Month: March 2024
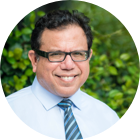
MMS • RSS
Posted on nosqlgooglealerts. Visit nosqlgooglealerts
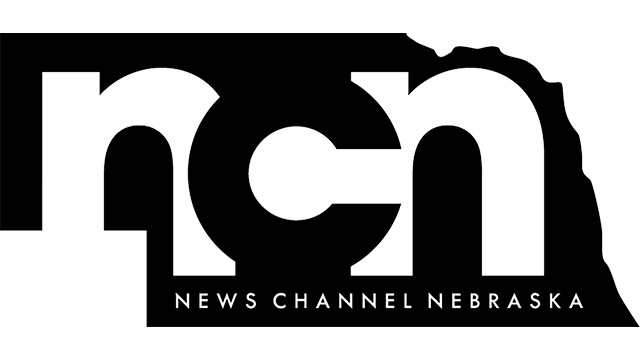
Press Release
This company simplifies data integration across relational and NoSQL databases, SaaS tools, and APIs.Peaka, a market-leading data integration platform, announces the launch of its zero-ETL solution, modernizing the…
WildFly 31 Delivers Support for Jakarta EE 10 and the New WildFly Glow Provisioning Tools
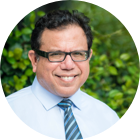
MMS • Shaaf Syed
Article originally posted on InfoQ. Visit InfoQ
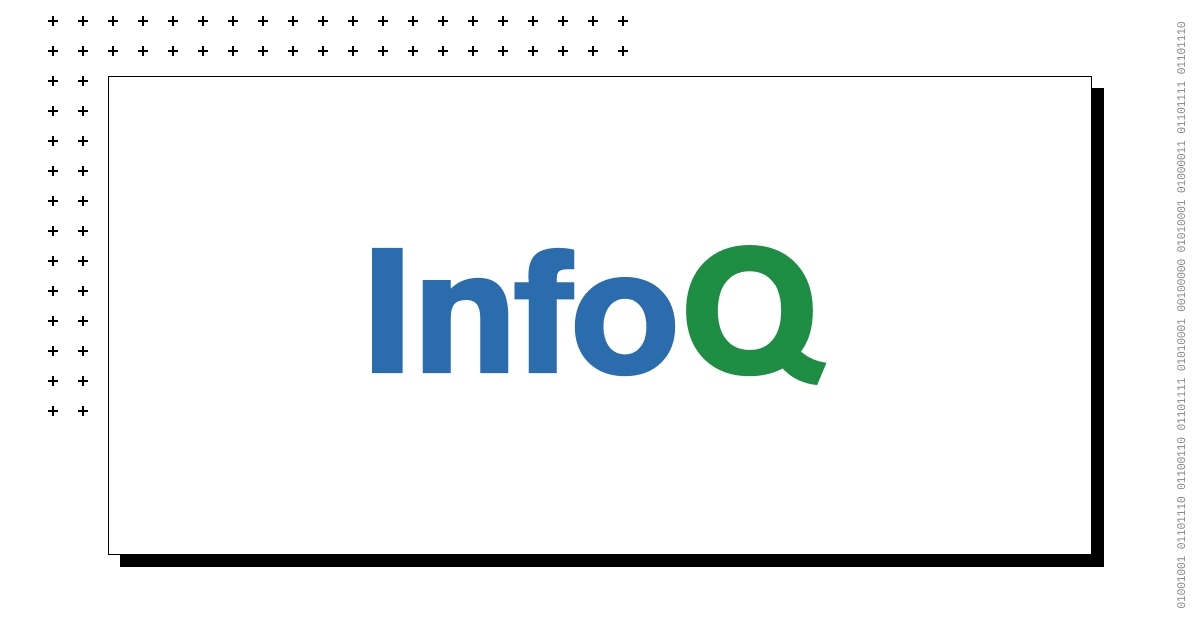
The WildFly community has released WildFly 31, which delivers support for Jakarta MVC 2.1, a CLI tool, and WildFly Glow, a Maven plugin that analyzes the usage of subsystems and suggests a more lightweight runtime, e.g., running in Docker containers. WildFly 31 also introduces stability levels so users can choose features more carefully for the different use cases. Other updates include an upgrade to MicroProfile 6.1, Hibernate 6.4.2, and JakartaEE 10. WildFly core now also supports JDK 21, the latest LTS version of the JDK.
According to Brian Stansberry, Sr. Principal Software Engineer at Red Hat, the journey to Glow started a couple of years ago by introducing Galleon, a provisioning tool enabling containerized deployments. Glow can scan the WAR file content and identify the required Galleon layers based on rulesets. e.g., jaxrs rule. WildFly Glow also detects, e.g., the data source being used and suggests the correct add-ons required. Once the identification process is complete, the correct Galleon layers are then selected. Furthermore, the Glow CLI enables users to provision a WildFly server, a bootable JAR or to produce a Docker container image that can run on orchestration platforms like Kubernetes.
WildFly 31 implements the JakartaEE 10 Platform, the Web Profile, and the Core Profile. The Core Profile can run with the latest LTS of JDK 21, whereas the JakartaEE 10 and Core Profile can run with JDK 11 and 17. The recommended version to run WildFly 31 is JDK 17. WildFly 31 also implements most of the core MicroProfile specifications having dropped support for MicroProfile Metrics in favor of Micrometer, rendering it incompatible with the MicroProfile specifications. The WildFly team has also shared the compatibility evidence for MicroProfile 6.1 that provides more details on the implemented specifications. Furthermore, this release also introduces two new quickstarts, Micrometer and MicroProfile LRA.
A new community feature allows users to export WildFly configuration so that any other instance of WildFly can be booted with it, thereby creating a configuration replica. Community features are part of the stability levels also introduced in this release. Stability levels (experimental, preview, community, default) give users more visibility into upcoming and experimental features and provide the ability to opt out of features.
The WildFly team also seems to have focused on more accessible learning options. All quickstarts are now deployable as ZIP files or bootable JARs. Where applicable, helm charts are used for deployment on Kubernetes derivatives like Red Hat OpenShift. Furthermore, the quickstarts also include tests such as smoke tests, the Getting Started maven archetype, the Get Started page, and user guides.
A detailed list of release notes is available on the WildFly release page.
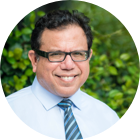
MMS • Thomas Betts
Article originally posted on InfoQ. Visit InfoQ
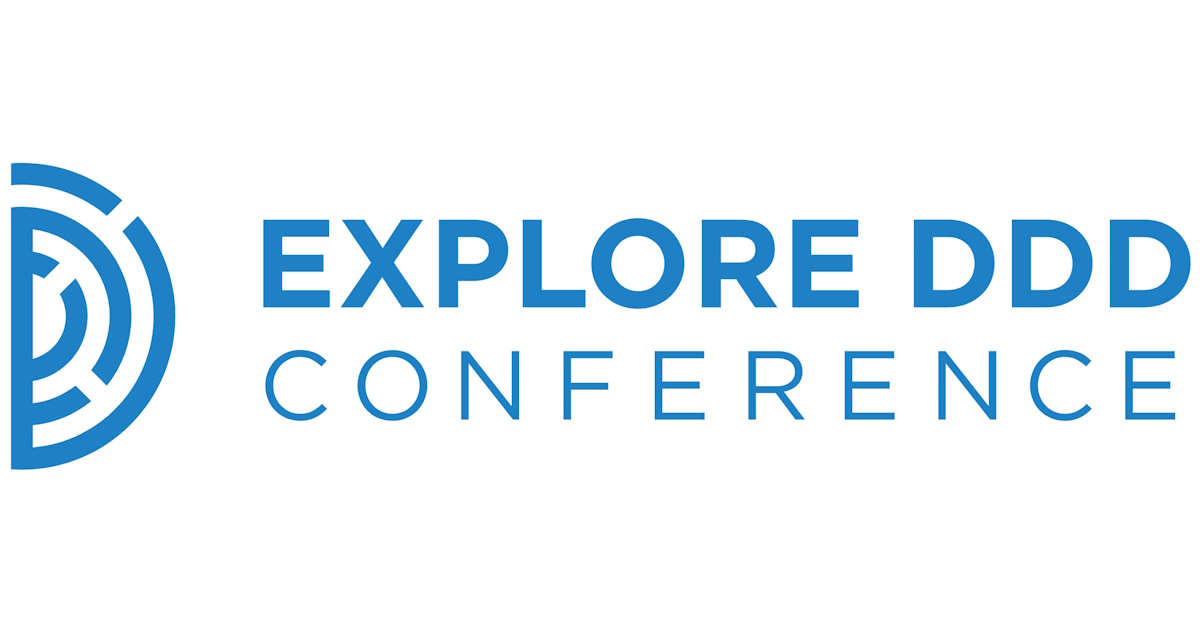
In his keynote presentation at Explore DDD, Eric Evans, author of Domain-Driven Design, argued that software designers need to look for innovative ways to incorporate large language models into their systems. He encouraged everyone to start learning about LLMs and conducting experiments now, and sharing the results and learnings from those experiments with the community.
Evans believes there can be good combinations of DDD and AI-oriented software. He said, “because some parts of a complex system never fit into structured parts of domain models, we throw those over to humans to handle. Maybe we’ll have some hard-coded, some human-handled, and a third, LLM-supported category.”
Using language familiar to DDD practitioners, he said a trained language model is a bounded context. Instead of using models trained on broad language and that are intended for general purposes, such as ChatGPT, training a language model on a ubiquitous language of a bounded context makes it far more useful for specific needs.
With generic LLMs, someone has to write careful, artificial prompts to achieve a desired response. Instead, Evans proposed having several fine-tuned models, each intended for a different purpose. He sees this as a strong separation of concerns. He predicts future domain modelers will identify tasks and subdomains that involve interpreting natural language input, and will naturally slot that into their designs. The infrastructure isn’t quite ready for that yet, but trends suggest it will come soon.
Evans emphasized that his thoughts must be considered in the context of when he was speaking, on March 14, 2024, because the landscape is changing so rapidly. Six months ago, he did not know enough about the subject, and a year from now his comments may be irrelevant. He compared our current situation to the late 90s, when he learned multiple ways to build a website, none of which would be applicable today.
Throughout the conference, other notable voices in the DDD community responded to Evans’ thoughts. Vaughn Vernon, author of Implementing Domain-Driven Design, was largely supportive of the idea of finding novel uses for LLMs beyond the common chatbot. In his vision for self-healing software, he sees a place for a tool like ChatGPT to respond to runtime exceptions, and be a “fix suggester” that automatically creates a pull request with suggested code to resolve the bug.
However, some people remain skeptical about all the benefits of LLMs. During a panel discussion on the intersection of DDD and LLMs, Chris Richardson, author of Microservice Patterns, expressed concerns about the high monetary and computing costs of LLMs. When Richardson wondered if any service that operated an LLM could ever turn a profit, Evans replied that fine tuning makes an inexpensive model cheaper and faster than an expensive model. Fellow panelist, Jessica Kerr, a principal developer advocate at Honeycomb.io, said, “we need to find what’s valuable, then make it economical.”
During his keynote, Evans went into detail about some of the experiments he conducted as part of his personal education into the capabilities of LLMs. At first, working with game designer Reed Berkowitz, he tried using ChatGPT to make a non-player character (NPC) respond to player input. An evolution of prompt engineering led him to the realization that the responses were more consistent if broken into smaller chunks, rather than one, long prompt. This approach follows his ideas of DDD breaking down complex problems.
The need for smaller, more specialized prompts naturally led to wanting a more specialized model, which would both provide better output while also being more performant and cost effective. His goal in explaining his research methods was to show how useful it can be to experiment with the technology. Although frustrating at times, the process was immensely rewarding, and many attendees said they could relate to the sense of satisfaction when you learn how to get something new working for the first time.
The Explore DDD conference took place in Denver, Colorado, from March 12-15, 2024. Most presentations during the conference were recorded, and will be posted to the @ExploreDDD YouTube channel over the next several weeks, and shared on the Explore DDD LinkedIn page, starting with the opening keynote by Eric Evans.
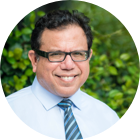
MMS • Sergio De Simone
Article originally posted on InfoQ. Visit InfoQ
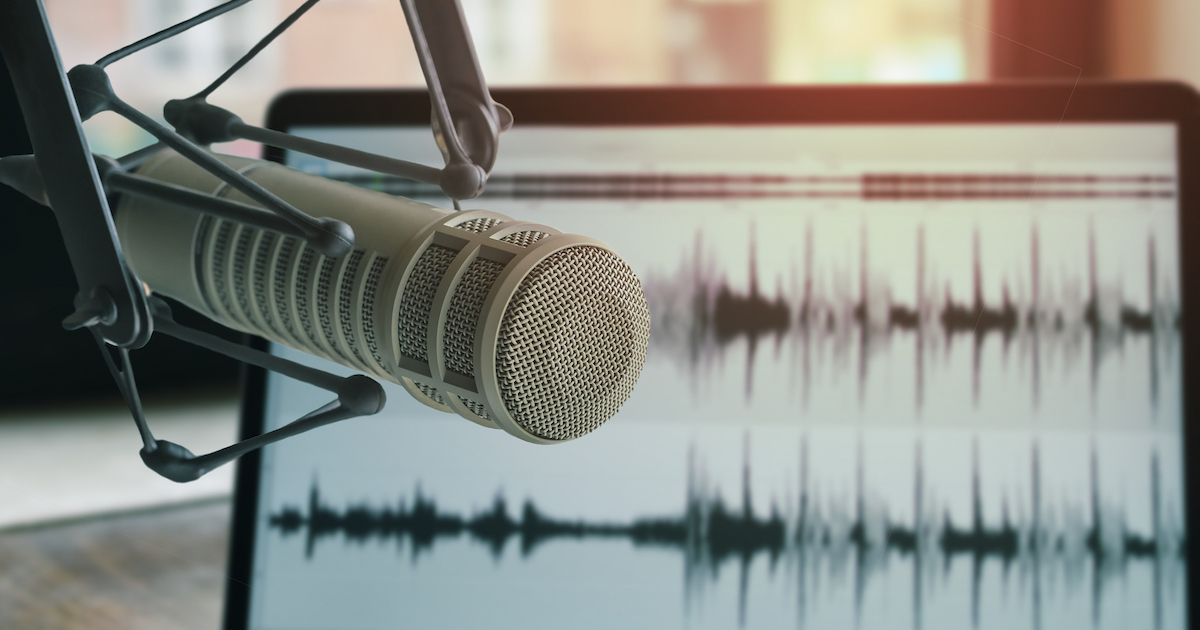
Google has announced a new text-to-speech engine for Wear OS, its Android variant aimed at smartwatches and other wearables, supporting over 50 languages and faster than its predecessor thanks to using smaller ML models.
According to Google’s Ouiam Koubaa and Yingzhe Li, the new text-to-speech engine is particularly geared for low-memory devices, such as those that power services for which wearables are most suited, including accessibility services, exercise apps, navigation-cues, and reading-aloud apps.
Text-to-speech turns text into natural-sounding speech across more than 50 languages powered by Google’s machine learning (ML) technology. The new text-to-speech engine on Wear OS uses smaller and more efficient prosody ML models to bring faster synthesis on Wear OS devices.
The new text-to-speech engine does not introduce new APIs to synthesize speech, meaning developers can keep using the previously existing speak method, along with the rest of the methods previously available.
Developers should keep in mind that the new engine takes about 10 seconds to get ready when the app is initialized. Therefore, apps that want to use speech right after launch is completed should initialize the engine as soon as possible by calling TextToSpeech(applicationContext, callback)
and synthesize the desired text from the passed callback
.
An additional caveat concerns the possibility that the new engine can synthesize speech in a language other than the user’s preferred language. This may happen, for example, when sending an emergency call, in which case the language corresponding to the actual locale the user is in is preferred over the user’s chosen UI language.
The new text-to-speech engine can be used on devices running Wear OS 4, released last July, or higher.
Besides text-to-speech synthesis, Wear OS also provides a speech recognition service through the SpeechRecognizer API, which is though not appropriate for continuous recognition since it relies on remote services.
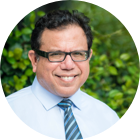
MMS • Aditya Kulkarni
Article originally posted on InfoQ. Visit InfoQ
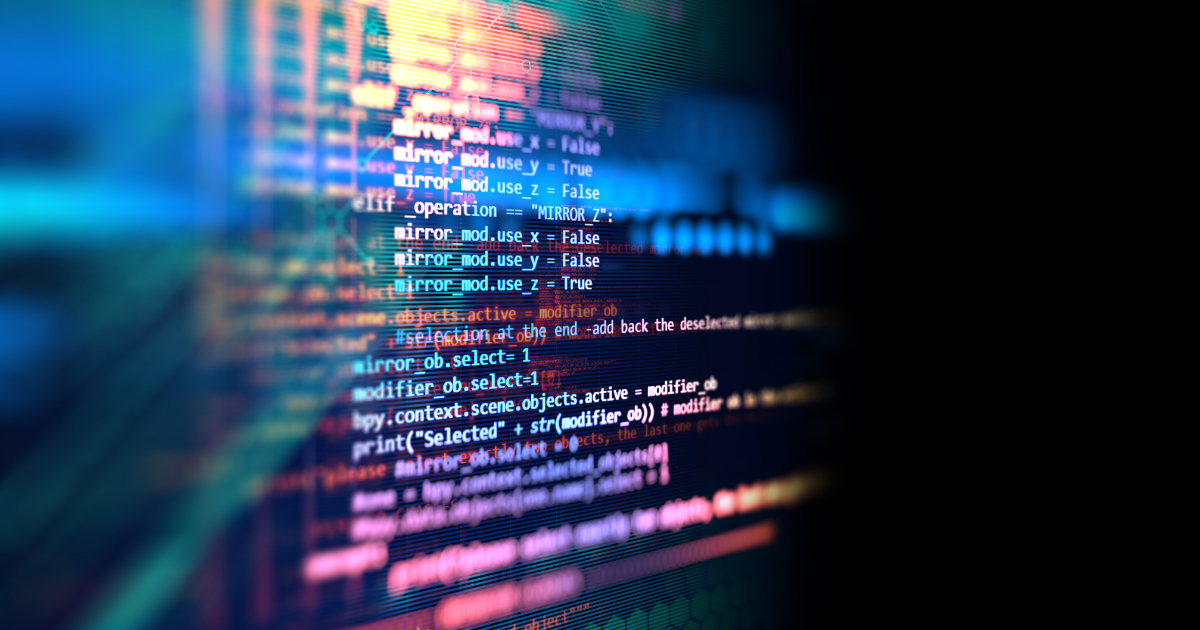
GitHub recently announced an enhancement to their GitHub Actions-hosted runners. Moving forward, workflows from public repositories on Linux or Windows that utilize GitHub’s default labels will be executed on the new 4-vCPU runners.
Larissa Fortuna, Product Manager at GitHub talked about the upgraded infrastructure in a blog post. This enhanced infrastructure allows for a performance increase of up to 25% on most CI/CD tasks, without needing any changes to configurations. Fortuna noted that GitHub Actions has been available at no cost for public repositories since its launch in 2019. Despite its widespread adoption by open source communities, the platform has consistently utilized the same 2-vCPU based virtual machines.
Starting December 1, 2023, GitHub started upgrading the Linux and Windows Action runners to 4-vCPU virtual machines with 16 GiB of memory, doubling their previous capacity. These enhancements will lead to quicker feedback on the pull requests and reduce the wait time for builds to complete. Teams handling larger workloads will benefit from machines that offer double the memory.
GitHub Actions has become essential for open-source projects that offer free, easy-to-use automation and build servers. This rapid adoption is also due to the open-source community and the GitHub Marketplace, which houses over 20,000 actions and apps. This allows developers of all organization sizes to enhance their workflows with GitHub Actions and Apps.
Earlier this year, GitHub made headlines as it integrated AI to enhance the developer experience on its platform, and offered interactive AI features through GitHub Copilot Enterprise and GitHub Copilot Chat. These tools, including a context-aware AI assistant powered by GPT-4, enable users to interact with Copilot directly in their browser and within their Integrated Development Environment (IDE), assisting with a wide range of tasks from explaining coding concepts to identifying security vulnerabilities and writing unit tests.
Martin Woodward, GitHub’s Vice President of Developer Relations, commented on the GitHub Actions-hosted runners, mentioning,
“GitHub is the home for the open source community, so I’m thrilled we’ve been able to give all open source projects access to Linux and Windows machines that have twice the v-CPUs, twice the memory and 10 times the storage for their builds, for free. This investment will give valuable time back to the maintainers of open source projects who benefited from over 7 billion build minutes with GitHub Actions in the past year alone.”
To start using the upgraded infrastructure, public repositories will need to execute the public workflows with any of the current Ubuntu or Windows labels, and they will automatically operate on the new 4-core hosted runners.
Java News Roundup: New JEP Drafts, Infinispan 15, Payara Platform, Alpaquita Containers with CRaC
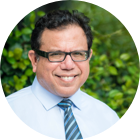
MMS • Michael Redlich
Article originally posted on InfoQ. Visit InfoQ
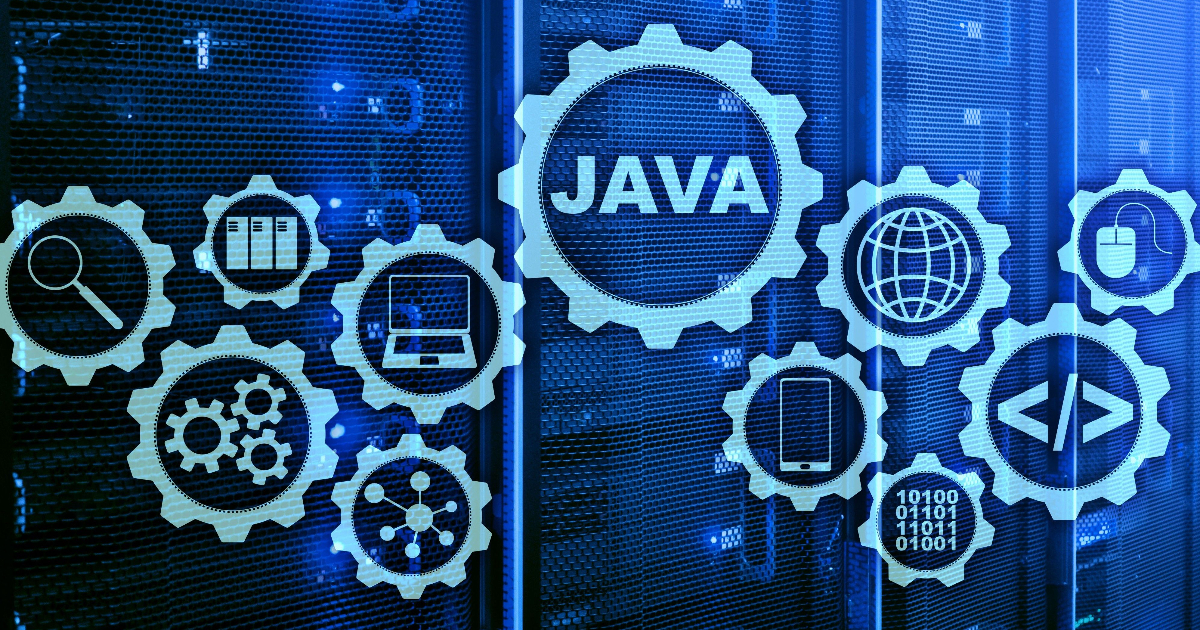
This week’s Java roundup for March 11th, 2024 features news highlighting: new JEP drafts, Stream Gatherers (Second Preview) and Hot Code Heap; Infinispan 15; the March 2024 edition of Payara Platform; Alpaquita Containers with CRaC; the first release candidate of JobRunr 7.0; and milestone and point releases for Spring projects, Quarkus, Helidon and Micronaut.
OpenJDK
Viktor Klang, Software Architect, Java Platform Group at Oracle, has introduced JEP Draft 8327844, Stream Gatherers (Second Preview), that proposes a second round of preview from the previous round, namely JEP 461, Stream Gatherers (Preview), to be delivered in the upcoming release of JDK 22. This will allow additional time for feedback and more experience with this feature with no user-facing changes over JEP 461. This feature was designed to enhance the Stream API to support custom intermediate operations that will “allow stream pipelines to transform data in ways that are not easily achievable with the existing built-in intermediate operations.” More details on this JEP may be found in the original design document and this InfoQ news story.
Dmitry Chuyko, Performance Architect at BellSoft, has introduced JEP Draft 8328186, Hot Code Heap, that proposes to “extend the segmented code cache with a new optional ‘hot’ code heap to compactly accommodate a part of non-profiled methods, and to extend the compiler control mechanism to mark certain methods as ‘hot’ so that they compile into the hot code heap.”
JDK 23
Build 14 of the JDK 23 early-access builds was made available this past week featuring updates from Build 13 that include fixes for various issues. More details on this release may be found in the release notes.
JDK 22
Build 36 remains the current build in the JDK 22 early-access builds. Further details on this build may be found in the release notes.
For JDK 23 and JDK 22, developers are encouraged to report bugs via the Java Bug Database.
BellSoft
BellSoft has released their Alpaquita Containers product, which includes Alpaquita Linux and Liberica JDK, with support for Coordinated Restore at Checkpoint (CRaC). Performance measurements reveal a 164x faster startup and 1.1x smaller images. InfoQ will follow up with a more detailed news story.
Spring Framework
Versions 6.1.5, 6.0.18 and 5.3.33 of Spring Framework have been released to primarily address CVE-2024-22259, Spring Framework URL Parsing with Host Validation (2nd report), a vulnerability in which applications that use the UriComponentsBuilder
class to parse an externally provided URL and perform validation checks on the host of the parsed URL, may be vulnerable to an open redirect attack or a server-side-request forgery attack if the URL is used after passing validation checks. New features include: allow the UriTemplate
class to be built with an empty template; and a refinement of the getContentLength()
method defined in classes that implement the HttpMessageConverter
interface to return value null safety. These versions will be shipped with the upcoming releases of Spring Boot 3.2.4 and Spring Boot 3.1.10, respectively. More details on these releases may be found in the release notes for version 6.1.5, version 6.0.18 and version 5.3.33.
The second milestone release of Spring Data 2024.0.0 provides new features: predicate-based QueryEngine for Spring Data Key-Value; and a transaction option derivation for MongoDB based on @Transactional
annotation labels. There were also upgrades to sub-projects such as: Spring Data Commons 3.3.0-M2; Spring Data MongoDB 4.3.0-M2; Spring Data Elasticsearch 5.3.0-M2; and Spring Data Neo4j 7.3.0-M2. More details on this release may be found in the release notes.
Similarly, versions 2023.1.4 and 2023.0.10 of Spring Data have been released providing bug fixes and respective dependency upgrades to sub-projects such as: Spring Data Commons 3.2.4 and 3.1.10; Spring Data MongoDB 4.2.4 and 4.1.10; Spring Data Elasticsearch 5.2.4 and 5.1.10; and Spring Data Neo4j 7.2.4 and 7.1.10. These versions may also be consumed by the upcoming releases of Spring Boot 3.2.4 and 3.1.10, respectively.
The release of Spring AI 0.8.1 delivers new features such as support for: the Google Gemini AI model; VertexAI Gemini Chat; Gemini Function Calling; and native compilation of Gemini applications. More details on this release may be found in the list of issues.
Payara
Payara has released their March 2024 edition of the Payara Platform that includes Community Edition 6.2024.3 and Enterprise Edition 6.12.0. Both editions feature notable changes such as: more control in system package selection with Apache Felix that streamlines configuration and reduces potential conflicts; a resolution for generated temporary files in the /tmp
folder upon deployment that weren’t getting deleted; and improved reliability to set the correct status when restarting a server instance from the Admin UI. More details on these releases may be found in the release notes for Community Edition 6.2024.3 and Enterprise Edition 6.12.0.
Micronaut
The Micronaut Foundation has released version 4.3.6 of the Micronaut Framework featuring Micronaut Core 4.3.11, bug fixes, improvements in documentation, and updates to modules: Micronaut Serialization, Micronaut Azure, Micronaut RxJava 3 and Micronaut Validation. Further details on this release may be found in the release notes.
Quarkus
Quarkus 3.2.11.Final, a maintenance LTS release, ships with dependency upgrades and security fixes to address:
- CVE-2024-25710, a denial of service caused by an infinite loop for a corrupted DUMP file.
- CVE-2024-1597, a PostgreSQL JDBC Driver vulnerability that allows an attacker to inject SQL if using
PreferQueryMode=SIMPLE
. - CVE-2024-1023, a memory leak due to the use of Netty
FastThreadLocal
data structures in Vert.x. - CVE-2024-1300, a memory leak when a TCP server is configured with TLS and SNI support.
- CVE-2024-1726 security checks for some inherited endpoints performed after serialization in RESTEasy Reactive may trigger a denial of service
More details on this release may be found in the changelog.
Helidon
The release of Helidon 4.0.6 provides notable changes such as: support for injecting instances of the UniversalConnectionPool
interface; a deprecation of the await(long, TimeUnit)
method defined in the Awaitable
interface in favor of await(Duration)
; and enhance the Status
class with additional standard HTTP status codes, namely: 203, Non-Authoritative Information; 207, Multi-Status; 507, Insufficient Storage; 508, Loop Detected; 510, Not Extended; and 511, Network Authentication Required. More details on this release may be found in the changelog.
Infinispan
Red Hat has released version 15.0.0 of Infinispan that delivers new features such as: a JDK 17 baseline; support for Jakarta EE; a connector to the Redis Serialization Protocol; support for distributed vector indexes and KNN queries; and improved tracing subsystem. More details on this release may be found in the release notes and InfoQ will follow up with a more detailed news story.
Micrometer
Version 1.13.0-M2 of Micrometer Metrics 1.13.0 delivers bug fixes, dependency upgrades and new features such as: align the JettyClientMetrics
class with Jetty 12; support for Prometheus 1.x; and support for the @Counted
annotation on classes with an update on the CountedAspect
class to handle when @Counted
annotates a class. More details on this release may be found in the release notes.
Similarly, versions 1.12.4 and 1.11.10 of Micrometer Metrics ship with bug fixes, dependency upgrades and a new feature in which the INSTANCE
field, defined in the DefaultHttpClientObservationConvention
class, was declared as final as being non-final seemed to be accidental. More details on these releases may be found in the release notes for version 1.12.4 and version 1.11.10.
Versions 1.3.0-M2, 1.2.4 and 1.1.11 of Micrometer Tracing provides bug fixes, dependency upgrades to Micrometer Metrics 1.13.0-M2, 1.12.4 and 1.11.10, respectively and a completed task that excludes the benchmarks module from the BOM because they are not published. More details on these releases may be found in the version 1.3.0-M2, version 1.2.4 and version 1.1.11.
Project Reactor
Project Reactor 2023.0.4, the fourth maintenance release, provides dependency upgrades to reactor-core 3.6.4
and reactor-netty 1.1.17
. There was also a realignment to version 2023.0.4 with the reactor-kafka 1.3.23
, reactor-pool 1.0.5
, reactor-addons 3.5.1
and reactor-kotlin-extensions 1.2.2
artifacts that remain unchanged. More details on this release may be found in the changelog.
Next, Project Reactor 2022.0.17, the seventeenth maintenance release, provides dependency upgrades to reactor-core 3.5.15
and reactor-netty 1.1.17
. There was also a realignment to version 2022.0.17 with the reactor-kafka 1.3.23
, reactor-pool 1.0.5
, reactor-addons 3.5.1
and reactor-kotlin-extensions 1.2.2
artifacts that remain unchanged. Further details on this release may be found in the changelog.
And finally, the release of Project Reactor 2020.0.42, codenamed Europium-SR42, provides dependency upgrades to reactor-core 3.4.36
and reactor-netty 1.0.43
and. There was also a realignment to version 2020.0.42 with the reactor-kafka 1.3.23
, reactor-pool 0.2.12
, reactor-addons 3.4.10
, reactor-kotlin-extensions 1.1.10
and reactor-rabbitmq 1.5.6
artifacts that remain unchanged. More details on this release may be found in the changelog.
Apache Software Foundation
Versions 5.0.0-alpha-7 and 4.0.20 of Apache Groovy feature bug fixes, dependency upgrades and an improvement to the getMessage()
method defined in the MissingMethodException
class to eliminate truncating the error message at 60 characters. More details on these releases may be found in the release notes for version 5.0.0-alpha-7 and version 4.0.20.
The release of Apache Camel 4.4.1 provides bug fixes, dependency upgrades and notable improvements such as: support for the ${}
expressions with dependencies in Camel JBang; and port validation in Camel GRPC should check if a port was specified. More details on this release may be found in the release notes.
Eclipse Foundation
Version 4.5.5 of Eclipse Vert.x has been released delivering notable changes such as: a deprecation of the toJson()
method defined in the Buffer
interface in favor of toJsonValue()
; a resolution to an OutOfMemoryException
after an update to the Certificate Revocation List; and a new requirement that an implementation of the CompositeFuture
interface must unregister itself against its component upon completion. More details on this release may be found in the release notes and list of deprecations and breaking changes.
The release of Eclipse Mojarra 4.0.6, the compatible implementation to the Jakarta Faces specification, ships with notable changes such as: ensure that the getViews()
method defined in the ViewHandler
class also returns programmatic facelets; and removal of the SKIP_ITERATION
enumeration as it was superseded by the VisitHint
enumeration. More details on this release may be found in the release notes.
Piranha
The release of Piranha 24.2.0 delivers notable changes to the Piranha CLI such as: the ability to generate a macOS GraalVM binary; and the addition of a version
and coreprofile
sub-commands. Further details on this release may be found in their documentation and issue tracker.
JobRunr
The first release candidate of JobRunr 7.0.0, a library for background processing in Java that is distributed and backed by persistent storage, features bug fixes, enhancements and new features: built-in support for virtual threads that are enabled by default when using JDK 21; and the InMemoryStorageProvider
class now allows for a poll interval as small as 200ms that is useful for testing. Breaking changes include: the delete(String id)
method in the JobScheduler
class has been renamed to deleteRecurringJob(String id)
; and updates to the StorageProvider
interface and the Page
and PageRequest
classes that include new features. More details on this release may be found in the release notes.
JBang
Version 0.115.0 of JBang delivers bug fixes and new features such as: arguments passed to an alias are now appended (instead of being replaced) to any arguments that are already defined in the alias; and support for specifying system properties when using the jbang app install
command. More details on this release may be found in the release notes.
LangChain4j
Version 0.28.0 of LangChain for Java (LangChain4j) provides many bug fixes, new integrations with Anthropic and Zhipu AI, and notable updates such as: a new Filter
API to support embedded stores like Milvus and Pinecone; the ability to load recursively and with glob/regex filtering with the FileSystemDocumentLoader
class; and an implementation of the missing parameters in the rest of the Azure OpenAI APIs with a clean up of the existing responseFormat
parameter so that all parameters are in the consistently in the same order. Further details on this release may be found in the release notes.
Java Operator SDK
The release of Java Operator SDK 4.8.1 features dependency upgrades and notable improvements such as: explicit use of the fixed thread pool; add logging for tracing issues with events; and changes to the primary to secondary index edge case for the dynamic mapper in the DefaultPrimaryToSecondaryIndex
class. More details on this release may be found in the release notes.
Gradle
The third release candidate of Gradle 8.7 provides continuous improvement in: support for Java 22 for compiling, testing, and running JVM-based projects; build cache improvements for Groovy DSL script compilation; and improvements to lazy configuration, error and warning messages, the configuration cache, and the Kotlin DSL. More details on this release may be found in the release notes.
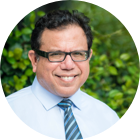
MMS • Debasish Ray Chawdhuri
Article originally posted on InfoQ. Visit InfoQ
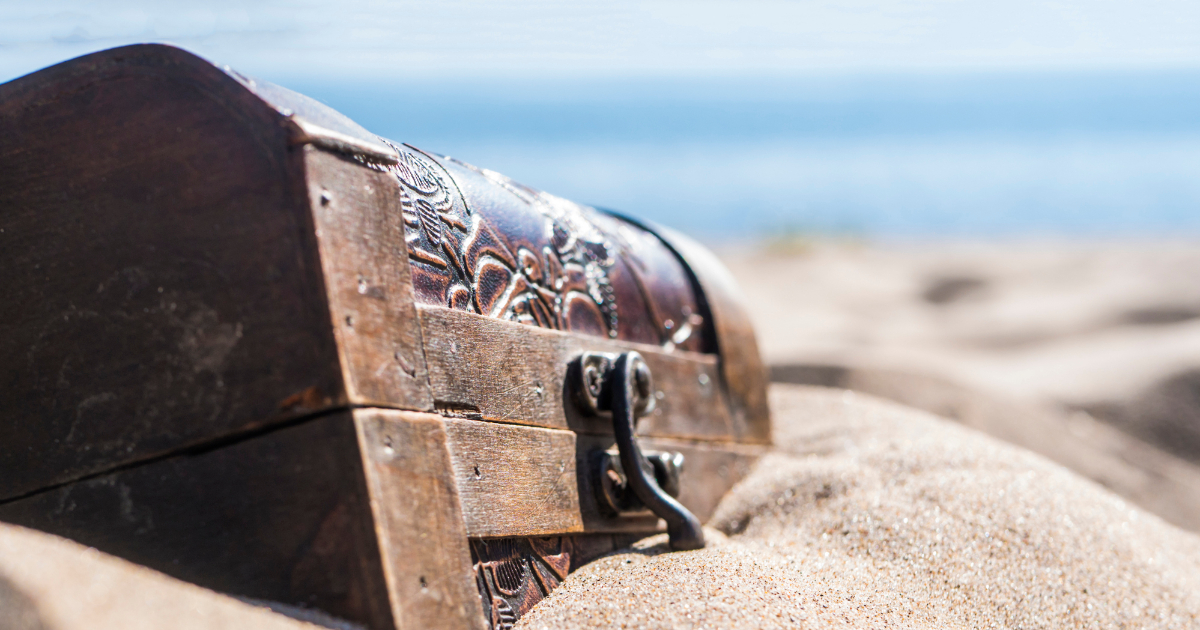
Key Takeaways
- There is a surge in interest in zero-knowledge proofs, particularly in the context of blockchain-based decentralized systems.
- Challenges exist in explaining zero-knowledge proofs, therefore most articles target either mathematically-inclined readers or offer examples limited to specific scenarios.
- Zero-knowledge proofs can be used to demonstrate knowledge of various secret solutions, such as hash preimages, private keys for public keys, or specific transactions for maintaining blockchain integrity.
- Zero-knowledge proofs can establish the reliability of a method without disclosing the method itself.
- Check out a simple algorithm to implement zero-knowledge proof using logic gates to any problem that requires hiding secrets.
Zero-knowledge proofs have come up with a lot of buzz in recent times, thanks to the advent of blockchain-based decentralized systems. For example, cryptocurrencies like ZCash and Monero provide private transactions on a public blockchain based on zero-knowledge proofs.
But what exactly is this magical cryptography that can provide the answer to any kind of privacy requirements on a blockchain? As expected, there is no shortage of articles trying to explain what a zero-knowledge proof is and how it works.
However, they are either targeted to a mathematically equipped audience or they are simple examples of specialized zero-knowledge proof systems. By specialized, I mean they are meant to accommodate only specific kinds of proofs. The question is: “what can you prove with a zero-knowledge proof?” The short answer is: “almost everything”. The long answer requires a little bit of explanation.
When we say ‘zero-knowledge proof’ in the context of cryptography, we mean the proof of the knowledge of a secret solution to a problem (or a puzzle). It can be the knowledge of the preimage of a hash function, or the knowledge of the private for a public key, or the knowledge of specific transactions that would maintain the integrity of a blockchain.
In this article, I will try to convince you that you can prove the knowledge of any such secret solution to any problem in zero-knowledge, i.e. without sharing anything about the secret. For example, if Alice knows the preimage of a specific SHA256 hash value, she can prove her knowledge to Bob without giving up even a single bit of the preimage.
A Simple Example
Let us consider a fictional story. Say Mary is an inventor of a mechanism to determine whether a very small amount of arsenic impurity is present in steel. She does not want to disclose it, but wants to get paid by the industry per test. Let Tom be a manager at a steel factory who has no reason to believe that Mary’s methods are correct. But, in case it is, Tom would like to buy her services. How would Mary convince Tom that her method is a hundred percent reliable without disclosing how she does it?
Mary proposes the following – Tom would prepare 128 samples of either pure steel or steel with the specified amount of arsenic impurity based on a coin toss. For each sample, Tom tosses a coin, if it is heads, he produces pure steel. If it is tails, he produces steel with arsenic impurity. He writes down which one is which and then hands the samples over to Mary. Mary can only see the sample identifiers without knowing which one has impurities. Now, her job is to tell which sample is of which kind, and Tom accepts her proof if she is correct in every case. Now, if Mary had no reliable method, and she was just guessing, then the probability of her being correct for every sample is only 2-128, which is very small. So, if she is correct in every case, Tom has every reason to believe that she does have a method to distinguish the pure samples from the impure samples.
This is an example of a zero-knowledge proof. Mary could convince Tom that her method works without disclosing how she is doing it. The question is, what kind of proofs can be provided in zero-knowledge. It turns out that almost anything that can be proven can be proven in zero-knowledge. Let us say that if you have the solution to a puzzle or you know the private key corresponding to a public key, all of these can be proven in zero-knowledge, i.e. without giving up the solution to the puzzle or your private key.
In this article, we will try to see that this is possible without having to go into the math required for the efficient zero-knowledge proof systems that are used in the industry.
Blind Dates
Let us imagine a different scenario. Mary and Tom went on a blind date and want to decide whether they want to go on a second date. However, it would be awkward if either of them wanted to go on a second date, but the other one did not. So, they have to ask each other in such a way that if either does want to go, the other cannot know that unless they want to go as well.
Essentially, we want to implement an AND gate, where each one wanting to go represents an input of 1 while not wanting to go is represented by a 0. If the output of the AND gate is 1, that means each input must also have been 1, so they both want to go. However, no one can know the other person’s input beyond what can be derived from their input and the result of the computation.
How can we achieve that? Let us assume that both of them are honest, i.e. they do what they are expected to do, but they are also inclined to remember anything they have seen. One solution then can be as follows. We assign each possible value of each input to the AND gate with a key. So, if Tom says 0, his input is represented by the key (K_T^0); on the contrary, if Tom says 1, his input is represented by the key (K_T^1). The superscripts here just represent the value the keys represent and are not exponents. Similarly, Mary’s inputs are (K_M^0) and (K_M^1) for 0 and 1 respectively. Let us now have four boxes, each of them can be opened by two keys used together, one from Tom’s keys and one from Mary’s keys. We can have the boxes as follows:
- Box (B_0) can be opened using (K_T^0) and (K_M^0) used together.
- Box (B_1) can be opened using (K_T^0) and (K_M^1) used together.
- Box (B_2) can be opened using (K_T^1) and (K_M^0) used together.
- Box (B_3) can be opened using (K_T^1) and (K_M^1) used together.
The boxes look identical from the outside, but they contain a piece of paper with a value printed on it. Each of (B_0),(B_1), and (B_2) contains a paper with a zero. (B_3) contains a paper with a 1.
Now, if Tom could somehow provide the key corresponding to his input and Mary hers, any of them could open the box that can be opened with those keys – by attempting to open each box with the pair of keys provided – and learn the outcome of their decisions. This does not tell the other person about their inputs because the keys are not marked with the values they represent, and the boxes look identical from the outside.
Either of them can be tasked with actually opening the box, as long as they are only allowed to try just one of their keys. If that person were allowed to try both of their keys, they could know which is the input of the other.
At the beginning of the protocol, one of them has to create the boxes and the keys. Let’s say Tom creates the boxes. Then he knows all the values and what each key means. Hence, if he is allowed to see which key Mary chooses, he would know what her decision was. Hence, if Tom creates the keys and the boxes, it must be Mary who opens the box. Now, there needs to be a way for Tom to give Mary the key corresponding to her choice so that she gets only that key, but Tom has no way of knowing which one she picked.
Note that Tom must not give Mary both keys because that would allow Mary to compute the function for both of her possible inputs, thus disclosing Tom’s input to her. So Tom puts each of Mary’s keys in an individual envelope and attaches a tag to each of them that tells Mary which is 0 and which is 1. Mary opens the one corresponding to her choice and removes the tag from the other envelope.
Then, they destroy the unopened envelopes in public and Mary opens the box that can be opened with the key provided by Tom and her own key. The piece of paper inside the box represents her answer, which she shows Tom. If Tom has signed each of the papers inside the boxes, there is no way for Mary to cheat by replacing a paper by her own. Tom can cheat by manipulating how he creates the boxes.
For example, Tom can choose to create the boxes in a way that both the boxes have 1 written on each piece of paper. This way the output would be 1 irrespective of the inputs. However, we have assumed Tom to be curious but honest at this point.
Now that I have discussed how to construct a protocol for an AND gate, I leave it to the reader to make the changes to implement a XOR gate and an OR gate.
Three-party Voting
Let us now consider a different scenario. Tom, Dick, and Harry want to decide which destination to visit for their vacation. There are two choices – 0 and 1. They decide that they must choose the location based on a majority vote. How can we get a Boolean circuit for a majority vote? The following circuit works as desired:
((T∧H)∨ (H ∧ D) ∨ (D∧T))
Here, (T) is the input of Tom, (D) is the input of Dick, and (H) is the input of Harry, where (∧) represents an AND gate and (∨) represents an OR gate.
If the formula returns 1, at least one of the clauses in the parentheses has to evaluate to 1. Hence, at least two of the inputs have to be 1. So, our vote can be evaluated with a circuit with three AND gates and two OR gates.
Let’s say, now, that we have to make sure that people do not learn about who voted for the less popular choice. To this aim we can use the boxes and keys approach as seen before but, here, we need to use the output of the AND gates as inputs to the OR gates. This is very simple to do: instead of having the pieces of paper with 0 or 1 written in the box, we put the keys corresponding to the input of the next gate. There is a key corresponding to the output 0 and one for the output 1 for the gate. But since there are four boxes, the boxes that represent the same output for the gate must contain duplicates of the same key.
The system described here is called Yao’s garbled circuit. Notice that this technique can be used for any computation using any Boolean circuit. Since all kinds of computations can be expressed in terms of Boolean circuits, we can make garbled circuits for any computation. The boxes are replaced by encryption and the keys are replaced by encryption keys in a cryptographic system. We will use this approach to build our zero-knowledge system.
Zero-knowledge Proof
We have already seen how to make a garbled circuit out of any circuit. How can we use it to make a zero-knowledge proof?
Let (f) be a function that takes a set of Boolean variables as input and outputs either 0 or 1. We can find such a function for anything we want to prove. For example, if the prover claims that he knows the factorization of a number (n), our (f(n,x)) will output 1 if and only if the input (x) represents two numbers greater than one whose product equals the input (n). We can represent the input numbers in binary, make a Boolean circuit to find the product of the number, and then make a circuit to check whether the output matches the binary representation of (n).
But how will the prover convince a verifier that he does know the factorization without giving the verifier any clue about what the factorization is? The following is a technique we can try to use:
- The prover makes a garbling of the circuit of (f) and gives it to the verifier.
- The prover then provides the keys corresponding to its input (x) to the verifier. Note that the verifier cannot tell whether the keys represent 0 or 1.
- The prover also gives the keys corresponding to the verifier’s input n with the envelope trick. But since the verifier does not need to hide (n), he can open the envelopes in public.
- The verifier runs the garbled circuit and checks if the final output is 1.
The technique certainly hides the prover’s input from the verifier, but it does not guarantee that the prover did not cheat by creating a garbled circuit of a function different from (f). To verify that the garbled circuit was created correctly, the verifier wants the prover to open all the boxes for all the gates in the circuit and also all the envelopes for the input keys. On the other hand, if the prover does that, the verifier will immediately know the prover’s input since he will know which of the prover’s input corresponds to which key.
The solution is as follows:
- The prover creates two copies of the garbled circuit, each with its unique set of keys.
- The prover puts both in public.
- The verifier chooses a random one to be opened by the prover.
- The prover has to open all the boxes and all the envelopes of the circuit copy chosen by the verifier.
- The verifier then evaluates the other copy with the keys provided by the prover and checks if it returns 1. [Note that the verifier does not have any secrets, so he can do the verification publicly and the prover also can make sure that the evaluation is correctly done by the verifier.]
Since each of the copies has a probability of 0.5 of being chosen to be opened, if the prover creates at least one of the garbled circuits incorrectly, he has a probability of 0.5 of being caught and losing his reputation. But what if that is not enough of a guarantee? What if we want almost a certainty of a dishonest prover being caught? Here is what we can do:
- The prover creates 256 copies of the garbled circuit, each with its unique set of keys.
- The prover puts all of them in public.
- The verifier chooses a random 128 of them to be opened by the prover.
- The prover has to open all the boxes and all the envelopes of the copies chosen by the verifier.
- The verifier then evaluates all the remaining copies with the keys provided by the prover and checks if all of them return 1.
Now, if any of the copies executed by the verifier is a correct circuit, the prover must provide the keys corresponding to the correct witness. Hence, if the prover does not have the factorization, the prover must make at least 128 copies incorrectly to have any chance of convincing the verifier. But, in that case, the prover will be caught unless the verifier chooses the correct ones to open. The number of ways the prover can choose 128 copies out of 250 is (begin{pmatrix} 256 128 end{pmatrix}). Only one of them would convince the verifier without the prover getting caught. The probability of that is (1/ begin{pmatrix} 256 128 end{pmatrix} <10^{-75}). So, the dishonest prover will almost certainly be caught. So, we have a zero-knowledge proof system for proving any secret knowledge that satisfies a given condition.
More Than a Zero-knowledge Proof
The above technique provides a way to prove the knowledge of any secret satisfying any condition in zero-knowledge. However, the proof is very large in the amount of communication that is needed and also in the amount of computation that the verifier needs to do. Modern zero-knowledge proof systems let you create very short proofs that can be verified with a very small amount of computation.
These techniques require the knowledge of finite fields, which are finite sets that allow addition, subtraction, multiplication, and division among the set elements; so I will not cover them here but the interested reader can learn more here.
In this article, we have introduced a simple way to achieve a zero-knowledge proof for a general boolean circuit. It shows the reader that anything that can be proved without zero-knowledge can be proved in zero-knowledge, since any function can be expressed in terms of a boolean circuit and then we can create a zero-knowledge proof as shown in the article.
However, this method is quite expensive in terms of communication complexity. In practical systems, succinct zero-knowledge (SNARK) proofs are used instead.
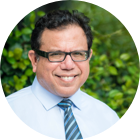
MMS • RSS
Posted on mongodb google news. Visit mongodb google news
MongoDB (NASDAQ:MDB) Full Year 2024 Results
Key Financial Results
-
Revenue: US$1.68b (up 31% from FY 2023).
-
Net loss: US$176.6m (loss narrowed by 49% from FY 2023).
-
US$2.48 loss per share (improved from US$5.03 loss in FY 2023).
All figures shown in the chart above are for the trailing 12 month (TTM) period
MongoDB Revenues and Earnings Beat Expectations
Revenue exceeded analyst estimates by 1.3%. Earnings per share (EPS) also surpassed analyst estimates by 12%.
In the last 12 months, the only revenue segment was Data Processing contributing US$1.68b. The largest operating expense was Sales & Marketing costs, amounting to US$782.8m (52% of total expenses). Over the last 12 months, the company’s earnings were enhanced by non-operating gains of US$57.1m. Explore how MDB’s revenue and expenses shape its earnings.
Looking ahead, revenue is forecast to grow 17% p.a. on average during the next 3 years, compared to a 9.1% growth forecast for the IT industry in the US.
Performance of the American IT industry.
The company’s shares are down 7.3% from a week ago.
Risk Analysis
Be aware that MongoDB is showing 2 warning signs in our investment analysis that you should know about…
Have feedback on this article? Concerned about the content? Get in touch with us directly. Alternatively, email editorial-team (at) simplywallst.com.
This article by Simply Wall St is general in nature. We provide commentary based on historical data and analyst forecasts only using an unbiased methodology and our articles are not intended to be financial advice. It does not constitute a recommendation to buy or sell any stock, and does not take account of your objectives, or your financial situation. We aim to bring you long-term focused analysis driven by fundamental data. Note that our analysis may not factor in the latest price-sensitive company announcements or qualitative material. Simply Wall St has no position in any stocks mentioned.
Article originally posted on mongodb google news. Visit mongodb google news
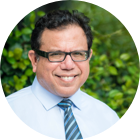
MMS • RSS
Posted on mongodb google news. Visit mongodb google news
In the latest trading session, MongoDB (MDB) closed at $213.46, marking a -0.06% move from the previous day. This change lagged the S&P 500’s daily gain of 0.53%. Meanwhile, the Dow gained 0.33%, and the Nasdaq, a tech-heavy index, lost 5.2%.
Coming into today, shares of the database platform had gained 9.45% in the past month. In that same time, the Computer and Technology sector gained 0.96%, while the S&P 500 gained 0.67%.
Wall Street will be looking for positivity from MongoDB as it approaches its next earnings report date. This is expected to be March 8, 2023. On that day, MongoDB is projected to report earnings of $0.07 per share, which would represent year-over-year growth of 177.78%. Meanwhile, our latest consensus estimate is calling for revenue of $335.84 million, up 26.02% from the prior-year quarter.
It is also important to note the recent changes to analyst estimates for MongoDB. These revisions typically reflect the latest short-term business trends, which can change frequently. With this in mind, we can consider positive estimate revisions a sign of optimism about the company’s business outlook.
Based on our research, we believe these estimate revisions are directly related to near-team stock moves. We developed the Zacks Rank to capitalize on this phenomenon. Our system takes these estimate changes into account and delivers a clear, actionable rating model.
Ranging from #1 (Strong Buy) to #5 (Strong Sell), the Zacks Rank system has a proven, outside-audited track record of outperformance, with #1 stocks returning an average of +25% annually since 1988. The Zacks Consensus EPS estimate remained stagnant within the past month. MongoDB is holding a Zacks Rank of #2 (Buy) right now.
Investors should also note MongoDB’s current valuation metrics, including its Forward P/E ratio of 337.03. For comparison, its industry has an average Forward P/E of 41.79, which means MongoDB is trading at a premium to the group.
The Internet – Software industry is part of the Computer and Technology sector. This group has a Zacks Industry Rank of 69, putting it in the top 28% of all 250+ industries.
The Zacks Industry Rank includes is listed in order from best to worst in terms of the average Zacks Rank of the individual companies within each of these sectors. Our research shows that the top 50% rated industries outperform the bottom half by a factor of 2 to 1.
Make sure to utilize Zacks.com to follow all of these stock-moving metrics, and more, in the coming trading sessions.
Want the latest recommendations from Zacks Investment Research? Today, you can download 7 Best Stocks for the Next 30 Days. Click to get this free report
MongoDB, Inc. (MDB) : Free Stock Analysis Report
To read this article on Zacks.com click here.
Article originally posted on mongodb google news. Visit mongodb google news
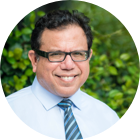
MMS • RSS
Posted on mongodb google news. Visit mongodb google news
Vanguard Group Inc. increased its position in shares of MongoDB, Inc. (NASDAQ:MDB – Free Report) by 0.3% during the 3rd quarter, Holdings Channel.com reports. The fund owned 6,648,265 shares of the company’s stock after acquiring an additional 16,590 shares during the period. Vanguard Group Inc. owned 0.09% of MongoDB worth $2,299,369,000 at the end of the most recent quarter.
Several other hedge funds have also recently bought and sold shares of the company. Jennison Associates LLC increased its holdings in MongoDB by 87.8% during the 3rd quarter. Jennison Associates LLC now owns 3,733,964 shares of the company’s stock worth $1,291,429,000 after purchasing an additional 1,745,231 shares during the period. State Street Corp increased its holdings in MongoDB by 1.8% during the 1st quarter. State Street Corp now owns 1,386,773 shares of the company’s stock worth $323,280,000 after purchasing an additional 24,595 shares during the period. 1832 Asset Management L.P. increased its holdings in MongoDB by 3,283,771.0% during the 4th quarter. 1832 Asset Management L.P. now owns 1,018,000 shares of the company’s stock worth $200,383,000 after purchasing an additional 1,017,969 shares during the period. Geode Capital Management LLC increased its holdings in MongoDB by 3.5% during the 2nd quarter. Geode Capital Management LLC now owns 1,000,665 shares of the company’s stock worth $410,567,000 after purchasing an additional 33,376 shares during the period. Finally, Norges Bank purchased a new stake in MongoDB during the 4th quarter worth about $147,735,000. Institutional investors and hedge funds own 88.89% of the company’s stock.
Insider Activity
In other news, CAO Thomas Bull sold 359 shares of the firm’s stock in a transaction that occurred on Tuesday, January 2nd. The stock was sold at an average price of $404.38, for a total value of $145,172.42. Following the completion of the sale, the chief accounting officer now directly owns 16,313 shares of the company’s stock, valued at approximately $6,596,650.94. The transaction was disclosed in a legal filing with the Securities & Exchange Commission, which can be accessed through this link. In related news, CRO Cedric Pech sold 1,248 shares of the firm’s stock in a transaction that occurred on Tuesday, January 16th. The stock was sold at an average price of $400.00, for a total value of $499,200.00. Following the completion of the transaction, the executive now directly owns 25,425 shares of the company’s stock, valued at approximately $10,170,000. The transaction was disclosed in a document filed with the Securities & Exchange Commission, which is accessible through the SEC website. Also, CAO Thomas Bull sold 359 shares of the firm’s stock in a transaction that occurred on Tuesday, January 2nd. The shares were sold at an average price of $404.38, for a total value of $145,172.42. Following the completion of the transaction, the chief accounting officer now directly owns 16,313 shares of the company’s stock, valued at $6,596,650.94. The disclosure for this sale can be found here. Insiders sold 54,607 shares of company stock valued at $23,116,062 in the last three months. 4.80% of the stock is currently owned by company insiders.
Analyst Ratings Changes
Several equities research analysts have weighed in on the company. Royal Bank of Canada upped their price target on MongoDB from $445.00 to $475.00 and gave the stock an “outperform” rating in a report on Wednesday, December 6th. Piper Sandler increased their price objective on MongoDB from $425.00 to $500.00 and gave the company an “overweight” rating in a report on Wednesday, December 6th. DA Davidson upgraded MongoDB from a “neutral” rating to a “buy” rating and increased their price objective for the company from $405.00 to $430.00 in a report on Friday, March 8th. Stifel Nicolaus reiterated a “buy” rating and issued a $450.00 price objective on shares of MongoDB in a report on Monday, December 4th. Finally, Guggenheim increased their price objective on MongoDB from $250.00 to $272.00 and gave the company a “sell” rating in a report on Monday, March 4th. One research analyst has rated the stock with a sell rating, three have given a hold rating and nineteen have given a buy rating to the company’s stock. According to MarketBeat.com, the company presently has a consensus rating of “Moderate Buy” and a consensus target price of $456.19.
Read Our Latest Stock Analysis on MDB
MongoDB Stock Down 3.7 %
MDB opened at $355.44 on Friday. The company has a debt-to-equity ratio of 1.18, a current ratio of 4.74 and a quick ratio of 4.74. The business has a 50 day simple moving average of $421.71 and a 200-day simple moving average of $391.90. The stock has a market capitalization of $25.65 billion, a P/E ratio of -143.32 and a beta of 1.24. MongoDB, Inc. has a 12 month low of $198.72 and a 12 month high of $509.62.
MongoDB Company Profile
MongoDB, Inc provides general purpose database platform worldwide. The company offers MongoDB Atlas, a hosted multi-cloud database-as-a-service solution; MongoDB Enterprise Advanced, a commercial database server for enterprise customers to run in the cloud, on-premise, or in a hybrid environment; and Community Server, a free-to-download version of its database, which includes the functionality that developers need to get started with MongoDB.
Featured Stories
Want to see what other hedge funds are holding MDB? Visit HoldingsChannel.com to get the latest 13F filings and insider trades for MongoDB, Inc. (NASDAQ:MDB – Free Report).
Receive News & Ratings for MongoDB Daily – Enter your email address below to receive a concise daily summary of the latest news and analysts’ ratings for MongoDB and related companies with MarketBeat.com’s FREE daily email newsletter.
Article originally posted on mongodb google news. Visit mongodb google news