Month: March 2024
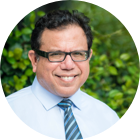
MMS • RSS
Posted on mongodb google news. Visit mongodb google news
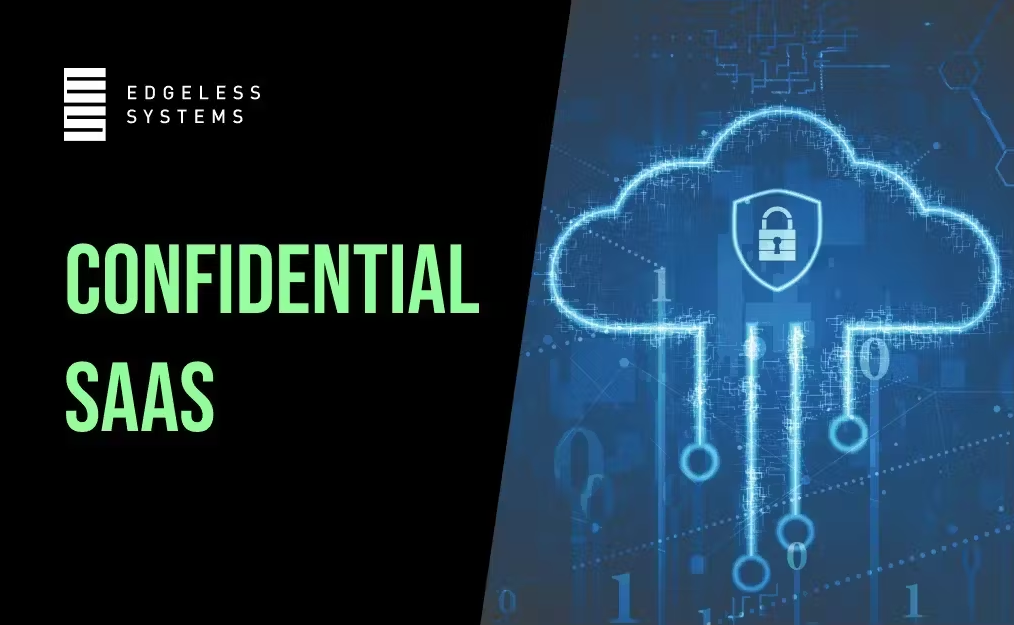
Confidential computing startup Edgeless Systems GmbH today announced a new solution designed to enhance the security of artificial intelligence applications by offering confidential AI capabilities that enable the sharing of sensitive data within AI services.
Called Continuum, the new service has been designed to protect user data within AI services by encrypting data. Doing so means that AI service providers and the underlying cloud infrastructure only ever see encrypted data that cannot be stolen or intercepted.
Edgeless Systems argues that since the release of OpenAI’s ChatGPT, generative AI has been transformative across industries and has found its way into many productivity-boosting products. However, the company claims that users such as enterprises, public sector entities and consumers have been reluctant to use AI services with sensitive data on concerns about data leaks and the potential for AI models to be further trained on their input data.
That’s where Continuum steps in. It offers cloud-based “confidential AI” services that address sensitive data concerns. The service ensures that user requests, or prompts, and the corresponding replies remain encrypted throughout within a given AI service, making plain-text data inaccessible to both the AI service provider and the underlying cloud infrastructure.
The service is also designed to protect the highly valuable weights of AI models in the same manner. To do so, Continuum leverages the confidential computing capabilities of Nvidia Corp.’s H100 GPUs along with cryptography and sandboxing technology to allow AI service providers to update models and code freely without compromising security.
Continuum is not solely focused on the end user, as it says AI service providers benefit from its straightforward integration into their existing software stacks, with protection for their AI models and intellectual property. Users of AI services gain from Continuum’s assurance of compliance and privacy, addressing concerns over data security and unauthorized use in future AI training.
“Continuum will significantly influence the generative AI market, allowing a wider range of enterprises, particularly those in regulated sectors, to confidently utilize offerings like chatbots,” said Chief Executive Felix Schuster. “This product embodies our mission to enable the use of cloud services without compromising on data security or compliance.”
Edgeless Systems is a venture capital-backed startup, having raised $6.72 million, according to Tracxn, including a round of $5 million in March 2023. Investors include SquareOne Holdings Inc., Inventures Private Ltd., Acequia Capital Management LLC, Venture Creator GmbH and SIX Group AG.
Image: Edgeless Systems
Your vote of support is important to us and it helps us keep the content FREE.
One click below supports our mission to provide free, deep, and relevant content.
Join our community on YouTube
Join the community that includes more than 15,000 #CubeAlumni experts, including Amazon.com CEO Andy Jassy, Dell Technologies founder and CEO Michael Dell, Intel CEO Pat Gelsinger, and many more luminaries and experts.
THANK YOU
Article originally posted on mongodb google news. Visit mongodb google news
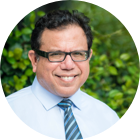
MMS • RSS
Posted on nosqlgooglealerts. Visit nosqlgooglealerts
MongoDB MDB released its fourth-quarter earnings report on Mar. 7. Here’s Morningstar’s take on MongoDB’s earnings and outlook for its stock.
Key Morningstar Metrics for MongoDB
What We Thought of MongoDB’s Q4 Earnings
- Since 2007, MongoDB has amassed millions of users of its document-based database, as workload shifts to the cloud have accelerated data collection and thus the need for architectures to store data. MongoDB has remained the most desirable database for professional developers to learn for years, according to Stack Overflow. We think such interest will persist as the firm’s more recent cloud database-as-a-service and data lake offerings help ensure its rich features transform to meet new technological needs.
- MongoDB stock has a high valuation and would need strong growth to be worth it. A big part of its thesis is a big growth runway as more companies switch from legacy database infrastructure providers to modern ones like MongoDB. The latest results call this into question somewhat. Our take is that the company will need to reaccelerate growth in 2025, or else the growth thesis will unravel. There’s no magic number, but we think that after falling to only 14% growth guidance for 2025, MongoDB needs to return to a topline growth rate of over 20% in 2026. Unless investors have good confidence that the firm can do this, they should be wary. Relatedly, as we have highlighted, investors have to believe the slowdown in current growth is due to one-time factors and not a sign of worsening competitive positioning.
- With significant opportunity remaining to convert customers to MongoDB’s cloud database-as-a-service product, Atlas, which represents over 60% of all revenue, along with brand-new opportunities for the company’s new Data Lake offering, we think it is set to grow at a robust pace and profit from such scale. We also believe MongoDB has a sticky customer base and could eventually merit a moat.
Fair Value Estimate for MongoDB
With its 3-star rating, we believe MongoDB’s stock is fairly valued compared with our long-term fair value estimate of $370 per share. Our valuation implies a forward fiscal-year enterprise value/sales of 16 times. Our assumptions are based on our expectations that MongoDB will achieve a compound annual growth rate of 21% over the next five years.
MongoDB is in its infancy but has a massive market opportunity and a large runway for growth, in our view. We expect this substantial growth to be driven by continued workload shifts to cloud environments, prompting the database market to grow robustly as companies realize how much easier it is to scale data storage in the cloud. In turn, this implies substantial usage growth per customer for MongoDB. Additionally, we think Atlas and Data Lake will bring in significant new revenue streams, as Atlas’ revenue eclipsed on-premises sales in early fiscal 2022.
We forecast that MongoDB’s gross margins will stay relatively the same, as its increasing mix of Atlas revenue is somewhat margin-dilutive in the near term. We expect GAAP operating margins to increase from negative 14% in fiscal 2024 to positive 32.5% in fiscal 2034 because of operating leverage as revenue growth exceeds operating expenses.
Read more about MongoDB’s fair value estimate.
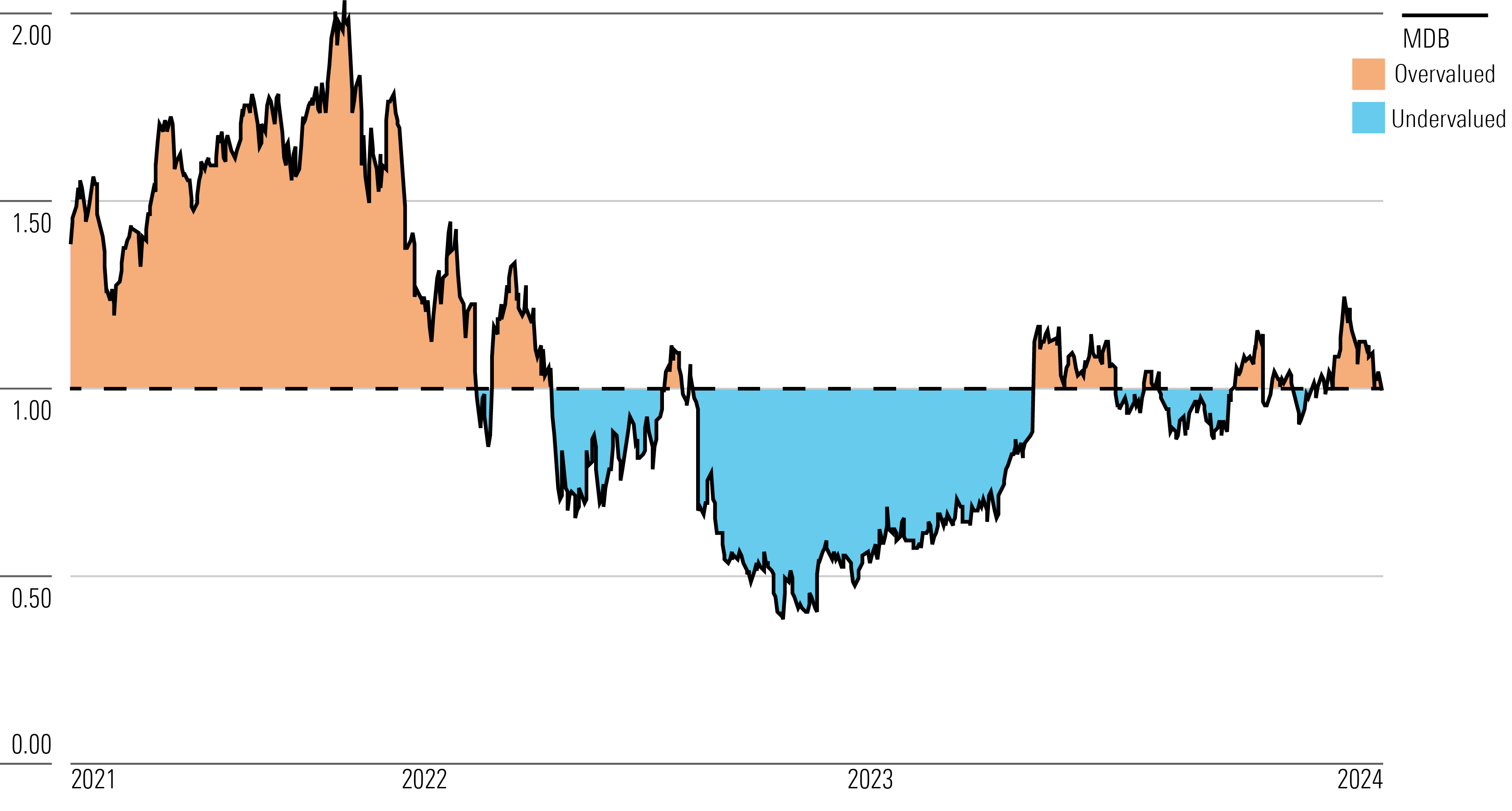
Economic Moat Rating
We do not assign MongoDB a moat. We think the company benefits from significant switching costs with the customers it has captured thus far. However, it is still in its customer acquisition phase, and it’s unclear whether this will lead to excess returns on invested capital over the next 10 years, as MongoDB lacks profitability and continues to aggressively spend on sales and marketing.
We believe MongoDB benefits from switching costs with its existing customer base, as we think data is increasingly mission-critical to enterprises, which makes choosing a database vendor an important task. With increasingly complex enterprise IT infrastructures, businesses need to think twice before switching databases, which are interconnected to many systems, thus requiring much time and money for reintegration.
MongoDB has not yet reached enough size and scale to generate excess returns on capital, even when considering a good portion of its sales and marketing costs as an asset with future benefits rather than a wasteful expense. We think it will take a few more years for MongoDB to reach such excess returns, as the company will likely continue to generate operating losses as it continues to invest in customer acquisition.
Read more about MongoDB’s moat rating.
Risk and Uncertainty
We assign MongoDB a High Uncertainty Rating due to its place in a technological landscape that could shift rapidly. However, we do not foresee any material environmental, social, or governance issues.
The firm faces uncertainty based on future competition. It runs the risk of Amazon AMZN encroaching on its abilities. Amazon’s DocumentDB service claims it can help companies deploy MongoDB at scale in the cloud, similar to Atlas. At the moment, DocumentDB has significant limitations compared with Atlas—with its inability to support rich data types, interoperability only with AWS, and only 63% compatibility with MongoDB. While many open-source software companies have suffered from Amazon reselling their software for a profit (for example, Elastic was repackaged into Amazon Elasticsearch), MongoDB is protected from this risk, as its software license bans Amazon from doing this.
On the ESG front, MongoDB is at risk of its stored data being compromised by breaches. If any of its security features were to fail, the company’s brand could suffer significantly, possibly leading to diminished future business.
Read more about MongoDB’s risk and uncertainty.
MDB Bulls Say
- MongoDB’s database is best-equipped to remove fears of vendor lock-in, and it’s poised for a strong future.
- Data Lake could gain significant traction, making MongoDB even stickier, as we believe data lakes have greater switching costs than databases. In turn, this could further boost returns on invested capital.
- MongoDB could eventually launch a data warehouse offering, which would further increase customer switching costs.
MDB Bears Say
- Document-based databases could decrease in popularity if a new NoSQL variation arises that better meets developer needs.
- Cloud service providers like AWS could catch up to MongoDB in terms of its rich features.
- If MongoDB can’t reaccelerate growth in fiscal 2026 after a slower 2025, its current valuation would seem too rich, and there could be a significant downside.
This article was compiled by Freeman Brou.
–>
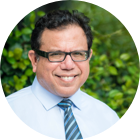
MMS • RSS
Posted on mongodb google news. Visit mongodb google news
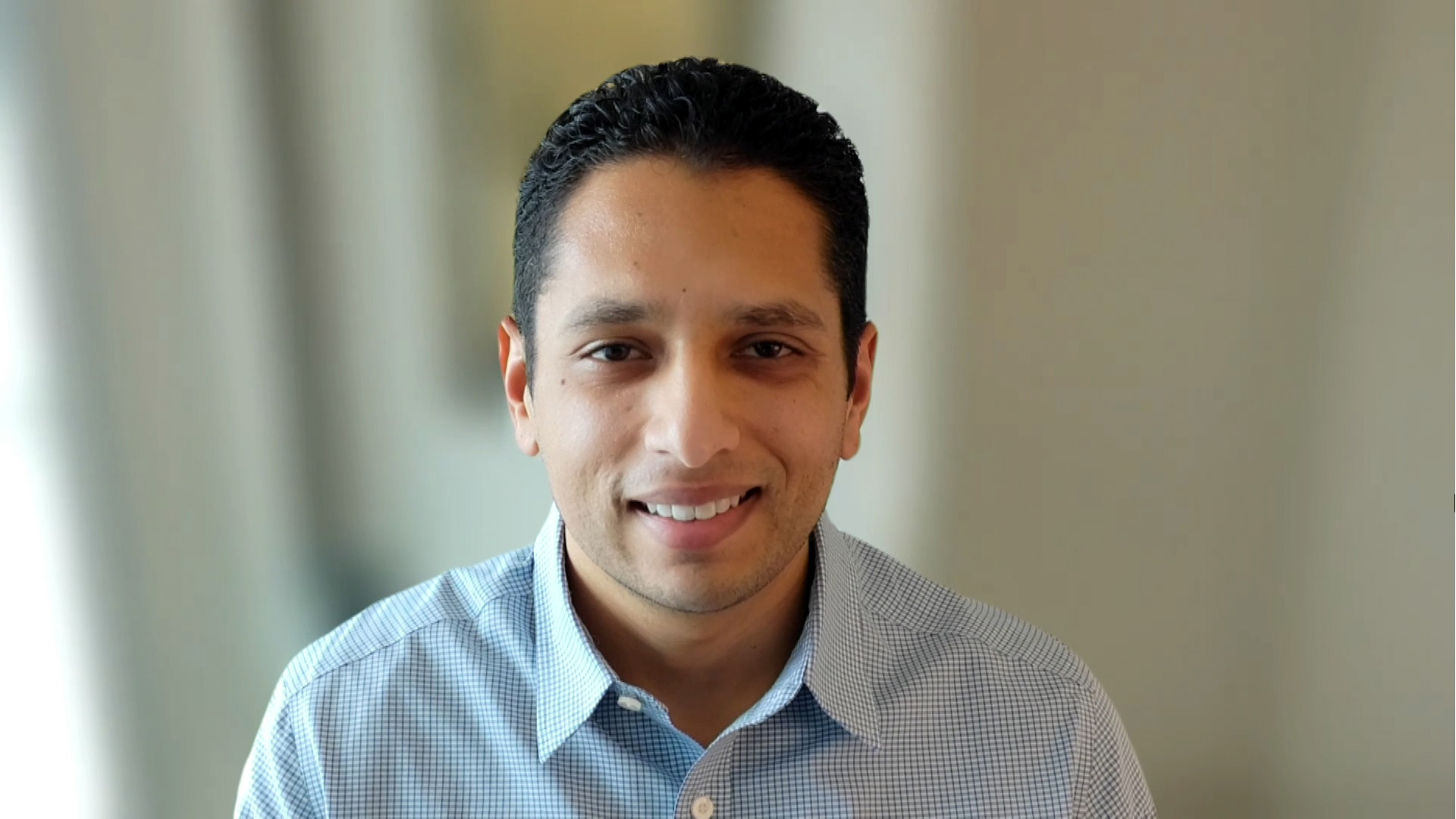
The enthusiasm around generative artificial intelligence is escalating daily, with increasing confidence in the improvement of language models across the industry. Within this dynamic landscape, MongoDB Inc. has established itself as an essential hub for developers, frequently organizing hackathons.
About two years ago, the company launched MongoDB Ventures. The thought at the time was to look across the customer base and think about a good way to introduce new technologies to it, according to Suraj Patel (pictured), head of MongoDB Ventures.
“Generally, we’re looking at a lot of infrastructure software, a lot of developer tools,” he said. “The last year and a half has been very exciting … focused a lot on looking at companies building in the AI space and, in particular, companies that are enabling developers, which is our key audience, to build with AI.”
Patel spoke with theCUBE Research analysts John Furrier, executive analyst, and Dave Vellante, co-founder and chief analyst, at the “Supercloud 6: AI Innovation” event, during an exclusive broadcast on SiliconANGLE Media’s livestreaming studio theCUBE. They discussed the evolving landscape of developer-focused ventures in the AI space and the integration of AI into enterprise applications.
New AI solutions rooted in developer innovation
Something that has emerged as a subject of conversation during Supercloud 6 coverage on theCUBE involves a new formula that enterprises are driving toward. It’s end-to-end new kinds of systems to power AI but needing to be developer-led. It begs the question: How does one become an enterprise motion end-to-end system that is developer-led? Such trends are exactly why MongoDB is positioned well as a venture organization, according to Patel.
“What we’re seeing is that, really, the products that a developer needs to … build AI into their applications, even if they’re at the enterprise, those products are often startups,” he said.
A lot of developers within the MongoDB ecosystem are using products such as LangChain and LlamaIndex, among others, according to Patel. These are integral products for those companies that need to build AI-infused applications.
“Now, the data in an enterprise, though, lives in proven database technologies like MongoDB, and that’s where the magic of the partnership with some of these younger companies really comes into play,” he said. “We work hand in hand with them; we figure out what are the points of connectivity that we can enable and how do we make it easier for developers to use these new innovative companies with their existing enterprise data and really bring the right context to these AI applications.”
Anyone spending a lot of time in the AI space and talking to enterprise customers in it right now often hears the same narrative, according to Patel. Where it felt like last year was a lot of prototypes with people trying applications for the first time, things are now maturing.
“They’re starting to think about, OK, well, if I’m using this model as part of my application, what is the long-term cost going to be of this model? And how am I going to deal with that from a budget perspective?” he said. “If I’m using this framework, am I confident that this framework is now going to scale? Or if I’m introducing AI powers into my application, which are by nature probabilistic, how can I design unit tests or different testing to make sure that the actual output of the application is as predictable as possible, as well as safe?”
Here’s the complete video interview, part of SiliconANGLE’s and theCUBE Research’s coverage of the “Supercloud 6: AI Innovation” event:
Photo: SiliconANGLE
Your vote of support is important to us and it helps us keep the content FREE.
One click below supports our mission to provide free, deep, and relevant content.
Join our community on YouTube
Join the community that includes more than 15,000 #CubeAlumni experts, including Amazon.com CEO Andy Jassy, Dell Technologies founder and CEO Michael Dell, Intel CEO Pat Gelsinger, and many more luminaries and experts.
THANK YOU
Article originally posted on mongodb google news. Visit mongodb google news
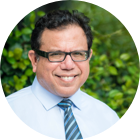
MMS • RSS
Posted on mongodb google news. Visit mongodb google news
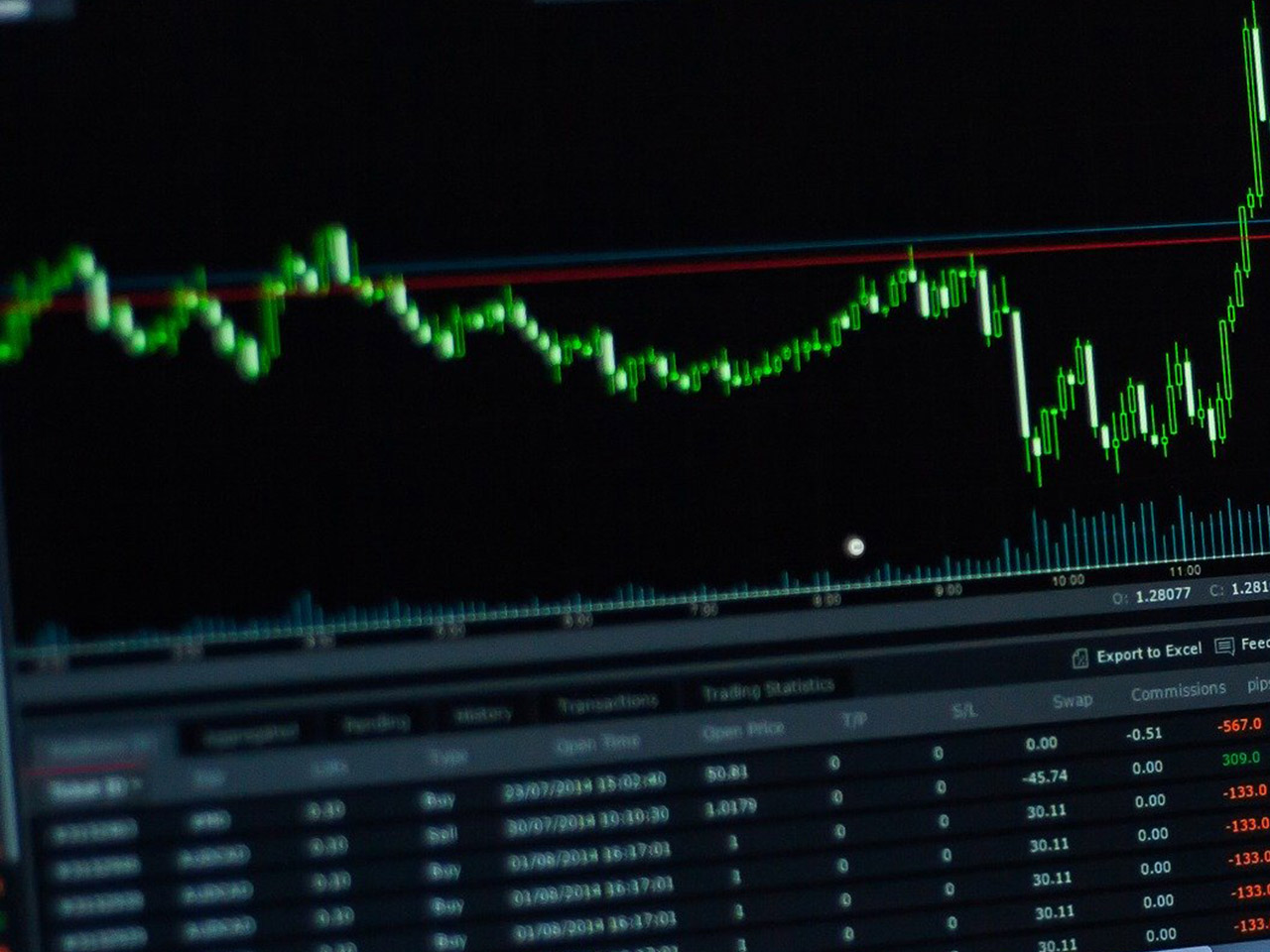
MongoDB, Inc. which can be found using ticker (MDB) have now 29 market analysts covering the stock. The analyst consensus now points to a rating of ‘buy’. The target price High/Low ranges between $700.00 and $272.00 calculating the mean target share price we have $455.58. (at the time of writing). Given that the stocks previous close was at $366.99 this indicates there is a potential upside of 24.1%. The day 50 moving average is $420.50 and the 200 moving average now moves to $387.84. The market cap for the company is 26.82B. The current share price for the company is: $368.64 USD
The potential market cap would be $33,288,477,733 based on the market consensus.
The company is not paying dividends at this time.
Other points of data to note are a P/E ratio of -, revenue per share of $23.62 and a -5.35% return on assets.
MongoDB, Inc. is a developer data platform company. Its developer data platform is an integrated set of databases and related services that allow development teams to address the growing variety of modern application requirements. Its core offerings are MongoDB Atlas and MongoDB Enterprise Advanced. MongoDB Atlas is its managed multi-cloud database-as-a-service offering that includes an integrated set of database and related services. MongoDB Atlas provides customers with a managed offering that includes automated provisioning and healing, comprehensive system monitoring, managed backup and restore, default security and other features. MongoDB Enterprise Advanced is its self-managed commercial offering for enterprise customers that can run in the cloud, on-premises or in a hybrid environment. It provides professional services to its customers, including consulting and training. It has over 40,800 customers spanning a range of industries in more than 100 countries around the world.
Article originally posted on mongodb google news. Visit mongodb google news
Presentation: Staff+ Engineering Beyond Big Techs: How to Succeed in the Technical Path on Non Tech Companies
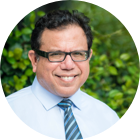
MMS • Loiane Groner
Article originally posted on InfoQ. Visit InfoQ
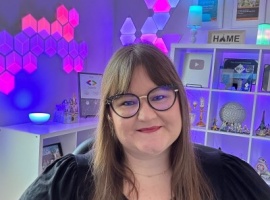
Transcript
Groner: I would like to share with you a little bit about a career as a staff engineer in non-technology companies. My name is Loiane Groner. I’m very passionate about working in technology. I’ve been on this road for over 15 years.
Whenever we talk about being a staff engineer, and we search online for job postings, we see a lot of articles and information about being staff engineers in big tech companies, especially the famous FAANG companies. However, this survey that was conducted by Oracle and Burning Glass Technologies from 2019 shows that approximately 90% of the jobs in the tech industry are in non-big tech companies, meaning they are not from the technology industry sector. If we take a look at this other chart, also published by Oracle and the Burning Glass Technologies from 2019, we see all these different industry sectors with a percentage of the job posts that we see they are tech related. This is just to emphasize that although we’re working in technology, the company that we work for might not necessarily be a technology company, meaning the vast majority of the IT jobs are outside of the tech industry. However, we almost don’t see a lot of material out there, talking about what comes after you’re a senior in these companies that are not related to technology. However, when it comes to career in non-tech companies, I’m sure that you have seen some diagram or career path that looks like the one that we have on the screen right now. This traditional career ladder, it’s what we call the manager’s path. After you become a senior engineer, you’re probably going to work as a tech lead for some time. If you want to take the next step into your career, the next step will be a manager level. If someone would like to continue working as a technical person beyond the senior level, especially the tech related companies, they created another path, which is what we call the staff engineering path. These individuals can continue climbing up the ladder, and progressing within their careers.
What Exactly is a Staff Engineer?
What exactly is a staff engineer that we talked about so much? We have the manager’s path, that we have agreed that this is pretty traditional. After the senior/tech lead level, instead of becoming a manager and having people reporting directly to you, there is what we call the staff-plus engineering path. In this path, you have the staff engineer, you have the senior staff engineer, principal engineer. Then if you want to continue, there’s also distinguished engineer, fellow engineer, as we have seen in some of the FAANG companies. This staff-plus track was designed to be able to retain talent, to be able to retain those people that want to continue working on a technical level. It doesn’t mean that because now you are in a technical track, and because you’re also not going to have any person reporting directly to you as you being their manager, it doesn’t mean that you won’t have to deal with people. This is still a leadership position, and you’re still going to help other people to grow within their careers as well. You’re just not going to be their manager. What exactly does it mean to be a staff engineer?
Three Pillars of Staff Engineer Roles
If you are on this path, or if you like to continue your career growing as a staff engineer in this staff-plus path, I highly recommend that you read these two books. They are amazing. The first one is the, “Staff Engineer.” There’s also a website that you can go into and read several articles, interviews, and guides. There’s also this other book published by Tanya Reilly, that is an individual guide, and has a lot of great insights as well. Tanya mentions in the book that there are three pillars that are related to being a staff engineer. The first one is big-picture thinking. The second one is project execution. The third one is leveling up. All these three pillars are based on the knowledge that you have acquired throughout your career, and also the experience. They have a big impact within the organization as well.
1. Big-Picture Thinking
Let’s dive into each one of them, and see also how these three pillars can be applied to non-technology companies. Let’s start with the big-picture thinking. This pillar means that you’re able to take a step back and see the whole picture, and to be able to make decisions that are going to be better for the organization. This means that you have to think beyond your current time. Whether that means if it’s a project that’s going to take years in order to be able to complete. If a system that you’re working on can be easily decommissioned. What exactly does your company need in the medium term, say, two, three years? Let’s see an example. This example is also from Tanya’s book, and I think it’s fantastic. When you are a senior engineer, or when you are a tech lead, and you work within a team, you have a problem to solve. You have different solutions, different approaches, or different tools that you might need to use, or implement to make your project successful. When you have all these options, usually when you’re a senior or a tech lead, you’re going to review all these options, and you’re going to make the decision based on what’s best for your team. This can be, for example, solution or approach A. This means that this is going to be easier for the team to implement, or it’s going to be quicker for the team to implement, meaning this has a higher value to the team. However, when we take a step back, and we review all the options and take the entire organization into consideration, this might not be the best solution. If we take a look, again, at both approaches, or both solutions that we have to consider, solution A and solution B, solution A although it might be better for the team might not be what’s best for the organization. For the organization, for example, in the long run, solution B might be better. For the organization, that’s going to have a higher value. It might take even more time for the team to implement that solution. Meaning it’s not what’s best for the team, but it’s what’s best for the organization. This is one of the reasons why it’s important to have staff engineers within your organization, so you can have people that are going to take a step back and analyze all the options available, and they’re going to make the best decision that’s possible.
Why is this important and how this correlates with the world that we’re living in today? If we take a look at this article that was published by Tech Brew back in November 2022, it says that many technology companies are cutting costs, and are aiming to do more with less. The Wall Street Journal also published an interview with Microsoft’s CEO. The CEO also mentioned that both their clients, Microsoft’s clients, and also Microsoft itself will have to do more with less. What does it mean to do more with less? Being a staff engineer in a non-technology company means that besides the technical expertise that you’re bringing to the table, you also learn about the business, the problems that the clients are facing. It’s also part of your job to help your clients to scale their business, especially in times of economic uncertainty. The staff engineers can play a key role within every organization. For example, my personal goal as an engineer is to make sure that the applications that I’m working on today will still be up and running in 10 years, and also, the decisions that I’m making will also help to scale the business. How the business can do more with less, and how can I help them using technology as a tool? That is the perspective that we need to have in order to make that happen.
When we say doing more with less, automation definitely plays a part in it. When we automate certain tasks, especially if those tasks are manual, this means that we are freeing up personnel so these personnel can work in different projects, or even take on new clients. Again, this goes for both technology and also for the business. Automation is one of the ways that we can help the business to do more with less. As a staff engineer, one of your jobs is to help to make decisions of tools that are going to be adopted at an organization level. Again, going back to the vision of the single team, if you leave it up for each individual team to make decisions on the tools and processes they’re going to adopt, each team might adopt a different tool or technology. Taking a step back, as a staff engineer, can we decide on a global tool that’s going to be used by everyone and even save some cost with it? Being a staff engineer, you might have an input on your department, within your organization, or even a global level or even in the tech industry. One of the other roles that a staff engineer also plays is to be able to define processes and best practices that are going to be adopted throughout your organization. This is another reason why non-tech companies should have a staff engineer path as well. Why? Because title matters. Having a title, the seniority means that you have a seat at the table. This means a lot. It is important to have the ability of providing an input so it can be taken into consideration. It doesn’t mean that whatever you say is what’s going to be adopted. It’s important to have the opportunity to have a say in it. This is one of the ways that non-tech companies can make sure that your voice is heard. This is really important.
When you work for companies that are not tech related, besides the technical knowledge that you bring to the table, you also have the knowledge about the business. You develop relationship with the clients, the business, the product owners, and other stakeholders as well. As we all know, it takes time to develop relationships. It’s not like, “You want to be friends,” and that’s a done deal. It really takes time until you can develop that partnership. In case someone decides to leave, training a new person can take time. It’s not like you’re starting from scratch. After all, you’re a senior. However, whenever you’re starting at a new company, there are all the internal processes of the company that you don’t know, and you have to learn. There are also all the relationships that you will have to develop. Having this knowledge about the business, knowing how companies work, this is also one of the reasons that for certain sectors in the industry, when you see a job description, you’ll probably see at the end of the job description, something like previous experience in the industry is a plus. Meaning that although some training is going to be required, because you’ll have to get used to the processes and the stakeholders, at least you’re not starting from scratch.
One of the takeaways is if you’re working for a non-technology company, and your company doesn’t have a staff-plus track yet, you start a discussion so this can be considered in the near future. For companies that still don’t have this path, depending on the size of the company, this is not something that you’re going to be doing in a week or even in a month. You’ll have to train managers on how to properly help staff engineers as well. It also requires some reorganizations within the company. If you have been through some reorgs within the company that you work for, you know that sometimes it might take a while until things go back to the normal track again. Having this path, it’s very important because this can help your company to retain talent as well.
2. Project Execution
If we go into the second pillar that Tanya describes in the book, we know that at a staff level, projects are going to be more complex. They’re going to involve more people. They’re going to involve more political capital. They’re going to require more influence and sometimes even culture change as well. As a staff engineer, what exactly is your role when it comes to project execution? I believe we can agree that we do have a pretty solid job description for junior engineers, mid-level senior engineers, tech leads, managers, and so on. What exactly is in a job description for a staff-plus engineer? Sometimes I like to joke with my colleagues at work that I don’t know what my title is anymore. One day, I’m helping with architecture, the other day, I’m helping define the strategy for long term. The next day, I’m helping the team to resolve a big production issue. The next day, I have a completely different task. Some days, I have no idea what my job title will be based on the tasks that I’m performing that day. I like to say that, today I’m going to be whatever you need me to be. Meaning that as a staff engineer, we do wear a lot of different hats, we play different roles depending on what the company needs. At the end of the day, your job as a staff engineer is to make sure that the organization is successful. You can be a technologist, but your job is to help the organization to achieve its goals. This means that this can be your team, your technology team, or it can be the business, the clients. As senior professionals, we do a lot of things that are not in our job description all the time. It might not be ideal, but if that’s what it takes, consider doing it.
Your work as a staff engineer needs to be important for the company. It doesn’t mean that your work should be playing with the latest and most modern technologies out there, meaning only doing fun stuff. Sometimes what’s important, it’s going to be the work that nobody sees. These important tasks might involve gathering data that doesn’t exist, going through old documents, combing through code that was written 10 years ago that nobody has touched. This is what we call the projects that nobody wants. Within a company, there are a number of tasks that they just need to get done, and somebody has to do it. This is still meaningful work. Meaningful work comes in many forms, and sometimes includes working in projects that nobody wants to work. Sometimes it also includes working with old boring technologies. There is one side where you need to keep your skills sharp, because this is how you’re going to be helping your organization to make strategic decisions. On the other hand, there is also all these other projects that they need to get done, and might involve old technologies. However, I’ve learned from experience that there is a lot to learn, even with old technologies. Sometimes I get surprised with the things that I didn’t know that I didn’t know. At the end of the day, it’s all about balance. There are still those projects that need to get done, and on the other hand we still want to play with the fun stuff.
3. Leveling Up
The third and last pillar is leveling up. With the increase in seniority, this means that you also have more responsibility with your hard skills, but also with the people around you. These responsibilities can include teaching, mentoring, and also the accidental influence of being a role model. After all, you are in a senior leadership position, and people will look up to you. As a staff engineer, it means that you don’t have direct reports. However, you’re also responsible for leveling up other people that work with you, within your team or within your organization. I like to include the teaching and mentoring into the community category. This can be done within your company or outside of your job responsibilities. I’m personally very passionate about this topic. This is a great way of teaching, giving back, leveling yourself up, as well as others. In my humble opinion, teaching is the best way to learn. This can be achieved in different ways. You can mentor other people. You can also contribute, writing blog posts, or that documentation in the Confluence, or wiki page of the company that you work for. You can record videos, publish them internally, or as well on the internet. One of the things that you can do, for example, is write a summary of everything that you have learned throughout this conference, or even put together a presentation of all the things that you have enjoyed and learned. Writing blog posts, presenting at conferences, can be a great way of building influence within the industry. Personally, sharing what I have learned with the community has opened many doors.
Just to give an example of how this works, and how this can be beneficial for both you and the company that you work for. Back in 2010, I was working with Ext JS, which is a frontend framework. I was writing blog posts back then of things that I was learning so I can deliver my tasks. I decided to put together a presentation and present it internally for the company that I used to work for. I continue writing blog posts. Eventually, to my surprise, I got an invite to write a book. That was a dream come true for me. I continued writing, and I did write more books because I really liked the process. This opened so many doors for me within the community. I started to get invited to present at conferences. I had the huge honor of presenting at QCon in Sao Paulo as well in Brazil. One year later, the company that I worked for, was deciding on a framework that we could start creating screens using HTML5. Again, to my surprise, and also a dream come true, I was invited to spend two weeks in New York, just training my peers. For me, this is amazing. I have no words to describe how thankful I am. I personally thought that I was living a dream or it was a movie, because it was really awesome.
For over 10 years, I’m still sharing a little bit of what I’m learning throughout my career. Because of this passion and commitment to the community, I was honored by some of these big tech companies that I’m a big fan of with some awards. I’m very thankful for it. Because of that, that continued to open more doors for me and I was able to present, for example, about Angular at QCon again. Later, the company that I worked for, was migrating from Ext JS to a different frontend framework, and I was able to provide my input on why we should adopt Angular and why it was good for us to do that. This is just an example of how contributions that you do outside your working hours can be beneficial for you, and also for the company that you work for. It’s a win-win situation. You might ask yourself that, especially in times like this, should we be sharing everything that we know, or should I just keep to myself so I can keep my job? My personal take on this is to just keep sharing. Great things can happen when you share what you know. You’re leveling up yourself and you’re also leveling up the people around you. This can be an opportunity to get more important projects, or even get a different role. Sharing will help you to keep growing within your career. Eder has presented an amazing talk about this subject as well, on how community contributions can help within your career. I highly recommend that you watch it.
One of the takeaways when you go back to work, just put together a summary of things that you have learned throughout this conference, or things that you have enjoyed, and present it back to your peers. This can be a great way to help you build your influence. Speaking about influence. Influence is one of the soft skills that you need to succeed in this career, however, it’s not the only one that you need. Every increase in seniority demands more soft skills. You’re going to be dealing with more people. When we make the shift from a senior or tech lead into the manager’s path, we’re going to shift our learning and we’re going to focus on learning more soft skills, because we’re going to be dealing a lot more with people. When you go into the staff path, besides your hard skills because you need to keep that technical knowledge growing, you also need to develop the soft skills. After all, you’re still a senior and you’re still in a leadership position as well. Meaning that soft skills are equally important as hard skills. Besides influence, communication is a must. The message that you’re trying to convey needs to be clear and precise. One of the challenges that some of us face, I know that I face this challenge every day, is how to do that in a second language. Do you know how smart I am in Portuguese? There you go. Influence is another one that is very important as well, not only to be able to provide your input, but also in case you need to get a sponsorship for a project, or a process that you’re trying to implement within your organization. Even though you are an individual contributor, your job is to do what’s best for the organization. This means that you’ll have to work with different teams. Teamwork is also essential.
Time management and delegating are also two important skills. Everybody’s time is valuable. As a staff engineer, you won’t have time to do every single thing that you would like to. You’ll have to choose what tasks are more important. How do you spend your time? Priorities might change, and you might not code as much as you’d like to. That’s very important to be able to know how to delegate and also how to manage your time. Again, even if you’re not a direct manager, but you can also help your peers with mentoring and coaching as well. Besides problem solving, critical thinking, that are also pretty basic soft skills. There is another soft skill that I think that is imperative, and that is learning how to disagree. Even though you are a staff engineer, it doesn’t mean that you can just simply pull rank. Sometimes your input, or the approach that you have suggested, are the ones that are going to be implemented. It’s very important to learn how to disagree, and disagree respectfully. Of course, technology is full of up and downs. It’s very important as well, to develop your emotional intelligence, so you are able to handle pressure and difficult situations that might come your way. Keep calm and carry on.
I also would like to leave this link for you because Charity presented an amazing talk as well at QCon, where she describes the importance of the manager and the staff engineer path pendulum. Right now, you might be a staff engineer, maybe your next role, you can be a manager, and vice versa. There are many things that you can learn by making the switch. The manager path is important because you want to continue developing the soft skills and learning how to deal with people. At the same time, you don’t want to be too long away from a technical path, so you can continue developing your hard skills as well. Again, at the end of the day, it’s all about balance. There are a lot of benefits of doing exactly this with your career. Of course, you also have the option to step back and not want to be a staff engineer anymore. You want to go back and be a tech lead, or being a senior engineer. That’s completely fine as well. Just remember that it’s your career, and you have to do what’s best for you as well.
Conclusion
Although we are very unique human beings, it’s almost impossible not to compare yourself with other people. However, don’t do that. It’s very bad for you. Instead, if you want to make sure that you’re making progress towards your career, compare yourself with you from yesterday. This way, you’re going to be able to see if you are indeed in the right path, or if there is anything that you have to change. Last but not least, although we are technologists, we’re attending a tech conference, we’re learning about new languages, architectures, and tools. Although we work with technology, it’s all about people. It’s about your clients, your team, your peers. It’s about being respectful and cultivating good relationships.
See more presentations with transcripts
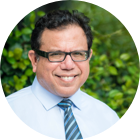
MMS • Renato Losio
Article originally posted on InfoQ. Visit InfoQ
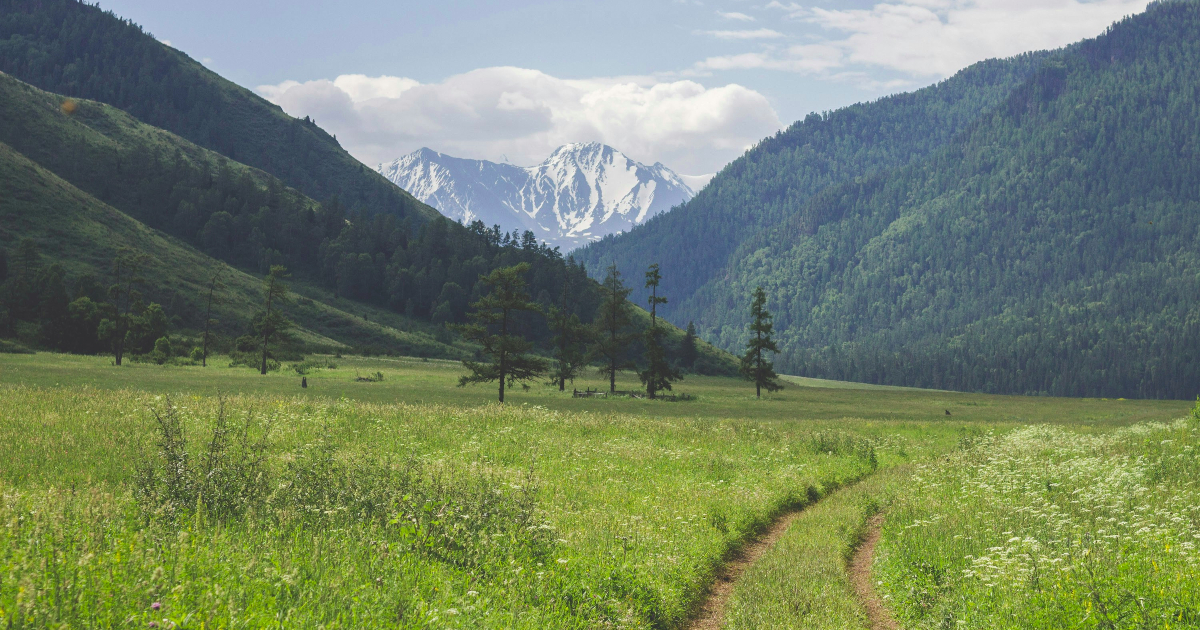
Recently, a former employee of F5 and main contributor of the Nginx project announced the fork Freenginx of the popular web server. The new project was started to address a security dispute and wants to be a drop-in replacement of Nginx, run by developers rather than corporate entities. Maxim Dounin, formerly principal software engineer at F5, provides insights into the fork:
Unfortunately, some new non-technical management at F5 recently decided that they know better how to run open-source projects. In particular, they decided to interfere with the security policy Nginx has used for years, ignoring both the policy and the developers’ position.
Originally developed by Igor Sysoev and currently maintained by F5, Nginx is open-source software for web serving, reverse proxying, caching, load balancing, and media streaming. According to the Web Server Survey, even two decades after its initial launch, Ngnix remains the leading web server serving 23.21% of all sites. In a popular thread on Hacker News, user sevg points out:
Worth noting that there are only two active “core” devs, Maxim Dounin (the OP) and Roman Arutyunyan. Maxim is the biggest contributor that is still active. Maxim and Roman account for basically 99% of the current development. So this is a pretty impactful fork.
In his announcement on the nginx-devel mailing list, Dounin highlights the initial dispute that prompted Nginx to issue a security patch addressing two critical vulnerabilities. He adds:
I am no longer able to control which changes are made in Nginx within F5, and I no longer see Nginx as a free and open-source project developed and maintained for the public good. As such, starting from today, I will no longer participate in Nginx development as run by F5. Instead, I am starting an alternative project, which is going to be run by developers, and not corporate.
Freenginx is not the first fork and drop-in replacement for Nginx: Angie was created by other Russian Nginx developers when F5 left Russia in 2020 and it is currently managed by the Russian company Web Server. Diogo Baeder, lead backend developer at DeepOpinion, comments:
Nginx is an incredible software and platform, but I was wondering if it wouldn’t be time to just bite the bullet and create a more modern solution based on Rust. Having a solution that follows a similar model, “understands” the Nginx configuration language, and reaches similar performance levels, but with the memory safety and wide community adoption of Rust could lead to an amazing new project – perhaps even doing to Nginx what Nginx did to Apache HTTP.
Vincentz Petzholtz, network engineer and architect, is less optimistic and adds:
Sometimes a fork is all you can do when a project is taken into a difficult direction. In the end, the users will vote with the adoption and install-base.
The first release version is Freenginx-1.25.4 under the same BSD license as Nginx. Dounin has provided access to a read-only Mercurial repository, discarding for now the option to migrate to GitHub. The project started a new developer mailing list.
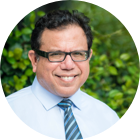
MMS • Anthony Alford
Article originally posted on InfoQ. Visit InfoQ
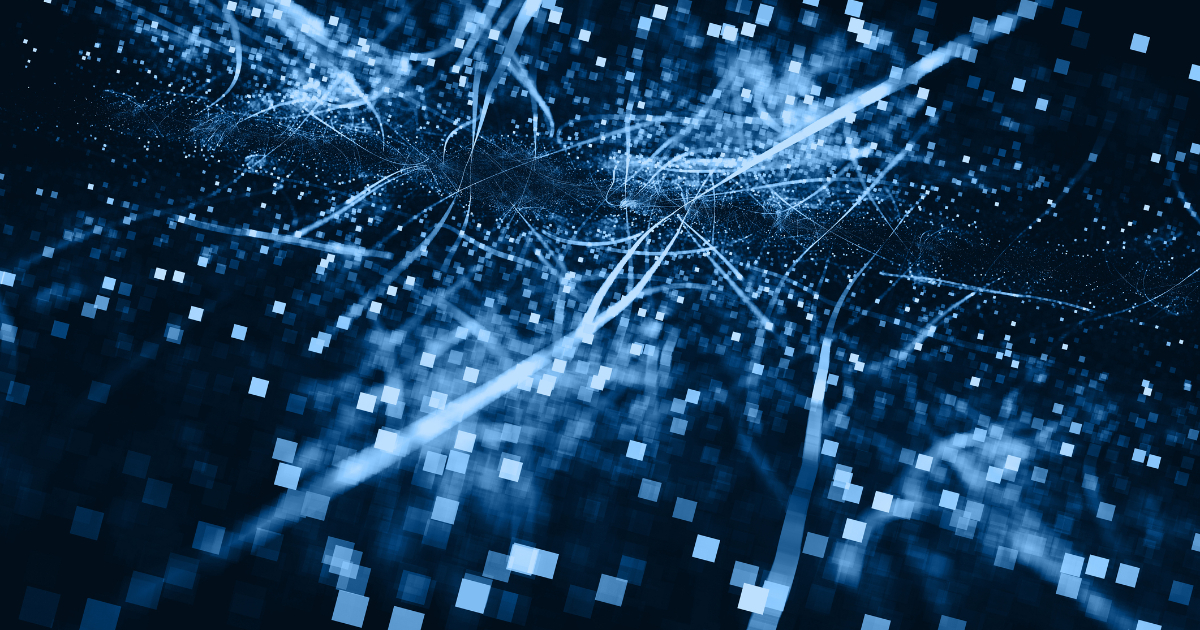
The RWKV Project recently open-sourced Eagle 7B, a 7.52B parameter large language model (LLM). Eagle 7B is trained on 1.1 trillion tokens of text in over 100 languages and outperforms other similarly-sized models on multilingual benchmarks.
Eagle 7B is based on the Receptance Weighted Key Value (RWKV) architecture, described as an attention-free Transformer that combines the benefits of both Transformers and recurrent neural networks (RNNs) while reducing their drawbacks; in particular, the model has no maximum input context length. The architecture has also been benchmarked as the most energy-efficient, measured by joules per token. Eagle 7B outperforms other 7B-parameter LLMs, including Mistral, Falcon, and Llama 2, on several multi-lingual benchmarks. The RWKV Project is supported by the Linux Foundation, and Eagle 7B carries the Apache 2.0 license, making it available for both personal and commercial use. According to the Project team:
RWKV opens a new route for scalable and efficient architectures to model complex relationships in sequential data. While many alternatives to Transformers have been proposed with similar claims, ours is the first to back up those claims with pretrained models with tens of billions of parameters.
Before Google published their work on Transformers, RNN-based models were the state-of-the-art solution for many AI applications, particularly in multilingual NLP domains such as translation. The Transformer was an attractive alternative, since training RNNs presents challenges, and their inherent serial nature makes them slower than Transformers. However, Transformers have their own drawbacks. In particular, their self-attention mechanism has quadratic complexity in both compute and storage, which limits their input context length.
To solve these problems, RWKV uses a variant of the Attention-Free Transformer (AFT), with a modification that allows the model to be formulated as an RNN. This formulation makes the model efficient during inference, when it is used for autoregressive generation. However, during training, many of the model’s matrix operations can be parallelized, as with a standard Transformer.
The RWKV architecture does have known limitations. While it does not have a maximum input context length, it may not perform as well as attention-based models on tasks that require “looking back” in a very long context. For the same reason, it also requires “carefully designed prompts,” as prompt information may be lost during inference.
In a discussion about Eagle 7B on Hacker News, one user touted its advantages:
These models don’t have a fixed context size and are progressively fine-tuned for longer and longer contexts. The context length also doesn’t impact inference cost. Another aspect of performance is not just how well does the trained model perform, but is it data efficient (performance per token trained)?
Lead RWKV developer Peng Bo posted about the model on X, showing its performance on what he called an “uncheatable” benchmark: calculating the model’s perplexity on new papers posted to arXiv:
Arxiv is the beginning. We can use latest news, github repos, arxiv paper, blog posts, new wiki entries, and more. The point is to benchmark LLMs on new data – although they can be polluted by ChatGPT too, it is still better than using very old (and actually noisy) evals.
The Eagle 7B code is available on GitHub, and the model weights on Huggingface.
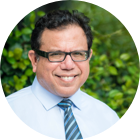
MMS • Stephanie Wang
Article originally posted on InfoQ. Visit InfoQ
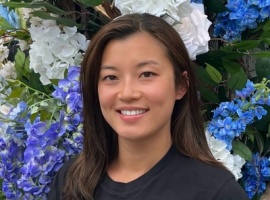
Transcript
Wang: My name is Stephanie Wang. Welcome to my talk about enabling remote query execution through DuckDB extensions. As the name implies, we will be talking a lot about DuckDB extensions, and a little bit about query executions, the internals of what happens when we run a query, and how we’re able to enable remote query execution through DuckDB extensions. We’ll probably go through a bit of information on what is DuckDB. Why are people so excited about it? We will zoom in on DuckDB extensions and understand how DuckDB query execution works locally. Understand why we want to enable remote DuckDB query execution, and how we are able to achieve it through DuckDB extensions. Finally, I will be sharing a little bit of my learnings and takeaways from developing with DuckDB and DuckDB extensions in the last year.
I am a founding software engineer at MotherDuck. MotherDuck is a company that we founded around a year ago, focusing on building a serverless DuckDB. Prior to coming to MotherDuck, I worked on BigQuery developer tools, mainly focusing on BigQuery client libraries for around three years. I worked on building sales and trading applications at Morgan Stanley for three years before that.
What Is DuckDB?
What is DuckDB? DuckDB is an in-process OLAP database. OLAP stands for online analytical processing. The main use case of DuckDB is focused on analytical use cases. The magic about DuckDB is that it can run inside Python or C++ and other languages. There is no external dependencies in this library. It also offers full SQL support. It can do very high-performance aggregations with the columnar vectorized execution engine. DuckDB was created at CWI, a Dutch research institution, by Hannes on the left, and Mark on the right. It is currently maintained by DuckDB Labs, and also DuckDB Foundation, as well as the community surrounding DuckDB. DuckDB has gained a lot of interest and popularity in the past few years. If you follow DB Engine Score, it has really skyrocketed over the last few years. This DB Engine Score is computed based on web content, Stack Overflow, job searches. On top of that, the GitHub repo stars now has reached over 10,000. To give you a basic idea, the Spark GitHub repository has around 35,000 stars, and the most popular repository in GCP has around 14,000 stars. This open source project is wildly popular amongst the community. Also, the DB Engine’s monthly growth is around 40%, and PyPy download is around 400,000. There are about 100,000 monthly unique visitors to duckdb.org.
Common DuckDB Use Cases
You may wonder, what are people using DuckDB for, this in-process OLAP database engine? There are two general ways that folks are using DuckDB for. I will break them down into the first category, which is human interactions. The second category which will be machine interactions. On the human interactions front, most users will use DuckDB in Python or Node or R notebooks. These users are mainly analysts or data scientists. An example is querying a parquet dataset that lives in S3. For example, if someone has a dataset that is already in S3, you can download this dataset locally to your computer, and connect to DuckDB via Python or R. You can start querying and getting query results from it. You can then move on to doing your dashboarding activities. You can share your Python notebook work in progress or results with your colleagues, very easily. This is a popular use case because it allows analysts and data scientists to iteratively work on the data in DuckDB, interwoven with other libraries like pandas. The support for pandas is very advanced in DuckDB. The other type of use case is machine interactions. Either using DuckDB as a persistent or ephemeral execution engine, and this use case is mostly covering data engineers. For example, you can spin up DuckDB in EC2 VM, or even on-premise. You could write some lambda functions that include DuckDB, and use it as your database. This allows you to read data directly from S3, or from your other sources that you download locally. As part of the CI build, for instance, DuckDB is able to deliver some output, typically in parquet format, or in other forms of query result sets.
What’s Under the Hood of DuckDB?
What is under the hood of DuckDB? How is it able to be in-process, so fast? Why are people so happy about it? Comparing some database engines, we’re familiar already with the row-based engines. These are great for transactional workloads. Because it’s very easy to insert new data into these row-based database engines, there is low memory usage for analytics, because you need to read each row at a time, and that really kills the performance. Some examples of row-based database engines include SQLite, PostgreSQL. In contrast to the row-based database engines, we have the columnar database engines. These are much better for analytics, because you can process only specific needed columns, because most data in a column is likely to be very similar. This allows you to potentially use efficient compression algorithms like dictionaries and run-length encoding for these repeated values. Good examples of these database engines are pandas, NumPy. DuckDB is built based on a vector-based query engine, and that’s a little bit different than what came before. This is a type of engine that is optimized for analytics workloads. Specifically, an example is aggregations, and it utilizes your local CPU very efficiently.
I want to talk a little bit more about cache locality. Cache locality is important because it needs to be considered to enable high performance in query engines. The closer you are to the CPU, the data can fit, which is L1 cache here, the faster the data can be accessed. L2 is slower, and L3 is slower yet. Then after that, main memory or disk. The cache sizes for my machine that I’m using here right now, for example, is pretty large. Imagine a vector database is like a big book with lots of words, and the CPU is like a reader. SIMD is like having the ability to read several words at once, instead of reading one word at a time. This helps the reader, which is the CPU, read the book, which is the vector database, much faster, which also means much better performance. Regarding cache locality, imagine the book which is the vector database is divided into several smaller books, which are caches in our example. The reader, which is the CPU, can quickly grab a smaller book, which is a cache, and find the words that they need. Cache locality means that the words used together are in the same smaller book. This makes it easier for the reader which is a CPU to find the words they need without searching through all the smaller books, which is all your caches, which speeds up the reading process, and improves performance. This is the basic reason why vector databases are very fast and super snappy. DuckDB, in particular, can process data that is larger than memory. It has a native storage format, which is columnar and partitioned. It stores the entire database, not just the tables within the database, and it offers ACID-compliant updates, when you need to make changes to your database. When it comes to data that is larger than memory, it spills overs to disk.
To use DuckDB, it is quite simple. Here’s an example of the CLI. You can go to the duckdb.org website, and download a CLI that is built for your local machine’s architecture. You can simply just click on it and start interacting with the DuckDB executable. You can start by running a common SQL command like CREATE TABLE. You can start just working on your datasets from there. Super easy, no need to set up Cloud Data Warehouse credentials, create account, and anything like that. It’s also very well integrated into Python and R, like I mentioned before. In Python, you can simply run a pip command to install DuckDB locally. You can import DuckDB, like you would normally do with any other Python libraries, and start writing Python commands and running SQLs using DuckDB.
DuckDB Extensions
Now maybe we can talk a little bit about working with DuckDB as a developer. As you’ve already seen, using DuckDB as a user, maybe as a data engineer, SQL analyst, or a data analyst is super easy. I want to just jump into the next topic about DuckDB extensions, and talk a little bit about how to develop on top of DuckDB. You will see that the process is also quite simple with these DuckDB extensions. First of all, you may wonder, what are extensions and why do we have extensions? Extensions are basically the parts of the code that are not built into the main source tree of DuckDB. This allows DuckDB to start from a lighter version of a database management system. The reality of a DBMS is that in most cases, people don’t need all the possible features of a DBMS. For example, most of the DuckDB users, for instance, will eventually scan a CSV file. They probably want that to be a part of the core of DuckDB. Some of them probably do not need to ever scan Postgres files. Postgres is built as a separate standalone extension, away from the DuckDB core. Instead of shipping a feature that’s only meant for a small percentage of DuckDB users, they’re able to ship a lighter version of DuckDB that contains only what is considered to be essential for the core users of DuckDB.
There is no external dependencies for DuckDB. However, you can have whatever dependencies you want for the extensions as a developer. For example, you want to leverage certain libraries to parse things or do other stuff, you can feel free to bring those in as part of your extension. For DuckDB core, there is no external dependencies. Having extensions built away from DuckDB core, also allows them to keep the binary size and compilation time in check. This is quite important. If you’re developing on top of DuckDB, you want to make sure that it’s super-fast to compile the entire DuckDB, so you can run your tests and see how things are performing. It also facilitates binary distribution because you have very fast compilation time and very small binary sizes. This allows you to be able to distribute this DuckDB database very easily. It also allows for private closed source additions on top of DuckDB. That’s something that we do at MotherDuck is we’ve developed very advanced DuckDB extensions, and that part of the changes are closed source and private. However, it leaves DuckDB core alone to be open sourced and completely separate. This allows us to develop without having to fork the main DuckDB repository. These are some of the motivations of why you now have extensions and what these are.
Some examples of the DuckDB extensions that already exist. First, these are distributed through the simple install and load commands, which means if you want to use a new DuckDB extension that’s already distributed, you can simply run, install something, and then load something. The extensions listed down here are the very common and DuckDB supported extensions. Fts and httpfs, for example, are very common. It allows you to read and write files over HTTPS connection, and you can do a full text search with the fts extension. On top of that, you can read JSON files with the JSON extension. You can read parquet files with the parquet extension. Similar goes for Postgres, sqlite_scanner. They even have support for Substrait, which is a separate open source project that’s focused on query plan standardization across the board. Of course, they have extensions for TPC-DS and TPC-H benchmarks as well. In order to use any of these extensions, like I said, is very simple. There are only two commands. The install command downloads and moves the extension to the DuckDB extension folder, which is shown over here. It’s super easy. The load commands is the one that actually loads this extension to a DuckDB binary. To run it, you can just run load and then provide the default directory or a full path to the extension, if it’s different than the default location of the extension. It may be different if you have built a custom extension in a separate location that you want to test. For testing purposes, whatnot, you could then load the extension from your other local locations, or it could be an S3 bucket that you’ve decided to upload your extension to.
There are two types of DuckDB extensions in general, signed versus unsigned. Any developer can create a DuckDB extension, and these can go to the unofficial Amazon S3 bucket, and these are considered unsigned extensions. That means the binaries has to be distributed by the producer of these extensions. You probably need to specify a different endpoint for installation. Same for load. There is no checks on the source code of these extensions from the DuckDB team. However, they do offer some boiler plate CI that does distribution of the extension for you. These are the unsigned DuckDB extensions. The signed DuckDB extensions on the other hand, are used for several core DuckDB functionalities. These are maintained and primarily developed by the DuckDB team. Like I mentioned earlier, it has support for reading from remote file systems like S3 over HTTP. It offers support for scanning over other data sources such as parquet, SQLite, and Postgres. It also can offer new scalar functions, aggregate, window, table functions, such as JSON, fts, and ICU. Through the ICU extensions, for instance, you can get time zone support and collation support. These are some of the examples of DuckDB extensions that are supported. The last one is the most recent addition, which is a geospatial extension that adds support for spatial data.
We just covered how to use DuckDB extensions as a user. How do you actually build a DuckDB extension? It’s actually super easy as well. Similar to how you would use DuckDB, which is through the install and load commands, there are also two key things to building a new DuckDB extension. DuckDB exposes two critical functions to allow you to start exposing an extension. The first one is version, and the second one is init. As a developer, you would have to then just add your logic after the init, and make sure that your version is compatible with whatever DuckDB binary it is that you’re building with. The version verifies the compilation and linking done against the same DuckDB version. This is critical because DuckDB is used as a library, so when you’re developing on top of it by building an extension, you want to make sure that everything can link correctly, and it is using the same exact version to avoid any conflict or compilation errors. Init is the method that actually loads your extension, and allows all your logic to be processed after that. All the extensions are written in C++. Most likely, your extension will be written in C++ as well. The beauty of these extensions is that you get access to all of DuckDB’s internal code, and you really start to appreciate how DuckDB is built like a library that is plug and play, and is very powerful.
Remote Query Execution, and Local Query Execution
Now let’s jump into an example of how we’re able to enable remote query execution through DuckDB extensions. We will first go over some basic query execution ideas and architecture. Then we’ll talk about why we need to run queries remotely, now that DuckDB is able to run queries very efficiently locally. Then we’ll take a look at how the architecture looks like for remote query execution. Then jump into a particular example for a deep dive, before we share some takeaways and learnings. Before we zoom in on to the remote query execution part of things, we can talk about the typical client server architecture for query execution. Typically, you would have a client-server architecture for running a query. You would have a client that is your application. Then you would have your database that lives maybe on your server side, which hosts your data. In between, you have the network that transports your API call and whatever you serialize into your binary data to the server. The database itself hosts the data, and also the query engine. The application logic is split between the application and the query engine. An issue about this particular model is that network can be slow and untrusted. Sometimes you will run into various issues by executing every single query over the wire. An in-process database, on the other hand, does not have those types of issues with network because everything is in-process in the applications process. SQLite is an example of an in-process database, where the database along with the data lives right inside some application’s process. They all live in the same process. You don’t have trust boundaries to traverse, and that allows you to execute queries super quickly. You have high bandwidth between all your components. Of course, the limitation is that the application and everything is done in-process, and you have to worry a lot about how to utilize the threads and the resources within your application. DuckDB is very similar in terms of architecture as SQLite. The difference is that SQLite is catered towards OLTP workload, which are transactional, but DuckDB is suited and built for really analytical workloads.
How does local query execution work in DuckDB? Maybe it helps to understand a little bit of under the hood mechanism of how DuckDB executes a query. DuckDB, in general, uses a very typical pipeline for query processing. First, a SQL query is input into DuckDB as a string. Then after that, the lexer and parser take this input string, and convert it into a set of statements, parse expressions, and table references. In the case of DuckDB, they use the Postgres parser. They have their custom transformer, which transforms Postgres Node statements into DuckDB understandable classes from there. Then, these statements will go into the planner, which then uses it to create an optimized logical query plan, which are also query trees. These logical query trees contain logical operations of a query execution. It basically describes what to do, and not necessarily how to do it. An example is, you would do a join, but at the logical planning step, you will now specify whether you’re doing a hash join or a merge join. Then, after that, this unoptimized query plan goes into the query optimizer, which then rewrites the logical query tree into another equivalent more optimized query tree, which is also called an optimized logical plan. How does the optimizer do that? It uses a mix of rule-based and cost-based optimizers. Then, finally, this optimized query plan will go into the physical planner, which then converts it into a physical plan for query execution, and from there on, you would build pipelines and execution would follow.
Using DuckDB, which is in-process, we’re able to create a hybrid database architecture where you can have an application that holds a local cache, and then you can then send requests over the network occasionally, if that’s necessary, to the server, which also has an instance of DuckDB that could run queries there, has data, or decide to send query results back to the client. In other words, the data and query engine live on both sides of the network boundary. You don’t have to pick and choose. You don’t have to only have the database live on the application side, or the database live on the server side, you can have the database live on both the application side and the server side. This client-side query engine, DuckDB, in this case, can enable better integration with the application itself because it would know the application better. It also allows you to better utilize the cache locally. The server DuckDB can run queries, just like how the client side would do. This opens up opportunities for query plan level optimization. To zoom in a little bit on how we can utilize DuckDB extensions, and the architecture, which I had just mentioned. You can have a client, and this could be your Jupyter Notebook, it could be your CLI, it could be Wasm even, something else. This is where you can have your DuckDB instance running. Another example is the web UI, you can have a DuckDB client extension running there as well. They can talk to the DuckDB that’s in the server that lives in the cloud. We can allow data persistence through another storage system, and then you can coordinate and orchestrate all the operations through a control plane. All of these clients can be doing similar stuff, sending requests to the cloud. You can cache things locally in one of these clients, and only make those over the wire RPC calls when it’s necessary.
How can we enable remote query execution? DuckDB offers many different extension entry points. There is a parser extension, for example, that allows you to intercept the parsing stage. There are other extensions as well where you can intercept anywhere you find appropriate. Typically, if you want to, the parser extension allows you to intercept and parse grammar that are not parsable by DuckDB. This is nice, because if it’s something that DuckDB already can parse, you typically do not need to parse those things again. If you want to add additional grammar support for your particular use case, then you can simply intercept at the parsing stage and introduce your own parser. An example could be if you want to enable multiple users using DuckDB scenario, to do something, then you may want to introduce a Create User grammar. For that to work, you’d have to parse the grammar yourself and transform it into something that DuckDB can understand, so we can go back to the query execution workflow. To do that, it’s very simple, you can just introduce your parser extension. Like I mentioned before, you just need to implement the version method and the init method, and your other logic would go into the init step for loading your actual extension. After that, there is an optimizer extension that DuckDB offers where you can intercept the query plan and introduce your own optimization logic. This could be that if you want to tell DuckDB that if you see this type of logical operator that requires data remotely, then do not execute this query locally, but instead execute this query remotely using the server-side DuckDB instance. That’s an example.
To enable this optimization logic that’s custom, it is probably likely that you’ll be introducing your own operators as well, and that is achievable through using the DuckDB operator extension. This allows you to create customized execution code and you can also patch any serialization issues, because, like I said, DuckDB’s architecture is in-process and fully local. They do not have the need to have to send requests over the wire, which means they do not have to serialize any of their data. For you to actually be able to run a query on the server side, you will need to perform this serialization step. You may have to then introduce your custom logic to serialize something into binary data format, so you can send that over the wire. You can use this to create local or remote logical operators, for example. What happens after that is that you can use these local and remote physical operators, which are produced after the logical planning step to build pipelines for actual query execution. In our case, we couple pretty tightly with the DuckDB pipeline construction. What we need is to really intercept the DuckDB scheduling process and achieve a low-level execution workflow. This allows us to, for example, parallel operators as we wish, and also handle data flow, so basically, sending data across the wire as part of the DuckDB query execution flow that they didn’t have before. You can also fetch data, get data as part of the physical planning step. You can make direct RPC calls over the wire to fetch data chunks or perform any create, update, delete operations using your custom physical operator. Finally, there’s also a DuckDB storage extension entry point where you can virtualize your catalog, and that can simplify the bind stage quite significantly, because you can confine everything to your virtual catalog when you create new database objects.
The motivation really, like what this allows us to achieve is, first, you can have faster execution speed, because the vast majority of data lives in the cloud. It makes sense to run computation closer to where the data resides. This can significantly reduce the amount of data that needs to be transferred across the network, the wire. It shields us from issues like unstable network, and also slow I/O. On top of that, part of the remote query results can be cached locally. If you’re smart about it, you could reuse these query results locally, to just leverage the local computation resources as opposed to having to do everything in the cloud. An example of using this is CTE. You can always CREATE TABLE. Then you can reuse the table or maybe make that into a materialized view, so you do not have to manually invalidate the table when the data in there gets updated. There is some cost reduction possible as well with enabling remote query execution through DuckDB. Because we’re already able to leverage the local compute resources, so we don’t have to run everything in the cloud, and that potentially can save some money in managing cloud resources. There could be some benefits regarding compliance, where sometimes users have data that they have locally, and they may not want them to leave their laptops. DuckDB enabling this type of hybrid execution allows them to just keep data where it is. The same idea applies for in the cloud scenario. If you have your data in the cloud, you can have that just there. You don’t have to move it around in order to execute a query.
Example
Maybe it helps to do another deep dive on an example of running a remote query using this DuckDB query engine. An example here offered is to detach a database. DuckDB recently introduced the support for multi-database interactions, where you can attach to and use multiple databases at once using a single DuckDB connection. From our end, we wanted to make sure that we can also detach a database which is previously attached, both locally in the DuckDB instance, and remotely in our DuckDB instance, that is in the cloud, because you want to make sure that you do this in both places. What happens is, first, we want to execute this SQL command locally. That basically comes for free, because DuckDB already supports this syntax. All we have to do is to check in our extension whether this database is attached in the local catalog or not, and if so, we will just detach this database locally. If it’s not, then we make an RPC call over the wire to detach this database remotely, when it is found in the remote catalog.
To make this work, there are two ways. The first is to hook into the DuckDB storage extension. The second is to go through the DuckDB Optimizer extension. To go through the DuckDB storage extension, we would essentially have to modify the DuckDB code, which is not very difficult, because DuckDB is very friendly to community contributions. If anything makes sense, they usually accept your contribution. In this particular case, we figured it might take more cycles for that to happen, so we went with implementing it using the DuckDB Optimizer extension, which essentially allows you to achieve the same effect. What you will do is you can intercept the query plan, when you see a logical detach operator. Then you can substitute this logical detach operator with your custom, local remote or, say, hybrid detach operator by extending the logical extension operator. This logical extension operator is something that we actually introduced to allow this level of flexibility where you can introduce any types of logical operators, that make sense for your execution workflow. In this logical operator, you can then make a create plan call to produce a custom physical local remote or, say, hybrid detach operator. As part of this physical operator, you can then perform the database detach logic by making that remote RPC call. If it makes sense, you can fetch data as part of get data, or you can receive back a Boolean indicating whether the detach happened successfully or not. Or maybe not. Maybe you just handle an exception on the client side and the server throws some type of exception. As you can see, it sounds complicated, but, really, because there are so many entry points into DuckDB, it is very flexible for you to intercept the logic and do your thing, and then fall back to the normal DuckDB execution flow.
Learnings and Takeaways
Finally, some learnings and takeaways. Hopefully, you’ve gotten the idea that DuckDB extensions are very powerful. You can do really crazy and advanced things with these extensions. There are lots of entry points where you can enter into the DuckDB code base, and really use it as a library to compose whatever you need, and then achieve whatever workflow you would like to achieve. Then fall back to DuckDB execution and get the results that you want. It’s very easy to get started. What I did to get started was to check out some popular extensions. An example is the sqlite_scanner extension. This is an out-of-tree extension, which means the code lives outside the core DuckDB source tree. You can also check out the httpfs extension, which is an in-tree extension. I think at this point, most likely, you will be developing an out-of-tree extension. Probably the SQLite or Postgres scanners are more relevant, but truly, both in-tree and out-of-tree extensions are very similar. They both have to implement the init and version methods. Then you would have your loading of the extension, and then you can do anything you like within your extension, and then just call DuckDB code wherever appropriate. There’s also an extension template that DuckDB Labs recently worked on, that helps you get quickly started with building an extension. You can also check out the automatic platform building, distributing CI that DuckDB offers, so you can get your binary distributed very easily.
A note is, DuckDB is evolving very quickly, and has a very vibrant developer community. If you feel like you’re developing your extension, and you need something changed in DuckDB, and we’ve done this many times over. For example, we needed to add more serialization logic for making RPC calls, and we’ve done that with them. Or, if you want to make a method virtual or public so that you can get access to it, if it makes sense, if it’s a common utility method, you can just go into the code base and make that change as appropriate. Really, anything else. Adding this extension operator, for example, is something else that we did that made sense for the open source community, as well as what we were doing at the time. You can feel free to make those contributions to the core DuckDB code base. With that said, DuckDB is evolving very quickly, which means your extension may no longer work if you don’t keep it up with the DuckDB versions that keep upgrading. A way to counteract that could be to build out CI, that test against DuckDB versions, and make sure that your extension is up to date with the code. Recently, for example, they’ve introduced their own unique pointer type, which overtakes the standard C++ library unique pointer. You would have to update your code accordingly. It also helps to keep an eye out on the DuckDB release notes. They will usually point out these critical changes. That helps you keep your extensions up to date. Finally, really, sky is the limit. There’s so much you can achieve with these extensions. It’s super fun to play around with it, and see how you can leverage the entire DuckDB code base, to achieve anything, really, that you would like to achieve with your extension. Let your creativity soar.
See more presentations with transcripts
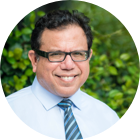
MMS • Dio Synodinos
Article originally posted on InfoQ. Visit InfoQ
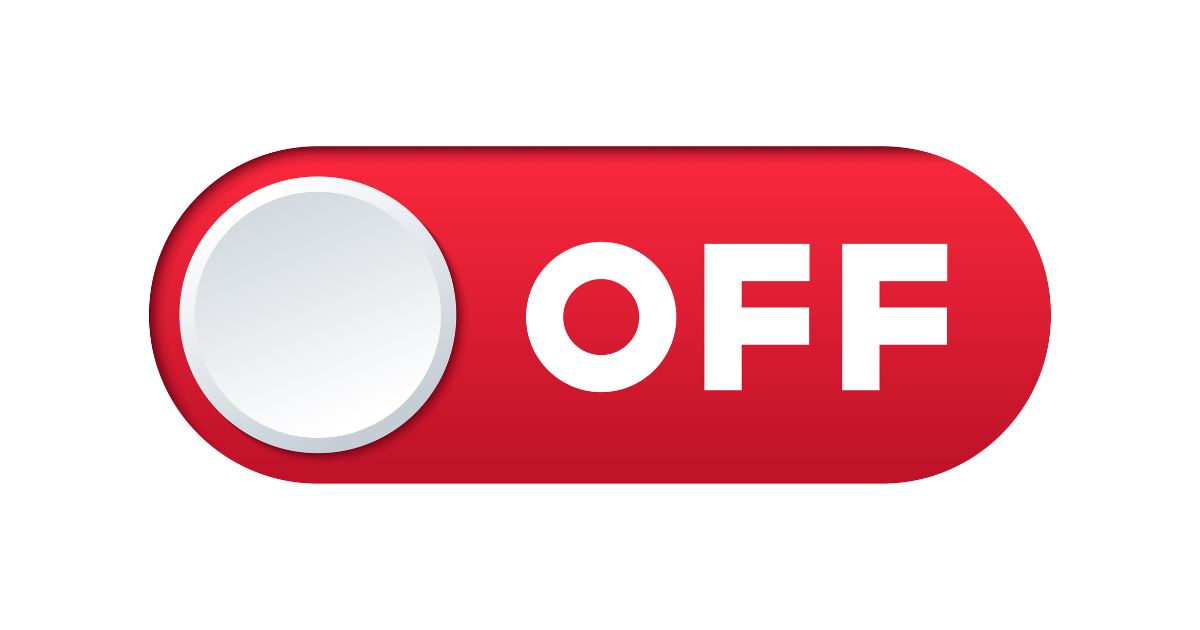
We are going to start phasing out reader commenting features across the website by March 18th, 2024. This will remove functionality like:
- Commenting on posts
- Following comment threads
- Getting notifications about comments from users you follow
The reasons for that are:
- Discussions happen elsewhere; there are dedicated services where communities have discussions like Social, Reddit, HN, and more.
- Spam has grown exponentially; fighting spam is becoming increasingly difficult for a small team like ours. Most of the comments we moderate are malicious attempts to build links and SEO hacks.
- Legal challenges: the legal requirements make user commenting practically impossible for international publishers.
We are planning on new features that will provide our community with more meaningful ways to engage with each other, especially around Trend Reports. So stay tuned!
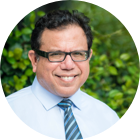
MMS • Sam Van Overmeire
Article originally posted on InfoQ. Visit InfoQ
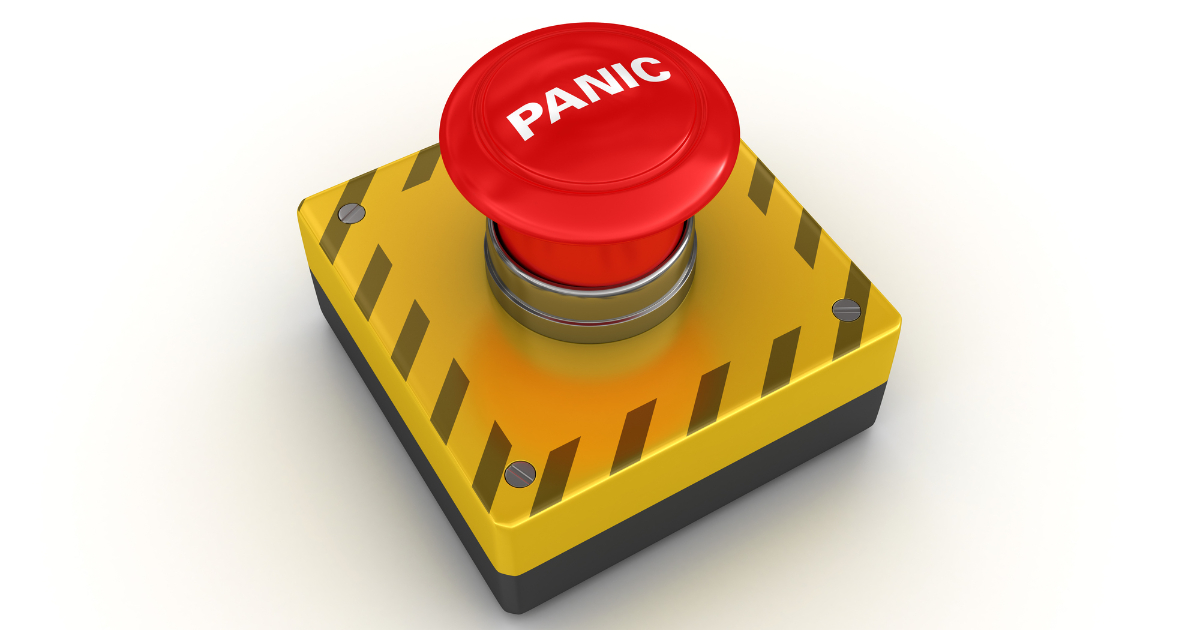
Key Takeaways
- Procedural macros can manipulate existing code, allowing us to replace, for example, panics with Errs.
- We can explore an entire function definition with the standard syn library, but if that function contains nested syntactic structures, doing so might require a lot of repetitive work.
- syn has additional functionality hidden behind feature flags, including the Fold and Visit traits.
- Fold and Visit allow us to recursively step through a function, so we only need to overwrite trait methods that are of interest to us.
Procedural macros are a powerful tool for manipulating Rust code. Programmers that want to write these macros will undoubtedly turn to libraries like syn
and quote
for parsing and outputting token streams. But in more advanced use cases, the standard tools that syn
provides might prove lacking, resulting in fragile, repetitive code.
In this article, we will demonstrate these shortcomings through a toy example, where we replace every panic in a function by an Err
. We will first show the kind of code you would normally write. Afterwards, we will turn to the Fold
trait, which makes the code for this kind of manipulation a lot more elegant.
Example use case: replacing panics
syn
, the standard Rust library for parsing procedural macro input, has a wide range of functions that can aid us in generating and transforming code. You can also use its standard functions for handling multiple, recursive manipulations. That will work just fine.
But we have to cover every possible case – and there could be a lot – ourselves. So you might regret your choice of tooling when you see how much code you have to write.
The wiser course of action is to turn to the library’s Fold
trait. Hidden behind a feature flag, it is an excellent choice for recursively changing code, offering multiple methods that allow you to ‘hook in’ to specific parts of your input.
To illustrate this trait’s usefulness, we can turn to an example from my book, Write Powerful Rust Macros: using a procedural macro to replace panics with the Err
enum variant in functions. The following code snippet shows the panic_to_result
macro in action:
#[panic_to_result] // use our macro
fn create_person_with_result(name: String, age: u32) -> Result {
if age > 30 {
panic!("I hope I die before I get old"); // <- panic will be replaced by an Err
}
Ok(Person {
name,
age,
})
}
fn main() {
// the assertion shows we got back an Err instead of a panic!
assert!(create_person_with_result("name".to_string(), 31).is_err());
}
Converting panics into errors is per se an interesting transformation. But the code’s primary goal was to serve as a guiding example for learning proper error handling in procedural macros, providing answers to questions like “How can I return errors from my macro?” “How do I point that error to a specific line in the user’s code?” etc.
We also briefly introduced the various error-handling styles of modern programming languages, including Rust — which relies heavily on Algebraic Data Types. Hence, getting the macro to work for every possible input was never the goal. In fact, the book’s code looks specifically for panics inside if
statements. This is convenient because that is where the panic occurs in our example. You can see it in the following snippet from the macro implementation:
match expression {
// check the if expressions for panics
Expr::If(mut ex_if) => {
let new_statements: Vec = // modify existing statements
ex_if.then_branch.stmts = new_statements; // and put them inside the if
Stmt::Expr(Expr::If(ex_if), token)
},
// return all other expressions without modification
_ => Stmt::Expr(expression, token)
}
This implementation has the advantage of going through the structure of a parsed function step by step. For learning purposes, that’s a good thing. The downside is that this solution lacks extensibility. Do we really want to check every possible type of input? Do we want to add a new match for while
expressions, and loop
, and … you get the point.
The Fold trait
The syn library has an advanced tool for use cases like this one, where we want to go through our input recursively: the Fold
trait.
Fold, which is hidden behind a feature flag, offers (according to the documentation):
“Syntax tree traversal to transform the nodes of an owned syntax tree. Each method of the Fold trait […] can be overridden to customize the behavior when transforming the corresponding type of node.”
That sounds useful. Another trait hidden behind a feature flag, Visit, is similar. But it uses a borrow of the tree and does not return anything — less suitable for what we want to do here.
Fold helps us visit every node in a program’s AST. And because it takes ownership of the syntax tree, we can modify those nodes, eventually resulting in a tree that has been altered to our liking. In our case, what we would like to do is walk the AST tree, replacing every panic with Err
. This, as you will see, requires surprisingly little code when using Fold.
Now, start by creating a new Rust library with cargo init --lib
and turn it into a procedural macro by setting proc-macro=true
in your Cargo.toml
. You will also need to add some dependencies
[dependencies]
quote = "1.0.33"
syn = { version = "2.0.39", features = ["fold", "full"]}
[lib]
proc-macro = true
syn and quote are two procedural macro classics, the former helping us with parsing, the latter turning our data into a TokenStream
that Rust can work with. The chosen syn features are important because Fold is hidden behind the fold
flag, while the fold::fold_stmt
function, that we will also be using, is activated by the full’
feature.
Next, we define our entry point, the function panic_to_result
, declared as an attribute macro. An attribute macro replaces the existing code with the code (as a stream of tokens) that we return from it as a stream of tokens. Hence, the output we generate here will replace the annotated function’s entire definition.
panic_to_result
first transforms the input into an ItemFn
, meaning we are expecting a function,. then it uses a custom struct and fold_item_fn
to fold the input, returning the result as a TokenStream
and passing the result to quote
.
use proc_macro::TokenStream;
use quote::quote;
#[proc_macro_attribute]
pub fn panic_to_result(_attr: TokenStream, input: TokenStream) -> TokenStream {
let item: ItemFn = syn::parse(input).unwrap(); // parse the input
let result = ChangePanicIntoResult.fold_item_fn(item); // fold it
quote!(#result).into() // and return the result
}
We are now getting closer to the key section of our implementation. But first, let us look at a helper function called extract_panic_content
. This function checks whether a given Macro
in the annotated function is a panic
, by comparing its identifier with “panic” as a string. If it is a panic
, we return its content, i.e. everything between the opening and closing parentheses. We will need that content soon enough to build a message for our Err
. In every other case, we return None
.
fn extract_panic_content(mac: &Macro) -> Option {
let does_panic = mac.path.segments.iter()
.any(|v| v.ident.to_string().eq("panic"));
if does_panic {
Some(mac.tokens.clone())
} else {
None
}
}
Now, let’s focus on the core of the macro, some 20 lines of code. We implement Fold
for our struct and overwrite the fold_stmt
method. Because a panic
is a Macro when parsed by syn
, we want to check that struct using our extract_panic_content
helper to verify whether it really is a panic
. If it is, we get back the content/message that was previously inside the `panic
`, which we quote to create the new Err
return value.
Finally, thanks to a little trick with parse2
, the generated tokens are converted into a statement, which we return from the function. A cool thing to notice here is that no type specification is needed here because Rust infers it based on the function’s output type. When we do not have a macro or a panic, we give back the existing Stmt
. Finally, fold::fold_stmt
is called to make sure syn keeps on folding.
use quote::quote;
use syn::{fold, ItemFn, Macro, Stmt};
use syn::fold::Fold;
struct ChangePanicIntoResult; // the struct that we were calling in the entry point
impl Fold for ChangePanicIntoResult {
fn fold_stmt(&mut self, stmt: Stmt) -> Stmt {
let new_statement: Stmt = match stmt {
Stmt::Macro(ref mac) => {
let output = extract_panic_content(&mac.mac); // helper to get the panic message
output
.map(|t| quote! {
return Err(#t.to_string());
})
.map(syn::parse2)
.map(Result::unwrap)
.unwrap_or(stmt)
}
// panics should be inside a 'Macro', so in every other case we return
_ => stmt
};
// keep folding
fold::fold_stmt(self, new_statement)
}
}
Things to note: while we called fold_item_fn
in our macro entry point, we did not implement it. The trait has a perfectly fine default implementation. So why did we implement fold_stmt
? Because we know syn parses the content of a function into a Block
, a vector of statements. That means the entire body consists of statements, including our panics
, and it’s there we should go looking for them!
This may raise further questions. Maybe you are now wondering why we did not just implement a fold_macro
if that exists. After all, a panic
is parsed as a Macro
in syn. And that, in fact, is what I originally wanted to do! Only to realize that I would be manipulating a macro and replacing it with an Err
, which is definitely not a macro. And, sadly, the fold_macro
definition specifies we have to return a macro.
Complete example
Let’s see our code in action. I have altered the previous example to include a loop. In our main, we will test all three possible paths.
use fold_macro::panic_to_result;
#[derive(Debug)]
pub struct Person {
name: String,
age: u32,
}
#[panic_to_result]
fn create_person_with_result(name: String, age: u32) -> Result {
// 'if' works
if age > 30 && age 50 {
panic!("This person is old... very old");
}
break
}
Ok(Person {
name,
age,
})
}
fn main() {
let first = create_person_with_result("name".to_string(), 20);
println!("{first:?}");
let second = create_person_with_result("name".to_string(), 40);
println!("{second:?}");
let third = create_person_with_result("name".to_string(), 51);
println!("{third:?}");;
}
As the output shows, what should have thrown wild panics has turned into docile Results
. Other things like, for example, nesting if
statements work as well.
Ok(Person { name: "name", age: 20 })
Err("I hope I die before I get old")
Err("This person is old... very old")
This is not a full-fledged solution for improving error handling with macros. For example, one drawback is that it only works with functions that already return a Result
. But it does the job as an example of how the Fold trait and a bit of custom code can accomplish some very powerful things.
Summary
In this article, we have reviewed how you can write advanced macros to step through Rust code and modify it, thanks to Fold.
We have seen how the standard tooling available in the syn crate allows us to easily transform functions. It can, for example, change an occurrence of a panic
into an Err
. What it lacks is an elegant way to recursively step through the entire function, automatically executing a change in every applicable location.
The Fold and Visit traits, hidden behind feature flags, solve this limitation. Fold can manipulate the abstract syntax tree of an existing function, and is ideal for our use case. It has many methods — with useful, albeit basic, default implementations — that allow you to handle every occurrence of a given type. For example, the fold_macro
lets you manipulate every macro within your function. Moreover, the fold_stmt
method helped us step through the entire function’s content, changing every panic with minimal effort.