Month: February 2024
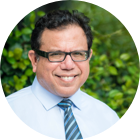
MMS • RSS
Posted on mongodb google news. Visit mongodb google news
Andar Capital Management HK Ltd raised its holdings in shares of MongoDB, Inc. (NASDAQ:MDB – Free Report) by 83.3% in the third quarter, according to its most recent Form 13F filing with the SEC. The institutional investor owned 22,000 shares of the company’s stock after buying an additional 10,000 shares during the period. MongoDB comprises approximately 19.2% of Andar Capital Management HK Ltd’s investment portfolio, making the stock its biggest position. Andar Capital Management HK Ltd’s holdings in MongoDB were worth $7,609,000 as of its most recent SEC filing.
A number of other hedge funds and other institutional investors have also recently added to or reduced their stakes in MDB. KB Financial Partners LLC acquired a new stake in shares of MongoDB during the 2nd quarter valued at about $27,000. BluePath Capital Management LLC acquired a new position in MongoDB during the third quarter valued at approximately $30,000. Parkside Financial Bank & Trust grew its holdings in MongoDB by 176.5% during the second quarter. Parkside Financial Bank & Trust now owns 94 shares of the company’s stock valued at $39,000 after purchasing an additional 60 shares during the period. AM Squared Ltd acquired a new position in MongoDB during the third quarter valued at approximately $35,000. Finally, Cullen Frost Bankers Inc. acquired a new position in MongoDB during the third quarter valued at approximately $35,000. Institutional investors and hedge funds own 88.89% of the company’s stock.
MongoDB Stock Performance
NASDAQ MDB traded down $3.59 during trading on Monday, reaching $497.31. The company’s stock had a trading volume of 611,248 shares, compared to its average volume of 1,349,371. The company has a current ratio of 4.74, a quick ratio of 4.74 and a debt-to-equity ratio of 1.18. The firm has a market capitalization of $35.89 billion, a price-to-earnings ratio of -193.00 and a beta of 1.24. MongoDB, Inc. has a 12 month low of $189.59 and a 12 month high of $509.62. The firm has a 50 day moving average price of $408.06 and a 200 day moving average price of $382.73.
MongoDB (NASDAQ:MDB – Get Free Report) last posted its quarterly earnings data on Tuesday, December 5th. The company reported $0.96 earnings per share (EPS) for the quarter, beating analysts’ consensus estimates of $0.51 by $0.45. MongoDB had a negative net margin of 11.70% and a negative return on equity of 20.64%. The firm had revenue of $432.94 million during the quarter, compared to the consensus estimate of $406.33 million. During the same quarter in the previous year, the firm posted ($1.23) EPS. The business’s revenue for the quarter was up 29.8% compared to the same quarter last year. As a group, equities analysts anticipate that MongoDB, Inc. will post -1.63 EPS for the current fiscal year.
Wall Street Analyst Weigh In
Several equities research analysts recently issued reports on the stock. Truist Financial reaffirmed a “buy” rating and set a $430.00 price target on shares of MongoDB in a report on Monday, November 13th. Royal Bank of Canada boosted their price target on shares of MongoDB from $445.00 to $475.00 and gave the company an “outperform” rating in a research note on Wednesday, December 6th. TheStreet upgraded shares of MongoDB from a “d+” rating to a “c-” rating in a research note on Friday, December 1st. UBS Group restated a “neutral” rating and set a $410.00 price target (down previously from $475.00) on shares of MongoDB in a research note on Thursday, January 4th. Finally, KeyCorp reduced their target price on shares of MongoDB from $495.00 to $440.00 and set an “overweight” rating for the company in a research note on Monday, October 23rd. One analyst has rated the stock with a sell rating, four have given a hold rating and twenty-one have assigned a buy rating to the company’s stock. According to data from MarketBeat, MongoDB has a consensus rating of “Moderate Buy” and a consensus target price of $429.50.
Read Our Latest Stock Report on MongoDB
Insider Activity
In related news, CAO Thomas Bull sold 359 shares of the stock in a transaction dated Tuesday, January 2nd. The shares were sold at an average price of $404.38, for a total value of $145,172.42. Following the completion of the transaction, the chief accounting officer now directly owns 16,313 shares in the company, valued at approximately $6,596,650.94. The sale was disclosed in a document filed with the SEC, which can be accessed through this hyperlink. In related news, CRO Cedric Pech sold 1,248 shares of the company’s stock in a transaction dated Tuesday, January 16th. The shares were sold at an average price of $400.00, for a total value of $499,200.00. Following the completion of the sale, the executive now directly owns 25,425 shares of the company’s stock, valued at $10,170,000. The transaction was disclosed in a legal filing with the SEC, which can be accessed through this hyperlink. Also, CAO Thomas Bull sold 359 shares of the company’s stock in a transaction that occurred on Tuesday, January 2nd. The stock was sold at an average price of $404.38, for a total value of $145,172.42. Following the completion of the sale, the chief accounting officer now owns 16,313 shares in the company, valued at approximately $6,596,650.94. The disclosure for this sale can be found here. Insiders have sold a total of 81,777 shares of company stock valued at $33,554,031 over the last quarter. Company insiders own 4.80% of the company’s stock.
MongoDB Profile
MongoDB, Inc provides general purpose database platform worldwide. The company offers MongoDB Atlas, a hosted multi-cloud database-as-a-service solution; MongoDB Enterprise Advanced, a commercial database server for enterprise customers to run in the cloud, on-premise, or in a hybrid environment; and Community Server, a free-to-download version of its database, which includes the functionality that developers need to get started with MongoDB.
Recommended Stories
This instant news alert was generated by narrative science technology and financial data from MarketBeat in order to provide readers with the fastest and most accurate reporting. This story was reviewed by MarketBeat’s editorial team prior to publication. Please send any questions or comments about this story to contact@marketbeat.com.
Before you consider MongoDB, you’ll want to hear this.
MarketBeat keeps track of Wall Street’s top-rated and best performing research analysts and the stocks they recommend to their clients on a daily basis. MarketBeat has identified the five stocks that top analysts are quietly whispering to their clients to buy now before the broader market catches on… and MongoDB wasn’t on the list.
While MongoDB currently has a “Moderate Buy” rating among analysts, top-rated analysts believe these five stocks are better buys.
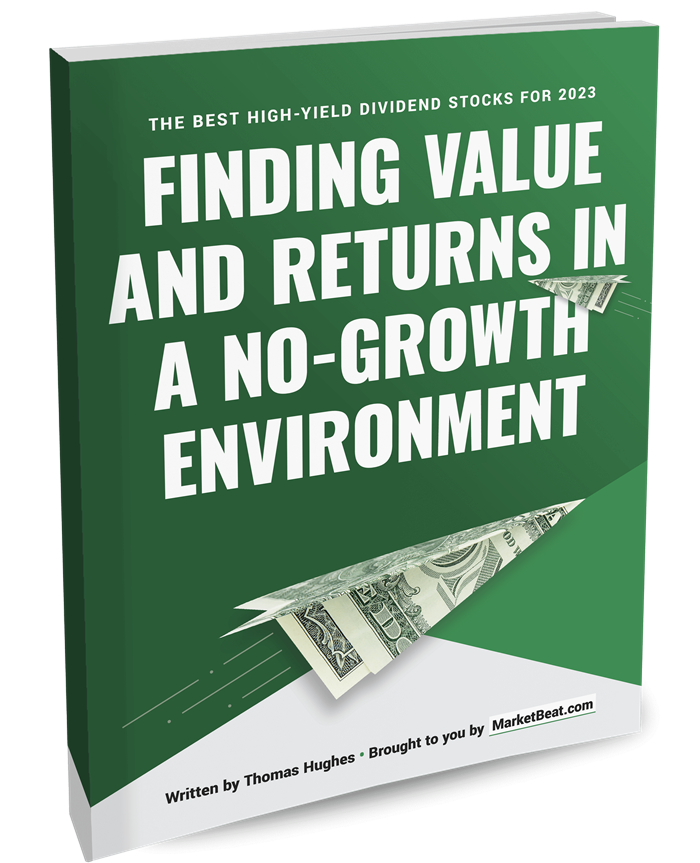
Looking to generate income with your stock portfolio? Use these ten stocks to generate a safe and reliable source of investment income.
Article originally posted on mongodb google news. Visit mongodb google news
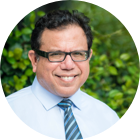
MMS • Joseph Lynch
Article originally posted on InfoQ. Visit InfoQ
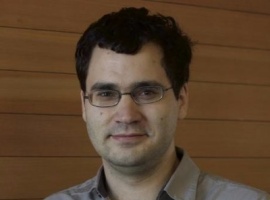
Transcript
Lynch: My name is Joseph Lynch. I’ll be talking about how Netflix ensures highly reliable online stateful systems. I’m a Principal Software Engineer at Netflix working on our data platform. I work on essentially every online system that stores state that you can imagine. If it stores data, I probably work with it. I’m an Apache Cassandra committer. I’ve worked across multiple different storage engines over many years. I’d like to talk to you about making stateful systems reliable. To do that, first, I want to define what does that even mean? Because I think that if you asked people, they might say something like, that means you have a lot of nines. Who’s heard that before? If you have a lot of nines, you’re highly reliable. Why think that your customers probably don’t care a whole lot about how many nines your datastore has. Instead, I think that they care, do the read and write operations succeed with the expected consistency model, so the semantics, within the designated latency service level objectives or goals? Always. A reliable stateful system has these properties. Instead of asking, how many nines do I have, instead, ask, how often do my systems fail? When they fail, how large is the blast radius? Then, finally, how long does it take us to recover from an outage. Then you’re going to spend money to make these go to zero. Either you’re going to overprovision computers, you’re going to pay a cloud provider to solve these problems for you, or you’re going to employ engineers to build cool resiliency techniques. Either way, this is the way that you make stateful systems reliable.
To drive this point home of why nines are insufficient, I want to consider three hypothetical stateful services. They all have equal number of nines. The first service fails just a little bit all the time, it never recovers. Maybe we need to invest in request hedging, or improving our tests to cover some bug. Service B occasionally fails cataclysmically. When service B fails, it recovers quickly but it still has a near 100% outage during that period. Perhaps we need to invest in load shedding or backpressure techniques. Finally, service C rarely fails, but when it does fail, it fails for a really long time. Maybe we didn’t detect the issue. Maybe we need better alerting or failover support. Or perhaps you don’t care about that service, and if it fails rarely, you’ll just bulkhead it from the rest of your system and be ok with it. The point that I’m trying to make is that different failure modes in your stateful services require different solutions. Throughout the rest of this talk, we’ll be exploring a multitude of techniques that we can use to try to make these properties go the direction that we want. We want less failure. When we do fail, we want to recover quickly, and we want to minimize the impact.
Netflix Online Stateful Scale
At Netflix, we use these techniques to reach very high scales of online stateful services. We have near caches, which live near to service hosts that handle billions of requests per second in sub-100-microsecond latency. We have remote caches based on Memcached that handles tens of millions of requests per second in 100-microsecond SLO targets, storing petabytes of data. Then, finally, the stateful databases under all of it is Apache Cassandra running in full 4-region active mode. In-region reader write consistency, single digit millisecond latency. We serve almost the entire planet except for a couple of places. We replicate our user state to four Amazon regions. This is a full active topology. Our video metadata is replicated even more, like if you actually look at the Open Connect CDN, it replicates state to hundreds of points of presence across the globe. We’re going to focus in this talk on the AWS control plane that runs in those four Amazon regions.
Reliable Stateful Servers
We’re going to combine three different chapters. We’re going to see how we build reliable stateful servers. How we pair those with reliable stateful clients. Then, finally, how we can design our APIs to use all of those reliability techniques. We’re going to cover five concepts in stateful servers. We’re going to talk about sharding, capacity planning, rapid responses via rapid upgrades, and then two techniques we can use to use caching to improve our reliability. First and most fundamental at Netflix is that our applications do not share caches or datastores. My team, the data platform team provides a managed experience where developers can spin up and spin down datastores on-demand as they need them. We appropriately size them for each use case. Let’s go back to that measurement technique. Before, when we had multi-tenant datastores that served multiple customers, we had rare failures that had large blast impact. When we moved to this technique, we now have more frequent failures but they have more isolated blast radius. You can see that already we’re making some tradeoffs amongst those metrics that are appropriate for our business.
We don’t want things to fail, so we want to make sure that each of these single tenant datastores is appropriately provisioned. That starts with capacity planning. It starts with understanding your hardware. For example, here, we’ve characterized two EC2 instances, and we’ve measured how quickly their disks can respond to real workloads. This is because less reliable disks, slower disks mean less reliable service. These are the foundations, this is the level of detail you have to go down to, in order to build up to a proper capacity model in order to minimize outages. For example, that little latency blip there on the p99 of that drive, that’s no good. You’re going to have SLO busters there. We have to combine this understanding of hardware with an understanding of our software. We program workload models per workload capacity models, which take into account a bunch of parameters about your workload, such as how many reads per second, or how large those reads are. Then we can use some fancy statistics, or a model that’s good enough, I think, a good enough model to output a cluster of computers that we call the least regretful choice. When we provision that cluster, we’re making a couple of tradeoffs. One of the ones that I want to call in particular, is that when we provision tier-3 datastores, we run those a lot hotter. We essentially save money on our tier-3s and our tier-2s. Then we spend it on our tier-0s. Our tier-0 datastores are overprovisioned relative to our expected workload. Those automatic capacity planning that we can continuously run on our database fleet allows us to shift risk around and save money on one part of our fleet and spend it to get reliability on another.
This also allows us to then take that cluster and replicate it to 12 Amazon availability zones spread across 4 regions. We do this for a few reasons. One of the most important ones is that we want to ensure that all of our microservices have local zone access to their data. Because as we’ll see throughout this talk, if you can keep no network connection, that’s the best. No network communication, most reliable. If you have to make a network call, make it in your local zone. If you have to cross zones, keep it in your region. Then, finally, sometimes you do have to go across region. Also, this allows us to have very highly reliable writes and read operations because we can use quorums to accept writes in any region. By having three copies in every region, we are able to provide a very high level of reliability. This minimizes the times that there are outages. Sometimes we have to fail over. This is a little diagram that shows the running Netflix system. At least at Netflix, most of our money is spent on our stateless services. We actually don’t spend as much money on our stateful services as we do on our stateless ones. They’re provisioned, typically, to handle around x over 4 of global traffic. Then, when U.S.-East-1 fails, because it’s U.S.-East-1, we have to fail out of that region. At Netflix, our resiliency team has built an amazing capability to evacuate an Amazon region in single digit minutes, we can have all of the traffic out of that region. That’s a problem for your datastores because they have to be ready to absorb a 33% hit of new traffic instantaneously. That’s even a problem for your stateless services because they can’t autoscale that fast. You have to reserve headroom.
Specifically with this architecture, you have to reserve 33% headroom. This actually is pretty ok, because the alternative where we shard our databases, and we run two copies instead of four, in that alternative, we have to reserve a lot more headroom. Specifically, we have to reserve 100% headroom. We have to prebuy a lot more reserved instances from Amazon for our stateless. We have to run continuously a lot more for our stateful if we were to copy the data less. This might not match your business. At least in Netflix’s case, paying that money to replicate the state is actually worth it, both in terms of reliability and because we can recoup the costs on stateless.
Sometimes even with all this capacity planning and sharding, bad things happen. When they do, you want to be able to deploy software and hardware quickly. For me, a lot of the reliability issues happen because of software that we write. We’ve carved mutation seams into our stateful images to allow us to more rapidly change software that doesn’t affect the availability of the system. Specifically, we’ve factored it into three components. We have the components in yellow, which we change frequently for software that we write like configuration agents or monitoring agents. We change them frequently, but they do not affect the availability of the datastore because we don’t have to bring down the Cassandra process or the Memcached process or what have you in order to change these. There’s no reliability impact. We can change these quite quickly. We couple the stateful process and the OS kernel together. Because if we have to upgrade, for example, Linux, we’re going to have to bring down the datastore image. When we do that, we’re taking a risk. Because remember earlier I talked about quorums, if we take down one member of the quorum, we’re running a risk, we could go down. We want to do this as fast as possible. We’ve released both a blog post as well as I’ve given a talk in the past about how we’re able to do this in mere minutes, going from one EC2 AMI to another atomically, similar to like a live CD, or Petitboot.
Then, finally, we have the state. This is the most problematic part of a stateful service. You don’t want to touch it that often. If you have to move state around, you’re going to have low reliability. Why is that? One reason is because of the bathtub curve. Every time that we move our state to a new instance, we’re taking a chance that that instance will fail young. The bathtub curve refers to hardware typically fails young and old. If we’ve got a good reliable database host, we really want to hold on to it, we don’t want to let go of it. If we do have to do that, we want to move the state as fast as possible. At Netflix we use snapshot restoration, where we suck down backups from S3 as fast as humanly possible onto new hot instances. Then we just share the deltas as we switch over. This does have a major reliability impact. Before I get to how we can minimize some of that reliability impact, I want to talk about monitoring. Because when you have stateful services, you have to care about how your drives are doing. At Netflix, when we’re launching those new instances, I mentioned the bathtub curve, we’re going to burn in the disks. We’re going to make sure that they can handle the type of load that we’re going to put on them. We call these pre-flight checks. Essentially, it’s like a little checklist of like, can I talk to the network? Can I talk to the disk? Is the disk capable of responding in under a millisecond?
Then, continuously, we’re monitoring errors and latency. These systems of monitoring, throw away thousands of EC2 instances on our fleet every year. Why might I do that? Because these things are just pending reliability problems. If your drive is degrading, it’s going to fail. If a drive fails, you’re going to have a bad day. It would be better to get off of it earlier. That doesn’t just apply to your hardware. It also applies to your software. Who has had a Java program go into an endless garbage collection death spiral? At Netflix, because we run so many JVM datastores like Elasticsearch and Cassandra, we run into what we call queries of death fairly frequently. What we did was we attached a monitoring agent to every JVM in our stateful fleet. That monitoring agent detects this condition within about two seconds. If our JVM has entered this death spiral, it immediately takes a heap dump, puts it in S3 so that we can analyze it later, and remediates the incident, it kills the process. In practice, this reduced 2000-plus SLO violations at Netflix in the first year that we deployed it. Because over the course of a year, when you have 2000-plus clusters, bad stuff happens, so you want to recover quickly. Little agents like these aren’t hard to add to your processes.
Let’s say that we’ve invested in all these things, and even then, we still have to be careful how quickly we do maintenance. On the x axis of this graph, we have how often we do maintenance on the Cassandra fleet. In the gray box, there are some parameters of this simulation, 2000 clusters, between 12 and 192 nodes. On the y axis we have how many outages we expect to cause through our maintenance. We can see using the naive data streaming approach where we move state between instances, we can only really do about quarterly maintenance before we start accepting more than one outage related to this maintenance per year. In contrast, if we can send data faster than we can get to monthly maintenance, we’re able to image our fleet even faster without causing too many outages. If we decouple compute from state and use EBS volumes and execute state movements with EBS swaps, we can get even farther.
Ultimately, to get to something like weekly level changes, or being able to roll out software changes faster, we need to use that in-place imaging technique that I talked about earlier. As far as I’m aware, this is the best way to do this. I’m super curious if anybody has other ways. At Netflix, we’ve taken this journey starting at the red, and then over time moving to the green so that we can recover faster from software issues.
Next, I’d like to talk about caching. Because at Netflix we treat caches like these materialized view engines. Services, if you think about it, they’re really performing complicated, almost joins. They’re talking to multiple datastores. They’re running business logic. They have some output of that computation. That output at least at Netflix doesn’t change that often. We put it in a cache, and then most read operations hit that cached view. Whenever the underlying data of that service changes the service recalculates the cache value and fills that cache. Some of you I hear what you’re saying, you’re going, no, the cache could go down. That is true. However, at Netflix, we’ve invested in making our caches highly reliable, for example, investing in things like cache warming. If a node fails, we repopulate it with the cached data before it goes into service, and by replicating it to every availability zone, so that if clients miss in one zone, they can retry against another. In practice, these caches are essentially your service at that point. Memcached can handle orders of magnitude more traffic than a Java service can.
One antipattern that we discourage is putting caches in front of datastores. Instead, we prefer to put them in front of the service. Because a cache in front of the service protects the service, which is at least for us, usually, the thing that fails first. The cache is cheap relative to the service. Stateless apps are quite expensive to operate. This technique can help us improve reliability by decreasing the amount of load that the datastores have to handle. It does shift load to caches, but like I mentioned, those are easier to make reliable. We can actually take this technique even further and move all the data into the client, we call this total near caching. Netflix has written a series of blog posts about this, as well as has an open source project called Hollow. The way this works is that the source of truth datastore isn’t even involved in the read path. All that it does is publish a snapshot up to some blob store like S3 through the producer. Then the video metadata service in this case, starts applying the delta to the in-memory dataset that it has, and then can atomically swap over from version 1 of the dataset to version 2. At Netflix, we like to treat Hollow almost like Git, but for your configuration data. This is the service that can handle billions of requests per second, because there is no network call, it’s all local to in-memory. It’s great. The more things that you can fit in this the better.
Reliable Stateful Clients
We’ve seen a number of techniques for how to make reliable stateful servers. None of those are any use if your clients are doing the wrong things. Let’s dive in to how to make reliable stateful clients. We’re going to cover six concepts. We’re going to talk about signaling, service level objectives per namespace and access pattern, hedged requests, exponential backoff, load unbalancing, and concurrency limits. Let’s start with signals, because at Netflix there historically was a big debate about tuning the timeouts and client tuning. What we realized on stateful services was that you can’t really set the correct timeout. Instead, you need to know who the client is, what shard of what datastore they’re talking to, and what data they’re trying to access. Then from those, you can deduce the service level objectives. In this example at the top box, we have a client that’s saying, I’d like to talk to the KeyValueService, the napa shard of that service in U.S.-East-1. In the bottom, we’re talking to a TimeSeries abstraction, hosting a totally different shard. Those signals start returning information to the client. For example, they might tell the client, I’d like you to chunk large payloads. Large payloads are really hard to make reliable, because you don’t know, is the network slow, or is it dropping packets? It’s difficult when you’re transmitting multi-megabytes of data at once to know if it’s latency or expected. In contrast, if the client chunks information to smaller pieces of work, you can individually retry and hedge those individual pieces of work. You can make progress in parallel. You can also go further and just compress stuff. At Netflix, we’ve used LZ4 compression from our stateful clients to compress large payloads before they’re sent. In practice, this reduces bytes sent by between 2x and 3x. Fewer bytes is more reliable. Why? Because every packet you send is an opportunity for a 200 millisecond SLO buster, when the Linux kernel waits a minimum of 200 milliseconds to retry that packet. If you can send fewer bytes, you’re going to have more reliability. Compression helps you with that. It also adds useful things like checksumming and other useful properties.
Finally, I want to talk about SLOs. Because in that signal’s endpoint, the services, the KeyValueService on the left and the TimeSeries Service on the right are going to communicate with the client, “This is what I think your target service level objective should be, and this is what the maximum should be.” You can think of target as like the number that we’re going to use hedges and retries to try to hit, and then max is like, after this, just timeout and go away. We’re also going to communicate the current concurrency limits. The server is saying, client, you’re allowed to hedge 50 concurrent requests against me, you’re allowed to send 1000 normal requests. If you’re interested about hedge requests, I highly recommend a wonderful article from Jeff Dean at Google called, “The Tail at Scale.” The general gist is that you send the request multiple times and whoever answers first, you take earlier. We can also tune these SLOs based on the particular namespace they’re trying to access. For example, in this case, we have two namespaces that are actually pointing at the same data, but one of them presents eventual consistency and the other one presents READ_YOUR_WRITE consistency. Eventual consistency can have a sub-millisecond latency SLO. It’s really hard to make a READ_YOUR_WRITES consistency model sub-millisecond. The SLO on the left can be stronger than the SLO on the right.
It actually gets a little more complicated. It’s not just the namespace, it’s also the client and their observed average latency. For example, if we have client A, and they’re making GET requests against namespace 1, if they’re observing 1 millisecond latency, then that means that in order to hit the SLO of 10 milliseconds, we’re going to have to send a hedge around 9 milliseconds. The same client might be doing PUT requests, and those are averaging 1.5 milliseconds. We have to hedge a little bit earlier, if we want to try to hit that 10 millisecond SLO. On the other hand, scan requests are real slow, they have an SLO of around 100 milliseconds. We don’t want to hedge until 77 milliseconds after that. Finally, client B might continuously be getting above the SLO latency, in which case hedging isn’t going to help you. Hedging is just a strategy to help you meet the SLO. If you’re always missing the SLO, there’s no point in doubling the load to your backend, just to try to meet something that you will fail at. This leads to what I call the three zones of dynamic hedging timeouts. The first zone is the zone of hope. This is the part where the client is observing latencies that are significantly under the SLO, specifically under half. Once you get to half, though, you’re now hedging 50% of your work, roughly. This is the part where you have to make a decision. You could either really try hard to make the SLO. This is the zone of last-ditch attempts. You could try really hard to make the SLO, in which case you’re going to be provisioning your backend for 50-plus percent extra load. Or you could just say like, let’s back off a bit and give the backend some room. At Netflix, when we hit the SLO itself, we back off entirely. This is because at least for us, when the backend service is on average above your SLO, there’s probably something wrong, and hedges probably aren’t going to help you out. You can see that in these three zones, we need to use different approaches to how we hedge against the downstream services, depending on whether we’re likely to get a positive result.
We also have to be really careful when we’re hedging requests. We have to use concurrency limiting to prevent too much load going to our backend services. Let’s walk through an example. In this case, client 1 sends two requests to server 1, which immediately goes into garbage collection pause because it’s Java. At that point, client 1 weights the SLO minus the average, and then says, ok, I’d like to hedge against server 2, maybe it’ll respond to me. At that point, it puts a hedge limiter in place. That 50 number we saw earlier, in this example, it’s 1. We’re allowing one outstanding hedge. When the client1 tries to hedge request B, instead of allowing that hedge to go through, we say, there might be something wrong with the backend, let’s just wait for it. Once we get the response back from server2 to request A, now the hedge limiter lifts, and a subsequent PUT from client1 to server1 is allowed to speculate. We can see in this example, if we had done nothing, all three of these requests would have hit that latency busting 24 millisecond GC Pause. Because of this hedging system, we were able to rescue two or three requests. This goes back to that, in this case, we couldn’t prevent the issue, we did have some SLO violation, but we minimized the impact. Fewer requests violated the SLO.
We can use similar techniques to make GC tolerant timeouts. If I’m implementing a naive timeout, I might set a single asynchronous future at 500 milliseconds. Then at the end of 500 milliseconds, I throw an exception. In our stateful clients, we use what we call GC tolerant timers, which is where we chain 4 async futures 125 milliseconds apart, the exact numbers don’t matter, you could do 3, you could do 2. What matters though, is that it’s more than one. This creates essentially a virtual clock that’s resilient to that client1 pausing. Let’s do an example where client1 sends a request to a stateful service, the service actually responds, but because that client was garbage collecting when it was responding, a naive timer would incorrectly throw an exception when it wakes up from the garbage collection. Contrast that with our GC tolerant timer, which correctly returns the result and does not pollute our logs with an incorrect error that misleads our investigators. Before we implemented this, we spent a significant amount of time tracking down services that thought their data source were slow, when in reality, their caches were actually quite fast, their key-value stores were quite fast, but their service was under load. This technique doesn’t again make the problem go away, but it does help you resolve it faster by preventing your operators from getting distracted. Then, finally, sometimes things are going to break and you’re going to get load shedding. When that happens, we do allow a retry against stateful services, we allow really just one retry. Although it is implemented generically to more than one. We use a slightly modified capped exponential backoff algorithm. The only real modification that’s worth pointing out here is that the first retry happens relevant to that SLO target. You can see how having those SLOs is allowing us to more intelligently retry against our backends. If we didn’t have those service level objectives, then we would have to take something that would probably mess up with our SLOs more frequently.
It wouldn’t be nice, though, if we didn’t send any requests to that slow server in the first place. That’s where load balancing, or I like to say, load unbalancing comes into play. At the bottom here, we have a bunch of resources about different load balancing strategies, including a simulation where you can reproduce these numbers. The end result is that the typical load balancing algorithms like random load balancing or round robin load balancing, they don’t actually work very well at avoiding slow servers. Instead, a lot of people at Netflix use choice of 2, the power of two random choices. We’ve actually recently rolled out, at Netflix, an improvement on choice of 2 called weighted choice of n. The way that works is by exploiting a priori knowledge about networks in the cloud. Specifically, this is three zones in U.S.-East-1, and those are the latencies between them. We know that because we have a replica of data in every zone, if we send the request to the same zone, we’re going to get a faster response. We don’t need anybody to tell us that. We know it, we can measure it. All we have to do is weight requests towards our local zone replica. We want this to degrade naturally, so like, what if we only have two copies, or what if we are in the wrong zone? Instead of just having a strict routing rule, we take our concurrency, and instead of just picking the two that have the less concurrency, we weight the concurrency by these factors. This sounds pretty cool. Does it work? Yes, it works really well. This is an example of us running a synthetic load test where we took a Cassandra client that was doing local1 reads, and we moved it from our weighted least load balancer algorithm to the out of the box trace of 2. Immediately we regressed from 500 microsecond latencies to 800 microsecond latencies. I’ll point something out, that’s client-side latency, that’s sub-millisecond latency from a database client to its server. This looked pretty positive, so we were like, we should put it in production. We saw a bunch of graphs like this, just dropping off a cliff. This is fantastic. We love it when our theory matches our measurement, matches production. If you’re curious to learn more, we talked about this at ApacheCon last year, and I’ve left links at the bottom.
Let’s put all these techniques together. Let’s look at a real-world KeyValueService issue, which did not turn into an incident. At time t1 at 7:02 a.m., an upstream client of this KeyValueService suffers a retry storm, and their traffic instantly doubles. Within 30 seconds, our additive increase, multiplicative decrease server concurrency limiters are shutting load off of the server trying to protect the backend from the sudden load spike. Meanwhile, those client hedges and exponential retries we talked about are mitigating the impact. This is such a small impact that we’re not violating any of our user visible SLOs at this point. The only problem that we have is that high latency impact. That’s because we went from 40% utilization to 80% utilization in 10 seconds. Finally, our server autoscaling system restores our latency SLO. This whole process from the client load doubling, to our server limiters tripping, to our client hedges and exponential retries mitigating, to the server autoscaling and finally restoring the SLO, takes about five minutes. There’s no human involved here. Everything happened as expected. We had an issue. We mitigated the impact. We recovered quickly. No human was involved.
Reliable Stateful APIs
I made a pretty important assumption when I was talking about hedging, and retrying, and breaking down work. What was that assumption? I assumed that the work was idempotent. That’s actually pretty tricky to build into your stateful APIs. I also assumed that your stateful APIs provided a fixed unit of work capability. Both of those have to be designed into your stateful APIs. Let’s see how that works in the real world, and we’ll motivate it with two real-world production examples in our KeyValueService and our TimeSeries service. Assume you have to retry, how do you make your mutable API safe? The answer is idempotency tokens. Who has used Stripe to charge a credit card? The way that works so that you don’t double charge your credit card is using an idempotency key. The client generates a unique ID that associates with that transaction. Even if the device has to retry it multiple times, you don’t have multiple charges. In concept, this is pretty simple, you generate some token. You send it with your mutative endpoint. Then if something bad happens, you just retry with the same idempotency token. You have to make sure that the backing storage engines implement this idempotency.
Let’s dive in and understand what kinds of tokens we might need. At Netflix, an idempotency token is generally a tuple of a timestamp and some form of token. Depending on the backend, these things are going to change. Let’s look at the simplest one. At Netflix we use a lot of Apache Cassandra. Apache Cassandra uses last write wins to duplicate things. As long as we send mutations with the same timestamp, they will deduplicate. We can use client monotonic timestamps, in this case, a microsecond timestamp with a little bit of randomness mixed into the last character just to prevent a little bit of duplication, and a random nonce. The combination of this uuid4 and a timestamp uniquely identifies that mutation. If we have a more consistent store, we might need to make a global monotonic timestamp within the same region. Perhaps we’re reaching out to a ZooKeeper cluster to acquire some lease, or perhaps we’re using a store like FoundationDB, which has a central timestamper. Then, finally, the most consistent option is to take a global transaction ID. That’s how a lot of transactional datastores work, for example, like MySQL or Postgres, where they actually associate a global transaction ID with a mutation. You have to somehow encapsulate that transaction ID or move it into your data model so that you can retry against those stores. Transactions don’t magically make your database isolated or idempotent. You have to actually put it in your data model and think about, I might have to retry this right, how would that look?
These three different options have a different consistency-reliability tradeoff. On the x axis here, we have the level of how strongly consistent we are, ranging from not at all consistent, all the way up to global linearizability. At the y axis we have the reliability of that technique. We’ll notice that the regional isolated tokens and the global isolated tokens have problems. Why do I say that they’re less reliable? It’s because they require communication across the network. A client monotonic clock requires no network connectivity for you to generate the next token. Contrast that with a local region token where you need at least a quorum in a region to vote, or a global one where you have to talk across a WAN network. At Netflix, we tried as much as possible to have client monotonic clocks. If you can make it less consistent, make it less consistent. Nobody knows if the recommendations at Netflix are the right recommendations at Netflix. Don’t bring down your service trying to get a global linearizable clock to use in your transactions.
I am going to be a little bit contrarian though, because earlier we heard about how clocks are problematic. If the clocks are outside of the cloud, I agree with that. However, we actually measured this. Across the Cassandra fleet at Netflix, over 25,000 virtual machines across over 20 instance families all with Nitro cards on them though, and we did not observe clock drifts beyond 1 millisecond. The only time that we observed clock drifts above 1 millisecond nonmonotonic was when VMs booted. The first about 2 to 3 minutes of a VM’s life, it has an inaccurate clock. Then after that, it’s accurate. How does this work? Atomic clocks, pretty cool. EC2 in every availability zone maintains a highly accurate clock source. Then using their Nitro system, they give your VM direct access to it. When I set out to write this memo, it was because the Cassandra community was proposing using clocks in a strongly consistent method. I was like, no, everybody in distributed systems know that clocks don’t work. Then we measured it. It turns out, they actually work pretty well. Surprising, unexpected, but it turns out accurate. I highly encourage you, if you are building a system based on clocks, measure, see what happens.
We’ve seen the concepts. How does this actually work in reality? Let’s dive into some examples of real-world APIs using these concepts. We’re going to start with the KeyValue Abstraction, which at Netflix offers developers a stable HashMap as a service, a two-level HashMap, and exposes a pretty simple crud interface. You can write items to the store, you can delete them, you can read them, and then you can scan all of the items across the entire outer map. Let’s dive in. Let’s look at PutItems. We can see immediately the idempotency token pops right out. The first required argument to write data to a store is you have to have an idempotency token, generated via one of those methods that we talked about earlier. We also see that in our list of items there’s a chunk number, and that facilitates the clients splitting up large payloads into multiple small ones, and then followed by a commit. On the read side, we see the same breaking down of work. A GetItems response does not guarantee that you will receive 1000 items, or 2000 items. Instead, it attempts to return a fixed size of data. This is because we want to be able to retry each component. You might say, gRPC, shouldn’t you be using streaming? That’s what we thought too. It didn’t work very well. The reason it didn’t work very well is because all of those resiliency techniques I talked about where you can retry individual pieces of work, and you can hedge and you can speculate, and you can retry, they don’t work in a streaming API. Instead, we’ve shifted almost all of our stateful APIs to paginated APIs that return fixed amounts of data. Then the client has to explicitly request the next page. This also has nice backpressure effects, where if the client isn’t asking for the next page, the datastore does not preemptively return it.
This does lead to one interesting failure mode, though, which is that because most storage engines implement pagination based on number of rows, and we want to implement pagination in terms of size, we have this mismatch, where the server might do many round trips to the datastore in order to accumulate a page, so many round trips that we actually bust the SLO. That’s pretty easy, because we know what the SLO is, so we can just stop paginating. At no point did our API promise that we would return a fixed amount of work, or that we perform a fixed number. Instead, we’d rather present progress to the client, and then let the client request the next page if they need it. In many cases, they’re just asking for the first item. Finally, let’s look at scan. Scan is just like GET, except that there’s no primary key. The only interesting thing in this API is that when you when you initiate a scan, the KeyValueService dynamically computes how many concurrent cursors it releases to that client. That’s the repeated StringValue next_page. For example, if the backend system is lowly loaded, then it might return a high concurrency like 16 concurrent cursors, that then the client could consume quite quickly. If a lot of clients are scanning, then it will only return a small number of cursors. This has a natural backpressure effect, where if a bunch of clients show up and start full table scanning this namespace, the KeyValueService protects itself, and it rate limits how quickly they can scan.
Let’s wrap it up. How do we put it all together? On the right side, we saw that idempotency token in use to deduplicate. In this case, the backend is some storage engine that implements the last write wins use case, so the timestamp is sufficient. We were able to chunk and break down large writes. On reads, we returned pages of work within this service level objective. We do not offer a service level objective across all pages. Because at least at Netflix, people can store gigabytes in a single key in our key-value store, we can’t offer an SLO on how quickly we’ll return gigabytes. We can offer a throughput SLO, which is a proxy for that individual page size limit. If you take that size and multiply it by the number of pages, that’s your throughput SLO. I think a key insight here is that we’re focusing on fixed size work, not fixed count work. As we mentioned earlier, every byte that we transmit either in writes or in reads is an opportunity for failure. That’s a nice example. You might say, that’s just one key-value API, what about other APIs? It turns out you can use these techniques in general. For example, at Netflix we have the TimeSeries Abstraction. This stores all of our trace information, PDS log files which are like playback log logs that tell us that somebody hit play. Just to demonstrate the scale of the service, this service handles between 20 and 30 million events per second, fully durably with retention for multiple weeks. That’s how the customer service agents know that your TV had that really odd error at 2:00 while you were watching Bridgerton.
How does this system work? We have our write EventRecords endpoint which takes a list of event records, and nowhere in this event record do we see an idempotency token. I promise you it’s there, just look a little closer. On the read side, it looks just like the GetItems. We have GetItems on the top and we have scan on the bottom, essentially reader specific event stream or read all of them. The idempotency token in this service is actually built into the contracted TimeSeries. Specifically, if you provide an event that has the same event time and the same unique event ID, then the backend storage of this deduplicates that operation. While it’s not called an idempotency token, it’s using the same concept. On the read side, again, we see that breaking down of a potentially very large response into multiple pages that can be consumed progressively. The one thing that I’d like to touch on on this service is that because of the massive scale here, developers asked us for an additional level of reliability. They were willing to trade off significant amounts of consistency in order to get it. Specifically, that’s that operation load on the left there which we all fire and forget, where the only thing that the TimeSeries client does is enqueue it into a buffer, and goes like, “Ok, I’m done.” It doesn’t even wait until it gets off the box. Is this durable? No. Would most database people say that that is an acceptable thing? Probably not. Our users really love that that could handle 20 million writes per second, and it worked all the time. They didn’t care if we dropped one service trace out of billions in that minute.
We do offer more consistent and less reliable options as we go to the left. For example, we can say, we’ll let you know when we have it enqueued into our in-memory queue on the TimeSeries server, or we’ll respond back to you once it is durable in storage. We can see again how depending on what your customer need, you can be more reliable by trading off consistency, or if they needed that consistency, you could just spend a lot of money. That’s another valuable way to get the type of reliability that you need. Let’s wrap it with TimeSeries. Again, we see the idempotency token on writes. We see multiple modes of acknowledgement. In this particular service we just ban large events. We can’t write large events to this, larger than 4 megabytes. Then reads return with pages within the SLO. Fixed size work, not fixed count.
With that, we’ve seen how we can make modifications to our stateful APIs to be able to use those client and server techniques that we covered earlier. I hope that some of these might help you improve the reliability of your stateful services.
Questions and Answers
Participant 1: How do we firstly enable technologies for the hedging and with the trace routing?
Lynch: The generic answer would be something like a service mesh, because that would allow you to implement those techniques once centrally in your proxy that does egress routing. You could implement your load balancing policy. That particular weighted trace event, that requires a little bit more metadata because we need to know information about where data is stored. It’s a little bit more complex to make generic, but you can do it. Hedging is very easy to make generic. You can throw that in service meshes. You can throw it in some generic IPC library that you distribute to all your clients. Hedging is easiest to implement in a language that has proper async futures, so like Rust, or Java, or C++ with futures. It’s really hard to implement in something like Python, although you can do it. That’s one of the reasons why I think I would probably lean towards some external sidecar that implements those resiliency techniques centrally.
Participant 2: Do you have any strategies for these server-side handling of the idempotency tokens?
Lynch: Many datastores have a built-in idempotency technique. For example, Apache Cassandra is last write wins. As long as you could present a mutation with the same timestamp. Other cases are more difficult and usually involve some form of data modeling. For example, let’s say you’ve got a transactional SQL store, you could implement idempotency by doing a transaction like a compare insert operation. Insert this if it doesn’t already exist. Insert if not exists is an idempotent operation. It’s definitely per storage engine. If you’re using Elasticsearch, the way that you implement idempotency for Elasticsearch is different from Cassandra which is different from Postgres.
Participant 2: At the app store, or datastore where there is a bit of like a serverless layer, or something?
Lynch: That’s actually one of the reasons why Netflix has been moving towards these online stateful abstractions. The KeyValue and TimeSeries Abstraction that I presented, one of their core reasons of being is to implement those idempotency techniques for people. When you send mutations to the KeyValueService or to the TimeSeries service, it handles like, I’m talking to DynamoDB, this is how DynamoDB does idempotency. I’m talking to Cassandra, this is how Cassandra implements idempotency. Although you certainly could do that in libraries, I just think it would be more difficult to maintain.
Participant 3: You mentioned decommissioning a couple thousand servers a year, in AWS that just means you give that back. Do you communicate that to AWS, or you just leave it for us blips to consume everything?
Lynch: It mostly comes back to us, actually. The first technique that we use is that we detach but don’t terminate yet. From an autoscaling group, we detach the instance but we don’t terminate it. That reserves it out of the pool. Amazon gives us a new instance which hopefully is healthy, then we release that one. It just goes back into the pool. The pre-flight checks help us not get it back. This is especially a problem for narrowly constrained instance families. For example, back when we used a lot of D2s, it was very common for us to get the same D2 back. That’s actually why we added the pre-flight checks because the pre-flight checks on our end would reject that hardware from reentering the fleet.
Participant 3: You store records inside [inaudible 00:46:10] or something?
Lynch: As far as I’m aware, there is no durable way to know that a new Amazon instance is an old one. If you have one instance ID and then you terminate it, and Amazon hands you back a new one, I’m not aware of how to know that it is degraded. We have talked with AWS about how to improve this communication capabilities. It would be nice if they had an API where we could be like, our monitoring systems have detected this degraded hardware. One thing I can say is that EC2 actually already knows a lot times that that drive is degraded, they just can’t pull it because there’s a user workload on it. Then you gather from their perspective, they don’t know if you’re using the drive. They might know that it’s throwing all these IO errors, but they don’t know if your workload cares about that, so they’re not going to just turn off your workload. In practice, Amazon actually catches a lot of that on their own.
Participant 4: You were talking about designing an API and designing clients and probably some [inaudible 00:47:21]. Are you usually providing like a set of APIs to the good uses of the stateful services, or they’re going to use stateful services the right way, how that relationship seems to work.
Lynch: We target an 80/20, so about 80% of services at Netflix go through those abstraction layers, in which case we offer them a lot of guarantees around API compatibility. About 20% of users do go directly to storage engines, in which case we send them the 25-page memo on like this is how you implement idempotency, this is how you don’t kill this datastore, this is how you do backups. A lot of folks do want that level of control at Netflix, and so we do give them that freedom. Although it is an 80/20. About 20% of users do direct data access and manage their own implementations of some of these. We do provide datastore client libraries as well, in all major supported languages. We definitely push people towards the APIs that we know have all the idempotency and resiliency techniques that we think are important. There are cases where, for example, we’ve worked to get some of these resiliency techniques. I’m actually working with a colleague right now to get that load balancer into the open source Cassandra driver, so we do sometimes bring that back to the open source community. It can be challenging because a lot of these techniques, when you don’t have a requirement for really high reliability, a lot of them don’t make sense to pay the engineering complexity. It makes sense for NASA to invest in reliability. If you’re showing cat pictures, maybe it doesn’t.
See more presentations with transcripts
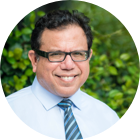
MMS • RSS
Posted on mongodb google news. Visit mongodb google news
Charles Schwab Investment Management Inc. raised its stake in shares of MongoDB, Inc. (NASDAQ:MDB – Free Report) by 1.0% during the third quarter, according to the company in its most recent Form 13F filing with the Securities and Exchange Commission (SEC). The firm owned 237,683 shares of the company’s stock after purchasing an additional 2,277 shares during the period. Charles Schwab Investment Management Inc. owned 0.33% of MongoDB worth $82,205,000 as of its most recent filing with the Securities and Exchange Commission (SEC).
A number of other hedge funds and other institutional investors have also added to or reduced their stakes in the stock. Blueshift Asset Management LLC purchased a new stake in MongoDB during the third quarter valued at approximately $902,000. Tanager Wealth Management LLP purchased a new stake in shares of MongoDB in the third quarter worth approximately $316,000. Banque Cantonale Vaudoise raised its holdings in shares of MongoDB by 10.4% in the third quarter. Banque Cantonale Vaudoise now owns 1,374 shares of the company’s stock worth $476,000 after buying an additional 129 shares during the period. Amalgamated Bank raised its holdings in shares of MongoDB by 4.7% in the third quarter. Amalgamated Bank now owns 7,548 shares of the company’s stock worth $2,611,000 after buying an additional 340 shares during the period. Finally, Western Wealth Management LLC raised its holdings in shares of MongoDB by 160.7% in the third quarter. Western Wealth Management LLC now owns 2,620 shares of the company’s stock worth $906,000 after buying an additional 1,615 shares during the period. 88.89% of the stock is currently owned by hedge funds and other institutional investors.
Analyst Ratings Changes
MDB has been the topic of several recent analyst reports. Mizuho increased their price target on MongoDB from $330.00 to $420.00 and gave the stock a “neutral” rating in a report on Wednesday, December 6th. KeyCorp reduced their price target on MongoDB from $495.00 to $440.00 and set an “overweight” rating on the stock in a report on Monday, October 23rd. Wells Fargo & Company started coverage on MongoDB in a research report on Thursday, November 16th. They set an “overweight” rating and a $500.00 target price for the company. Royal Bank of Canada lifted their target price on MongoDB from $445.00 to $475.00 and gave the company an “outperform” rating in a research report on Wednesday, December 6th. Finally, Capital One Financial raised MongoDB from an “equal weight” rating to an “overweight” rating and set a $427.00 target price for the company in a research report on Wednesday, November 8th. One equities research analyst has rated the stock with a sell rating, four have assigned a hold rating and twenty-one have issued a buy rating to the company. According to MarketBeat.com, the company currently has a consensus rating of “Moderate Buy” and an average price target of $429.50.
Read Our Latest Analysis on MongoDB
Insider Activity
In other news, CAO Thomas Bull sold 359 shares of the business’s stock in a transaction dated Tuesday, January 2nd. The stock was sold at an average price of $404.38, for a total value of $145,172.42. Following the completion of the transaction, the chief accounting officer now owns 16,313 shares of the company’s stock, valued at approximately $6,596,650.94. The sale was disclosed in a legal filing with the SEC, which is accessible through this link. In related news, CAO Thomas Bull sold 359 shares of the company’s stock in a transaction dated Tuesday, January 2nd. The stock was sold at an average price of $404.38, for a total value of $145,172.42. Following the completion of the transaction, the chief accounting officer now owns 16,313 shares of the company’s stock, valued at approximately $6,596,650.94. The transaction was disclosed in a legal filing with the Securities & Exchange Commission, which is accessible through the SEC website. Also, CRO Cedric Pech sold 1,248 shares of the company’s stock in a transaction dated Tuesday, January 16th. The stock was sold at an average price of $400.00, for a total value of $499,200.00. Following the completion of the transaction, the executive now directly owns 25,425 shares of the company’s stock, valued at approximately $10,170,000. The disclosure for this sale can be found here. Insiders sold a total of 81,777 shares of company stock valued at $33,554,031 in the last quarter. 4.80% of the stock is currently owned by company insiders.
MongoDB Stock Performance
MongoDB stock opened at $500.90 on Monday. The company has a current ratio of 4.74, a quick ratio of 4.74 and a debt-to-equity ratio of 1.18. The business’s 50-day simple moving average is $408.06 and its two-hundred day simple moving average is $382.88. MongoDB, Inc. has a fifty-two week low of $189.59 and a fifty-two week high of $507.25.
MongoDB (NASDAQ:MDB – Get Free Report) last posted its quarterly earnings results on Tuesday, December 5th. The company reported $0.96 earnings per share for the quarter, beating the consensus estimate of $0.51 by $0.45. The business had revenue of $432.94 million for the quarter, compared to the consensus estimate of $406.33 million. MongoDB had a negative return on equity of 20.64% and a negative net margin of 11.70%. The company’s quarterly revenue was up 29.8% compared to the same quarter last year. During the same quarter in the previous year, the business earned ($1.23) EPS. As a group, sell-side analysts forecast that MongoDB, Inc. will post -1.63 EPS for the current year.
About MongoDB
MongoDB, Inc provides general purpose database platform worldwide. The company offers MongoDB Atlas, a hosted multi-cloud database-as-a-service solution; MongoDB Enterprise Advanced, a commercial database server for enterprise customers to run in the cloud, on-premise, or in a hybrid environment; and Community Server, a free-to-download version of its database, which includes the functionality that developers need to get started with MongoDB.
Recommended Stories
Want to see what other hedge funds are holding MDB? Visit HoldingsChannel.com to get the latest 13F filings and insider trades for MongoDB, Inc. (NASDAQ:MDB – Free Report).
Receive News & Ratings for MongoDB Daily – Enter your email address below to receive a concise daily summary of the latest news and analysts’ ratings for MongoDB and related companies with MarketBeat.com’s FREE daily email newsletter.
Article originally posted on mongodb google news. Visit mongodb google news
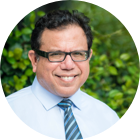
MMS • RSS
Posted on mongodb google news. Visit mongodb google news
Shell Asset Management Co. cut its stake in shares of MongoDB, Inc. (NASDAQ:MDB – Free Report) by 2.2% in the third quarter, according to the company in its most recent disclosure with the Securities and Exchange Commission (SEC). The fund owned 1,350 shares of the company’s stock after selling 30 shares during the period. Shell Asset Management Co.’s holdings in MongoDB were worth $467,000 as of its most recent SEC filing.
Other institutional investors have also made changes to their positions in the company. KB Financial Partners LLC acquired a new stake in shares of MongoDB during the second quarter valued at about $27,000. Bessemer Group Inc. purchased a new position in shares of MongoDB in the fourth quarter valued at approximately $29,000. BluePath Capital Management LLC purchased a new position in shares of MongoDB in the third quarter valued at approximately $30,000. Cullen Frost Bankers Inc. purchased a new position in shares of MongoDB in the third quarter valued at approximately $35,000. Finally, AM Squared Ltd purchased a new position in shares of MongoDB in the third quarter valued at approximately $35,000. 88.89% of the stock is currently owned by institutional investors and hedge funds.
MongoDB Trading Up 5.4 %
MDB stock opened at $500.90 on Monday. The company has a debt-to-equity ratio of 1.18, a current ratio of 4.74 and a quick ratio of 4.74. MongoDB, Inc. has a twelve month low of $189.59 and a twelve month high of $507.25. The company’s 50 day moving average is $408.06 and its two-hundred day moving average is $382.88.
MongoDB (NASDAQ:MDB – Get Free Report) last announced its earnings results on Tuesday, December 5th. The company reported $0.96 EPS for the quarter, beating analysts’ consensus estimates of $0.51 by $0.45. The business had revenue of $432.94 million for the quarter, compared to analysts’ expectations of $406.33 million. MongoDB had a negative net margin of 11.70% and a negative return on equity of 20.64%. The company’s quarterly revenue was up 29.8% on a year-over-year basis. During the same period in the previous year, the company posted ($1.23) EPS. As a group, equities research analysts expect that MongoDB, Inc. will post -1.63 EPS for the current fiscal year.
Wall Street Analyst Weigh In
Several research analysts have recently commented on the stock. Barclays raised their price objective on shares of MongoDB from $470.00 to $478.00 and gave the company an “overweight” rating in a research note on Wednesday, December 6th. Mizuho raised their price objective on shares of MongoDB from $330.00 to $420.00 and gave the company a “neutral” rating in a research note on Wednesday, December 6th. JMP Securities reissued a “market outperform” rating and issued a $440.00 price objective on shares of MongoDB in a research note on Monday, January 22nd. Needham & Company LLC reissued a “buy” rating and issued a $495.00 price objective on shares of MongoDB in a research note on Wednesday, January 17th. Finally, DA Davidson reiterated a “neutral” rating and issued a $405.00 price target on shares of MongoDB in a research note on Friday, January 26th. One investment analyst has rated the stock with a sell rating, four have assigned a hold rating and twenty-one have issued a buy rating to the company. According to data from MarketBeat, the stock currently has an average rating of “Moderate Buy” and an average target price of $429.50.
Read Our Latest Research Report on MongoDB
Insider Activity
In other MongoDB news, CRO Cedric Pech sold 1,248 shares of the business’s stock in a transaction on Tuesday, January 16th. The shares were sold at an average price of $400.00, for a total value of $499,200.00. Following the transaction, the executive now directly owns 25,425 shares of the company’s stock, valued at approximately $10,170,000. The sale was disclosed in a filing with the Securities & Exchange Commission, which is accessible through this link. In other MongoDB news, CRO Cedric Pech sold 1,248 shares of the business’s stock in a transaction on Tuesday, January 16th. The shares were sold at an average price of $400.00, for a total value of $499,200.00. Following the transaction, the executive now directly owns 25,425 shares of the company’s stock, valued at approximately $10,170,000. The sale was disclosed in a filing with the Securities & Exchange Commission, which is accessible through this link. Also, CEO Dev Ittycheria sold 33,000 shares of the business’s stock in a transaction on Thursday, February 1st. The shares were sold at an average price of $405.77, for a total transaction of $13,390,410.00. Following the completion of the transaction, the chief executive officer now directly owns 198,166 shares in the company, valued at $80,409,817.82. The disclosure for this sale can be found here. Insiders have sold 81,777 shares of company stock valued at $33,554,031 in the last ninety days. 4.80% of the stock is currently owned by company insiders.
MongoDB Profile
MongoDB, Inc provides general purpose database platform worldwide. The company offers MongoDB Atlas, a hosted multi-cloud database-as-a-service solution; MongoDB Enterprise Advanced, a commercial database server for enterprise customers to run in the cloud, on-premise, or in a hybrid environment; and Community Server, a free-to-download version of its database, which includes the functionality that developers need to get started with MongoDB.
Featured Stories
Want to see what other hedge funds are holding MDB? Visit HoldingsChannel.com to get the latest 13F filings and insider trades for MongoDB, Inc. (NASDAQ:MDB – Free Report).
Receive News & Ratings for MongoDB Daily – Enter your email address below to receive a concise daily summary of the latest news and analysts’ ratings for MongoDB and related companies with MarketBeat.com’s FREE daily email newsletter.
Article originally posted on mongodb google news. Visit mongodb google news
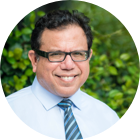
MMS • A N M Bazlur Rahman
Article originally posted on InfoQ. Visit InfoQ
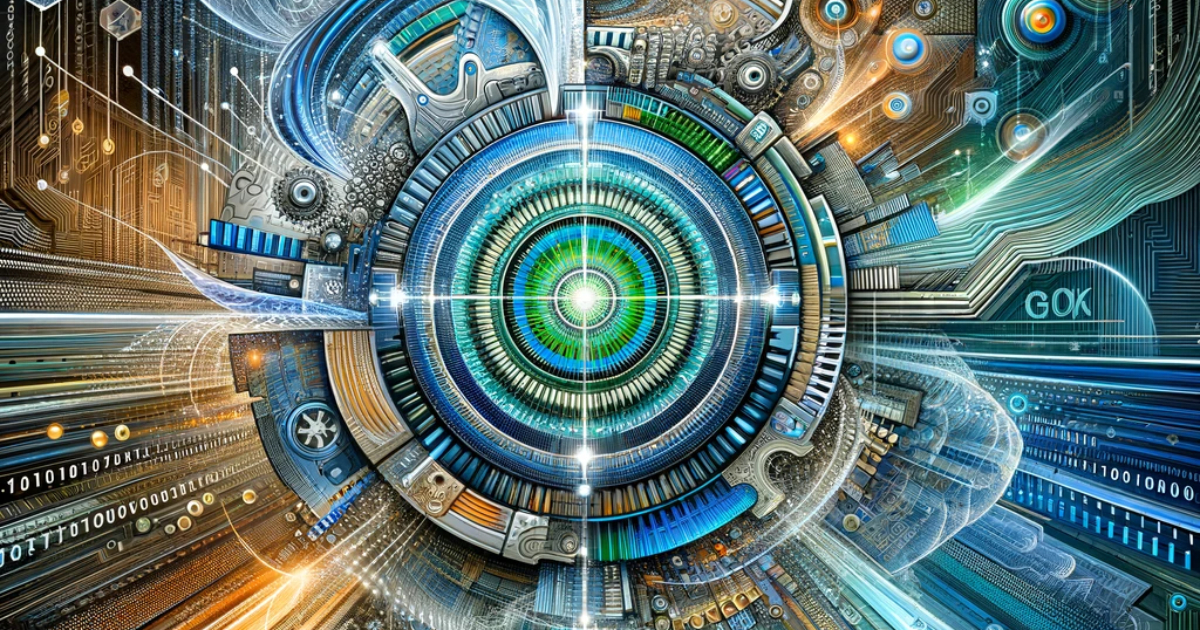
JEP 460, Vector API (Seventh Incubator), has been Closed / Delivered for JDK 22. This JEP, under the auspices of Project Panama, incorporates enhancements in response to feedback from the previous six rounds of incubation: JEP 448, Vector API (Sixth Incubator), delivered in JDK 21; JEP 438, Vector API (Fifth Incubator), delivered in JDK 20; JEP 426, Vector API (Fourth Incubator), delivered in JDK 19; JEP 417, Vector API (Third Incubator), delivered in JDK 18; JEP 414, Vector API (Second Incubator), delivered in JDK 17; and JEP 338, Vector API (Incubator), delivered as an incubator module in JDK 16. The most significant change from JEP 448 includes an enhancement to the JVM Compiler Interface (JVMCI) to support Vector API values.
This API aims to provide developers with a clear and concise way to express vector computations that can be compiled at runtime into optimal vector instructions on supported CPU architectures, thereby achieving superior performance compared to scalar computations. The latest iteration, proposed for JDK 22, includes minor enhancements and bug fixes, notably extending support for vector access to heap MemorySegments
backed by an array of any primitive element type. This is a significant improvement over the previous limitation to byte arrays.
The core objectives of the Vector API are to offer a platform-agnostic interface that enables efficient implementation on multiple CPU architectures, including x64 and AArch64, and to ensure reliable runtime compilation and performance. This reliability is essential for developers to confidently use vector operations, expecting them to map closely to hardware vector instructions like Streaming SIMD Extensions (SSE), Advanced Vector Extensions (AVX) on x64, and NEON, SVE on AArch64. Furthermore, the API is designed to degrade gracefully on architectures that don’t support certain vector instructions, ensuring functionality without compromising performance significantly.
To illustrate the concept, consider a traditional scalar approach for adding two arrays of integers:
public void scalarAddition(int[] a, int[] b, int[] result) {
for (int i = 0; i < a.length; i++) {
result[i] = a[i] + b[i];
}
}
While straightforward, this approach operates on one pair of integers at a time, which is not optimal on modern CPUs with vector processing capabilities.
Now, the above method can be rewritten using the Vector API to leverage Single Instruction, Multiple Data (SIMD) capabilities for parallel computation:
import jdk.incubator.vector.IntVector;
import jdk.incubator.vector.VectorSpecies;
public void vectorAddition(int[] a, int[] b, int[] result) {
final VectorSpecies species = IntVector.SPECIES_PREFERRED;
int length = species.loopBound(a.length);
for (int i = 0; i < length; i += species.length()) {
IntVector va = IntVector.fromArray(species, a, i);
IntVector vb = IntVector.fromArray(species, b, i);
IntVector vc = va.add(vb);
vc.intoArray(result, i);
}
// Handle remaining elements
for (int i = length; i < a.length; i++) {
result[i] = a[i] + b[i];
}
}
In this vectorized version, the IntVector
class from the Vector API is used perform addition on multiple integers at once. The SPECIES_PREFERRED
static field represents the optimal vector shape for the current CPU architecture, determining the number of integers processed in a single operation. The loopBound
method calculates the upper bound for the loop to ensure safe vector operations within the array size. Inside the loop, Vectors va
and vb
are created from the input arrays and added together using the add
method, which performs the addition in parallel across the vector lanes. The result is then stored back into the result array with the intoArray
method. Any remaining elements not covered by the vector operations are processed using a scalar loop, ensuring all elements are correctly added.
The example above illustrates the API’s power by comparing a scalar computation with its vectorized counterpart using the Vector API. The vectorized version demonstrates a significant performance improvement by operating on multiple elements in parallel, showcasing the potential for enhancing Java application performance in areas such as machine learning, cryptography, and linear algebra.
One of the long-term goals of the Vector API is to align with Project Valhalla, aiming to change the API’s current value-based classes to value classes for enhanced performance and memory efficiency. This change will allow for the manipulation of class instances without object identity, significantly benefiting vector computations in Java.
The Vector API introduces an abstract class, Vector
, and its subclasses for specific primitive types to represent vectors and perform operations. These operations are classified as lane-wise, affecting individual elements, or cross-lane, affecting the vector as a whole. The API’s design focuses on reducing its surface area while maintaining the flexibility to perform a wide range of vector operations, including arithmetic, conversions, and permutations.
The re-incubation of the Vector API in JDK 22 reflects an ongoing commitment to optimizing Java’s performance capabilities in response to modern hardware advancements. This effort highlights the Java community’s dedication to improving computational efficiency and developer productivity through continued innovation.
Editor’s Note:
Minor cosmetic tweak was done.
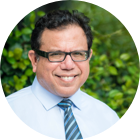
MMS • Sergio De Simone
Article originally posted on InfoQ. Visit InfoQ
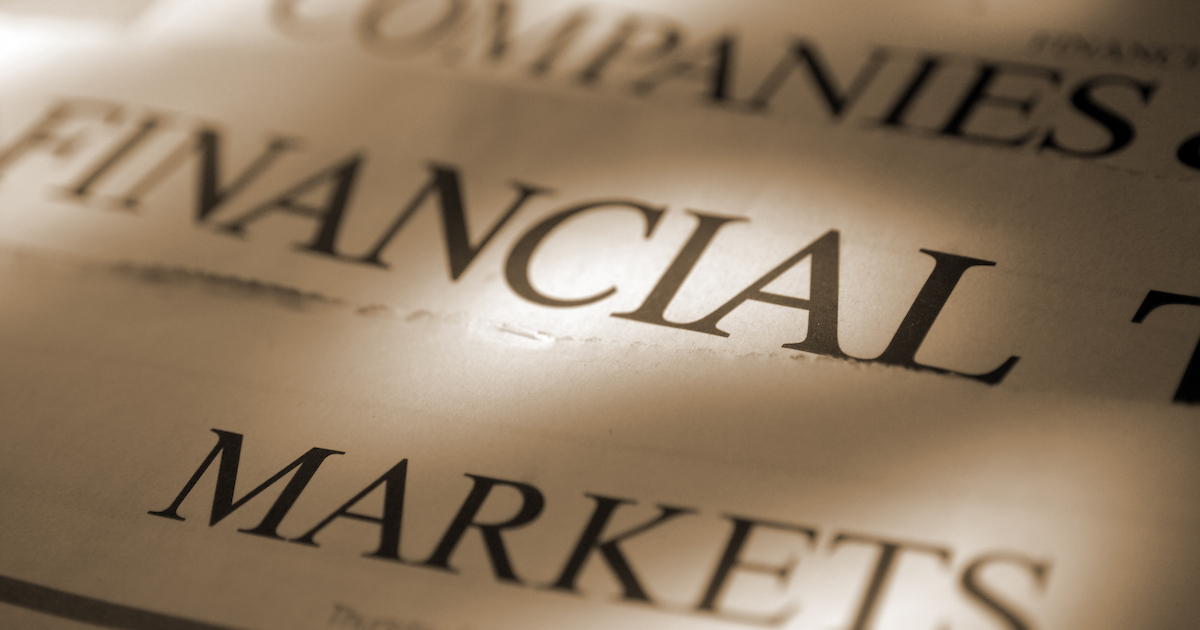
Responding to the European Commission Digital Markets Act (DMA), which aims to regulate the “gatekeeper power” of digital companies, Apple has opened up the possibility for developers to distribute their iPhone apps through alternative marketplaces in EU countries. Developers, though, will be required to pay a new Core Technology Fee if they do.
To be DMA compliant, Apple is introducing three key changes for iPhone apps in the EU: allowing the use of alternative marketplaces; allowing collection of payments through alternative payment systems; and allowing browsers to use alternative rendering engines to WebKit. In addition, users will be allowed to select their default browser.
While Apple’s announcement could sound “game changing” on the surface, the reality is more complex than that. In fact, to comply with DMA, Apple has published a set of complex rules, policies, and new APIs developers should adopt. Tellingly, in Apple’s own wording those rules and policies are meant to “safeguard” from the new risks that DMA poses to EU users, including:
threats like malware or malicious code, and risks of installing apps that misrepresent their functionality or the responsible developer.
Most significantly, if iOS developers want to opt into the new DMA-compliant rules, they will be required to pay a new Core Technology Fee (CTF) for every install over the first 1 million. Apple goes to a great length to define the concept of “first annual install” to clarify how installs are calculated and to ensure that no two installs by the same user can be counted as two distinct “first annual installs”:
A first annual install may result from an app’s first-time install, a reinstall, or an update from any iOS app distribution option — including the App Store, an alternative app marketplace, TestFlight, an App Clip, volume purchases through Apple Business Manager and Apple School Manager, and/or a custom app.
The first million “first annual installs” will be free for all developers. Beyond that threshold, developers will pay a Core Technology Fee of €0.50 for each additional “first annual install”. This will apply to free as well as paid apps.
Developers can choose to remain under the current, non-DMA rules, which means everything will remain as it is —in short, free apps will be free to both users and their developers, while paid apps and in-app purchases will pay according to the 30%/15% rule— and there will be no Core Technology Fee.
If developers adopt the new DMA-rules, they will have several options to choose from.
First, they can decide to stay with the App Store as the only distribution channel for their apps while leveraging the opportunity of using alternative Web engines. In this case, developers can still use Apple’s payment system and pay 20%/13% commission (instead of the usual 30%/15% split) + CTF. Additionally, they can integrate alternative payment systems and/or external links to websites and pay 17%/10% percent + CTF.
As a second option, they can distribute their apps through alternative marketplaces. In this case, they will not be allowed to use Apple’s payment system and their only monetary obligation to Apple will be the CTF.
From a technical point of view, marketplaces will be implemented through third-party apps which will be themselves available on the App Store. No provision is given for installing apps directly from a developer’s website, anyway, and the requirements to run a marketplace appear quite exacting.
Surprisingly, even apps to be distributed through alternative marketplaces will be subject to “notarization” by Apple. This will take the form of an app review aiming to ensure the app is secure, is not misrepresenting things, is safe, and protects privacy. The rules used for review will be entirely different and most specifically Apple will not object to the app’s content, which means apps with adult content will not be rejected, as it happens for the App Store.
Apple has always considered sideloading on iOS detrimental to users’ interests due to the risks in terms of privacy and security it poses. So it comes as no surprise that they are now trying to make it not easy for developers to use alternative marketplaces.
Reviewing Apple’s announcement, long-time technology investor and writer M.G. Siegler observed most rules seem convoluted changes to ensure the status quo actually doesn’t change much. He also predicts Apple is going to likely be at war with the EU over these matters for years.
As Apple-focused tech blogger John Gruber remarks, Apple’s new rules and policies are just an attempt to be compliant with DMA, while preserving what the company sees as its interests. Ironically, he adds, Apple’s “dealings with the European Commission sound exactly like App Store developers’ dealings with Apple. Do all the work to build it first, and only then find out if it passes muster”.
According to Tim Sweeney, Epic Games founder and CEO, Apple is “forcing developers to choose between App Store exclusivity and the store terms, which will be illegal under DMA”. Despite all the hindrances he sees in Apple’s proposed solution to DMA compliance, he says Epic is “determined to launch on iOS and Android and enter the competition to become the #1 multi-platform software store, on the foundation of payment competition, 0%-12% fees, and exclusive games like Fortnite.”
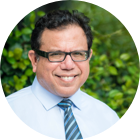
MMS • Almir Vuk
Article originally posted on InfoQ. Visit InfoQ
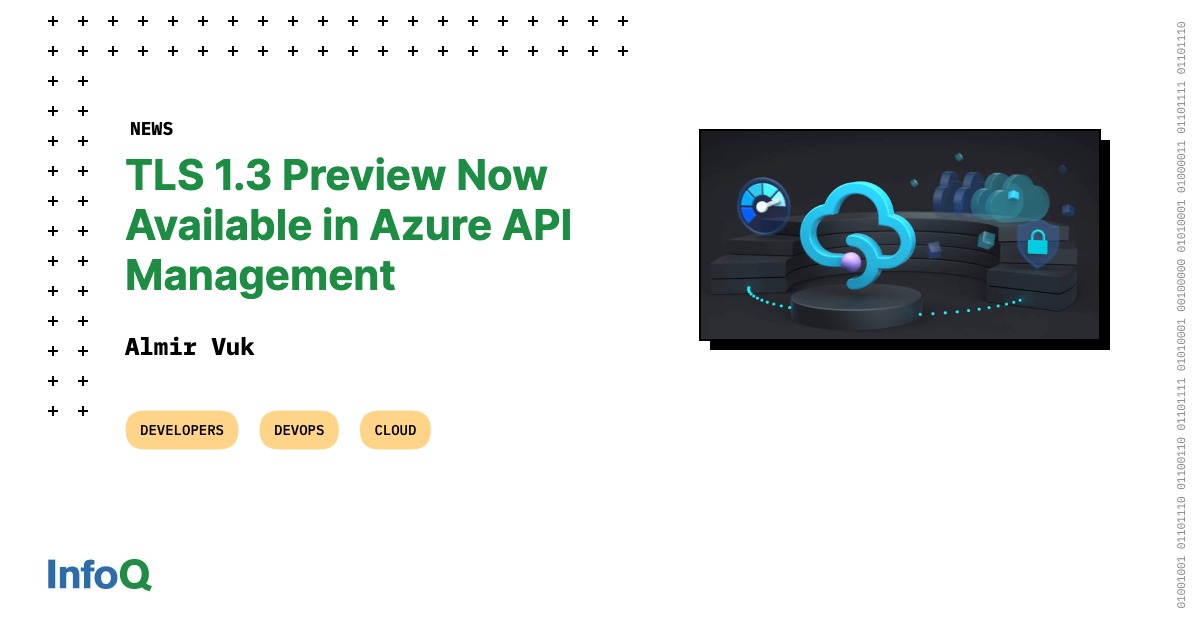
Azure API Management introduced TLS 1.3 support in the V1 and V2 tiers during the initial week of February 2024. As reported, the rollout will occur progressively across regions. Inbound traffic for both V1 and V2 tiers will inherently support TLS 1.3 for incoming requests from API clients.
As reported, for outbound traffic in V1 tiers, manual activation of TLS 1.3 will be required, while V2 tiers will receive support for outbound traffic with TLS 1.3 in a subsequent update. Additionally, an update will be released in the coming weeks for the enabling or disabling of ciphers of outbound traffic through various channels such as the Azure Portal, ARM API, CLIs, and SDKs.
TLS 1.3 represents the latest iteration of the widely used security protocol on the internet. It secures communication channels between endpoints by encrypting data, thus superseding outdated cryptographic algorithms, bolstering security compared to older versions, and prioritizing encryption throughout the handshake process.
Unlike previous versions, TLS 1.3 ensures confidentiality in client authentication without the need for additional round trips or CPU costs. At the same time, it enhances security measures significantly.
According to Microsoft, integrating API clients or services with TLS 1.3 protocol should not pose any issues for those employing client libraries like browsers or .NET HTTP clients. However, the manual configuration of TLS handshakes for clients connected to Azure API Management warrants review to ensure compatibility with TLS 1.3.
Developers are strongly encouraged to test TLS 1.3 in their applications and services. The simplified list of supported cipher suites reduces complexity and guarantees specific security features such as forward secrecy (FS).
Regarding the impact of TLS 1.3 Impact on API Clients, Fernando Mejia from Microsoft stated the following:
We do not expect TLS 1.3 support to negatively impact customers. TLS 1.2 clients will continue to work as expected. However, client certificate renegotiation is not allowed with TLS 1.3, if your Azure API Management instance relies on client certificate renegotiation for receiving and validating client certificates, your instance of API Management will not be updated to enable TLS 1.3 by default and will default to TLS 1.2 to avoid any impact on your API clients.
The protocol enables encryption earlier in the handshake, providing better confidentiality and preventing interference from poorly designed middle boxes. TLS 1.3 encrypts the client certificate, so client identity remains private, and renegotiation is not required for secure client authentication.
In addition to the announcement, the original blog post includes an informative FAQ section addressing common questions from the community regarding the addition of TLS 1.3 support. One such question is, What to expect with the initial TLS 1.3 (preview) support?
Beginning February 5th, some customers may begin to see incoming client requests using TLS 1.3 handshakes if the clients also support TLS 1.3. Customers using Azure API Management will not have control over when the update arrives, it will be part of a general release. You can expect these TLS 1.3 handshakes to stabilize by the end of March 2024.
Lastly, Microsoft encourages users to provide feedback on the TLS 1.3 preview in Azure API Management. For questions, users can seek answers from community experts on Microsoft Q&A. Also, users with support plans requiring technical assistance can create a support request using Azure Portal.
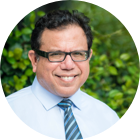
MMS • RSS
Posted on mongodb google news. Visit mongodb google news
Amalgamated Bank boosted its position in MongoDB, Inc. (NASDAQ:MDB – Free Report) by 4.7% in the third quarter, according to the company in its most recent Form 13F filing with the Securities & Exchange Commission. The firm owned 7,548 shares of the company’s stock after purchasing an additional 340 shares during the period. Amalgamated Bank’s holdings in MongoDB were worth $2,611,000 as of its most recent filing with the Securities & Exchange Commission.
A number of other hedge funds have also recently added to or reduced their stakes in MDB. Vanguard Group Inc. increased its holdings in MongoDB by 2.1% in the first quarter. Vanguard Group Inc. now owns 5,970,224 shares of the company’s stock valued at $2,648,332,000 after buying an additional 121,201 shares in the last quarter. Jennison Associates LLC increased its holdings in MongoDB by 87.8% in the third quarter. Jennison Associates LLC now owns 3,733,964 shares of the company’s stock valued at $1,291,429,000 after buying an additional 1,745,231 shares in the last quarter. State Street Corp increased its holdings in MongoDB by 1.8% in the first quarter. State Street Corp now owns 1,386,773 shares of the company’s stock valued at $323,280,000 after buying an additional 24,595 shares in the last quarter. 1832 Asset Management L.P. increased its holdings in MongoDB by 3,283,771.0% in the fourth quarter. 1832 Asset Management L.P. now owns 1,018,000 shares of the company’s stock valued at $200,383,000 after buying an additional 1,017,969 shares in the last quarter. Finally, Geode Capital Management LLC increased its holdings in MongoDB by 3.5% in the second quarter. Geode Capital Management LLC now owns 1,000,665 shares of the company’s stock valued at $410,567,000 after buying an additional 33,376 shares in the last quarter. Institutional investors and hedge funds own 88.89% of the company’s stock.
MongoDB Stock Up 5.4 %
MDB opened at $500.90 on Monday. The firm has a 50 day moving average price of $408.06 and a 200 day moving average price of $382.88. The company has a market cap of $36.15 billion, a PE ratio of -189.73 and a beta of 1.24. MongoDB, Inc. has a 1 year low of $189.59 and a 1 year high of $507.25. The company has a debt-to-equity ratio of 1.18, a quick ratio of 4.74 and a current ratio of 4.74.
MongoDB (NASDAQ:MDB – Get Free Report) last posted its earnings results on Tuesday, December 5th. The company reported $0.96 earnings per share (EPS) for the quarter, topping analysts’ consensus estimates of $0.51 by $0.45. The firm had revenue of $432.94 million during the quarter, compared to the consensus estimate of $406.33 million. MongoDB had a negative net margin of 11.70% and a negative return on equity of 20.64%. The company’s revenue was up 29.8% on a year-over-year basis. During the same quarter in the prior year, the company earned ($1.23) EPS. Sell-side analysts expect that MongoDB, Inc. will post -1.63 earnings per share for the current year.
Analyst Upgrades and Downgrades
A number of brokerages have issued reports on MDB. DA Davidson reaffirmed a “neutral” rating and issued a $405.00 target price on shares of MongoDB in a report on Friday, January 26th. Barclays raised their price objective on shares of MongoDB from $470.00 to $478.00 and gave the stock an “overweight” rating in a research note on Wednesday, December 6th. UBS Group reissued a “neutral” rating and set a $410.00 price objective (down from $475.00) on shares of MongoDB in a research note on Thursday, January 4th. Truist Financial reissued a “buy” rating and set a $430.00 price objective on shares of MongoDB in a research note on Monday, November 13th. Finally, Needham & Company LLC reissued a “buy” rating and set a $495.00 price objective on shares of MongoDB in a research note on Wednesday, January 17th. One investment analyst has rated the stock with a sell rating, four have assigned a hold rating and twenty-one have assigned a buy rating to the company. According to MarketBeat, the stock has a consensus rating of “Moderate Buy” and an average target price of $429.50.
Read Our Latest Report on MongoDB
Insiders Place Their Bets
In other MongoDB news, Director Dwight A. Merriman sold 6,000 shares of the company’s stock in a transaction that occurred on Monday, February 5th. The stock was sold at an average price of $437.99, for a total value of $2,627,940.00. Following the completion of the transaction, the director now owns 1,168,784 shares in the company, valued at approximately $511,915,704.16. The transaction was disclosed in a document filed with the SEC, which can be accessed through this hyperlink. In other MongoDB news, Director Dwight A. Merriman sold 6,000 shares of the company’s stock in a transaction that occurred on Monday, February 5th. The stock was sold at an average price of $437.99, for a total value of $2,627,940.00. Following the completion of the transaction, the director now owns 1,168,784 shares in the company, valued at approximately $511,915,704.16. The transaction was disclosed in a document filed with the SEC, which can be accessed through this hyperlink. Also, Director Dwight A. Merriman sold 1,000 shares of the business’s stock in a transaction dated Monday, January 22nd. The stock was sold at an average price of $420.00, for a total value of $420,000.00. Following the completion of the transaction, the director now owns 528,896 shares in the company, valued at approximately $222,136,320. The disclosure for this sale can be found here. Insiders sold a total of 81,777 shares of company stock worth $33,554,031 in the last 90 days. 4.80% of the stock is owned by company insiders.
About MongoDB
MongoDB, Inc provides general purpose database platform worldwide. The company offers MongoDB Atlas, a hosted multi-cloud database-as-a-service solution; MongoDB Enterprise Advanced, a commercial database server for enterprise customers to run in the cloud, on-premise, or in a hybrid environment; and Community Server, a free-to-download version of its database, which includes the functionality that developers need to get started with MongoDB.
See Also
Want to see what other hedge funds are holding MDB? Visit HoldingsChannel.com to get the latest 13F filings and insider trades for MongoDB, Inc. (NASDAQ:MDB – Free Report).
Receive News & Ratings for MongoDB Daily – Enter your email address below to receive a concise daily summary of the latest news and analysts’ ratings for MongoDB and related companies with MarketBeat.com’s FREE daily email newsletter.
Article originally posted on mongodb google news. Visit mongodb google news
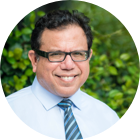
MMS • Matt Saunders
Article originally posted on InfoQ. Visit InfoQ
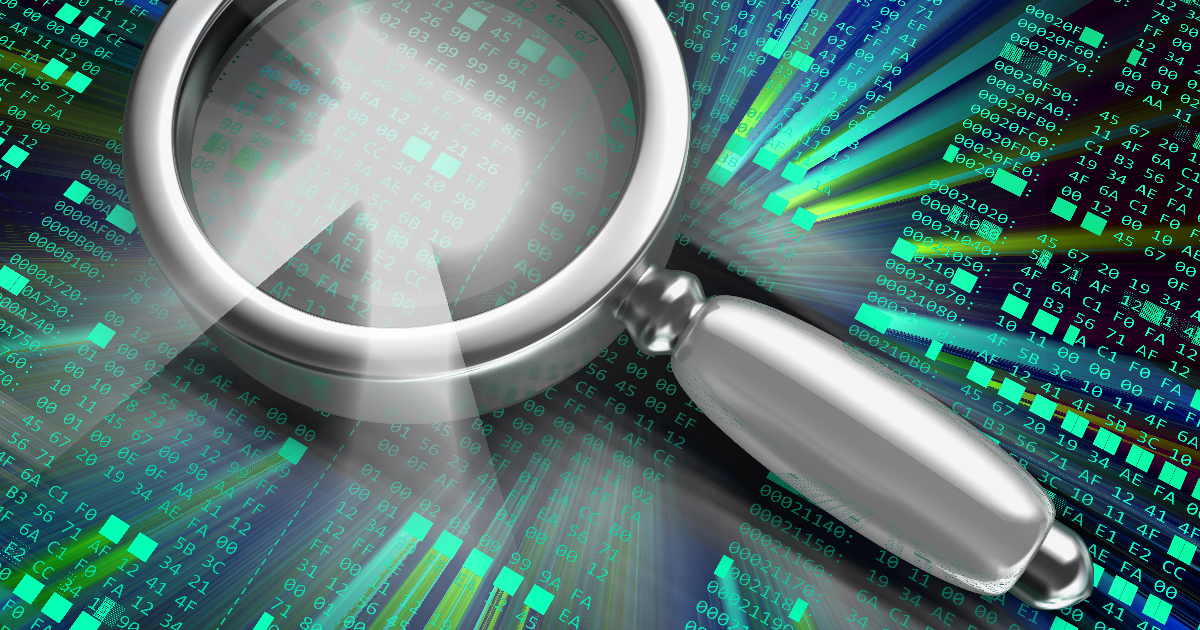
Infrastructure automation software company HashiCorp have announced a limited beta phase for HCP Vault Radar, a Software-as-a-Service (SaaS) based secrets discovery product. HCP Vault Radar is a secret scanning product that focuses on the proactive discovery of unmanaged or leaked secrets, allowing organizations to take swift action if secret information is exposed.
Following an alpha stage that began in October 2023, this beta release showcases new capabilities and integrations designed to bolster security for organizations managing sensitive information. The beta release adds role and attribute-based access controls (RBAC/ABAC). RBAC allows organizations to grant access by roles, while ABAC offers highly granular controls governing access based on user and object characteristics, action types, and more. These features enhance the ability to manage permissions, audit privileges, and comply with regulatory requirements efficiently.
HCP Vault Radar supports secret scanning from both a command line interface (CLI) and the HCP portal. The beta release expands on the data sources that can be used, now including Git-based version control systems, AWS Parameter Store, Confluence, Docker images, and Terraform Cloud and Terraform Enterprise. This addition enables users to scan a broader range of platforms than before.
Radar categorizes and ranks exposed data based on its level of risk, and as well as secrets also looks for PII (personally identifiable information) and non-inclusive language, scoring these risks appropriately. This allows DevOps and Security teams to prioritize remediation efforts effectively.
HCP Vault Radar also integrates with HashiCorp Vault, to allow Radar to scan supported data sources for leaked secrets actively in use within Vault. By cross-referencing with either Vault Enterprise or Vault Community, HCP Vault Radar provides an enhanced risk rating for discovered secrets. This prioritization ensures that organizations can address the most critical issues promptly.
HCP Vault Radar builds on HashiCorp Vault’s secrets lifecycle management functionality. Its automated scanning and ongoing detection capabilities empower organizations to be proactive in identifying and remediating unmanaged secrets before they pose a security risk. The product is currently in a private beta program, and organizations interested in participating can sign up for updates to be considered for inclusion.
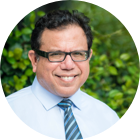
MMS • Michael Redlich
Article originally posted on InfoQ. Visit InfoQ
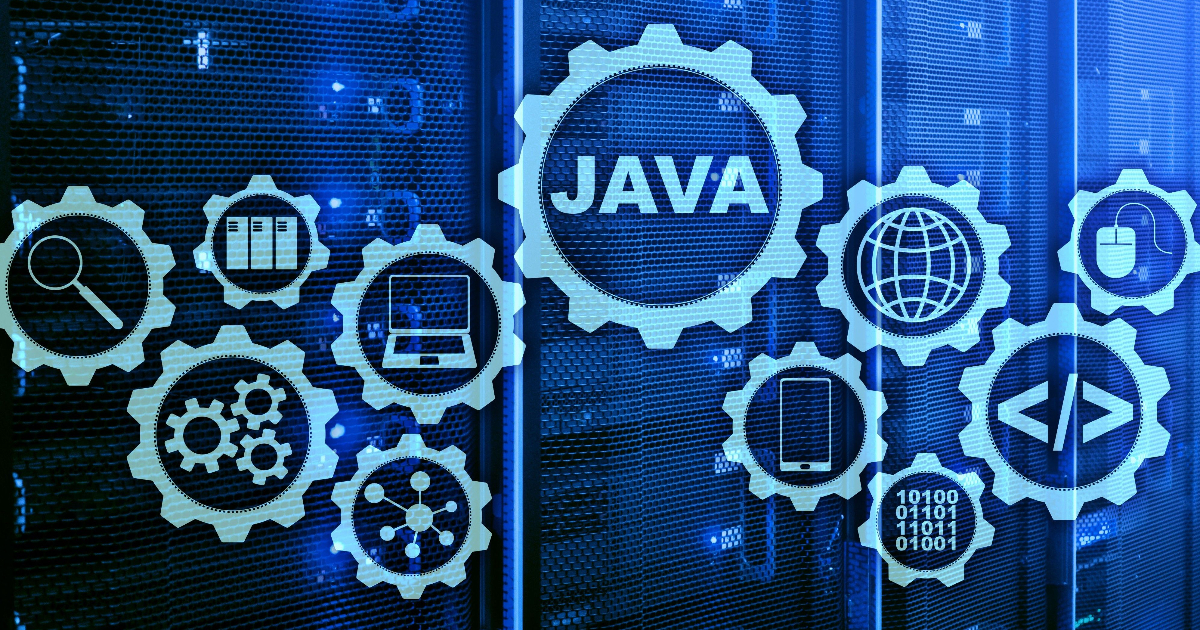
This week’s Java roundup for February 5th, 2024 features news highlighting: the first release candidate of JDK 22, JBoss Enterprise Application Platform 8.0, IBM Semeru Runtimes first quarter 2024 updates, LangChain4j 0.27.0, and multiple point releases for Micronaut, Helidon and Eclipse Vert.x.
JDK 23
Build 9 of the JDK 23 early-access builds was made available this past week featuring updates from Build 8 that include fixes for various issues. More details on this release may be found in the release notes.
JDK 22
Build 35 of the JDK 22 early-access builds was also made available this past week featuring updates from Build 34 that include fixes to various issues. Further details on this build may be found in the release notes.
As per the JDK 22 release schedule, Mark Reinhold, chief architect, Java Platform Group at Oracle, formally declared that JDK 21 has entered its first release candidate as there are no unresolved P1 bugs in Build 35. The anticipated GA release is scheduled for March 19, 2024.
The final set of 12 features for the GA release in March 2024 will include:
For JDK 23 and JDK 22, developers are encouraged to report bugs via the Java Bug Database.
Jakarta EE 11
In his weekly Hashtag Jakarta EE blog, Ivar Grimstad, Jakarta EE developer advocate at the Eclipse Foundation, has provided an update on the road to Jakarta EE 11 with a more formal milestone release plan that follows up on the recent release of Jakarta EE 11-M1. Developers can expect milestones 2, 3 and 4 to be released in March, April and May 2024, respectively. As Grimstad stated: “It is the first time we are using Milestones for a Jakarta EE release. Hopefully, it will turn out to be a good idea that will help us complete the release as planned in June/July this year [2024].”
Eclipse GlassFish
The second milestone release of GlassFish 8.0.0 delivers notable changes such as: removal of CDI tests using the @ManagedBean
annotation due to the Jakarta Managed Beans specification having been removed from the Jakarta EE Platform; a resolution to the startserv
script that incorrectly reported a bash syntax error; and a resolution to the ConcurrentModificationException
in the context map propagator. More details on this release may be found in the release notes.
Spring Framework
The release of Spring Tools 4.21.1 features bug fixes, early access builds for Eclipse 2024-03 milestone, and improvements: availability of viewing and editing of log levels in VSCode for live running Spring Boot apps, if enabled on the the application via Spring Boot Actuators; and the ability to show “Refactor Preview” in VSCode before applying the changes from OpenRewrite recipes. Further details on this release may be found in the release notes.
JBoss Enterprise Application Platform
Red Hat has released version 8.0 of the JBoss Enterprise Application Platform extends Java support in the cloud with security enhancements, improved cloud workflow tools, and compatibility with Jakarta EE 10, contributing to streamlined application modernization for customers and continued support for enterprise Java application development. InfoQ will follow up with a more detailed news story.
Micronaut
The Micronaut Foundation has released version 4.3.0 of the Micronaut Framework featuring Micronaut Core 4.3.4, bug fixes, dependency upgrades and updates to modules such as: Micronaut GCP, Micronaut Liquibase, Micronaut Data and Micronaut Validation. New modules, Micronaut Chatbots and Micronaut EclipseStore, were also introduced in this version. More details on this release may be found in the release notes.
Similarly, the follow up release of Micronaut Framework 4.3.1 features Micronaut Core 4.3.5, bug fixes, dependency upgrades and updates to modules: Micronaut Security, Micronaut Data, and Micronaut Logging. Further details on this release may be found in the release notes.
IBM Semeru Runtimes
IBM has released version 9.0 of Universal Base Image (UBI) minimal Liberty container images, a stripped down image which allows for the production of smaller application images, with support for Semeru Runtimes Java 21 JRE. The UBI 9 minimal images are available starting with the release of Open Liberty 24.0.0.1.
IBM has also released the 1Q2024 quarterly update of the Semeru Runtime, Open Edition versions 21.0.2, 17.0.10, 11.0.22 and 8.0.402 based on Eclipse OpenJ9 0.43 and OpenJDK jdk-21.0.2+13, jdk-17.0.10+7, jdk-11.0.22+7 and jdk8u402-b06, respectively. This release contains the latest CPU and security fixes from the Oracle Critical Patch Update for January 2024.
Quarkus
Red Hat has released version 3.7.2 of Quarkus with notable changes such as: a resolution to SSL requests hang when an endpoint returns a CompletableFuture
; always execute the OpenIDConnectSecurityFilter
class at runtime to ensure that the OpenAPI document will use the runtime value of the quarkus.oidc.auth-server-url
property; and a new CheckCrossReferences
class to check the cross references of canonical IDs. More details on this release may be found in the changelog.
Helidon
The release of Helidon 4.0.5 delivers notable resolutions to issues such as: tests defined in the KafkaSeTest
class failing on Windows OS; a NullPointerException
from a user test by adding a null
check for the resource path in the HelidonTelemetryContainerFilter
class; and problems handling character encodings in URIs. Further details on this release may be found in the changelog.
Similarly, Helidon 3.2.6 has been released providing dependency upgrades and notable changes such as: manually count the number of offered tasks instead of relying solely on the inaccurate value returned by the getActiveCount()
method defined in the ThreadPool
class; and a resolution to the currentSpan()
method defined in the TracerProviderHelper
class throwing a NullPointerException
in situations where an implementation of the TracerProvider
class is null
. More details on this release may be found in the changelog.
Hibernate
The release of Hibernate ORM 6.4.4.Final ships with dependency upgrades and resolutions to issues such as: a NullPointerException
upon using the default instance of the BytecodeProvider
interface after upgrading WildFly to Hibernate 6.4.3; a memory leak using the BasicTypeRegistry
class; and an IllegalStateException
due to an unsupported combination of tuples in queries. Further details on this release may be found in the list of issues.
Eclipse Vert.x
Versions 4.5.3 and 4.4.8 of Eclipse Vert.x both ship with notable resolutions to issues such as: CVE-2024-1300, a vulnerability that allows an attacker to send Transport Layer Security (TLS) client “hello” messages with fake server names, triggering a JVM out-of-memory error due to a memory leak in TCP servers configured with support for TLS and Server Name Indication (SNI); a NullPointerException
in the decodeError()
method defined in the PgDecoder
class; and temporary files generated by the WebClient
interface not being removed. More details on these releases may be found in the release notes for version 4.5.3 and version 4.4.8.
Eclipse JKube
The release of Eclipse JKube 1.16.0 provides notable changes such as: support for the Spring Boot application properties placeholders generated from the configuration; a new JKubeArchiveDecompressor
class with initial support for .tgz
and .zip
files; and support for parsing Docker image names with IPv6 address literals. Further details on this release may be found in the release notes.
Infinispan
The release of Infinispan 14.0.24 provides many dependency upgrades and improvements: prevent leaks from an implementation of the Java MBeanServer
interface from within the HotRodClient
class; and enable the Insights Java Client by default. More details on this release may be found in the release notes.
LangChain for Java
Version 0.27.0 of LangChain for Java (LangChain4j) provides many bug fixes, new integrations with Infinispan and MongoDB, and notable updates such as: improved support for AstraDB and Cassandra; a fallback strategy for the LanguageModelQueryRouter
class; and an enhance to the ServiceOutputParser
class that allows the outputFormatInstructions()
method to document nested objects in the jsonStructure()
method. Further details on this release may be found in the release notes.
JHipster
Version 1.4.0 of JHipster Lite have been released to deliver bug fixes, dependency upgrades and new features/enhancements such as: a new module to auto-enable Spring local profile for development; support for programmatically declare dependencies in a Maven profile; and a rename of getJavaVersion()
method to javaVersion()
to align with the JHipster Lite convention of not using the get
prefix. More details on this release may be found in the release notes.
Testcontainers for Java
The release of Testcontainers for Java 1.19.5 ships with a downgrade of Apache Commons Compress from version 1.25.0 to version 1.24.0 to avoid classpath conflicts due to a recent change in Commons Compress 1.25.0. Further details on this release may be found in the release notes.
Java Operator SDK
The release of Java Operator SDK 4.8.0 features notable changes such as: improved logging of conflicting exceptions; the ability for multiple controllers reconciling same resource type, but with different labels; and a resolution to multiple dependents of same type exceptions. More details on this release may be found in the release notes.
Multik
Version 0.2.3 of Multik, the multidimensional array library for Kotlin, has been migrated to Kotlin 1.9.22. New features include: use of pinned arrays to optimize the sin
, cos
, log
, exp
functions in Kotlin/Native; implementation of complex numbers in Kotlin/Native using the Vector128
class; and enhanced performance on Windows OS and Apple Silicon processors. Further details on this release may be found in the release notes.
Gradle
Gradle 7.6.4, the fourth maintenance release, features: an upgrade to Apache Ant 1.10.14 to address CVE-2020-11979, a vulnerability in which an attacker can inject modified source files into a build process due to the fixcrlf
task deleting temporary files and creating new ones without the permissions assigned to the current user; an upgrade to Google Guava 32.1.1 to address CVE-2023-2976, a vulnerability in the FileBackedOutputStream
class that allows other users and apps on the machine with access to the default Java temporary directory to be able to access the files created by the class; and an upgrade to Apache Ivy 2.5.2 to address CVE-2022-46751, a vulnerability in Apache Ivy in which an attacker can exfiltrate data, access resources only the machine running Ivy, or disturb the execution of Ivy in different ways due to improper restriction of XML External Entity Reference. More details on this release may be found in the release notes.