You can see how he starts with the blueprints that are artfully shaded and realistically rendered. In the end, he boils it down to its true essence of just 12 lines. He has a U suggesting the horns, a short line for the tail, and a smattering of black and white dots for the skin. In the end, he simplifies the bull to its essence. Steve Jobs says it takes a lot of hard work to make something simple, to truly understand the underlying challenges, and then come up with the elegant solutions. That’s what Picasso had done. That’s how Steve Jobs designed. In fact, when Apple trains its new hires, it includes a presentation of Picasso’s blueprints. This is why we see Apple create simple designs of its mouse, its Apple TV remote, and its iMac.
Decoupling and Decentralizing Identity
Bringing this back to our topic of today, let’s take these design principles of discovering the essence and detangling our systems. Let’s tease apart the essentials of the software and services that we offer today. Once we do so, we can recompose and recreate with Alicia as a new center. Let’s start by pulling out and decoupling identity. Once decoupled and removed from the centralized silo, we recompose it with decentralization, and Alicia centricity in mind. Alicia decides how to identify herself online. It could be via email, telephone number, even government or institution issued IDs. She can reuse her chosen identity across platforms and applications. Here’s an example UI where Alicia controls the binding of her own selected identity with a specific application. She minimizes what identity information to share, and perhaps even leveraging progressive proof of information.
Decoupling and Decentralizing Application Service
That was decoupling and decentralizing identity, now let’s look at doing the same for application services. Let’s first decouple application services from that tightly coupled silo, and then we compose in a decentralized way. The application, they’re now so decoupled from the underlying data storage. Alicia now decides which application she uses and when, and to what extent. This means if Alicia’s photo application chooses just to go out of business, her photos, they still remain with her. She can change to another photo app and not worry about losing any data. She more easily experiments, shops around, and tries different photo apps seamlessly, all the while her photos remain with her. She decides for herself which messaging app she uses. Her social circle, they can choose other apps if they want. The decentralized messaging apps, they interoperate with standard communication protocols. At the end of the day, it’s Alicia and her own experiences and choices. The applications serve her, not the other way around.
As another example, let’s take calendar apps. Today, our single day is broken up across multiple calendars on Google, Apple, Microsoft, and other provider services. If the calendar app were truly decentralized, it wouldn’t matter what app other people use. Instead, the calendar apps, they use data standards to interoperate. Alicia’s app, it uses a standard to access her own day. Her colleague’s calendar app accesses Alicia’s calendar using the same standard. In the end, Alicia and her contacts have freedom to choose their own individual calendar apps.
Decoupling and Decentralizing Data
That was about decoupling and decentralizing application services. Now it’s time for data. We see that data has multiple facets. We can separate data storage from data access from learning. Once decoupled, we recompose decentralized. Alicia controls the storage. Alicia controls the access, who, which users, which service providers. What, the granularity of access: fine grained, or coarse grained. When, Alicia expires access when she wants to. She can turn it on and off at her will. Alicia, she controls learning, how her own data is used for personalization via machine learning. Here are some sample UIs for Alicia’s control, for data storage, backups, data longevity. For an application’s access, she has time limited and fine-grained control. Similarly, for access to her data by other users as well. When machine learning is decentralized, Alicia chooses whether and which collaborative model to leverage. She may also derive her own local generated model from a provider’s model.
Coming Expectations – Privacy Requirements
You see, we walked through what happens when identity, application, and data essentials are decoupled, then decentralized. Here’s a reminder of what such an architecture looks like in a decentralized world where Alicia is in the center. Whether you agree or not, these are coming expectations. Privacy by design are seven principles adopted in 2010 by the International Assembly of Privacy Commissioners and data protection authorities. A few notable ones include privacy by default, privacy embedded into the design and not bolted on as an afterthought, or respecting privacy with the interests of the user at the center. OWASP, in addition to their security list, they have started publishing the top 10 privacy risks since 2014, with their own ideas for countermeasures. Examples, instead of your site requiring users to consent on everything, have users consent for each purpose, like consent specifically for keeping profiling for ads, distinct from consent for the use of the website. Better yet, minimize what data you’re collecting in the first place. Ensure that your site sufficiently deletes personal data after it’s needed, or the user requests to delete it. You do so proactively with data retention, archival, and deletion policies. Also, you can ensure that users, they always have access, and can change and delete their own data when they want to.
While OWASP suggests providing users with an email form to make their request for this, with decentralized architecture the user’s data is in their control the whole time. Finally, if we don’t hear privacy demands from the collective voices of consumers, nor the voices of international assemblies and nonprofit communities like these, then we’re sure to feel the slap on the wrist from upcoming global legal regulations. They’re going to come with enforced sanctions and fines. New global privacy laws are emerging even beyond GDPR, most notably from EU, Brazil, Canada, and California. For example, EU’s Digital Marketing Act or DMA, it has provisions for ensuring data interoperability, data portability, and data access specially for users of big tech platforms. As another example, EU’s Data Governance Act or DGA, it provides a legal framework for common data sharing spaces within Europe to increase data sharing across organizations, especially in industries such as finance, health, and the environment. We can try to bolt on support of these requirements, but privacy by design, architecturally, it will truly enable the ideals and essence of these policies and principles.
Real-World Efforts Today
You may ask, what real-world efforts exist today, so we may practically support these requirements? Let’s look at each of the essentials individually. I’ll give you a few examples of emerging technologies in each category. For identity, we have W3C’s decentralized identity or DID, which Thoughtworks is implementing as a proof of concept for the Singapore government. With DIDs, there’s no need for a centralized authority or identity management. DID is a persistent, globally unique identifier, following a URI scheme. DID refers to a DID subject, which can be a user and, in our case, Alicia. The DID resolves to a DID document that describes the DID subject. The resolution of the DID uses a verifiable data registry that maps to the method in the DID’S URI. Then the DID’s corresponding document is controlled by what’s called a DID controller. You see, there’s no centralized controller, there’s no centralized resolver in this process. Plus, to limit exposure of a user’s data, a DID controller can use zero knowledge proofs for selective and progressive disclosure of the user’s information. Finally, I’ve also listed OpenID here as a secondary alternative if you really can’t make the case for DIDs today in your org. OpenID is an open standard for federated identity. Let’s just take a moment to reflect on the impact of all of this for Alicia. If all sites invested in just this one essential of identity and decentralizing it, Alicia’s password management goes down to near or absolute zero.
Now let’s move on to application services as a next essential. Foremost, you have SOLID and PODs as new standards actively being created by Tim Berners-Lee, the founder of the web, with his team at Inrupt, starting in 2018. PODs or Personal Online Datastore is a proposed standard for storing personal data in user or organization specific stores. SOLID, or Social Linked Data is their standard for communicating across PODs and cross-linking data. This is a web centric, decentralized architecture using HTTP, web ID, web ACL. Secondly, for decentralized applications to interoperate, we need data standards as a common language. Schema.org is a place to find what exists already, and if something’s not there, you can contribute new ones. There are also industry specific standards such as FHIR for healthcare, or TM Forum for telecom, and IDMP for the identification of medicinal products. Another exciting emerging technology in this area is 2019 published research initiative called Local-first, it’s by Ink & Switch in Germany. Local-first apps, they keep their data in local storage on each device, and the data it’s synchronized across all the users’ devices. Yes, like Groove, the network is optional.
Local-first, it leverages CRDT, or Conflict-Free Replicated Data types. What CRDT does is it allows decentralized apps to merge data without conflicts. It provides strong eventual consistency guarantees, where over time all nodes eventually converge without data loss. Essentially, in async and decentralized scenarios, it fundamentally reframes race conditions. To get a super, very brief idea of how, first think about what model it may use to notify other nodes when a change happens. A few options for synchronization models could be, denote the full new state of an object to other nodes, or specify what operation to run on the object so other nodes run that same operation. Or, just transfer only a diff, or delta of the new state from the previous state. Regardless of the synchronization model, to provide strong eventual consistency, the object’s underlying data structure uses different types of tools. For example, if we all JSON base changes, they can be reduced to commutative operations. A plus B is the same as B plus A. Once you’ve done that, it doesn’t matter in what order a node receives changes, it’ll all be additive. Another tool is to use a convergence heuristic, such as last writer wins. To support that, you need to timestamp each change. If either of those don’t work for a data type or object, then you could use a version vector or a vector clock to keep track of where other nodes are at by field or by event. Such techniques, they have to make use of UUIDs to identify each field and maybe also make use of data versioning. As you can see, this design is very different from server-based designs that rely on a single centralized server to mitigate merge conflicts.
For storage, the local-only movement supports efforts to decouple and decentralize storage. By keeping data local only, organizations, they can avoid liability issues and even government subpoena of data. Of course, we mentioned peer-to-peer technologies earlier when we were describing Groove. Now for access, let’s look at what these technologies can do in terms of decoupling access from other essentials, so that it results in separation of powers. For example, the open source Signal Protocol, which is used by multiple messaging apps today, including Signal, WhatsApp, and Google, it provides end-to-end encryption of the messages. Access to the underlying message is not shared with the application provider, or any cloud storage provider. Then, you have technologies such as Vault, 1Password, and HSM, and they separate the storage of secrets from the storage of data. Finally, OPA, or Open Policy Agent, is an open source tool that enables you to separate your access policies from the rest of your application logic and data. Its architecture is a great example of Picasso based decomplecting of essentials, resulting in a powerful and flexible foundation. In fact, you can see how easily our own decomposition of essentials maps well with OPA’s own primitives.
For learning, I was pleasantly surprised to learn about the many emerging technologies in decentralized learning from my colleague in Berlin, Katharine Jarmul. She can tell you detail about federated learning, differential privacy, secure multi-party computation, secure aggregation, TinyML. Many of us, including my prior self, assume we need big data for deep learning and analysis to provide personalized user experiences and business analytics. However, this list of technologies, they prove us wrong. As you can see, there’s a plethora of emerging technologies just waiting for you to leverage, contribute to, and advance.
Practical Steps Forward
Many of these technologies, they’re in the innovative and early adopter stages. If the maturity of these are at all a hindrance for you and for your own organization, then here are some actual practical steps you can take even today. For identity, think about whether your org still requires users to create passwords, specifically for your org. If so, can you influence your company to support federated identity and single sign-on instead? There are multiple third-party identity providers now in the market such as Okta, and ForgeRock, and Auth0, who support OpenID Connect. Once we’ve leveraged an IdP, identity provider, then integrate them with preexisting identity providers, such as social login or SAML integration that may be already existing with a user’s employer. It would be even better if you can propose doing a proof of concept or pilot with a standard decentralized identity technology such as DIDs, and digital wallets. For application services, could your organization shift to leveraging existing interoperable standards in your industry? Previously, I mentioned a few industry-specific standards such as FHIR. If standards aren’t available for your industry, pick the business case for being an industry leader, and publishing standards into a framework such as schema.org. For storage, have you looked at where your users’ personal data is propagated, sent, stored? How are we keeping track of this? Could you, in fact, minimize replicating users’ data into more places than it really needs to be.
To explain what I mean, let’s say you have multiple frontends and backends in a microservice and micro-frontend architecture. Backends may be using cloud storage technologies, while frontends may be leveraging browser or device local storage. Rather than having all of your users’ profile information propagated throughout all of your backend services and their databases, minimize replicating the user’s data to only where it needs to be, perhaps only to the specific micro-frontend that displays a user’s profile. Even then, perhaps the profile information is in the JSON Web Token provided by your organization’s own identity provider, and so it doesn’t even need to be stored on the frontend separately. Then, when your organization matures to using an external identity provider or a decentralized identity, the users personal identifiers can be ephemeral and not even stored within your organization’s own application storages.
Coming back to our list, where we were looking at practical steps for decentralized storage, make the business case for supporting data portability and making user data exportable. Doing so now provides your organization optionality, before the upcoming data privacy laws give you little time. For access, check assumptions that you have today. Can you build in measures today to automatically expire access, or remove data after a period of time? Or can you allow more fine-grained access to data, so it’s not all or nothing? Finally, move away from entangling your application and access. You can use well regarded policy as code technologies like OPA, Open Policy Agent that are already available. OPA aligns with this decoupled architecture that was put forth by a XACML standard, which is Extensible Access Control Markup Language. By using OPA’s model architecture in your organization, that’s a great practical step to decouple policy enforcement from policy decision from policy administration and from policy information. You can see how this decoupling maps very well to our own decentralized modeling essentials. I hope that gives you a good list of practical ideas that you can start even today, that will be directionally aligned to the promises of this decentralized architecture. If we collaborate on getting to this, starting today, we are on the path of an industry-wide rearchitecture.
Human-Centric Architecture
Then, fast forward a few decades. Now, Alicia is at the center. She controls her own data and the choices surrounding it. Our industry is reset with the centralization pendulum shifted to a new and better balance. We have a user centric foundation to meet the underlying needs of Alicia’s communities and spheres. Alicia’s memberships and belongings are rooted in her own self, her own growth of her own identity. It’s not chosen by others and broken into disparate pieces throughout the internet. This pivotal recentering transforms data ownership for whole organizations, cities, and states. Users, communities, organizations, nations, we have expanded it to the global landscape where now we’re in a place where human-centric architecture is at the heart. Technology serves Alicia, not the other way around.
Questions and Answers
Knecht: I think you mentioned a lot of different options for here are the different ways that folks could start to move in a user-centered architecture direction. Just to tie it back to the practical security track, and getting very opinionated actionable steps here, if you had to choose one of those things, what’s the most important step that we can take or technology that we should embrace to enable a user-centered architecture?
Asthagiri: I do think the most important long run is to tackle that decentralized application essential, where we do start really decoupling where data lives and the ownership of that, and really get the data in the hands of the users when they need it, and to that extent. For the short term, I think what could be most important, is really tackling the identity dimension. With decentralized identity, removing a lot of the security issues that we get even from password management, humans being the weakest point in managing passwords, but it’s just not user friendly in so many ways. I think that just tackling that, when there’s already so many technical advances in terms of single sign-on providers, whether it’s Okta, or Auth0, or ForgeRock, or whatever you may choose to use as your technology, but getting us to move towards a single sign-on experience for our users and then eventually decentralized identity.
Knecht: One of the foundations of DDD is that the same concept like identity, for example, means different things, depending on the subdomain using it. How do you handle avoiding a huge blob as identity containing all information necessary for services that might be different and are presented differently from one service to another without it growing out of proportion? Especially if identity is handled and stored outside of services from different companies, if we want one change to update them all?
Asthagiri: Domain-driven design, yes, it does speak about polysemes, and basically each bounded context having its own semantic for a particular term. You use here, as an example, the term identity. Identity is a cross-cutting concern within organizations also. It’s a cross-cutting architectural concern. Therefore, typically, even within an organization that’s providing an application service, you would have a single identity provider in order to scale the organization, because you don’t want your each of the microservices developing its own identity management solution, and so forth. I think what you’re pointing out is more about the data about an identity. You’re perfectly right. The way that I’m thinking about it is that you do need a hybrid architecture. When we’re shifting from one side of the pendulum to the other, we need to find a balance where we’re doing both decentralized and centralized and using each of them where it makes sense. When you have data standards that you want to develop, so in this particular case, if the users have a separate decentralized identity provider for themselves, that would be the system of record. That would be the place where a lot of their identity data is there. However, in the application organizations and stuff, there will be a relationship with the user. For instance, in terms of, let’s say, my purchase of a particular product, or my history with that organization and application, some of those things, I don’t necessarily want to store in a separate place, however, you want to still be mindful for privacy. I think there is a hybrid approach where some non-PII related, still user related, though, information can still be stored in the application. We still want to be very mindful about thinking about privacy-first design. If the data that is being still stored in the application layer, can be retained only sufficiently for its needs, and then you expire that data. All of those techniques, we want to start being able to exercise those muscles. Always, whenever we’re thinking, ok, data, where should it be stored? Is it in the user side, is it on the application side, for how long? All those things we would want to tease apart as we look through it.
Knecht: I think this is a very ambitious future that you’re proposing here with decentralized design and so many different domains in places. I’m curious, your thoughts on maybe what are the biggest challenges, and what are some contributing factors to why we have the currently prevalent non-user centric architectures that we have? What are the ways that we can break those things down?
Asthagiri: I was pleasantly surprised in terms of when I was doing research for this talk on things that I had found where there were already a lot of emerging technologies in this area. Then I was even more pleasantly surprised after my talk, some of them, people were telling me, have you heard about “The Intention Economy,” by Doc Searls. People were telling me about this Internet Identity workshop, which is an un-conference where people have been meeting for a couple of years to talk about this, and all the different angles to it. Then there’s user driven services, which is trying to tackle the user experience aspect of this. I do think that this is not something that could just be addressed, technically. It’s an architecture talk on this. It’s not going to move the needle, just by itself. It is a holistic movement where the business incentive, the legal compliances, the user experience and helping users understand what’s happening today. Right now, even when we talk about privacy to certain users, they’re complacent. They think that, ok, this just has to be that way. Or they don’t even understand the level and extent to which their data is being shared. With surveillance movements happening in our own country and elsewhere, where, if I want to be able to go to a mother’s home for whatever reason, people would be able to find out. My geolocation is available in so many different ways today, and through so many different apps. There’s an educational component for the users as well. I think just holistically addressing each of these different aspects, and then being able to bring some of this forth. I do think that that Internet Identity workshop is a great one too, where if people would like to learn a little bit more, they do have proceedings from past workshops, where a lot of thought leaders in the space come together and talk through it.
See more presentations with transcripts
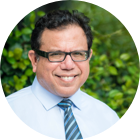
MMS • RSS
Posted on mongodb google news. Visit mongodb google news
MongoDB, Inc. (NASDAQ:MDB – Get Free Report) was the target of some unusual options trading activity on Wednesday. Traders purchased 23,831 put options on the company. This represents an increase of 2,157% compared to the average volume of 1,056 put options.
Analyst Ratings Changes
MDB has been the topic of several recent analyst reports. Needham & Company LLC boosted their price target on MongoDB from $430.00 to $445.00 and gave the company a “buy” rating in a research note on Friday, September 1st. Sanford C. Bernstein boosted their price target on shares of MongoDB from $424.00 to $471.00 in a research report on Sunday, September 3rd. 58.com reaffirmed a “maintains” rating on shares of MongoDB in a report on Monday, June 26th. Morgan Stanley boosted their target price on MongoDB from $440.00 to $480.00 and gave the stock an “overweight” rating in a report on Friday, September 1st. Finally, Scotiabank started coverage on MongoDB in a research report on Tuesday, October 10th. They issued a “sector perform” rating and a $335.00 price objective for the company. One research analyst has rated the stock with a sell rating, three have given a hold rating and twenty-two have given a buy rating to the company’s stock. According to data from MarketBeat, MongoDB has a consensus rating of “Moderate Buy” and an average target price of $416.31.
View Our Latest Stock Report on MongoDB
Insider Activity at MongoDB
In related news, Director Dwight A. Merriman sold 6,000 shares of the business’s stock in a transaction dated Friday, August 4th. The stock was sold at an average price of $415.06, for a total transaction of $2,490,360.00. Following the completion of the transaction, the director now owns 1,207,159 shares of the company’s stock, valued at approximately $501,043,414.54. The transaction was disclosed in a legal filing with the Securities & Exchange Commission, which is available through the SEC website. In related news, Director Dwight A. Merriman sold 6,000 shares of the company’s stock in a transaction on Friday, August 4th. The shares were sold at an average price of $415.06, for a total transaction of $2,490,360.00. Following the completion of the transaction, the director now directly owns 1,207,159 shares in the company, valued at approximately $501,043,414.54. The transaction was disclosed in a filing with the SEC, which is available through the SEC website. Also, Director Dwight A. Merriman sold 2,000 shares of the firm’s stock in a transaction on Tuesday, October 10th. The stock was sold at an average price of $365.00, for a total transaction of $730,000.00. Following the completion of the sale, the director now owns 1,195,159 shares in the company, valued at $436,233,035. The disclosure for this sale can be found here. In the last three months, insiders have sold 187,984 shares of company stock worth $63,945,297. 4.80% of the stock is owned by insiders.
Institutional Investors Weigh In On MongoDB
Several institutional investors and hedge funds have recently added to or reduced their stakes in the business. GPS Wealth Strategies Group LLC acquired a new position in shares of MongoDB during the 2nd quarter worth about $26,000. KB Financial Partners LLC acquired a new position in shares of MongoDB during the 2nd quarter worth about $27,000. Capital Advisors Ltd. LLC increased its stake in shares of MongoDB by 131.0% during the 2nd quarter. Capital Advisors Ltd. LLC now owns 67 shares of the company’s stock worth $28,000 after purchasing an additional 38 shares during the last quarter. Bessemer Group Inc. acquired a new position in shares of MongoDB during the 4th quarter worth about $29,000. Finally, Global Retirement Partners LLC increased its stake in shares of MongoDB by 346.7% during the 1st quarter. Global Retirement Partners LLC now owns 134 shares of the company’s stock worth $30,000 after purchasing an additional 104 shares during the last quarter. 88.89% of the stock is owned by institutional investors.
MongoDB Trading Down 1.9 %
MDB opened at $358.68 on Thursday. The stock has a market capitalization of $25.59 billion, a P/E ratio of -103.66 and a beta of 1.13. MongoDB has a 12 month low of $135.15 and a 12 month high of $439.00. The firm’s fifty day simple moving average is $359.87 and its 200 day simple moving average is $337.61. The company has a current ratio of 4.48, a quick ratio of 4.48 and a debt-to-equity ratio of 1.29.
MongoDB (NASDAQ:MDB – Get Free Report) last posted its quarterly earnings data on Thursday, August 31st. The company reported ($0.63) EPS for the quarter, beating the consensus estimate of ($0.70) by $0.07. MongoDB had a negative net margin of 16.21% and a negative return on equity of 29.69%. The business had revenue of $423.79 million for the quarter, compared to analysts’ expectations of $389.93 million. As a group, research analysts anticipate that MongoDB will post -2.17 EPS for the current fiscal year.
About MongoDB
MongoDB, Inc provides general purpose database platform worldwide. The company offers MongoDB Atlas, a hosted multi-cloud database-as-a-service solution; MongoDB Enterprise Advanced, a commercial database server for enterprise customers to run in the cloud, on-premise, or in a hybrid environment; and Community Server, a free-to-download version of its database, which includes the functionality that developers need to get started with MongoDB.
Featured Stories
Receive News & Ratings for MongoDB Daily – Enter your email address below to receive a concise daily summary of the latest news and analysts’ ratings for MongoDB and related companies with MarketBeat.com’s FREE daily email newsletter.
Article originally posted on mongodb google news. Visit mongodb google news
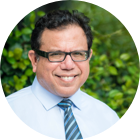
MMS • RSS
Posted on mongodb google news. Visit mongodb google news
MongoDB, Inc. (NASDAQ:MDB – Get Free Report) saw unusually large options trading activity on Wednesday. Stock investors bought 36,130 call options on the stock. This is an increase of 2,077% compared to the average volume of 1,660 call options.
MongoDB Stock Performance
MDB stock opened at $358.68 on Thursday. The firm has a market cap of $25.59 billion, a price-to-earnings ratio of -103.66 and a beta of 1.13. The company has a quick ratio of 4.48, a current ratio of 4.48 and a debt-to-equity ratio of 1.29. MongoDB has a twelve month low of $135.15 and a twelve month high of $439.00. The business’s fifty day simple moving average is $359.87 and its two-hundred day simple moving average is $337.61.
MongoDB (NASDAQ:MDB – Get Free Report) last announced its quarterly earnings results on Thursday, August 31st. The company reported ($0.63) EPS for the quarter, beating the consensus estimate of ($0.70) by $0.07. The firm had revenue of $423.79 million for the quarter, compared to analyst estimates of $389.93 million. MongoDB had a negative return on equity of 29.69% and a negative net margin of 16.21%. Analysts predict that MongoDB will post -2.17 earnings per share for the current year.
Insider Buying and Selling
In other MongoDB news, CRO Cedric Pech sold 308 shares of the business’s stock in a transaction on Wednesday, September 27th. The shares were sold at an average price of $326.27, for a total transaction of $100,491.16. Following the transaction, the executive now directly owns 34,110 shares of the company’s stock, valued at approximately $11,129,069.70. The sale was disclosed in a legal filing with the SEC, which can be accessed through the SEC website. In other news, Director Dwight A. Merriman sold 6,000 shares of the business’s stock in a transaction on Friday, August 4th. The shares were sold at an average price of $415.06, for a total transaction of $2,490,360.00. Following the sale, the director now owns 1,207,159 shares in the company, valued at approximately $501,043,414.54. The sale was disclosed in a document filed with the SEC, which is accessible through this link. Also, CRO Cedric Pech sold 308 shares of the business’s stock in a transaction on Wednesday, September 27th. The shares were sold at an average price of $326.27, for a total transaction of $100,491.16. Following the sale, the executive now owns 34,110 shares in the company, valued at approximately $11,129,069.70. The disclosure for this sale can be found here. Insiders have sold a total of 187,984 shares of company stock valued at $63,945,297 over the last three months. 4.80% of the stock is owned by company insiders.
Institutional Investors Weigh In On MongoDB
A number of institutional investors have recently added to or reduced their stakes in MDB. GPS Wealth Strategies Group LLC acquired a new stake in MongoDB in the second quarter worth about $26,000. KB Financial Partners LLC acquired a new stake in MongoDB in the second quarter worth about $27,000. Capital Advisors Ltd. LLC boosted its holdings in MongoDB by 131.0% in the second quarter. Capital Advisors Ltd. LLC now owns 67 shares of the company’s stock worth $28,000 after acquiring an additional 38 shares in the last quarter. Bessemer Group Inc. acquired a new stake in MongoDB in the fourth quarter worth about $29,000. Finally, Global Retirement Partners LLC boosted its holdings in MongoDB by 346.7% in the first quarter. Global Retirement Partners LLC now owns 134 shares of the company’s stock worth $30,000 after acquiring an additional 104 shares in the last quarter. Institutional investors own 88.89% of the company’s stock.
Analyst Ratings Changes
A number of brokerages recently issued reports on MDB. Needham & Company LLC upped their price target on shares of MongoDB from $430.00 to $445.00 and gave the stock a “buy” rating in a research note on Friday, September 1st. Scotiabank initiated coverage on shares of MongoDB in a research report on Tuesday, October 10th. They set a “sector perform” rating and a $335.00 price target for the company. VNET Group reissued a “maintains” rating on shares of MongoDB in a report on Monday, June 26th. 22nd Century Group restated a “maintains” rating on shares of MongoDB in a research note on Monday, June 26th. Finally, Truist Financial upped their price target on MongoDB from $420.00 to $430.00 and gave the stock a “buy” rating in a research note on Friday, September 1st. One analyst has rated the stock with a sell rating, three have given a hold rating and twenty-two have issued a buy rating to the stock. According to data from MarketBeat, the company presently has a consensus rating of “Moderate Buy” and a consensus target price of $416.31.
Read Our Latest Research Report on MongoDB
About MongoDB
MongoDB, Inc provides general purpose database platform worldwide. The company offers MongoDB Atlas, a hosted multi-cloud database-as-a-service solution; MongoDB Enterprise Advanced, a commercial database server for enterprise customers to run in the cloud, on-premise, or in a hybrid environment; and Community Server, a free-to-download version of its database, which includes the functionality that developers need to get started with MongoDB.
See Also
Receive News & Ratings for MongoDB Daily – Enter your email address below to receive a concise daily summary of the latest news and analysts’ ratings for MongoDB and related companies with MarketBeat.com’s FREE daily email newsletter.
Article originally posted on mongodb google news. Visit mongodb google news