Vector databases can’t solve modern data challenges any better than traditional SQL or NoSQL databases can.
Jun 3rd, 2024 10:34am by
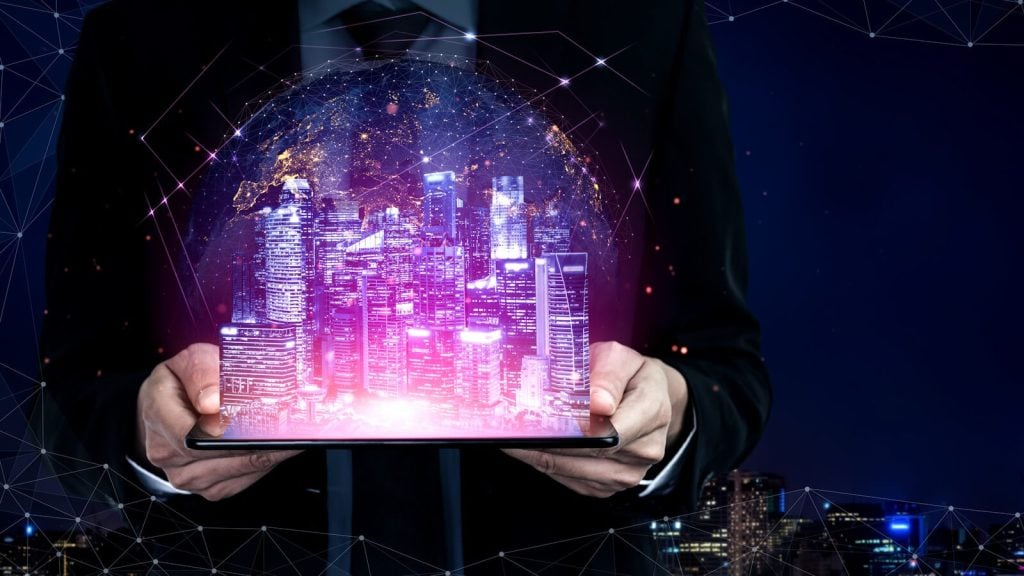
Featured image by Unsplash+ in collaboration with Getty Images.
With the rise of AI, vector databases have gained significant attention due to their ability to efficiently store, manage and retrieve large-scale, high-dimensional data. This capability is crucial for AI and generative AI (GenAI) applications that deal with unstructured data such as text, images and videos.
The main logic behind a vector database is to provide similarity search capabilities, rather than keyword search, as traditional databases provide. This concept has been widely adopted to boost the performance of large language models (LLMs), particularly following the release of ChatGPT.
The biggest issue with LLMs is that they require substantial resources, time and data for fine-tuning. Which makes it very difficult to keep them updated. This is why when you query LLMs about recent events, they often provide answers that are factually incorrect, nonsensical or disconnected from the input prompt, leading to “hallucinations.”
One solution is retrieval-augmented generation (RAG), which augments an LLM by integrating up-to-date information retrieved from an external knowledge base. Specialized vector databases are designed to handle vectorized data efficiently and provide robust semantic search capabilities. These databases are optimized for storing and retrieving high-dimensional vectors, which are very important for making similarity searches. The speed and efficiency of vector databases have made them an integral part of RAG systems.
The hype around vector databases has led many people to suggest that traditional databases might be replaced by vector databases. Instead of storing data in traditional (SQL or NoSQL) databases, could you store an organization’s entire data set in a vector database and retrieve it using natural language instead of writing manual queries?
But vector databases don’t function like traditional databases. As Qdrant CTO Andrey Vasnetsov wrote, “the majority of vector databases are not databases in this sense. It is more accurate to call them search engines.” This is because their main purpose is to provide optimized search functionalities, and they are not designed to support basic features like keyword search or SQL queries.
Limitations of Specialized Vector Databases
As use cases grew and people focused on the scalability of their applications, the limitations of vector databases became more visible. Developers soon realized they still need the features of a full-text search engine along with vector search. For example, filtering search results based on specific criteria is very difficult with vector databases. These databases also lack direct matches for exact phrases, which are crucial for many tasks.
Limited Support for Complex Queries
Complex queries often involve multiple conditions, joins and aggregations, making them challenging for specialized vector databases. These databases provide limited support for complex queries through metadata filtering. However, metadata storage is very limited in vector databases, which restricts users’ ability to make a wide range of complex queries.
In contrast, SQL databases are designed to handle extensive storage and processing, allowing efficient execution of complex queries involving multiple conditions, joins and aggregations. This makes SQL databases far more versatile and capable when it comes to handling complex data retrieval and manipulation tasks.
Data-Type Limitations
Specialized vector databases also face data-type limitations. They are designed to store vectors and minimal metadata, which restricts their flexibility. This focus on vectors means they cannot handle the wide variety of data types SQL databases can, such as integers, strings and dates, which allows more complex and varied data operations.
Overall, specialized vector databases have a very narrow focus. Their architecture is optimized primarily for semantic search rather than broader data management needs. This restricts their functionality to perform a wide range of tasks that are easily handled by more versatile systems like SQL databases. In addition, their inability to store and manage different data types beyond vectors makes them less suitable for general-purpose database tasks. Vector databases work well for RAG applications, but they are not versatile enough for broader use cases.
Integration Challenges
Integrating specialized vector databases into existing IT infrastructures is fraught with challenges. Compatibility issues often arise due to the inherent differences between specialized vector databases and existing systems, necessitating significant data transformation and potential data loss or corruption. Ensuring interoperability with legacy systems and maintaining data consistency and integrity are also complex tasks. Moreover, the integration process requires specialized skill sets, which may not be readily available within an organization, leading to high training costs and a steep learning curve.
Furthermore, the financial implications of integration are substantial. Costs include software licensing, hardware upgrades, personnel training and ongoing maintenance. Additionally, existing applications may need to be modified or rewritten to interact with the vector database, which is a costly and risky process with the potential for introducing new bugs or performance issues. The need for continuous support and updates for the specialized vector database can also lead to long-term financial commitments.
Data Processing Requires a Hybrid Approach
The foundations of a specialized vector database are vector storage and vector search, primarily for RAG applications. However, traditional databases should also be able to handle vectors, and vector search is a query-processing approach, not a foundation for a new way of processing data.
RAG is a popular AI technique that benefits from vector databases. While vector databases are great for semantic searches and handling high-dimensional data, their focused capabilities often overlook an organization’s operational and functional needs. This can limit their use in broader applications with diverse operational and functional requirements.
Likewise, traditional databases have attempted to incorporate vector storage and vector search features to offer an efficient solution for large-scale processing of complex data types. For example, PostgreSQL and Elasticsearch have introduced vector search capabilities. However, their vector search performance is not as good and lags behind specialized vector databases like Pinecone and Qdrant. For example, Qdrant achieves a mean latency of only 45.23ms with a precision rate of 0.9822. In comparison, although robust, OpenSearch records a higher latency of 53.89ms and a slightly lower precision of 0.9823. Complete benchmarks are available in GitHub.
The architecture of specialized vector databases is specifically designed to handle high-dimensional vector data efficiently, but traditional databases are mainly built for relational data and don’t naturally support the specific needs of vector search.
Another option is adding vector extensions to your current database or search engine. This approach directly supports business needs by merging the strengths and flexibility of traditional databases with the advanced features of modern vector searches.
A hybrid model can align more closely with a business’ diverse data handling requirements and streamline its data infrastructure. This can reduce operational costs and complexity, ultimately leading to a more scalable and efficient solution that meets the comprehensive data processing needs of the organization.
SQL Vector Databases Bridge the Gap
SQL has been the backbone of scalable applications for half a century, and its integration with vector search features is poised to bridge the gap between traditional and modern data processing needs. Integrating SQL with vectors will improve data modeling flexibility and make development easier. This will enable the system to handle complex queries involving structured data, vector data, keyword searches and joined queries across multiple tables.
While specialized vector databases excel in handling high-dimensional data with precision and speed, integrating vector search into SQL databases presents a compelling alternative. It offers a balance between the efficiency required for complex data type processing at scale and the convenience of working within a familiar and widely adopted framework. This integration solves many challenges that specialized vector databases face, like slow iteration, inefficient querying and high costs of managing a separate database. By embracing SQL vector databases, enterprises can harness the power of SQL’s proven scalability and reliability, while gaining advanced capabilities needed to tackle the multifaceted challenges of modern data processing.
Conclusion
Relying entirely on a specialized vector database that only processes vectors limits how flexible your data management strategy can be. A multi-functional or integrated vector database provides a more promising solution.
MyScaleDB, an open source SQL vector database, not only efficiently manages vectors but also functions as a traditional database, making it suitable for a wide range of applications.
Built on ClickHouse, MyScale combines the strengths of traditional SQL databases with the capabilities of vector databases, efficiently storing and managing high-dimensional vectors using SQL for GenAI applications. It is also the first SQL vector database to outperform specialized vector databases in both performance and cost-effectiveness, debunking the myth that integrated vector databases are inherently less efficient than alternatives.
Having a database that can manage both traditional and vector data is crucial in today’s AI tech world. This approach ensures scalability, flexibility and cost-effectiveness, eliminating the need to manage multiple systems. By opting for a versatile database, you can prepare your data infrastructure for the future and meet modern applications’ increasing requirements.
YOUTUBE.COM/THENEWSTACK
Tech moves fast, don’t miss an episode. Subscribe to our YouTube
channel to stream all our podcasts, interviews, demos, and more.
Created with Sketch.