Month: May 2024
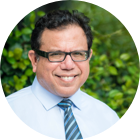
MMS • RSS
Posted on mongodb google news. Visit mongodb google news
Orion Portfolio Solutions LLC lessened its stake in shares of MongoDB, Inc. (NASDAQ:MDB – Free Report) by 7.2% in the 4th quarter, according to its most recent disclosure with the SEC. The institutional investor owned 5,789 shares of the company’s stock after selling 447 shares during the period. Orion Portfolio Solutions LLC’s holdings in MongoDB were worth $2,191,000 at the end of the most recent quarter.
Other institutional investors also recently bought and sold shares of the company. Blue Trust Inc. increased its holdings in MongoDB by 937.5% during the fourth quarter. Blue Trust Inc. now owns 83 shares of the company’s stock valued at $34,000 after buying an additional 75 shares during the period. Huntington National Bank increased its holdings in MongoDB by 279.3% during the third quarter. Huntington National Bank now owns 110 shares of the company’s stock valued at $38,000 after buying an additional 81 shares during the period. Parkside Financial Bank & Trust increased its holdings in MongoDB by 38.3% during the third quarter. Parkside Financial Bank & Trust now owns 130 shares of the company’s stock valued at $45,000 after buying an additional 36 shares during the period. Beacon Capital Management LLC increased its holdings in MongoDB by 1,111.1% during the fourth quarter. Beacon Capital Management LLC now owns 109 shares of the company’s stock valued at $45,000 after buying an additional 100 shares during the period. Finally, Raleigh Capital Management Inc. grew its holdings in MongoDB by 156.1% in the third quarter. Raleigh Capital Management Inc. now owns 146 shares of the company’s stock worth $50,000 after purchasing an additional 89 shares during the last quarter. 89.29% of the stock is owned by hedge funds and other institutional investors.
Insiders Place Their Bets
In other news, CAO Thomas Bull sold 170 shares of the business’s stock in a transaction dated Tuesday, April 2nd. The shares were sold at an average price of $348.12, for a total transaction of $59,180.40. Following the completion of the sale, the chief accounting officer now owns 17,360 shares of the company’s stock, valued at $6,043,363.20. The transaction was disclosed in a filing with the Securities & Exchange Commission, which can be accessed through the SEC website. In other news, CAO Thomas Bull sold 170 shares of the business’s stock in a transaction dated Tuesday, April 2nd. The shares were sold at an average price of $348.12, for a total transaction of $59,180.40. Following the completion of the sale, the chief accounting officer now owns 17,360 shares of the company’s stock, valued at $6,043,363.20. The transaction was disclosed in a filing with the Securities & Exchange Commission, which can be accessed through the SEC website. Also, CEO Dev Ittycheria sold 17,160 shares of the business’s stock in a transaction dated Tuesday, April 2nd. The stock was sold at an average price of $348.11, for a total transaction of $5,973,567.60. Following the sale, the chief executive officer now directly owns 226,073 shares of the company’s stock, valued at approximately $78,698,272.03. The disclosure for this sale can be found here. Over the last quarter, insiders sold 46,802 shares of company stock worth $16,514,071. Company insiders own 3.60% of the company’s stock.
Wall Street Analyst Weigh In
Several research firms have issued reports on MDB. Guggenheim lifted their price target on shares of MongoDB from $250.00 to $272.00 and gave the stock a “sell” rating in a report on Monday, March 4th. Redburn Atlantic reaffirmed a “sell” rating and issued a $295.00 price target (down from $410.00) on shares of MongoDB in a report on Tuesday, March 19th. Citigroup lifted their price target on shares of MongoDB from $515.00 to $550.00 and gave the stock a “buy” rating in a report on Wednesday, March 6th. Needham & Company LLC reaffirmed a “buy” rating and issued a $465.00 price target on shares of MongoDB in a report on Friday, May 3rd. Finally, Stifel Nicolaus reaffirmed a “buy” rating and issued a $435.00 price target on shares of MongoDB in a report on Thursday, March 14th. Two investment analysts have rated the stock with a sell rating, four have assigned a hold rating and twenty have given a buy rating to the company. Based on data from MarketBeat, MongoDB presently has a consensus rating of “Moderate Buy” and an average price target of $444.57.
Get Our Latest Analysis on MDB
MongoDB Trading Down 0.6 %
Shares of MongoDB stock opened at $333.99 on Thursday. The firm has a 50-day simple moving average of $356.71 and a two-hundred day simple moving average of $392.33. MongoDB, Inc. has a fifty-two week low of $275.76 and a fifty-two week high of $509.62. The company has a current ratio of 4.40, a quick ratio of 4.40 and a debt-to-equity ratio of 1.07.
MongoDB (NASDAQ:MDB – Get Free Report) last issued its quarterly earnings data on Thursday, March 7th. The company reported ($1.03) earnings per share for the quarter, missing analysts’ consensus estimates of ($0.71) by ($0.32). The firm had revenue of $458.00 million during the quarter, compared to analyst estimates of $431.99 million. MongoDB had a negative return on equity of 16.22% and a negative net margin of 10.49%. Equities research analysts forecast that MongoDB, Inc. will post -2.53 earnings per share for the current year.
MongoDB Company Profile
MongoDB, Inc, together with its subsidiaries, provides general purpose database platform worldwide. The company provides MongoDB Atlas, a hosted multi-cloud database-as-a-service solution; MongoDB Enterprise Advanced, a commercial database server for enterprise customers to run in the cloud, on-premises, or in a hybrid environment; and Community Server, a free-to-download version of its database, which includes the functionality that developers need to get started with MongoDB.
Featured Stories
Receive News & Ratings for MongoDB Daily – Enter your email address below to receive a concise daily summary of the latest news and analysts’ ratings for MongoDB and related companies with MarketBeat.com’s FREE daily email newsletter.
Article originally posted on mongodb google news. Visit mongodb google news
General Availability of Deployment Stacks for Azure Resource Management Replacing Blueprints
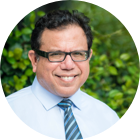
MMS • Steef-Jan Wiggers
Article originally posted on InfoQ. Visit InfoQ
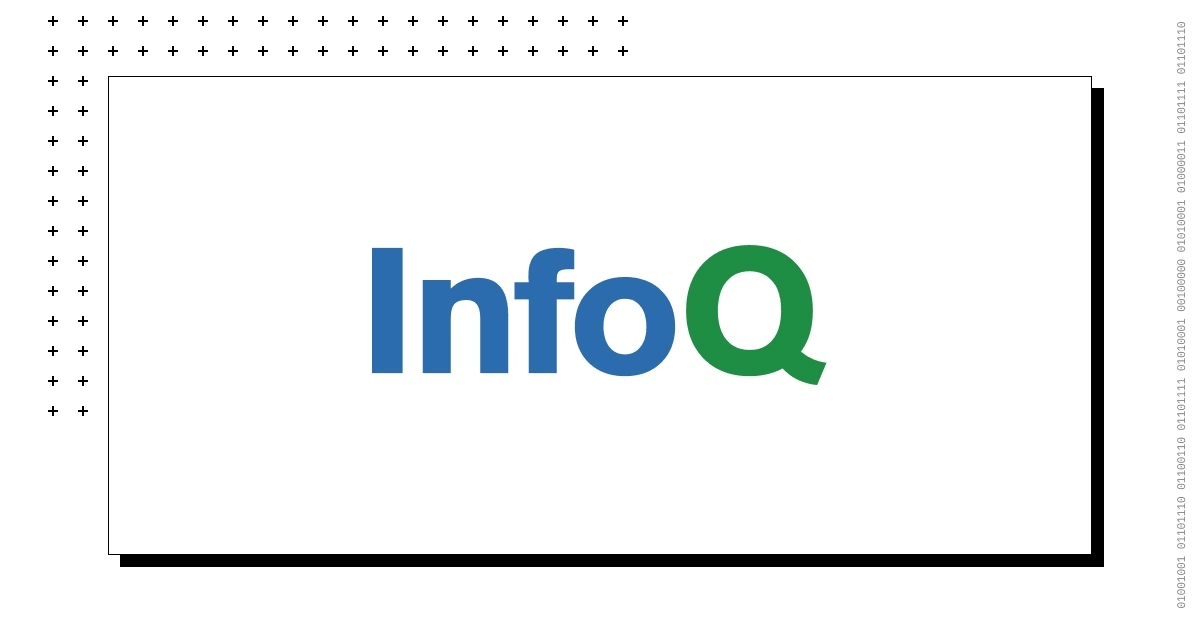
Deployment Stacks, a new resource type for managing a collection of Azure resources as a single unit, is now generally available (GA). It allows for faster updates and deletions while also preventing unwanted changes to those resources.
The GA release follows last year’s public preview and can be considered the follow-up of Azure Blueprints, which will be deprecated by July 2026 and never reached the GA status. The company states customers should migrate their blueprint definitions and assignments to Template Specs and Deployment Stacks.
Ryan Yates, a freelance consultant and former MVP, tweeted:
This has been a long time coming, but it’s good that it’s finally GA.
A deployment stack is a method of deploying ARM Templates or Bicep files, tracking resources in a “managedResources” list. Beyond the capabilities of conventional deployments, deployment stacks bring two main capabilities to Azure:
- “ActionOnUnmanage“: Specifies the action to take when a managed resource becomes unmanaged, either deleting or detaching the resource.
- “DeleteResources“: Deletes unmanaged resources, detaching Resource Groups and Management Groups.
Other settings include “DeleteAll,” “DetachAll,” “DenySettingsMode,” “DenyDelete,” “DenyWriteAndDelete,” and “None“.
A deployment stack can be created at different scopes, such as Resource Group, Subscription, and Management Group scope. To create a deployment stack, a user will need the following information:
- A main template, main.bicep or azuredeploy.json, that defines the “managedResources” to be created by the deployment stack. Think about which resources that share the same lifecycle can be defined into a deployment stack (e.g., networking resources, DevTest environments, Applications). For example, here is “mainAppInfra.bicep”:
- This file deploys a storage account and a virtual network to different resource groups.
targetScope = 'subscription'
param resourceGroupName1 string = 'testapp-storage'
param resourceGroupName2 string = 'testapp-network'
param resourceGroupLocation string = deployment().location
//Create Resource Groups
resource testrg1 'Microsoft.Resources/resourceGroups@2021-01-01' = {
name: resourceGroupName1
location: resourceGroupLocation
}
resource testrg2 'Microsoft.Resources/resourceGroups@2021-01-01' = {
name: resourceGroupName2
location: resourceGroupLocation
}
//Create Storage Accounts
module firstStorage 'multistorage.bicep' = if (resourceGroupName1 == 'testapp-storage') {
name: uniqueString(resourceGroupName1)
scope: testrg1
params: {
location: resourceGroupLocation
}
}
//Create Virtual Networks
module firstVnet 'multinetwork.bicep' = if (resourceGroupName2 == 'testapp-network') {
name: uniqueString(resourceGroupName2)
scope: testrg2
params: {
location: resourceGroupLocation
}
}
Example of mainAppInfra.bicep (Source: Microsoft Tech Community blog post)
- Next, users choose the “ActionOnUnmanage” setting of “DeleteResources,” “DeleteAll,” or “DetachAll.”
- Subsequently, the “DenySettingsMode” setting of “DenyDelete,” “DenyWriteAndDelete,” or “None.”
- And finally, the scope of the deployment stack and the target scope of its deployment.
An Azure CLI command to create a deployment stack at subscription scope would look as follows:
az stack sub create --name "DevTestEnvStack" --template-file "mainAppInfra.bicep" --location "westus2" --action-on-unmanage "deleteResources" --deny-settings-mode "denyDelete"
Removing a resource in the template and redeploying it with the same CLI Command results in a resource that is no longer being managed, which can be observed in the “deletedResources” array property of the deployment stack response. Beyond deciding on the behavior for “actionOnUnmanage,” users must also define what deny settings mode the deployment stack should use. This enables guardrails to help protect “managedResources” against unwanted changes.
Users can also view the Deployment Stack and its contents in the portal by navigating to the specified scope > settings > deployment stacks.
Google Cloud Platform (GCP) and Amazon Web Services (AWS) offer similar capabilities to Azure Deployment Stacks. With GCP, users can leverage Google Cloud Deployment Manager and Terraform as the primary tools for deploying and managing infrastructure. In contrast, with AWS, users have AWS CloudFormation, AWS CDK, and Terraform, which offer similar functionality. Note that Terraform is also an option for Azure, as it can be considered cloud platform agnostic.
Itamar Hirosh, a senior cloud Solution architect at Microsoft, concluded in a technical blog post on Deployment Stacks:
By leveraging Azure Deployment Stacks, we can effectively manage and secure our Terraform backend state storage accounts without falling into the trap of infinite state file loops. This approach not only centralizes the management of backend components but also adds an additional layer of security through deny assignments, preventing unauthorized access and modifications.
Lastly, besides the documentation, more details on Deployment Stacks are available on GitHub.
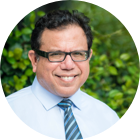
MMS • Jay Alammar
Article originally posted on InfoQ. Visit InfoQ
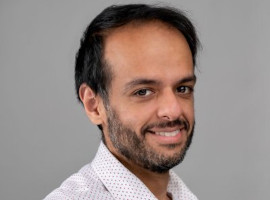
Transcript
Alammar: It is a delight to be sharing some ideas about what I find to be one of the most fascinating topics in maybe all of technology. What a time for what we call AI or language AI. It’s been a roller-coaster ride if you’ve been paying attention the last few years. This is a pattern that you can see, just in computing in general, every 10, 15 years, you have this new paradigm that opens up new ways of doing things. The rise of the personal computers, the creation of the web browser, the smartphones. Every one of these just creates new ways where people can communicate with computers and ways they can use them. That also changes definitions of jobs, entire industries as well. Now we stand at this era or time where this generative AI or AI in general, or possibly generative chat are this new mode that is probably going to be as big as these previous technological shifts. I even sometimes like to take a further step back and say, if you look at the human species, in general, jumps in language technology can be milestones for the species as a whole. You can point at the invention of writing, which is just this jump in human language technology. That’s technically what we call the beginning of history is this one jump that we use language in a specific way, and then that’s what we call history. Then, about 400, 500 years ago, the printing press, this device made it possible for so many people to have books. Before this invention, if you wanted a copy of a book, you needed to go and copy it yourself or pay somebody to copy it by hand. Once this way of automating books came around, you didn’t need to be rich anymore to be able to own a book. Then that opens the door for the next technological revolution, the renaissance. Pretty soon, we’re just launching Teslas into space within the span of a couple of years. I have talks from two years ago, where I say, this jump isn’t going to be as big as these two. We don’t really know, there’s a lot of hype. I would like to cut through the hype. Two years ago, we had a less than maybe 20% chance, it’s possible that this is going to be as big. Now I put it at less than 40% chance. It’s definitely going to change so many different things, but we’ll see if it lives up to this too.
Background
My name is Jay. I blogged for the last 9 or 10 years about machine learning, and language processing, and large language models. The most read article is The Illustrated Transformer that explains this neural network architecture that launched to this explosion in AI capability over the last few years. It has about 3 million page views or so. I’m the author of this upcoming O’Reilly book called “Hands-On Large Language Models.” Already you can find the first five or six chapters on the O’Reilly platform. I work at Cohere. Cohere is a large language model company, it’s one of the earliest large language model companies. I’ve been training these models for about four years. It was started by one of the co-authors of the transformer paper, Aidan Gomez. I joined because I was absolutely fascinated about this technology. I wanted to see how this cool machine learning trick would make its way into industry. How will it become products? How will it become features? That’s what we’re going to be sharing here, some of the things that I learned from what we see in Cohere and how people adopt these products. You’re definitely in the right place. This is the right time to start to think about how to build the intelligent systems of the future using technologies like these.
Useful Perspectives of Language AI
When I used to go and give talks two years ago, I had to introduce to people what a large language model is. That was not a common piece of software knowledge. Now, everywhere I go, developers or non-developers, everybody has interacted or had some sense of what is a chat model, what is a ChatGPT, what is a large language model that you can talk to. How we talk about them is a little bit different. Now what we try to do is to try to raise the awareness that, ok, you’ve interacted with a generative chat model, a large language model, we suggest that you don’t think of it as a black box, we suggest that you don’t think of it as a digital brain, regardless of how coherent and how well written it might be. The useful perspective we advise everybody, and developers specifically, is to think about it in terms of what can you build with this. What kinds of capabilities do these give you, or adds to your toolbox as a developer? How can you think about it more than just this box that you send some text in, and you get some text out. That’s what we’re going to be talking about. When we talk about the language side of it, you can talk about two major families of capabilities that these models bring to you. These can be language understanding capabilities. That’s the first group of capabilities that language models provide to you. It can also be language generation capabilities. That’s the other group. Even if you’re not dealing specifically with text generation, who here has Midjourney, or Sora for image generation, or Stable Diffusion for video generation. All of these models, even though they are generating things other than text, they have a language model embedded in them in the beginning of that flow, because you’re giving them a prompt, and that understanding of a prompt is the use of a language model for understanding, not necessarily for generation.
For your toolbox, I want to break this down further. Generative chat is only one of multiple things that you can do with generation. Text generation can be summarization, so taking a large article and providing a two or three sentence summary, is a generative task. Copywriting, if you tell the model to write me an email that says this, or write new variations of this type of text, that’s another generation text. These are in addition to generative chat. One of the main messages here is, don’t be limited to chat only. Everything you can do with generation can go into a lot of different generation tasks. A lot of them should probably be offline. You shouldn’t always lock yourself into, I’m going to be always thinking about online chat, right this very minute. Then, even when you think about these other non-chat generative capabilities, you’re only thinking about one of the things that AI can do. In fact, some of the most dramatic and most reliable use cases that AI has right now that has even progressed the last few years, are things like search, or text classification, or categorizations. Large language models, or language models, in general, small and large, are some of the best tools that we have for that. Think beyond just generating text. I think it’s a little bit unfortunate that this wave is being called generative AI, because some of the most robust applications are really more on the understanding, the representation, the search systems. We will talk about these and where their capabilities lie. This idea of search by meaning or using a language model for search is one of the most reliable applications of large language models. If you’ve tried to build with large language models, reliability becomes something that you care about a little bit because there’s so many cherry-picked examples that you’ve come across in social media. If you try to build that into a product, you realize that that works 6 out of 10 times, or 3 out of 10 times depending on that use case. Reliability is important when you think about robustness.
Semantic Search
When you think about search, don’t think only about building the next Google Search. Search is this major feature of every application that you interact with, every company needs to search their own internal documents. A lot of search systems are broken. They need to be improved. You can almost never find what you want. How language models can help here is with this idea of semantic search. Let’s take a query, for example. You want to search for Brazil to USA travel. Before language models, most search was based on keyword relevance. It will break it down and compare the keywords in the query to keywords in the document. If a system is not very well tuned, it might give you this article, which has all the same keywords, but the order of the two countries is flipped, and so that document really does not become a relevant document for that search. If you use language models for that search, they have a better ability to catch the intention and the context that you’re using these words for. That’s the idea of using language models for search. Two main concepts to think about for semantic search, for using language models for search, one is called dense retrieval. Who has built anything with embedding search, like a vector search database or Pinecone? That is what’s called dense retrieval. Then the other one is called reranking.
Let’s take a quick look at how those are. You have a query, you send it to a search system, in this case, a vector database, or it can be a database with vector search capabilities. There are features of Postgres, for example, that can do something like that. That vector database has access to a text archive, and then produces a number of search results. This is the search formula basically. Behind the scenes, how dense retrieval works is that it takes the query, it sends it to a language model that provides something called an embedding. Language models, you can have them generate text, but you can also have them generate another thing, which is a list of numbers that capture the meaning of that text. That’s what’s called an embedding vector. Here you have the query, you send it to the model, the model gives you maybe 300 numbers, like a vector size, that is a numeric representation that is useful for downstream software applications. Then you simply send that vector to this vector database, and it finds the nearest neighbors to that point, and those will tend to be the best search results for that query. This model has to be tuned for search specifically. You can’t just get any embedding models. An embedding model used for search has to be tuned in a specific way for search. There’s a step before that of chunking the text and embedding the archive beforehand, so that is step 0. If you’d like to play around with embeddings, we’ve open sourced, we’ve released the embeddings of all of Wikipedia. These are 250 million embedding vectors of Wikipedia in 300 languages. It’s the English but also every other Wikipedia out there. It’s on Hugging Face, you can download it and play around with it. You can build incredible things with it. That is dense retrieval.
The other idea is called reranking. Reranking is really the fastest way to inject the intelligence of a language model into a existing search system. It works, basically, in this two-step pipeline. You don’t have to remove your existing search system. If you’re using Elastic, or any other search system, that can be your first step of your search pipeline. That is the system that queries or searches your million or a billion documents, and gets you the top 100 results. Then those 100 results, we pass to a language model, and it just changes the order, it outputs the same 100. It says, this article that you told me is number 33, is actually the most relevant to this query, so I’m going to make it number one. This is the only one call at the end of that search pipeline that tends to dramatically improve the search system regardless, whether you’re using Elastic, whether you’re using embedding search, whether you’re using hybrid search of the two, just reranking just gives you that uplift very quickly. This is a language model that works in what’s called a cross-encoder fashion. To the language model, we present the query and the document, and it just outputs a score of relevance. Even if you’re familiar with embeddings, this works better than embeddings, because the language model at the time of scoring has access to all of the text of the query and of the document. It’s able to give a better result, because of that information that it has, while embeddings actually work on these very compressed spaces of the two vectors that can’t encode all the information in the text. That’s why reranking tends to work better than embeddings but it happens to be a second stage because it can’t operate on a million documents. You need a funnel to choose the top 10 or 100 results to get to. Here you get to see the uplift that you can get. These are three different datasets. The light one is keyword search, so that’s Elastic or BM25. The one next to it, this is search by embeddings. This is Elastic plus reranking. Whether you’re using keyword search or embedding search, a reranker is one of the fastest ways to improve the search system that you’re building with. The y axis here is accuracy. Who is familiar enough with what that means? What is search accuracy? That is very good, because I have a couple of slides on what that means. Because coming into this, I saw a lot of people wave hands about search accuracy, but I wanted a clear definition. We’ll get to that. These are two kinds of language models. These are not generative language models, but these are ways of using language models for search. Embeddings and reranking are these tools.
Search is Vital for the Future of Generation Models
Even if you’re interested in generation specifically, search is one of the most important things for the future of generation models. This is an example that showcases why that is. Let’s say you have two questions. You have a question where you send a question to a language model. Then you have another scenario where you send it the same question, but you actually give it some context, you maybe give it the answer, before you give it the question. Models would tend to answer number two better than number one, regardless of the question. If you give the model the answer plus the question, it will probably have a better answer for you. This is one of the first things that people first realized when these models came out. They called them search killers. People were asking these models questions and relying on how factual their information are. A lot of people got into trouble for trusting how coherent those models are, and ascribing authority to their information retrieval. Then that turned out to be a mistake. Everybody now knows about this problem called hallucinations. Models will tend to give you an answer, maybe they might be a little bit too confident about it. You should not rely on a language model, specifically, only a language model for factual retrieval, you need better tools. You need to augment it somehow. That is the method here. When you’re relying on the model for information, you’re relying on information that is stored within the parameters of the model, and so you’re always going to be using larger models. The information is always going to be outdated, because, how can you inject new information to the model? You have to train it for another nine months. While in this other paradigm, you actually augment the model with an external data source where you can inject the information at question time, whenever somebody asks you, the model is able to retrieve the information that is needed, and then that informs it. You get so many different benefits from this. You get much smaller models, because you’re not storing ridiculous amounts of information in this inefficient storage mechanism and loading them to GPU memory all the time. You can have up to date information, you can change it by the hour. You can give different users the ability to access different data sources. Even it gives you more freedom of what systems you can build with a system like this, but it also gives you explainable sources. Which documents did the model look at before it answered this question? That gives you a little bit more transparency into the behavior of the model. Then, that can also help you debug, did the problem come from the search step or from the generation step?
Advanced Retrieval-Augmented Generation (Query Rewriting, Tool Use, and Citations)
This is what retrieval-augmented generation is. It is this intersection of search and generation. It’s by far the highest in-demand use case that we see in industry and enterprises that we talk with. One way to look at it is like this command is the LLM we use at Cohere. You’re tying your LLM with a data source that is able to access and retrieve information from. This is one way of thinking. The conversation is not just with the model itself, but it’s with a model that is connected, that is grounded in a data source that is able to access that information. Now you have these two steps. Before the question is answered, it goes through a retrieval or a search step and then you go through a generation step. These are the two basic steps of the retrieval pipeline. Then another way of seeing it more clearly is you do a search step and then you get the top 3 documents, for example, or 10 documents. You put those in the prompt with the question. You present that to the language model. Then that’s how it’s answered. This is also the most basic formulas of RAG or retrieval-augmented generation. Then you get even better results if you have a reranker in the step, because if you’re only giving 10 documents and the 11th document is really the one that has the right information, but it’s beyond that cutoff line, the model is not going to be able to generate the right answer. The area where so many failures in RAG happen tend to be more in the retrieval side, prior to the generation step. Now you have these two language models in the retrieval step, in addition to the generation model in the generation step. That’s one of the first ways that you can build a RAG system.
One challenge is, after you build a system like this, and because you have in mind that people will ask direct questions that can be answered with one piece of information. You roll this into production. Then you find people really ask questions like this. They say, “We have an essay due tomorrow. We have to write about some animal. I love penguins. I could write about them. I could also write about dolphins. Are they animals? Maybe. Let’s do dolphins. Where do they live for example?” As a software engineer, is it fair for you to take this and throw it at Elastic and say, deal with this. It really is not. This is one of the first ways to improve initial RAG systems, which is to go through this step called query rewriting. This is an example of how that works. When a language model gets a question like this, you can write a query using a generative language model that says, to answer this question, this is the query that I need to search for. To answer this, it says, where do dolphins live? It’s just extracted that piece of information, and then that is what is relevant for that component, which is the search for the system. Now you have query rewriting, as this step with a generation model. You can do it with a language model with a prompt, but in the API that we built, we have a specific parameter for it that we optimize, but you can use it in different ways. This is how it works. It outputs the search query, and then you can throw it at your search system.
Now we have a text generation LLM in your retrieval step, doing that initial query rewriting step. We’ll take it a few steps further. We’re building up to the most advanced uses of LLMs, step by step. What about a question like this, if somebody says, compare the financial results of NVIDIA in 2020 versus 2023. You might get this query, and you might be able to find a document that already has the information about NVIDIA’s result in 2020 and 2023. There’s a very good chance that you will not find one single document that does this. One way to improve that is to say, actually, to answer this, I need two queries. I need to search for NVIDIA results 2020, and then 2023. These are two separate results. You get two separate sets of results and documents back. Then you synthesize them and present them to the model. This is what’s called multi-query RAG. Another way of looking at it is like this. You have the question, you send it to the language model, and then the language model says, I’m going to hit this data source. Where this data source can be whatever you want. It can be the web. It can be your Notion. It can be Slack. It can be whatever is relevant for you. Then the information is retrieved. Then you have this answering phase, what’s called a grounded generation. This is where you present the model with the documents in the context and it’s able to answer.
Another optimization for search is what if you build this search system and somebody says, hi, or hello? Do you need to really search your database for the model to answer hi, or hello, or something like that? You really don’t. When you’re doing this query rewriting, you can build that awareness in the language model to say, I need to search for this, or I need to search for these two queries, or I do not need to make a search to answer this question. Here you’re starting to see the model become a switch statement or an if else where the model is starting to be controlling the flow a little bit of how to deal with this. It has a couple of options, so it can either search or it can generate directly without search. As you get more greedy and comfortable with these new primitives of using language models, you start to say, if I can search one source, why can I not search multiple sources? Why can’t I give the model the ability to search my Notion, but also my ERP or my CRM, and later models start to build in these capabilities, where the model can route. It’s not necessarily tied to one data source.
This should give you a sense of, this is a new way of doing software. It’s really different when you think about these things. It can get a little bit more complex. Once you have a question like this, where you can say, who are the largest car manufacturers in 2023? Do they each make EVs or not? There’s probably not one document on the web, or anywhere that can answer this question. One thing the LLM can do is to say, first, I will search the web for the largest car manufacturers in 2020. I need one piece of information first. Then after it gets that piece of information, it says, now I need to search, Toyota electric vehicles, Volkswagen electric vehicles, Hyundai electric vehicles. The model is now continuing to control the flow of asking follow-up questions and making follow-up searches by itself before outputting the final result. This is what’s called multi-hop RAG. Where the model can make multiple jumps, and it’s determining, is this enough information or should I go and do something else to produce this final answer? Let’s take one last mental jump, to say, when you search the web or when you search Notion, you are invoking a function like this. You’re saying, I needed to search Notion, so call, search Notion, this function and give it these parameters. If you have models that are capable enough in doing something like this reliably and accurately enough, what is to prevent you from saying, not only do you search or retrieve information from Notion, how about you post this to Notion? The models are now able to just call APIs to actually do things, to inject information, to interact with the other systems and make these sorts of interactions.
Now here you’re starting to see this new thing emerge. Now we’re beyond what LLMs are. This is a new thing. This is the LLM-backed agent. These are some of the most futuristic things that you can see or think about in LLMs. It’s never too early, they will continue to get better. Then, now you’re having LLMs as the heart of a piece of software that is able to read and write from multiple sources, that is able to use tools. It can use the Python CLI. It can use calculators. It can use email. It can use other things. Now you have this piece of software that is a little bit more intelligent. This can be connected to different sources. You’re starting to see more of what it’s able to do. It’s an important piece of a place to invest in where things will go in the future. A lot of the agents that you see right now are maybe toys or just they show you the potential. This will take a few months or years to come through, but you can already see it showing its potential in solving problems. It’s just this extension of, if you made the jump from LLMs to RAG, and then from RAG to multi-step and multi-hop RAG, tool use is the next abstraction because you’re using the same thing. Instead of the search engine being your tool, it’s another system or another API or another piece of software.
Then, we also always advocate for citations. If you can have a system that provides the citations for which spans in the text refer to which documents. We need to give our users the ability to verify the models up, to not completely trust it. Citations as a feature is highly recommended in building these systems. On the Cohere side, we’ve built this series of models, Command R and Command R+. We’ve released the weights. You can download them, you can run them on your laptop. They’re super optimized for RAG and for all of these use cases that we talked about. They’re on Hugging Face. If you have the GPUs, you can probably run them on CPUs as well, but you need a little bit of memory. You can download the quantized models. If you search Cohere on Hugging Face, you can download these models and start playing with all of these ideas of RAG, multi-step, multi-hop, and the final one, tool use. Developers love it. Now you have a shape like this where you have retrieval that has three language models in it: there’s an embedding language model, there’s a reranking language model, and there is a generation language model. You know why that generation model is in the search step. Then you have this generation step that does this grounded generation, hopefully, with citations that will be used.
Evaluation
Last note on evaluation. We talked about accuracy. This is from one of the chapters of the upcoming book. Let’s talk about this accuracy metric of search. You have one query. You have two systems that you want to compare the quality of. From each one, you will get three results. Let’s say you have this query, and then search system 1 gives you these results where number one is relevant, two is not relevant, and three is irrelevant, so two out of three. Search system number 2 gives you two non-relevant results and one relevant one. Who says that search system 1 is better? Who says that system 2 is better? Search system 1 got two out of three correct, so that’s maybe a two out of three accuracy for this query, while one got one out of three accuracy. Search system 1 is actually better. That’s one metric of evaluating search, which is accuracy. Another one is like this, what about both of them got you one, but one out of the three as relevant. The first system placed it right at the top, it said, this is number one. The second system said, this is number three. Who says system 1 is better? Who says system 2 is better? System 1 is better, because the relevant response is higher rated. There’s another span of metrics. What this assumes is that you have this, that you have a test suite for your data. If you’re thinking about building RAG systems, or evaluating search systems for your use case, it’s good for you to develop something like this internally, which is, you have your documents. Let’s say you have a set of queries that are relevant for them. Then you have these relevance judgments of, is this document relevant for this query or not, for all of them? This is how you would tend to evaluate at least the retrieval step. For end-to-end RAG, there are other methods. This is one way. You can use language models to actually generate the queries for that. I hope by now you can think about them as just these multi problem-solving tools. This builds up to this metric called mean average precision, which is one of the metrics that you can use for search that thinks about the scores and the ranking.
Summary
If I want you to take away two things out of this, one, large language models are not this black box of text in, text out. They are a collection of tools in your grasp. We talked about so many of these ways to make them useful for embedding, for search, for classification, for retrieval, for generation, for query rewriting. Think of them as just this new class of software that you’re able to build with. Then this idea of this new form that they’re taking, this new also class of software, which is the LLM-backed agent that is able to communicate with external data sources and tools, and use them a little bit more successfully and in successive steps. We have a guide on how to build something that plugs all of these tools together. You can just search RAG chatbot on the blog, https://txt.cohere.com/rag-chatbot. We have a resource called LLM.University, where we have about seven or eight modules about lessons, very highly accessible. Very visual, with videos in a lot of them. It’s completely free.
Best Practices and Pitfalls of Building Enterprise Grade RAG Systems
Luu: Given your experience in all these areas and working on RAGs and such, it’s not easy to build RAG based systems at an enterprise grade level. What have you seen as some of the best practices and pitfalls that companies or folks have run into?
Alammar: The earlier you invest, the better, because there is definitely a lot of learning that goes into building these systems. Having the people who experiment with these internally and realize very quickly or very early their failure points, is important to build continually. A few concrete things are, yes, embeddings are great. It’s probably even better to have a hybrid search system where you’re doing both keyword and embeddings at the same time. You shouldn’t just rely on one of these methods. Then with all search systems, you can also inject other signals into that that would be relevant. I really love software testing in, let’s say, unit tests. I think we should do a lot more of that in machine learning. If you have some of that as a cache of tests that are relevant for you as behaviors, because even when you’re using managed language models, their behavior might change with the next version, and the next version. You need to bring these solid software methodologies of doing. Can you catch a regression in one behavior or another? Building those solid software engineering practices into machine learning is something that people in AI should be doing a lot.
Questions and Answers
Participant 1: The multi-hop RAG, do you think that it’s relevant to incorporate that into the first classification call that you talked about, the rewrite plus the skip? Because it sounds very beefy for the model to understand that actually a flow is needed. Would you separate that out? How would you tackle that?
Alammar: In machine learning, most answers are, it depends. If you’re doing a highly latency sensitive system, the query rewriting needs to be the fastest that you can be. I think you should just measure how latency sensitive the use case is, and then go on that. If it’s highly latency sensitive, you might go with a smaller model for the first step. Then for the multi-step, you call it when you need it. The entire ethos of this is to use the best tool for each thing and not throw everything at a massive model that can do everything, but can do it very expensively, it will need so many GPUs. Yes, always advocating using the smallest type of model that is capable to solve your task.
Participant 2: What’s your favorite framework? Because there’s quite a few frameworks out there at the moment, and I’m going from one to the other to the other making forks everywhere.
Alammar: I’m always trying different frameworks. I cannot necessarily recommend anyone right now. We’re working a little bit with LangChain, because LangChain started soon, and it just makes it convenient for people to get started with it. I also like to do a lot of my work in just Python strings and just call the APIs and build those things. The frameworks might just make it easier for you to download a template, play around with it, see where it breaks. Don’t always think that it will take you all the way to production, because it will not always do that. As long as it helped you get your hands dirty and play with. Very convenient to learn with. It’s still very volatile. There’s so much development very quickly that is happening. I wouldn’t say there’s major dominant ones. LangChain has made its name, but there continues to be newer frameworks that specialize in the different use cases.
Participant 3: I have a question on definitions. One year ago, when I learned about language models, it was quite mentally easy for me to picture that, they were taught with text messages and were able to answer questions, and so on. Nowadays, there’s these agents and all that control logic that you were also talking about, about splitting questions and so on. What’s your definition of a language model? Does it include all that control logic, and stuff like that? What do you think?
Alammar: That is a great thing. Because even before large language models came, language model is a very well-defined system in statistics, which is a system that is able to predict the next word successfully. That is the language modeling objective of even how these models are created in the first step. What we found out recently is that if you train a large enough language model and a large enough and clean enough dataset, it captures behaviors that really surprise you. If you’ve trained it on a dataset that has a lot of factual information, the model will not only pick up language, it will pick up facts. If you train it on a lot of code and give it the right tokenization, it’s going to be able to generate code. It becomes these new capabilities based on how you train it and how you clean the data and how you optimize for it. It becomes a general problem-solving piece of software that is able to stretch the imagination and do more things than just generate coherent language or text. The nature of language models is fascinating.
Participant 4: You mentioned pitfalls, especially when people are initially playing with these kinds of systems. What’s one that you think you would call out that people could keep eyes open before they play? What’s the one that you see as most common to people, pitfall or limitation that it would be nice to know about beforehand?
Alammar: Overly trusting the model. This is a punishment that we get by making models that are so good. Once models became not gibberish creators, people started to give them more authority than they should. That nature of it is a little different. That trust in companies or in people who are new in making applications for it, can say, ok, I can interact with this system on this website. Let me take that same system and make it a chatbot on my public website to talk with the world. Over-trusting in these probabilistic systems without guardrails, without the domain knowledge. Cybersecurity gives you a lot of paranoia, because you need to think about the different ways where your system can be broken. I think we need a lot more of that in deployment of large language models.
Participant 5: These models are probabilistic. They’re not deterministic. If that’s the case, would you ever put them closer to your systems of record with that diagram you showed of them interacting with the world and more APIs and plugins? Would you ever put them closer to systems of record? How long before you think that’s possible, or feasible?
Alammar: Yes, it depends on the use case, really. There are a lot of use cases where they are ready now. If you’re doing things that are, for example, recommendations to an expert that can spot the failure modes, or if you have the correct guardrails. It’s supposed to output to JSON, can you check, is it valid JSON or not? The more you build these actual practices of that domain, yes, they can solve a lot of problems right now. Then the more high risk the use case is the more care and attention that needs to be there. The more we need to think about humans in the loop, and safe deployment options. It depends really on the use case. We know a lot of tools that increase the probability of success. There are things like majority voting, for example. We have a model up with the response three or five times and seeing, do they agree with each other or not? There are tools of improving that. You as the creator have the responsibility of setting that threshold and having enough of guardrails to make sure that you get the response and the result and the behavior that your system needs.
See more presentations with transcripts
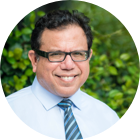
MMS • Edin Kapic
Article originally posted on InfoQ. Visit InfoQ
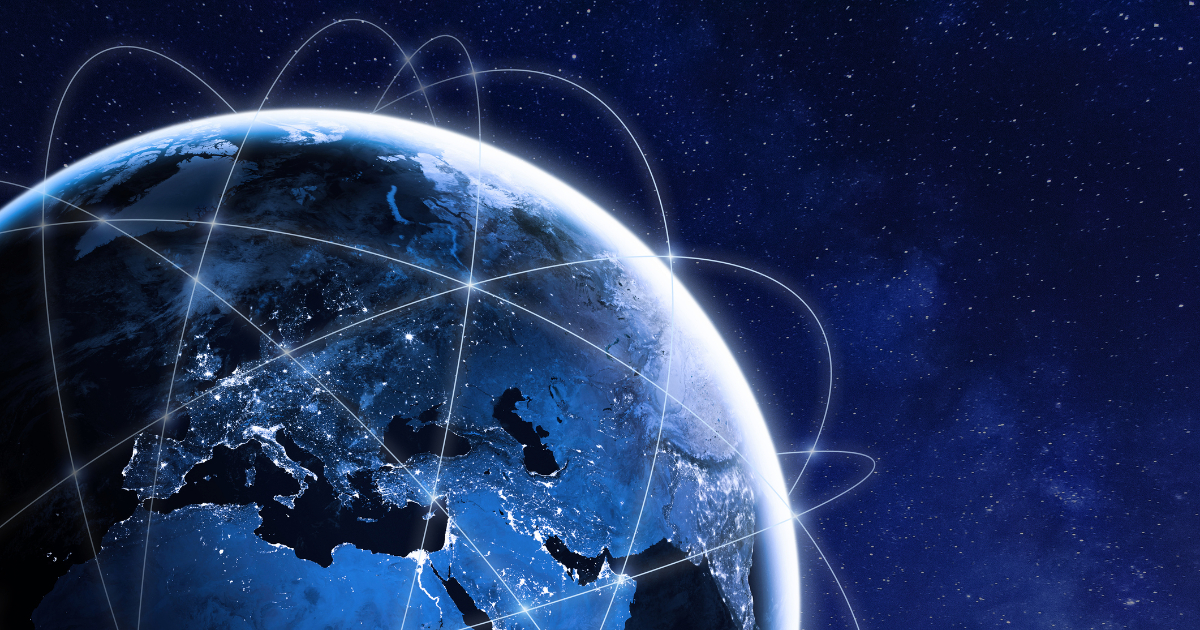
The latest version of OpenSilver 2.2, a remake of Microsoft’s retired Silverlight web application framework, includes support for migrating applications created with Visual Studio LightSwitch that targeted Silverlight.
OpenSilver was launched in October 2021 by a French company, Userware. It is an open-source, MIT-licensed reimplementation of Silverlight. OpenSilver compiles .NET and XAML code into HTML5 and WebAssembly, reimplementing standard and selected third-party controls. It allows developers to reuse instead of rewriting their legacy Silverlight or XAML applications. The latest update to OpenSilver was version 2.1 in February 2024, with the support for F# language.
Visual Studio LightSwitch was a framework for the rapid creation of business data-centric applications with visual drag-and-drop designers and support for C# and XAML. It was programmed with data-access abstraction via Entities and used the Model-View-ViewModel (MVVM) paradigm for the UI. It would generate a Silverlight application as an output. With the demise of Silverlight, the only available alternative for developers would be to rewrite their existing LightSwitch applications.
OpenSilver 2.2 can now migrate LightSwitch applications without changing the code. The generated files for the LightSwitch project can be imported into an empty OpenSilver project. By referencing a NuGet package called “LightSwitch Compatibility Pack”, the original application files will be compiled into static HTML and JS files. This NuGet package is licensed, and the developers can sign up for a trial.
To illustrate the migration, Userware migrated a sample LightSwitch application to the OpenSilver version that can be run in the browser without a Silverlight plugin. The source code for both applications is available on GitHub.
For the moment, the migration doesn’t allow the modification of screens created by LigthSwitch. The developers have to make the change in LightSwitch and import the application files again into the OpenSilver project. The interactive editor is on the OpenSilver roadmap.
The reactions from developers have been generally positive. On Reddit, a corporate user called Yukti_Solutions says that “massive selling points are the cost savings plus the fact that migrated apps still work and look the same”.
The OpenSilver 2.2 press release states that the roadmap will include a WYSIWYG XAML designer with full drag-and-drop support, as current support is limited to rendering the XAML in the Forms Designer. Future support for .NET MAUI, XAML Hot Reload, macOS, VS Code and Rider are mentioned, too.
The OpenSilver source code is available on GitHub. The repository containing OpenSilver has 928 stars and has been forked 115 times. Beyond the Userware developer team, there are other active contributions to the project, with a total of 48 contributors. According to the OpenSilver website, companies that rely on this framework include Bayer, TATA, KPMG, and others.
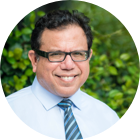
MMS • RSS
Posted on mongodb google news. Visit mongodb google news
MongoDB, Inc. (NASDAQ:MDB – Get Free Report) was the recipient of unusually large options trading activity on Wednesday. Investors bought 23,831 put options on the company. This represents an increase of 2,157% compared to the typical volume of 1,056 put options.
Insider Transactions at MongoDB
In other MongoDB news, CRO Cedric Pech sold 1,430 shares of the business’s stock in a transaction on Tuesday, April 2nd. The shares were sold at an average price of $348.11, for a total transaction of $497,797.30. Following the completion of the sale, the executive now directly owns 45,444 shares in the company, valued at approximately $15,819,510.84. The sale was disclosed in a document filed with the SEC, which is available through this link. In other news, Director Dwight A. Merriman sold 6,000 shares of the business’s stock in a transaction on Friday, May 3rd. The shares were sold at an average price of $374.95, for a total transaction of $2,249,700.00. Following the completion of the sale, the director now owns 1,148,784 shares of the company’s stock, valued at approximately $430,736,560.80. The sale was disclosed in a legal filing with the SEC, which is available at this hyperlink. Also, CRO Cedric Pech sold 1,430 shares of the firm’s stock in a transaction on Tuesday, April 2nd. The stock was sold at an average price of $348.11, for a total value of $497,797.30. Following the completion of the transaction, the executive now directly owns 45,444 shares in the company, valued at $15,819,510.84. The disclosure for this sale can be found here. Insiders sold 46,802 shares of company stock worth $16,514,071 over the last three months. 3.60% of the stock is currently owned by company insiders.
Institutional Investors Weigh In On MongoDB
Several institutional investors have recently bought and sold shares of the company. Norges Bank purchased a new position in shares of MongoDB in the fourth quarter worth about $326,237,000. Jennison Associates LLC lifted its stake in MongoDB by 14.3% in the 1st quarter. Jennison Associates LLC now owns 4,408,424 shares of the company’s stock worth $1,581,037,000 after purchasing an additional 551,567 shares in the last quarter. Axiom Investors LLC DE acquired a new position in shares of MongoDB during the 4th quarter worth approximately $153,990,000. Swedbank AB acquired a new stake in shares of MongoDB in the first quarter valued at approximately $91,915,000. Finally, Clearbridge Investments LLC lifted its position in MongoDB by 109.0% during the first quarter. Clearbridge Investments LLC now owns 445,084 shares of the company’s stock worth $159,625,000 after buying an additional 232,101 shares in the last quarter. 89.29% of the stock is currently owned by institutional investors and hedge funds.
MongoDB Stock Performance
MongoDB stock traded down $19.70 during mid-day trading on Thursday, hitting $314.29. The company had a trading volume of 2,015,488 shares, compared to its average volume of 1,306,049. MongoDB has a 12-month low of $275.76 and a 12-month high of $509.62. The company’s fifty day moving average is $356.71 and its 200-day moving average is $392.33. The company has a current ratio of 4.40, a quick ratio of 4.40 and a debt-to-equity ratio of 1.07.
MongoDB (NASDAQ:MDB – Get Free Report) last posted its earnings results on Thursday, March 7th. The company reported ($1.03) EPS for the quarter, missing analysts’ consensus estimates of ($0.71) by ($0.32). MongoDB had a negative net margin of 10.49% and a negative return on equity of 16.22%. The business had revenue of $458.00 million for the quarter, compared to analyst estimates of $431.99 million. On average, research analysts expect that MongoDB will post -2.53 earnings per share for the current year.
Wall Street Analyst Weigh In
A number of research firms recently weighed in on MDB. Truist Financial increased their price objective on shares of MongoDB from $440.00 to $500.00 and gave the stock a “buy” rating in a research report on Tuesday, February 20th. Monness Crespi & Hardt raised shares of MongoDB to a “hold” rating in a research note on Tuesday. Loop Capital initiated coverage on shares of MongoDB in a report on Tuesday, April 23rd. They issued a “buy” rating and a $415.00 price target on the stock. Tigress Financial lifted their price objective on shares of MongoDB from $495.00 to $500.00 and gave the company a “buy” rating in a report on Thursday, March 28th. Finally, Redburn Atlantic restated a “sell” rating and issued a $295.00 target price (down from $410.00) on shares of MongoDB in a research note on Tuesday, March 19th. Two research analysts have rated the stock with a sell rating, four have assigned a hold rating and twenty have issued a buy rating to the company’s stock. Based on data from MarketBeat, the stock presently has a consensus rating of “Moderate Buy” and an average price target of $444.57.
View Our Latest Stock Analysis on MongoDB
About MongoDB
MongoDB, Inc, together with its subsidiaries, provides general purpose database platform worldwide. The company provides MongoDB Atlas, a hosted multi-cloud database-as-a-service solution; MongoDB Enterprise Advanced, a commercial database server for enterprise customers to run in the cloud, on-premises, or in a hybrid environment; and Community Server, a free-to-download version of its database, which includes the functionality that developers need to get started with MongoDB.
Featured Stories
Before you consider MongoDB, you’ll want to hear this.
MarketBeat keeps track of Wall Street’s top-rated and best performing research analysts and the stocks they recommend to their clients on a daily basis. MarketBeat has identified the five stocks that top analysts are quietly whispering to their clients to buy now before the broader market catches on… and MongoDB wasn’t on the list.
While MongoDB currently has a “Moderate Buy” rating among analysts, top-rated analysts believe these five stocks are better buys.
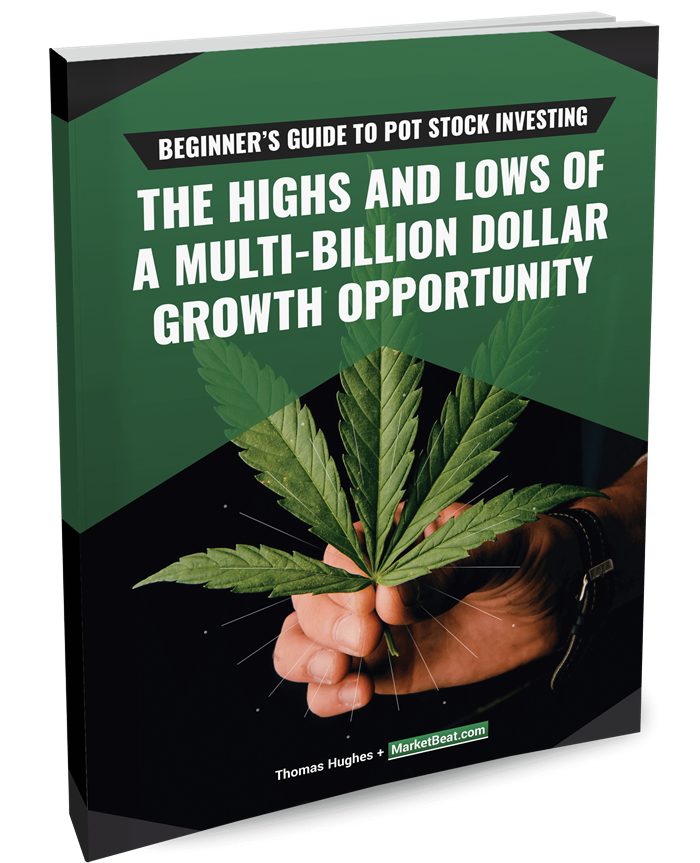
Click the link below and we’ll send you MarketBeat’s guide to pot stock investing and which pot companies show the most promise.
Article originally posted on mongodb google news. Visit mongodb google news
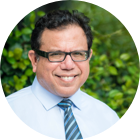
MMS • RSS
Posted on mongodb google news. Visit mongodb google news
MongoDB, Inc. (NASDAQ:MDB – Get Free Report) was the recipient of unusually large options trading activity on Wednesday. Traders purchased 36,130 call options on the stock. This represents an increase of approximately 2,077% compared to the average volume of 1,660 call options.
Analyst Ratings Changes
A number of equities analysts have recently weighed in on MDB shares. Tigress Financial upped their price target on shares of MongoDB from $495.00 to $500.00 and gave the stock a “buy” rating in a research note on Thursday, March 28th. Bank of America cut their price target on MongoDB from $500.00 to $470.00 and set a “buy” rating for the company in a research note on Friday, May 17th. KeyCorp dropped their price objective on MongoDB from $490.00 to $440.00 and set an “overweight” rating on the stock in a report on Thursday, April 18th. Truist Financial lifted their target price on shares of MongoDB from $440.00 to $500.00 and gave the company a “buy” rating in a research note on Tuesday, February 20th. Finally, Loop Capital initiated coverage on shares of MongoDB in a research note on Tuesday, April 23rd. They set a “buy” rating and a $415.00 price target on the stock. Two analysts have rated the stock with a sell rating, three have given a hold rating and twenty have issued a buy rating to the stock. Based on data from MarketBeat.com, the company presently has an average rating of “Moderate Buy” and an average price target of $444.57.
Insiders Place Their Bets
In other news, Director Dwight A. Merriman sold 6,000 shares of the business’s stock in a transaction on Friday, May 3rd. The stock was sold at an average price of $374.95, for a total transaction of $2,249,700.00. Following the sale, the director now directly owns 1,148,784 shares of the company’s stock, valued at $430,736,560.80. The sale was disclosed in a legal filing with the Securities & Exchange Commission, which can be accessed through the SEC website. In other MongoDB news, Director Dwight A. Merriman sold 1,000 shares of MongoDB stock in a transaction that occurred on Monday, April 1st. The shares were sold at an average price of $363.01, for a total transaction of $363,010.00. Following the transaction, the director now directly owns 523,896 shares in the company, valued at approximately $190,179,486.96. The transaction was disclosed in a filing with the SEC, which is available at the SEC website. Also, Director Dwight A. Merriman sold 6,000 shares of the stock in a transaction on Friday, May 3rd. The shares were sold at an average price of $374.95, for a total value of $2,249,700.00. Following the completion of the sale, the director now owns 1,148,784 shares of the company’s stock, valued at approximately $430,736,560.80. The disclosure for this sale can be found here. Over the last 90 days, insiders have sold 46,802 shares of company stock worth $16,514,071. 3.60% of the stock is owned by insiders.
Institutional Trading of MongoDB
Hedge funds and other institutional investors have recently modified their holdings of the stock. Cetera Advisors LLC increased its position in MongoDB by 106.9% in the first quarter. Cetera Advisors LLC now owns 1,558 shares of the company’s stock worth $559,000 after purchasing an additional 805 shares during the period. Cetera Investment Advisers boosted its stake in shares of MongoDB by 327.6% in the 1st quarter. Cetera Investment Advisers now owns 10,873 shares of the company’s stock valued at $3,899,000 after purchasing an additional 8,330 shares during the last quarter. Atria Investments Inc grew its position in shares of MongoDB by 1.2% during the 1st quarter. Atria Investments Inc now owns 3,259 shares of the company’s stock worth $1,169,000 after buying an additional 39 shares during the period. Swedbank AB bought a new stake in shares of MongoDB during the first quarter worth approximately $91,915,000. Finally, LRI Investments LLC acquired a new position in MongoDB in the first quarter valued at approximately $106,000. Institutional investors and hedge funds own 89.29% of the company’s stock.
MongoDB Stock Down 0.6 %
Shares of NASDAQ:MDB opened at $333.99 on Thursday. The company has a market capitalization of $24.32 billion, a PE ratio of -134.67 and a beta of 1.19. The company has a debt-to-equity ratio of 1.07, a quick ratio of 4.40 and a current ratio of 4.40. The company’s fifty day moving average is $356.71 and its 200-day moving average is $392.33. MongoDB has a 52 week low of $275.76 and a 52 week high of $509.62.
MongoDB (NASDAQ:MDB – Get Free Report) last announced its earnings results on Thursday, March 7th. The company reported ($1.03) EPS for the quarter, missing the consensus estimate of ($0.71) by ($0.32). The business had revenue of $458.00 million during the quarter, compared to analyst estimates of $431.99 million. MongoDB had a negative return on equity of 16.22% and a negative net margin of 10.49%. Equities research analysts predict that MongoDB will post -2.53 earnings per share for the current fiscal year.
About MongoDB
MongoDB, Inc, together with its subsidiaries, provides general purpose database platform worldwide. The company provides MongoDB Atlas, a hosted multi-cloud database-as-a-service solution; MongoDB Enterprise Advanced, a commercial database server for enterprise customers to run in the cloud, on-premises, or in a hybrid environment; and Community Server, a free-to-download version of its database, which includes the functionality that developers need to get started with MongoDB.
Featured Articles
Receive News & Ratings for MongoDB Daily – Enter your email address below to receive a concise daily summary of the latest news and analysts’ ratings for MongoDB and related companies with MarketBeat.com’s FREE daily email newsletter.
Article originally posted on mongodb google news. Visit mongodb google news
Chicory – a WebAssembly Interpreter Written Purely in Java With Zero Native Dependencies
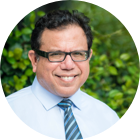
MMS • Olimpiu Pop
Article originally posted on InfoQ. Visit InfoQ
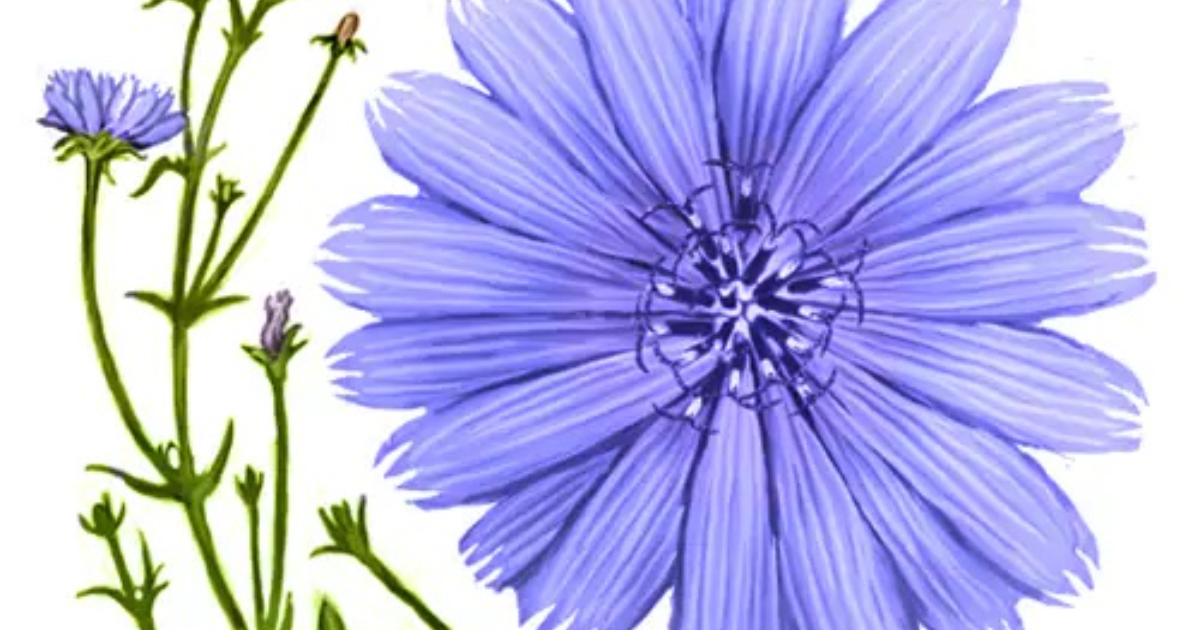
Recently, multiple languages have begun supporting compilation to WebAssembly (Wasm), allowing developers to build real polyglot systems. Chicory is a Wasm interpreter for the JVM with zero native dependencies and can run on any JVM. As wazero in the Go ecosystem, Chicory promises developers to safely interact with libraries written in any language supported by the Wasm ecosystem.
The initiators of the project, Benjamin Eckel, chief technical officer at Dylibso, and Andrea Peruffo, principal software engineer at RedHat, motivated their decision to build a WebAssembly interpreter on top of the JVM with the following statement:
Eckel & Peruffo: Wasm was born on the web, hence for safety reasons it has a sandboxed memory model which prevents modules from reading memory or jumping to code outside their scope. By default, Wasm will not allow you to access system resources such as files or networks. Also, it has no concepts like objects or heap meaning it can run low-level languages very efficiently. This makes Wasm an ideal runtime for running untrusted/third-party code written in various languages.
Chicory is similar to Graal’s WebAssembly implementation, except that it only requires a library as a jar, and doesn’t have native dependencies. Presumably, it should be possible to run it on any JVM, GraalVM included. Peruffo points to wazero as inspiration – Chicory’s “distant cousin from the Go ecosystem”.
To get started, just add the chicory dependency to a project, followed by loading the Wasm file and its instantiation:
import com.dylibso.chicory.runtime.ExportFunction;
import com.dylibso.chicory.wasm.types.Value;
import com.dylibso.chicory.runtime.Module;
import com.dylibso.chicory.runtime.Instance;
import java.io.File;
// point this to your path on disk
Module module = Module.builder(new File("./factorial.wasm")).build();
Instance instance = module.instantiate();
The Module
class, the module instance, is just the “inert” code while the Instance
class, the instance, is the Wasm virtual machine that loaded the code and can be run. Developers can invoke a function exported by the module.
ExportFunction iterFact = instance.export("iterFact");
Value result = iterFact.apply(Value.i32(5))[0];
System.out.println("Result: " + result.asInt()); // should print 120 (5!)
When coding, developers should be aware of a couple of the Wasm particularities: the methods might return multiple results and it supports just basic integer and float primitives. More complex types can be passed by transferring pointers. Using the low-level API, to provide a string, developers can do the following:
import com.dylibso.chicory.runtime.Memory;
Memory memory = instance.memory();
String message = "Hello, World!";
int len = message.getBytes().length;
// allocate {len} bytes of memory, this returns a pointer to that memory
int ptr = alloc.apply(Value.i32(len))[0].asInt();
// We can now write the message to the module's memory:
memory.writeString(ptr, message);
Chicory provides the Memory
class which allows developers to allocate, read and write different values to the Wasm program’s memory.
By default, Wasm programs are sandboxed (they cannot affect the “outside world”) and can’t do anything except compute. Developers can use a “native function” if required. The HostFunction
class, written in Java, is available to be called from the WebAssembly code. Peruffo pointed out that the use cases where Chicory could be used “are endless”: it was used to help with the JRuby distribution or even to “even to run Doom” on the JVM.
At this point, Chicory passes the WebAssembly test suite meaning that it can run “any correct Wasm project”. As pointed out by Peruffo, “This includes 100% of the V1 specification (but no SIMD support) on the happy path”. The next focus will be on making the runtime safe (incorrect programs will crash according to the spec) and later performant (JMH benchmarks were added to the project, to be able to spot any “performance” regressions).
Even if multiple Wasm runtimes are available, the project’s ambition is to become the “de facto” standard WebAssembly runtime for the JVM ecosystem. Other important milestones are “becoming production ready” before its first anniversary (September 2024) and “to be fast” and compatible by the end of 2024. These imply the creation of an AOT compiler that creates JVM bytecode and support for WebAssembly System Interface Preview 1(WASI), Single Instruction Multiple Data and Garbage Collection support. Those interested in contributing can interact via the project’s GitHub page or zulip chat.
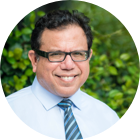
MMS • RSS
Posted on mongodb google news. Visit mongodb google news
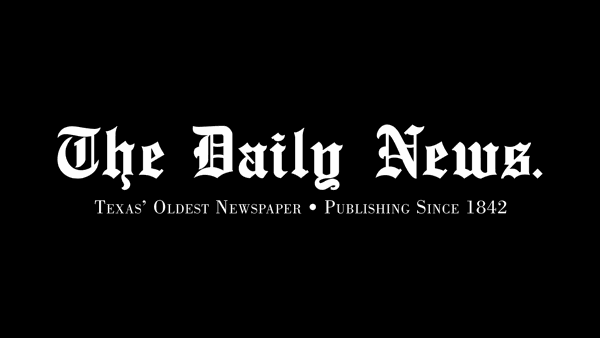
NEW YORK, May 29, 2024 /PRNewswire/ — MongoDB, Inc. (NASDAQ: MDB) today announced that it will present at two upcoming conferences: the Baird 2024 Global Consumer, Technology & Services Conference in New York, NY and the 44th Annual William Blair Growth Stock Conference in Chicago, IL.
- Chief Operating Officer and Chief Financial Officer, Michael Gordon, and Senior Vice President of Finance, Serge Tanjga, will present at the Baird Conference on Tuesday, June 4, 2024 at 1:25 PM Eastern Time.
- Mr. Gordon and Mr. Tanjga will present at the William Blair Conference on Wednesday, June 5, 2024 at 4:40 PM Central Time (5:40 PM Eastern Time).
Article originally posted on mongodb google news. Visit mongodb google news
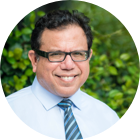
MMS • RSS
Posted on mongodb google news. Visit mongodb google news
Database software company MongoDB (MDB) will be reporting earnings tomorrow after market close. Here’s what you need to know.
MongoDB beat analysts’ revenue expectations by 5.2% last quarter, reporting revenues of $458 million, up 26.8% year on year. It was a weaker quarter for the company, with management forecasting growth to slow and underwhelming revenue guidance for the next quarter. It added 80 enterprise customers paying more than $100,000 annually to reach a total of 2,052.
Is MongoDB a buy or sell going into earnings? Read our full analysis here, it’s free.
This quarter, analysts are expecting MongoDB’s revenue to grow 19.4% year on year to $439.9 million, slowing from the 29% increase it recorded in the same quarter last year. Adjusted earnings are expected to come in at $0.37 per share.
The majority of analysts covering the company have reconfirmed their estimates over the last 30 days, suggesting they anticipate the business to stay the course heading into earnings. MongoDB has a history of exceeding Wall Street’s expectations, beating revenue estimates every single time over the past two years by 7.2% on average.
Looking at MongoDB’s peers in the data storage segment, some have already reported their Q1 results, giving us a hint as to what we can expect. Commvault Systems delivered year-on-year revenue growth of 9.7%, beating analysts’ expectations by 5.1%, and DigitalOcean reported revenues up 11.9%, topping estimates by 1.2%. Commvault Systems traded up 3.2% following the results while DigitalOcean was also up 15.7%.
Read our full analysis of Commvault Systems’s results here and DigitalOcean’s results here.
Investors in the data storage segment have had steady hands going into earnings, with share prices up 1.3% on average over the last month. MongoDB is down 8.1% during the same time and is heading into earnings with an average analyst price target of $444.4 (compared to the current share price of $335.56).
Unless you’ve been living under a rock, it should be obvious by now that generative AI is going to have a huge impact on how large corporations do business. While Nvidia and AMD are trading close to all-time highs, we prefer a lesser-known (but still profitable) semiconductor stock benefitting from the rise of AI. Click here to access our free report on our favorite semiconductor growth story.
Article originally posted on mongodb google news. Visit mongodb google news
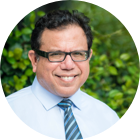
MMS • RSS
Posted on mongodb google news. Visit mongodb google news
NEW YORK, May 29, 2024 /PRNewswire/ — MongoDB, Inc. (NASDAQ: MDB) today announced that it will present at two upcoming conferences: the Baird 2024 Global Consumer, Technology & Services Conference in New York, NY and the 44th Annual William Blair Growth Stock Conference in Chicago, IL.
-
Chief Operating Officer and Chief Financial Officer, Michael Gordon, and Senior Vice President of Finance, Serge Tanjga, will present at the Baird Conference on Tuesday, June 4, 2024 at 1:25 PM Eastern Time.
-
Mr. Gordon and Mr. Tanjga will present at the William Blair Conference on Wednesday, June 5, 2024 at 4:40 PM Central Time (5:40 PM Eastern Time).
A live webcast of each presentation will be available on the Events page of the MongoDB investor relations website at https://investors.mongodb.com/news-events/events. A replay of the webcasts will also be available for a limited time.
About MongoDB
Headquartered in New York, MongoDB’s mission is to empower innovators to create, transform, and disrupt industries by unleashing the power of software and data. Built by developers, for developers, MongoDB’s developer data platform is a database with an integrated set of related services that allow development teams to address the growing requirements for today’s wide variety of modern applications, all in a unified and consistent user experience. MongoDB has tens of thousands of customers in over 100 countries. The MongoDB database platform has been downloaded hundreds of millions of times since 2007, and there have been millions of builders trained through MongoDB University courses. To learn more, visit mongodb.com.
Investor Relations
Brian Denyeau
ICR for MongoDB
646-277-1251
ir@mongodb.com
Media Relations
MongoDB
communications@mongodb.com
View original content to download multimedia:https://www.prnewswire.com/news-releases/mongodb-inc-to-present-at-the-baird-2024-global-consumer-technology–services-conference-and-the-44th-annual-william-blair-growth-stock-conference-302158593.html
SOURCE MongoDB, Inc.
Article originally posted on mongodb google news. Visit mongodb google news