Month: June 2024
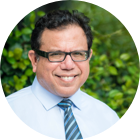
MMS • RSS
Posted on mongodb google news. Visit mongodb google news
MongoDB, Inc. (NASDAQ:MDB – Get Free Report) saw unusually large options trading on Wednesday. Stock traders bought 23,831 put options on the company. This is an increase of approximately 2,157% compared to the typical daily volume of 1,056 put options.
MongoDB Stock Performance
MDB stock traded up $3.63 during mid-day trading on Thursday, hitting $244.15. The company had a trading volume of 2,169,590 shares, compared to its average volume of 1,534,545. The firm has a market cap of $17.91 billion, a PE ratio of -85.59 and a beta of 1.13. The stock’s fifty day moving average price is $307.97 and its two-hundred day moving average price is $368.31. The company has a debt-to-equity ratio of 0.90, a quick ratio of 4.93 and a current ratio of 4.93. MongoDB has a 12 month low of $214.74 and a 12 month high of $509.62.
MongoDB (NASDAQ:MDB – Get Free Report) last issued its quarterly earnings data on Thursday, May 30th. The company reported ($0.80) earnings per share (EPS) for the quarter, hitting analysts’ consensus estimates of ($0.80). The company had revenue of $450.56 million for the quarter, compared to the consensus estimate of $438.44 million. MongoDB had a negative return on equity of 14.88% and a negative net margin of 11.50%. On average, analysts forecast that MongoDB will post -2.67 earnings per share for the current fiscal year.
Wall Street Analysts Forecast Growth
A number of analysts have recently weighed in on MDB shares. Oppenheimer reduced their price target on shares of MongoDB from $480.00 to $300.00 and set an “outperform” rating for the company in a research note on Friday, May 31st. JMP Securities dropped their price objective on MongoDB from $440.00 to $380.00 and set a “market outperform” rating on the stock in a research note on Friday, May 31st. Bank of America reduced their target price on shares of MongoDB from $500.00 to $470.00 and set a “buy” rating for the company in a report on Friday, May 17th. Canaccord Genuity Group cut their price objective on MongoDB from $435.00 to $325.00 and set a “buy” rating on the stock in a report on Friday, May 31st. Finally, Guggenheim raised MongoDB from a “sell” rating to a “neutral” rating in a research note on Monday, June 3rd. One research analyst has rated the stock with a sell rating, five have issued a hold rating, nineteen have issued a buy rating and one has assigned a strong buy rating to the stock. According to MarketBeat, MongoDB currently has a consensus rating of “Moderate Buy” and an average target price of $361.30.
Read Our Latest Stock Analysis on MongoDB
Insiders Place Their Bets
In related news, Director Dwight A. Merriman sold 1,000 shares of the firm’s stock in a transaction on Wednesday, May 1st. The stock was sold at an average price of $379.15, for a total transaction of $379,150.00. Following the transaction, the director now directly owns 522,896 shares in the company, valued at $198,256,018.40. The sale was disclosed in a document filed with the Securities & Exchange Commission, which is accessible through the SEC website. In other news, Director Dwight A. Merriman sold 6,000 shares of the firm’s stock in a transaction on Friday, May 3rd. The stock was sold at an average price of $374.95, for a total transaction of $2,249,700.00. Following the transaction, the director now directly owns 1,148,784 shares of the company’s stock, valued at $430,736,560.80. The sale was disclosed in a filing with the Securities & Exchange Commission, which is available through the SEC website. Also, Director Dwight A. Merriman sold 1,000 shares of MongoDB stock in a transaction dated Wednesday, May 1st. The shares were sold at an average price of $379.15, for a total transaction of $379,150.00. Following the sale, the director now owns 522,896 shares in the company, valued at $198,256,018.40. The disclosure for this sale can be found here. Insiders have sold a total of 59,976 shares of company stock valued at $19,525,973 in the last quarter. Insiders own 3.60% of the company’s stock.
Institutional Inflows and Outflows
Institutional investors and hedge funds have recently made changes to their positions in the company. Transcendent Capital Group LLC purchased a new position in MongoDB during the fourth quarter valued at $25,000. Blue Trust Inc. raised its holdings in MongoDB by 937.5% in the fourth quarter. Blue Trust Inc. now owns 83 shares of the company’s stock worth $34,000 after buying an additional 75 shares during the last quarter. YHB Investment Advisors Inc. bought a new position in shares of MongoDB in the first quarter worth approximately $41,000. Parkside Financial Bank & Trust increased its position in shares of MongoDB by 38.3% during the third quarter. Parkside Financial Bank & Trust now owns 130 shares of the company’s stock valued at $45,000 after acquiring an additional 36 shares in the last quarter. Finally, Beacon Capital Management LLC lifted its holdings in shares of MongoDB by 1,111.1% during the fourth quarter. Beacon Capital Management LLC now owns 109 shares of the company’s stock valued at $45,000 after purchasing an additional 100 shares in the last quarter. Hedge funds and other institutional investors own 89.29% of the company’s stock.
MongoDB Company Profile
MongoDB, Inc, together with its subsidiaries, provides general purpose database platform worldwide. The company provides MongoDB Atlas, a hosted multi-cloud database-as-a-service solution; MongoDB Enterprise Advanced, a commercial database server for enterprise customers to run in the cloud, on-premises, or in a hybrid environment; and Community Server, a free-to-download version of its database, which includes the functionality that developers need to get started with MongoDB.
Recommended Stories
Before you consider MongoDB, you’ll want to hear this.
MarketBeat keeps track of Wall Street’s top-rated and best performing research analysts and the stocks they recommend to their clients on a daily basis. MarketBeat has identified the five stocks that top analysts are quietly whispering to their clients to buy now before the broader market catches on… and MongoDB wasn’t on the list.
While MongoDB currently has a “Moderate Buy” rating among analysts, top-rated analysts believe these five stocks are better buys.
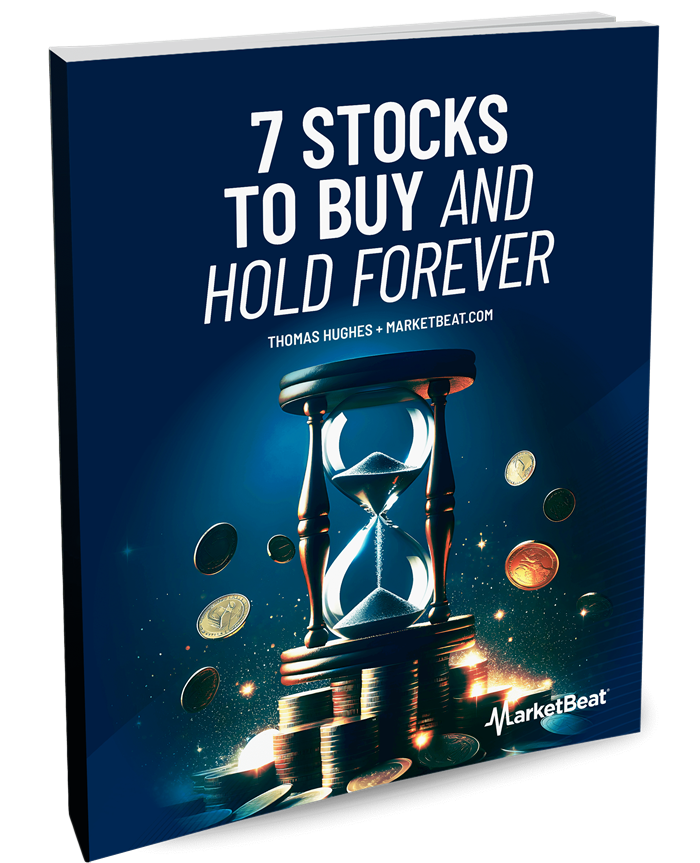
Click the link below and we’ll send you MarketBeat’s list of seven stocks and why their long-term outlooks are very promising.
Article originally posted on mongodb google news. Visit mongodb google news
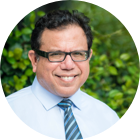
MMS • RSS
Posted on mongodb google news. Visit mongodb google news
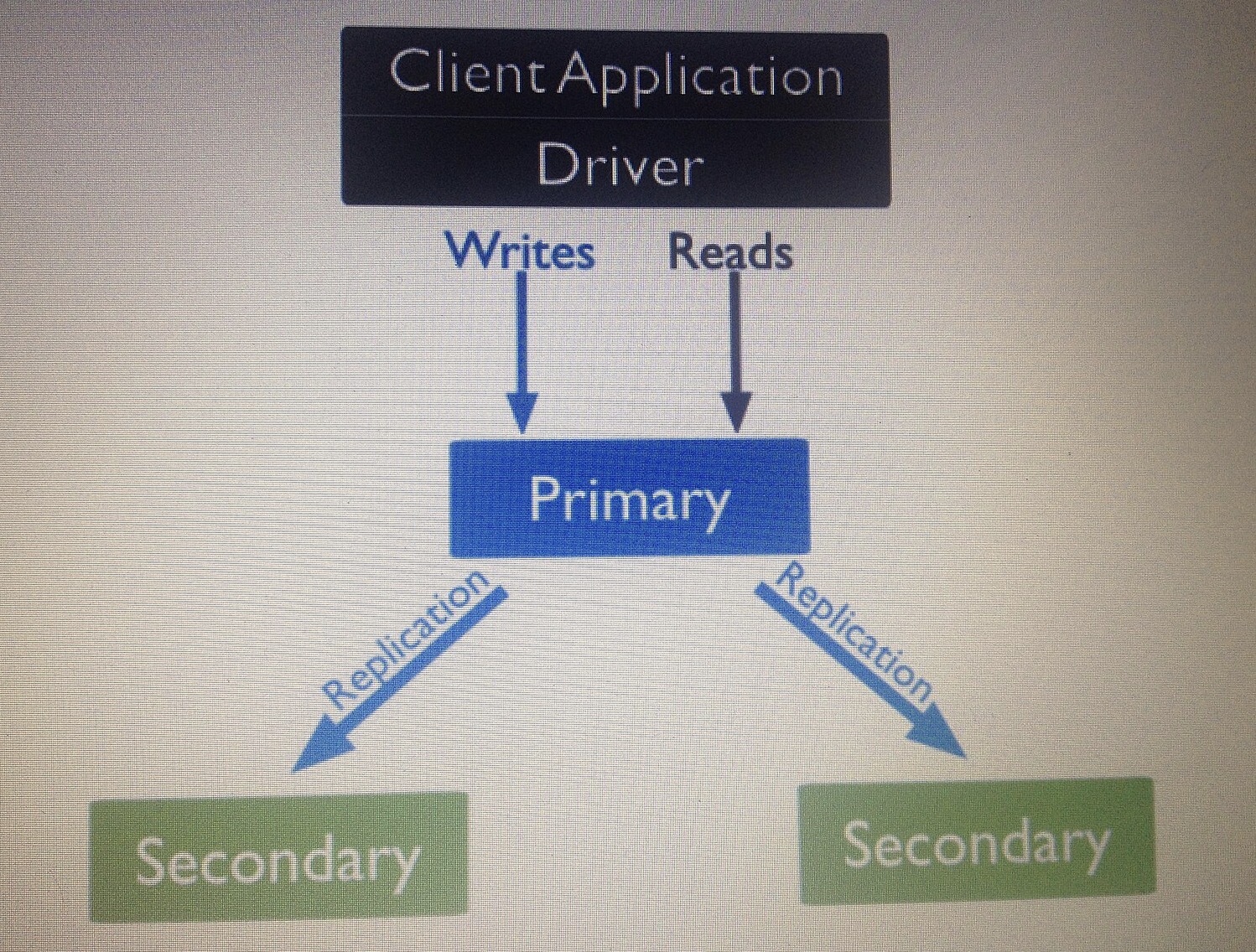
The mobile landscape mainly relies on efficient information retrieval. Users expect lightning-fast searches that deliver not just exact matches, but relevant results that understand the intent behind a query. MongoDB addresses this need with Atlas Vector Search, a powerful tool for developers to build intelligent search functionalities into their applications.
What is Atlas Vector Search?
Atlas Vector Search is a feature within the MongoDB Atlas database platform that allows developers to perform vector searches on their data. Unlike traditional keyword-based searches, vector searches delve deeper, analyzing the semantic meaning behind textual content. This is achieved by converting text into numerical representations called vectors. These vectors capture the relationships between words, enabling Atlas Vector Search to identify documents with similar meanings even if they don’t contain the exact keywords.
The Role of Large Language Models (LLMs)
Large language models (LLMs) are crucial in generating the vector representations that power Atlas Vector Search. An extensive look at large language models on MongoDB explains that these complex AI models are trained on massive amounts of text data. It allows them to capture the nuances of language and encode semantic relationships between words. Developers can then use pre-trained LLMs or fine-tune them for specific use cases within their mobile apps. For instance, an e-commerce app can refine an LLM to understand the particular terminology used in product descriptions and user queries related to fashion.
Integrating Atlas Vector Search into Mobile Apps
There are two primary approaches to integrate Atlas Vector Search into mobile applications:
- Server-Side Integration: In this approach, the mobile app sends search queries to a server that houses the Atlas Search engine. The server performs the vector search on the database and returns the most relevant results to the app. This method offers greater flexibility and computational power, particularly for complex search functionalities.
- Mobile SDKs: MongoDB offers mobile SDKs for popular frameworks like Flutter and React Native. These SDKs allow developers to embed vector search capabilities directly within the mobile app itself. This approach can be advantageous for scenarios where offline functionality or low latency is crucial.
For iOS development, Atlas Vector Search can integrate seamlessly with Apple’s SwiftUI framework, allowing developers to build intuitive and visually appealing search interfaces. SwiftUI’s declarative nature makes it easy to present search results in a dynamic and user-friendly manner.
Additionally, iOS developers can leverage the power of Core ML, Apple’s machine learning framework, to create custom LLMs tailored to their specific app’s needs. This combination of Atlas Vector Search, SwiftUI, and Core ML empowers iOS developers to craft mobile search experiences that are not only powerful but also aesthetically pleasing and in line with the native look and feel of the iOS platform. The result is powerful iPhone apps for purposes such as learning, shopping, and travel.
For Android, Atlas Vector Search integrates smoothly with Jetpack Compose, the modern UI toolkit for building Android apps. Jetpack Compose’s composable functions enable developers to construct dynamic and visually appealing search interfaces.
Android developers can also leverage TensorFlow Lite, a lightweight mobile version of the TensorFlow machine learning framework, to deploy custom LLMs directly on the device. This empowers offline search functionalities and reduces reliance on constant server communication. By combining Atlas Vector Search with Jetpack Compose and TensorFlow Lite, Android developers can create intelligent and user-centric search experiences that are performant even on devices with limited resources.
Considerations for Mobile Implementation
When using Atlas Vector Search for mobile, developers need to consider certain factors during implementation:
- Device Limitations: Mobile devices have resource constraints compared to servers. It’s essential to optimize vector search queries and data structures to ensure smooth performance on various devices.
- Data Privacy: When dealing with user data, security and privacy are paramount. Developers must adhere to best practices for data anonymization and encryption when working with vector representations.
- Offline Functionality: For situations where internet connectivity might be limited, developers can explore caching mechanisms to provide a baseline search experience even when offline.
Final Thoughts
Atlas Vector Search signifies a shift towards a more intelligent and user-centric approach to mobile search. With the power of vector representations and large language models, developers can build applications that not only understand user queries but also anticipate their needs. As mobile technology continues to evolve, Atlas Vector Search holds immense potential to shape the future of how users interact with information on their devices.
For more information on the latest tech news, gadget and apps, take a thorough look at our Technology Archives here on Explosion.
Article originally posted on mongodb google news. Visit mongodb google news
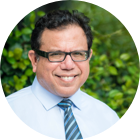
MMS • Ben Linders
Article originally posted on InfoQ. Visit InfoQ
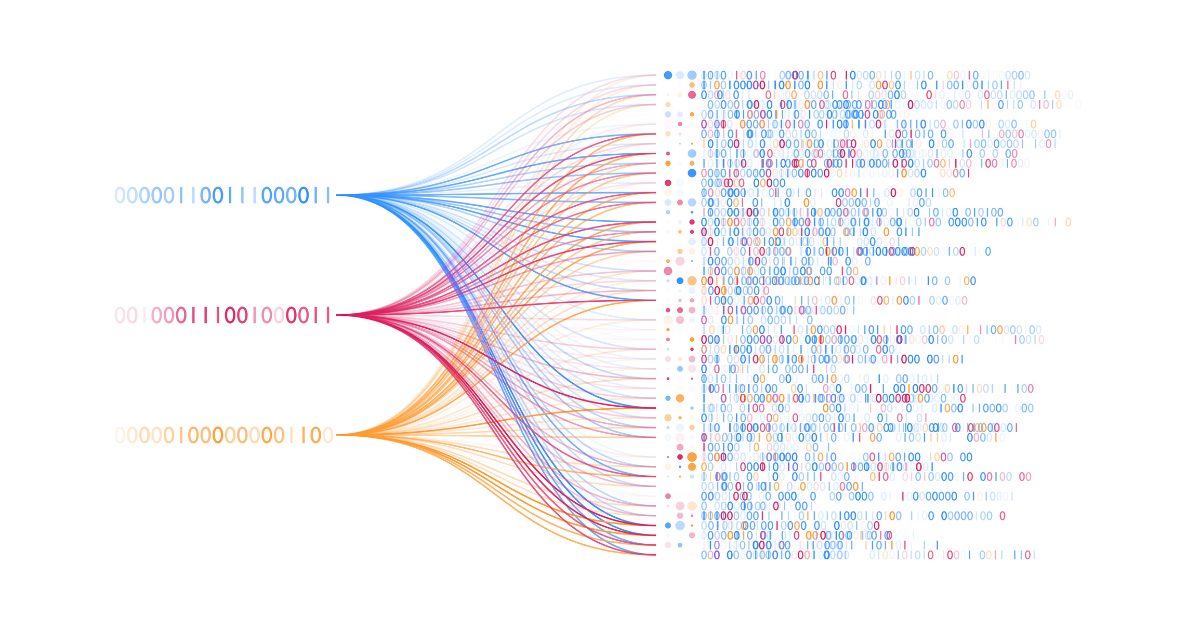
A challenge that companies often face when exploiting their data in data warehouses or data lakes is that ownership of analytical data is weak or non-existent, and quality can suffer as a result. A data mesh is an organizational paradigm shift in how companies create value from data where responsibilities go back into the hands of producers and consumers.
Matthias Patzak gave a talk about data mesh platforms at FlowCon France.
One of the biggest challenges that companies face when they want to exploit their data and become data-driven is the quality of the data they collect, as Patzak explained:
Have you ever heard the phrase “data is the new oil”? In the late 2000s, it was argued that all data should be stored because it is a valuable resource. But who trusts a 5-year-old S3 bucket when you don’t really know who stored what data and why?
Patzak argued that data is more like wine. Some data, like wine, must be consumed quickly or it will go bad. Other data, if stored and handled properly, can age very well and even increase in value and quality with age, he said.
The fundamental problem, Patzak mentioned, is that ownership of analytical data is often weak or non-existent, and quality suffers as a result. Analytical data is generated by transactional systems. However, the people who know and own these systems and the underlying processes are not responsible for the analytical application of their data, Patzak said. It is typically extracted, transformed, loaded into data warehouses or data lakes, and made available for use by a centralized, highly specialized department. These specialists often don’t have a real sense of ownership, either, he added.
A data mesh is a distributed data infrastructure that puts the responsibility for using and creating value from data back in the hands of the producers and consumers of that data, Patzak said. It eliminates the specialized data organization as a proxy and bottleneck in the communication between producers and consumers. At the heart of this distributed data infrastructure are data products that create tangible business value in their own right.
To build a data mesh, you’d create a domain-oriented architecture in which each business unit manages its data as a product, using a self-service infrastructure and tools for cataloging, sharing, and governance, as Patzak explained:
This self-service infrastructure is built by a data mesh platform and includes cloud services, data orchestration tools, and CI/CD pipelines, supported by federated governance policies for security and quality, and observability systems for monitoring.
Patzak mentioned that access is controlled by robust security mechanisms, and the entire data infrastructure is automated and maintained through Infrastructure as Code practices. Crucially, domain teams are equipped with the necessary skills through targeted enablement and training programs provided by the platform teams, ensuring that the technical setup facilitates a culture of autonomy, quality and collaboration, he added.
The benefits of a data mesh are faster implementation times and less cognitive load for producers and consumers, consistent tools, and standards for the company, Patzak concluded.
InfoQ interviewed Matthias Patzak about creating a data mesh platform.
InfoQ: What’s needed to create a data mesh platform and what benefits does a platform bring?
Matthias Patzak: From a technical point of view, everything is available to build the core services of a data mesh platform. This is just busy work. As with any platform, the challenge lies in ensuring that the platform services are accepted and used by the users. This is achieved by letting the platform users prioritize the platform backlog and by involving developers from the user teams in the development of platform services by means of job rotation.
InfoQ: What’s your advice to organizations that want to exploit their data using a data mesh?
Patzak: Don’t boil the ocean! Start small with a specific use case and pair of open minded producers and consumers and leverage the decentralised approach of data mesh. Even start before you are ready and become ready by starting. Finally, develop the platform in parallel with specific use cases.
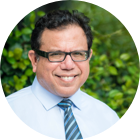
MMS • RSS
Posted on mongodb google news. Visit mongodb google news
Bendigo and Adelaide Bank, based in Australia, has partnered with MongoDB to modernise its core banking technology with MongoDB Atlas as the keystone of an ambitious application modernisation initiative.
During the initiative, the bank reduced the development time required to migrate a core banking application off of a legacy relational database to MongoDB Atlas by up to 90%.
Bendigo and Adelaide Bank migrated onto MongoDB Atlas at one-tenth of the cost of a traditional legacy-to-cloud migration.
Also, the bank automated repetitive developer tasks with new AI tooling in order to accelerate developers’ pace of innovation. For example, AI-powered automations reduced time spent running application test cases from over 80 hours to just five minutes.
In less than three months, the bank modernised its agent delivery system (ADS), a legacy retail banking application, on MongoDB Atlas with the help of MongoDB Relational Migrator and generative AI-assisted modernization tools.
ADS is a retail teller application for the bank’s agent branches, and is used in communities where digital banking functionality is made available from non-bank businesses, like newsagents or pharmacies.
Prior to partnering with MongoDB, the bank ran the system on a legacy relational database that lacked the flexibility to easily evolve to reflect the complex data mapping requirements that today’s modern applications demand.
Because of these database-related challenges, the bank’s analysts and developers were committing significant effort to complex database management tasks in order to keep the application running.
Specifically, the bank sought to adopt a new microservices architecture to help integrate its data and a robust API ecosystem — a series of connective mechanisms that enable easy data exchange — to facilitate fast, easy data flow between the bank’s applications, third parties, and consumers.
The bank chose MongoDB Atlas as the underlying platform for its tech stack because of its ability to manage the bank’s real-time, operational data on a unified platform, and because of the intuitive developer experience offered by MongoDB’s document model, which maps to how developers think and code, enabling them to build applications faster.
With this migration, Bendigo and Adelaide Bank has eliminated volumes of routine code writing and programming work with automated generative AI tooling, which has empowered the bank’s developers to innovate with increased agility and more quickly deploy highly-available and performant application features that enhance end-user experiences.
“Now, our lean, highly skilled team can ask MongoDB Atlas and the generative AI tooling MongoDB’s Professional Services team built for us to do really smart things, leaving our developers free to focus on other ways they can deliver great outcomes for our customers,” said Andrew Cresp, CIO at Bendigo and Adelaide Bank.
“We’ve started by modernising our most critical applications, and next, we intend to tackle a number of other outdated legacy applications across the bank, putting us on track to meet our target of 50 percent of our critical workloads in the cloud by the end of the year,” said Cresp.
Article originally posted on mongodb google news. Visit mongodb google news
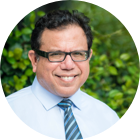
MMS • Steef-Jan Wiggers
Article originally posted on InfoQ. Visit InfoQ
Google Cloud has announced a significant update to its Cloud Storage services by introducing the Hierarchical Namespace (HNS). Now available in preview, this new feature allows users to organize their storage buckets in a hierarchical file system structure, enhancing performance, consistency, and manageability.
The hierarchical namespace enables users to organize their data more effectively by creating directories and nested subdirectories within storage buckets. This logical structuring mirrors traditional file systems, making it easier for users to manage and access their data. The hierarchical organization simplifies data management and improves performance, particularly for workloads requiring extensive directory and file operations.
Vivek Saraswat, a group product manager, and Zhihong Yao, a staff software engineer, both at Cloud Storage at Google, write:
A bucket with a hierarchical namespace has storage folder resources backed by an API, and the new ‘Rename Folder’ operation recursively renames a folder and its contents as a metadata-only operation. This ensures the process is fast and atomic, improving performance and consistency for folder-related operations compared to existing buckets.
In addition, Richard Seroter, chief evangelist at Google Cloud, tweeted:
.. create a more functional “tree” of objects. This improves how you interact with “folders,” improves performance, and more.
Left: Cloud Storage bucket with a flat hierarchy and simulated folders. Right: Bucket with hierarchical namespace organized into a tree-like structure (Source: Google Cloud blog post)
The introduction of HNS is particularly beneficial for scenarios that require high performance and manageability, such as big data analytics, content management systems, and large-scale application deployments. For example, a media company managing vast libraries of video files can use HNS to organize content by project, date, or type, improving accessibility and processing efficiency.
Users can create new buckets with HNS enabled or migrate existing buckets to utilize the hierarchical namespace. Google Cloud provides comprehensive documentation and tools to facilitate this transition. Users can allow HNS through the Google Cloud Console, command-line interface, or API, offering flexibility in managing their storage resources.
Patrick Haggerty, a director of Google cloud learning at ROI Training, listed in a LinkedIn post the pros and cons of the HNS feature in Google Cloud Storage:
Pros:
- If you rename a folder, it doesn’t have to move or rewrite files anymore.
- New API operations to manipulate folders.
- Faster initial QPS (x8) on read/write operations.
- Works with the managed folder thing for folder permissions.
Cons:
- Must be enabled when the bucket is created.
- No support for versioning, locks, retention, or file-level ACLs.
- Extra charge for the feature (pricing not announced).
Other Hyperscalers like Microsoft and AWS also offer the HNS feature in their storage services. For instance, in Azure Data Lake Storage Gen2, HNS organizes objects/files within an account into a hierarchy of directories and nested subdirectories. Meanwhile, in Amazon S3, Directory Buckets organize data hierarchically into directories instead of the flat storage structure of general-purpose buckets.
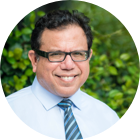
MMS • Ghida Ibrahim
Article originally posted on InfoQ. Visit InfoQ
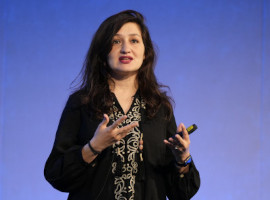
Transcript
Ibrahim: My name is Ghida. I’m a chief architect and head of data at Sector Alarm, which is one of Europe’s leading providers of smart alarm solution. It’s a Norwegian company, not present in the UK in case you didn’t hear of it. Before that, I spent 6 years at Meta/Facebook as a technical lead working on infrastructure optimization.
I’m going to talk about how AIOps, which refers to using AI and advanced analytics for managing IT operations at scale, is really useful in the context of ML operations. This talk basically is based on my previous experience, previous to my current role, especially at Meta, even though I have to emphasize that this is not necessarily a Meta related talk or a talk sponsored by Meta. It’s just inspired by my work there and also my work during my PhD on multi-cloud optimization.
Outline
First of all, I’ll introduce the concept of AIOps, what do we mean by AIOps? Then I will talk about the transition that we have seen from DevOps towards MLOps. How MLOps is very different or is different in some ways, to DevOps. Where do orchestrators use, both for DevOps and MLOps, fail, and why AIOps could be actually the solution here. Then, we’ll dive deeper into specific use cases of how to use AIOps for improving ML operations at scale, and specific use cases related to trend forecasting, workload orchestration, anomaly detection, and root causing.
What is AIOps?
What is AIOps? AIOps is AI operations. It refers to the use of AI, machine learning, and advanced analytics to enhance and automate various aspects related to IT operations and infrastructure management. You can think of anything related to forecasting trend, whether this is like the compute needs of a given application, the storage data needs, the network bandwidth needs. It could refer to orchestrating load. If you have different workloads, how do you decide which type of infrastructure to use for a specific workload and where to direct this workload in real time as well? Also, there is a lot of work related to anomaly detection, and how can we detect that something went wrong, whether it is at the application level or at the infrastructure level, and how to root cause it efficiently without producing a lot of low-noise signals to development teams?
From DevOps to MLOps: Where Do Current Orchestrators Fall Short?
In this section, I’ll try to give an overview of the evolution from DevOps towards MLOps, and where do current orchestrators, which introduce some level of intelligence here, fall short. DevOps is a set of practices that combine software development and IT operations. The goal here is to shorten the system’s development lifecycle and provide continuous delivery of high software quality. I’m sure you’re all familiar with this diagram, like starting with planning what to code, coding, building, testing, releasing, deploying, operating, monitoring. This is an example using Azure as a platform. Here you see that as a developer, you could be using a tool like Visual Studio to do your coding. Then you would be committing it to GitHub, for instance. You would be using GitHub Actions to do things like building your code or testing it as well. Then, once it’s ready, you can maybe transform it into a container. You can push it into a container registry, or you can deploy it to an orchestrator, like Kubernetes, for instance. Kubernetes is not the only choice here, just like an illustrative example here. You can deploy it also to other platforms or other services, like App Service, for instance. After that you have a bunch of monitoring capabilities tools: Azure Monitor, App Insights. In every cloud provider environment, you would find something similar to what I have just presented here in terms of giving developers tools to code, to test, to build, to deploy, to monitor.
What is MLOps? MLOps is a set of practices and tools that aim at combining machine learning and development operation to streamline the deployment, scaling, monitoring, and management of machine learning models in production environments. What does this mean? It means that we need to cover different steps, starting with data management. Machine learning is very heavy on data. It really relies on identifying patterns in data. Data management, data processing, getting the data ready for training, and then streaming data in real time for inference and serving purposes is a very important component of MLOps. You have the part related to feature engineering, deciding on the future, creating a new future, doing a bunch of transformation in the data to create these features. You have the model building itself, which we refer to as ML training. Really deciding on the model that we want to use, the hyperparameters, maybe fine-tuning, maybe comparing different models and deciding at the end, what do we want to go for? Of course, the evaluation part of this. Once we have made this decision, you have the ML model deployment or serving, monitoring and production, and optimization and fine-tuning. Of course, this is a cycle because how the model performs in production would influence what kind of data we decide to collect in the future. We would need to make sure that the collection of data is always up to date, feature engineering are revisited, the model is retrained. This is really a loop here. MLOps is quite like a multi-step process, a multi-step workflow.
What’s the difference between MLOps and DevOps? DevOps mainly enables the deployment of a given application, probably a containerized application within a given cluster. Whereas MLOps deals with an entire end-to-end workflow that has a lot of dependencies, can have complex logic. Most importantly, the different steps that I just presented, from data management to model training to feature engineering to model serving, have different infrastructure and provisioning requirements. In a way, MLOps is quite more complex of DevOps. Of course, it can leverage DevOps to handle individual ML tasks. For instance, we can use it for training or for serving. It’s not only DevOps, DevOps is just an enabler, is just a part of the entire workflow, of the entire equation. This is an example actually of an MLOps workflow that I took from a very interesting book by a writer called Chip [inaudible 00:08:15], about enabling ML systems design. I really recommend reading this book. It could be something like this. We could decide to pull data from a data warehouse. We featurize the data. We use different models. We compare these two models, and we evaluate them in real time. Then we decide which one to use. This logic is really not as simple to be deployed only in DevOps, so we really need to support complex logic and complex workflows here.
Where do current orchestrators fall short? Here, I have taken a bunch of orchestrators, like the most popular one, at least, for both DevOps and MLOps. I did lightweight comparative studies of these different orchestrators and where do they fall short in terms of enabling this entire workflow to operate smoothly. Kubernetes would be the most popular DevOps orchestrator. Of course, it has many advantages in terms of enabling, orchestrating IT applications and increment cluster and not worrying about a bunch of stuff, from scaling to failure management, to updating the code, all of these things. Still, it has its own limitation. Some of these limitations are related to the fact that we’re dealing with a mono-cluster deployment. This cluster has intrinsic and similar capabilities, like it’s a CPU cluster or GPU cluster, or a certain type of GPU or a certain type of CPU. It’s really a mono-cluster configuration deployment here. You still have to configure yourself as a software engineer the container and decide which container would go together into one pod. Autoscaling is not always supported in Kubernetes. Failure can be detected at nodes, but it’s not necessarily root caused and it’s not detected at the application level, which in itself can be a limitation. Because if there is a problem that is impacting all nodes, and all nodes keep failing, we can redirect the load to another node, but we’re really not resolving the problem here. At the end, cost efficiency or resources efficiency and service QoS are not really priority here. It’s really not a priority to decide, is this cluster best suited from a latency perspective for this kind of workload or not? This extra logic is not really there. Now comes a series of MLOps orchestrator, that have evolved over time. We started with Airflow, which enables what we call configuration as code. Basically, you can code your workflow, or configure it, that like, let’s start with this task and then continue to that task, and for this task, use this infrastructure. The problem with Airflow is that it enables a monolithic deployment of workflows. An entire workflow, including all the steps that I just mentioned, would be deployed in just one application, or one container, which is not always the most optimal thing to do, given that every step is separated. We want to limit this high dependency, high tight coupling between all these steps. Also, because these different steps have different infrastructure requirements, so maybe this is not the best solution from a provisioning perspective. Also, Airflow has limitations in terms of workflow customization and handling complex workflows. For instance, if we look at what I just presented here, this kind of workflow, here we have a certain complex logic, which is an if-then logic. This would not be supported in Airflow. Same thing, for instance, if we have a workflow that depends on, how many lines of data we have. Depending on the number of instances we have in the data, we decide to go for a model over another. Airflow would not enable this. These are the main limitations of Airflow that led to a next generation or a new generation of MLOps orchestrators, like Prefect and Argo, that obviously introduced a lot of improvement in terms of customization, in terms of making or supporting more dynamic workflows, more parameterized workflows. Still had also their own limitations, especially in terms of messy configuration. For instance, if we look at an orchestrator like Prefect, you still have to write yourself your own YAML file, and you have to also attach Dockerfiles to run specific steps in your workflow on a Docker container, for instance. It’s really a lot of configuration. It requires a lot of expertise. It requires a lot of knowledge. The infrastructure configuration is still pretty much manual. You need to decide how many GPUs or TPUs or CPUs you need, and what type. Also, these two tools here, or these two orchestrators have limited testing capabilities in the sense that it only runs in production. It didn’t offer developers that much flexibility in terms of testing their workflow. This is when a new generation of MLOps orchestrators came, like Kubeflow and Metaflow. Kubeflow is more popular than Metaflow. They solved the problem of limiting testing capabilities by enabling developers to run workflows from a notebook, both in production and in dev environments. A really smooth experience here for developers. There are still messy configuration components remaining here, especially for Kubeflow, where you really need to create a different YAML file and Dockerfile for each part of your process, and stitch them together in a Python file. This is quite a lot of configuration. The infrastructure configuration as well is remaining pretty much manual. This is where current orchestrators, even though they brought the developer community a long way and adapted to the ML needs, fall short. This is where introducing an extra abstraction level that we refer to as AIOps could be very beneficial.
AIOps for MLOps: Relevant Use Cases
In this section, we’re going to dive a bit into AIOps and what do we mean by it, and what is this extra layer that we’re referring to, and what kind of functionalities is enabling, and what kind of abstraction is enabling. Most importantly, what AI techniques or analytics techniques are relevant there. For instance, if we look at ML pipelines. As I mentioned, we have different components from data extraction, transformation loading, feature engineering, ML training, ML serving. We have the underlying resources, which can be on-premise, in the cloud. It could be actually a hybrid setting where a part of the resources are on-premise, a part are in the cloud. It could be a multi-cloud native setup. It could be really anything. You don’t really need to be dependent on one cloud in this instance. The resources here could be physical infrastructure resources, like EC2 instances for AWS, like virtual machines. It could be platform resources like EKS or AKS, basically Kubernetes orchestrator, or other tools like AWS batch processing tool. It could be Software as a Service resources like Vertex AI, or Amazon SageMaker that offer a really high level of abstraction. Still, for us, as developers, trying to deploy machine learning models, these are all resources that we need to access, and that can be anywhere, in any cloud provider. Actually, some cloud provider could be better for ETL, other could be better for ML training. We need this layer in the middle to do this kind of matching between the needs of our ML pipeline, and what resources are best suited for this, both from a performance perspective, but also from a cost perspective for us, as like a business client.
The AIOps layer is really this layer on the middle doing this abstraction and doing this matching between the needs and the resources. It’s doing a bunch of things, from capacity scaling to workload orchestration, and anomaly detection and root causing. We will dive next to each of these use cases, and see what kind of AI techniques could be used there. If we start with capacity scaling, I think the first use case would be what we call workload profile prediction. What do we mean by workload profile prediction? It’s basically being able to tell based on the definition or the description of a given task, and if there are any past demand patterns or data that we have, also this. What would be the capacity needs of this task in particular in our ML workflow? It would look something like that. You would have the task SLAs, so service level agreement that are dependent on the task. For instance, if we’re talking about data transformation, it will be about the data volume, the original schema of the data, the target schema of the data. We’ll see an example. If we’re talking about ML training, it could be about the data itself, but also about the models that we want to experiment with. If it is about ML serving, it could be about the number of concurrent requests that we’re looking at, and what kind of latency expectations or other expectations we have in mind, and past demand patterns. What this engine should be able to tell us would be, how much compute do we need? What type of compute do we need? Is it CPU, is it GPU? Within CPU, GPU, we have different options. Even NVIDIA has different machines available for these purposes. What kind of machine is best suited for our workload? How many cores do we need? How much data capacity do we need? Is it best to have memory or on disk? Is it best to structure this data in SQL, non-SQL format? Most importantly, the network, so the input-output bandwidth, whether it is within a given instance, so between the memory and the compute, or between the disk and a GPU or CPU instance. This engine will really do this. For doing that, we can use a number of techniques. One technique would be time-series forecasting, especially if we have previous patterns of our application or of our task. We can use this to predict the future. The other thing would be doing some inference. We already have a lot of ML tasks deployed already in production by engineers and developers, and a lot of testing have taken place around this. What would be the best deployment choice to have here? We can use this knowledge actually to do inference in the future about similar tasks, like in the same way Netflix works in a sense that, based on what you have watched in the past, or based on what other people have watched, you get this recommendation. Same logic we can use here, to infer that for this specific task that is similar to that task that we know of in the past, we think that these resources and infrastructure is best suited. These are techniques that we can use in this context.
If we go into specific examples, let’s take a task that consists of transforming medical training data for ML training purposes. We would have the initial data state, the volume, and the target data state or schema. Ideally, this engine would output the storage capacity required in terabyte or petabyte. The storage capacity type, so it could be that we want to use a data warehouse infrastructure for storing original and target data. We want to use memory in between in the transformation process. Also, we could need compute for doing these ETL jobs. For instance, here, the prediction could be the number of data warehouse units that we need. What do we think would be the data warehouse unit expected utilization over time, so that we don’t provision a given number at all times? We can just scale it up and down, or basically schedule an autoscaling operation. Another example of a task would be, now we have transformed the data, now let’s do the training. Let’s predict cancer odds based on patient data. Here, we can say, we want to experiment with XGBoost, or a tree-based model. Or we can say, actually, we don’t know what would be the best model to use here. If we know, we can provide model hyperparameters. We can say that we want to repeat this training every day, or every week, or at any other cadency that we prefer. We can give a description of the training data, which is the data that we just transformed in the previous step. This forecasting engine will give us like, the compute type for this is like, we need to use GPUs. Let’s use NVIDIA H100. This is how many instances we need for really enabling these operations. What would be basically the use at different time slots? Because, for instance, training is something that we don’t do online, it’s an offline operation, so it doesn’t really require capacity usage at all times. Now that we have built our model, we want to deploy it to production. The task description here would be to deploy a cancer odds prediction model in production, or what we refer to as ML serving. Here, again, it could be that the compute type is CPU, let’s use devices or machines like NVIDIA Triton, and this is how much compute we need. Also, here, one component would be the compute location, because here we’re talking about serving. Maybe latency is an important component, or having like a real time reply maybe in this use case, is an important component. Or the capacity forecasting engine would be able to tell from the task description, what are the requirements of this task in terms of latency or in terms of other QoS requirements, and take this into account when suggesting or outputting a certain infrastructure that can support that.
The second application or use cases where we can use AI for enabling MLOps operations at scale, is optimizing workload placement. Now that we have the workload profiles associated with the different tasks in our end-to-end ML workflow, we want to do what we call orchestration. Orchestration here is a bit different than the orchestration that we talk about when we talk about Kubernetes, for instance. What we mean by orchestration here is that we have different types of workloads from ETL, to feature engineering to training an ML model to ML serving. These workloads have different requirements. Also, they run at different time slots. For instance, ML training happens once a day. ML servings happens in real time, like every second. What we’re trying to do here is that, we have all these workloads, we know that these are the requirements. How can we aggregate these workloads and decide where to direct them in real time? The first step here is to do workload aggregation, which refers really to multiplexing workloads with similar capacity type and geo needs. For instance, if we know that these different workloads all needs the same type of CPU, and we can use the same CPU cluster, and actually one workload needs these CPU machines at a certain time, but another worker at other times where they’re not needed by the first task, then this constitutes the perfect use case for multiplexing these two workloads together and optimizing our infrastructure usage. This is the multiplexing that happens here, really based on similar capacity type, and also geolocation needs. The other step would be to aggregate workloads to cluster assignments. Now we know that, workload 1 and workload 3, I can batch together, because they have similar needs. Now, this is my infrastructure, these are my clusters. I have two AWS clusters, three Azure clusters, whatever, how can I best do this assignment of workloads to clusters? In this case, you can use something like operations research or constrained optimization for assigning these aggregated workloads to a cluster. What optimization here refers to is trying to maximize the number of workloads we’re serving, and also the fact that we’re serving them with a high level of SLA, ideally, really close to 100%, while being aware of the infrastructure requirement, and while trying also to keep the cost below a certain level. Here, what we’re trying to achieve is to really have a certain objective, which could be, let’s achieve all our SLAs, or let’s maximize our SLA achievement. Our constraints would be the capacity that we have, and the cost. It could be the other way around, maybe our objective is to minimize the cost, and the constraint that we have is more that our SLA should be more than this. Maybe we’re happy with only 99% SLA, we’re not really interested with 99.999%, or something like that. It really depends on our priority as a company, as a user. We really can use here some kind of optimization to do this assignment. Then, based on the outcome of this assignment, we might decide that, for ETL, let’s use AWS clusters in Brazil. For ML serving, let’s use Azure in Western Europe. Based on this offline calculation, we do the direction of the workload in real time. Of course, things can change. Of course, our decision on how to direct workloads could be influenced by the fact that some clusters suddenly became unavailable. We need to react to this as well in real time. At least this provides us with a high-level direction on what to do if everything were to be fine, and what is the most optimal thing to do here.
The third use case is, now we predicted the workload profiles, and then we decided how to aggregate the workloads and where to direct them, to which type of cluster. Now we have all this in place, things can go wrong, things can fail. How do we detect that things fail as soon as possible, preferably before end users detect this themselves? Most importantly, how do we root cause? A bunch of motivation here exists, and I try to group it into cluster. First of all, we want to reduce the on-call fatigue. We don’t want like 1000 alarms about a similar thing. We want to group similar alarms, we want to minimize the false positive. If there is a small alarm about something, but actually the end user will not be impacted, maybe we don’t want to bother the engineering teams with it yet. Second thing, we want to reduce the time to detection. Ideally, detecting issues before users are impacted. Most importantly, we want to understand what has gone wrong, so we can learn from it and make sure that it doesn’t propagate, so we can stop it. What can be done here? We can be looking at a bunch of signals from clusters’ health, so really physical clusters have infrastructure metrics, or KPIs, to also more application-level or task-specific KPIs that are relevant for different tasks. For instance, if we’re looking at ETL, it could be the number of data rows that we have. If it suddenly increased too much or suddenly decreased too much, it could be an indicator that something wrong has happened. If we’re looking at feature engineering, if suddenly we see a big drop or increase in our features, this also could be an indication that something wrong has happened. If we’re looking at ML training, it could be the change of the model precision that we have, could be also an important metric to look at. We don’t need to wait until the entire model has been retrained and we’ve lost the precision. Even if we lost the precision on a batch, we can use this as a signal. Finally, for something like ML serving, we can look at the number of concurrent requests. If we see that the number of concurrent requests have dropped, with respect to the same time of the day, a day back, or a year back, or a week back, or whatever, then we can also detect this outlier behavior.
Here, all of these kinds of elements or components will generate alarm signals when something goes wrong. We can use anomaly detection and leverage something like hypothesis testing to ask ourselves, is this behavior of the cluster, or of the model, or of the data normal given what we have seen in the past or not, and compute what we call a z-score. If the score is high, this means that something wrong is happening, and this is an outlier behavior. The second thing would be maybe to implement in our logic, some kind of human knowledge saying, “This is normal, this is not very normal.” This can be hard coded, or can be expert knowledge, put into our code or into our engine. Then the third step would be to do anomaly clustering. Can we have all these alarms? Are there any similarities between the alarms that we’re seeing? Can we refer them to one task, or can we refer them to one type of user device, or one type of infrastructure like a given provider, like Azure, for instance, or a given cluster, in particular? Can we do this kind of grouping, which can help us really simplify the way we’re doing things and simplify or reduce the fatigue of our on-call team. Then, this would lead to what we call a super alarm. An alarm that really aggregates many alarms, and it has a summary of many wrong things going on. We can do what we call an alarm root causing. Mainly the idea here is a tree-based model, like, what is the most common thing between these different alarms? We’ve seen this alarm for all users that have iOS devices, what wrong thing could have happened? Let’s start with hypothesis 1. If this is not the case, let’s start with hypothesis 2, and so on. This root causing can help us boil down what exactly went wrong, and how basically to fix this. Here, as you can see, we have used a bunch of techniques in this process, from hypothesis testing, to clustering, to tree-based models, to expert networks as well, for automating the generation and root causing of high-signal, low-noise alarms. This is just an illustration about really like, how can we leverage AI and advanced analytics and ML for really improving the way we deploy ML workflows?
Conclusion
As we have seen in this presentation, AIOps is key for tackling the increasing complexity of ML workflows. Given how complex ML workflow is, given the dependencies, given the different requirements, infrastructure requirements, we need this extra level of abstraction for tackling this increasing complexity and making things easier for developers. It enables abstracting complex decisions and operations from capacity scaling to workload orchestration and performance monitoring. I personally believe that when implemented correctly by existing cloud providers, or even new platform providers, because this really creates a space for new kinds of operators beyond cloud providers to provide this service that can be multi-cloud, hybrid, AIOps has the potential to further democratize ML and LLM adoption. As we have seen in the past year or so, LLM really democratized the deployment of applications. You can simply deploy applications by creating smart prompts. I think why not do the same for deploying ML and abstracting all this complexity that we have around ML operations by creating this AIOps empowered layer, where we can use a bunch of techniques from inference to time-series forecasting, to optimization.
Questions and Answers
Participant 1: Is this an intended direction that you see that the industry should take, or are you aware of existing solutions or platforms that already propose these functionalities?
Ibrahim: I think that this is a direction that we should be taking. I’m not aware of public solutions that do this kind of abstraction. What I know is that, for instance, at Facebook where I was, there were dedicated teams for creating an extra intelligence beyond the cluster level around how we should be scaling capacity, where we should actually be placing our data centers or our point of presence. How do we detect alarms better? How do we best orchestrate different workloads in a way that really allow us to manage the infrastructure better and reduce the cost? Because reducing costs have been obviously a very important theme for many big tech companies over the past years. I’m not aware of solutions that do this at this level of abstraction. Obviously, we have the orchestrators which I presented and probably there are many more that I haven’t necessarily used, or I’m not completely aware of, but I think this is still pretty technical, this is still pretty low level. There is another layer of abstraction that can be added here. This is exactly what I’m talking about here.
Nardon: In my company, we actually developed ML models to detect capacity and try to increase the cluster as predicting when we needed more power. You have an experience with Facebook, but if you know how is the landscape for AIOps from other companies. Are these something that big companies are looking at and having teams for that? It seems it’s something that each company is doing their own models. It’s a landscape to invest on, it seems?
Ibrahim: I think that maybe cloud providers are best positioned to do this, and obviously some big tech companies that have their own clouds, like Facebook, which has an internal cloud. They are best positioned to do that. I think there is also a role for new players that are cloud agnostic or cloud native to emerge within this landscape, especially these different steps as I mentioned, could lead to decisions like, we don’t want to use just one cloud provider. We want really our solution to be multi-cloud or hybrid. I think there is room for new types of players to come in and establish themselves beyond a given cloud provider or like big tech company.
Nardon: It’s a good business to invest for those who are thinking about starting a startup.
See more presentations with transcripts
MongoDB, Inc. Investigation Ongoing: Contact Levi & Korsinsky About Potential Securities … – WATE
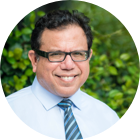
MMS • RSS
Posted on mongodb google news. Visit mongodb google news
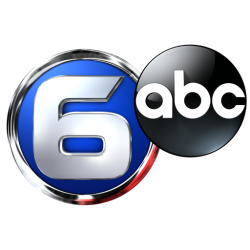
NEW YORK, NY / ACCESSWIRE / June 26, 2024 / Levi & Korsinsky notifies investors that it has commenced an investigation of MongoDB, Inc. (“Mongo”) (NASDAQ:MDB) concerning possible violations of federal securities laws.
On March 7, 2024, Mongo reported strong Q4 2024 results and then announced lower than expected full-year guidance for 2025. Mongo attributed it to the Company’s change in its “sales incentive structure” which led to a decrease in revenue related to “unused commitments and multi-year licensing deals.”
Following this news, Mongo’s stock price fell by $28.59 per share to close at $383.42 per share.
Later, on May 30, 2024, Mongo further lowered its guidance for the full year 2025 attributing it to “macro impacting consumption growth.” Analysts commenting on the reduced guidance questioned if changes made to the Company’s marketing strategy “led to change in customer behavior and usage patterns.” To obtain additional information, go to:
https://zlk.com/pslra-1/mongodb-inc-lawsuit-submission-form?prid=87691&wire=1
or contact Joseph E. Levi, Esq. either via email at jlevi@levikorsinsky.com or by telephone at (212)363-7500.
WHY LEVI & KORSINSKY: Over the past 20 years, Levi & Korsinsky LLP has established itself as a nationally-recognized securities litigation firm that has secured hundreds of millions of dollars for aggrieved shareholders and built a track record of winning high-stakes cases. The firm has extensive expertise representing investors in complex securities litigation and a team of over 70 employees to serve our clients. For seven years in a row, Levi & Korsinsky has ranked in ISS Securities Class Action Services’ Top 50 Report as one of the top securities litigation firms in the United States. Attorney Advertising. Prior results do not guarantee similar outcomes.
CONTACT:
Levi & Korsinsky, LLP
Joseph E. Levi, Esq.
Ed Korsinsky, Esq.
33 Whitehall Street, 17th Floor
New York, NY 10004
jlevi@levikorsinsky.com
Tel: (212)363-7500
Fax: (212)363-7171
https://zlk.com/
SOURCE: Levi & Korsinsky, LLP
View the original press release on accesswire.com
View the original press release on accesswire.com
Article originally posted on mongodb google news. Visit mongodb google news
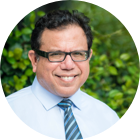
MMS • RSS
Posted on mongodb google news. Visit mongodb google news
MongoDB, Inc. (NASDAQ:MDB – Get Free Report) was the target of unusually large options trading activity on Wednesday. Investors purchased 36,130 call options on the company. This is an increase of approximately 2,077% compared to the typical daily volume of 1,660 call options.
MongoDB Stock Up 6.1 %
Shares of MongoDB stock opened at $240.52 on Thursday. The company has a debt-to-equity ratio of 0.90, a current ratio of 4.93 and a quick ratio of 4.93. MongoDB has a 1 year low of $214.74 and a 1 year high of $509.62. The firm’s 50-day moving average is $307.97 and its two-hundred day moving average is $368.31. The stock has a market cap of $17.64 billion, a price-to-earnings ratio of -85.59 and a beta of 1.13.
MongoDB (NASDAQ:MDB – Get Free Report) last issued its earnings results on Thursday, May 30th. The company reported ($0.80) earnings per share (EPS) for the quarter, hitting the consensus estimate of ($0.80). MongoDB had a negative net margin of 11.50% and a negative return on equity of 14.88%. The company had revenue of $450.56 million for the quarter, compared to analysts’ expectations of $438.44 million. As a group, research analysts expect that MongoDB will post -2.67 earnings per share for the current fiscal year.
Insiders Place Their Bets
In other MongoDB news, CRO Cedric Pech sold 1,430 shares of the firm’s stock in a transaction on Tuesday, April 2nd. The shares were sold at an average price of $348.11, for a total value of $497,797.30. Following the sale, the executive now owns 45,444 shares of the company’s stock, valued at approximately $15,819,510.84. The sale was disclosed in a legal filing with the SEC, which is available through this hyperlink. In other news, CRO Cedric Pech sold 1,430 shares of MongoDB stock in a transaction dated Tuesday, April 2nd. The shares were sold at an average price of $348.11, for a total transaction of $497,797.30. Following the sale, the executive now owns 45,444 shares of the company’s stock, valued at approximately $15,819,510.84. The sale was disclosed in a legal filing with the Securities & Exchange Commission, which is available at the SEC website. Also, Director John Dennis Mcmahon sold 10,000 shares of MongoDB stock in a transaction dated Monday, June 24th. The shares were sold at an average price of $228.00, for a total transaction of $2,280,000.00. Following the sale, the director now directly owns 20,020 shares in the company, valued at approximately $4,564,560. The disclosure for this sale can be found here. Insiders have sold a total of 59,976 shares of company stock valued at $19,525,973 in the last quarter. Insiders own 3.60% of the company’s stock.
Institutional Trading of MongoDB
A number of institutional investors have recently bought and sold shares of MDB. PNC Financial Services Group Inc. raised its position in MongoDB by 7.2% during the 3rd quarter. PNC Financial Services Group Inc. now owns 2,474 shares of the company’s stock worth $856,000 after buying an additional 166 shares during the last quarter. Clearbridge Investments LLC increased its holdings in shares of MongoDB by 4.4% during the 3rd quarter. Clearbridge Investments LLC now owns 1,949 shares of the company’s stock valued at $674,000 after purchasing an additional 82 shares in the last quarter. LPL Financial LLC increased its holdings in shares of MongoDB by 20.6% during the 3rd quarter. LPL Financial LLC now owns 31,145 shares of the company’s stock valued at $10,772,000 after purchasing an additional 5,329 shares in the last quarter. Mariner LLC increased its holdings in shares of MongoDB by 10.2% during the 3rd quarter. Mariner LLC now owns 7,047 shares of the company’s stock valued at $2,437,000 after purchasing an additional 653 shares in the last quarter. Finally, Northern Trust Corp increased its holdings in shares of MongoDB by 5.5% during the 3rd quarter. Northern Trust Corp now owns 448,035 shares of the company’s stock valued at $154,957,000 after purchasing an additional 23,270 shares in the last quarter. 89.29% of the stock is currently owned by institutional investors and hedge funds.
Analyst Ratings Changes
Several research firms recently commented on MDB. Redburn Atlantic reissued a “sell” rating and set a $295.00 price objective (down previously from $410.00) on shares of MongoDB in a research note on Tuesday, March 19th. Truist Financial decreased their target price on shares of MongoDB from $475.00 to $300.00 and set a “buy” rating for the company in a report on Friday, May 31st. Canaccord Genuity Group decreased their target price on shares of MongoDB from $435.00 to $325.00 and set a “buy” rating for the company in a report on Friday, May 31st. Guggenheim raised shares of MongoDB from a “sell” rating to a “neutral” rating in a report on Monday, June 3rd. Finally, KeyCorp decreased their target price on shares of MongoDB from $490.00 to $440.00 and set an “overweight” rating for the company in a report on Thursday, April 18th. One research analyst has rated the stock with a sell rating, five have assigned a hold rating, nineteen have given a buy rating and one has given a strong buy rating to the company. Based on data from MarketBeat, MongoDB presently has a consensus rating of “Moderate Buy” and a consensus price target of $361.30.
Get Our Latest Research Report on MongoDB
About MongoDB
MongoDB, Inc, together with its subsidiaries, provides general purpose database platform worldwide. The company provides MongoDB Atlas, a hosted multi-cloud database-as-a-service solution; MongoDB Enterprise Advanced, a commercial database server for enterprise customers to run in the cloud, on-premises, or in a hybrid environment; and Community Server, a free-to-download version of its database, which includes the functionality that developers need to get started with MongoDB.
Further Reading
Receive News & Ratings for MongoDB Daily – Enter your email address below to receive a concise daily summary of the latest news and analysts’ ratings for MongoDB and related companies with MarketBeat.com’s FREE daily email newsletter.
Article originally posted on mongodb google news. Visit mongodb google news
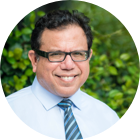
MMS • RSS
Posted on mongodb google news. Visit mongodb google news
MongoDB, Inc. (NASDAQ:MDB – Get Free Report) was the target of some unusual options trading activity on Wednesday. Stock traders acquired 23,831 put options on the company. This represents an increase of 2,157% compared to the typical volume of 1,056 put options.
MongoDB Stock Up 6.1 %
MDB opened at $240.52 on Thursday. MongoDB has a 52 week low of $214.74 and a 52 week high of $509.62. The company has a quick ratio of 4.93, a current ratio of 4.93 and a debt-to-equity ratio of 0.90. The firm has a 50-day simple moving average of $307.97 and a two-hundred day simple moving average of $368.31. The company has a market capitalization of $17.64 billion, a PE ratio of -85.59 and a beta of 1.13.
MongoDB (NASDAQ:MDB – Get Free Report) last announced its quarterly earnings results on Thursday, May 30th. The company reported ($0.80) EPS for the quarter, meeting analysts’ consensus estimates of ($0.80). MongoDB had a negative return on equity of 14.88% and a negative net margin of 11.50%. The company had revenue of $450.56 million during the quarter, compared to analyst estimates of $438.44 million. Analysts forecast that MongoDB will post -2.67 earnings per share for the current fiscal year.
Analysts Set New Price Targets
A number of equities research analysts have issued reports on the company. Truist Financial cut their price target on MongoDB from $475.00 to $300.00 and set a “buy” rating for the company in a research note on Friday, May 31st. Monness Crespi & Hardt raised shares of MongoDB to a “hold” rating in a report on Tuesday, May 28th. Mizuho dropped their price target on shares of MongoDB from $380.00 to $250.00 and set a “neutral” rating on the stock in a research note on Friday, May 31st. Stifel Nicolaus reduced their price objective on MongoDB from $435.00 to $300.00 and set a “buy” rating for the company in a research note on Friday, May 31st. Finally, Barclays lowered their target price on MongoDB from $458.00 to $290.00 and set an “overweight” rating on the stock in a research report on Friday, May 31st. One analyst has rated the stock with a sell rating, five have given a hold rating, nineteen have given a buy rating and one has issued a strong buy rating to the company’s stock. According to MarketBeat, MongoDB presently has a consensus rating of “Moderate Buy” and a consensus target price of $361.30.
Get Our Latest Research Report on MDB
Insider Buying and Selling at MongoDB
In other MongoDB news, CEO Dev Ittycheria sold 17,160 shares of the firm’s stock in a transaction dated Tuesday, April 2nd. The shares were sold at an average price of $348.11, for a total transaction of $5,973,567.60. Following the completion of the sale, the chief executive officer now directly owns 226,073 shares in the company, valued at approximately $78,698,272.03. The transaction was disclosed in a document filed with the SEC, which can be accessed through the SEC website. In other news, CEO Dev Ittycheria sold 17,160 shares of the business’s stock in a transaction that occurred on Tuesday, April 2nd. The shares were sold at an average price of $348.11, for a total transaction of $5,973,567.60. Following the completion of the transaction, the chief executive officer now directly owns 226,073 shares of the company’s stock, valued at $78,698,272.03. The transaction was disclosed in a filing with the Securities & Exchange Commission, which can be accessed through the SEC website. Also, Director Dwight A. Merriman sold 1,000 shares of the firm’s stock in a transaction that occurred on Monday, April 1st. The stock was sold at an average price of $363.01, for a total transaction of $363,010.00. Following the completion of the sale, the director now owns 523,896 shares in the company, valued at approximately $190,179,486.96. The disclosure for this sale can be found here. In the last ninety days, insiders sold 59,976 shares of company stock worth $19,525,973. 3.60% of the stock is owned by corporate insiders.
Institutional Inflows and Outflows
A number of institutional investors and hedge funds have recently modified their holdings of the business. Vanguard Group Inc. grew its holdings in shares of MongoDB by 1.0% in the first quarter. Vanguard Group Inc. now owns 6,910,761 shares of the company’s stock valued at $2,478,475,000 after purchasing an additional 68,348 shares in the last quarter. Jennison Associates LLC grew its holdings in shares of MongoDB by 14.3% in the first quarter. Jennison Associates LLC now owns 4,408,424 shares of the company’s stock valued at $1,581,037,000 after purchasing an additional 551,567 shares in the last quarter. Norges Bank purchased a new position in shares of MongoDB in the fourth quarter valued at $326,237,000. Champlain Investment Partners LLC lifted its position in MongoDB by 22.4% in the first quarter. Champlain Investment Partners LLC now owns 550,684 shares of the company’s stock worth $197,497,000 after buying an additional 100,725 shares during the last quarter. Finally, First Trust Advisors LP boosted its stake in MongoDB by 59.3% during the fourth quarter. First Trust Advisors LP now owns 549,052 shares of the company’s stock worth $224,480,000 after buying an additional 204,284 shares during the period. 89.29% of the stock is owned by hedge funds and other institutional investors.
MongoDB Company Profile
MongoDB, Inc, together with its subsidiaries, provides general purpose database platform worldwide. The company provides MongoDB Atlas, a hosted multi-cloud database-as-a-service solution; MongoDB Enterprise Advanced, a commercial database server for enterprise customers to run in the cloud, on-premises, or in a hybrid environment; and Community Server, a free-to-download version of its database, which includes the functionality that developers need to get started with MongoDB.
Featured Articles
Receive News & Ratings for MongoDB Daily – Enter your email address below to receive a concise daily summary of the latest news and analysts’ ratings for MongoDB and related companies with MarketBeat.com’s FREE daily email newsletter.
Article originally posted on mongodb google news. Visit mongodb google news
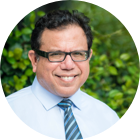
MMS • RSS
Posted on mongodb google news. Visit mongodb google news
MongoDB, Inc. (NASDAQ:MDB – Get Free Report) Director John Dennis Mcmahon sold 10,000 shares of MongoDB stock in a transaction on Monday, June 24th. The shares were sold at an average price of $228.00, for a total value of $2,280,000.00. Following the sale, the director now directly owns 20,020 shares of the company’s stock, valued at approximately $4,564,560. The transaction was disclosed in a document filed with the Securities & Exchange Commission, which is accessible through the SEC website.
MongoDB Trading Up 6.1 %
MDB stock traded up $13.91 during trading on Wednesday, reaching $240.52. The company had a trading volume of 2,504,639 shares, compared to its average volume of 1,529,366. MongoDB, Inc. has a 52 week low of $214.74 and a 52 week high of $509.62. The company has a market cap of $17.64 billion, a P/E ratio of -85.59 and a beta of 1.13. The business has a 50 day moving average of $307.97 and a 200 day moving average of $368.31. The company has a debt-to-equity ratio of 0.90, a current ratio of 4.93 and a quick ratio of 4.93.
MongoDB (NASDAQ:MDB – Get Free Report) last announced its quarterly earnings data on Thursday, May 30th. The company reported ($0.80) EPS for the quarter, meeting the consensus estimate of ($0.80). The business had revenue of $450.56 million during the quarter, compared to analyst estimates of $438.44 million. MongoDB had a negative return on equity of 14.88% and a negative net margin of 11.50%. As a group, sell-side analysts forecast that MongoDB, Inc. will post -2.67 earnings per share for the current fiscal year.
Analysts Set New Price Targets
MDB has been the topic of a number of research analyst reports. KeyCorp decreased their price target on shares of MongoDB from $490.00 to $440.00 and set an “overweight” rating on the stock in a report on Thursday, April 18th. Redburn Atlantic restated a “sell” rating and set a $295.00 price target (down previously from $410.00) on shares of MongoDB in a research report on Tuesday, March 19th. Loop Capital cut their price objective on MongoDB from $415.00 to $315.00 and set a “buy” rating for the company in a report on Friday, May 31st. Canaccord Genuity Group reduced their price objective on MongoDB from $435.00 to $325.00 and set a “buy” rating on the stock in a research report on Friday, May 31st. Finally, Oppenheimer dropped their target price on MongoDB from $480.00 to $300.00 and set an “outperform” rating for the company in a research report on Friday, May 31st. One investment analyst has rated the stock with a sell rating, five have issued a hold rating, nineteen have issued a buy rating and one has issued a strong buy rating to the company. According to MarketBeat, MongoDB currently has an average rating of “Moderate Buy” and a consensus price target of $361.30.
Check Out Our Latest Stock Report on MongoDB
Institutional Inflows and Outflows
A number of large investors have recently added to or reduced their stakes in MDB. Cetera Advisors LLC raised its holdings in MongoDB by 106.9% in the 1st quarter. Cetera Advisors LLC now owns 1,558 shares of the company’s stock worth $559,000 after acquiring an additional 805 shares during the last quarter. Cetera Investment Advisers raised its stake in MongoDB by 327.6% during the 1st quarter. Cetera Investment Advisers now owns 10,873 shares of the company’s stock valued at $3,899,000 after buying an additional 8,330 shares during the last quarter. Atria Investments Inc increased its position in MongoDB by 1.2% during the first quarter. Atria Investments Inc now owns 3,259 shares of the company’s stock valued at $1,169,000 after acquiring an additional 39 shares during the last quarter. Swedbank AB bought a new position in shares of MongoDB in the 1st quarter worth approximately $91,915,000. Finally, LRI Investments LLC purchased a new position in shares of MongoDB during the 1st quarter worth $106,000. Hedge funds and other institutional investors own 89.29% of the company’s stock.
About MongoDB
MongoDB, Inc, together with its subsidiaries, provides general purpose database platform worldwide. The company provides MongoDB Atlas, a hosted multi-cloud database-as-a-service solution; MongoDB Enterprise Advanced, a commercial database server for enterprise customers to run in the cloud, on-premises, or in a hybrid environment; and Community Server, a free-to-download version of its database, which includes the functionality that developers need to get started with MongoDB.
Recommended Stories
Before you consider MongoDB, you’ll want to hear this.
MarketBeat keeps track of Wall Street’s top-rated and best performing research analysts and the stocks they recommend to their clients on a daily basis. MarketBeat has identified the five stocks that top analysts are quietly whispering to their clients to buy now before the broader market catches on… and MongoDB wasn’t on the list.
While MongoDB currently has a “Moderate Buy” rating among analysts, top-rated analysts believe these five stocks are better buys.
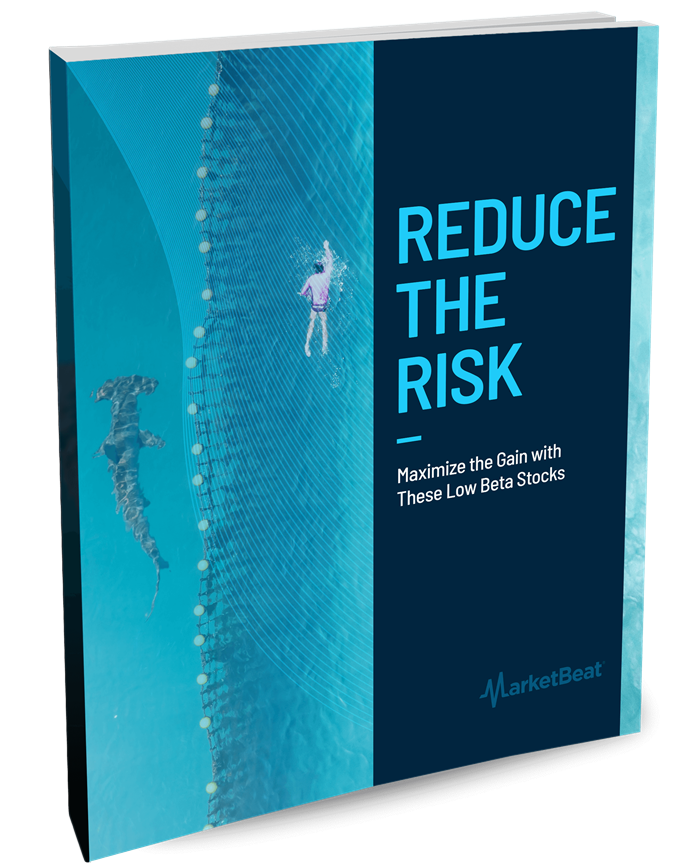
Market downturns give many investors pause, and for good reason. Wondering how to offset this risk? Click the link below to learn more about using beta to protect yourself.
Article originally posted on mongodb google news. Visit mongodb google news